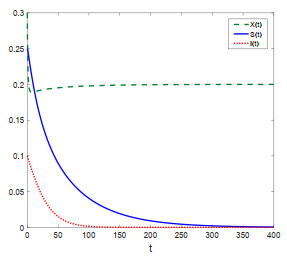
Chronic pain research, with a specific focus on the brain-derived neurotrophic factor (BDNF), has made impressive progress in the past decade, as evident in the improved research quality and increased publications. To better understand this evolving landscape, a quantitative approach is needed. The main aim of this study is to identify the hotspots and trends of BDNF in chronic pain research. We screened relevant publications from 2013 to 2022 in the Scopus database using specific search subject terms. A total of 401 documents were selected for further analysis. We utilized several tools, including Microsoft Excel, Harzing's Publish or Perish, and VOSViewer, to perform a frequency analysis, citation metrics, and visualization, respectively. Key indicators that were examined included publication growth, keyword analyses, topmost influential articles and journals, networking by countries and co-citation of cited references. Notably, there was a persistent publication growth between 2015 and 2021. “Neuropathic pain” emerged as a prominent keyword in 2018, alongside “microglia” and “depression”. The journal Pain® was the most impactful journal that published BDNF and chronic pain research, while the most influential publications came from open-access reviews and original articles. China was the leading contributor, followed by the United States (US), and maintained a leadership position in the total number of publications and collaborations. In conclusion, this study provides a comprehensive list of the most influential publications on BDNF in chronic pain research, thereby aiding in the understanding of academic concerns, research hotspots, and global trends in this specialized field.
Citation: Che Aishah Nazariah Ismail, Rahimah Zakaria, Khairunnuur Fairuz Azman, Nazlahshaniza Shafin, Noor Azlina Abu Bakar. Brain-derived neurotrophic factor (BDNF) in chronic pain research: A decade of bibliometric analysis and network visualization[J]. AIMS Neuroscience, 2024, 11(1): 1-24. doi: 10.3934/Neuroscience.2024001
[1] | Kawkab Al Amri, Qamar J. A Khan, David Greenhalgh . Combined impact of fear and Allee effect in predator-prey interaction models on their growth. Mathematical Biosciences and Engineering, 2024, 21(10): 7211-7252. doi: 10.3934/mbe.2024319 |
[2] | Saheb Pal, Nikhil Pal, Sudip Samanta, Joydev Chattopadhyay . Fear effect in prey and hunting cooperation among predators in a Leslie-Gower model. Mathematical Biosciences and Engineering, 2019, 16(5): 5146-5179. doi: 10.3934/mbe.2019258 |
[3] | Dirk Stiefs, Ezio Venturino, Ulrike Feudel . Evidence of chaos in eco-epidemic models. Mathematical Biosciences and Engineering, 2009, 6(4): 855-871. doi: 10.3934/mbe.2009.6.855 |
[4] | Yuhong Huo, Gourav Mandal, Lakshmi Narayan Guin, Santabrata Chakravarty, Renji Han . Allee effect-driven complexity in a spatiotemporal predator-prey system with fear factor. Mathematical Biosciences and Engineering, 2023, 20(10): 18820-18860. doi: 10.3934/mbe.2023834 |
[5] | Yuanfu Shao . Bifurcations of a delayed predator-prey system with fear, refuge for prey and additional food for predator. Mathematical Biosciences and Engineering, 2023, 20(4): 7429-7452. doi: 10.3934/mbe.2023322 |
[6] | Hongqiuxue Wu, Zhong Li, Mengxin He . Dynamic analysis of a Leslie-Gower predator-prey model with the fear effect and nonlinear harvesting. Mathematical Biosciences and Engineering, 2023, 20(10): 18592-18629. doi: 10.3934/mbe.2023825 |
[7] | Ranjit Kumar Upadhyay, Swati Mishra . Population dynamic consequences of fearful prey in a spatiotemporal predator-prey system. Mathematical Biosciences and Engineering, 2019, 16(1): 338-372. doi: 10.3934/mbe.2019017 |
[8] | Shunyi Li . Hopf bifurcation, stability switches and chaos in a prey-predator system with three stage structure and two time delays. Mathematical Biosciences and Engineering, 2019, 16(6): 6934-6961. doi: 10.3934/mbe.2019348 |
[9] | Rongjie Yu, Hengguo Yu, Chuanjun Dai, Zengling Ma, Qi Wang, Min Zhao . Bifurcation analysis of Leslie-Gower predator-prey system with harvesting and fear effect. Mathematical Biosciences and Engineering, 2023, 20(10): 18267-18300. doi: 10.3934/mbe.2023812 |
[10] | Wanxiao Xu, Ping Jiang, Hongying Shu, Shanshan Tong . Modeling the fear effect in the predator-prey dynamics with an age structure in the predators. Mathematical Biosciences and Engineering, 2023, 20(7): 12625-12648. doi: 10.3934/mbe.2023562 |
Chronic pain research, with a specific focus on the brain-derived neurotrophic factor (BDNF), has made impressive progress in the past decade, as evident in the improved research quality and increased publications. To better understand this evolving landscape, a quantitative approach is needed. The main aim of this study is to identify the hotspots and trends of BDNF in chronic pain research. We screened relevant publications from 2013 to 2022 in the Scopus database using specific search subject terms. A total of 401 documents were selected for further analysis. We utilized several tools, including Microsoft Excel, Harzing's Publish or Perish, and VOSViewer, to perform a frequency analysis, citation metrics, and visualization, respectively. Key indicators that were examined included publication growth, keyword analyses, topmost influential articles and journals, networking by countries and co-citation of cited references. Notably, there was a persistent publication growth between 2015 and 2021. “Neuropathic pain” emerged as a prominent keyword in 2018, alongside “microglia” and “depression”. The journal Pain® was the most impactful journal that published BDNF and chronic pain research, while the most influential publications came from open-access reviews and original articles. China was the leading contributor, followed by the United States (US), and maintained a leadership position in the total number of publications and collaborations. In conclusion, this study provides a comprehensive list of the most influential publications on BDNF in chronic pain research, thereby aiding in the understanding of academic concerns, research hotspots, and global trends in this specialized field.
The effect of disease on eco-epidemiology system is a significant topic from both mathematical and ecological perspectives. The disease factor usually leads to a more complex and diverting dynamics than those in the disease-free system [1,2]. Within the interactions between predator and prey, the disease could only spread in prey or predator population, also could spread between prey and predator [3,4,5]. Birds (particularly pelicans) infect vibrio and die by preying on vibrio-infected fish (particularly tilapia) at the Salton Sea in the desert of Southern California [3], which is an example of disease spreads amongst the prey. For the disease in predator, taking fox rabies as an example, foxes (Vulpis) infect rabies and transmit to other foxes or their prey rabbits by biting in Europe and North America [6]. More relevant examples could be found in [7]. From the mathematical epidemiology point of view, one needs much more attention in the dynamics of infected predator to observe whether the presence of the prey allows the survival of a part of the predator population [8].
A variety of diseased predator models have been proposed to study the complex interaction between prey and predator with infected diseases [2,9,10] and the reference therein. Most common epidemic model applied in predator-prey interactions is the SI-type, i.e., the predator population Y(t) is divided into two sub-classes, namely susceptible predator S(t) and infected predator I(t), respectively [10,11,12]. The infection term could be mass-action term (bilinear form) βSI or saturation form βSIS+I [4]. The infected predators usually behave differently with susceptible ones, and suffer an additional death rate. In a epidemic model, the global dynamics are usually determined by the basic reproduction number R0, i.e., the disease will dies out in the population when R0≤1, and the disease will persist in the population when R0>1. However, the basic reproduction number is no longer a threshold parameter determining the global dynamics in diseased predator models, on the contrary, the dynamics are relatively comprehensive and unexpected.
Predation is the key force in a prey-predator interaction, which could affect the size of prey population by direct hunting [9,13,14,15], and elicit a variety of anti-predator responses [16,17,18]. Consequently, prey tends to alter behaviors in a certain extent, such as change of habitat, foraging activity, vigilance, physiological changes. This anti-predator behaviors accelerate the extinction, evolution and development of prey population in the long run. Under the risk of predation, prey may reduce its foraging activity in order to stay alert, leading to starvation which impacts on population growth [19,20]. Therefore, an immediately result of anti-predator behaviors is the reduction of prey growth rate, which is the cost for prey in prey defense [19,21,22,23,24,25,26].
Consider a simple birth-death process of the prey X(t) with the cost of anti-predator behaviors [27]:
dXdt=[F(k,Y)a]X−dX, |
where X,Y represent the density of the prey and predator, respectively. a is the birth rate of prey, d is the natural death rate of prey. F(k,Y) accounts for the cost of anti-predator defence due to fear, the parameter k reflects the level of fear which drives anti-predator behaviors of prey. The fear factor F(k,Y) has some specific assumptions under the ecological motions, for details see [20,27].
To derive a simple diseased predator model incorporating the anti-predator defence due to fear, we adopted the following fear effect term F(k,Y):
F(k,Y)=11+kY=11+k(S+I). |
Based on the results in [4,9,11], we can obtain the eco-epidemiological system with cost of anti-predator behaviors as following system of nonlinear differential equations:
{dXdt=rX1+k(S+I)−rX2K−aXS1+bX,dSdt=eaXS1+bX−d1S−βSI,dIdt=βSI−d2I, | (1.1) |
where X,S,I represent the density of prey, susceptible predator and infected predator at time t, respectively. r is the intrinsic growth rate of prey, K is the carrying capacity of the prey, a is the predation coefficient, b is the predators handling time of a prey, e is the biomass conversion constant, β is the transmissibility coefficient. d1 and d2 are the mortality rates of the susceptible predator and infected predator, and naturally d1<d2.
This paper consists of six sections. In the next section, we prove the positivity and boundedness of the solution of system (1.1). In Section 3, we provide the existence conditions of the equilibria of the model. We analyze the stability of equilibria and show the occurrence of Hopf bifurcation in Section 4. In Section 5, the correctness of the theoretical proof is illustrated by numerical simulation. Finally, we summarize our results with ecological interpretations in Section 6.
In view of the ecological significance, we only consider the solutions (X(t),S(t),I(t)) of system (1.1) on
R3+={(X(t),S(t),I(t))∈R3+:X(t)≥0,S(t)≥0,I(t)≥0}. |
Theorem 2.1. Each solution of system (1.1) with initial value (X(0),S(0),I(0))∈R3+ is positive and ultimately bounded.
Proof. Since the right-hand side of system (1.1) is completely continuous and locally Lipschitzian on R3+, the solution (X(t),S(t),I(t)) with initial condition (X(0),S(0),I(0))∈R3+ exists and is unique on R3+.
By integrating, it follows from system (1.1) that
X(t)=X(0)exp{t∫0(r1+k(S(τ)+I(τ))−rX(τ)K−aS(τ)1+bX(τ))dτ}≥0,S(t)=S(0)exp{t∫0(eaX(τ)1+bX(τ)−d1−βI(τ))dτ}≥0,I(t)=I(0)exp{t∫0(βS(τ)−d2)dτ}≥0. |
Hence, the solution (X(t),S(t),I(t)) of system (1.1) with the initial condition (X(0),S(0),I(0))∈R3+ remains positive.
From the first equation of (1.1), we can obtain
dXdt=rX1+k(S+I)−rX2K−aXS1+bX≤rX−rX2K=rX(1−XK), |
then
lim supt→∞X(t)≤K. |
Let N(t)=eX(t)+S(t)+I(t), we can get
dNdt=erX1+k(S+I)−erX2K−d1S−d2I≤erX−erX2K−d1S−d2I≤erX(1−XK)+ed1X−d1N≤eK(r+d1)24r−d1N, |
then
lim supt→∞N(t)≤eK(r+d1)24rd1. |
This ends the proof.
Remark 2.2. From Theorem 2.1, we know that all positive solutions of system (1.1) with initial conditions (X(0),S(0),I(0))∈R3+ are defined in the following positive bounded invariant:
Γ:={(X(t),S(t),I(t))∈R3+:0≤X(t)≤K,0≤eX(t)+S(t)+I(t)≤eK(r+d1)24rd1}. |
System (1.1) possesses at most three boundary equilibria:
(i) Trivial equilibrium: E0=(0,0,0);
(ii) Axial equilibrium: E1=(K,0,0);
(iii) Planar equilibrium: E2=(X2,S2,0) exists if ea−bd1>0 and K>d1ea−bd1, where
X2=d1ea−bd1,S2=−[K(ea−bd1)2+rd1ke]+√[K(ea−bd1)2−rd1ke]2+4K2kre(ea−bd1)32Kk(ea−bd1)2. | (3.1) |
For epidemic models, the most critical problem is the threshold property for the extinction and persistence of the disease, which is generally governed by the basic reproduction number R0. The basic reproduction number can be interpreted as the expected number of secondary cases produced, in a completely susceptible population, by a typical infected individual during its entire period of infectiousness. Following [28], we define the basic reproduction number for the predator population in the system (1.1) by
R0:=βS2d2, |
where S2 is given by (3.1).
Next, we mainly focus on the existence of positive equilibrium E3=(X3,S3,I3) of system (1.1). The coordinates X3,S3,I3 are positive solutions to the following system of equilibrium equations:
{r1+k(S3+I3)−rX3K−aS31+bX3=0,eaX31+bX3−d1−βI3=0,βS3−d2=0. |
Thus,
S3=d2β,I3=X3(ea−bd1)−d1(bX3+1)β, |
and X3 is the positive root of (3.2) in (X2,+∞):
Q(X)=m3X3+m2X2+m1X+m0=0, | (3.2) |
where
m3:=−bβr(k(ea−bd1)+b(kd2+β)),m2:=βr(Kb2β−k(ea−bd1)−2b(kd2+β)+bkd1),m1:=(−kd2a(ea−bd1)−b(akd22+aβd2−2β2r))K−βr(−kd1+kd2+β),m0:=−K(−ad2(kd1−kd2−β)−β2r). |
If ea−bd1>0 and r>ad2(β+k(d2−d1))β2, we have
m3<0,m0>0. |
By Descartes' rule of signs, system (1.1) has at least one positive equilibrium E3.
Hence, we have the following results on the existence of the positive equilibrium. It is worthy to note that the positive equilibrium is not unique due to the impact of fear effect k.
Theorem 3.1. If ea−bd1>0 and r>ad2(β+k(d2−d1))β2, then system (1.1) has at least one positive equilibrium E3=(X3,S3,I3), where S3=S2R0, I3=X3(ea−bd1)−d1(bX3+1)β and X3 is the positive root of (3.2) in (X2,+∞).
Regarding the local stability of trivial equilibrium E0 and axial equilibrium E1, we have the following results. The proof is standard, so we omit it here.
Theorem 4.1. For system (1.1),
(i) The trivial equilibrium E0=(0,0,0) is unstable;
(ii) If one of the following inequalities holds:
(ii-1) ea−bd1<0;
(ii-2) ea−bd1>0 and K<d1ea−bd1,
then the axial equilibrium E1=(K,0,0) is stable; while E1=(K,0,0) is unstable if ea−bd1>0 and K>d1ea−bd1.
Secondly, we will show the local stability of the planar equilibrium E2 of system (1.1). For convenience, set
r1:=d2(ea−bd1)βe,r2:=d2(ea−bd1)(ea+bd1)aβe2,K1:=ea+bd1b(ea−bd1),K2:=βerd1(ea−bd1)(βer−d2(ea−bd1)),k1:=Kb(ea−bd1)2(Kb(ea−bd1)−(ea+bd1))ae2r(ea+bd1),k2:=−β((ea−bd1)(d2(ea−bd1)−βer)K+βerd1)(Kd2(ea−bd1)2+βerd1)d2. | (4.1) |
Theorem 4.2. For system (1.1), assume that ea−bd1>0. If one of the following inequalities holds:
(Ⅰ) r≤r1 and one of the following inequalities holds:
(Ⅰ-1) d1ea−bd1<K≤K1;
(Ⅰ-2) K>K1 and k>k1;
(Ⅱ) r1<r<r2 and one of the following inequalities holds:
(Ⅱ-1) d1ea−bd1<K≤K1;
(Ⅱ-2) K1<K and k>max{k1,k2};
(Ⅲ) r>r2 and one of the following inequalities holds:
(Ⅲ-1) d1ea−bd1<K≤K2;
(Ⅲ-2) K2<K and k>max{k1,k2},
then equilibrium E2 is stable; otherwise, it is unstable.
Proof. The Jacobian matrix of system (1.1) at E2 is given by
J2=(a11a12a13a210−βS200βS2−d2), |
where
a11:=X2(−rK+abS2(1+bX2)2),a12:=−krX2(1+kS2)2−aX21+bX2,a13:=−krX2(1+kS2)2,a21:=eaS2(1+bX2)2. |
Hence, the characteristic equation of J2 is given as
f(λ)(λ−βS2+d2)=0, | (4.2) |
where
f(λ):=λ2−a11λ−a12a21. |
Clearly, one can see that J2 has three eigenvalues λ1, λ2 and λ3=βS2−d2. Since a12<0,a21>0, then −a12a21>0.
From (3.1), we can obtain
a11=X2(−rK+abS2(1+bX2)2)=X2Φ2Kka2e2, |
where
Φ:=ab√(K(ea−bd1)2−rd1ke)2+4K2kre(ea−bd1)3−ab(K(ea−bd1)2+rd1ke)−2rka2e2. |
Note that the sign of Φ depends on
˜Φ:=a2b2((K(ea−bd1)2−rd1ke)2+4K2kre(ea−bd1)3)−(ab(K(ae−bd1)2+rd1ke)+2rka2e2)2=4a2kerP(k), |
where
P(k):=−ae2r(ea+bd1)k+Kb(ea−bd1)2(Kb(ea−bd1)−(ea+bd1)). |
One can obtain that P(k) is decreasing with respect to k. If K≤ea+bd1b(ea−bd1) holds, we have P(0)≤0, which means that P(k)<0 for all k>0; if K>ea+bd1b(ea−bd1) and k>k1 hold, we can get P(k)<0. Therefore, when one of the following inequalities holds:
(i) K≤ea+bd1b(ea−bd1);
(ii) K>ea+bd1b(ea−bd1) and k>k1,
we can obtain a11<0, which implies that the real parts of λ1 and λ2 are all negative.
It follows from system (3.1) that
βS2−d2=−β[K(ea−bd1)2+rd1ke]+β√[K(ea−bd1)2−rd1ke]2+4K2kre(ea−bd1)32Kk(ea−bd1)2−d2=Θ2Kk(ea−bd1)2, |
where
Θ:=−K(ea−bd1)2(2kd2+β)−βekrd1+β√(K(ea−bd1)2−rd1ke)2+4K2kre(ea−bd1)3. |
Note that the sign of Θ depends on
˜Θ:=β2(K(ea−bd1)2−rd1ke)2+4β2K2kre(ea−bd1)3−(K(ea−bd1)2(2kd2+β)+βekrd1)2=−4Kk(ae−bd1)2[(Kd2(ea−bd1)2+βerd1)d2k+β((ea−bd1)(d2(ea−bd1)−βer)K+βerd1)]. |
Then if one of the following inequalities holds:
(Ⅰ) ea−bd1>0 and r≤d2(ea−bd1)βe;
(Ⅱ) ea−bd1>0, r>d2(ea−bd1)βe and one of the following inequalities:
(Ⅱ-1) K≤βerd1(ea−bd1)(βer−d2(ea−bd1));
(Ⅱ-2) K>βerd1(ea−bd1)(βer−d2(ea−bd1)) and k>k2:=−β((ea−bd1)(d2(ea−bd1)−βer)K+βerd1)(Kd2(ea−bd1)2+βerd1)d2,
we have λ3=βS2−d2<0.
Thus, we can arrive at the conclusion.
It should be pointed out that another way to state Theorem 4.2 is as follows.
Remark 4.3. For system (1.1), assume that ea−bd1>0 and R0<1. If one of the following inequalities:
(Ⅰ) d1ea−bd1<K≤K1;
(Ⅱ) K>K1 and k>k1
holds, then the planar equilibrium E2 is stable; otherwise, it is unstable.
Next, we will show the local stability of the positive equilibrium E3 of system (1.1).
The Jacobian matrix of system (1.1) at E3 is given by
J3=(b11b12b13b210−d20βI30), |
where
b11=X3(−rK+abS3(1+bX3)2),b12=−krX3(1+k(S3+I3))2−aX31+bX3<0,b13=−krX3(1+k(S3+I3))2<0,b21=eaS3(1+bX3)2>0. | (4.3) |
The characteristic equation of J3 is given as
λ3+A1λ2+A2λ+A3=0, | (4.4) |
where
A1=−b11,A2=βd2I3−b12b21,A3=−b11βd2I3−b13b21βI3. | (4.5) |
Note that if A1>0 holds, then b11<0, which means that A3>0. According to Routh-Hurwitz criterion, the positive equilibrium E3 is locally asymptotically stable when A1>0 and A1A2−A3>0.
Therefore, we can establish the following statement.
Theorem 4.4. Assume that ea−bd1>0 and r>ad2(β+k(d2−d1))β2 hold. The positive equilibrium E3 of system (1.1) is locally asymptotically stable if A1>0 and A1A2−A3>0, where Ai,i=1,2,3 is defined as in (4.5). Otherwise, it is unstable.
Remark 4.5. Theorem 4.4 gives a sufficient condition about the stability of the positive equilibrium E3 for system (1.1). However, the complexity of model (1.1) leads to the failure to theoretically demonstrate how the fear factor affects the stability of the positive equilibrium. This will be discussed later through numerical simulations.
In this subsection, we take k as the bifurcation parameter. The characteristic equation of system (1.1) at E3 is (4.4), and Ai(k),i=1,2,3 are defined as (4.5).
Theorem 4.6. Hopf bifurcation near the positive equilibrium E3 for system (1.1) occurs whenever the critical parameter k attains the value k=kh in the domain:
Ω={kh∈R+:Δ(kh):=[A1(k)A2(k)−A3(k)]|k=kh=0withA2(kh)>0,[dΔ(k)dk]|k=kh≠0}. |
Proof. If k=kh, the characteristic Eq (4.4) equals
λ3+A1(kh)λ2+A2(kh)λ+A3(kh)=0, | (4.6) |
then (4.6) can be factorized as
(λ2+A2(kh))(λ+A1(kh))=0. | (4.7) |
Clearly, (4.7) has three roots: λ1=i√A2(kh), λ2=−i√A2(kh) and λ3=−A1(kh). The roots are of the form λ1=p1(k)+ip2(k), λ2=p1(k)−ip2(k) and λ3=−p3(k), where pi(k)(i=1,2,3) are real numbers.
From the characteristic Eq (4.4), we can get
dλdk=−λ2A′1+λA′2+A′33λ2+2A1λ+A2, | (4.8) |
where ′=ddk. Substituting λ=i√A2 into (4.8), we obtain that
A′3−A2A′1+iA′2√A22(A2−iA1√A2)=−dΔ(k)dk2(A21+A2)+i[√A2A′22A2−A1√A2dΔ(k)dk2A2(A21+A2)], |
which implies that
[dRe(λ)dk]|k=kh=−dΔ(k)dk2(A21+A2)|k=kh. |
By using monotonicity condition in the real part of the complex root dRe(λ)dk|k=kh≠0, the transversality condition dΔ(k)dk|k=kh≠0 can be obtained to ensure the existence of Hopf bifurcation.
Results from numerical simulations are provided in this section to demonstrate our theoretical results. As we will show, the observations shed lights on the impact of fear factor. We choose the parameters of system (1.1) as follows:
r=0.8,a=0.2,b=0.1,e=0.9,d1=0.05,β=0.1,d2=0.053. | (5.1) |
Then we have
ea−bd1=0.175>0,d1ea−bd1=0.286,r1=d2(ea−bd1)βe=0.103,r2=d2(ea−bd1)(ea+bd1)aβe2=0.106,K1=ea+bd1b(ea−bd1)=10.571,K2=βerd1(ea−bd1)(βer−d2(ea−bd1))=0.328. |
Example 5.1 (The stability of E1).
We adopt K=0.2,k=0.01, then system (1.1) has trivial equilibrium E0=(0,0,0) and axial equilibrium E1=(0.2,0,0). In this case, one can know that the conditions of Theorem 4.1 are satisfied, which means that E1 is locally asymptotically stable. The numerical results are shown in Figure 1.
Example 5.2 (The impacts of K and k on the stability of E2).
In this example, we will choose three values of carrying capacity K for numerical experiments. We conclude that the carrying capacity and fear effect are other key factors related to the extinction of infected predators, in addition to the basic reproduction number R0.
Firstly, we take K=0.3<K2, then we have k=0.1 which yields that R0=0.263<1. In this case, system (1.1) has trivial equilibrium E0=(0,0,0), axial equilibrium E1=(0.3,0,0), and planar equilibrium E2=(0.286,0.139,0). By Theorem 4.5, E2 is locally asymptotically stable, see Figure 2(a). Thus, when the carrying capacity of the prey K is small, no matter what the level of fear k is, the small size of prey population will lead to the extinction of infected predators.
Secondly, for comparison, we take K2<K=15, then
k1=Kb(ea−bd1)2(Kb(ea−bd1)−(ea+bd1))ae2r(ea+bd1)=0.149,k2=−β((ea−bd1)(d2(ea−bd1)−βer)K+βerd1)(Kd2(ea−bd1)2+βerd1)d2=10.873. |
Choosing k=0.1<max{k1,k2} which yields R0=5.792>1, then we have
A1=0.25791>0,A1A2−A3=0.00523>0. |
In this case, system (1.1) has trivial equilibrium E0=(0,0,0), axial equilibrium E1=(15,0,0), planar equilibrium E2=(0.286,3.070,0), and positive equilibrium E3=(7.351,0.530,7.125). By Theorem 4.5, E2=(0.286,3.070,0) is unstable. On the contrary, E3=(7.351,0.530,7.125) is locally asymptotically stable. The numerical simulation is shown in Figure 2(b).
Finally, we take K2<K=60, then we have
k1=Kb(ea−bd1)2(Kb(ea−bd1)−(ea+bd1))ae2r(ea+bd1)=6.629,k2=−β((ea−bd1)(d2(ea−bd1)−βer)K+βerd1)(Kd2(ea−bd1)2+βerd1)d2=12.238. |
Choosing k=30>max{k1,k2} which yields that R0=0.649<1, system (1.1) has trivial equilibrium E0=(0,0,0), axial equilibrium E1=(60,0,0), and planar equilibrium E2=(0.286,0.344,0). By Theorem 4.5, E2 is locally asymptotically stable, see Figure 2(c). Thus, when the carrying capacity of the prey K is relatively large, a high level of fear k will lead to the extinction of infected predators.
Example 5.3 (The impact of k on the stability of E3). We adopt K=60, then we have kh=0.26. In the next, we will choose three values of k, corresponding to the local stability of E3, Hopf bifurcation, and instability of E3, to illustrate the impact of fear factor on the population dynamics.
Firstly, we take k=0.1<kh which yields that R0=5.881>1, then system (1.1) has trivial equilibrium E0=(0,0,0), axial equilibrium E1=(60,0,0), planar equilibrium E2=(0.286,3.117,0) and a unique positive equilibrium E3=(24.047,0.530,12.213). In this case, we obtain that
A1=0.29863>0,A1A2−A3=0.00065>0, |
which means that E3 is local asymptotically stable. The numerical results are shown in Figure 3.
Secondly, we take k=0.26=kh which yields that R0=4.682>1, then system (1.1) has trivial equilibrium E0=(0,0,0), axial equilibrium E1=(60,0,0), planar equilibrium E2=(0.286,2.481,0) and a unique positive equilibrium E3=(12.975,0.530,9.665). In this case, we obtain that
A1=0.14694>0,A1A2−A3=0, |
which means that system (1.1) undergoes a Hopf bifurcation and there is a limit cycle around E3. The numerical results and the bifurcation diagrams of system (1.1) with respect to the parameter k are shown in Figures 4 and 5, respectively. Comparing Figures 3 and 4(a), one can see that there are two different implications induced by the fear factor k: the first is that the stability of E3 converts from stable into unstable, and the second is the decrease of values of X3 and I3 of E3.
Finally, we take k=0.5>kh which yields that R0=3.821>1, then system (1.1) has trivial equilibrium E0=(0,0,0), axial equilibrium E1=(60,0,0), planar equilibrium E2=(0.286,2.025,0) and a unique positive equilibrium E3=(7.685,0.530,7.322). In this case, we can obtain that
A1=0.07642>0,A1A2−A3=−0.00051<0, |
which means that E3 is unstable. The numerical results are shown in Figure 6. One can find that the difference between Figures 4 and 6 is the decrease of values of E3 from (12.975,0.530,9.665) to (7.685,0.530,7.322), which is induced by the impact of the feat factor.
In this paper, we explored a predator-prey model that incorporates infectious disease in predator population and the cost of anti-predator behaviors. The cost of anti-predator behaviors is measured by a fear effect k leading to an reduction of prey's birth rate. We fulfill a complete stability analysis of equilibria for system (1.1) and show that the system (1.1) exhibits the Hopf bifurcation. Biologically, we focus on the impact of fear effect on the population dynamics. As we will see later, the cost of a high level of fear effect is disastrously. The main findings are summarized in the following.
1) Small size of prey population leads to the extinction of infected predators.
If the carrying capacity K is relatively small, the planar equilibrium E2 is stable, see Figure 2(a). Thus, no matter what the level of fear effect k is, a small size of prey population will lead to the extinction of infected predators.
2) Low level of the fear effect doesn't impact on the population dynamics.
If the level of fear effect k<kh, the positive (coexistence) equilibrium E3 is stable, see Figure 3. Hence, we conclude that a small fear effect k is not the key disturbance and does not change the coexistence dynamics of system (1.1). However, the densities of the prey and infected predator gradually decrease as k increasing.
3) Certain medium level of the fear effect lead to periodic oscillation.
If k=kh, the fear effect can destabilize the stability of E3 and will benefit the occurrence of periodic oscillation. In other words, system (1.1)undergoes a limit cycle, see Figures 4 and 5.
4) High level of the fear effect leads to complex dynamics and the infected predator can go to extinction.
If k>kh, E3 is unstable, see Figure 6. Therefore, a large fear effect k persistently and dramatically influence the population dynamics of prey and predator. Furthermore, if the level of the fear factor k is extremely high, the planar equilibrium E2 is stable, see Figure 2(c). The prey will respond to perceived predation risk and show a variety of anti-predator responses, dramatically decreasing the recruitment of susceptible predator, which will lead to an extinction of infected predator.
The authors would like to thank the editor and the referees for their helpful comments. This research was supported by the National Natural Science Foundation of China (Grant No. 12171192, 12031020 and 12071173), the Science and Technology Research Projects of the Education Office of Jilin Province, China (JJKH20211033KJ), the Technology Development Program of Jilin Province, China (20210508024RQ) and Huaian Key Laboratory for Infectious Diseases Control and Prevention, China (HAP201704).
The authors declare that there are no conflicts of interest regarding the publication of this paper.
[1] |
Şentürk İA, Şentürk E, Üstün I, et al. (2023) High-impact chronic pain: evaluation of risk factors and predictors. Korean J Pain 36: 84-97. https://doi.org/10.3344/kjp.22357 ![]() |
[2] |
Hutton D, Mustafa A, Patil S, et al. (2023) The burden of Chronic Pelvic Pain (CPP): Costs and quality of life of women and men with CPP treated in outpatient referral centers. Plos One 18: e0269828. https://doi.org/10.1371/journal.pone.0269828 ![]() |
[3] |
Nahin RL, Feinberg T, Kapos FP, et al. (2023) Estimated Rates of Incident and Persistent Chronic Pain Among US Adults, 2019-2020. JAMA Netw Open 6: e2313563. https://doi.org/10.1001/jamanetworkopen.2023.13563 ![]() |
[4] |
Collier R (2018) A short history of pain management. Can Med Assoc J 190: E26-E27. https://doi.org/10.1503/cmaj.109-5523 ![]() |
[5] |
Ikeda K, Hazama K, Itano Y, et al. (2020) Development of a novel analgesic for neuropathic pain targeting brain-derived neurotrophic factor. Biochem Biophys Res Commun 531: 390-395. https://doi.org/10.1016/j.bbrc.2020.07.109 ![]() |
[6] |
Bathina S, Das UN (2015) Brain-derived neurotrophic factor and its clinical implications. Arch Med Sci 11: 1164-1178. https://doi.org/10.5114/aoms.2015.56342 ![]() |
[7] |
Sikandar S, Minett MS, Millet Q, et al. (2018) Brain-derived neurotrophic factor derived from sensory neurons plays a critical role in chronic pain. Brain 141: 1028-1039. https://doi.org/10.1093/brain/awy009 ![]() |
[8] |
Sosanya NM, Garza TH, Stacey W, et al. (2019) Involvement of brain-derived neurotrophic factor (BDNF) in chronic intermittent stress-induced enhanced mechanical allodynia in a rat model of burn pain. BMC Neurosci 20: 1-18. https://doi.org/10.1186/s12868-019-0500-1 ![]() |
[9] |
Eaton MJ, Blits B, Ruitenberg MJ, et al. (2002) Amelioration of chronic neuropathic pain after partial nerve injury by adeno-associated viral (AAV) vector-mediated over-expression of BDNF in the rat spinal cord. Gene Ther 9: 1387-1395. https://doi.org/10.1038/sj.gt.3301814 ![]() |
[10] |
Thomas Cheng H (2010) Spinal cord mechanisms of chronic pain and clinical implications. Curr Pain Headache Rep 14: 213-220. https://doi.org/10.1007/s11916-010-0111-0 ![]() |
[11] |
Ding X, Cai J, Li S, et al. (2015) BDNF contributes to the development of neuropathic pain by induction of spinal long-term potentiation via SHP2 associated GluN2B-containing NMDA receptors activation in rats with spinal nerve ligation. Neurobiol Dis 73: 428-451. https://doi.org/10.1016/j.nbd.2014.10.025 ![]() |
[12] |
Zhou LJ, Yang T, Wei X, et al. (2011) Brain-derived neurotrophic factor contributes to spinal long-term potentiation and mechanical hypersensitivity by activation of spinal microglia in rat. Brain Behav Immun 25: 322-334. https://doi.org/10.1016/j.bbi.2010.09.025 ![]() |
[13] |
Zhou W, Xie Z, Li C, et al. (2021) Driving effect of BDNF in the spinal dorsal horn on neuropathic pain. Neurosci Lett 756: 135965. https://doi.org/10.1016/j.neulet.2021.135965 ![]() |
[14] |
Coull JAM, Beggs S, Boudreau D, et al. (2005) BDNF from microglia causes the shift in neuronal anion gradient underlying neuropathic pain. Nature 438: 1017-1021. https://doi.org/10.1038/nature04223 ![]() |
[15] |
Thakkar B, Acevedo EO (2023) BDNF as a biomarker for neuropathic pain: Consideration of mechanisms of action and associated measurement challenges. Brain Behav 13: e2903. https://doi.org/10.1002/brb3.2903 ![]() |
[16] |
Cao T, Matyas JJ, Renn CL, et al. (2020) Function and mechanisms of truncated BDNF receptor TrkB.T1 in neuropathic pain. Cells 9: 1194. https://doi.org/10.3390/cells9051194 ![]() |
[17] |
He T, Wu Z, Zhang X, et al. (2022) A bibliometric analysis of research on the role of BDNF in depression and treatment. Biomolecules 12: 1464. https://doi.org/10.3390/biom12101464 ![]() |
[18] |
Abramo G, D'Angelo CA, Viel F (2011) The field-standardized average impact of national research systems compared to world average: the case of Italy. Scientometrics 88: 599-615. https://doi.org/10.1007/s11192-011-0406-x ![]() |
[19] |
Ahmad R, Azman KF, Yahaya R, et al. (2023) Brain-derived neurotrophic factor (BDNF) in schizophrenia research: a quantitative review and future directions. AIMS Neurosci 10: 5-32. https://doi.org/10.3934/Neuroscience.2023002 ![]() |
[20] |
Fei X, Wang S, Li J, et al. (2022) Bibliometric analysis of research on Alzheimer's disease and non-coding RNAs: opportunities and challenges. Front Aging Neurosci 14: 1037068. https://doi.org/10.3389/fnagi.2022.1037068 ![]() |
[21] | Martínez-Ezquerro JD, Michán L, Rosas-Vargas H (2016) Bibliometric analysis of the BDNF Val66Met polymorphism based on Web of Science, Pubmed, and Scopus databases. Paper presented at: 29th National Congress of Biochemistry, Mexican Society of Biochemistry (SMB) 2012 . https://doi.org/10.7490/f1000research.1113470.1 |
[22] |
Othman Z, Abdul Halim AS, Azman KF, et al. (2022) Profiling the research landscape on cognitive aging: A bibliometric analysis and network visualization. Front Aging Neurosci 14: 876159. https://doi.org/10.3389/fnagi.2022.876159 ![]() |
[23] |
Zhu J, Liu W (2020) A tale of two databases: The use of Web of Science and Scopus in academic papers. Scientometrics 123: 321-335. https://doi.org/10.1007/s11192-020-03387-8 ![]() |
[24] |
Pranckutė R (2021) Web of Science (WoS) and Scopus: The titans of bibliographic information in today's academic world. Publications 9: 12. https://doi.org/10.3390/publications9010012 ![]() |
[25] |
Ferrini F, De Koninck Y (2013) Microglia control neuronal network excitability via BDNF signaling. Neural Plast 2013: 429815. https://doi.org/10.1155/2013/429815 ![]() |
[26] |
Liu Y, Zhou L-J, Wang J, et al. (2017) TNF-α differentially regulates synaptic plasticity in the hippocampus and spinal cord by microglia-dependent mechanisms after peripheral nerve injury. J Neurosci 37: 871-881. https://doi.org/10.1523/JNEUROSCI.2235-16.2016 ![]() |
[27] |
Gomes C, Ferreira R, George J, et al. (2013) Activation of microglial cells triggers a release of brain-derived neurotrophic factor (BDNF) inducing their proliferation in an adenosine A2A receptor-dependent manner: A2A receptor blockade prevents BDNF release and proliferation of microglia. J Neuroinflamm 10: 1-13. https://doi.org/10.1186/1742-2094-10-16 ![]() |
[28] |
Taves S, Berta T, Chen G, et al. (2013) Microglia and spinal cord synaptic plasticity in persistent pain. Neural Plast 2013: 753656. https://doi.org/10.1155/2013/753656 ![]() |
[29] |
Yalcin I, Barthas F, Barrot M (2014) Emotional consequences of neuropathic pain: insight from preclinical studies. Neurosci Biobehav Rev 47: 154-164. https://doi.org/10.1016/j.neubiorev.2014.08.002 ![]() |
[30] |
Taylor AMW, Castonguay A, Taylor AJ, et al. (2015) Microglia disrupt mesolimbic reward circuitry in chronic pain. J Neurosci 35: 8442-8450. https://doi.org/10.1523/JNEUROSCI.4036-14.2015 ![]() |
[31] |
Khan N, Smith MT (2015) Neurotrophins and neuropathic pain: role in pathobiology. Molecules 20: 10657-10688. https://doi.org/10.3390/molecules200610657 ![]() |
[32] |
Nijs J, Meeus M, Versijpt J, et al. (2015) Brain-derived neurotrophic factor as a driving force behind neuroplasticity in neuropathic and central sensitization pain: a new therapeutic target?. Expert Opin Ther Targets 19: 565-576. https://doi.org/10.1517/14728222.2014.994506 ![]() |
[33] |
Zhou L-J, Peng J, Xu Y-N, et al. (2019) Microglia are indispensable for synaptic plasticity in the spinal dorsal horn and chronic pain. Cell Rep 27: 3844-3859. https://doi.org/10.1016/j.celrep.2019.05.087 ![]() |
[34] |
Richner M, Ulrichsen M, Elmegaard SL, et al. (2014) Peripheral nerve injury modulates neurotrophin signaling in the peripheral and central nervous system. Mol Neurobiol 50: 945-970. https://doi.org/10.1007/s12035-014-8706-9 ![]() |
[35] |
Chaplan SR, Bach FW, Pogrel JW, et al. (1994) Quantitative assessment of tactile allodynia in the rat paw. J Neurosci Methods 53: 55-63. https://doi.org/10.1016/0165-0270(94)90144-9 ![]() |
[36] |
Decostered I, Woolf CJ (2000) Spared nerve injury: an animal model of persistent peripheral neuropathic pain. Pain 87: 149-158. https://doi.org/10.1016/S0304-3959(00)00276-1 ![]() |
[37] |
Bennett GJ, Xie Y-K (1988) A peripheral mononeuropathy in rat that produces disorders of pain sensation like those seen in man. Pain 33: 87-107. https://doi.org/10.1016/0304-3959(88)90209-6 ![]() |
[38] | Ribeiro VGC, Lacerda ACR, Santos JM, et al. (2021) Efficacy of whole-body vibration training on brain-derived neurotrophic factor, clinical and functional outcomes, and quality of life in women with fibromyalgia syndrome: a randomized controlled trial. J Healthc Eng 2021: 7593802. https://doi.org/10.1155/2021/7593802 |
[39] |
Sheng J, Liu S, Wang Y, et al. (2017) The link between depression and chronic pain: neural mechanisms in the brain. Neural Plast 2017: 9724371. https://doi.org/10.1155/2017/9724371 ![]() |
[40] |
Zhang S-B, Zhao G-H, Lv T-R, et al. (2023) Bibliometric and visual analysis of microglia-related neuropathic pain from 2000 to 2021. Front Mol Neurosci 16: 1142852. https://doi.org/10.3389/fnmol.2023.1142852 ![]() |
[41] |
Du H, Wu D, Zhong S, et al. (2022) miR-106b-5p attenuates neuropathic pain by regulating the P2x4 receptor in the spinal cord in mice. J Mol Neurosci 72: 1764-1778. https://doi.org/10.1007/s12031-022-02011-z ![]() |
[42] |
Kohno K, Tsuda M (2021) Role of microglia and P2X4 receptors in chronic pain. Pain Rep 6: e864. https://doi.org/10.1097/PR9.0000000000000864 ![]() |
[43] | Biagioli M, Lippman A (2020) Gaming the metrics: Misconduct and manipulation in academic research. London: MIT Press 1-21. |
[44] |
Caon M, Trapp J, Baldock C (2020) Citations are a good way to determine the quality of research. Phys Eng Sci Med 43: 1145-1148. https://doi.org/10.1007/s13246-020-00941-9 ![]() |
[45] |
Chuang K-Y, Ho Y-S (2014) A bibliometric analysis on top-cited articles in pain research. Pain Med 15: 732-744. https://doi.org/10.1111/pme.12308 ![]() |
[46] |
Thelwall M, Sud P (2022) Scopus 1900–2020: Growth in articles, abstracts, countries, fields, and journals. Quant Sci 3: 37-50. https://doi.org/10.1162/qss_a_00177 ![]() |
[47] |
Xiong H-Y, Liu H, Wang X-Q (2021) Top 100 most-cited papers in neuropathic pain from 2000 to 2020: a bibliometric study. Front Neurol 12: 765193. https://doi.org/10.3389/fneur.2021.765193 ![]() |
[48] |
Chou C-Y, Chew SSL, Patel DV, et al. (2009) Publication and citation analysis of the Australian and New Zealand Journal of Ophthalmology and Clinical and Experimental Ophthalmology over a 10-year period: the evolution of an ophthalmology journal. Clin Experiment Ophthalmol 37: 868-873. https://doi.org/10.1111/j.1442-9071.2009.02191.x ![]() |
[49] |
Li X, Zhu W, Li J, et al. (2021) Prevalence and characteristics of chronic Pain in the Chinese community-dwelling elderly: a cross-sectional study. BMC Geriatr 21: 1-10. https://doi.org/10.1186/s12877-021-02432-2 ![]() |
[50] | Surwase G, Saga A, Kadermani BS, et al. (2011) Co-citation analysis: An overview. In: Paper Presented at: Beyond Librarianship: Creativity, Innovation and Discovery, Mumbai (India), 16–17 September 2011 . |
[51] |
Argüelles JC, Argüelles-Prieto R (2019) The impact factor: implications for research policy, editorial rules and scholarly reputation. FEMS Microbiol Lett 366: fnz132. https://doi.org/10.1093/femsle/fnz132 ![]() |
[52] |
Romanelli JP, Gonçalves MCP, de Abreu Pestana LF, et al. (2021) Four challenges when conducting bibliometric reviews and how to deal with them. Environ Sci Pollut Res 28: 60448-60458. https://doi.org/10.1007/s11356-021-16420-x ![]() |
[53] | Gingras Y (2016) Bibliometrics and research evaluation: Uses and abuses. London: MIT Press Pp 1-89. |
[54] |
Zimmermann M (1983) Ethical guidelines for investigations of experimental pain in conscious animals. Pain 16: 109-110. https://doi.org/10.1016/0304-3959(83)90201-4 ![]() |
[55] |
Groth R, Aanonsen L (2002) Spinal brain-derived neurotrophic factor (BDNF) produces hyperalgesia in normal mice while antisense directed against either BDNF or trkB, prevent inflammation-induced hyperalgesia. Pain 100: 171-181. https://doi.org/10.1016/0304-3959(83)90201-4 ![]() |
[56] |
Hargreaves K, Dubner R, Brown F, et al. (1988) A new and sensitive method for measuring thermal nociception in cutaneous hyperalgesia. Pain 32: 77-88. https://doi.org/10.1016/0304-3959(88)90026-7 ![]() |
[57] |
Smith P (2014) BDNF: no gain without pain?. Neurosci 283: 107-123. https://doi.org/10.1016/j.neuroscience.2014.05.044 ![]() |
[58] |
Geng S-J, Liao F-F, Dang W-H, et al. (2010) Contribution of the spinal cord BDNF to the development of neuropathic pain by activation of the NR2B-containing NMDA receptors in rats with spinal nerve ligation. Exp Neurol 222: 256-266. https://doi.org/10.1016/j.expneurol.2010.01.003 ![]() |
[59] |
Kim SH, Chung JM (1992) An experimental model for peripheral neuropathy produced by segmental spinal nerve ligation in the rat. Pain 50: 355-363. https://doi.org/10.1016/0304-3959(92)90041-9 ![]() |
[60] |
Merighi A, Salio C, Ghirri A, et al. (2008) BDNF as a pain modulator. Prog Neurobiol 85: 297-317. https://doi.org/10.1016/j.pneurobio.2008.04.004 ![]() |
[61] |
Pezet S, McMahon SB (2006) Neurotrophins: mediators and modulators of pain. Annu Rev Neurosci 29: 507-538. https://doi.org/10.1146/annurev.neuro.29.051605.112929 ![]() |
[62] |
Tsuda M, Shigemoto-Mogami Y, Koizumi S, et al. (2003) P2X4 receptors induced in spinal microglia gate tactile allodynia after nerve injury. Nature 424: 778-783. https://doi.org/10.1038/nature01786 ![]() |
[63] |
Coull JAM, Boudreau D, Bachand K, et al. (2003) Trans-synaptic shift in anion gradient in spinal lamina I neurons as a mechanism of neuropathic pain. Nature 424: 938-942. https://doi.org/10.1038/nature01868 ![]() |
1. | Chunmei Zhang, The effect of the fear factor on the dynamics of an eco-epidemiological system with standard incidence rate, 2024, 9, 24680427, 128, 10.1016/j.idm.2023.12.002 | |
2. | Hongqiuxue Wu, Zhong Li, Mengxin He, Dynamic analysis of a Leslie-Gower predator-prey model with the fear effect and nonlinear harvesting, 2023, 20, 1551-0018, 18592, 10.3934/mbe.2023825 | |
3. | Zhuoying Zhao, Xinhong Zhang, Unraveling the transmission mechanism of animal disease: Insight from a stochastic eco-epidemiological model driven by Lévy jumps, 2025, 191, 09600779, 115859, 10.1016/j.chaos.2024.115859 | |
4. | Huazhou Mo, Yuanfu Shao, Stability and bifurcation analysis of a delayed stage-structured predator–prey model with fear, additional food, and cooperative behavior in both species, 2025, 2025, 2731-4235, 10.1186/s13662-025-03879-y |