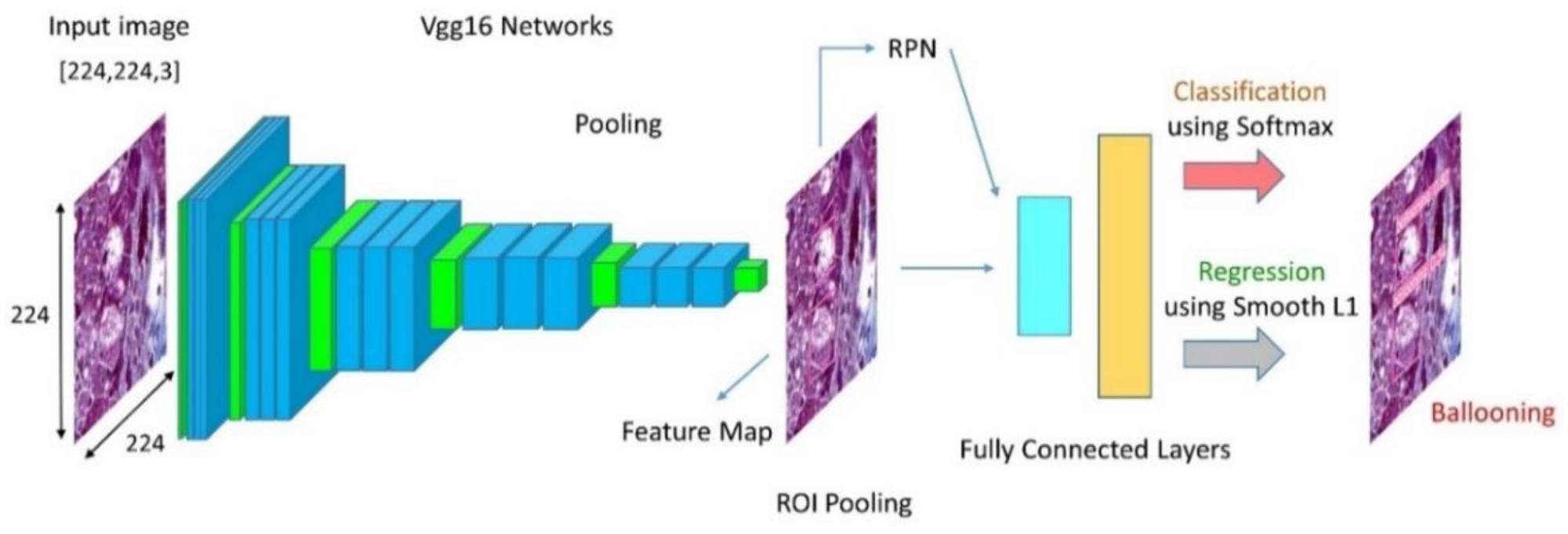
Accurate detection of non-alcoholic fatty liver disease (NAFLD) through biopsies is challenging. Manual detection of the disease is not only prone to human error but is also time-consuming. Using artificial intelligence and deep learning, we have successfully demonstrated the issues of the manual detection of liver diseases with a high degree of precision. This article uses various neural network-based techniques to assess non-alcoholic fatty liver disease. In this investigation, more than five thousand biopsy images were employed alongside the latest versions of the algorithms. To detect prominent characteristics in the liver from a collection of Biopsy pictures, we employed the YOLOv3, Faster R-CNN, YOLOv4, YOLOv5, YOLOv6, YOLOv7, YOLOv8, and SSD models. A highlighting point of this paper is comparing the state-of-the-art Instance Segmentation models, including Mask R-CNN, U-Net, YOLOv5 Instance Segmentation, YOLOv7 Instance Segmentation, and YOLOv8 Instance Segmentation. The extent of severity of NAFLD and non-alcoholic steatohepatitis was examined for liver cell ballooning, steatosis, lobular, and periportal inflammation, and fibrosis. Metrics used to evaluate the algorithms' effectiveness include accuracy, precision, specificity, and recall. Improved metrics are achieved by optimizing the hyperparameters of the associated models. Additionally, the liver is scored in order to analyse the information gleaned from biopsy images. Statistical analyses are performed to establish the statistical relevance in evaluating the score for different zones.
Citation: Soumyajit Podder, Abhishek Mallick, Sudipta Das, Kartik Sau, Arijit Roy. Accurate diagnosis of liver diseases through the application of deep convolutional neural network on biopsy images[J]. AIMS Biophysics, 2023, 10(4): 453-481. doi: 10.3934/biophy.2023026
[1] | Dafina Xhako, Niko Hyka, Elda Spahiu, Suela Hoxhaj . Medical image analysis using deep learning algorithms (DLA). AIMS Biophysics, 2025, 12(2): 121-143. doi: 10.3934/biophy.2025008 |
[2] | Soumyajit Podder, Somnath Bhattacharjee, Arijit Roy . An efficient method of detection of COVID-19 using Mask R-CNN on chest X-Ray images. AIMS Biophysics, 2021, 8(3): 281-290. doi: 10.3934/biophy.2021022 |
[3] | Muhammad Asif Zahoor Raja, Adeeba Haider, Kottakkaran Sooppy Nisar, Muhammad Shoaib . Intelligent computing knacks for infected media and time delay impacts on dynamical behaviors and control measures of rumor-spreading model. AIMS Biophysics, 2024, 11(1): 1-17. doi: 10.3934/biophy.2024001 |
[4] | Natarajan Mala, Arumugam Vinodkumar, Jehad Alzabut . Passivity analysis for Markovian jumping neutral type neural networks with leakage and mode-dependent delay. AIMS Biophysics, 2023, 10(2): 184-204. doi: 10.3934/biophy.2023012 |
[5] | Manuel Bedrossian, Marwan El-Kholy, Daniel Neamati, Jay Nadeau . A machine learning algorithm for identifying and tracking bacteria in three dimensions using Digital Holographic Microscopy. AIMS Biophysics, 2018, 5(1): 36-49. doi: 10.3934/biophy.2018.1.36 |
[6] | Mehmet Yavuz, Kübra Akyüz, Naime Büşra Bayraktar, Feyza Nur Özdemir . Hepatitis-B disease modelling of fractional order and parameter calibration using real data from the USA. AIMS Biophysics, 2024, 11(3): 378-402. doi: 10.3934/biophy.2024021 |
[7] | Warin Rangubpit, Sunan Kitjaruwankul, Panisak Boonamnaj, Pornthep Sompornpisut, R.B. Pandey . Globular bundles and entangled network of proteins (CorA) by a coarse-grained Monte Carlo simulation. AIMS Biophysics, 2019, 6(2): 68-82. doi: 10.3934/biophy.2019.2.68 |
[8] | Swarnava Biswas, Debajit Sen, Dinesh Bhatia, Pranjal Phukan, Moumita Mukherjee . Chest X-Ray image and pathological data based artificial intelligence enabled dual diagnostic method for multi-stage classification of COVID-19 patients. AIMS Biophysics, 2021, 8(4): 346-371. doi: 10.3934/biophy.2021028 |
[9] | Dinesh Bhatia, Tania Acharjee, Shruti Shukla, Monika Bhatia . Nano-technological advancements in multimodal diagnosis and treatment. AIMS Biophysics, 2024, 11(4): 464-507. doi: 10.3934/biophy.2024026 |
[10] | Kazushige Yokoyama, Christa D. Catalfamo, Minxuan Yuan . Reversible peptide oligomerization over nanoscale gold surfaces. AIMS Biophysics, 2015, 2(4): 649-665. doi: 10.3934/biophy.2015.4.649 |
Accurate detection of non-alcoholic fatty liver disease (NAFLD) through biopsies is challenging. Manual detection of the disease is not only prone to human error but is also time-consuming. Using artificial intelligence and deep learning, we have successfully demonstrated the issues of the manual detection of liver diseases with a high degree of precision. This article uses various neural network-based techniques to assess non-alcoholic fatty liver disease. In this investigation, more than five thousand biopsy images were employed alongside the latest versions of the algorithms. To detect prominent characteristics in the liver from a collection of Biopsy pictures, we employed the YOLOv3, Faster R-CNN, YOLOv4, YOLOv5, YOLOv6, YOLOv7, YOLOv8, and SSD models. A highlighting point of this paper is comparing the state-of-the-art Instance Segmentation models, including Mask R-CNN, U-Net, YOLOv5 Instance Segmentation, YOLOv7 Instance Segmentation, and YOLOv8 Instance Segmentation. The extent of severity of NAFLD and non-alcoholic steatohepatitis was examined for liver cell ballooning, steatosis, lobular, and periportal inflammation, and fibrosis. Metrics used to evaluate the algorithms' effectiveness include accuracy, precision, specificity, and recall. Improved metrics are achieved by optimizing the hyperparameters of the associated models. Additionally, the liver is scored in order to analyse the information gleaned from biopsy images. Statistical analyses are performed to establish the statistical relevance in evaluating the score for different zones.
Non-alcoholic fatty liver disease (NAFLD) is a worldwide issue that affects more than 10% of people globally [1]. The incidence of NAFLD exceeds 30% in industrialized nations, where corpulence and its accompanying illnesses of diabetes and metabolic disorders are prominent. In both adults and children with fatty liver disease, a liver biopsy examination remains an essential tool in clinical practice and scientific research. The ever-increasing complexity and number of complaints related to liver diseases pose a new set of challenges in efficiently detecting such diseases. NAFLD is characterized by an accumulation of excess adipose tissue in the hepatic region that is not induced by alcohol. NAFLD is a liver ailment marked by steatosis, which is one of the most frequent liver abnormalities in obese people. A fatty liver is characterized as one in which fat constitutes greater than 5% to 10% of the liver [2]. A liver biopsy is the benchmark process to quantify hepatic steatosis. A biopsy is an invasive technique (i.e., surgery) that has a significant chance of catastrophic consequences, including discomfort, internal bleeding, infection, and organ harm. The purpose of a liver biopsy is to provide crucial information for patient treatment, clinical trials, and continuing research on specific liver illnesses [3]. Though there are no symptoms associated with NAFLD, alcoholic fatty liver disease (AFLD), nonalcoholic steatohepatitis (NASH), and acute fatty liver of pregnancy (AFLP) are distinct forms of fatty liver diseases [3],[4]. Among these, NAFLD encloses a broad clinical spectrum, spanning from bland steatosis to NASH, that might advance to liver cirrhosis and hepatic carcinoma (HCC). If NAFLD advances to cirrhosis, then fluid retention, internal bleeding, and loss of healthy liver function may occur.
Alcohol retention prevents the liver from properly metabolizing fat, which results in AFLD. NASH develops when there is an abundance of liver fat and hepatic inflammation. It causes inflammation of the liver, which can ultimately result in cirrhosis and liver failure. Hepatitis B and C are two liver disorders brought on by viral infections [5]. There are two types of NAFLD detection methods: invasive and noninvasive. NAFLD is frequently detected using invasive techniques such as biopsies as well as non-invasive techniques such as ionizing radiation, computerized tomography (CT), magnetic resonance imaging (MRI), ultrasonography (USG), and liver enzyme tests. To find abdominal bleeding or injury, CT scans are used. This painless, non-invasive method of identifying internal damage may help save the lives of patients [6].
Due to the large sample size, the current procedures for the pathological diagnosis of the hepatic tissue are unable efficient, cost effective, and fast paced, which in turn demands new technology or methods to be imparted for the detection of liver diseases. Efficient liver disease diagnostic techniques using artificial intelligence (AI) is becoming indispensable. Recently, AI and deep learning have found tremendous applications in medical imaging. Sethunath et al. [7] executed a supervised machine learning algorithm to detect different areas in mouse liver biopsy pictures. Owjimehr et al. [8] proposed wavelet packet transforms to identify liver illness in ultrasound pictures. Additionally, computer-aided design (CAD) or deep learning algorithms have been utilized to diagnose NAFLD patients using ultrasound pictures [9]–[11]. Automated segmentation of the carotid intima-media thickness in ultrasound images was performed using a fast fuzzy c-mean clustering technique [12]. Tsiplakidou et al. [3] introduced a thresholding approach in which the fatty sections of images are monitored and assessed depending on the eccentricity of the area, whereas Liquori et al. [13] demonstrated the recognition of fat zones based on color homogeneity and circular shapes.
To identify hepatic steatosis, certain powerful machine learning methods have been applied. Additionally, convolutional neural networks (CNNs) have been utilized to automatically identify polyps in the colon [14]–[17]. Mulay et al. [6] accomplished liver segmentation utilizing MRI [18],[19] and CT data employing HEDMask-R-CNNs (holistically-nested edge detection mask-region-convolution neural network). Glomerulus detection in kidney biopsy images was performed utilizing a Mask R-CNN [20]. For hepatic segmentation from CT scan images, Cohen et al. [21] applied CNN. Along with the aforementioned studies, Tang et al. [22] executed Faster R-CNN and DeepLab for autonomous liver segmentation. Guo et al. [23] employed a deep learning method for hepatic steatosis segmentation to predict steatosis using boundary boxes and classification probability. From medical imaging, AI has always been a key component in illness detection [24],[25]. Utilizing Mask R-CNN, Podder et al. [26] identified COVID-19 using chest X-ray images with a great degree of accuracy and specificity. Applications of the You Only Look Once (YOLO) algorithm include skin lesion segmentation [27], identification of blood cells from human blood smears [28],[29], liver detection [30], and cholelithiasis and gallstone categorization in CT images [30].
Therefore, as far as NAFLD is concerned, the present status of the application of AI and deep learning has not been robustly explored; moreover, we have attempted various techniques of AI and deep learning on biopsy images of the liver. This research presents a methodology for accurately diagnosing hepatic steatosis that makes use of SSD (single shot multibox detector) [31], Faster R-CNN [32], and YOLO [33]. The application of these techniques is compared for a more effective and practical use. The proposed networks are not specific to solely liver biopsy images. Instead, these networks can also be applied to all kinds of microscopic images from slides.
Semantic segmentation is a deep learning technique that labels or categorizes each pixel in an image. Semantic segmentation, also referred to as image segmentation, is the technique of gathering areas of an image which correspond to the identical object class [34]. The use of semantic segmentation is found in medical imaging and diagnostics, self-driving cars, and facial recognition, and is a sophisticated technique for segmenting images that deals with finding instances of objects and identifying their boundaries with an image. In the case of segmentation, each object of interest that occurs in an image is both recognized and separated. Instance segmentation is crucial for autonomous vehicles, medical imaging, disease detection from microfluidic devices [35], and satellite photography. Instance segmentation is supported by the U-Net [36], Mask R-CNN [37], and numerous members of the YOLO family.
A deep convolutional network known as Faster R-CNN contains two stages and uses an end-to-end network with high accuracy. The network is capable of predicting the positions of myriad quickly and precisely. Each predictor improves the overall recall while predicting the particular object size, aspect ratio, or category, resulting in an overall improvement in recall. Along with object detection, Faster R-CNN utilizes a region proposal network to produce object proposals.
YOLO utilizes a single neural network to perform detection across the entire image. The network splits the picture into regions and forecasts the probability and bounding boxes for every region [38]. The forecast, which has the maximum IoU (Intersection over Union) with the ground truth, allocates a predictor for each prediction of an object. By employing SSD, we can recognize multiple items inside an image with just one shot, as opposed to RPN-based systems such as the R-CNN series, which require two shots-one for making region suggestions and for recognizing the item of each proposal. It uses multi-scale features and default boxes for higher efficiency. Identifying things simply involves predicting their class and placement within a given area.
An overview of the proposed methods and the biopsy dataset required for fatty liver disease detection is described below.
The dataset contains 21,435 images of liver biopsies. It is an open-source collection of data and is accessible in the Open Science Framework (https://osf.io/p48rd/) [39]. These anatomical images were captured on a Zeiss AxioScan Z1 scanner (Carl Zeiss, Jena, Germany), which is a high-definition color camera containing a 20× objective for bright field microscopy illumination and images with a pixel resolution of 0.22 m/px [40]. The images captured were 897 × 897 px2 in a BiggTIFF format. The sections of the liver were obtained from mice of various ages. The obtained sample was from an axial slice in the center of the liver lobe, which was a 3 µm thin section. It included both healthy and NAFLD/NASH liver. Additionally, Masson's trichrome staining was performed on the slides.
From the dataset, as large as 5,348 biopsy images were chosen for use in training, testing, and validating the Faster R-CNN, SSD, and YOLO algorithms. For this experiment, 4,000 images were taken for training, whereas 800 images were utilized for testing and 548 images were utilized for validation. The biopsy image dataset contained four classes, with 1,000 images each for the training dataset: ballooning, steatosis, inflammation, and fibrosis. On a single graphics processing unit (GPU), tests were conducted (16 GB RAM, NVIDIA GeForce RTX 3080 Ti) for the dataset. These images were annotated by skilled pathologists [41]. Data preprocessing included resizing the images to 299×299 px2 for evaluation. Augmentations of the dataset were performed to boost its size and enhance training effectiveness. In this study, different augmentation techniques such as rotation, flipping, resizing, and gaussian blurring were considered.
Faster R-CNN is an algorithm used in object recognition that predicts the object's location utilizing the RPN (region proposal network). Fast R-CNN utilizes only region of interest (ROI) pooling, which consumes more time as compared to Faster R-CNN (which utilizes RPNs), and thus directly produces region proposals. RPN has been used in Faster R-CNN to supplement the selective search method employed by Fast R-CNN. VGG-16 was used to acquire an accurate valuation of an image. By its pooling layer, the ROI generates a feature map of uniform size [42]. The method was tested using the PASCAL VOC 2007 dataset.
The ROI pooling layer receives bounding boxes of numerous forms and dimensions. For each anchor, the ROI pooling layer extracts fixed size feature maps. A fully connected layer with a Softmax activation function and a linear regression layer receives the feature maps. Finally, it separates fatty liver cells and forecasts the bounding boxes for the cells that have been found. The classification and bounding box regression losses are combined in the following multi-task loss function:
where i and pi represent the anchor's index and the predicted likelihood that the anchor is a fatty liver tissue, respectively. The value of the ground truth label, represented by
The following equation indicates the regression loss:
where R indicates smooth L1 function. Figure 1 depicts the Faster R-CNN architectural layout. The accurate diagnosis of fat tissues in the liver is accomplished using Faster R-CNN.
SSD is another useful technique to identify steatosis in the liver. SSD is a form of convolutional neural network dependent on the feed-forward convolutional neural network, in which the nodes do not form a loop, which creates fixed-sized bounding boxes and a score for the liver tissues to be recognized within those boxes. To obtain the final detections, the non-max suppression step is followed. In contrast to CNN, SSD separately detects objects using multi-scale feature maps.
The base network, the additional feature extraction layer, and the prediction layer make up the SSD architecture. The initial layer of any conventional image categorization within the neural network is the base network. The feature maps are derived utilizing VGG-16.
At the conclusion, the convolutional layers take the place of completely linked layers. SSD generates anchor boxes and predicts their categories and offsets using feature maps, which are fundamentally based on many scales, as shown in Figure 2. The loss function consolidates the classification loss and bounding box regression loss [43]:
The classification loss is calculated using the subsequent equation:
where
The regression loss is shown by the following equation:
where g indicates ground truth boxes, l indicates predicted boxes,
You Only Look Once, also known as YOLO [44], is a relatively recent strategy that relies on regression. For the entire image under study, YOLO is used to forecast classes and boundary boxes in only one algorithm run. Its most common application is real-time object detection. YOLOv5, YOLOv7, and YOLOv8 all include instance segmentation as an extra feature with a rather high mAP (mean average precision) for each model.
A new era in object identification and segmentation began with the introduction of anchor boxes for YOLOv2 in 2017. On top of the current YOLO model, several enhancements were made. Its successor, YOLOv3, which generated predictions at three different granularity levels, was created in 2018. The newer YOLO models focused on advances such as feature aggregations and architectural improvements enabled by PyTorch in YOLOv4 and YOLOv5, respectively.
Other notable mentions in this category of algorithms with performance enhancements include PP-YOLO, Scaled YOLOv4, and PP-YOLOv2. In accordance with modifications in its architecture, the YOLOv6 algorithm also included a decoupled head, which has proven to increase its performance. YOLOv7 has a shorter gradient in the back propagation layers, thereby increasing the efficiency of the algorithm. Currently, YOLOv8 is among the most reliable algorithms in the world of computer vision, alongside an association of a tracking component.
The image is initially scaled to 224×224 pixels. Then, the picture is divided into 7 × 7 grid cells, each of which is responsible for estimating bounding boxes. Non-max suppression removes the bounding boxes that has the maximum common area and the boxes with a low likelihood of containing the classes. The anchor box allows the YOLO algorithm to identify several objects that are centered in a single grid cell [39],[45]. The method employs a single neural network for detection. The architecture of YOLO is illustrated in Figure 3.
At first, the model was tested on the PASCAL VOC detection dataset. The network design includes 24 convolutional layers and two fully connected layers for prediction. We have only one class for identifying hepatic steatosis. For the anchor box, the bounding box parameters and prediction probability are determined. As a result of the presence of five anchor boxes, it is likelihood that the object will be present in the grid cell pc; and therefore, the object's central coordinates (x, y) corresponding to the cell's top left corner of the predicted class, as well as the length and width of the rectangle's enclosing box can be calculated. The bounding box represents the newly identified fatty hepatic tissues. Consequently, if NF is the number of filters in the final convolution layer, NA is the number of anchor boxes and NC is the number of classes, which is summarized in the following equation:
The YOLO algorithm, which is used to automatically diagnose fatty liver, uses an acceptable threshold. The typical absolute difference between our guess and the underlying data at different thresholds levels is used to compute the threshold value. The final generated prediction boxes on the photos tally the number of fatty liver tissues in the output. The settings define the boundary boundaries that surround each uncovered tissue.
The total loss consists of classification, localization, and confidence losses combined. The total loss function calculated in YOLO algorithm is given by the following:
where either
In this paper, the instance segmentation algorithms explored for the diagnosis of liver conditions include Mask R-CNN, U-Net, YOLOv5 Instance Segmentation, YOLOv7 Instance Segmentation, and YOLOv8 Instance Segmentation. The family of YOLO algorithm frameworks has shown consistent improvement, not just in image recognition, but also in instance segmentation. Within the duration of a couple of years, YOLO models have gained significant acclaim, not just from the community of people working in the field of computer vision, but also from associations of medical science because of their high accuracy of detection and faster processing of videos. The primary importance of using a YOLO model is its small size, which enables them to deploy in resource-constrained parallel computing edge devices while still allowing faster inference speeds.
The aforementioned algorithms work both on images and videos. Therefore, they may also find applications in various AI-aided medical imaging applications in X-ray, Ultrasound, CT, MRI, positron emission tomography (PET), single photon emission computed tomography (SPECT), and video applications in surgical endoscopy and capsule endoscopy [48]. The Mask R-CNN architecture has an extra layer for the prediction of the segmentation on top of the layers in Faster R-CNN. Thus, bounding boxes are generated along with masks for the ROIs. It consists of several layers including the convolutional layer, pooling layer, and fully connected layer. U-Net is one of the most popular algorithms for instance segmentation, and the derived characteristics have grown more abstract as the neural networks have grown even deeper. U-Net consists of several up-sampling and down-sampling steps.
A grade is the global measures of liver cells and inflammatory response due to injury which shows potentially changeable characteristics. The stage is an evaluation of the position of fibrosis [49] and constructive alteration; therefore, it is practically reversible. The grade describes the quantity [50], whereas the stage does not. The stage only provides information on the parenchymal location of collagen and matrix buildup, as well as modifications to the vascular/constructive system. Compared to staging, morphological measurement of fibrosis in hepatic disorders necesstitates a particular strategy that yields prominent but more contrasting information. There are three types of grades in scoring of liver: mild, moderate and severe [51],[52]. However, scoring cannot be applied to stages due to the inclusion of fibrosis location and constructive modifications when present, such as in cirrhosis.
Scoring can be performed after Hematoxylin and Eosin or Masson's trichrome staining for the evaluation of several biopsies from patients in clinical trials. The histological features observed in the human liver are steatosis, lobular inflammation, ballooning, periportal inflammation, and fibrosis. The unweighted total NAFLD Activity Score (NAS) is independently calculated for each lesion. The value of NAS spans from 0−8. It comprises of steatosis (0-3), lobular inflammation (0-3), and hepatocyte ballooning (0-2) [53]. Ballooning injury & steatosis mainly contribute to inflammation & fibrosis in the NAFLD score framework [39]. Further morphological structures include acidophil bodies, Mallory-Denk bodies and the zonal location of steatosis. The staging of fibrosis progresses from none to portal or periportal. It might advance to bridging fibrosis and consequently cirrhosis in a linear manner. In NASH and NAFLD, the fibrosis progresses from none to perisinusoidal. Then, it advances to periportal or bridging. Bridging fibrosis might lead to cirrhosis of the liver [54]. At the moment, the most popular grading scale is the NAS.
The distinct types of scoring frameworks are the Brunt system, NASH-CRN system & SAF system. Brunt et al. [50] divided the micro-inflammatory grades of NASH into grades: 1,2, and 3 [55]. Overall, they suggested a fibrosis severity and location-based rating system: zone 3 of stage 1 perisinusoidal fibrosis; stage 2 portal fibrosis along with the aforementioned stage 1; stage 3 bridging fibrosis in addition to stage 2; and stage 4 is cirrhosis. Stage 1, zone 3 is divided into the subcategories 1A, 1B, and 1C, which correspond to mild, moderate, and only portal/periportal, respectively [56]. Sorely obese patients and pediatric patients sporadically manifest fibrosis [57]. The NASH Clinical Research Network (NASH-CRN) formulated the NAS for clinical exploration. The primary objective of the NAS is to access the etiological changes in the patient's liver with time. Recent work has utilized the criterion value of NAS, particularly NAS ≤ 5, as a substitute for the cytological determination of NASH. The SAF activity score is used to calculate hepatocyte ballooning and lobular inflammation, and a score of ≥ 3 indicates either bridging fibrosis or cirrhosis.
The variables are continuous and is evidenced by mean and standard deviation. In order to predict quantitative data, percentages are used with the numbers. A paired sample T-Test is used to contrast the normally disctributed continuous variables. A one-way analysis of variance (UNIANOVA) was executed for the NAFLD diagnosis of different classes [58]. Weighted Kappa scores can be of two types: Inter-Rater & Intra-Rater. The intraclass correlation coefficient was obtained from the component of the variance model. The histological characteristics obtained from the diagnosis of steatohepatitis can be performed using the Chi-square test. A Chi-Square Test is an examination for autonomy; it indicates if there is a link but does not indicate the strength of the association. We measured the effect size using Cramer's V. Fisher's exact test and the Chi-Square test were performed using a Yates' correction test for the data. The p-values can be obtained from Mantel Haenszel χ2 test for satisfying 2 × 2 tables. The IBM SPSS Version 27 and Graph Pad Prism software were used for statistical evaluation [55].
In statistics, the intraclass correlation coefficient is a summarization of data that can be applied when numerical quantifications are made on units that are assembled into groups. It expresses the units in the same group that are highly similar to each other. The inter-rater reliability determines the degree of consistency or reliability in a process. The inter-rater reliability is also estimated with Cohen's Kappa. Cohen's Kappa allows us to evaluate the inter-rater reliability when we have nominal or ordinal variables. We want to determine the –inter-rater reliability between these two classes. The Kruskal-Wallis Test is a one-way ANOVA's non-parametric counterpart. The dependent variable must be continuous, observations must be independent, there must be no notable outliers, homogeneity of variance, and an independent variable must exist with two or more categorical groups. Additionally, the dependent variable must have a distribution that is roughly normal at each level of the independent variable. Now, the assumptions for the Kruskal-Wallis test are slightly different. The data points must be independent of one another, there must be five data points in each sample, participants must be chosen at random from the population, and the sample size must be roughly equal. Both the normality of the distribution and the equality of the variances are not requirements.
For the Wilcoxon W, if the asymptotic significance value is 0.05 or less, then there is a significant difference between the two scores. The Z-score is a numerical computation indicating a value's linkage to the mean of a batch of values. Determination of the Z-score is performed with regard to standard deviations from the average. If the Z-score is zero, it implies that the value of the data element is similar to the average value. Multivariate associations with the identification of steatohepatitis were evaluated utilizing multinomial logistic regression models, which can produce an odds ratio with 95% confidence intervals. Additionally, these regression models were utilized to calculate the p-values. After performing a Bonferroni correction for numerous comparisons, a p-value of 0.025 was regarded to be important.
Although the liver biopsy is the gold standard for detecting liver steatosis, it is insufficient in determining the disease's frequency in a given population [59]. Steatosis refers to the buildup of triglycerides as macromolecules within the cytoplasm of hepatocytes. This condition must be present for any form of NAFLD to exist. A macrovesicular steatosis pattern will be present in the fat. Large vacuoles, called fat vacuoles, usually contain a macrovesicular steatosis pattern in each cytoplasm and push the nucleus to one side. On one hand, some regions have medium-sized fat droplets, while others have very minute ones. This study uses metrics often utilized for object detection tasks. The four rectangle-shaped coordinates (x,y,w,h) of the identified bounding boxes constitute the model's output, the parameters of which are shown below.
True Positive (TP): the accurate recognition outcome if the recognized box corresponds to fatty liver tissue ground truth.
False Positive (FP): the improper recognition outcome where the identified box lies outside fatty liver tissue ground truth.
True Negative (TN): no recognition for images in which fatty liver cells are not present.
False Negative (FN): no recognition for images in which fatty liver cells are present.
To evaluate the performance, these parameters are used to calculate the following metrics [24]:
The diagnostic performance of various algorithms for fatty liver disease has been illustrated in Table 1. The model's performance is reflected by its value, which should be as near to 1 as possible.
Method | TP | FP | TN | FN | AC (%) | SP (%) | PR (%) | RC (%) | F1 (%) | F2 (%) |
Faster R-CNN | 710 | 21 | 69 | 0 | 97.375 | 76.67 | 97.127 | 100 | 98.543 | 99.411 |
SSD | 653 | 10 | 134 | 3 | 98.375 | 93.055 | 98.491 | 99.543 | 99.014 | 99.33 |
YOLO v3 | 728 | 17 | 55 | 0 | 97.875 | 76.39 | 97.718 | 100 | 98.846 | 99.535 |
YOLO v4 | 731 | 11 | 57 | 1 | 98.5 | 83.823 | 98.517 | 99.863 | 99.185 | 99.591 |
YOLO v5 | 793 | 1 | 6 | 0 | 99.875 | 85.714 | 99.874 | 100 | 99.937 | 99.975 |
YOLO v6 | 744 | 12 | 42 | 2 | 98.25 | 77.78 | 98.413 | 99.732 | 99.068 | 99.465 |
YOLO v7 | 746 | 11 | 38 | 5 | 98 | 77.551 | 98.547 | 99.334 | 98.939 | 99.176 |
YOLO v8 | 795 | 1 | 4 | 0 | 99.875 | 80 | 99.874 | 100 | 99.937 | 99.975 |
For 8,000 iterations, the average loss are found to be 0.383155 and 1.779745 for YOLOv3 and YOLOv4, respectively. For 100 epochs, the total loss for YOLOv5, YOLOv6, YOLOv7, and YOLOv8 algorithms are 0.0247318, 2.3778, 0.03914, and 0.023472, respectively. The mAP value for YOLOv8 is found to be 99.1%, which is higher than the mAP value of all other algorithms which are used to test the models. The IoU value obtained is 0.999 for YOLOv8, thereby indicating that the predicted bounding box is quite close to the ground truth bounding box. The TP, TN, FP, and FN for the YOLOv8 algorithm are 99.375%, 0.125%, 0.5%, and 0%, respectively. The accuracy, specificity, precision, recall, F1-score, and F2-score are 99.875%, 80%, 99.874%, 100%, 99.937%, and 99.975%, respectively. The FP is considerably low while testing the YOLOv8 model. A threshold value of 30% is utilized while testing every algorithm. The batch size of 16 is taken for training all the models. The performance comparison of the instance segmentation models is illustrated in Table 2.
The analysis demonstrates that the suggested method can measure hepatic steatosis and properly identify fat. For quicker processing, the suggested approach has been improved. Table 3 displays the evaluation of the performance of various methods and a comparison of mAP and IoU. Figure 4 displays the results of Faster R-CNN, SSD, YOLOv3, and YOLOv4 for various classes. These models can be deployed effectively and efficiently on a server, mobile device, or edge device. According to the instance segmentation findings, the Mask R-CNN's AC and SP are 97% and 81.905%, respectively, which are much better than all other methods.
Method | TP | FP | TN | FN | AC (%) | SP (%) | PR (%) | RC (%) | F1 (%) | F2 (%) |
Mask R-CNN | 690 | 19 | 86 | 5 | 97 | 81.905 | 97.32 | 99.281 | 98.291 | 98.883 |
U-Net | 623 | 25 | 144 | 8 | 95.875 | 85.207 | 96.142 | 99.732 | 97.904 | 98.993 |
YOLO v5 | 694 | 16 | 39 | 51 | 91.625 | 70.909 | 97.746 | 93.154 | 95.395 | 94.038 |
YOLO v7 | 578 | 37 | 48 | 137 | 78.25 | 56.471 | 93.984 | 80.839 | 86.917 | 83.165 |
YOLO v8 | 710 | 24 | 32 | 34 | 92.75 | 57.143 | 96.73 | 95.43 | 96.076 | 95.687 |
Model | mAP (%) | IoU |
Faster R-CNN | 49.2 | 0.971 |
SSD | 53.1 | 0.98 |
YOLOv3 | 54.3 | 0.977 |
YOLOv4 | 65.6 | 0.984 |
YOLOv5 | 98.9 | 0.999 |
YOLOv6 | 56.4 | 0.982 |
YOLOv7 | 59.6 | 0.979 |
YOLOv8 | 99.1 | 0.999 |
The hallmark of ballooning in the liver is an enlarged hepatocyte with a rarefied cytoplasm. With hematoxylin and eosin staining, the detection is challenging; therefore, our deep learning methodology is found to be useful for a proper diagnosis. Fibrosis in NASH can be either perisinusoidal or pericellular. The infiltration of mixed inflammatory cells that characterizes lobular inflammation in NASH is often modest, while periportal inflammation is rare in NASH and occurs mainly in other hepatic disorders such as hepatitis C and autoimmune hepatitis [56]. In NAFLD patients, liver steatosis manifests as either little or big fat droplets.
According to the correlation values from the paired sample T-test, a patient with low ballooning scores is very likely to have low inflammation scores, and vice versa. Likewise, a patient with low inflammation scores is very likely to have low steatosis scores, and vice versa. However, for a patient with low ballooning score, a high steatosis score is very certain, and vice versa. Similarly, for a patient with low ballooning score, a high fibrosis score is very certain, and vice versa. UNIANOVA is performed to check if there is a difference among the classes, and the post hoc Bonferroni test is performed to discover the degree of difference between the classes. The intraclass correlation coefficient is 0.333, which is also statistically significant.
When evaluated for the association of ballooning with inflammation, ballooning with steatosis, ballooning with fibrosis, inflammation with steatosis, inflammation with fibrosis, and steatosis with fibrosis, the Pearson Chi-Square values are 71.498, 22.295, 51.678, 78.567, 131.217, and 131.217, respectively. All of them are found to be statistically significant, and therefore, we can conclude that there is a significant association between the classes. The Cramer's value is found to be such that there is a small to moderate effect of each class on the other class. Here it is established with the intraclass correlation coefficient, which is 0.667 when the scores for all four classes are taken into consideration. It indicates that 66.7% of the consistency is noted among the statistically significant scores. We see that for a single measurement, the intraclass correlation coefficient is 0.333, which is also statistically significant.
The highest correlation is observed between the inflammation score and the fibrosis score, which is 0.516; the lowest correlation is observed between the ballooning score and the steatosis score, which is 0.003. Between the four classes, we have a moderate inter-rater reliability. When comparing scores of ballooning with inflammation, inflammation with steatosis, inflammation with fibrosis, and steatosis with fibrosis, the Cohen's Kappa value are 0.205, 0.187, 0.191, and 0.347, respectively, which are found to be statistically significant, and the rest are found statistically insignificant.
The Kruskal-Wallis H for ballooning, fibrosis, inflammation, and steatosis are 71.739, 86.237, 121.546, and 76.626, respectively. The Mann-White U-Test is performed and the values are found to be statistically significant for all classes. The Wilcoxon W is 21 for the ballooning score, 29 for the inflammation score, 35 for the steatosis score, 33 for the fibrosis score, and the Z-score is -3.028 for the ballooning score, which is statistically significant, -0.202 for the inflammation score, -0.213 for steatosis score, and -0.601 for fibrosis score, which are not statistically significant.
From the multinomial logistic regression model, we can conclude that when steatosis is from 5% to 33%, we observe inflammation with no foci, < 2 foci, and 2 to 4 foci. When steatosis is greater than 33% to 66%, we see inflammation < 2 foci per 200x field and 2 to 4 foci per 200x field. If steatosis is from 5% to 33%, we observe no ballooning. However, if steatosis is greater than 33% to 66%, a few ballooning cells occur. When there are numerous ballooning cells, we observe < 2 foci per 200x field [60]. On the other hand, when there are few ballooning cells, we observe no fibrosis, perisinusoidal or periportal fibrosis, perisinusoidal, and periportal fibrosis. Additionally, when there are a lot of ballooning cells, we observe perisinusoidal or periportal fibrosis, perisinusoidal, and periportal fibrosis, and bridging fibrosis. From the analysis, we observed that when there is no inflammation. When there is no fibrosis and when steatosis is < 5%, we observe perisinusoidal and periportal fibrosis.
For the purpose of detecting the fat automatically in the liver from B-mode ultrasound image sequences, Byra et al. [2] used an Inception-ResNet-v2 deep convolutional neural network that had been previously trained on the ImageNet dataset. They achieved an accuracy of 90.9% and a specificity of 94.1%. By incorporating migration learning into the DenseNet model, Yang et. al. [46] developed a deep learning-based technique to grade liver steatosis. Then, the system's efficiency was confirmed by using it to grade actual cases of liver steatosis. The model has an accuracy of about 88.5% and a specificity of about 80%. A customized CNN deep learning model was developed by Arjmand et. al. with an accuracy and specificity of 95% and 98.3%, respectively [61],[62].
A CNN to assess scores of the liver was developed by Heinemann et. al. [39] with an accuracy of 90.63%. Ugail et. al. [63] demonstrated a deep learning algorithm to classify livers suitable for transplantation, while achieving a high accuracy of 99.63%. Gaber et. al. designed a voting-based classifier and machine learning algorithm, which are used to construct a computer-aided diagnosis method that classifies hepatic tissues as either fatty or normal, utilizing attributes extracted from ultrasound images [64] with an accuracy of 95.71% and specificity of 94.44%. A random forest model by Wu et. al. [65] showed a high accuracy and specificity of 86.48% and 85.89%, respectively.
In this work, for the YOLOv8 algorithm, the larger model YOLOv8-x is used as a pretrained weight. Different state-of-the-art algorithms such as YOLOv5, YOLOv6, YOLOv7, and YOLOv8 have been used to obtained the following output, as shown in Figure 5. A better architecture could emerge by fine-tuning the hyperparameters associated with an architecture. The architecture, such as Faster R-CNN, YOLOv8, and Mask R-CNN, is fine-tuned with several hyperparameters, and the results of this tuning are shown in Figure 6. The onfidence threshold is set to 80%. YOLOv8 performs better in terms of Average Precision on the MS COCO dataset. The hyperparameters are fine-tuned with PyTorch and it is observed that the losses are reduced (See Figure 6). The batch size, learning rate, number of epochs, anchor boxes, and IoU threshold can be adjusted for each specific application. In this work, the hyperparameters are tuned for an improved accuracy that includes setting the initial learning rate to 0.01, SGD momentum to 0.937, and warmup epochs to 3. The IoU (intersection over union) training threshold is set to 0.2 and the anchor multiple thresholds is set to 4 for better mAP. These results are shown in Table 3. The investigation on the fine-tuning of the hyperparameters yields a higher accuracy and decreased training time. The performance of the instance segmentation algorithms is illustrated in Figure 7 and Figure 8, respectively. Table 4 compares various efficient AI-based models for detecting liver diseases.
It is quite possible to evaluate the overall steatosis percentage from the biopsy images according to physican's requirement. To determine the percentage of steatosis on a slide, the average of the marks is obtained and compared to the overall area of the image. Thus, the percentage of steatosis obtained:
Model used | Reference | AC (%) | SP (%) | PR (%) | RC (%) | F1 (%) |
YOLOv5 | Our model | 99.875 | 85.714 | 99.874 | 100 | 99.937 |
Steatosis detection using eccentricity and roundness | [3] Tsiplikadou et al. | 97.78 | - | 100 | 97.78 | - |
Transfer learning to extract CNN-based features | [2] Byra et al. | 90.9 | 94.1 | - | 89.5 | - |
Mask R-CNN ResNet50 | [23] Guo et al. | - | - | 75.87 | 60.66 | 65.88 |
Supervised machine learning model | [7] Sethunath et al. | 84.26 | - | 95.01 | 94.23 | - |
Improved DenseNet | [66] Yang et al. | 88.49 | 81.6 | - | 95.44 | - |
Deep learning using classification network | [10] Cao et al. | 95.45 | - | - | - | - |
Curvelet Transform and Entropy feature extraction | [11] Acharya et al. | 97.33 | 100 | - | 96 | - |
ROI select and SVM classifier | [8] Owjimehr et al. | 97.9 | - | 92.7 | 100 | - |
CNN SGDM model | [61] Arjmand et al. | 95 | 98.3 | 95 | 95 | 95 |
Trained CNN | [39] Heinemann et al. | 90.63 | - | - | - | - |
ImageNet model with SVM classifier | [63] Ugail et al. | 99.63 | - | - | - | - |
CAD model with voting-based classifier | [64] Gaber et al. | 95.71 | 94.44 | 94.28 | 97.05 | 95.64 |
Random forest model | [65] Wu et al. | 86.48 | 85.89 | - | 87.16 | - |
The scoring of the liver according to histology is illustrated in Figure 12. The scoring system definitions, scores, and total number of detections done are illustrated in Table 5. Table 6 indicates the Wilcoxon W, Kruskal-Wallis H, and Z-score of the different liver conditions. The different classes for ballooning, steatosis, inflammation, fibrosis, and background classes for YOLOv5 algorithm are shown in the Confusion Matrix in Figure 13. The graphs in Figure 14 show the variation of F1-score vs Confidence, Precision vs Confidence, Precision vs Recall, and Recall vs Confidence for YOLOv5 algorithm.
Histology type | Definition | Scores of liver | Number of images |
Low- to medium-power evaluation of parenchymal involvement by steatosis | |||
Steatosis Grade | <5% | 0 | 145 |
5%-33% | 1 | 35 | |
>33%-66% | 2 | 72 | |
>66% | 3 | 6 | |
None | 0 | 129 | |
Perisinusoidal or periportal | 1 | 31 | |
Fibrosis Stage | Perisinusoidal and portal/periportal | 2 | 31 |
Bridging fibrosis | 3 | 52 | |
Cirrhosis | 4 | 15 | |
Lobular inflammation | No foci | 0 | 70 |
<2 foci per 200 × field | 1 | 51 | |
2-4 foci per 200 × field | 2 | 89 | |
>4 foci per 200 × field | 3 | 48 | |
Ballooning | None | 0 | 172 |
Few balloon cells | 1 | 30 | |
Many cells/prominent ballooning | 2 | 56 |
Item | Wilcoxon W | Kruskal-Wallis H | Z-score |
Steatosis score | 35 | 76.626 | -0.213 |
Ballooning score | 21 | 71.739 | -3.028 |
Inflammation score | 29 | 121.546 | -0.202 |
Fibrosis score | 33 | 86.237 | -0.601 |
A liver biopsy procedure is commonly conducted on NAFLD patients to either confirm or rule out the diagnosis, identify any associated liver diseases, and determine the degree of liver liver damage, if any, for treatment and prognosis. The biopsy images used in this work are high-resolution (2×magnification) images, which are acquired using a microscope from a pathological laboratory to achieve a higher degree of accuracy. The key benefit of the suggested method over reported ones is that the processing time is same even though we are using biopsy images of a higher resolution. It is to be noted here that obtaining high-resolution images (which are demonstrated in this article) takes time and requires advanced equipment [67]. As a result, processing high-quality images takes much less time and requires low computing power. Another benefit of the suggested method is that the entire process is automated without manual involvement.
Recently, there have been significant advancements in the field of computer vision. It is utilized for a variety of practical applications, including disease diagnosis and therapy. Our models are designed to provide a more user-friendly technique for liver disease diagnosis while reducing the loss of efficiency due to the lack of data, as we are using a large number of samples. To evaluate which technique is more accurate and efficient, we made comparisons among the networks under consideration. Compared to other algorithms, YOLOv5, YOLOv6, YOLOv7, and YOLOv8 have faster training, testing and outperform them regarding their mAP and IoU values (note that our algorithms are fine-tuned with the associated hyperparameters). The YOLO algorithms depend on the PyTorch framework. Utilizing a large dataset of liver biopsy images, the full training and testing process is conducted on a single GPU for 100 epochs and the results are found to be robust.
Deep learning frameworks have been the fastest-growing approach for biomedical image analysis. The baseline histological criteria for NASH diagnosis, listed most recently by the American Association for the Study of Liver Diseases (AASLD) suggestions are steatosis, lobular inflammation, and ballooning in the liver [51],[68]. Our suggested methodology makes it simple to identify hepatic steatosis from liver biopsy images. The overall loss for each method is determined to be quite minimal. An accurate diagnosis of steatosis is crucial for understanding the pathophysiology of the condition and evaluating the effectiveness of therapeutic treatment. A radiologist may take enough time to study a patient's image, depending on how challenging a case is; however, the deep learning model requires only a few seconds. In the future, clinical routines may combine deep learning algorithms and CAD technologies [64].
With Faster R-CNN, the region proposals' bottleneck is removed. In order to improve the robustness of region proposals, the learned RPN is used, which enhances the overall accuracy of object detection. SSD benefits from eliminating proposal generation and uses just one deep neural network. The SSD algorithm's performance is quite dependable because it utilizes default boxes with different aspect ratios for every feature map position. On the other hand, YOLO has the benefit of simultaneously completing the bounding box and class forecasting. The mAP values and accuracy of the YOLO algorithms are found to be higher than those of other cutting-edge algorithms. Given that the processing time is reduced and the images are easily obtained, the suggested technique is simple enough to incorporate into ordinary clinical practice. The algorithms utilized in this study could be applied in other investigations to pinpoint additional stomach problems.
Artificial intelligence is gaining popularity in medical imaging due to its improved performance in image-recognition techniques. This study is used to understand different patients with different degrees of hepatic steatosis. We are able to obtain a promising degree of accuracy in the testing phase with faster prediction based on the number of annotations in biopsy images during training. Based on convolutional neural network models such Faster R-CNN, YOLO, and SSD, the suggested technique displays specificity, accuracy, and recall for fatty liver diagnosis. For Faster R-CNN, YOLOv8, and SSD, the accuracy is 97.375%, 99.875%, and 98.375%, respectively. Hence, the algorithms are proven to reduce human error in steatosis detection.
The authors declare they have not used Artificial Intelligence (AI) tools in the creation of this article.
[1] |
Berthold MR, Feelders A, Krempl G Advances in Intelligent Data Analysis XVIII (2020). https://doi.org/10.1007/978-3-030-44584-3 ![]() |
[2] | Byra M, Styczynski G, Szmigielski C, et al. (2018) Transfer learning with deep convolutional neural network for liver steatosis assessment in ultrasound images. Int J Comput Ass Rad 13: 1895-1903. https://doi.org/10.1007/s11548-018-1843-2 |
[3] |
Tsiplakidou M, Tsipouras M, Giannakeas N, et al. (2017) Automated detection of liver histopathological findings based on biopsy image processing. Information 8: 36. https://doi.org/10.3390/info8010036 ![]() |
[4] |
Siddiqui MS, Vuppalanchi R, Van Natta ML, et al. (2019) Vibration-controlled transient elastography to assess fibrosis and steatosis in patients with nonalcoholic fatty liver disease. Clin Gastroenterol H 17: 156-163. https://doi.org/10.1016/j.cgh.2018.04.043 ![]() |
[5] |
Lin J (2014) Virus-related liver cirrhosis: Molecular basis and therapeutic options. World J Gastroentero 20: 6457. https://doi.org/10.3748/wjg.v20.i21.6457 ![]() |
[6] | Mulay S, Deepika G, Jeevakala S, et al. (2019) Liver segmentation from multimodal images using HED-mask R-CNN. Multiscale Multimodal Medical Imaging : 68-75. https://doi.org/10.1007/978-3-030-37969-8_9 |
[7] |
Sethunath D, Morusu S, Tuceryan M, et al. (2018) Automated assessment of steatosis in murine fatty liver. PLos One 13: e0197242. https://doi.org/10.1371/journal.pone.0197242 ![]() |
[8] |
Owjimehr M, Danyali H, Helfroush M (2015) An improved method for liver diseases detection by ultrasound image analysis. J Med Signals Sens 5: 21. https://doi.org/10.4103/2228-7477.150387 ![]() |
[9] | Huang Q, Zhang F, Li X (2018) Machine learning in ultrasound computer-aided diagnostic systems: a survey. BioMed Res Int 2018: 5137904. https://doi.org/10.1155/2018/5137904 |
[10] |
Cao W, An X, Cong L, et al. (2019) Application of deep learning in quantitative analysis of 2-dimensional ultrasound imaging of nonalcoholic fatty liver disease. J Ultras Med 39: 51-59. https://doi.org/10.1002/jum.15070 ![]() |
[11] |
Acharya UR, Raghavendra U, Fujita H, et al. (2016) Automated characterization of fatty liver disease and cirrhosis using curvelet transform and entropy features extracted from ultrasound images. Comput Biol Med 79: 250-258. https://doi.org/10.1016/j.compbiomed.2016.10.022 ![]() |
[12] |
Naik VN, Gamad RS, Bansod PP (2022) Effect of despeckling filters on the segmentation of ultrasound common carotid artery images. Biomed J 45: 686-695. https://doi.org/10.1016/j.bj.2021.07.002 ![]() |
[13] | Liquori GE, Calamita G, Cascella D, et al. (2009) An innovative methodology for the automated morphometric and quantitative estimation of liver steatosis. Histol Histopathol 24: 49-60. https://doi.org/10.14670/HH-24.49 |
[14] |
Qadir HA, Balasingham I, Solhusvik J, et al. (2020) Improving automatic polyp detection using CNN by exploiting temporal dependency in colonoscopy video. IEEE J Biomed Health 24: 180-193. https://doi.org/10.1109/JBHI.2019.2907434 ![]() |
[15] |
Shin Y, Qadir HA, Aabakken L, et al. (2018) Automatic colon polyp detection using region based deep CNN and post learning approaches. IEEE Access 6: 40950-40962. https://doi.org/10.1109/ACCESS.2018.2856402 ![]() |
[16] |
Zhang X, Chen F, Yu T, et al. (2019) Real-time gastric polyp detection using convolutional neural networks. PLos One 14: e0214133. https://doi.org/10.1371/journal.pone.0214133 ![]() |
[17] |
Nogueira-Rodríguez A, Domínguez-Carbajales R, Campos-Tato F, et al. (2021) Real-time polyp detection model using convolutional neural networks. Neural Comput Appl 34: 10375-10396. https://doi.org/10.1371/journal.pone.0214133 ![]() |
[18] |
Lundervold AS, Lundervold A (2019) An overview of deep learning in medical imaging focusing on MRI. Z Med Phys 29: 102-127. https://doi.org/10.1016/j.zemedi.2018.11.002 ![]() |
[19] |
Zhen S, Cheng M, Tao Y, et al. (2020) Deep learning for accurate diagnosis of liver tumor based on magnetic resonance imaging and clinical data. Front Oncol 10: 680. https://doi.org/10.3389/fonc.2020.00680 ![]() |
[20] |
Yang CK, Lee CY, Wang HS, et al. (2022) Glomerular disease classification and lesion identification by machine learning. Biomed J 45: 675-685. https://doi.org/10.1016/j.bj.2021.08.011 ![]() |
[21] |
Ben-Cohen A, Diamant I, Klang E, et al. (2016) Fully convolutional network for liver segmentation and lesions detection. Deep Learning and Data Labeling for Medical Applications : 77-85. https://doi.org/10.1007/978-3-319-46976-8_9 ![]() |
[22] | Tang W, Zou D, Yang S, et al. (2018) DSL: Automatic liver segmentation with faster R-CNN and deepLab. Artificial Neural Networks and Machine Learning–ICANN : 137-147. https://doi.org/10.1007/978-3-030-01421-6_14 |
[23] | Guo X, Wang F, Teodoro G, et al. (2019) Liver steatosis segmentation with deep learning methods. 2019 IEEE 16th International Symposium on Biomedical Imaging (ISBI 2019) . https://doi.org/10.1109/ISBI.2019.8759600 |
[24] |
Li Q, Dhyani M, Grajo JR, et al. (2018) Current status of imaging in nonalcoholic fatty liver disease. World J Hepatol 10: 530-542. https://doi.org/10.4254/wjh.v10.i8.530 ![]() |
[25] |
Zhou JH, Cai JJ, She ZG, et al. (2019) Noninvasive evaluation of nonalcoholic fatty liver disease: Current evidence and practice. World J Gastroentero 25: 1307-1326. https://doi.org/10.3748/wjg.v25.i11.1307 ![]() |
[26] |
Podder S, Bhattacharjee S, Roy A (2021) An efficient method of detection of COVID-19 using mask R-CNN on chest X-Ray images. AIMS Biophys 8: 281-290. https://doi.org/10.3934/biophy.2021022 ![]() |
[27] |
Ünver HM, Ayan E (2019) Skin lesion segmentation in dermoscopic images with combination of YOLO and GrabCut algorithm. Diagnostics 9: 72. https://doi.org/10.3390/diagnostics9030072 ![]() |
[28] |
Alam MM, Islam MT (2019) Machine learning approach of automatic identification and counting of blood cells. Healthc Technol Lett 6: 103-108. https://doi.org/10.1049/htl.2018.5098 ![]() |
[29] |
Elsalamony HA (2016) Healthy and unhealthy red blood cell detection in human blood smears using neural networks. Micron 83: 32-41. https://doi.org/10.1016/j.micron.2016.01.008 ![]() |
[30] |
Xia K, Yin H (2019) Liver detection algorithm based on an improved deep network ccombined wwith edge perception. IEEE Access 7: 175135-175142. https://doi.org/10.1109/ACCESS.2019.2953517 ![]() |
[31] | Liu W, Anguelov D, Erhan D, et al. (2016) SSD: Single shot multibox detector. Computer Vision – ECCV 2016: 21-37. https://doi.org/10.1007/978-3-319-46448-0_2 |
[32] |
Ren S, He K, Girshick R, et al. (2017) Faster r-cnn: Towards real-time object detection with region proposal networks. IEEE T Pattern Anal 39: 1137-1149. https://doi.org/10.1109/TPAMI.2016.2577031 ![]() |
[33] | Redmon J, Divvala S, Girshick R, et al. (2016) You only look once: Unified, real-time object detection. 2016 IEEE Conference on Computer Vision and Pattern Recognition (CVPR) . https://doi.org/10.1109/CVPR.2016.91 |
[34] |
Shotton J, Kohli P (2014) Semantic image segmentation. Computer Vision : 713-716. https://doi.org/10.1007/978-0-387-31439-6_251 ![]() |
[35] |
Tsai H-F, Podder S, Chen P-Y (2023) Microsystem advances through integration with artificial intelligence. Micromachines 14: 826. https://doi.org/10.3390/mi14040826 ![]() |
[36] |
Ronneberger O, Fischer P, Brox T (2015) U-Net: Convolutional networks for biomedical image segmentation. Lecture Notes in Computer Science : 234-241. https://doi.org/10.1007/978-3-319-24574-4_28 ![]() |
[37] | He K, Gkioxari G, Dollar P, et al. (2017) Mask R-CNN. 2017 IEEE International Conference on Computer Vision (ICCV) . https://doi.org/10.1109/ICCV.2017.322 |
[38] |
Antonello M, Chiesurin S, Ghidoni S (2020) Enhancing semantic segmentation with detection priors and iterated graph cuts for robotics. Eng Appl Artif Intel 90: 103467. https://doi.org/10.1016/j.engappai.2019.103467 ![]() |
[39] |
Heinemann F, Birk G, Stierstorfer B (2019) Deep learning enables pathologist-like scoring of NASH models. Sci Rep 9: 18454. https://doi.org/10.1038/s41598-019-54904 ![]() |
[40] |
Heinemann F, Gross P, Zeveleva S, et al. (2022) Deep learning-based quantification of NAFLD/NASH progression in human liver biopsies. Sci Rep 12: 19236. https://doi.org/10.1038/s41598-022-23905-3 ![]() |
[41] | Kůrková V, Manolopoulos Y, Hammer B, et al. (2018) Lecture notes in computer science. Artificial Neural Networks and Machine Learning – ICANN 2018 . https://doi.org/10.1007/978-3-030-01421-6 |
[42] |
Amjoud AB, Amrouch M (2023) Object detection using deep learning, CNNs and vision transformers: a review. IEEE Access 11: 35479-35516. https://doi.org/10.1109/ACCESS.2023.3266093 ![]() |
[43] |
Han X, Zhong Y, Zhang L (2017) An efficient and robust integrated geospatial object detection framework for high spatial resolution remote sensing imagery. Rem Sens 9: 666. https://doi.org/10.3390/rs9070666 ![]() |
[44] |
Alam MM, Islam MT (2019) Machine learning approach of automatic identification and counting of blood cells. Healthc Technol Lett 6: 103-108. https://doi.org/10.1049/htl.2018.5098 ![]() |
[45] |
Pang S, Ding T, Qiao S, et al. (2019) A novel YOLOv3-arch model for identifying cholelithiasis and classifying gallstones on CT images. PLos One 14: e0217647. https://doi.org/10.1371/journal.pone.0217647 ![]() |
[46] | Eldho A, Francis T, Hari CV YOLO based Logo detection, 2019 9th International Conference on Advances in Computing and Communication (ICACC) (2019). https://doi.org/10.1109/ICACC48162.2019.8986207 |
[47] |
Saponara S, Elhanashi A, Gagliardi A (2021) Implementing a real-time, AI-based, people detection and social distancing measuring system for Covid-19. J Real-Time Image Pr 18: 1937-1947. https//doi.org/10.1007/s11554-021-01070-6 ![]() |
[48] | Hussain S, Mubeen I, Ullah N, et al. (2022) Modern diagnostic imaging technique applications and risk factors in the medical field: a review. BioMed Res Int 2022: 1-19. https://doi.org/10.1155/2022/5164970 |
[49] |
Amin J, Anjum MA, Sharif M, et al. (2022) Liver tumor localization based on YOLOv3 and 3D-semantic segmentation using deep neural networks. Diagnostics 12: 823. https://doi.org/10.3390/diagnostics12040823 ![]() |
[50] |
Kleiner DE, Makhlouf HR (2016) Histology of nonalcoholic fatty liver disease and nonalcoholic steatohepatitis in adults and children. Clin Liver Dis 20: 293-312. https://doi.org/10.1016/j.cld.2015.10.011 ![]() |
[51] |
Brunt EM, Kleiner DE, Wilson LA, et al. (2011) Nonalcoholic fatty liver disease (NAFLD) activity score and the histopathologic diagnosis in NAFLD: distinct clinicopathologic meanings. Hepatology 53: 810-820. https://doi.org/10.1002/hep.24127 ![]() |
[52] |
Chalasani N, Wilson L, Kleiner DE, et al. (2008) Relationship of steatosis grade and zonal location to histological features of steatohepatitis in adult patients with non-alcoholic fatty liver disease. J Hepatol 48: 829-834. https://doi.org/10.1016/j.jhep.2008.01.016 ![]() |
[53] |
Chalasani NP, Sanyal AJ, Kowdley KV, et al. (2009) Pioglitazone versus vitamin E versus placebo for the treatment of non-diabetic patients with non-alcoholic steatohepatitis: PIVENS trial design. Contemp Clin Trials 30: 88-96. https://doi.org/10.1016/j.cct.2008.09.003 ![]() |
[54] |
Angulo P, Kleiner DE, Dam-Larsen S, et al. (2015) Liver fibrosis, but no other histologic features, is associated with long-term outcomes of patients with nonalcoholic fatty liver disease. Gastroenterology 149: 389-397. https://doi.org/10.1053/j.gastro.2015.04.043 ![]() |
[55] |
Takahashi Y, Dungubat E, Kusano H, et al. (2023) Artificial intelligence and deep learning: New tools for histopathological diagnosis of nonalcoholic fatty liver disease/nonalcoholic steatohepatitis. Comput Struct Biotec 21: 2495-2501. https://doi.org/10.1016/j.csbj.2023.03.048 ![]() |
[56] |
Zambrano-Huailla R, Guedes L, Stefano JT, et al. (2020) Diagnostic performance of three non-invasive fibrosis scores (Hepamet, FIB-4, NAFLD fibrosis score) in NAFLD patients from a mixed Latin American population. Ann Hepatol 19: 622-626. https://doi.org/10.1016/j.aohep.2020.08.066 ![]() |
[57] |
Brown GT, Kleiner DE (2016) Histopathology of nonalcoholic fatty liver disease and nonalcoholic steatohepatitis. Metabolism 65: 1080-1086. https://doi.org/10.1016/j.metabol.2015.11.008 ![]() |
[58] |
Kleiner DE, Brunt EM, Van Natta M, et al. (2005) Design and validation of a histological scoring system for nonalcoholic fatty liver disease. Hepatology 41: 1313-1321. https://doi.org/10.1002/hep.20701 ![]() |
[59] |
Chen JR, Chao YP, Tsai YW, et al. (2020) Clinical value of information entropy compared with deep learning for ultrasound grading of hepatic steatosis. Entropy 22: 1006. https://doi.org/10.3390/e22091006 ![]() |
[60] |
Rantakokko P, Männistö V, Airaksinen R, et al. (2015) Persistent organic pollutants and non-alcoholic fatty liver disease in morbidly obese patients: a cohort study. Environ Health 14: 79. https://doi.org/10.1186/s12940-015-0066-z ![]() |
[61] |
Arjmand A, Angelis CT, Christou V, et al. (2019) Training of deep convolutional neural networks to identify critical liver alterations in histopathology image samples. Appl Sci 10: 42. https://doi.org/10.3390/app10010042 ![]() |
[62] |
Adegun A, Viriri S (2020) Deep learning techniques for skin lesion analysis and melanoma cancer detection: a survey of state-of-the-art. Artif Intell Rev 54: 811-841. https://doi.org/10.1007/s10462-020-09865-y ![]() |
[63] |
Ugail H, Abubakar A, Elmahmudi A, et al. (2022) The use of pre-trained deep learning models for the photographic assessment of donor livers for transplantation. Artif Intell Surg 2: 101-119. https://doi.org/10.20517/ais.2022.06 ![]() |
[64] |
Gaber A, Youness HA, Hamdy A, et al. (2022) Automatic classification of fatty liver disease based on supervised learning and genetic algorithm. Appl Sci 12: 521. https://doi.org/10.3390/app12010521 ![]() |
[65] |
Wu CC, Yeh WC, Hsu WD, et al. (2019) Prediction of fatty liver disease using machine learning algorithms. Comput Meth Prog Bio 170: 23-29. https://doi.org/10.1016/j.cmpb.2018.12.032 ![]() |
[66] | Yang R, Zhou Y, Liu W, et al. (2022) Study on the grading model of hepatic steatosis based on improved densenet. J Healthc Eng 2022: 1-8. https://doi.org/10.1155/2022/9601470 |
[67] |
Feng B, Ma XH, Wang S, et al. (2021) Application of artificial intelligence in preoperative imaging of hepatocellular carcinoma: Current status and future perspectives. World J Gastroentero 27: 5341-5350. https://doi.org/10.3748/wjg.v27.i32.5341 ![]() |
[68] |
Kistler KD, Brunt EM, Clark JM, et al. (2011) Physical activity recommendations, exercise intensity, and histological severity of nonalcoholic fatty liver disease. Am J Gastroenterol 106: 460-468. https://doi.org/10.1038/ajg.2010.488 ![]() |
1. | R. Saranya, R. Jaichandran, A dense kernel point convolutional neural network for chronic liver disease classification with hybrid chaotic slime mould and giant trevally optimizer, 2025, 102, 17468094, 107219, 10.1016/j.bspc.2024.107219 |
Method | TP | FP | TN | FN | AC (%) | SP (%) | PR (%) | RC (%) | F1 (%) | F2 (%) |
Faster R-CNN | 710 | 21 | 69 | 0 | 97.375 | 76.67 | 97.127 | 100 | 98.543 | 99.411 |
SSD | 653 | 10 | 134 | 3 | 98.375 | 93.055 | 98.491 | 99.543 | 99.014 | 99.33 |
YOLO v3 | 728 | 17 | 55 | 0 | 97.875 | 76.39 | 97.718 | 100 | 98.846 | 99.535 |
YOLO v4 | 731 | 11 | 57 | 1 | 98.5 | 83.823 | 98.517 | 99.863 | 99.185 | 99.591 |
YOLO v5 | 793 | 1 | 6 | 0 | 99.875 | 85.714 | 99.874 | 100 | 99.937 | 99.975 |
YOLO v6 | 744 | 12 | 42 | 2 | 98.25 | 77.78 | 98.413 | 99.732 | 99.068 | 99.465 |
YOLO v7 | 746 | 11 | 38 | 5 | 98 | 77.551 | 98.547 | 99.334 | 98.939 | 99.176 |
YOLO v8 | 795 | 1 | 4 | 0 | 99.875 | 80 | 99.874 | 100 | 99.937 | 99.975 |
Method | TP | FP | TN | FN | AC (%) | SP (%) | PR (%) | RC (%) | F1 (%) | F2 (%) |
Mask R-CNN | 690 | 19 | 86 | 5 | 97 | 81.905 | 97.32 | 99.281 | 98.291 | 98.883 |
U-Net | 623 | 25 | 144 | 8 | 95.875 | 85.207 | 96.142 | 99.732 | 97.904 | 98.993 |
YOLO v5 | 694 | 16 | 39 | 51 | 91.625 | 70.909 | 97.746 | 93.154 | 95.395 | 94.038 |
YOLO v7 | 578 | 37 | 48 | 137 | 78.25 | 56.471 | 93.984 | 80.839 | 86.917 | 83.165 |
YOLO v8 | 710 | 24 | 32 | 34 | 92.75 | 57.143 | 96.73 | 95.43 | 96.076 | 95.687 |
Model | mAP (%) | IoU |
Faster R-CNN | 49.2 | 0.971 |
SSD | 53.1 | 0.98 |
YOLOv3 | 54.3 | 0.977 |
YOLOv4 | 65.6 | 0.984 |
YOLOv5 | 98.9 | 0.999 |
YOLOv6 | 56.4 | 0.982 |
YOLOv7 | 59.6 | 0.979 |
YOLOv8 | 99.1 | 0.999 |
Model used | Reference | AC (%) | SP (%) | PR (%) | RC (%) | F1 (%) |
YOLOv5 | Our model | 99.875 | 85.714 | 99.874 | 100 | 99.937 |
Steatosis detection using eccentricity and roundness | [3] Tsiplikadou et al. | 97.78 | - | 100 | 97.78 | - |
Transfer learning to extract CNN-based features | [2] Byra et al. | 90.9 | 94.1 | - | 89.5 | - |
Mask R-CNN ResNet50 | [23] Guo et al. | - | - | 75.87 | 60.66 | 65.88 |
Supervised machine learning model | [7] Sethunath et al. | 84.26 | - | 95.01 | 94.23 | - |
Improved DenseNet | [66] Yang et al. | 88.49 | 81.6 | - | 95.44 | - |
Deep learning using classification network | [10] Cao et al. | 95.45 | - | - | - | - |
Curvelet Transform and Entropy feature extraction | [11] Acharya et al. | 97.33 | 100 | - | 96 | - |
ROI select and SVM classifier | [8] Owjimehr et al. | 97.9 | - | 92.7 | 100 | - |
CNN SGDM model | [61] Arjmand et al. | 95 | 98.3 | 95 | 95 | 95 |
Trained CNN | [39] Heinemann et al. | 90.63 | - | - | - | - |
ImageNet model with SVM classifier | [63] Ugail et al. | 99.63 | - | - | - | - |
CAD model with voting-based classifier | [64] Gaber et al. | 95.71 | 94.44 | 94.28 | 97.05 | 95.64 |
Random forest model | [65] Wu et al. | 86.48 | 85.89 | - | 87.16 | - |
Histology type | Definition | Scores of liver | Number of images |
Low- to medium-power evaluation of parenchymal involvement by steatosis | |||
Steatosis Grade | <5% | 0 | 145 |
5%-33% | 1 | 35 | |
>33%-66% | 2 | 72 | |
>66% | 3 | 6 | |
None | 0 | 129 | |
Perisinusoidal or periportal | 1 | 31 | |
Fibrosis Stage | Perisinusoidal and portal/periportal | 2 | 31 |
Bridging fibrosis | 3 | 52 | |
Cirrhosis | 4 | 15 | |
Lobular inflammation | No foci | 0 | 70 |
<2 foci per 200 × field | 1 | 51 | |
2-4 foci per 200 × field | 2 | 89 | |
>4 foci per 200 × field | 3 | 48 | |
Ballooning | None | 0 | 172 |
Few balloon cells | 1 | 30 | |
Many cells/prominent ballooning | 2 | 56 |
Item | Wilcoxon W | Kruskal-Wallis H | Z-score |
Steatosis score | 35 | 76.626 | -0.213 |
Ballooning score | 21 | 71.739 | -3.028 |
Inflammation score | 29 | 121.546 | -0.202 |
Fibrosis score | 33 | 86.237 | -0.601 |
Method | TP | FP | TN | FN | AC (%) | SP (%) | PR (%) | RC (%) | F1 (%) | F2 (%) |
Faster R-CNN | 710 | 21 | 69 | 0 | 97.375 | 76.67 | 97.127 | 100 | 98.543 | 99.411 |
SSD | 653 | 10 | 134 | 3 | 98.375 | 93.055 | 98.491 | 99.543 | 99.014 | 99.33 |
YOLO v3 | 728 | 17 | 55 | 0 | 97.875 | 76.39 | 97.718 | 100 | 98.846 | 99.535 |
YOLO v4 | 731 | 11 | 57 | 1 | 98.5 | 83.823 | 98.517 | 99.863 | 99.185 | 99.591 |
YOLO v5 | 793 | 1 | 6 | 0 | 99.875 | 85.714 | 99.874 | 100 | 99.937 | 99.975 |
YOLO v6 | 744 | 12 | 42 | 2 | 98.25 | 77.78 | 98.413 | 99.732 | 99.068 | 99.465 |
YOLO v7 | 746 | 11 | 38 | 5 | 98 | 77.551 | 98.547 | 99.334 | 98.939 | 99.176 |
YOLO v8 | 795 | 1 | 4 | 0 | 99.875 | 80 | 99.874 | 100 | 99.937 | 99.975 |
Method | TP | FP | TN | FN | AC (%) | SP (%) | PR (%) | RC (%) | F1 (%) | F2 (%) |
Mask R-CNN | 690 | 19 | 86 | 5 | 97 | 81.905 | 97.32 | 99.281 | 98.291 | 98.883 |
U-Net | 623 | 25 | 144 | 8 | 95.875 | 85.207 | 96.142 | 99.732 | 97.904 | 98.993 |
YOLO v5 | 694 | 16 | 39 | 51 | 91.625 | 70.909 | 97.746 | 93.154 | 95.395 | 94.038 |
YOLO v7 | 578 | 37 | 48 | 137 | 78.25 | 56.471 | 93.984 | 80.839 | 86.917 | 83.165 |
YOLO v8 | 710 | 24 | 32 | 34 | 92.75 | 57.143 | 96.73 | 95.43 | 96.076 | 95.687 |
Model | mAP (%) | IoU |
Faster R-CNN | 49.2 | 0.971 |
SSD | 53.1 | 0.98 |
YOLOv3 | 54.3 | 0.977 |
YOLOv4 | 65.6 | 0.984 |
YOLOv5 | 98.9 | 0.999 |
YOLOv6 | 56.4 | 0.982 |
YOLOv7 | 59.6 | 0.979 |
YOLOv8 | 99.1 | 0.999 |
Model used | Reference | AC (%) | SP (%) | PR (%) | RC (%) | F1 (%) |
YOLOv5 | Our model | 99.875 | 85.714 | 99.874 | 100 | 99.937 |
Steatosis detection using eccentricity and roundness | [3] Tsiplikadou et al. | 97.78 | - | 100 | 97.78 | - |
Transfer learning to extract CNN-based features | [2] Byra et al. | 90.9 | 94.1 | - | 89.5 | - |
Mask R-CNN ResNet50 | [23] Guo et al. | - | - | 75.87 | 60.66 | 65.88 |
Supervised machine learning model | [7] Sethunath et al. | 84.26 | - | 95.01 | 94.23 | - |
Improved DenseNet | [66] Yang et al. | 88.49 | 81.6 | - | 95.44 | - |
Deep learning using classification network | [10] Cao et al. | 95.45 | - | - | - | - |
Curvelet Transform and Entropy feature extraction | [11] Acharya et al. | 97.33 | 100 | - | 96 | - |
ROI select and SVM classifier | [8] Owjimehr et al. | 97.9 | - | 92.7 | 100 | - |
CNN SGDM model | [61] Arjmand et al. | 95 | 98.3 | 95 | 95 | 95 |
Trained CNN | [39] Heinemann et al. | 90.63 | - | - | - | - |
ImageNet model with SVM classifier | [63] Ugail et al. | 99.63 | - | - | - | - |
CAD model with voting-based classifier | [64] Gaber et al. | 95.71 | 94.44 | 94.28 | 97.05 | 95.64 |
Random forest model | [65] Wu et al. | 86.48 | 85.89 | - | 87.16 | - |
Histology type | Definition | Scores of liver | Number of images |
Low- to medium-power evaluation of parenchymal involvement by steatosis | |||
Steatosis Grade | <5% | 0 | 145 |
5%-33% | 1 | 35 | |
>33%-66% | 2 | 72 | |
>66% | 3 | 6 | |
None | 0 | 129 | |
Perisinusoidal or periportal | 1 | 31 | |
Fibrosis Stage | Perisinusoidal and portal/periportal | 2 | 31 |
Bridging fibrosis | 3 | 52 | |
Cirrhosis | 4 | 15 | |
Lobular inflammation | No foci | 0 | 70 |
<2 foci per 200 × field | 1 | 51 | |
2-4 foci per 200 × field | 2 | 89 | |
>4 foci per 200 × field | 3 | 48 | |
Ballooning | None | 0 | 172 |
Few balloon cells | 1 | 30 | |
Many cells/prominent ballooning | 2 | 56 |
Item | Wilcoxon W | Kruskal-Wallis H | Z-score |
Steatosis score | 35 | 76.626 | -0.213 |
Ballooning score | 21 | 71.739 | -3.028 |
Inflammation score | 29 | 121.546 | -0.202 |
Fibrosis score | 33 | 86.237 | -0.601 |