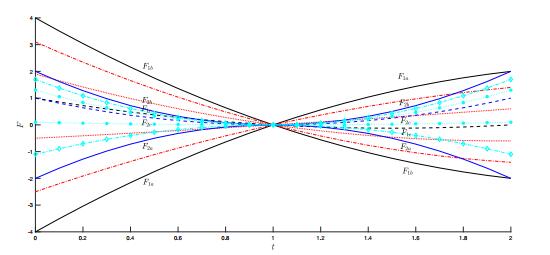
Obesity is a complex and multifactorial disease marker, which has become a major threat to cardiovascular health. We sought to assess the correlation of obesity and other cardio-metabolic risk factors in patients seen at the outpatient specialist clinic in Ghana.
A prospective cross-sectional study was conducted on 395 patients at Precise Specialist Clinic in Kumasi, Ghana. A standardized questionnaire was used to obtain demographic, anthropometric and clinical data of patients. Fisher's exact test for statistical significance at a 95% confidence interval was used to evaluate associations between categorical variables. The associations between obesity indices and cardiovascular disease risk factors were analyzed by Pearson's correlation.
Of the 395 participants, 187 were males and 208 were females. The mean (± standard deviation) age of study participants was 59.29 (± 13.93); more than half of the participants were between 50 and 69 years. The mean BMI of male participants was significantly lower than the mean BMI of female participants (28.18 kg/m2 vs 31.16 kg/m2, P-value < 0.0001). Gender was significantly associated with the weight categories (P = 0.0144). Obesity was seen more in females (49.0%) than in males (35.8%). The Pearson correlation analysis also showed a significant positive correlation between obesity, increasing systolic blood pressure (r = 0.1568, P-value = 0.0018) and increasing diastolic blood pressure (r = 0.2570, P-value < 0.0001).
Obesity was found to be significantly associated with female gender, increasing age, increasing systolic blood pressure, and increasing diastolic blood pressure. Efforts to step-up preventive measures to reduce the increasing prevalence of obesity in Ghana are highly recommended.
Citation: Isaac Kofi Owusu, Emmanuel Acheamfour-Akowuah, Lois Amoah-Kumi, Yaw Amo Wiafe, Stephen Opoku, Enoch Odame Anto. The correlation between obesity and other cardiovascular disease risk factors among adult patients attending a specialist clinic in Kumasi. Ghana[J]. AIMS Medical Science, 2023, 10(1): 24-36. doi: 10.3934/medsci.2023003
[1] | Jiani Jin, Haokun Qi, Bing Liu . Hopf bifurcation induced by fear: A Leslie-Gower reaction-diffusion predator-prey model. Electronic Research Archive, 2024, 32(12): 6503-6534. doi: 10.3934/era.2024304 |
[2] | Maurıicio F. S. Lima, Jaume Llibre . Hopf bifurcation for a class of predator-prey system with small immigration. Electronic Research Archive, 2024, 32(7): 4604-4613. doi: 10.3934/era.2024209 |
[3] | Jialu Tian, Ping Liu . Global dynamics of a modified Leslie-Gower predator-prey model with Beddington-DeAngelis functional response and prey-taxis. Electronic Research Archive, 2022, 30(3): 929-942. doi: 10.3934/era.2022048 |
[4] | Ruizhi Yang, Dan Jin . Dynamics in a predator-prey model with memory effect in predator and fear effect in prey. Electronic Research Archive, 2022, 30(4): 1322-1339. doi: 10.3934/era.2022069 |
[5] | Yujia Xiang, Yuqi Jiao, Xin Wang, Ruizhi Yang . Dynamics of a delayed diffusive predator-prey model with Allee effect and nonlocal competition in prey and hunting cooperation in predator. Electronic Research Archive, 2023, 31(4): 2120-2138. doi: 10.3934/era.2023109 |
[6] | Yuan Tian, Hua Guo, Wenyu Shen, Xinrui Yan, Jie Zheng, Kaibiao Sun . Dynamic analysis and validation of a prey-predator model based on fish harvesting and discontinuous prey refuge effect in uncertain environments. Electronic Research Archive, 2025, 33(2): 973-994. doi: 10.3934/era.2025044 |
[7] | Xianyi Li, Xingming Shao . Flip bifurcation and Neimark-Sacker bifurcation in a discrete predator-prey model with Michaelis-Menten functional response. Electronic Research Archive, 2023, 31(1): 37-57. doi: 10.3934/era.2023003 |
[8] | Yuan Tian, Yang Liu, Kaibiao Sun . Complex dynamics of a predator-prey fishery model: The impact of the Allee effect and bilateral intervention. Electronic Research Archive, 2024, 32(11): 6379-6404. doi: 10.3934/era.2024297 |
[9] | Xuemin Fan, Wenjie Zhang, Lu Xu . Global dynamics of a predator-prey model with prey-taxis and hunting cooperation. Electronic Research Archive, 2025, 33(3): 1610-1632. doi: 10.3934/era.2025076 |
[10] | Yichao Shao, Hengguo Yu, Chenglei Jin, Jingzhe Fang, Min Zhao . Dynamics analysis of a predator-prey model with Allee effect and harvesting effort. Electronic Research Archive, 2024, 32(10): 5682-5716. doi: 10.3934/era.2024263 |
Obesity is a complex and multifactorial disease marker, which has become a major threat to cardiovascular health. We sought to assess the correlation of obesity and other cardio-metabolic risk factors in patients seen at the outpatient specialist clinic in Ghana.
A prospective cross-sectional study was conducted on 395 patients at Precise Specialist Clinic in Kumasi, Ghana. A standardized questionnaire was used to obtain demographic, anthropometric and clinical data of patients. Fisher's exact test for statistical significance at a 95% confidence interval was used to evaluate associations between categorical variables. The associations between obesity indices and cardiovascular disease risk factors were analyzed by Pearson's correlation.
Of the 395 participants, 187 were males and 208 were females. The mean (± standard deviation) age of study participants was 59.29 (± 13.93); more than half of the participants were between 50 and 69 years. The mean BMI of male participants was significantly lower than the mean BMI of female participants (28.18 kg/m2 vs 31.16 kg/m2, P-value < 0.0001). Gender was significantly associated with the weight categories (P = 0.0144). Obesity was seen more in females (49.0%) than in males (35.8%). The Pearson correlation analysis also showed a significant positive correlation between obesity, increasing systolic blood pressure (r = 0.1568, P-value = 0.0018) and increasing diastolic blood pressure (r = 0.2570, P-value < 0.0001).
Obesity was found to be significantly associated with female gender, increasing age, increasing systolic blood pressure, and increasing diastolic blood pressure. Efforts to step-up preventive measures to reduce the increasing prevalence of obesity in Ghana are highly recommended.
Abbreviations: FFIDDCs: fuzzy fractional implicit delay differential coupled systems; TFFs: triangular fuzzy functions; MDs: membership degrees; IVP: initial value problem; FDDEs: fuzzy delay differential equations; FDEs: fractional differential equations; DFDEs: fractional differential equations with time delay; FFDEs: fuzzy fractional differential equations; FCFDIVP: fuzzy coupled fractional initial value problem with delays; MF: membership function; TFN: triangular fuzzy number
In an ecosystem, there exist universally competitions between two species, which is very important. And they tend to interact with each other so that there are some relationships such as predation or symbiosis among them (i.e. coupled systems) (see [9,39]). In addition, the delay and ambiguity may appear because of different reasons such as physical properties of equipment used in the system, signal transmission or measurement of system variables (see [14]), so we need to take them into account. Thus, in this paper, motivated by the work of Dong et al. [13] and Fatullayev et al. [14], we come up with the following problem of fuzzy fractional implicit delay differential coupled systems (FFIDDCs):
{cDαx(t)=m1(t)y(t−τ)+~F1(t,y(t),cDαx(t)),t>0,cDβy(t)=m2(t)x(t−τ)+~F2(t,x(t),cDβy(t)),t>0,x(t)=~Φ1(t),−τ≤t≤0,y(t)=~Φ2(t),−τ≤t≤0, | (1.1) |
and explore existence and uniqueness result of solution for the FFIDDC (1.1), where cDαf(t) denotes α-order Caputo fractional derivative of function f(t)∈C1α for 0<α<1 (see [22]), τ is the value of time delay, ~Φ1(t) and ~Φ2(t) are triangular fuzzy functions (TFFs) defined on [−τ,0], ~F1(t,y(t),cDαx(t)) and ~F2(t,x(t),cDβy(t)) are also TFFs defined on (0,∞)×R×R, and m1(t) and m2(t) are continuous crisp functions. The concept of TFFs used in this paper was first proposed by Gasilov et al. [17], then it has been widely employed [7,16,27]. Unlike in the past, Gasilov et al. [17] thought that a fuzzy function is a fuzzy bunch of real functions rather than a fuzzy number-valued function. Each of these real functions has a certain membership degree. The new concept of TFFs broadened the way (see [14]), and so we deal with fuzzy functions and fuzzy problems.
Remark 1.1. In this paper, we consider the problem with Caputo fractional derivative, which is based on Riemann-Liouville fractional derivative [22]. We note that for temporal variable, the Caputo fractional derivative has been widely recognized in real application. For example, the Caputo fractional derivative of a constant is 0, but in the case of finite number at the lower limit of the interval, the Riemann-Liouville fractional derivative of a constant is not equal to 0. And the initial conditions given by Riemann-Liouville method cannot be explained physically. For more related work, see [28,34]. However, can one discuss the existence and uniqueness results for the solutions of the FFIDDC (1.1) with Riemann-Liouville fractional derivative or Hadamard fractional derivative? These are usually presented as important and significative problems in future research.
Some special cases of the FFIDDC (1.1) are listed as follows:
(i) If τ=0,0≤t≤1 and αj=1, where αj is the membership degrees (MDs) of ~Fj for j=1,2, the FFIDDC (1.1) can be rewritten as the problem investigated by Dong et al. [13]:
{cDαx(t)=f(t,y(t),cDαx(t)),0≤t≤1,cDβy(t)=g(t,x(t),cDβy(t)), 0≤t≤1,x(0)=x(0),y(0)=y(0). |
Making full use of fixed point theorem and the vector form of Gronwall inequality, Dong et al. [13] gained existence and uniqueness of solution to the initial value problem (IVP), and discussed the estimation of the solution.
(ii) If α=β=1, the FFIDDC (1.1) reduces to the following fuzzy implicit delay differential coupled systems:
{x′(t)=m1(t)y(t−τ)+~F1(t,y(t),x′(t)),t>0,y′(t)=m2(t)x(t−τ)+~F2(t,x(t),y′(t)),t>0,x(t)=~Φ1(t),−τ≤t≤0,y(t)=~Φ2(t),−τ≤t≤0, | (1.2) |
which is brand new and is worth studying in the future.
We note that the system (1.2) is an extension of the linear inhomogeneous fuzzy delay differential equations (FDDEs) considered by Fatullayev et al. [14]:
{x′(t)=n(t)x(t)+m(t)x(t−τ)+˜F(t),t>0,x(t)=˜Φ(t),−τ≤t≤0. |
The authors presented a method to represent the solution of FDDE as a fuzzy set of real functions, and proved existence and uniqueness of solution for FDDE involving TFFs. Furthermore, Fatullayev et al. [14] showed clearly that the proposed method can be extended to the system of FDDEs by using the research results of Gasilov and Amrahov [15]. The purpose of this paper is to discuss existence and uniqueness of solution for the FFIDDC (1.1).
Comparing with the simpler integer-order differential equations, fractional differential equations (FDEs) have more wide applications and play an important role in engineering, physics, finance and other fields. Nowadays, FDEs have been used to more accurately describe the dynamics of many systems when several complex phenomena in numerous seemingly diverse and widespread fields of science and engineering is modeled and investigated. See, for example, [3,6,22,28,34] and references therein. In addition, to study ecosystem problems in biology, one often discovers that an ecosystem does not have only a single species. That is to say, there must be multiple species, so there exists competition among them and coupling is taken into account (see [9,39]). Coupling relationship refers to the interaction and mutual influence between two or more objects. In fact, the coupled systems of FDEs have been studied by multitudinous researchers. See, for example, [2,38] and the references cited therein. Recently, by applying standard fixed point theorems for multivalued maps, existence of solutions for coupled systems of fractional differential inclusions with coupled boundary conditions was obtained by Ahmad et al. [5].
In science and engineering and other practical applications, time delay usually occurs on account of manual measurement, signal transmission, aging of equipments and so on (see [30]). Thereby, in recent years, the scholars have been interested in solving the fractional differential equations with time delay (DFDEs) (see, for instance, [30,35] and the references therein). Brzdek and Eghbali [10] considered the Ulam's stability of DFDEs and also proved that under some appropriate assumptions, each approximate solution of DFDEs is close to its only exact solution. In order to acquire existence of solutions for a time dependent delay differential equation with constant delay, Tabassum et al. [33] extended the previous approaches about fuzzy weakly contraction mapping principle. Of course, there are not just one type of FDEs, whether they are linear or nonlinear. And the common ones are Caputo type, Riemann-Liouville type and Hadamard type (see [11,21,36]). Moreover, the delay is not necessarily finite, it can be also infinite. Some researchers dedicated themselves to the study of FDEs with infinite delay. Banach fixed point theorem and the nonlinear alternative of Leray-Schauder type initiated by Granas and Dugundji [18], had been utilized by Benchohra et al. [8] to investigate existence results of FDEs with infinite delay.
Certainly, in actual application, there are not only time delay, but also exists the possibility of ambiguity. Thus, one needs to take the fuzzy into account, and then the fuzzy fractional differential equations (FFDEs) are proposed. Agarwal et al. [1] put forward a notion of fuzzy Riemann-Liouville differentiability based on Hukuhara differentiability, which can be employed to solve the IVPs of FFDEs. After that, many investigators have extended the concepts and expression forms of FFDEs, and bent themselves to obtain existence and uniqueness of solution for FFDEs. These theories are also well applied in real life. See, for example, [24,25,29] and the references therein. As Fatullayev et al. [14] pointed out, "the types of fuzzy items are more important than their numbers when a fuzzy problem needs to be solved". In fact, we can easily deal with n-th order homogeneous linear differential equations with fuzzy initial values (i.e. the problem with n fuzzy inputs) by using Zadeh's extension principle. But if a fuzzy forcing function appears in the problem, even for the delay differential equation of first order (i.e. with 2 fuzzy inputs), it will be indistinct how to use the principle.
It is well know that explicit equations are special cases of implicit equations, and the implicit equations are more general (see [12]). Cubiotti and Yao [12] introduced and studied a class of implicit second-order ordinary differential equations with known endpoint values. They also proved existence results under two conditions. However, as Hoa and Vu [21] stated briefly, "to the best of our knowledge, there exists no literature devoted to the uncertain fractional implicit differential equation in the fuzzy setting with the concepts of Riemann-Liouville, Caputo, Hadamard fractional derivatives". And then, Hoa and Vu [21] proposed some existence results for solutions of the fuzzy fractional implicit differential equations associated with the fuzzy Caputo-type, fuzzy Riemann-Liouville-type and fuzzy Hadamard-type concepts of fractional derivative, respectively. Very recently, Son and Dong [31] proposed global existence and some properties of solutions for the nonlocal problem of implicit fuzzy fractional differential systems. For more work on various forms of implicit FDE models, one can refer to [26,19,20] and the references therein.
The major methods of this paper are as follows:
(i) We generalize TFF (Definition 2.1 of [17]) to a group of TFFs (see Example 2.1).
(ii) To solve the FFIDDC (1.1), we use the method of steps proposed by Fatullayev et al. [14]. Firstly, we figure out the solution of the FFIDDC (1.1) on interval [0,τ]. Afterwards, we similarly find the solution on interval [τ,2τ]. And as we go along, eventually, the solution on interval [0,+∞) can be obtained.
(iii) Because of the fuzzy coupled fractional initial value problem with delays (FCFDIVP) (3.1), which is the identical transformation of the FFIDDC (1.1), is linear, the superposition principle can be used to work out it. As a consequence, we can decompose the FCFDIVP (3.1) into three subproblems (3.4)-(3.6) and solve them separately.
The remainder of this paper is organized as follows. The necessary preliminaries about the fuzzy theory that we are going to use are listed in Section 2. In Section 3, we introduce the concept of solution for the FFIDDC (1.1) and describe how to get it. Ultimately, a solution algorithm is proposed. In Section 4, we present an example to show that the hypotheses in all theorems can be met. Finally, in Section 5, we summarize the research results of this paper, and provide the content that one can study in the future.
Based on the work of Zadeh [37], we define a fuzzy set ˜A as a pair of the universal set U and the membership function (MF) μ:U→[0,1]. The MF of a fuzzy set ˜A can be denoted as μ˜A. For each x∈U, the numerical value μ˜A(x) is called the MD of x in ˜A. The crisp set supp(˜A)={x∈U∣μ˜A(x)>0} is called the support of ˜A.
After that, let U be the set of real numbers R, and a,c and b be real numbers which meet a≤c≤b. Then the set ˜u with MF
μ(x)={x−ac−a, a<x<c,1,x=c,b−xb−c, c<x<b,0,otherwise |
is called a triangular fuzzy number (TFN) and we denote it as ˜u=(a,c,b). On the grounds of the geometric interpretation, the number c is called the vertex of ˜u, we denote u_=a and ¯u=b to represent the left and the right end-points of ˜u, respectively. Frequently, we express ˜u=(a,c,b) as ˜u=ucr+˜uun. Here, ucr=c is the crisp part and ˜uun=(a−c,0,b−c) is the uncertain part of ˜u.
It is also useful to represent the fuzzy sets though their α-cuts. For each α∈(0,1], the crisp set Aα={x∈U∣μ˜A(x)≥α} is called the α-cut of ˜A. For α=0, the A0=closure(supp(˜A)).
For the TFN ˜u=(a,c,b), the α-cuts are intervals uα=[uα_,¯uα], where uα_=a+α(c−a) and ¯uα=b+α(c−b). These formulas can be rewritten as uα_=c+(1−α)(a−c) and ¯uα=c+(1−α)(b−c). Therefore, uα=[uα_,¯uα]=c+(1−α)[a−c,b−c]. From here we can see that an α-cut is homothetic to [a,b] (which is the 0-cut) with center c and with ratio (1−α).
There are different notions about the fuzzy functions. In this study, we use the concept of the fuzzy function which was brought forward by Gasilov et al. [17], namely, fuzzy function is a bunch of fuzzy real functions. As a value ˜F(t) of a fuzzy bunch ˜F at time t, we understand the fuzzy set, which elements are the values of the real functions at t, with the higher MD of the corresponding functions. Mathematically,
μ˜F(t)(x)=α ⇔ ∃y(⋅):(μ˜F(y)=α∧y(t)=x)∧∀z(⋅):(μ˜F(z)>α→z(t)≠x), |
where "∧" and "→" are the logical conjunction and implication symbols, respectively.
Definition 2.1. ([17]) Let U be set of continuous functions defined on an interval I, and Fa(⋅),Fc(⋅),Fb(⋅)∈U. We call the fuzzy subset ˜F of U, determined by the MF as follows:
μ˜F(y(⋅))={α,y=Fa+α(Fc−Fa)and0<α≤1,α,y=Fb+α(Fc−Fb)and0<α≤1,0,otherwise, |
as TFF and denote it as ˜F=⟨Fa,Fc,Fb⟩.
According to this definition, a TFF is a fuzzy set (or, fuzzy bunch) of real functions. Among them only two functions have the MD α: the functions y1=Fa+α(Fc−Fa) and y2=Fb+α(Fc−Fb).
Referring to the example of TFF given by Gasilov et al. [16], we give the following example of a group of TFFs.
Example 2.1. In Figure 1, we depict a group of TFFs as
{~F1=⟨F1a,F1c,F1b⟩~F2=⟨F2a,F2c,F2b⟩ |
where
F1a(t)=−t2+5t−4 (MD is 0, the black curve that is at bottom on [0,1] and at upper on [1,2]);
F1c(t)=0.5t2−1.5t+1 (MD is 1, the black dashed line);
F1b(t)=t2−5t+4 (MD is 0, the black curve that is at upper on [0,1] and at bottom on [1,2]);
F2a(t)=−2t2+4t−2 (MD is 0, the blue curve that is at bottom on [0,2]);
F2c(t)=t2−2t+1 (MD is 1, the blue dashed line);
F2b(t)=2t2−4t+2 (MD is 0, the blue curve that is at upper on [0,2]).
The TFF ~F1 with MDs 0.7 and 0.3 are depicted by red dotted and dashed-dotted lines, respectively. And the TFF ~F2 with MDs 0.7 and 0.3 are described by cyan dotted and dashed-dotted lines which are marked, respectively.
The value of a TFF at a time t∈I can be expressed by the following formula:
˜F(t)=(min{Fa(t),Fc(t),Fb(t)},Fc(t),max{Fa(t),Fc(t),Fb(t)}). |
We can easily find out that this value is a TFN, and a TFF ˜F=⟨Fa,Fb,Fc⟩ is not a fuzzy number-valued function. Actually, it is a fuzzy subset of the universe of continuous functions. Each element of this fuzzy subset is a real function with a certain MD.
If Fa(t)≤Fc(t)≤Fb(t) for all t∈I, then the TFF ˜F=⟨Fa,Fc,Fb⟩ is regular TFF on I, and we have ˜F(t)=(Fa(t),Fc(t),Fb(t)). Further, when a TFF ˜F is not regular, we call it as non-regular TFF. It means that for a non-regular TFF, in general, ˜F(t)≠(Fa(t),Fc(t),Fb(t)). And the graphs of functions Fa,Fc and Fb can interchanged as t goes. In Figure 1, the TFFs ~F1 and ~F2 are non-regular and regular on [0,2], respectively. Without loss of generality, no matter regular TFFs or non-regular TFFs, main results and the algorithm in this paper are all valid. In the sequel, we assume that TTFs are regular.
Now, we will consider the FFIDDC (1.1) in this section. With respect to the FFIDDC (1.1), we can solve it by using the method of steps introduced by Fatullayev et al. [14].
First of all, we deal with the FFIDDC (1.1) on interval [0,τ]. Because x(t−τ)=~Φ1(t−τ),y(t−τ)=~Φ2(t−τ) for t∈[0,τ], the FFIDDC (1.1) is equivalent to FCFDIVP as follows:
{cDαx(t)=~G1(t,y(t),cDαx(t)),cDβy(t)=~G2(t,x(t),cDβy(t)),x(0)=~Φ1(0),y(0)=~Φ2(0), | (3.1) |
where
~G1(t,y(t),cDαx(t))=m1(t)~Φ2(t−τ)+~F1(t,y(t),cDαx(t)),~G2(t,x(t),cDβy(t))=m2(t)~Φ1(t−τ)+~F2(t,x(t),cDβy(t)). |
Thus, we transform the FCFDIVP (3.1) in matrix form:
{D⋅Z(t)=˜G(⋅),Z(0)=˜Φ(0). | (3.2) |
Here, Z(t)=(x(t)y(t)), D=(cDα00cDβ), ˜G(⋅)=(~G1(t,y(t),cDαx(t))~G2(t,x(t),cDβy(t))) and ˜Φ(0)=(~Φ1(0)~Φ2(0)).
Definition 3.1. For the problem (3.2), the fuzzy set ˜Z with MF
μ˜Z(Z(⋅))=min{μ˜Φ(ϕ(0)),μ˜G(D⋅Z(t))} | (3.3) |
is called to be a solution of the problem (3.2), which is also a solution to the FCFDIVP (3.1), where ϕ(0)=Z(0).
As for the formula (3.3), let Z(t) be a functional matrix. We determine ϕ(0)=Z(0) for t∈[0,τ] and calculate μ1≜μ˜Φ(ϕ(0)). After that, we compute g(⋅)=D⋅Z(t) on interval [0,τ] and determine μ2≜μ˜G(g(⋅)). Finally, we calculate the MD μ as μ=min{μ1,μ2}. We assign the number μ as the MD of Z(t). We define the set of all functional matrices such as Z(t) with their MDs μ as the fuzzy solution ˜Z.
According to Definition 3.1., the solution ˜Z is a fuzzy bunch of real functional matrices, which consists of functional matrices such as Z(t). If a functional matrix Z(t) satisfies
{D⋅Z(t)=g(⋅),Z(0)=ϕ(0) |
for some functional matrices g∈supp(˜G) and ϕ(0)∈supp(˜Φ(0)), then it has a positive MD.
Let us represent ~G1=g1cr+~g1 (crisp part + uncertainty), where g1cr=G1c and ~g1=⟨g1a,0,g1b⟩=⟨G1a−G1c,0,G1b−G1c⟩. Similarly, ~G2=g2cr+~g2, here g2cr=G2c and ~g2=⟨g2a,0,g2b⟩.
Assume that ~Φ1 and ~Φ2 are regular TFFs. Then ~Φ1(0) and ~Φ2(0) are TFNs. Further, ~Φ1(0)=ϕ1cr(0)+~ϕ1(0), here ϕ1cr(0)=Φ1c(0) and ~ϕ1(0)=(ϕ1a(0),0,ϕ1b(0))=(Φ1a(0)−Φ1c(0),0,Φ1b(0)−Φ1c(0)). In the same way, ~Φ2(0)=ϕ2cr(0)+~ϕ2(0), here ϕ2cr(0)=Φ2c(0) and ~ϕ2(0)=(ϕ2a(0),0,ϕ2b(0)).
Since the FCFDIVP (3.1) is linear, we can solve it using the superposition principle. This follows that in order to find the solution of the FCFDIVP (3.1), we can separately consider the following subproblems:
(1) The associated crisp problem:
{cDαx(t)=g1cr(t,y(t),cDαx(t)),cDβy(t)=g2cr(t,x(t),cDβy(t)),x(0)=ϕ1cr(0),y(0)=ϕ2cr(0). | (3.4) |
(2) The problem with initial TFFs:
{cDαx(t)=0,cDβy(t)=0,x(0)=~ϕ1(0),y(0)=~ϕ2(0). | (3.5) |
(3) The problem with fuzzy source functions and zero initial functions:
{cDαx(t)=~g1(t,y(t),cDαx(t)),cDβy(t)=~g2(t,x(t),cDβy(t)),x(0)=0,y(0)=0. | (3.6) |
By solving the above three subproblems (3.4)-(3.6), the solution of the FFIDDC (1.1) on interval [0,τ] can be obtained. Similarly, at the next step, we can find the solution on interval [τ,2τ]. Hence, we can conclude that the solution of the FFIDDC (1.1) exists for t∈[0,∞) using the method of steps [14].
In the sequel, we make clear how to solve each of these three subproblems (3.4)-(3.6).
To solve the problem (3.4), we provide the following theorem.
Theorem 3.1. For the problem (3.4), we can get a unique solution to it, which is denoted as Zcr(t)=(xcr(t)ycr(t)), when the following conditions fulfill:
(H1) g1cr,g2cr:[0,τ]×R×R→R are continuous.
(H2) There exist constants Ki,Li∈(0,1) for i=1,2 such that
0<max{K2+K1Γ(β+1),L2+L1Γ(α+1)}<1 |
and for each x1,x2,y1,y2∈R,
∣g1cr(t,x1,y1)−g1cr(t,x2,y2)∣≤K1∣x1−x2∣+K2∣y1−y2∣,∣g2cr(t,x1,y1)−g2cr(t,x2,y2)∣≤L1∣x1−x2∣+L2∣y1−y2∣. |
Proof. The proof is similar to Theorem 3.1 of Dong et al. [13] and it is omitted.
In this subsection, we will give solvability of the problem (3.5) as follows.
Theorem 3.2. Consider the problem (3.5), where ~ϕ1(0)=(ϕ1a(0),0,ϕ1b(0)),~ϕ2(0)=(ϕ2a(0),0,ϕ2b(0)). If Za(t)=(xa(t)ya(t)) and Zb(t)=(xb(t)yb(t)) are solutions of the problem
{cDαx(t)=0,cDβy(t)=0,x(0)=ϕ1(0),y(0)=ϕ2(0) | (3.7) |
for (ϕ1(0)ϕ2(0))=(ϕ1a(0)ϕ2a(0)) and (ϕ1(0)ϕ2(0))=(ϕ1b(0)ϕ2b(0)), respectively, then the problem (3.5) has a unique solution ~Zϕ, which is a TFF matrix given by
~Zϕ=⟨Za,0,Zb⟩. | (3.8) |
Proof. On the one hand, according to Definition 3.1., it is easy for us to know that each Z(t) with non-zero MD from the bunch ~Zϕ is a solution of the problem (3.7) for some (ϕ1(0)ϕ2(0)) from (~ϕ1(0)~ϕ2(0)).
On the other hand, in terms of Definition 2.1, the bunch ~ϕ1=⟨ϕ1a,0,ϕ1b⟩ consists of functions kϕ1a and kϕ1b ([0,1]∋k=1−α) owing to ϕ1c=0. And the bunch ~ϕ2=⟨ϕ2a,0,ϕ2b⟩ is similar to the former.
Moreover, if a Z(t) is a solution of the problem (3.7), then kZ(t) is a solution of the same equation with (kϕ1(0)kϕ2(0)) taken instead of (ϕ1(0)ϕ2(0)).
From the above reasoning we can get the conclusion that the bunch ~Zϕ consists of kZa and kZb. Therefore, the bunch ~Zϕ is a TFF matrix determined to be ~Zϕ=⟨Za,0,Zb⟩.
Actually, we can get the solutions Za(t)=(ϕ1a(0)ϕ2a(0)) and Zb(t)=(ϕ1b(0)ϕ2b(0)).
We can express the value of the TFF matrix ~Zϕ (3.8) at a time t by the formula ~Zϕ(t)=(min{Za(t),0,Zb(t)},0,max{Za(t),0,Zb(t)}). Note that this value is a matrix of TFNs.
As for the solution of the problem (3.6), we give the theorem as follows.
Theorem 3.3. In the problem (3.6), assume ~g1=⟨g1a,0,g1b⟩,~g2=⟨g2a,0,g2b⟩, where (g1ag2a) and (g1bg2b) separately satisfy the conditions (H1) and (H2) of Theorem 3.1. If Zu(t)=(xu(t)yu(t)) and Zv(t)=(xv(t)yv(t)) are solutions of the following problem:
{cDαx(t)=g1(t,y(t),cDαx(t)),cDβy(t)=g2(t,x(t),cDβy(t)),x(0)=0,y(0)=0 | (3.9) |
for (g1g2)=(g1ag2a) and (g1g2)=(g1bg2b) in several, then the problem (3.6) has a unique solution ~Zg, which is a TFF matrix given by
~Zg=⟨Zu,0,Zv⟩. | (3.10) |
Proof. Since g1a and g2a satisfy (H1) and (H2), it follows from Theorem 3.1 that there will be a unique solution when (g1g2)=(g1ag2a) in (3.9). By the same token, from the assumption for (g1g2)=(g1bg2b) in the problem (3.9), only one solution will exist in this case. Thus, the proof can be done by the same way as in Theorem 3.2.
Thus, we can also express the value of the TFF matrix ~Zg (3.10) at a time t by ~Zg(t)=(min{Zu(t),0,Zv(t)},0,max{Zu(t),0,Zv(t)}), which is a matrix of TFNs.
By integrating Theorems 3.1-3.3 above, we can obtain the following solution algorithm for solving the FFIDDC (1.1).
Algorithm 3.1. Step 1. Using the method of steps and dealing with the FFIDDC (1.1) on interval [0,τ], transform the FFIDDC (1.1) into the FCFDIVP (3.1).
Step 2. Represent the initial values and source functions as
~G1=g1cr+⟨g1a,0,g1b⟩,~G2=g2cr+⟨g2a,0,g2b⟩,~Φ1(0)=ϕ1cr(0)+(ϕ1a(0),0,ϕ1b(0)),~Φ2(0)=ϕ2cr(0)+(ϕ2a(0),0,ϕ2b(0)). |
Step 3. Find the solution Zcr(t) of the problem (3.4).
Step 4. Seek the solutions Za(t) and Zb(t) of the problem (3.7) in regard to (ϕ1(0)ϕ2(0))=(ϕ1a(0)ϕ2a(0)) and (ϕ1(0)ϕ2(0))=(ϕ1b(0)ϕ2b(0)), respectively, and define
~Zϕ(t)=(min{Za(t),0,Zb(t)},0,max{Za(t),0,Zb(t)}). |
Step 5. Solve the problem (3.9) and denote the solutions by Zu(t) and Zv(t), corresponding to (g1g2)=(g1ag2a) and (g1g2)=(g1bg2b), respectively, and let
~Zg(t)=(min{Zu(t),0,Zv(t)},0,max{Zu(t),0,Zv(t)}). |
Step 6. Construct the unique solution of the FFIDDC (1.1) on interval [0,τ] as follows:
˜Z(t)=Zcr(t)+~Zϕ(t)+~Zg(t). |
Similarly, we can find the unique solution on interval [τ,2τ], ⋯. In consequence, we obtain a unique solution of the FFIDDC (1.1) which exists at t≥0.
In this section, referring to Example 1 in Fatullayev et al. [14], we take an example to clarify that the hypotheses in Theorems 3.1-3.3 can be satisfied, which can be also employed to verify the main results presented in Dong et al. [13].
Example 4.1. Let us solve the problem
{cD12x(t)=costy(t−π2)+~F1(t,y(t),cD12x(t)),t>0,cD12y(t)=sintx(t−π2)+~F2(t,x(t),cD12y(t)),t>0,x(t)=~Φ1(t),−π2≤t≤0,y(t)=~Φ2(t),−π2≤t≤0, | (4.1) |
where
~F1=f1cr+⟨f1a,0,f1b⟩,~F2=f2cr+⟨f2a,0,f2b⟩,~Φ1=ϕ1cr+⟨ϕ1a,0,ϕ1b⟩,~Φ2=ϕ2cr+⟨ϕ2a,0,ϕ2b⟩ |
with
f1cr=−0.5siny(t)+10−3sincD12x(t),f2cr=0.7sinx(t)−10−3sincD12y(t),f1a=−0.15sintcost, f1b=0.15sintcost,f2a=0.15sintcost,f2b=−0.15sintcost,ϕ1cr=cost, ϕ1a=0.15sint−0.25cost, ϕ1b=−0.15sint+0.25cost,ϕ2cr=sint, ϕ2a=0.15cost−0.25sint, ϕ2b=−0.15cost+0.25sint. |
In the sequel, we only deal with the problem (4.1) on interval [0,π2]. The unique solution on interval [τ,2τ], ⋯ can be likewise found.
Firstly, the problem (4.1) can be transformed as the problem
{cD12x(t)=~G1(t,y(t),cD12x(t)),cD12y(t)=~G2(t,x(t),cD12y(t)),x(0)=~Φ1(0),y(0)=~Φ2(0), | (4.2) |
where
~G1(t,y(t),cD12x(t))=cost~Φ2(t−π2)+~F1(t,y(t),cD12x(t)),~G2(t,x(t),cD12y(t))=sint~Φ1(t−π2)+~F2(t,x(t),cD12y(t)). |
Then, after performing some arithmetics, one can get
~G1=g1cr+⟨g1a,0,g1b⟩,~G2=g2cr+⟨g2a,0,g2b⟩,~Φ1(0)=ϕ1cr(0)+~ϕ1(0),~Φ2(0)=ϕ2cr(0)+~ϕ2(0), |
where
g1cr=−cos2t−0.5siny(t)+10−3sincD12x(t),g2cr=sin2t+0.7sinx(t)−10−3sincD12y(t),g1a=0.25cos2t,g1b=−0.25cos2t,g2a=−0.25sin2t,g2b=0.25sin2t,ϕ1cr(0)=1,~ϕ1(0)=(−0.25,0,0.25),ϕ2cr(0)=0,~ϕ2(0)=(−0.15,0,0.15). |
Next, we respectively solve the three subproblems of the problem (4.2) on [0,π2] one by one.
(i) With respect to g1cr and g2cr, we can rewrite them in another forms:
g1cr(t,u,v)=−cos2t−0.5sinu+10−3sinv,g2cr(t,m,n)=sin2t+0.7sinm−10−3sinn, |
where
u=y(t),v=cD12x(t),m=x(t),n=cD12y(t). |
We can easily see that g1cr,g2cr:[0,π2]×R×R→R are continuous and satisfy (H1).
Moreover, taking K1=0.5, K2=10−3, L1=0.7 and L2=10−3, then we have
max{K2+K1Γ(12+1),L2+L1Γ(12+1)}=max{10−3+0.5Γ(32),10−3+0.7Γ(32)}=max{0.5718,0.8000}=0.8000∈(0,1), |
and
∣g1cr(t,u1,v1)−g1cr(t,u2,v2)∣≤K1∣u1−u2∣+K2∣v1−v2∣,∣g2cr(t,m1,n1)−g2cr(t,m2,n2)∣≤L1∣m1−m2∣+L2∣n1−n2∣ |
for i=1,2 and any ui, vi, mi, ni∈R. Hence, it is easy to see that (H2) holds.
To sum up, all the hypotheses (H1) and (H2) in Theorem 3.1 are satisfied and we can obtain a unique solution Zcr(t), which is illustrated in Figure 2 to the following associated crisp subproblem via using the "L1 method" due to Li and Zeng [23]:
{cD12x(t)=g1cr(t,y(t),cD12x(t))=−cos2t−0.5siny(t)+10−3sincD12x(t),cD12y(t)=g2cr(t,x(t),cD12y(t))=sin2t+0.7sinx(t)−10−3sincD12y(t),x(0)=ϕ1cr(0)=1,y(0)=ϕ2cr(0)=0, | (4.3) |
which is the first subproblem of the problem (4.2).
From Figure 2, one can easily see that the order of magnitude of the ordinate value in Figure 2 is 108, and xcr and ycr established by relying on the "L1 method" discretization [23] of the Caputo fractional derivatives in (4.3), are growing very fast, and after iterating 43 times (t≈0.66), the values of xcr and ycr approach 1 and 27.88, respectively. Since the ordinate value of Figure 2 is too large to observe between t=0.6 and t=0.7, we give the local graph Figure 3 for the crisp discretized solutions to the problem (4.3) on the ordinate value interval [0,100], which right endpoint is far less than that of the ordinate value interval [0,4×108] in Figure 2. We note that Figure 3 locally shows the unique solution Zcr(t)=(xcr(t)ycr(t))=(127.88) of the problem (4.3) at t≈0.66∈[0,π2].
(ii) Solve the following crisp problem:
{cD12x(t)=0,cD12y(t)=0,x(0)=ϕ1(0),y(0)=ϕ2(0) |
for (ϕ1(0)ϕ2(0))=(−0.25−0.15) and (ϕ1(0)ϕ2(0))=(0.250.15), and find the solutions Za(t)=(−0.25−0.15) and Zb(t)=(0.250.15), respectively.
Letting ~ϕ1(0)=(−0.25,0,0.25) and ~ϕ2(0)=(−0.15,0,0.15), then it follows that the solution to the second subproblem of the problem (4.2) as hereunder mentioned:
{cD12x(t)=0,cD12y(t)=0,x(0)=~ϕ1(0),y(0)=~ϕ2(0) | (4.4) |
is the TFF matrix ~Zϕ=⟨Za,0,Zb⟩, which is graphed in Figure 4, and one knows that
~Zϕ(t)=(min{Za(t),0,Zb(t)},0,max{Za(t),0,Zb(t)}). |
Hence, Theorem 3.2 holds and there is one and only solution to the problem (4.4). Indeed, since the solutions Za(t) and Zb(t) are all constant matrices in Figure 4, it is easy to see that the values of ~xϕ1 and ~yϕ2 are all constants when MD α∈[0,1] is determined. That is, the values of ~xϕ1 and ~yϕ2 remain constant as t increases. For example, ~xϕ1a=0.25, ~xϕ1b=−0.25, ~yϕ2a=0.15 and ~yϕ2b=−0.15 when α=0.
(iii) Consider the following crisp problem:
{cD12x(t)=g1(t,y(t),cD12x(t)),cD12y(t)=g2(t,x(t),cD12y(t)),x(0)=0,y(0)=0 |
for (g1g2)=(g1ag2a) and (g1g2)=(g1bg2b), and find the solutions Zu(t)=(xu(t)yu(t)) and Zv(t)=(xv(t)yv(t)), respectively. That is, one solves the following crisp problems:
{cD12x(t)=g1a(t,y(t),cD12x(t))=0.25cos2t,cD12y(t)=g2a(t,x(t),cD12y(t))=−0.25sin2t,x(0)=0,y(0)=0 | (4.5) |
and
{cD12x(t)=g1b(t,y(t),cD12x(t))=−0.25cos2t,cD12y(t)=g2b(t,x(t),cD12y(t))=0.25sin2t,x(0)=0,y(0)=0. | (4.6) |
As for the problem (4.5), g1a and g2a can be written in following forms:
g1a(t,u,v)=0.25cos2t,g2a(t,m,n)=−0.25sin2t, |
where
u=y(t),v=cD12x(t),m=x(t),n=cD12y(t). |
It is clear to find that g1a,g2a:[0,π2]×R×R→R are continuous.
On the other hand, let K1=0.4, K2=0.1, L1=0.6 and L2=0.2. Then
max{K2+K1Γ(12+1),L2+L1Γ(12+1)}=max{0.1+0.4Γ(32),0.2+0.6Γ(32)}=max{0.5514,0.8770}=0.8770∈(0,1), |
and for each u1, u2, v1, v2, m1, m2, n1, n2∈R,
∣g1a(t,u1,v1)−g1a(t,u2,v2)∣≤K1∣u1−u2∣+K2∣v1−v2∣,∣g2a(t,m1,n1)−g2a(t,m2,n2)∣≤L1∣m1−m2∣+L2∣n1−n2∣. |
According to the above, all the hypotheses (H1) and (H2) hold. And that on the basis of Theorem 3.1, we can obtain the unique solution Zu(t) to the last subproblem of the problem (4.2) involving fuzzy source functions and zero initial functions as follows:
{cD12x(t)=⟨g1a,0,g1b⟩,cD12y(t)=⟨g2a,0,g2b⟩,x(0)=0,y(0)=0. | (4.7) |
Similarly, we can conclude that the problem (4.6) also has a unique solution Zv(t). It follows that the solution to the problem (4.7) is the TFF matrix ~Zg=⟨Zu,0,Zv⟩, which is depicted in Figure 5, and we have
~Zg(t)=(min{Zu(t),0,Zv(t)},0,max{Zu(t),0,Zv(t)}). |
As a consequence, Theorem 3.3 holds and there is a unique solution to the problem (4.7). Moreover, it can be seen that, in Figure 5, the images of ~xg1 and ~yg2 are respectively symmetric when the corresponding MDs are chosen. For MD α∈[0,1), as t increases, the upper branch of ~xg1 firstly increases and then decreases (t≈0.69 is the turning point), and the upper branch of ~yg2 gets bigger and bigger. Especially, the values of ~xg1 and ~yg2 are constant 0 when the MD α=1. However, the ordinate value of Figure 5 is of order of magnitude 10−1 and very small compared with that in Figure 2, so it has little effect on the final solution ˜Z(t).
In conclusion, by solving the problems (4.3), (4.4) and (4.7), it follows from Algorithm 3.1 that we can find the unique solution of the problem (4.1) on interval [0,π2] as
˜Z(t)=Zcr(t)+~Zϕ(t)+~Zg(t), |
which can be found from Figure 6. It is easy to note that the image of fuzzy solution ˜Z(t) in Figure 6 has a similar trend to that of the crisp solution Zcr(t) in Figure 2. That is because when MD is fixed, the unique solution ˜Z(t) of the problem (4.1) is composed of three parts and the value of the crisp solution Zcr(t) for the problem (4.3) is too large (order of magnitude 108 of the ordinate value for Figure 2). Further, since the ordinate value of Figure 6 is very big (order of magnitude 108) and the curves of ˜x,˜y can not be distinguished obviously, a local graph Figure 7 of Figure 6 is given for the fuzzy solutions of the problem (4.1) when the ordinate value interval is restricted to [0,30]. Choosing specially MD α=0, we have ~xϕ1a=0.93, ~xϕ1b=1.07, ~yϕ2a=27.68 and ~yϕ2b=28.08.
Before the end of this section, we remark that the unique solution of the problem (4.1) on interval [π2,π], ⋯ can be found in the same way. Therefore, one can find the unique solution of the problem (4.1) for t>0 via Algorithm (3.1).
In this paper, inspired by Fatullayev et al. [14] and Dong et al. [13], by using the methods of steps and separating fuzzy items, we solved the implicit coupled systems of fuzzy fractional delay differential equations with fuzzy initial values and source functions (FFIDDC) (1.1). Under the conditions (H1) and (H2), and based on the concept of TFFs and examples in [16,17], we obtained existence and uniqueness of solution for the FFIDDC (1.1). We note that the solution is a triangular fuzzy function matrix which is composed of real functional matrices. Furthermore, an algorithm for solution procedure and an example for graphical confirmation of explicit solution were given.
As Remark 1.1 mentioned, except for considering the problem (1.1) with Caputo fractional derivative, we will explore the problem of form (1.1) with fractional derivatives of Riemann-Liouville or Hadamard in the future. Recently, there are more and more researchers to explore the relevant (fractional) differentiable set-value problems and impulsive problems, such as Amrahov et al. [4] and Sun et al. [32]. Therefore, for further study, we can introduce the set-valued delay and the impulse into the FFIDDC (1.1), and extend the methods proposed in this paper to solve the new problems associated with set-values or impulsive elements.
We are grateful to the anonymous referees and editors for their valuable comments and helpful suggestions to improve the quality of this paper.
This work was partially supported by the Sichuan Science and Technology Program (2019YJ0541) the Opening Project of Sichuan Province University Key Laboratory of Bridge Non-destruction Detecting and Engineering Computing (2019QZJ03) and the Innovation Fund of Postgraduate, Sichuan University of Science & Engineering (y2020080).
All authors declare no conflicts of interest in this paper.
[1] |
Gordon-Larsen P, Heymsfield SB (2018) Obesity as a disease, not a behavior. Circulation 137: 1543-1545. https://doi.org/10.1161/CIRCULATIONAHA.118.032780 ![]() |
[2] |
Jastreboff AM, Kotz CM, Kahan S, et al. (2019) Obesity as a disease: The obesity society 2018 position statement. Obesity 27: 7-9. https://doi.org/10.1002/oby.22378 ![]() |
[3] | World Health OrganizationObesity and overweight. Available from: https://www.who.int/news-room/fact-sheets/detail/obesity-and-overweight |
[4] |
Valavanis IK, Mougiakakou SG, Grimaldi KA, et al. (2010) A multifactorial analysis of obesity as CVD risk factor: use of neural network based methods in a nutrigenetics context. BMC Bioinformatics 11: 453. https://doi.org/10.1186/1471-2105-11-453 ![]() |
[5] |
van der Sande MAB, Ceesay SM, Milligan PJM, et al. (2001) Obesity and undernutrition and cardiovascular risk factors in rural and urban Gambian communities. Am J Public Health 91: 1641-1644. https://doi.org/10.2105/ajph.91.10.1641 ![]() |
[6] | Popkin BM (2003) Dynamics of the nutrition transition and its implications for the developing world. Forum Nutr 56: 262-264. |
[7] |
Hall ME, do Carmo JM, da Silva AA, et al. (2014) Obesity, hypertension, and chronic kidney disease. Int J Nephrol Renov Dis 7: 75-88. https://doi.org/10.2147/IJNRD.S39739 ![]() |
[8] |
Eckel RH, Kahn SE, Ferrannini E, et al. (2011) Obesity and type 2 diabetes: what can be unified and what needs to be individualized?. J Clin Endocrinol Metab 96: 1654-1663. https://doi.org/10.1210/jc.2011-0585 ![]() |
[9] |
Farzadfar F, Finucane MM, Danaei G, et al. (2011) National, regional, and global trends in serum total cholesterol since 1980: systematic analysis of health examination surveys and epidemiological studies with 321 country-years and 3·0 million participants. Lancet 377: 578-586. https://doi.org/10.1016/S0140-6736(10)62038-7 ![]() |
[10] |
Lyall DM, Celis-Morales C, Ward J, et al. (2017) Association of body mass index with cardiometabolic disease in the UK Biobank: A mendelian randomization study. JAMA Cardiol 2: 882-889. https://doi.org/10.1001/jamacardio.2016.5804 ![]() |
[11] |
Ofori-Asenso R, Agyeman AA, Laar A, et al. (2016) Overweight and obesity epidemic in Ghana—a systematic review and meta-analysis. BMC Public Health 16: 1239. https://doi.org/10.1186/s12889-016-3901-4 ![]() |
[12] |
Unger T, Borghi C, Charchar F, et al. (2020) 2020 International Society of Hypertension Global Hypertension Practice Guidelines. Hypertension 75: 1334-1357. https://doi.org/10.1161/HYPERTENSIONAHA.120.15026 ![]() |
[13] |
Alberti KG, Zimmet PZ (1998) Definition, diagnosis and classification of diabetes mellitus and its complications. Part 1: diagnosis and classification of diabetes mellitus provisional report of a WHO consultation. Diabet Med 15: 539-553. https://doi.org/10.1002/(SICI)1096-9136(199807)15:7<539::AID-DIA668>3.0.CO;2-S ![]() |
[14] |
Grundy SM, Stone NJ, Bailey AL, et al. (2019) 2018 AHA/ACC/AACVPR/AAPA/ABC/ACPM/ADA/AGS/APhA/ASPC/NLA/PCNA Guideline on the management of blood cholesterol: A report of the American College of Cardiology/American Heart Association task force on clinical practice guidelines. J Am Coll Cardiol 73: e285-e350. https://doi.org/10.1016/j.jacc.2018.11.003 ![]() |
[15] |
Ritchie SA, Connell JMC (2007) The link between abdominal obesity, metabolic syndrome and cardiovascular disease. Nutr Metab Cardiovasc Dis 17: 319-326. https://doi.org/10.1016/j.numecd.2006.07.005 ![]() |
[16] |
Kales SN, Polyhronopoulos GN, Aldrich JM, et al. (1999) Correlates of body mass index in hazardous materials firefighters. J Occup Environ Med 41: 589-595. ![]() |
[17] | Donkor N, Farrell K, Ocho O, et al. (2020) Correlates of obesity indices and cardiovascular disease risk factors among Trinidadian nurses. Int J Africa Nurs Sci 12: 100194. https://doi.org/10.1016/j.ijans.2020.100194 |
[18] |
Cil H, Bulur S, Türker Y, et al. (2012) Impact of body mass index on left ventricular diastolic dysfunction. Echocardiography 29: 647-651. https://doi.org/10.1111/j.1540-8175.2012.01688.x ![]() |
[19] | Kossaify A, Nicolas N (2013) Impact of overweight and obesity on left ventricular diastolic function and value of tissue Doppler echocardiography. Clin Med Insights Cardiol 7: 43-50. https://doi.org/10.4137/CMC.S11156 |
[20] |
Reynolds K, Gu D, Whelton PK, et al. (2007) Prevalence and risk factors of overweight and obesity in China. Obesity 15: 10-18. https://doi.org/10.1038/oby.2007.527 ![]() |
[21] |
Gu D, Reynolds K, Wu X, et al. (2005) Prevalence of the metabolic syndrome and overweight among adults in China. Lancet 365: 1398-1405. https://doi.org/10.1016/S0140-6736(05)66375-1 ![]() |
[22] |
An R, Xiang X (2016) Age–period–cohort analyses of obesity prevalence in US adults. Public Health 141: 163-169. https://doi.org/10.1016/j.puhe.2016.09.021 ![]() |
[23] |
Yusuf S, Hawken S, Ounpuu S, et al. (2005) Obesity and the risk of myocardial infarction in 27,000 participants from 52 countries: A case-control study. Lancet 366: 1640-1649. https://doi.org/10.1016/S0140-6736(05)67663-5 ![]() |
[24] |
Liang X, Chen X, Li J, et al. (2018) Study on body composition and its correlation with obesity: A cohort study in 5121 Chinese Han participants. Medicine 97: e10722. https://doi.org/10.1097/MD.0000000000010722 ![]() |
[25] |
Moghaddam AA, Woodward M, Huxley R (2007) Obesity and risk of colorectal cancer: A meta-analysis of 31 studies with 70,000 events. Cancer Epidemiol Biomarkers Prev 16: 2533-2547. https://doi.org/10.1158/1055-9965.EPI-07-0708 ![]() |
[26] |
Valdes AM, Andrew T, Gardner JP, et al. (2005) Obesity, cigarette smoking, and telomere length in women. Lancet 366: 662-664. https://doi.org/10.1016/S0140-6736(05)66630-5 ![]() |
[27] | Maltais ML, Desroches J, Dionne IJ (2009) Changes in muscle mass and strength after menopause. J Musculoskelet Neuronal Interact 9: 186-197. |
[28] |
Crawford SL, Casey VA, Avis NE, et al. (2000) A longitudinal study of weight and the meno-pause transition: results from the Massachusetts Women's Health Study. Menopause 7: 96-104. https://doi.org/10.1097/00042192-200007020-00005 ![]() |
[29] |
Sun J, Zhou W, Gu T, et al. (2018) A retrospective study on association between obesity and cardiovascular risk diseases with aging in Chinese adults. Sci Rep 8: 5806. https://doi.org/10.1038/s41598-018-24161-0 ![]() |
[30] |
Abubakari AR, Lauder W, Agyemang C, et al. (2008) Prevalence and time trends in obesity among adult West African populations: A meta-analysis. Obes Rev 9: 297-311. https://doi.org/10.1111/j.1467-789X.2007.00462.x ![]() |
[31] | Benkeser RM, Biritwum R, Hill AG (2012) Prevalence of overweight and obesity and perception of healthy and desirable body size in Urban, Ghanaian women. Ghana Med J 46: 66-75. |
[32] | Aryeetey RNO (2016) Perceptions and experiences of overweight among women in the Ga East District, Ghana. Front Nutr 3: 13. https://doi.org/10.3389/fnut.2016.00013 |
[33] | Appiah CA, Steiner-Asiedu M, Otoo GE (2014) Predictors of overweight/obesity in Urban Ghanaian women. Int J Clin Nutr 2: 60-68. https://doi.org/10.12691/ijcn-2-3-3 |
[34] | Donkor N, Farrell K, Constable A, et al. (2015) Cardiovascular and type 2 diabetes risk factors in Liberian nurses. Int J Africa Nurs Sci 4: 1-6. https://doi.org/10.1016/j.ijans.2015.11.001 |
[35] |
Akpa OM, Made F, Ojo A, et al. (2020) Regional patterns and association between obesity and hypertension in Africa. Evidence from the H3Africa CHAIR study. Hypertension 75: 1167-1178. https://doi.org/10.1161/HYPERTENSIONAHA.119.14147 ![]() |
[36] |
Akil L, Ahmad HA (2011) Relationships between obesity and cardiovascular diseases in four Southern states and Colorado. J Health Care Poor Underserved 22: 61-72. https://doi.org/10.1353/hpu.2011.0166 ![]() |
[37] |
Poston WSC, Haddock CK, Jahnke SA, et al. (2011) The prevalence of overweight, obesity, and substandard fitness in a population-based firefighter cohort. J Occup Environ Med 53: 266-273. https://doi.org/10.1097/JOM.0b013e31820af362 ![]() |
[38] |
Sekokotla MA, Goswami N, Sewani-Rusike CR, et al. (2017) Prevalence of metabolic syndrome in adolescents living in Mthatha, South Africa. Ther Clin Risk Manag 13: 131-137. https://doi.org/10.2147/TCRM.S124291 ![]() |
[39] |
Choi B, Steiss D, Garcia-Rivas J, et al. (2016) Comparison of body mass index with waist circumference and skinfold-based percent body fat in firefighters: adiposity classification and associations with cardiovascular disease risk factors. Int Arch Occup Environ Health 89: 435-448. https://doi.org/10.1007/s00420-015-1082-6 ![]() |
[40] |
Soteriades ES, Hauser R, Kawachi I, et al. (2005) Obesity and cardiovascular disease risk factors in firefighters: A prospective cohort study. Obes Res 13: 1756-1763. https://doi.org/10.1038/oby.2005.214 ![]() |
[41] |
Clark S, Rene A, Theurer WM, et al. (2002) Association of body mass index and health status in firefighters. J Occup Environ Med 44: 940-946. https://doi.org/10.1097/00043764-200210000-00013 ![]() |
[42] |
Owusu IK, Acheamfour-Akowuah E (2018) Pattern of cardiovascular diseases as seen in an out-patient cardiac clinic in Ghana. World J Cardiovasc Dis 8: 70-84. https://doi.org/10.4236/wjcd.2018.81008 ![]() |
[43] | Acheamfour-Akowuah E, Owusu IK (2016) Prevalence and correlates of Electrocardiographic left ventricular hypertrophy in hypertensive patients at a specialist clinic in Techiman, Ghana. IOSR J Dent Med Sci 15: 100-109. https://doi.org/10.9790/0853-151103100109 |
[44] | Owusu IK, Boakye YA (2013) Prevalence and aetiology of heart failure in patients seen at a teaching hospital in Ghana. J Cardiovasc Dis Diagn 1: 131. https://doi.org/10.4172/2329-9517.1000131 |
[45] |
da Silva AA, do Carmo J, Dubinion J, et al. (2009) The role of the sympathetic nervous system in obesity-related hypertension. Curr Hypertens Rep 11: 206-211. https://doi.org/10.1007/s11906-009-0036-3 ![]() |
[46] |
Lambert GW, Straznicky NE, Lambert EA, et al. (2010) Sympathetic nervous activation in obesity and the metabolic syndrome—causes, consequences and therapeutic implications. Pharma-col Ther 126: 159-172. https://doi.org/10.1016/j.pharmthera.2010.02.002 ![]() |
[47] |
Aghamohammadzadeh R, Heagerty AM (2012) Obesity-related hypertension: epidemiology, pathophysiology, treatments, and the contribution of perivascular adipose tissue. Ann Med 44: S74-84. https://doi.org/10.3109/07853890.2012.663928 ![]() |
[48] |
Hall JE, da Silva AA, do Carmo JM, et al. (2010) Obesity-induced hypertension: role of sympathetic nervous system, leptin, and melanocortins. J Biol Chem 285: 17271-17276. https://doi.org/10.1074/jbc.R110.113175 ![]() |
[49] |
Byczek L, Walton SM, Conrad KM, et al. (2004) Cardiovascular risks in firefighters: implications for occupational health nurse practice. AAOHN J 52: 66-76. ![]() |
[50] |
Tokgozoglu L, Orringer C, Ginsberg HN, et al. (2022) The year in cardiovascular medicine 2021: dyslipidaemia. Eur Heart J 43: 807-817. https://doi.org/10.1093/eurheartj/ehab875 ![]() |
[51] |
McAloon CJ, Osman F, Glennon P, et al. (2016) Chapter 4—Global Epidemiology and Incidence of Cardiovascular Disease. Cardiovascular Diseases. Boston: Academic Press 57-96. https://doi.org/10.1016/B978-0-12-803312-8.00004-5 ![]() |
1. | Martha Álvarez–Ramírez, Johanna D. García–Saldaña, Mario Medina, Global Dynamics and Integrability of a Leslie-Gower Predator–Prey Model with Linear Functional Response and Generalist Predator, 2024, 23, 1575-5460, 10.1007/s12346-024-01155-0 | |
2. | Cahit Köme, Yasin Yazlik, Stability, bifurcation analysis and chaos control in a discrete predator–prey system incorporating prey immigration, 2024, 70, 1598-5865, 5213, 10.1007/s12190-024-02230-0 | |
3. | Maurıicio F. S. Lima, Jaume Llibre, Hopf bifurcation for a class of predator-prey system with small immigration, 2024, 32, 2688-1594, 4604, 10.3934/era.2024209 |