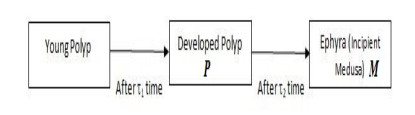
Epidemiological and environmental studies demonstrated that the rate of cancer mortality in the Acerra area, better known as "Triangle of Death", and, more in general, in the Neapolitan metropolitan territory are higher than the regional average values. In the "Triangle of Death" the higher rate of mortality has been mostly related to the presence of toxic wastes illegally buried in agricultural areas which have been contaminating soils and groundwater for decades. Thus, collecting a total of 154 samples over an area of about 100 km2, a detailed study was carried out to assess the geochemical-environmental conditions of soils aiming at defining the environmental hazard proceeding from 15 potentially toxic elements (PTEs), 9 polycyclic aromatic hydrocarbons (PAHs) and 14 organochlorine pesticides (OCPs) related with soil contamination. The study was also targeted at discriminating the contamination sources of these pollutants. Results showed that 9 PTEs, 5 PAHs and 6 OCPs are featured by concentrations higher than the guideline values established by the Italian Environmental laws, especially in the proximities of inhabited centers and industrial areas. The contamination source analysis revealed that, as regards the concentrations of chemical elements, they have a dual origin due to both the natural composition of the soils (Co-Fe-V-Tl-Be) and the pressure exerted on the environment by anthropic activities such as vehicular traffic (Pb-Zn-Sb-Sn) and agricultural practices (Cu-P). As far as organic compounds are concerned, the source of hydrocarbons can be mainly attributed to the combustion of biomass (i.e., grass, wood and coal), while for pesticides, although the use of some of them has been prohibited in Italy since the 1980s, it has been found that they are still widely used by local farmers.
Citation: Stefano Albanese, Annalise Guarino. Assessing contamination sources and environmental hazards for potentially toxic elements and organic compounds in the soils of a heavily anthropized area: the case study of the Acerra plain (Southern Italy)[J]. AIMS Geosciences, 2022, 8(4): 552-578. doi: 10.3934/geosci.2022030
[1] | Hongying Shu, Wanxiao Xu, Zenghui Hao . Global dynamics of an immunosuppressive infection model with stage structure. Mathematical Biosciences and Engineering, 2020, 17(3): 2082-2102. doi: 10.3934/mbe.2020111 |
[2] | Hongbin Guo, Michael Yi Li . Global dynamics of a staged progression model for infectious diseases. Mathematical Biosciences and Engineering, 2006, 3(3): 513-525. doi: 10.3934/mbe.2006.3.513 |
[3] | Yijun Lou, Bei Sun . Stage duration distributions and intraspecific competition: a review of continuous stage-structured models. Mathematical Biosciences and Engineering, 2022, 19(8): 7543-7569. doi: 10.3934/mbe.2022355 |
[4] | Jia Li . Malaria model with stage-structured mosquitoes. Mathematical Biosciences and Engineering, 2011, 8(3): 753-768. doi: 10.3934/mbe.2011.8.753 |
[5] | Wei Feng, Michael T. Cowen, Xin Lu . Coexistence and asymptotic stability in stage-structured predator-prey models. Mathematical Biosciences and Engineering, 2014, 11(4): 823-839. doi: 10.3934/mbe.2014.11.823 |
[6] | Sophia R.-J. Jang . Discrete host-parasitoid models with Allee effects and age structure in the host. Mathematical Biosciences and Engineering, 2010, 7(1): 67-81. doi: 10.3934/mbe.2010.7.67 |
[7] | Shunyi Li . Hopf bifurcation, stability switches and chaos in a prey-predator system with three stage structure and two time delays. Mathematical Biosciences and Engineering, 2019, 16(6): 6934-6961. doi: 10.3934/mbe.2019348 |
[8] | Zhisheng Shuai, P. van den Driessche . Impact of heterogeneity on the dynamics of an SEIR epidemic model. Mathematical Biosciences and Engineering, 2012, 9(2): 393-411. doi: 10.3934/mbe.2012.9.393 |
[9] | Asma Alshehri, John Ford, Rachel Leander . The impact of maturation time distributions on the structure and growth of cellular populations. Mathematical Biosciences and Engineering, 2020, 17(2): 1855-1888. doi: 10.3934/mbe.2020098 |
[10] | Jianxin Yang, Zhipeng Qiu, Xue-Zhi Li . Global stability of an age-structured cholera model. Mathematical Biosciences and Engineering, 2014, 11(3): 641-665. doi: 10.3934/mbe.2014.11.641 |
Epidemiological and environmental studies demonstrated that the rate of cancer mortality in the Acerra area, better known as "Triangle of Death", and, more in general, in the Neapolitan metropolitan territory are higher than the regional average values. In the "Triangle of Death" the higher rate of mortality has been mostly related to the presence of toxic wastes illegally buried in agricultural areas which have been contaminating soils and groundwater for decades. Thus, collecting a total of 154 samples over an area of about 100 km2, a detailed study was carried out to assess the geochemical-environmental conditions of soils aiming at defining the environmental hazard proceeding from 15 potentially toxic elements (PTEs), 9 polycyclic aromatic hydrocarbons (PAHs) and 14 organochlorine pesticides (OCPs) related with soil contamination. The study was also targeted at discriminating the contamination sources of these pollutants. Results showed that 9 PTEs, 5 PAHs and 6 OCPs are featured by concentrations higher than the guideline values established by the Italian Environmental laws, especially in the proximities of inhabited centers and industrial areas. The contamination source analysis revealed that, as regards the concentrations of chemical elements, they have a dual origin due to both the natural composition of the soils (Co-Fe-V-Tl-Be) and the pressure exerted on the environment by anthropic activities such as vehicular traffic (Pb-Zn-Sb-Sn) and agricultural practices (Cu-P). As far as organic compounds are concerned, the source of hydrocarbons can be mainly attributed to the combustion of biomass (i.e., grass, wood and coal), while for pesticides, although the use of some of them has been prohibited in Italy since the 1980s, it has been found that they are still widely used by local farmers.
Jellyfish plays an important role in the marine ecosystems as a keystone species and a potential resource for human consumption [1]. The amount of jellyfish has been significantly increasing in many waters since 1980s [2]. Jellyfish can be found in many regions worldwide such as Japan [3], the China Seas [4], the Mediterranean Sea [5], Taiwan [6], Southampton Water and Horsea Lake, England [7]. It can survive in a wide range of water temperatures $ (0- 36\; ^{\circ}C) $ and salinities $ (3- 36\%) $ [8,9].
Jellyfish has a complex life history with several different phases: planula, polyp, strobila, ephyra and medusa [10]. The polyp and medusa are two main stages of the life cycle of jellyfish. Medusae are dioecious, the sperm combines with egg to form a planula, which normally settles to the bottom and then occur metamorphosis of planula into tentacles-ring polyp (or scyphistoma) [11]. For Aurelia aurita jellyfish, the scyphistoma produces external outgrowths asexually by budding, the vitally asexual reproduction of polyp ($ 94\% $), stolon ($ 5\% $) and podocysts ($ 1\% $) [3]. The scyphistoma changes into strobila (strobilating polyp) through strobilation, which is asexual reproduction by division into segments developing into ephyra. After liberating from strobila, the ephyra becomes adult medusa. In addition, strobila reverts into the initial scyphistoma [11]. Since jellyfish has a distinct mobility patterns in different phases of its life history, it is interesting to take these facts into account for model formulation.
Temperature has a great effect on variations of jellyfish populations [12] as the asexual reproduction rate and strobilation rate depend on the functions of temperatures [11]. Global warming has affected the increase of jellyfish populations because it might cause the distribution, growth, and ephyrae production of medusae [13]. The rapid strobilation might be proceeded at the warmer temperature, but the continuous high temperature results in the fewer budding and increased mortality [6]. Hence the population explosions of polyps and medusae might be caused at the appropriate increase of temperature, but rising temperatures lead to the decreased populations.
Many approaches for jellyfish have been developed to discuss the nature of the correlations between environmental indices and population abundance [6,14]. In particular, mathematical modeling is one of the important tools in analyzing the dynamical properties in aquatic systems. In [15], Oguz et al. presented food web model with an anchovy population and bioenergetics-based weight growth model governed by system of differential equations. In [16], Melica et al. conducted that the dynamics of polyps population by the logistic model. In [11], Xie et al. proposed the following two-dimensional dynamic model of scyphozoan jellyfish:
$ \begin{equation} \begin{aligned} \frac{dP}{dt} = & \alpha(T)P+s_1\gamma M-d_1P-d_2P-b_1P^2, \\ \frac{dM}{dt} = & s_2\beta(T)nP-d_3M-d_4M-b_2M^2, \end{aligned} \end{equation} $ | (1.1) |
where $ P(t) $ and $ M(t) $ are the population sizes of polyps and medusae at time $ t $, respectively. For system (1.1), by using the Bendixson-Dulac's negative criterion and Poincare-Bendixson Theorem, the conditions for the global asymptotical stability of the equilibria $ E_0 $, $ E_1 $ and $ E^* $ are given. The effects of temperature, substrate and predation on the population sizes of scyphozoan were investigated by numerical simulations.
Although multiple progresses have been seen in the above work of [11], for system (1.1), it is assumed that each population preserves an equal density dependent rate and each individual has the same opportunity to compete for their common resources during the whole life history. Unfortunately, this is not realistic due to the life history of jellyfish which has a diverse mobility body structures in different stages. The immature stages of jellyfish are much weaker than the mature stages and so they cannot compete for their common resources. Jellyfish reaches maturity after surviving the immature stages. Therefore, it is realistic and interesting for us to construct the stage-structured jellyfish model that exhibits a diversity between these different stages.
Recently, population dynamic models with stage structure and time delays have attracted more attention from authors [17,18,19,20,21,22,23,24]. For instance, Aiello and Freedman proposed a stage-structured model of single species containing of the immature and mature stages and using a discrete time delay taken from birth to maturity [18]. Liu et al. showed that the global stability for the two competitive Lotka-Volterra system with time delay that denotes the time taken from birth to maturity. They proposed that the stage structure is one of the main reasons that cause permanence and extinction for the two competitive system [23]. There have been an increasing interest and progress in the study of the above stage-structured models which assume all individuals are in the same species that require the analogous amount of time to become mature at the same age. Unlike birds and mammals, jellyfish species have the different mobility shapes in the distinctive stages of its life cycle. Thus, the previous methods and techniques cannot be applied exactly to our system because we classify the single species jellyfish into two-stage structure. Mathematically, the proposed model has two delay terms and the equations are matched with each other, which is not similar with the previous models [18,23,24].
In this paper, we will propose a time-delayed jellyfish model with stage structure and will investigate how the stage structure parameters and temperature affect the dynamical behaviors of system (2.2). Our main purpose is to study the population dynamics of jellyfish for the largest surviving probability as well as for final population numbers. To find the largest surviving probability, we will take the global asymptotical behaviors of the model by applying the monotone dynamical properties (for reference, see [25] and [26,p. 90]).
This paper is organized as follows: in Section 2, we propose the model and show that the solutions are positiveness and ultimately bounded. The main results of this paper are presented in Section 3. In Section 4, we perform numerical simulations to explore the effects of two delays and temperature on the dynamics. Section 5 is the brief discussion of our results.
The life history of jellyfish is divided into two main stages; polyp and medusa. The larval stage of polyp is planula and the elementary phase of medusa is ephyra. Let $ P(t) $ and $ M(t) $ be the population size or number of polyps and medusae at time $ t $, respectively. The model is based on the following assumptions and the diagram in Figure 1:
$ \rm(A1) $ $ \tau_1 $ is the length of the stage from the young polyp to the mature polyp. The immature polyp reproduces asexually at time $ t-\tau_1 $ and surviving from time $ t-\tau_1 $ to $ t $ is $ e^{-(d_1+d_2)\tau_1} $.
$ \rm(A2) $ $ \tau_2 $ is the time lag taken from the developed polyp to ephyra (incipient medusa), i.e., the developed polyp reaches ephyra after surviving this stage. Denote $ \tau = \max\lbrace{\tau_1, \tau_2}\rbrace $.
$\rm (A3) $ Its maturity is denoted by $ \tau = \max\lbrace{\tau_1, \tau_2}\rbrace, \tau > 0 $.
$ \rm(A4) $ Each population competes for their common resources.
$ \rm(A5) $ Each population has its own natural death rate, the mortality of polyp is varied by the factors of silt coverage or nudibranch consumption while that of medusa is because of different types of predators.
By the preceding assumptions, we get the following polyp-medusa population with stage structure:
$ \begin{equation} \begin{array}{rcl} \frac{dP}{dt}& = &\alpha(T)e^{-(d_1+d_2)\tau_1}P(t-\tau_1)+s_1\gamma M-d_1P-d_2P-b_1P^2, \\[6pt] \frac{dM}{dt}& = &s_2\beta(T)ne^{-(d_3+d_4)\tau_2}P(t-\tau_2)-d_3M-d_4M-b_2M^2. \end{array} \end{equation} $ | (2.1) |
As pointed out in [11], $ \alpha(T) $ denotes the asexual reproduction rate affected by temperature, involving budding, stolon and podocyst et al., $ s_1 $ is the survival and metamorphosis rate of planula, $ \gamma $ represents the sexual reproduction rate, $ b_1 $ and $ b_2 $ denote the density-dependent rates of polyps and medusae respectively, $ s_2 $ is the survival and development rate of ephyra, $ \beta(T) $ is the strobilation rate affected by temperature and $ n $ is strobilation times. Assuming that the death rate of the immature population is proportional to the existing immature population with proportionality constants $ d_i > 0 $, i = 1, 2, 3, 4. The loss of polyp is either due to natural death rate $ d_1 $ or due to the factors of silt coverage $ d_2 $ and $ \tau_1 $ is the time taken from the immature polyp to the mature one; thus $ e^{-(d_1+d_2)\tau_1} $ is the survival probability of each immature polyp to reach the mature one. The death of medusa is either from natural fatality rate $ d_3 $ or because of the predations $ d_4 $ and $ \tau_2 $ is the time length between the developed polyp and ephyra (incipient medusa); thus $ e^{-(d_3+d_4)\tau_2} $ is the survival rate of each developed polyp to reach the ephyra (incipient medusa) population. As it takes a few days in the larval stage of jellyfish life, the permanence and extinction criteria for the stage structured model are independent in this larval stage.
For the goal of simplicity, we denote $ a = \alpha(T), $ $ b = s_1\gamma, $ $ d = d_1+d_2 $, $ c = s_2\beta(T)n, $ $ d_* = d_3+d_4 $. Thus the following system can be obtained from system (2.1).
$ \begin{equation} \begin{array}{ll} \frac{dP}{dt} & = ae^{-\zeta_1}P(t-\tau_1)+bM-dP-b_1P^2, \\[6pt] \frac{dM}{dt} & = ce^{-\zeta_2}P(t-\tau_2)-d_*M-b_2M^2, \end{array} \end{equation} $ | (2.2) |
where $ \zeta_1 = d\tau_1 $ and $ \zeta_2 = d_*\tau_2 $. Denote $ \zeta_1 $ and $ \zeta_2 $ the degrees of the stage structure.
Let $ X = \mathcal{C}([-\tau, 0], \mathbb{R}^2) $ be the Banach space of all continuous function from $ [-\tau, 0] $ to $ \mathbb{R}^2 $ equipped with the supremum norm, where $ \tau = \max\lbrace{\tau_1, \tau_2}\rbrace $. By the standard theory of functional differential equations (see, for example, Hale and Verduyn Lunel [27]), for any $ \psi\in\mathcal{C}([-\tau, 0], \mathbb{R}^2) $, there exists a unique solution $ Y(t, \psi) = (P(t, \psi), M(t, \psi)) $ of system (2.2); which satisfies $ Y_0 = \psi $.
For system (2.2), we consider the initial conditions to either the positive cone $ X^+ = \{\psi\in X|\mbox{ $\psi_i(\theta)\ge 0$ for all $\theta\in[-\tau, 0]$, $i = 1, 2$ }\} $ or the subset of $ X^+ $ of functions which are strictly positive at zero, $ X_0^+ = \lbrace{\psi\in X^+|\psi_i(0) > 0, i = 1, 2}\rbrace $.
Lemma 2.1. For equation
$ \begin{equation} \frac{d\overline{W}}{dt} = ae^{-\zeta_1}\overline{W}(t-\tau_1)+\frac{bce^{-\zeta_2}}{d_*}\overline{W}(t-\tau_2)-\frac{B}{2}\overline{W}^2, \end{equation} $ | (2.3) |
where $ a, b, c, d_*, B > 0 $, $ \tau = \max\lbrace{\tau_1, \tau_2}\rbrace, \tau > 0 $ and $ \overline{W}(0) > 0 $ and $ \overline{W}(\theta)\ge 0 $, $ \theta\in[-\tau, 0] $, we have: $ \lim_{t\to\infty}\overline{W}(t) = \frac{2d_*ae^{-\zeta_1}+2bce^{-\zeta_2}}{d_*B} $ if $ d_*ae^{-\zeta_1}+bce^{-\zeta_2} > 0 $.
Proof. By using the similar argument of Lu et al. [28,Proposition 1], we will prove that $ \overline{W}(t) > 0 $, for all $ t\ge0 $. Otherwise, there exists some constant $ \acute{t}_0 > 0 $ such that $ \min \lbrace{\overline{W}(\acute{t}_0)}\rbrace = 0. $ Let $ t_0 = \inf \lbrace{\acute{t}_0: \overline{W}(\acute{t}_0) = 0}\rbrace. $ Then we have that $ \min \lbrace{\overline{W}(t_0)} = 0\rbrace $ and $ \min \lbrace{\overline{W}(t)}\rbrace > 0 $, $ \forall t \in [0, t_0) $. From system (2.3), we have
$ \begin{equation} \begin{aligned} \overline{W}(t)& = \overline{W}(0)e^{-\int_0^t \frac{B}{2}\overline{W}(\vartheta)d\vartheta}+ae^{-\zeta_1}\int_0^t \overline{W}(\omega-\tau_1)e^{-\int_\omega^t \frac{B}{2}\overline{W}(\vartheta)d\vartheta}d\omega\\ &+\frac{bce^{-\zeta_2}}{d_*}\int_0^t \overline{W}(\omega-\tau_2)e^{-\int_\omega^t \frac{B}{2}\overline{W}(\vartheta)d\vartheta}d\omega. \end{aligned} \end{equation} $ | (2.4) |
Incorporation initial conditions and Eq (2.4), we get $ \overline{W}(t_0) > 0 $, contradicting $ \min \lbrace{\overline{W}(t_0)}\rbrace = 0 $. Consequently, $ \overline{W}(t) > 0 $ for all $ t \ge 0 $.
Let $ \overline{W}^* = \frac{2d_*ae^{-\zeta_1}+2bce^{-\zeta_2}}{d_*B} $ denotes the unique positive equilibrium of system (2.3). Denote $ u(t) = \overline{W}(t)-\overline{W}^* $, thus system (2.3) takes the form as
$ \begin{equation} \begin{aligned} \frac{du}{dt} = ae^{-\zeta_1}u(t-\tau_1)+\frac{bce^{-\zeta_2}}{d_*}u(t-\tau_2)-\frac{B}{2}u^2(t)-B\overline{W}^*u(t). \end{aligned} \end{equation} $ | (2.5) |
Constructing the Lyapunov functional
$ \begin{equation*} \begin{aligned} V(u, u_\tau) = \frac{1}{2}u^2(t)+\frac{1}{2}ae^{-\zeta_1}\int_{t-\tau_1}^{t}u^2(s)ds+\frac{1}{2}\frac{bce^{-\zeta_2}}{d_*}\int_{t-\tau_2}^{t}u^2(s)ds, \end{aligned} \end{equation*} $ |
we have
$ \begin{equation*} \begin{aligned} \left.\frac{dV}{dt}\right|_{(2.5)} = &ae^{-\zeta_1}u(t)u(t-\tau_1)+\frac{bce^{-\zeta_2}}{d_*}u(t)u(t-\tau_2)-\frac{B}{2}u^3(t)-B\overline{W}^*u^2(t)+\frac{1}{2}ae^{-\zeta_1}u^2(t)\\ &-\frac{1}{2}ae^{-\zeta_1}u^2(t-\tau_1)+ \frac{1}{2}\frac{bce^{-\zeta_2}}{d_*}u^2(t)-\frac{1}{2}\frac{bce^{-\zeta_2}}{d_*}u^2(t-\tau_2)\\ \le&\frac{1}{2} ae^{-\zeta_1}u^2(t)+\frac{1}{2} ae^{-\zeta_1}u^2(t-\tau_1)+\frac{1}{2}\frac{bce^{-\zeta_2}}{d_*}u^2(t)+\frac{1}{2}\frac{bce^{-\zeta_2}}{d_*}u^2(t-\tau_2)-\frac{B}{2}u^3(t)\\ &-B\overline{W}^*u^2(t)+\frac{1}{2}ae^{-\zeta_1}u^2(t)-\frac{1}{2}ae^{-\zeta_1}u^2(t-\tau_1)+ \frac{1}{2}\frac{bce^{-\zeta_2}}{d_*}u^2(t)-\frac{1}{2}\frac{bce^{-\zeta_2}}{d_*}u^2(t-\tau_2)\\ = &ae^{-\zeta_1}u^2(t)+\frac{bce^{-\zeta_2}}{d_*}u^2(t)-B\overline{W}^*u^2(t)-\frac{B}{2}u^3(t)\\ = &(ae^{-\zeta_1}+\frac{bce^{-\zeta_2}}{d_*}-\frac{B}{2}\overline{W}^*)u^2(t)-(\overline{W}^*+u(t))\frac{B}{2}u^2(t)\\ = &-\frac{B}{2}\overline{W}(t)u^2(t)\le 0, \end{aligned} \end{equation*} $ |
which is negative definite and $ \left.\frac{dV}{dt}\right|_{(2.5)} = 0 $ if and only if $ u = 0 $. By Lyapunov-LaSalle invariance principle ([29,Theorem 2.5.3]), we get $ \lim_{t\to\infty}\overline{W}(t) = \overline{W}^* = \frac{2d_*ae^{-\zeta_1}+2bce^{-\zeta_2}}{d_*B} $, this proves Lemma 2.1.
Lemma 2.2. Given system (2.2), then:
(I) Under the initial conditions, all the solutions of system (2.2) are positive for all $ t\ge 0 $.
(II) Solutions of system (2.2) are ultimately bounded.
Proof. (I) We start with proving the positivity of solutions by using the similar argument of Lu et al. [28,Proposition 1]. We will prove that $ P(t) > 0 $, $ M(t) > 0 $ for $ t\ge 0 $. Otherwise, there exists some constant $ \tilde{t}_0 > 0 $ such that $ \min\lbrace{P(\tilde{t}_0), M(\tilde{t}_0)\rbrace = 0} $. Let $ t_0 = \inf\lbrace{\tilde{t}_0:P(\tilde{t}_0) = 0, M(\tilde{t}_0) = 0}\rbrace $. Then we have that $ \min\lbrace{P(t_0), M(t_0)}\rbrace = 0 $ and $ \min\lbrace{P(t), M(t)}\rbrace > 0 $, $ \forall t\in[0, t_0) $. From system (2.2), we have
$ \begin{equation} \left\{ \begin{aligned} P(t) = &P(0)e^{-\int_{0}^{t}(d+b_1P(\eta))d\eta}+ae^{-\zeta_1} \int_{0}^{t}P(\kappa-\tau_1)e^{-\int_{\kappa}^{t}(d+b_1P(\eta))d\eta} d\kappa\\ &+b\int_{0}^{t}M(\kappa)e^{-\int_{\kappa}^{t}(d+b_1P(\eta))d\eta} d\kappa, \\ M(t) = &M(0)e^{-\int_{0}^{t}(d_*+b_2M(\eta))d\eta} +ce^{-\zeta_2} \int_{0}^{t}P(\kappa-\tau_2)e^{-\int_{\kappa}^{t}(d_*+b_2M(\eta))d\eta} d\kappa. \end{aligned} \right. \end{equation} $ | (2.6) |
Incorporation initial conditions and Eq (2.6), we obtain $ P(t_0) > 0 $ and $ M(t_0) > 0 $, contradicting $ \min\lbrace{P(t_0), M(t_0)}\rbrace = 0 $. Consequently, $ P(t) > 0 $, $ M(t) > 0 $ for all $ t\ge 0 $.
(II) We show that the boundedness of solutions as follows.
Let $ W = \frac{d_*}{b}P+M $. By system (2.2), we have
$ \begin{equation*} \begin{aligned} \left.\frac{dW}{dt}\right|_{(2.2)} = &ae^{-\zeta_1}\frac{d_*}{b}P(t-\tau_1)+ce^{-\zeta_2}P(t-\tau_2)-\frac{dd_*}{b}P-\frac{d_*b_1}{b}P^2-b_2M^2\\ = &ae^{-\zeta_1}[\frac{d_*}{b}P(t-\tau_1)+M(t-\tau_1)]-ae^{-\zeta_1}M(t-\tau_1)+\frac{bce^{-\zeta_2}}{d_*}[\frac{d_*}{b}P(t-\tau_2)+M(t-\tau_2)]\\ &-\frac{bce^{-\zeta_2}}{d_*}M(t-\tau_2)-\frac{dd_*}{b}P-\frac{bb_1}{d_*}(\frac{d_*}{b}P)^2-b_2M^2\\ \le&ae^{-\zeta_1}W(t-\tau_1)+\frac{bce^{-\zeta_2}}{d_*}W(t-\tau_2)-B[(\frac{d_*}{b}P)^2+M^2], \end{aligned} \end{equation*} $ |
where $ B: = \min{\{\frac{bb_1}{d_*}, b_2}\} $.
$ \frac{dW}{dt}\le ae^{-\zeta_1}W(t-\tau_1)+\frac{bce^{-\zeta_2}}{d_*}W(t-\tau_2)-\frac{B}{2}W^2, $ |
where $ -2((\frac{d_*}{b}P)^2+M^2)\le -(\frac{d_*}{b}P+M)^2 $.
Consider the equation
$ \begin{equation} \frac{d\overline{W}}{dt} = ae^{-\zeta_1}\overline{W}(t-\tau_1)+\frac{bce^{-\zeta_2}}{d_*}\overline{W}(t-\tau_2)-\frac{B}{2}\overline{W}^2. \end{equation} $ | (2.7) |
By using Lemma 2.1 and Comparison Theorem, we get
$ \limsup_{t\to\infty}W(t)\le \frac{2d_*ae^{-\zeta_1}+2bce^{-\zeta_2}}{d_*B} $, which implies $ P(t) $ and $ M(t) $ are ultimately bounded. This completes the proof of Lemma 2.2.
The equilibria $ (P, M) $ of system (2.2) satisfies the following system
$ \begin{equation} \begin{aligned} ae^{-\zeta_1}P+bM-dP-b_1P^2 = 0, \\ ce^{-\zeta_2}P-d_*M-b_2M^2 = 0. \end{aligned} \end{equation} $ | (2.8) |
System (2.2) has the equilibria $ E_0 = (0, 0) $ for all parameter values and $ E_1 = (\frac{ae^{-\zeta_1}-d}{b_1}, 0) $ if $ ae^{-\zeta_1}-d > 0 $ and $ c = 0 $.
Since Eq (2.8) can be rewritten as
$ \begin{equation} \begin{aligned} (ae^{-\zeta_1}-d-b_1P)P& = -bM, \ \ ce^{-\zeta_2}P& = (d_*+b_2M)M. \end{aligned} \end{equation} $ | (2.9) |
When $ PM\neq 0 $, from Eq (2.9) it follows that
$ \begin{equation} \frac{ae^{-\zeta_1}-d-b_1P}{ce^{-\zeta_2}}+\frac{b}{d_*+b_2M} = 0. \end{equation} $ | (2.10) |
Further, substituting $ P = \frac{(d_*+b_2M)M}{ce^{-\zeta_2}} $ into Eq (2.10) and we get
$ \frac{ae^{-\zeta_1}-d-b_1 \frac{(d_*+b_2M)M}{ce^{-\zeta_2}}}{ce^{-\zeta_2}}+\frac{b}{d_*+b_2M} = 0. $ |
Set
$ F(M): = \frac{ae^{-\zeta_1}-d-b_1 \frac{(d_*+b_2M)M}{ce^{-\zeta_2}}}{ce^{-\zeta_2}}+\frac{b}{d_*+b_2M}, $ |
thus $ F(M) $ is a decreasing function of $ M $ for any $ M > 0 $.
Noting that the continuity and monotonicity of $ F(M) $ and that $ F(+\infty) < 0 $, furthermore since one can get $ F(0) > 0 $ provided that
$ \begin{equation} (ae^{-\zeta_1}-d)d_*+bce^{-\zeta_2} > 0, \ \ c\neq 0 \end{equation} $ | (2.11) |
hold true, therefore we conclude that system (2.2) admits a unique positive equilibrium given Eq (2.11) is satisfied.
The purpose of this section is to study the global stability of system (2.2).
Now we consider the local stability of the equilibria. The characteristic equation of system (2.2) takes the form as follows;
$ \begin{equation*} \det(\lambda I-G-H_1e^{-\lambda \tau_1}-H_2e^{-\lambda \tau_2}) = 0, \end{equation*} $ |
where
$ \begin{equation*} G = \begin{pmatrix} -d-2b_1P &b\\ 0&-d_*-2b_2M \end{pmatrix}, H_1 = \begin{pmatrix} ae^{-\zeta_1} &0\\ 0&0 \end{pmatrix}, H_2 = \begin{pmatrix} 0 &0\\ ce^{-\zeta_2}&0 \end{pmatrix}. \end{equation*} $ |
Lemma 3.1. Suppose that $ (ae^{-\zeta_1}-d)d_*+bce^{-\zeta_2} < 0 $, then the equilibrium $ E_0 = (0, 0) $ of system (2.2) is locally asymptotically stable.
Proof. The characteristic equation of system (2.2) at the equilibrium $ E_0 $ is as follows:
$ \begin{equation} C(\lambda): = (\lambda+d_*)(\lambda+d-ae^{-\zeta_1-\lambda\tau_1})-bce^{-\zeta_2-\lambda\tau_2} = 0. \end{equation} $ | (3.1) |
To show that it is asymptotically stable under assumption $ (ae^{-\zeta_1}-d)d_*+bce^{-\zeta_2} < 0 $, we just need to prove that the solutions of the characteristic equation $ C(\lambda) = 0 $ must have negative real parts. Let $ \lambda = u+iv $, where $ u $ and $ v $ are real numbers. Denote
$ \begin{equation*} \begin{aligned} A_1 = &u+d_*, \qquad &B_1 = &v, \\ A_2 = &u+d-ae^{-\zeta_1-u\tau_1}\cos(v\tau_1), \qquad &B_2 = &v+ae^{-\zeta_1-u\tau_1}\sin(v\tau_1), \\ C_1 = &bce^{-\zeta_2-u\tau_2}\cos(v\tau_2), \qquad &C_2 = &-bce^{-\zeta_2-u\tau_2}\sin(v\tau_2). \end{aligned} \end{equation*} $ |
Substituting $ \lambda $ by $ u+iv $ into Eq (3.1)
$ \begin{equation*} \begin{aligned} A_1A_2-B_1B_2 = C_1, \quad A_1B_2+A_2B_1 = C_2. \end{aligned} \end{equation*} $ |
Then
$ \begin{equation} (A_1A_2)^2+(B_1B_2)^2+(A_1B_2)^2+(A_2B_1)^2 = (C_1)^2+(C_2)^2. \end{equation} $ | (3.2) |
Assume that $ u\ge 0 $, then we get
$ A_1\ge d_* > 0, \quad A_2\ge d-ae^{-\zeta_1} > 0. $ |
Hence
$ \begin{equation} \begin{aligned} (A_1A_2)^2 > ((d-ae^{-\zeta_1})d_*)^2. \end{aligned} \end{equation} $ | (3.3) |
$ \begin{equation*} (A_1A_2)^2+(B_1B_2)^2+(A_1B_2)^2+(A_2B_1)^2 \ge (A_1A_2)^2 > ((d-ae^{-\zeta_1})d_*)^2. \end{equation*} $ |
From Eq (3.2), we obtain
$ \begin{equation*} \begin{aligned} (C_1)^2+(C_2)^2&\ge (A_1A_2)^2 > ((d-ae^{-\zeta_1})d_*)^2\\ (bce^{-\zeta_2})^2 (\cos^2(v\tau_2)+\sin^2(v\tau_2))&\ge (A_1A_2)^2 > ((d-ae^{-\zeta_1})d_*)^2\\ (bce^{-\zeta_2})^2&\ge (A_1A_2)^2 > ((d-ae^{-\zeta_1})d_*)^2. \end{aligned} \end{equation*} $ |
Hence Eq (3.3) contradicts to the assumption $ (ae^{-\zeta_1}-d)d_*+bce^{-\zeta_2} < 0 $, thus $ u < 0 $, which means $ \lambda $ must have negative real parts. This proves Lemma 3.1.
Lemma 3.2. Suppose that $ ae^{-\zeta_1}-d > 0 $ and $ c = 0 $, then the equilibrium $ E_1 = (\frac{ae^{-\zeta_1}-d}{b_1}, 0) $ of system (2.2) is locally asymptotically stable.
Proof. The characteristic equation of system (2.2) at the equilibrium $ E_1 $ is
$ \begin{equation} \begin{aligned} X(\lambda): = (\lambda+d_*)(\lambda-d+2ae^{-\zeta_1}-ae^{-\zeta_1-\lambda\tau_1}) = 0. \end{aligned} \end{equation} $ | (3.4) |
Then $ \lambda = -d_* $ is a negative root of the equation $ X(\lambda) = 0 $. Let $ \lambda-d+2ae^{-\zeta_1}-ae^{-\zeta_1-\lambda\tau_1} = 0 $; then if the root is $ \lambda = u+iv $, we have $ u+2ae^{-\zeta_1}-d-ae^{-\zeta_1-u\tau_1}\cos(v\tau_1) = 0 $. Assume that $ u\ge 0 $, then $ u+2ae^{-\zeta_1}-d-ae^{-\zeta_1-u\tau_1}\cos(v\tau_1)\ge ae^{-\zeta_1}-d > 0 $ is a contradiction, hence $ u < 0 $. This shows that all the roots of $ X(\lambda) = 0 $ must have negative real parts, and therefore $ E_1 $ is locally asymptotically stable. This proves Lemma 3.2.
Lemma 3.3. Suppose that $ (ae^{-\zeta_1}-d)d_*+bce^{-\zeta_2} > 0 $ and $ c\neq0 $, then the equilibrium $ E^* = (P^*, M^*) $ of system (2.2) is locally asymptotically stable.
Proof. The characteristic equation of system (2.2) at the equilibrium $ E^* $ is
$ \begin{equation*} \begin{aligned} (\lambda+d+2b_1P^*-ae^{-\zeta_1-\lambda\tau_1})(\lambda+d_*+2b_2M^*)-bce^{-\zeta_2-\lambda\tau_2} = 0. \end{aligned} \end{equation*} $ |
To show that it is asymptotically stable under $ (ae^{-\zeta_1}-d)d_*+bce^{-\zeta_2} > 0 $, we just need to prove that the solutions of the characteristic equation must have negative real parts. Let $ \lambda = u+iv $ where $ u $ and $ v $ are real numbers. Denote
$ \begin{aligned} D_1 = &u+d+2b_1P^*-ae^{-\zeta_1-u\tau_1}\cos(v\tau_1), &E_1 = &v+ae^{-\zeta_1-u\tau_1}\sin(v\tau_1), \\ D_2 = &u+d_*+2b_2M^*, &E_2 = &v, \\ F_1 = &bce^{-\zeta_2-u\tau_2}\cos(v\tau_2), &F_2 = &-bce^{-\zeta_2-u\tau_2}\sin(v\tau_2). \end{aligned} $ |
Substituting $ \lambda $ by $ u+iv $ into the above equation.
$ \begin{equation*} \begin{aligned} D_1D_2-E_1E_2 = F_1, \quad D_1E_2+D_2E_1 = F_2. \end{aligned} \end{equation*} $ |
Then
$ \begin{equation} \begin{aligned} (D_1D_2)^2+(E_1E_2)^2+(D_1E_2)^2+(D_2E_1)^2 = (F_1)^2+(F_2)^2. \end{aligned} \end{equation} $ | (3.5) |
Assume that $ u\ge 0 $, then we get
$ \begin{equation*} \begin{aligned} D_1&\ge d+2b_1P^*-ae^{-\zeta_1} > d+2b_1 \frac{ae^{-\zeta_1}-d}{b_1}-ae^{-\zeta_1} = ae^{-\zeta_1}-d > 0, \\ D_2&\ge d_*+2b_2M^* = d_*+2b_2\frac{b_1(P^*)^2-(ae^{-\zeta_1}-d)P^*}{b_1}\\ & > d_*+2b_2 \frac {b_1(\frac{ae^{-\zeta_1}-d}{b_1})^2-(ae^{-\zeta_1}-d)(\frac{ae^{-\zeta_1}-d}{b_1})}{b_1} = d_* > 0. \end{aligned} \end{equation*} $ |
Hence
$ \begin{equation*} (D_1D_2)^2 > ((ae^{-\zeta_1}-d)d_*)^2. \end{equation*} $ |
$ \begin{equation*} (D_1D_2)^2+(E_1E_2)^2+(D_1E_2)^2+(D_2E_1)^2 \ge (D_1D_2)^2 > ((ae^{-\zeta_1}-d)d_*)^2. \end{equation*} $ |
By Eq (3.5), we get
$ \begin{equation*} \begin{aligned} (F_1)^2+(F_2)^2\ge (D_1D_2)^2 & > ((ae^{-\zeta_1}-d)d_*)^2\\ (bce^{-\zeta_2})^2 (\cos^2(v\tau_2)+\sin^2(v\tau_2))&\ge (D_1D_2)^2 > ((ae^{-\zeta_1}-d)d_*)^2\\ (bce^{-\zeta_2})^2\ge (D_1D_2)^2& > ((ae^{-\zeta_1}-d)d_*)^2. \end{aligned} \end{equation*} $ |
By assumption $ (ae^{-\zeta_1}-d)d_*+bce^{-\zeta_2} > 0 $, which is a contradiction, thus $ u $ must be negative real parts. This completes the proof of Lemma 3.3.
Before the details, we will present the notion from the literature [25]. We define
$ x_t\in\mathcal{C}([-\tau, 0], \mathbb{R}^2), $ |
by $ x_t(\theta) = x(t+\theta), \forall \theta\in[-\tau, 0] $. Consider a delay system
$ \begin{equation} \begin{aligned} x'(t) = f(x_t), \end{aligned} \end{equation} $ | (3.6) |
for which uniqueness of solutions is assumed, $ x(t, \psi) $ designates the solution of Eq (3.6) with initial condition $ x_0 = \psi $ $ (\psi\in\mathcal{C}) $.
A non-negative equilibrium $ v = (v_p, v_m)\in \mathbb{R}^2 $ of system (2.2) is said to be globally attractive if $ Y(t)\to v $ as $ t\to\infty $, for all admissible solutions $ Y(t) $ of system (2.2). We say that $ v $ is globally asymptotically stable if it is stable and globally attractive.
System (2.2) is written as Eq (3.6),
$ \begin{equation} \begin{aligned} f_1(\psi) = &\psi_1(0)[-d-b_1\psi_1(0)]+ae^{-\zeta_1}\psi_1(-\tau_1)+b\psi_2(0), \\ f_2(\psi) = &\psi_2(0)[-d_*-b_2\psi_2(0)]+ce^{-\zeta_2}\psi_1(-\tau_2). \end{aligned} \end{equation} $ | (3.7) |
Observe that system (2.2) is cooperative, i.e., $ Df_i(\psi)\varphi\ge 0 $, for all $ \psi, \varphi\in X^+ $ with $ \varphi_i(0) = 0, i = 1, 2 $. This implies that $ f $ satisfies quasi-monotonicity condition [26,p. 78]. Typically, in population dynamics the stability of equilibria is closely related to the algebraic properties of some kinds of competition matrix of the community. Denote
$ \begin{equation*} A = \begin{pmatrix} ae^{-\zeta_1}-d&0\\ 0&-d_* \end{pmatrix}, \quad D = \begin{pmatrix} 0&b\\ ce^{-\zeta_2}&0 \end{pmatrix}. \end{equation*} $ |
For convenience, we shall refer to $ N = A+D $ as the (linear) community matrix:
$ \begin{equation} N = \begin{pmatrix} ae^{-\zeta_1}-d&b\\ ce^{-\zeta_2}&-d_* \end{pmatrix}. \end{equation} $ | (3.8) |
Since $ D\ge 0 $, the matrix $ N $ in Eq (3.8) is called cooperative. If $ D $ is irreducible, then the matrix $ N $ in Eq (3.8) is also irreducible; in this case, system (2.2) is called an irreducible system [26,p. 88], and the semiflow $ \psi\mapsto Y_t(\psi) $ is eventually strongly monotone. $ f = (f_1, f_2)^T:\mathbb{R}^2 \to \mathbb{R}^2 $ is strictly sublinear, i.e., for any $ P\gg 0, M\gg 0 $ and any $ \alpha\in(0, 1), $
$ \begin{equation*} \begin{aligned} f_1(\alpha P, \alpha M) = &\alpha P[-d-b_1\alpha P]+ae^{-\zeta_1}\alpha P(t-\tau_1)+b\alpha M\\ > & \alpha [P(-d-b_1P)+ae^{-\zeta_1} P(t-\tau_1)+b M] = \alpha f_1(P, M), \\ f_2(\alpha P, \alpha M) = &\alpha M[-d_*-b_2\alpha M]+ce^{-\zeta_2}\alpha P(t-\tau_2)\\ > &\alpha [M(-d_*-b_2M)+ce^{-\zeta_2}P(t-\tau_2)] = \alpha f_2(P, M). \end{aligned} \end{equation*} $ |
Cooperative $ DDEs $ satisfying these sublinearity conditions have significant properties [30,Proposition 4.3].
Recall that the stability modulus of square matrix $ N $ in Eq (3.8), denoted by $ s(N) $, is defined by $ s(N) = \max \{Re \lambda:\mbox{ $\lambda$ is an eigenvalue of $N$ }\rbrace $. If the matrix $ N $ in Eq (3.8) has nonnegative off diagonal elements and is irreducible, then $ s(N) $ is a simple eigenvalue of the matrix $ N $ with a (componentwise) positive eigenvector (see, e.g., [31,Theorem A.5]).
The matrix $ N $ in Eq (3.8) is
$ \begin{equation*} N = \begin{pmatrix} ae^{-\zeta_1}-d&b\\ ce^{-\zeta_2}&-d_* \end{pmatrix}, \end{equation*} $ |
then we can easily get the following:
$ s(N) > 0 $ if and only if $ (ae^{-\zeta_1}-d)d_*+bce^{-\zeta_2} > 0 $ and $ s(N) < 0 $ if and only if $ (ae^{-\zeta_1}-d)d_*+bce^{-\zeta_2} < 0 $.
Definition 3.4. [32] A square matrix $ A = [a_{ij}] $ with non-positive off diagonal entries, i.e., $ a_{ij}\le 0 $ for all $ i\neq j $, is said to be an M-matrix if all the eigenvalues of $ A $ have a non-negative real part, or equivalently, if all its principal minors are non-negative, and $ A $ is said to be a non singular M-matrix if all the eigenvalues of $ A $ have positive real part, or, equivalently, if all its principal minors are positive.
Theorem 3.5. Suppose that $ (ae^{-\zeta_1}-d)d_*+bce^{-\zeta_2} < 0 $, then the equilibrium $ E_0 $ of system (2.2) is globally asymptotically stable.
Proof. Let $ P(t, l) $, $ M(t, k) $ be the solutions of system (2.2) with $ P(0+\theta, l) = l $, $ M(0+\theta, k) = k $ for $ \theta\in[-\tau, 0] $. Note that $ f_1(l) = l[-d+b+ae^{-\zeta_1}-b_1l] < 0 $ for $ l > 0 $ sufficiently large and $ f_2(k) = k[-d_*+ce^{-\zeta_2}-b_2k] < 0 $ for $ k > 0 $ sufficiently large. Hence we can easily conclude that all admissible solutions of system (2.2) are bounded [26,Corollary 5.2.2]. We have $ s(N) < 0 $ if and only if $ (ae^{-\zeta_1}-d)d_*+bce^{-\zeta_2} < 0 $. By the assumption $ (ae^{-\zeta_1}-d)d_*+bce^{-\zeta_2} < 0 $, we observed that it is equivalent to having $ -N $ a non singular M-matrix. Since matrix $ -N $ is a non singular M-matrix, there exists the equilibrium $ v = (v_p, v_m)\in \mathbb{R}^2, v > 0, $ such that $ Nv < 0 $, hence we get
$ \begin{equation} \begin{aligned} ae^{-\zeta_1}v_p-dv_p+bv_m < 0, \\ ce^{-\zeta_2}v_p-d_*v_m < 0. \end{aligned} \end{equation} $ | (3.9) |
Let $ P(t)\ge 0 $, $ M(t)\ge 0 $ be solutions of system (2.2). Denote $ y_p(t) = \frac{P(t)}{v_p} $ and $ y_m(t) = \frac{M(t)}{v_m} $, thus system (2.2) takes the form as
$ \begin{equation} \begin{aligned} y_p'(t) = &y_p(t)[-d-b_1y_p(t)v_p]+ae^{-\zeta_1}y_p(t-\tau_1)+\frac{bv_m}{v_p}y_m(t), \\ y_m'(t) = &y_m(t)[-d_*-b_2y_m(t)v_m]+ce^{-\zeta_2}\frac{v_p}{v_m}y_p(t-\tau_2). \end{aligned} \end{equation} $ | (3.10) |
It suffices to prove that $ (L_p, L_m): = \limsup_{t\to\infty} (y_p(t), y_m(t)) = (0, 0) $. Let $ L_p: = \limsup\lbrace{y_p(t)}\rbrace $, $ L_m: = \limsup\lbrace{y_m(t)}\rbrace $, $ \tilde{L}: = \max\lbrace{L_p, L_m}\rbrace $ and suppose that $ \tilde{L} > 0 $. From Eq (3.9), we can choose $ \varepsilon > 0 $ such that
$ \begin{equation*} \begin{aligned} \tilde{L}[-d-b_1\tilde{L}v_p+ae^{-\zeta_1}+\frac{bv_m}{v_p}]+\varepsilon[ae^{-\zeta_1}+\frac{bv_m}{v_p}] = :\gamma_p < 0, \\ \tilde{L}[-d_*-b_2\tilde{L}v_m+ce^{-\zeta_2}\frac{v_p}{v_m}]+\varepsilon[ce^{-\zeta_2}\frac{v_p}{v_m}] = :\gamma_m < 0. \end{aligned} \end{equation*} $ |
Let $ T > 0 $ be such that $ y_p(t)\le \tilde{L}+\varepsilon $, $ y_m(t)\le \tilde{L}+\varepsilon $ for all $ t > T-\tau $ and the cases of $ y_p(t) $ and $ y_m(t) $ are separated as eventually monotone and not eventually monotone. By [26,Proposition 5.4.2], if $ y_p(t) $ and $ y_m(t) $ are eventually monotone, then $ y_p(t)\to \tilde{L} $ and $ y_m(t)\to \tilde{L} $ as $ t\to \infty $ for $ t\ge T $ and we obtain
$ \begin{equation} \begin{aligned} y'_p(t)&\le y_p(t)[-d-b_1y_p(t)v_p]+ae^{-\zeta_1}(\tilde{L}+\varepsilon)+(\tilde{L}+\varepsilon)\frac{bv_m}{v_p}\to \gamma_p, \\ y'_m(t)&\le y_m(t)[-d_*-b_2y_m(t)v_m]+ce^{-\zeta_2}\frac{v_p}{v_m}(\tilde{L}+\varepsilon)\to \gamma_m \text{ as } t\to\infty. \end{aligned} \end{equation} $ | (3.11) |
Since $ \gamma_p < 0 $ and $ \gamma_m < 0 $, these imply that $ \lim_{t\to \infty} (y_p(t), y_m(t)) = -\infty $, which is impossible. By using the similar argument of Aiello and Freedman [18,Theorem 2], if $ y_p(t) $ and $ y_m(t) $ are not eventually monotone, there is a sequence $ t_n\to\infty $ such that $ y_p(t_n)\to \tilde{L}, $ $ y'_p(t_n) = 0 $ and $ y_m(t_n)\to \tilde{L}, $ $ y'_m(t_n) = 0 $. We obtain (3.11) with $ t $ replaced by $ t_n $, again a contradiction. This proves $ \lim_{t\to \infty} (y_p(t), y_m(t)) = (0, 0) $. Using Lemma 3.1, we complete the proof of Theorem 3.5.
Theorem 3.6. Suppose that $ ae^{-\zeta_1}-d > 0 $ and $ c = 0 $, then the equilibrium $ E_1 $ of system (2.2) is globally asymptotically stable.
Proof. If $ c = 0 $, the second equation of system (2.2) becomes
$ \begin{equation} M'(t) = -d_*M-b_2M^2, \end{equation} $ | (3.12) |
For the independent subsystem (3.12), it is obvious that $ \lim_{t\to \infty} M(t) = 0 $.
Then the first equation of system (2.2) becomes
$ \begin{equation} P'(t) = ae^{-\zeta_1}P(t-\tau_1)-dP(t)-b_1P^2(t). \end{equation} $ | (3.13) |
Let $ \varepsilon > 0 $ be sufficiently small and $ L > 0 $ be sufficiently large such that $ \varepsilon\le P(t)\le L $, $ t\in[-\tau, 0] $, and
$ ae^{-\zeta_1}\varepsilon-d\varepsilon-b_1\varepsilon^2 > 0, \quad ae^{-\zeta_1}L-dL-b_1L^2 < 0. $ |
Let $ P_\varepsilon(t) $ and $ P_L(t) $ be the solutions of Eq (3.13) with $ P_\varepsilon(t) = \varepsilon $ and $ P_L(t) = L $ for $ t\in[-\tau, 0] $. From the monotone properties of the equation [26], the function $ P_\varepsilon(t) $ is increasing and $ P_L(t) $ is decreasing for $ t\ge 0 $ and
$ P_\varepsilon(t)\le P(t)\le P_L(t), t\ge 0. $ |
It therefore follows that
$ \begin{equation*} \begin{aligned} \frac{ae^{-\zeta_1}-d}{b_1} = \lim\limits_{t\to\infty} P(t)\le \lim\limits_{t\to\infty} P_L(t) = \frac{ae^{-\zeta_1}-d}{b_1} \end{aligned} \end{equation*} $ |
because the only equilibrium of the equation between $ \varepsilon $ and $ L $ is $ \frac{ae^{-\zeta_1}-d}{b_1} $. Using Lemma 3.2, we complete the proof of Theorem 3.6.
Lemma 3.7. Suppose there is a positive equilibrium $ (P^*, M^*) $ of system (2.2), and that $ (ae^{-\zeta_1}-d)d_*+bce^{-\zeta_2} > 0 $ and $ c\neq 0 $. Then all solutions $ P(t, \psi_1) $, $ M(t, \psi_2) $ of system (2.2) with $ \psi_i\in X_0^+, i = 1, 2 $ satisfy $ \liminf_{t\to\infty}(P(t, \psi_1), M(t, \psi_2))\ge (P^*, M^*) $.
Proof. For $ (P^*, M^*) $ an equilibrium of system (2.2), we have
$ \begin{equation} \begin{aligned} ae^{-\zeta_1}-d+b\frac{M^*}{P^*} = b_1P^* > 0, \\ -d_*+ce^{-\zeta_2}\frac{P^*}{M^*} = b_2M^* > 0. \end{aligned} \end{equation} $ | (3.14) |
Denote $ \bar{P}(t) = \frac{P(t)}{P^*} $ and $ \bar{M}(t) = \frac{M(t)}{M^*} $ in system (2.2), and dropping the bar for simplicity, we get
$ \begin{equation} \begin{aligned} P'(t) = &P(t)[-d-b_1P(t)P^*]+ae^{-\zeta_1}P(t-\tau_1)+\frac{bM^*}{P^*}M(t), \\ M'(t) = &M(t)[-d_*-b_2M(t)M^*]+ce^{-\zeta_2}\frac{P^*}{M^*}P(t-\tau_2). \end{aligned} \end{equation} $ | (3.15) |
For the solutions $ P(t) = P(t, \psi_1) $ and $ M(t) = M(t, \psi_2) $ of Eq (3.15) with $ \psi_i\in X_0^+ $ for $ i = 1, 2 $, we first claim that $ (l_p, l_m): = \liminf_{t\to\infty}(P(t), M(t)) > (0, 0) $. Otherwise, there exist $ \delta\in(0, 1) $ and $ t_0 > \tau $ such that $ \tilde{l} = \min\lbrace{(P(t), M(t)):t\in[0, t_0]}\rbrace $ and $ \tilde{l} < \delta $. By using Eq (3.14),
$ \begin{equation*} \begin{aligned} P'(t_0)& = P(t_0)[-d-b_1P(t_0)P^*]+ae^{-\zeta_1}P(t_0-\tau_1)+\frac{bM^*}{P^*}M(t_0)\\ &\ge \tilde{l}[-d-b_1\tilde{l}P^*]+ae^{-\zeta_1}\tilde{l}+\frac{bM^*}{P^*}\tilde{l} \\ & = \tilde{l}(b_1P^*-b_1\tilde{l}P^*) = \tilde{l}b_1P^*(1-\tilde{l}) > 0, \\ M'(t_0)& = M(t_0)[-d_*-b_2M(t_0)M^*]+ce^{-\zeta_2}\frac{P^*}{M^*}P(t_0-\tau_2)\\ &\ge \tilde{l}[-d_*-b_2\tilde{l}M^*]+ce^{-\zeta_2}\frac{P^*}{M^*}\tilde{l} \\ & = \tilde{l}(b_2M^*-b_2\tilde{l}M^*) = \tilde{l}b_2M^*(1-\tilde{l}) > 0. \end{aligned} \end{equation*} $ |
But these are not possible. Since the definition of $ t_0 $, $ P'(t_0)\le 0 $ and $ M'(t_0)\le 0 $.
Next we prove that $ (l_p, l_m)\ge(1, 1) $. Choose $ \tilde{l} = \min \lbrace{l_p, l_m}\rbrace $ and suppose that $ \tilde{l} < 1 $. Let $ T > 0 $ and $ \varepsilon > 0 $ be chosen so that $ P(t)\ge \tilde{l}-\varepsilon $ and $ M(t)\ge \tilde{l}-\varepsilon $ for all $ t > T-\tau $.
$ \begin{equation*} \begin{aligned} \tilde{l}b_1P^*(1-\tilde{l})-\varepsilon[ae^{-\zeta_1}\tilde{l}+\frac{bM^*}{P^*}] = :n_p > 0, \\ \tilde{l}b_2M^*(1-\tilde{l})-\varepsilon[ce^{-\zeta_2}\frac{P^*}{M^*}] = :n_m > 0. \end{aligned} \end{equation*} $ |
By [26,Proposition 5.4.2], if $ P(t) $ and $ M(t) $ are eventually monotone, then $ P(t)\to \tilde{l} $ and $ M(t)\to \tilde{l} $ and for $ t\ge T $, we have
$ \begin{equation*} \begin{aligned} P'(t)&\ge P(t)[-d-b_1P(t)P^*]+ae^{-\zeta_1}(\tilde{l}-\varepsilon)+(\tilde{l}-\varepsilon)\frac{bM^*}{P^*}\to n_p, \\ M'(t)&\ge M(t)[-d_*-b_2M(t)M^*]+(\tilde{l}-\varepsilon)ce^{-\zeta_2}\frac{P^*}{M^*} \to n_m \text{ as } t\to\infty, \end{aligned} \end{equation*} $ |
leading to $ P(t)\to \infty $ and $ M(t)\to \infty $ as $ t\to \infty $, contradicting $ \tilde{l} < 1 $. By using the similar argument of Aiello and Freedman [18,Theorem 2], if $ P(t) $ and $ M(t) $ are not eventually monotone, there is a sequence $ t_n\to \infty $ such that $ P(t_n)\to \tilde{l}, $ $ P'(t_n) = 0 $ and $ M(t_n)\to \tilde{l}, $ $ M'(t_n) = 0 $. For $ t_n\ge T $, we obtain the above inequalities $ t_n $ instead of $ t $, which yield that $ 0 = P'(t_n)\ge n_p $ and $ 0 = M'(t_n)\ge n_m $, again contradicting $ \tilde{l} < 1 $. This proves that $ \tilde{l}\ge 1 $.
Theorem 3.8. Suppose that $ (ae^{-\zeta_1}-d)d_*+bce^{-\zeta_2} > 0 $ and $ c\neq 0 $, then the equilibrium $ E^* $ of system (2.2) is globally asymptotically stable.
Proof. For $ (P^*, M^*) $ of system (2.2), after the changes $ P(t)\mapsto \frac{P(t)}{P^*} $ and $ M(t)\mapsto \frac{M(t)}{M^*} $, consider system (3.15) with positive equilibrium $ (1, 1)\in \mathbb{R}^2 $. In view of Lemmas 3.3 and 3.7, we only need to prove that $ (L_p, L_m): = \limsup_{t\to \infty}(P(t), M(t))\le (1, 1) $ and any positive solution $ P(t) $, $ M(t) $ of Eq (3.15).
For the sake of contradiction, suppose that $ \tilde{L} = \max \lbrace{L_p, L_m}\rbrace > 1 $. Choose $ \varepsilon > 0 $ and $ t > \tau $, such that $ P(t)\le \tilde{L}+\varepsilon $ and $ M(t)\le \tilde{L}+\varepsilon $ for all $ t > T-\tau $ and
$ \begin{equation*} \begin{aligned} \tilde{L}b_1P^*(1-\tilde{L})+\varepsilon[ae^{-\zeta_1}\tilde{L}+\frac{bM^*}{P^*}] = :&N_p < 0, \\ \tilde{L}b_2M^*(1-\tilde{L})+\varepsilon[ce^{-\zeta_2}\frac{P^*}{M^*}] = :& N_m < 0. \end{aligned} \end{equation*} $ |
Separating the cases of $ P(t) $ and $ M(t) $ eventually monotone and not eventually monotone, and reasoning as in the proofs of Theorem 3.5 and Lemma 3.7, we obtain a contradiction, thus $ \tilde{L}\le 1 $. Finally we get $ \lim_{t\to \infty}(P(t), M(t)) = (P^*, M^*) $. Using Lemma 3.3, we complete the proof of Theorem 3.8.
Remark 1. Note that when $ \tau_1 = \tau_2 = 0 $, system (2.2) becomes system (1.1). Theorems 4–6 in [11] are the corresponding results of Theorems 3.5, 3.6 and 3.8 for system (2.2), respectively. Our main results not only extend the results in [11] but also generalize the related results into the stage-structured system with two delays. But the proof methods of our results are quite different to those in [11].
In this section, we numerically simulate the dynamics of system (2.2) for a range of parameters which are the same as those in [11]. In this paper, we add the values of two delays $ \tau_1 $ and $ \tau_2 $ from [10,33]. The parameters are given in Table 1.
Parameter | Ranges | Ref. | Unit | data 1 | data 2 |
$ \alpha(T) $ | $ 0.03\sim0.15^{a} $ | [14] | ind $ \cdot $ d$ ^{-1} $ $ P^{-1} $ | 0.12 | 0.15 |
$ \beta(T) $ | $ 0.065\sim0.139^{a} $ | [14] | ind $ \cdot $ d$ ^{-1} $time$ ^{-1} $ $ P^{-1} $ | 0.108 | 0.122 |
$ \gamma $ | $ 19\sim178^{a} $ | [33,34] | ind $ \cdot $ d$ ^{-1} $ $ M^{-1} $ | 100 | 170 |
$ s_1 $ | $ 0.001\sim0.3^{b} $ | [34,35] | no unit | 0.008 | 0.01 |
$ s_2 $ | $ 0.01\sim0.8^{b} $ | [34] | no unit | 0.2 | 0.8 |
$ n $ | $ 1\sim2 $ | [14] | times | 1 | 1 |
$ d_1 $ | $ 0\sim0.028^{a, b} $ | [6,14] | d$ ^{-1} $ | 0.0001 | 0.0001 |
$ d_2 $ | $ 0.0001\sim0.3^{b} $ | [34] | d$ ^{-1} $ | 0.0001 | 0.0001 |
$ d_3 $ | $ 0.004\sim0.02^{a} $ | [34,36] | d$ ^{-1} $ | 0.006 | 0.004 |
$ d_4 $ | $ 0.0001\sim0.8^{b} $ | [1] | d$ ^{-1} $ | 0.0001 | 0.0001 |
$ b_1 $ | $ 0.00001\sim0.1^{b} $ | [3,37] | d$ ^{-1} $ind$ ^{-1} $ | 0.0012 | 0.0001 |
$ b_2 $ | $ 0\sim0.1^{b} $ | d$ ^{-1} $ind$ ^{-1} $ | 0.0001 | 0.0001 | |
$ \tau_1 $ | $ 30\sim120^{b} $ | [10,33] | d | 120 | 120 |
$ \tau_2 $ | $ 60\sim300^{b} $ | [10,33] | d | 90 | 150 |
Values signatured by $ ^{a} $ are from experimental data with unit innovation and those signatured by $ ^{b} $ are estimated from references.
The left figure of Figure 2 shows that the positive equilibrium $ E^* $ of system (2.2) is globally asymptotically stable under different initial values. The left figure and right figure of Figure 2 take the parameters data 1 and data 2, respectively. Figure 2 shows that the population sizes change with respect to environmental indices but do not depend on the initial values. The population explosion occurs even though the initial values $ (P = 0, M = 2) $ are small (see the right figure of Figure 2). The numbers of two stages in the right figure of Figure 2 are larger than those in the left figure of Figure 2 because the reproduction is high while the destructions and competitions are low in the right figure of Figure 2. The trajectories of the right figure of Figure 2 finally tend towards a higher population level up to 10–15 times than the trajectories in the left figure of Figure 2 (in the corresponding Figure 3 of [11], the populations of Figure 3(b) is higher 30–50 times than Figure 3(a)) although the initial values $ (0, 2) $ are of equal values.
Based on data 2, Figure 3 illustrates how time delays affect the population dynamics. In the left figure of Figure 3, we fix the delay $ \tau_2 $ as the best fit value and increase the delay $ \tau_1\in [30,120] $. We find that the populations are slightly fallen over the longer period $ \tau_1 $ (see the left figure of Figure 3). This is because of the lack of needed temperature and resources and so the asexual reproduction period is long, and the results of the population are low. When we fix the delay $ \tau_1 $ and change the delay $ \tau_2 $ from $ 60 $ to $ 300 $, the populations are significantly decreased over the longer period $ \tau_2 $ (see the right figure of Figure 3). Overall, Figure 3 can be seen that the peaks of population abundance occur at the small $ \tau_1 $ and $ \tau_2 $ while the longer maturation periods may be responsible for the lower populations.
Figure 4 depicts the effect of temperature $ T\in [7,36] $ on the populations. Temperature is the impact factor that affects the asexual reproduction and strobilation of the jellyfish. In [11], Xie et al. presented that
$ \alpha(T) = \frac{1.9272}{T^3-30.3904T^2+294.7234T-871.29}+0.0378, $ |
$ \beta(T) = 0.1430 \text{ exp } \lbrace{-(\frac{T-16.8108}{10.5302})^2}\rbrace. $ |
In Figure 4, the numbers of polyp reach a peak at $ 12.5\; ^{\circ}C $, which correlates with the maximum budding rate of experimental data [14] and then gradually declined over the high temperature. From $ 12.5\; ^{\circ}C $ to $ 16.8\; ^{\circ}C $ is the maximum level of the number of medusae which is different from the experimental result $ 15\; ^{\circ}C $ [14]. Figure 4 reveals that an appropriate increase of temperature might cause a large increase in the number of populations but the rise of temperatures would result in the fewer populations. Comparing the corresponding Figure (4d) in [11] with the right figure of Figure 4 in this paper, we find out that we can exactly see the peak populations due to the stage structure and can exactly know the effects of temperature on the population dynamics because the temperature is considered up to $ 36\; ^{\circ}C $ in this paper.
In this paper, we propose and analyze a delayed jellyfish model with stage structure, which is an extension of ODE model studied by Xie et al. in [11]. We have investigated how the phenomena of budding and strobilation influence the population dynamics of the jellyfish population. $ \tau_1 $ stands the time needed from the stage of the young polyp to the developed polyp and $ \tau_2 $ stands the time taken from the mature polyp to ephyra (incipient medusa). We have developed the systematic analysis of the model in both theoretical and numerical ways.
We have proved the global stability of the equilibria under suitable conditions. Our results not only extend but also improve some related results of literature [11]. Our Theorems 3.5, 3.6 and 3.8 straightly extend the corresponding Theorems 4–6 in [11], respectively. Comparing the corresponding Theorems 4–6 in [11] for the ODE system (1.1) with Theorem 3.5, 3.6 and 3.8 for system (2.2), we find out that there are two extra terms $ e^{-d\tau_1} $ and $ e^{-d_*\tau_2} $ in our permanence and extinction criteria, i.e., the surviving probability of each immature population to develop into mature, which obtains due to the stage structure. From our results, we find that the jellyfish population will be extinct in the large immature mortality rate $ d, d_* $ or the long maturation $ \tau_1, \tau_2 $. Thus we may suggest that the proper increases of $ d\tau_1 $ and $ d_*\tau_2 $ have a negative effect of jellyfish population.
Biologically, our results suggest that (i) jellyfish species go extinct if the survival rate of polyp during cloning and the survival rate of the incipient medusa during strobilation are less than their death rates; (ii) polyps will continue and there is no complement from polyp to medusa if the survival rate of polyp during cloning is larger than its death rate and the temperature is not enough to strobilate; (iii) both polyp and medusa will survive in a certain ideal environment and our result converges to the positive constant when the survival rate of polyp during cloning and the survival rate of the incipient medusa during strobilation are larger than their death rates.
Besides the above systematic theoretical results, we have performed the numerical simulations to support the theoretical results. Our numerical results suggest that the positive equilibrium is globally asymptotically stable under distinctive initial values and the population sizes don't deal with the initial values but they change with respect to environmental factors. In Figures 3 and 4, our results suggest that the abundance in population occurs at the smaller periods $ \tau_1 $ and $ \tau_2 $ whereas the longer periods $ \tau_1 $ and $ \tau_2 $ will lower the peak population of polyp and medusa. In addition to the problem due to increasing $ \tau_1 $ and $ \tau_2 $, the increase of temperatures might cause the outburst of the population dynamics. If there is much higher temperature, the population rate leads to decline. Since temperature has a great impact on jellyfish population, it is interesting for one to consider the populations under the relevance to temperature. We leave this interesting problem as our future work.
We would like to take this chance to thank the editor and the anonymous referees for their very valuable comments, which led to a significant improvement of our previous versions. The authors would like to thank Dr. Zhanwen Yang for his warm help on the numeric simulations. Z. Win, B. Tian and S. Liu are supported by the Natural Science Foundation of China (NSFC) (No. 11871179, 11771374, 91646106).
All authors declare no conflicts of interest in this paper.
[1] | Albanese S, Breward N (2011) Sources of Anthropogenic Contaminants in the Urban Environment, In: Johnson CC, Author, Mapping the chemical environment of urban areas, Wiley, 116–127. |
[2] |
Albanese S, Cicchella D (2012) Legacy problems in urban geochemistry. Elements 8: 423–428. https://doi.org/10.2113/gselements.8.6.423 doi: 10.2113/gselements.8.6.423
![]() |
[3] | WHO, Air quality guidelines for particulate matter, ozone, nitrogen dioxide and sulphur dioxide—Global update 2005—Summary of risk assessment. World Health Organization, Geneva, Switzerland, 2006. |
[4] | Ali H, Khan E, Ilahi I (2019) Environmental chemistry andecotoxicology of hazardous heavy metals: environmental per-sistence, toxicity, and bioaccumulation. J Chem, 1–14. |
[5] | Liu J, Goyer RA, Waalkes MP (2007) Toxic effects of metals, In: Klaassen CD, Author, Casarett and Doull's Toxicology: The Basic Science of Poisons, 7 Eds., New York: McGraw-Hill Professional, 931–980. |
[6] |
Antoniadis V, Shaheen SM, Levizou E, et al. (2019) A critical prospective analysis of the potential toxicity of trace element regulation limits in soils worldwide: Are they protective concerning health risk assessment?—A review. Environ Int 127: 819–847. https://doi.org/10.1016/j.envint.2019.03.039 doi: 10.1016/j.envint.2019.03.039
![]() |
[7] | Fengpeng H, Zhihuan Z, Yunyang W, et al. (2009) Polycyclic aromatic hydrocarbons in soils of Beijing and Tianjin region: Vertical distribution, correlation with TOC and transport mechanism. J Environ Sci 21: 675–685. |
[8] |
Manoli E, Kouras A, Samara C (2004) Profile analysis of ambient and source emitted particle-bound polycyclic aromatic hydrocarbons from three sites in northern Greece. Chemosphere 56: 867–878. https://doi.org/10.1016/j.chemosphere.2004.03.013 doi: 10.1016/j.chemosphere.2004.03.013
![]() |
[9] | ATSDR, Toxicity of Polycyclic Aromatic Hydrocarbons (PAHs). Agency for Toxic Substances and Disease Registry (ATSDR), Atlanta GA, 2009. Available from: https://www.atsdr.cdc.gov/csem/pah/docs/pah.pdf. |
[10] | Eisler R (2000) Handbook of Chemical Risk Assessment: Health Hazards to Humans, Plants and Animals, Boca Raton: Lewis Publishers. |
[11] | USEPA, Ambient water quality criteria for polynuclear aromatic hydrocarbons. United States Environmental Protection Agency (USEPA), Washington, DC, 1980. Available from: https://www.epa.gov/sites/default/files/2019-03/documents/ambient-wqc-pah-1980.pdf. |
[12] | Sparling DW (2016) Ecotoxicology Essentials. Polycyclic Aromatic Hydrocarbons. 1 Eds., Amsterdam: Elsevier, 193–221. |
[13] | Schaefer C, Peters P, Miller RK (2015) Drugs During Pregnancy and Lactation. 3 Eds., Amsterdam: Elsevier. |
[14] | ISPRA, Impatto sugli ecosistemi e sugli esseri viventi delle sostanze sintetiche utilizzate nella profilassi anti-zanzara. Istituto Superiore per la Protezione e la Ricerca Ambientale (ISPRA), 2015. Available from: https://www.isprambiente.gov.it/files/pubblicazioni/quaderni/ambiente-societa/Quad_AS_10_15_ProfilassiAntiZanzare.pdf. |
[15] |
Lushchak VI, Matviishyn TM, Husak VV, et al. (2018) Pesticide toxicity: A mechanistic approach. EXCLI J 1: 1101–1136. https://doi.org/10.17179/excli2018-1710 doi: 10.17179/excli2018-1710
![]() |
[16] |
Balmer JE, Morris AD, Hung H, et al. (2019) Levels and trends of current-use pesticides (CUPs) in the arctic: an updated review, 2010–2018. Emerg Contam 5: 70–88. https://doi.org/10.1016/j.emcon.2019.02.002 doi: 10.1016/j.emcon.2019.02.002
![]() |
[17] | Sparling DW (2016) Ecotoxicology Essentials. Organochlorine Pesticides. 1 Eds., Amsterdam: Elsevier, 69–107. |
[18] |
Kim KH, Kabir E, Jahan SA (2017) Exposure to pesticides and the associated human health effects. Sci Total Environ 575: 525–535. https://doi.org/10.1016/j.scitotenv.2016.09.009 doi: 10.1016/j.scitotenv.2016.09.009
![]() |
[19] | Stockholm Convention on Persistent Organic Pollutants, Worldwide found, Stockholm convention "new POPs": screening additional POPs candidates, 2005. Available from: http://chm.pops.int/TheConvention/ThePOPs/TheNewPOPs/tabid/2511/Default.aspx. |
[20] | Stockholm Convention on Persistent Organic Pollutants, 2011. Available from: http://chm.pops.int/. |
[21] | IARC, Monographs evaluate DDT, lindane, and 2, 4-D. Press Release Number 236. International Agency for Research on Cancer (IARC), 2015. Available from: https://www.iarc.who.int/wp-content/uploads/2018/07/pr236_E.pdf. |
[22] |
Senior K, Mazza A (2004) Italian "Triangle of death" linked to waste crisis. Lancet Oncol 5: 525–527. https://doi.org/10.1016/s1470-2045(04)01561-x doi: 10.1016/s1470-2045(04)01561-x
![]() |
[23] | Flora A (2015) La Terra dei Fuochi: ambiente e politica industriale nel Mezzogiorno. Riv Econ Del Mezzogiorno Trimest Svimez 1–2: 89–122. |
[24] |
Albanese S, De Vivo B, Lima A, et al. (2007) Geochemical background and baseline values of toxic elements in stream sediments of Campania region (Italy). J Geochem Explor 93: 21–34. https://doi.org/10.1016/j.gexplo.2006.07.006 doi: 10.1016/j.gexplo.2006.07.006
![]() |
[25] | Albanese S, De Luca ML, De Vivo B, et al. (2008) Relationships between heavy metals distribution and cancer mortality rates in the Campania Region, Italy, In: De Vivo B, Belkin HE, Lima A, Authors, Environmental Geochemistry: Site Characterization, Data Analysis and Case Histories, Amsterdam: Elsevier, 391–404. |
[26] | Albanese S, Cicchella D, De Vivo B, et al. (2011) Advancements in urban geochemical mapping of the Naples metropolitan area: color composite maps and results from an urban brownfield site, In: Johnson CC, Demetriades A, Locutura J, Authors, Mapping The Chemical Environment of Urban Areas, UK: John Wiley Publisher, 410–423. |
[27] |
Bove M, Ayuso RA, De Vivo B, et al. (2011) Geochemical and isotopic study of soils and waters from an Italian contaminated site: Agro Aversano (Campania). J Geochem Explor 109: 38–50. https://doi.org/10.1016/j.gexplo.2010.09.013 doi: 10.1016/j.gexplo.2010.09.013
![]() |
[28] |
Catani V, Zuzolo D, Esposito L, et al. (2020) A New Approach for Aquifer Vulnerability Assessment: the Case Study of Campania Plain. Water Resour Manage 34: 819–834. https://doi.org/10.1007/s11269-019-02476-5 doi: 10.1007/s11269-019-02476-5
![]() |
[29] |
Cicchella D, De Vivo B, Lima A, et al. (2008) Heavy metal pollution and Pb isotopes in urbansoils of Napoli, Italy. Geochem Explor Environ Anal 8: 103–112. https://doi.org/10.1144/1467-7873/07-148 doi: 10.1144/1467-7873/07-148
![]() |
[30] | De Vivo B, Lima A, Albanese S, et al. (2006) Atlante geochimico-ambientale dei suoli dell'area urbana e della provincia di Napoli, Roma: Aracne Editrice. |
[31] | De Vivo B, Lima A, Albanese S, et al. (2016) Atlante geochimico-ambientale dei suoli della Campania, Roma: Aracne Editrice. |
[32] | De Vivo B, Albanese S, Lima A, et al. (2021) Composti Organici Persistenti: Idrocarburi Policiclici Aromatici, Policlorobifenili, Pesticidi, Monitoraggio Geochimico-Ambientale dei suoli della Regione Campania, Roma: Aracne Editrice. |
[33] | De Vivo B, Cicchella D, Lima A, et al. (2021) Elementi Potenzialmente Tossici e loro Biodisponibilità, Elementi Maggiori e in Traccia; distribuzione in suoli superficiali e profondi, Monitoraggio Geochimico-Ambientale dei suoli della Regione Campania, Roma: Aracne Editrice. |
[34] | De Vivo B, Cicchella D, Albanese S, et al. (2022) Idrocarburi Policiclici Aromatici (IPA), Policlorobifenili (PCB), Pesticidi (OCP), Eteri di Polibromobifenili (PBDE), Elementi Potenzialmente Tossici (EPT), Monitoraggio geochimico-ambientale della matrice aria della Regione Campania. Il Piano Campania Trasparente, Roma: Aracne Editrice. |
[35] |
Grezzi G, Ayuso RA, De Vivo B, et al. (2011) Lead isotopes in soils and ground waters as tracers of the impact of human activities on the surface environment: the Domizio-flegreo littoral (Italy) case study. J Geochem Explor 109: 51–58. https://doi.org/10.1016/j.gexplo.2010.09.012 doi: 10.1016/j.gexplo.2010.09.012
![]() |
[36] | Lima A, Giaccio L, Cicchella D, et al. (2012) Atlante geochimico ambientale del S.I.N-Litorale Domizio flegreo e Agro aversano, Roma: Aracne editrice. |
[37] |
Minolfi G, Albanese S, Lima A, et al. (2018) Human health risk assessment in Avellino-Salerno metropolitan areas, Campania Region, Italy. J Geochem Explor 195: 97–109. https://doi.org/10.1016/j.gexplo.2017.12.011 doi: 10.1016/j.gexplo.2017.12.011
![]() |
[38] |
Minolfi G, Petrik A, Albanese S, et al. (2019) The distribution of Pb, Cu and Zn in topsoil of the Campanian Region, Italy. Geochem: Explor Environ Anal 19: 205–215. https://doi.org/10.1144/geochem2017-074 doi: 10.1144/geochem2017-074
![]() |
[39] |
Petrik A, Albanese S, Lima A, et al. (2018) The spatial pattern of beryllium and its possible origin using compositional data analysis on a high-density topsoil data set from the Campania Region (Italy). Appl Geochem 91: 162–173. https://doi.org/10.1016/j.apgeochem.2018.02.008 doi: 10.1016/j.apgeochem.2018.02.008
![]() |
[40] |
Petrik A, Thiombane M, Albanese S, et al. (2018) Source patterns of Zn, Pb, Cr and Ni potentially toxic elements (PTEs) through a compositional discrimination analysis: A case study on the Campanian topsoil data. Geoderma 331: 87–99. https://doi.org/10.1016/j.geoderma.2018.06.019 doi: 10.1016/j.geoderma.2018.06.019
![]() |
[41] |
Petrik A, Albanese S, Lima A, et al. (2018) Spatial pattern recognition of arsenic in topsoil using high-density regional data. Geochem Explor Environ Anal 18: 319–330. https://doi.org/10.1144/geochem2017-060 doi: 10.1144/geochem2017-060
![]() |
[42] |
Petrik A, Albanese S, Lima A, et al. (2018) Spatial pattern analysis of Ni and its concentrations in topsoils in the Campania region (Italy). J Geochem Explor 195: 130–142. https://doi.org/10.1016/j.gexplo.2017.09.009 doi: 10.1016/j.gexplo.2017.09.009
![]() |
[43] |
Petrik A, Thiombane M, Lima A, et al. (2018) Soil Contamination Compositional Index: a new approach to quantify contamination demonstrated by assessing compositional source patterns of potentially toxic elements in the Campania Region (Italy). Appl Geochem 96: 264–276. https://doi.org/10.1016/j.apgeochem.2018.07.014 doi: 10.1016/j.apgeochem.2018.07.014
![]() |
[44] |
Qu C, Albanese S, Chen W, et al. (2016) The status of organochlorine pesticide contamination in the soils of the Campanian Plain, southern Italy, and correlations with soil properties and cancer risk. Environ Pollut 216: 500–511. https://doi.org/10.1016/j.envpol.2016.05.089 doi: 10.1016/j.envpol.2016.05.089
![]() |
[45] |
Qu C, Albanese S, Lima A, et al. (2017) Residues of hexachlorobenzene and chlorinated cyclodiene pesticides in the soils of the Campanian Plain, southern Italy. Environ Pollut 231: 1497–1506. https://doi.org/10.1016/j.envpol.2017.08.100 doi: 10.1016/j.envpol.2017.08.100
![]() |
[46] |
Qu C, Albanese S, Lima A, et al. (2019) The occurrence of OCPs, PCBs, and PAHs in the soil, air, and bulk deposition of the Naples metropolitan area, southern Italy: Implications for sources and environmental processes. Environ Int 124: 89–97. https://doi.org/10.1016/j.envint.2018.12.031 doi: 10.1016/j.envint.2018.12.031
![]() |
[47] |
Qu C, Albanese S, Lima A, et al. (2018) Polycyclic aromatic hydrocarbons in the sediments of the Gulfs of Naples and Salerno, Southern Italy: status, sources and ecological risk. Ecotoxicol Environ Saf 161: 156–163. https://doi.org/10.1016/j.ecoenv.2018.05.077 doi: 10.1016/j.ecoenv.2018.05.077
![]() |
[48] |
Qu C, De Vivo B, Albanese S, et al. (2021) High spatial resolution measurements of passive-sampler derived air concentrations of persistent organic pollutants in the Campania region, Italy: Implications for source identification and risk analysis. Environ Pollut 286: 117248. https://doi.org/10.1016/j.envpol.2021.117248 doi: 10.1016/j.envpol.2021.117248
![]() |
[49] | Qu C, Doherty AL, Xing X, et al. (2018) Polyurethane foam-based passive air samplers in monitoring persistent organic pollutants: Theory and application, In: De Vivo B, Belkin HE, Lima A, Authors, Environmental Geochemistry—Site Characterization, Data Analysis and Case Histories, Elsevier. |
[50] |
Qu C, Sun Y, Albanese S, et al. (2018) Organochlorine pesticides in sediments from Gulfs of Naples and Salerno, Southern Italy. J Geochem Explor 195: 87–96. https://doi.org/10.1016/j.gexplo.2017.12.010 doi: 10.1016/j.gexplo.2017.12.010
![]() |
[51] |
Rezza C, Albanese S, Ayuso R, et al. (2018) Geochemical and Pb isotopic characterization of soil, groundwater, human hair, and corn samples from the Domizio Flegreo and Agro Aversano area (Campania region, Italy). J Geochem Explor 184: 318–332. https://doi.org/10.1016/j.gexplo.2017.01.007 doi: 10.1016/j.gexplo.2017.01.007
![]() |
[52] |
Rezza C, Petrik A, Albanese S, et al. (2018) Mo, Sn and W patterns in topsoils of the Campania Region, Italy. Geochem Explor Environ Anal 18: 331–342. https://doi.org/10.1144/geochem2017-061 doi: 10.1144/geochem2017-061
![]() |
[53] |
Thiombane M, Albanese S, Di Bonito M, et al. (2019) Source patterns and contamination level of polycyclic aromatic hydrocarbons (PAHs) in urban and rural areas of Southern Italian soils. Environ Geochem Health 41: 507–528. https://doi.org/10.1007/s10653-018-0147-3 doi: 10.1007/s10653-018-0147-3
![]() |
[54] |
Thiombane M, Martín-Fernández JA, Albanese S, et al. (2018) Exploratory analysis of multielement geochemical patterns in soil from the Sarno River Basin (Campania region, southern Italy) through Compositional Data Analysis (CODA). J Geochem Explor 195: 110–120. https://doi.org/10.1016/j.gexplo.2018.03.010 doi: 10.1016/j.gexplo.2018.03.010
![]() |
[55] |
Thiombane M, Petrik A, Di Bonito M, et al. (2018) Status, sources and contamination levels of organochlorine pesticide residues in urban and agricultural areas: a preliminary review in central–southern Italian soils. Environ Sci Pollut Res 25: 26361–26382. https://doi.org/10.1007/s11356-018-2688-5 doi: 10.1007/s11356-018-2688-5
![]() |
[56] |
Zuzolo D, Cicchella D, Albanese S, et al. (2018) Exploring uni-element geochemical data under a compositional perspective. Appl Geochemistry 91: 174–184. https://doi.org/10.1016/j.apgeochem.2017.10.003 doi: 10.1016/j.apgeochem.2017.10.003
![]() |
[57] |
Zuzolo D, Cicchella D, Doherty AL, et al. (2018) The distribution of precious metals (Au, Ag, Pt, and Pd) in the soils of the Campania Region (Italy). J Geochem Explor 192: 33–44. https://doi.org/10.1016/j.gexplo.2018.03.009 doi: 10.1016/j.gexplo.2018.03.009
![]() |
[58] |
Zuzolo D, Cicchella D, Lima A, et al. (2020) Potentially toxic elements in soils of Campania region (Southern Italy): Combining raw and compositional data. J Geochem Explor 213: 106524. https://doi.org/10.1016/j.gexplo.2020.106524 doi: 10.1016/j.gexplo.2020.106524
![]() |
[59] |
Albanese S, Taiani MV, De Vivo B, et al. (2013) An environmental epidemiological study based on the stream sediment geochemistry of the Salerno province (Campania region, Southern Italy). J Geochem Explor 131: 59–66. https://doi.org/10.1016/j.gexplo.2013.04.002 doi: 10.1016/j.gexplo.2013.04.002
![]() |
[60] |
Giaccio L, Cicchella D, De Vivo B, et al. (2012) Does heavy metals pollution affects semen quality in men? A case of study in the metropolitan area of Naples (Italy). J Geochem Explor 112: 218–225. https://doi.org/10.1016/j.gexplo.2011.08.009 doi: 10.1016/j.gexplo.2011.08.009
![]() |
[61] |
Albanese S, Fontaine B, Chen W, et al. (2015) Polycyclic aromatic hydrocarbons in the soils of a densely populated region and associated human health risks: the Campania Plain (Southern Italy) case study. Environ Geochem Health 37: 1–20. https://doi.org/10.1007/s10653-014-9626-3 doi: 10.1007/s10653-014-9626-3
![]() |
[62] |
De Vivo B, Rolandi G, Gans PB, et al. (2001) New constraints on the pyroclastic eruptive history of the Campanian volcanic Plain (Italy). Mineral Petrol 73: 47–65. https://doi.org/10.1007/s007100170010 doi: 10.1007/s007100170010
![]() |
[63] | di Gennaro A (2002) I sistemi di terre della Campania, Firenze: Risorsa srl Selca. |
[64] | Budetta P, Celico P, Corniello A, et al. (1994) Carta idrogeologica della Campania 1/200.000 e relativa memoria illustrativa, Atti IV Geoengineering International Congress: Soil and Groundwater Protection, Geda, 565–586. |
[65] | Celico P, de Paola P (1992) La falda dell'area napoletana: ipotesi sui meccanismi naturali di protezione e sulle modalità di inquinamento. Gruppo Scient. It. Studi e Ricerche. Atti Giornate di studio "Acque per uso potabile-Proposte per la tutela ed il controllo della qualità", 387–412. |
[66] | Celico P, de Gennaro M, Esposito L, et al. (1994) La falda ad oriente della città di Napoli: idrodinamica e qualità delle acque. Geol Rom 30: 653–660. |
[67] | Celico P, Esposito L, Guadagno FM (1997) Sulla qualità delle acque sotteranee nell'acquifero del settore orientale della Piana Campana. Geologia Tecnica ed Ambientale 4: 17–27. |
[68] | Corniello A, Ducci D, Napolitano P (1997) Comparison between parametric methods to evaluate aquifer pollution vulnerability using a GIS: an example in the "Piana Campana", southern Italy, Engineering Geology and the Environment, Rotterdam: Balkema, 1721–1726. |
[69] | Corniello A, Ducci D (2009) Possible sources of nitrate in groundwater of Acerra area (Piana Campana). Eng Hydro Environ Geol 12: 155–164. |
[70] | D'Alisa G, Burgalassi D, Healy H, Walter M, (2010) Conflict in Campania: Waste emergency or crisis of democracy. Ecol Econ 70: 239–249. |
[71] | Salminen R, Tarvainen T, Demetriades A, et al. (1998) FOREGS Geochemical Mapping Field Manual. Geological Survey of Finland, Espoo Guide 47. Available from: http://www.gtk.fi/foregs/eochem/fieldmanan.pdf. |
[72] | Legislative Decree 152/2006 Decreto Legislativo 3 aprile, Norme in materia ambientale. Gazzetta Ufficiale della Repubblica Italiana, 2006. Available from: https://www.gazzettaufficiale.it/dettaglio/codici/materiaAmbientale. |
[73] | Ministerial Decree 46/2019 Decreto Ministeriale 1 marzo, Regolamento relativo agli interventi di bonifica, di ripristino ambientale e di messa in sicurezza, d'emergenza, operativa e permanente, delle aree destinate alla produzione agricola e all'allevamento, ai sensi dell'articolo 241 del D.Lgs 152/2006. Gazzetta Ufficiale della Repubblica Italiana, 2019. Available from: https://www.gazzettaufficiale.it/eli/id/2019/06/07/19G00052/sg. |
[74] | Cheng Q (1994) Multifractal modelling and spatial analysis with GIS: Gold potential estimation in the Mitchell-Sulphurets area. Northwestern British Columbia. Unpublished PhD thesis. University of Ottawa, Ottawa, 268. |
[75] |
Cheng Q (1999) Spatial and scaling modelling for geochemical anomaly separation. J Geochem Explor 65: 175–194. https://doi.org/10.1016/S0375-6742(99)00028-X doi: 10.1016/S0375-6742(99)00028-X
![]() |
[76] |
Cheng Q, Agterberg FP, Ballantyne SB (1994) The separation of geochemical anomalies from background by fractal methods. J Geochem Explor 51: 109–130. https://doi.org/10.1016/0375-6742(94)90013-2 doi: 10.1016/0375-6742(94)90013-2
![]() |
[77] |
Cheng Q, Agteberg FP, Bonham-Carter GF (1996) A spatial analysis method for geochemical anomaly separation. J Geochem Explor 56: 183–195. https://doi.org/10.1016/S0375-6742(96)00035-0 doi: 10.1016/S0375-6742(96)00035-0
![]() |
[78] | Cheng Q, Xu Y, Grunsky E (1999) Integrated spatial and spectrum analysis for geochemical anomaly separation, Proceedings of the International Association for Mathematical Geology Meeting, Trondheim (Norway), 87–92. |
[79] |
Cheng, Q, Xu Y, Grunsky E (2000) Integrated spatial and spectrum method for geochemical anomaly separation. Nat Resour Res 9: 43–56. https://doi.org/10.1023/A:1010109829861 doi: 10.1023/A:1010109829861
![]() |
[80] | Cheng Q, Bonham-Carter GF, Raines GL (2001) GeoDAS: A new GIS system for spatial analysis of geochemical data sets for mineral exploration and environmental assessment. 20th Int Geochem Explor Symposium, 42–43. |
[81] |
Lima A, De Vivo B, Cicchella D, et al. (2003) Multifractal IDW interpolation and fractal filtering method in environmental studies: an application on regional stream sediments of Campania Region (Italy). Appl Geochem 18: 1853–1865. https://doi.org/10.1016/S0883-2927(03)00083-0 doi: 10.1016/S0883-2927(03)00083-0
![]() |
[82] | Cicchella D, De Vivo B, Lima A (2005) Background and baseline concentration values of harmful elements in the volcanic soils of metropolitan and Provincial areas of Napoli (Italy). Geochem Explor Environ Anal 5: 1–12. |
[83] |
Zuo R, Wang J (2019) ArcFractal: An ArcGIS Add-In for Processing Geoscience Data Using Fractal/Multifractal Models. Nat Resour Res 29: 3–12. https://doi.org/10.1007/s11053-019-09513-5 doi: 10.1007/s11053-019-09513-5
![]() |
[84] |
Zhengle X, Zhigao S, Zhang H, et al. (2014) Contamination assessment of arsenic and heavy metals in a typical abandoned estuary wetland-a case study of the Yellow River Delta Nature Reserve. Environ Monit Assess 186: 7211–7232. https://doi.org/10.1007/s10661-014-3922-3 doi: 10.1007/s10661-014-3922-3
![]() |
[85] |
Golia EE, Dimirkou A, Floras SA (2015) Spatial monitoring of arsenic and heavy metals in the Almyros area, Central Greece. Statistical approach for assessing the sources of contamination. Environ Monit Assess 187: 399–412. https://doi.org/10.1007/s10661-015-4624-1 doi: 10.1007/s10661-015-4624-1
![]() |
[86] |
Bourliva A, Christoforidis C, Papadopoulou L, et al. (2017) Characterization, heavy metal content and health risk assessment of urban road dusts from the historic center of the city of Thessaloniki, Greece. Environ Geochem Health 39: 611–634. https://doi.org/10.1007/s10653-016-9836-y doi: 10.1007/s10653-016-9836-y
![]() |
[87] | Field A (2009) Discovering Statistics Using SPSS, 3 Eds., London: Sage Publications Ltd. |
[88] |
Jolliffe IT (1972) Discarding variables in a principal component analysis, Ⅰ: Artificial data. J R Stat Soc Appl Stat Ser C 21: 160–173. https://doi.org/10.2307/2346488 doi: 10.2307/2346488
![]() |
[89] | Jolliffe IT (1986) Principal component analysis, New York: Springer. |
[90] |
Tobiszewski M, Namiesnik J (2011) PAH diagnostic ratios for the identification of pollution emission sources. Environ Pollut 162: 110–119. https://doi.org/10.1016/J.ENVPOL.2011.10.025 doi: 10.1016/J.ENVPOL.2011.10.025
![]() |
[91] |
Mostert MMR, Ayoko GA, Kokot S (2010) Application of chemometrics to analysis of soil pollutants. Trends Anal Chem 29: 430–435. https://doi.org/10.1016/j.trac.2010.02.009 doi: 10.1016/j.trac.2010.02.009
![]() |
[92] |
Zhang W, Zhang S, Wan C, et al. (2008) Source diagnostics of polycyclic aromatic hydrocarbons in urban road runoff, dust, rain and canopy throughfall. Environ Pollut 153: 594–601. https://doi.org/10.1016/j.envpol.2007.09.004 doi: 10.1016/j.envpol.2007.09.004
![]() |
[93] |
Pies C, Hoffmann B, Petrowsky J, et al. (2008) Characterization and source identification of polycyclic aromatic hydrocarbons (PAHs) in river bank soils. Chemosphere 72: 1594–1601. https://doi.org/10.1016/j.chemosphere.2008.04.021 doi: 10.1016/j.chemosphere.2008.04.021
![]() |
[94] |
Akyüz M, Çabuk H (2010) Gas and particle partitioning and seasonal variation of polycyclic aromatic hydrocarbons in the atmosphere of Zonguldak, Turkey. Sci Total Environ 408: 5550–5558. https://doi.org/10.1016/j.scitotenv.2010.07.063 doi: 10.1016/j.scitotenv.2010.07.063
![]() |
[95] |
De La Torre-Roche RJ, Lee W-Y, Campos-Díaz SI (2009) Soil-borne polycyclic aromatic hydrocarbons in El Paso, Texas: analysis of a potential problem in the United States/Mexico border region. J Hazard Mater 163: 946–958. https://doi.org/10.1016/j.jhazmat.2008.07.089 doi: 10.1016/j.jhazmat.2008.07.089
![]() |
[96] |
Yunker MB, Macdonald RW, Vingarzan R, et al. (2002) PAHs in the Fraser River basin: a critical appraisal of PAH ratios as indicators of PAH source and composition. Org Geochem 33: 489–515. https://doi.org/10.1016/S0146-6380(02)00002-5 doi: 10.1016/S0146-6380(02)00002-5
![]() |
[97] |
Katsoyiannis A, Terzi E, Cai QY (2007) On the use of PAH molecular diagnostic ratios in sewage sludge for the understanding of the PAH sources. Is this use appropriate? Chemosphere 69: 1337–1339. https://doi.org/10.1016/j.chemosphere.2007.05.084 doi: 10.1016/j.chemosphere.2007.05.084
![]() |
[98] |
Jiang YF, Wang XT, Jia Y, et al. (2009) Occurrence, distribution and possible sources of organochlorine pesticides in agricultural soil of Shanghai, China. J Hazard Mater 170: 989–997. https://doi.org/10.1016/j.jhazmat.2009.05.082 doi: 10.1016/j.jhazmat.2009.05.082
![]() |
[99] |
Qiu X, Zhu T, Yao B, et al. (2005) Contribution of dicofol to the current DDT pollution in China. Environ Sci Technol 39: 4385–4390. https://doi.org/10.1021/es050342a doi: 10.1021/es050342a
![]() |
[100] |
Iwata H, Tanabe S, Ueda K, et al. (1995) Persistent organochlorine residues in Air, Water, Sediments, and Soils from the Lake Baikai Region, Russia. Environ Sci Technol 29: 792–801. https://doi.org/10.1021/es00003a030 doi: 10.1021/es00003a030
![]() |
[101] |
Zhang ZL, Huang J, Yu G, et al. (2004) Occurrence of PAHs, PCBs and organochlorine pesticides in Tonghui River of Beijing, China. Environ Pollut 130: 249–261. https://doi.org/10.1016/j.envpol.2003.12.002 doi: 10.1016/j.envpol.2003.12.002
![]() |
[102] |
Zhang A, Liu W, Yuan H, et al. (2011) Spatial distribution of hexachlorocyclohexanes in agricultural soils in Zhejiang province, China, and correlations with elevation and temperature. Environ Sci Technol 45: 6303–6308. https://doi.org/10.1021/es200488n doi: 10.1021/es200488n
![]() |
[103] |
Bidleman TF, Jantunen LLM, Helm PA, et al. (2000) Chlordane enantiomers and temporal trends of chlordane isomers in Arctic air. Environ Sci Technol 36: 539–544. https://doi.org/10.1021/es011142b doi: 10.1021/es011142b
![]() |
[104] | WHO, Environmental Health Criteria 40: Endosulfan. World Health Organization, Geneva, 1984. Available from: https://apps.who.int/iris/bitstream/handle/10665/39390/WHO_EHC_40.pdf?sequence=1&isAllowed=y. |
[105] | Albanese S, Guarino A, Pizzolante A, et al. (2022) The use of natural geochemical background values for the definition of local environmental guidelines: the case study of the Vesuvian plain, In: Baldi D, Uricchio F, Authors, Le bonifiche ambientali nell'ambito della transizione ecologica, Società Italiana di Geologia Ambientale (SIGEA) and Consiglio Nazionale delle Ricerche (CNR), 15–25. |
[106] |
Aruta A, Albanese S, Daniele L, et al. (2022). A new approach to assess the degree of contamination and determine sources and risks related to PTEs in an urban environment: the case study of Santiago (Chile). Environ Geochem Health. https://doi.org/10.1007/s10653-021-01185-6 doi: 10.1007/s10653-021-01185-6
![]() |
[107] |
Stančić Z, Fiket Ž, Vuger A (2022) Tin and Antimony as Soil Pollutants along Railway Lines-A Case Study from North-Western Croatia. Environments 9: 10. https://doi.org/10.3390/environments9010010 doi: 10.3390/environments9010010
![]() |
[108] |
Wuana RA, Okieimen FE (2011) Heavy metals in contaminated soils: a review of sources, chemistry, risks and best available strategies for remediation. Isrn Ecology 2011: 2090–4614. https://doi.org/10.5402/2011/402647 doi: 10.5402/2011/402647
![]() |
[109] |
Qiu YW, Zhang G, Liu GQ, et al. (2009) Polycyclic aromatic hydrocarbons (PAHs) in the water column and sediment core of Deep Bay, South China. Estuar Coast Shelf Sci 83: 60–66. https://doi.org/10.1016/j.ecss.2009.03.018 doi: 10.1016/j.ecss.2009.03.018
![]() |
[110] | ATSDR, Toxicological Profile for DDT, DDE, and DDD. Dept Health Human Services. Agency for Toxic Substances and Disease Registry (ATSDR), Atlanta GA, 2002. Available from: https://www.atsdr.cdc.gov/toxprofiles/tp35.pdf. |
1. | Zin Thu Win, Boping Tian, Population dynamical behaviors of jellyfish model with random perturbation, 2023, 28, 1531-3492, 2994, 10.3934/dcdsb.2022201 | |
2. | Da Song, Wentao Fu, Meng Fan, Kun Li, Ecological effect of seasonally changing temperature on the life cycle of Aurelia aurita, 2025, 501, 03043800, 111014, 10.1016/j.ecolmodel.2024.111014 |
Parameter | Ranges | Ref. | Unit | data 1 | data 2 |
$ \alpha(T) $ | $ 0.03\sim0.15^{a} $ | [14] | ind $ \cdot $ d$ ^{-1} $ $ P^{-1} $ | 0.12 | 0.15 |
$ \beta(T) $ | $ 0.065\sim0.139^{a} $ | [14] | ind $ \cdot $ d$ ^{-1} $time$ ^{-1} $ $ P^{-1} $ | 0.108 | 0.122 |
$ \gamma $ | $ 19\sim178^{a} $ | [33,34] | ind $ \cdot $ d$ ^{-1} $ $ M^{-1} $ | 100 | 170 |
$ s_1 $ | $ 0.001\sim0.3^{b} $ | [34,35] | no unit | 0.008 | 0.01 |
$ s_2 $ | $ 0.01\sim0.8^{b} $ | [34] | no unit | 0.2 | 0.8 |
$ n $ | $ 1\sim2 $ | [14] | times | 1 | 1 |
$ d_1 $ | $ 0\sim0.028^{a, b} $ | [6,14] | d$ ^{-1} $ | 0.0001 | 0.0001 |
$ d_2 $ | $ 0.0001\sim0.3^{b} $ | [34] | d$ ^{-1} $ | 0.0001 | 0.0001 |
$ d_3 $ | $ 0.004\sim0.02^{a} $ | [34,36] | d$ ^{-1} $ | 0.006 | 0.004 |
$ d_4 $ | $ 0.0001\sim0.8^{b} $ | [1] | d$ ^{-1} $ | 0.0001 | 0.0001 |
$ b_1 $ | $ 0.00001\sim0.1^{b} $ | [3,37] | d$ ^{-1} $ind$ ^{-1} $ | 0.0012 | 0.0001 |
$ b_2 $ | $ 0\sim0.1^{b} $ | d$ ^{-1} $ind$ ^{-1} $ | 0.0001 | 0.0001 | |
$ \tau_1 $ | $ 30\sim120^{b} $ | [10,33] | d | 120 | 120 |
$ \tau_2 $ | $ 60\sim300^{b} $ | [10,33] | d | 90 | 150 |
Parameter | Ranges | Ref. | Unit | data 1 | data 2 |
$ \alpha(T) $ | $ 0.03\sim0.15^{a} $ | [14] | ind $ \cdot $ d$ ^{-1} $ $ P^{-1} $ | 0.12 | 0.15 |
$ \beta(T) $ | $ 0.065\sim0.139^{a} $ | [14] | ind $ \cdot $ d$ ^{-1} $time$ ^{-1} $ $ P^{-1} $ | 0.108 | 0.122 |
$ \gamma $ | $ 19\sim178^{a} $ | [33,34] | ind $ \cdot $ d$ ^{-1} $ $ M^{-1} $ | 100 | 170 |
$ s_1 $ | $ 0.001\sim0.3^{b} $ | [34,35] | no unit | 0.008 | 0.01 |
$ s_2 $ | $ 0.01\sim0.8^{b} $ | [34] | no unit | 0.2 | 0.8 |
$ n $ | $ 1\sim2 $ | [14] | times | 1 | 1 |
$ d_1 $ | $ 0\sim0.028^{a, b} $ | [6,14] | d$ ^{-1} $ | 0.0001 | 0.0001 |
$ d_2 $ | $ 0.0001\sim0.3^{b} $ | [34] | d$ ^{-1} $ | 0.0001 | 0.0001 |
$ d_3 $ | $ 0.004\sim0.02^{a} $ | [34,36] | d$ ^{-1} $ | 0.006 | 0.004 |
$ d_4 $ | $ 0.0001\sim0.8^{b} $ | [1] | d$ ^{-1} $ | 0.0001 | 0.0001 |
$ b_1 $ | $ 0.00001\sim0.1^{b} $ | [3,37] | d$ ^{-1} $ind$ ^{-1} $ | 0.0012 | 0.0001 |
$ b_2 $ | $ 0\sim0.1^{b} $ | d$ ^{-1} $ind$ ^{-1} $ | 0.0001 | 0.0001 | |
$ \tau_1 $ | $ 30\sim120^{b} $ | [10,33] | d | 120 | 120 |
$ \tau_2 $ | $ 60\sim300^{b} $ | [10,33] | d | 90 | 150 |