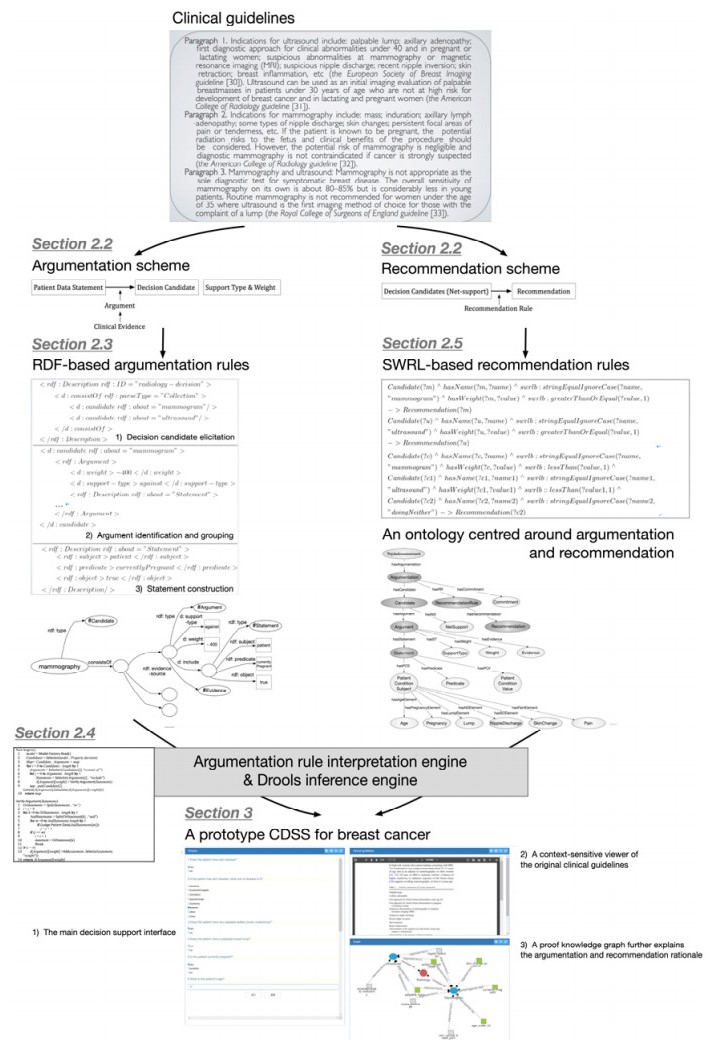
In clinical decision support, argumentation plays a key role while alternative reasons may be available to explain a given set of signs and symptoms, or alternative plans to treat a diagnosed disease. In literature, this key notion usually has closed boundary across approaches and lacks of openness and interoperability in Clinical Decision Support Systems (CDSSs) been built. In this paper, we propose a systematic approach for the representation of argumentation, their interpretation towards recommendation, and finally explanation in clinical decision support. A generic argumentation and recommendation scheme lays the foundation of the approach. On the basis of this, argumentation rules are represented using Resource Description Framework (RDF) for clinical guidelines, a rule engine developed for their interpretation, and recommendation rules represented using Semantic Web Rule Language (SWRL). A pair of proof knowledge graphs are made available in an integrated clinical decision environment to explain the argumentation and recommendation rationale, so that decision makers are informed of not just what are recommended but also why. A case study of triple assessment, a common procedure in the National Health Service of UK for women suspected of breast cancer, is used to demonstrate the feasibility of the approach. In conducting hypothesis testing, we evaluate the metrics of accuracy, variation, adherence, time, satisfaction, confidence, learning, and integration of the prototype CDSS developed for the case study in comparison with a conventional CDSS and also human clinicians without CDSS. The results are presented and discussed.
Citation: Liang Xiao, Hao Zhou, John Fox. Towards a systematic approach for argumentation, recommendation, and explanation in clinical decision support[J]. Mathematical Biosciences and Engineering, 2022, 19(10): 10445-10473. doi: 10.3934/mbe.2022489
[1] | Bo An . Construction and application of Chinese breast cancer knowledge graph based on multi-source heterogeneous data. Mathematical Biosciences and Engineering, 2023, 20(4): 6776-6799. doi: 10.3934/mbe.2023292 |
[2] | Colella Ylenia, De Lauri Chiara, Improta Giovanni, Rossano Lucia, Vecchione Donatella, Spinosa Tiziana, Giordano Vincenzo, Verdoliva Ciro, Santini Stefania . A Clinical Decision Support System based on fuzzy rules and classification algorithms for monitoring the physiological parameters of type-2 diabetic patients. Mathematical Biosciences and Engineering, 2021, 18(3): 2654-2674. doi: 10.3934/mbe.2021135 |
[3] | Alfonso Maria Ponsiglione, Carlo Ricciardi, Giovanni Improta, Giovanni Dell'Aversana Orabona, Alfonso Sorrentino, Francesco Amato, Maria Romano . A Six Sigma DMAIC methodology as a support tool for Health Technology Assessment of two antibiotics. Mathematical Biosciences and Engineering, 2021, 18(4): 3469-3490. doi: 10.3934/mbe.2021174 |
[4] | Yifan Wang, Lu Zhang, Yan Li, Fei Wu, Shiyu Cao, Feng Ye . Predicting the prognosis of HER2-positive breast cancer patients by fusing pathological whole slide images and clinical features using multiple instance learning. Mathematical Biosciences and Engineering, 2023, 20(6): 11196-11211. doi: 10.3934/mbe.2023496 |
[5] | Cyrille Agossou, Mintodê Nicodème Atchadé, Aliou Moussa Djibril, Svetlana Vladimirovna Kurisheva . Mathematical modeling and machine learning for public health decision-making: the case of breast cancer in Benin. Mathematical Biosciences and Engineering, 2022, 19(2): 1697-1720. doi: 10.3934/mbe.2022080 |
[6] | Mohamad Al Bannoud, Tiago Dias Martins, Silmara Aparecida de Lima Montalvão, Joyce Maria Annichino-Bizzacchi, Rubens Maciel Filho, Maria Regina Wolf Maciel . Integrating biomarkers for hemostatic disorders into computational models of blood clot formation: A systematic review. Mathematical Biosciences and Engineering, 2024, 21(12): 7707-7739. doi: 10.3934/mbe.2024339 |
[7] | Venkatesan Rajinikanth, Seifedine Kadry, Ramya Mohan, Arunmozhi Rama, Muhammad Attique Khan, Jungeun Kim . Colon histology slide classification with deep-learning framework using individual and fused features. Mathematical Biosciences and Engineering, 2023, 20(11): 19454-19467. doi: 10.3934/mbe.2023861 |
[8] | Jian-Di Li, Gang Chen, Mei Wu, Yu Huang, Wei Tang . Downregulation of CDC14B in 5218 breast cancer patients: A novel prognosticator for triple-negative breast cancer. Mathematical Biosciences and Engineering, 2020, 17(6): 8152-8181. doi: 10.3934/mbe.2020414 |
[9] | Lili Jiang, Sirong Chen, Yuanhui Wu, Da Zhou, Lihua Duan . Prediction of coronary heart disease in gout patients using machine learning models. Mathematical Biosciences and Engineering, 2023, 20(3): 4574-4591. doi: 10.3934/mbe.2023212 |
[10] | Vitaliy Yakovyna, Natalya Shakhovska . Modelling and predicting the spread of COVID-19 cases depending on restriction policy based on mined recommendation rules. Mathematical Biosciences and Engineering, 2021, 18(3): 2789-2812. doi: 10.3934/mbe.2021142 |
In clinical decision support, argumentation plays a key role while alternative reasons may be available to explain a given set of signs and symptoms, or alternative plans to treat a diagnosed disease. In literature, this key notion usually has closed boundary across approaches and lacks of openness and interoperability in Clinical Decision Support Systems (CDSSs) been built. In this paper, we propose a systematic approach for the representation of argumentation, their interpretation towards recommendation, and finally explanation in clinical decision support. A generic argumentation and recommendation scheme lays the foundation of the approach. On the basis of this, argumentation rules are represented using Resource Description Framework (RDF) for clinical guidelines, a rule engine developed for their interpretation, and recommendation rules represented using Semantic Web Rule Language (SWRL). A pair of proof knowledge graphs are made available in an integrated clinical decision environment to explain the argumentation and recommendation rationale, so that decision makers are informed of not just what are recommended but also why. A case study of triple assessment, a common procedure in the National Health Service of UK for women suspected of breast cancer, is used to demonstrate the feasibility of the approach. In conducting hypothesis testing, we evaluate the metrics of accuracy, variation, adherence, time, satisfaction, confidence, learning, and integration of the prototype CDSS developed for the case study in comparison with a conventional CDSS and also human clinicians without CDSS. The results are presented and discussed.
Evidence-based medicine promotes conscious and explicit use of the best evidence for clinical decision-making [1]. Clinical Decision Support Systems (CDSSs) can be developed that match evidence-based guidelines to patient conditions and generates customized recommendations. As alternative reasons may explain a given set of signs and symptoms, and alternative plans may treat a diagnosed disease, a key component in CDSSs is actually argumentation. The goal of argumentation is generating the most appropriate recommendations and better still, convincing the decision makers what has been recommended. It is therefore crucial that argumentation has its representation in compliance with clinical evidence, interpretation in an automatic manner, and explanation readily available in the very decision-making context.
The representation of argumentation shall suit clinical decision support, and encapsulate the decision rationale as described in clinical evidence. However, the majority of the existing clinical decision languages such as Arden syntax [2], Guideline Interchange Format (GLIF) [3], PROforma [4], and even agent-oriented paradigm of Goal-Norm-Agreement-Plan-Belief (GNAPB) [5] remain independent and proprietary in their nature. This results in their closed boundary and lack of openness and interoperability in CDSSs been built. Thus, in the past, numerous difficulties have been encountered in their integration with local environments such as Electronic Health Record (EHR) systems, or in joining external knowledge exchange processes when required. The lack of semantic interoperation inevitably hinders the adoption of CDSSs and eventually leads to their failure. In addition, explanation is also important to CDSSs but which is, sadly, often missing in the literature. The result is that, there are often rare opportunities for decision makers to fully exploit the decision options and the underlying recommendation rationale in the due process of decision-making. They may feel hesitate in committing to appropriate decisions.
It has been increasingly realized that issues such as interoperability and explain-ability place a tremendous burden upon local advocators and potential users, and it may lead to the reluctance of the use of CDSSs. In this work, an investigation of argumentation-centered decision support is carried out. We propose an approach for the representation of RDF-based argumentation rules, in an attempt to address the interoperability issue. A generic rule engine is developed to support their interpretation. The decision recommendation processes are accompanied by proof knowledge graphs, addressing the explain-ability issue. A prototype is built as a demonstration of the approach, using a case study of the triple assessment of women suspected of breast cancer.
Argumentation is an activity that puts forward propositions to justify or refute standpoints prior to reaching a rational judgment [6]. Argumentation systems can help to capture and model the discussions involved in meetings or conversations whereas decisions need to be made. Early in the 60s, an argumentation-based approach of Issue Based Information System (IBIS) [7] was invented. It is designed to solve what Rittel has defined as the "wicked problems" [8]—problems that exist in the real world and could not be solved by formal models. Since the middle of 2000s, IBIS-related approaches have increasingly gained popularity, as they have been applied in developing various kinds of computer-based systems for collaborative problem solving. Of special note are systems using a "Dialogue Mapping" [9] technique, which can be employed to facilitate with translating participants' comments into the IBIS scheme, with key notations of Questions, Ideas, Pros and Cons. First, a Question or a problem-to-solve is raised, shown as a question mark in a diagram. Then, a number of responding Ideas or possible solutions to the problem are proposed, shown as bulb marks and pointed to the question. Finally, under each Idea, the Pros and Cons or the arguments support or object to the corresponding solution are put forward, shown as plus and minus marks and pointed to the idea. Any of these can be further questioned and this makes a growing tree structure. Eventually, a decision is chosen as one of the Ideas on the root Question. The contributions of participants are represented progressively and informally using free-text labeled nodes and edges in an argumentation diagram.
In the 2010s, major institutions such as MIT Centre for Collective Intelligence advocate the use of IBIS argumentation scheme, as opposed to collocated meetings which are impractical, expensive, and with limited breadth of interaction, as well as social media which are unsystematic, poorly-organized, and with a wide range of quality [11]. Some techniques and tools are developed to support the scheme. Compendium augments human dialogue and shared cognition in organizations [12], hence facilitating Computer-Supported Collaborative Argumentation (CSCA). Deliberatorium uses attention mediation metrics to enhance argumentation effectiveness [13], via the synthesis of human communities' creativity and computer systems' data analysis productivity. Such kind of fusion has been considered as an important part of what is envisioned as "programming the global brain" [14] or building up the "superminds" [15]. The idea is that networked humans and computers will bring together collective intelligence in the emergent era.
However, all above studies have the disadvantages in those human users and their experience are the primary concern. An argument map is central to the design, and it has a simple and systematic structure that encourages clarity and reduces redundancy. Nevertheless, they are left in a form just for human comprehension and will gradually lose value since they are not automatically interpretable by machine or put in an integrated decision support environment. More importantly, as argument maps will inevitably evolve, it will be more and more difficult to maintain the link between the argument structures and their implications in the real world continuously.
It is only around 2010s till this day that the propositions of representing arguments in a way that both semantically rich and computationally enabled are put forward. Initiatives such as the Argument Web [16,17,18] are aimed at the storage, visualisation, and sharing of argument structures, using an Argument Interchange Format (AIF) [19]. While largely written in natural language, the AIF description can be specified in OWL and RDF, thus facilitating argument exchange across domains.
In the clinical domain, argumentation is helpful in formalizing human cognition and reasoning about the best clinical decisions to make. One notable model is logic of argument (LA) [10], developed in the 2000s, which underpins the guideline representation language of PROforma. LA includes three parts of an argument: claim, grounds, and confidence. Claims can be alternative clinical options to believe such as diagnosis or treatment. Grounds and confidence can be built up, e.g., via judging symptoms in support or oppose a certain diagnosis, etc. In fact, the model shares some similarities with the IBIS scheme, whereas Questions are the decisions to make, Ideas are the decision options, Pros and Cons are the arguments. LA is also closely related with Toulmin's influential, yet more generic argumentation scheme [29] that establishes formal theoretical ground to argue about certain claims to believe, e.g., decision options to commit to.
LA and other approaches were designed at the time that decision support systems are mostly proprietary, with closed boundary and limited request of interoperability. Unfortunately, the lack of a counterpart open argument structure as AIF in the clinical domain results in the adoption of traditional CDSSs increasingly difficult. It has been well recognized that the semantic integration of argumentation in clinical decision support as well as the underlying clinical datasets should be made straightforward to enable knowledge sharing and reasoning across clinical domains and boundaries. In fact, the triple structure of RDF is a natural candidate for representing propositions formally [20], and RDF graphs are a promising alternative to existing argument graph representations. It has been demonstrated that Toulmin's argument scheme can be defined using a generic ontology [21], which could later guide the representation and interpretation of arguments in interchangeable RDF structures. Related techniques have also been applied to maintain semantic relationships among clinical concepts [22], acquire semantically enriched data for clinical queries [23] or storage [24], and towards clinical recommendations using SWRL [25].
We believe the synthesis of conventional argument theories with Semantic Web-oriented knowledge representations and inference machinery, as advocated by this study, will advance a promising, open and interoperable clinical decision support paradigm. In the age of Web, encoding semantic domain knowledge offers a standard approach for managing software complexity continuously, as business logic is decoupled from code and in an easily configurable manner. One may even envision that one day, a kind of "Semantic Argument Web" as an extension of the Semantic Web will augment the flexibly, understandability, and reasoning ability of the Web to a much deeper extent. It is sensible, thus far, to advocate a systematic approach to represent RDF-based argumentation knowledge for nationally or internationally recognized clinical guidelines and use them to drive decision support.
Many CDSSs fail to explain to human decision makers the decision rationale behind the recommendation. While humans have insufficient confidence or feel uncomfortable about what are suggested, the advices may potentially be obsolete, unfortunately.
In the opposite side, the benefits are evident. The PHI method has been proposed in [26] to enhance IBIS, and it integrates argumentation into a context to detect and critique suboptimal solutions made by decision makers. The communication between humans and problem-domains is improved, during which crucial decision-making won't be disrupted by argumentation but supported so via critical reflection in an integrated environment. In a study of tour recommender systems [27], it is found that entity-based explanations with ontology classes and sentence-based explanations can enhance user satisfaction towards the recommended tours. In the clinical domain, PANDEX [28] is a genetic prenatal consultation application designed to calculate the optimal treatment strategy with an explanatory infrastructure to assist patients and care providers to reach their shared decisions. It has been demonstrated that the graphical tools embedded in the system can facilitate patients to fully understand the recommended strategy, while their personal medical data or preferences are changed the corresponding effects on the recommended strategy can be further explored.
An overview of the methods proposed in this paper is shown in Figure 1. A generic argumentation and recommendation scheme is put forward (Section 2.2). This lays the foundation of representing clinical rationale so that generic clinical evidence can be applied to specific patient circumstance and generating customized decisions. The representation of argumentation rules is demonstrated using the scheme and a case study of triple assessment (Section 2.3). A systematic representation process is introduced, as well as the additional expressive power in representing recommendations against overscreening, and the attacking relationships among arguments. A rule engine is developed to support the interpretation of RDF-based argumentation rules (Section 2.4). The representation of SWRL-based recommendation rules is demonstrated, along with its supporting ontology (Section 2.5). These lead to the development of a prototype CDSS (Section 3).
A scheme for argumentation rules is proposed here on the basis of an adaptation and extension of the influential Toulmin's general argument scheme [29], most suitable for complex real-world situations in which no absolute solution to a problem is available. The scheme is designed specifically to suit the domain of clinical decision support. It is shown in the upper part of Figure 2 that the original scheme can be stated as a Conclusion being established on the basis of a Fact supported by a Warrant, with potentially additional Backing, Rebuttal, and Qualifier elements. It is shown in the lower part of Figure 2 that our argumentation rule scheme can be stated as a Decision Candidate (Conclusion or Claim) being asserted by Patient Data Statement (Fact, Ground, or Data), for reasons given in the Argument (Warrant, linking the Ground to the Claim). Such an argument can have a Support Type (Pros or Cons) & Weight (Qualifier or Probability), and usually supported by a Clinical Evidence (Backing).
It is essential that the argumentation holds the whole structure together. The Backing component may represent the rationale behind clinical guidelines published nationally or internationally, or synthesized from statistical analysis of clinical trails in very large scales. The Rebuttal element may or may not present in this scheme. For example, one may specify an argument against a decision candidate, effectively the same as attacking one of its supporting arguments. We believe this argumentation rule scheme is generic and simple enough while used in representing and executing clinical guidelines. The recommendation scheme will be detailed later.
Next, we demonstrate the representation of argumentation rules using the above scheme around a case study of Triple Assessment. This is a common procedure in the National Health Service of UK for women suspected with breast cancer. The major decisions that need to be made are: 1) clinical and genetic risk assessment, 2) imaging assessment by mammography or ultrasound and 3) pathology assessment by core biopsy, fine needle aspiration, skin biopsy, etc. Only after making these decisions will a multidisciplinary team be able to make a management decision about whether to refer a patient for treatment of a tumor. In this study, the actual argumentation rule specifications are derived directly from well-established clinical guidelines in natural language. An important portion of them for imaging assessment are aggregated and summarized in Figure 3.
The key elements of the argumentation rule scheme referred in Figure 2 can be defined more precisely as follows:
1) Candidate (Decision Candidate): A proposed decision option in a form of belief (e.g., whether a disease or not), action (e.g., treatment or discharge), or plan (e.g., surgery or chemotherapy), etc.
2) Argument: A proposition that argues about a Candidate with a support type of either "for" (support) or "against" (oppose) with a weight indicating its strength, or alternatively "confirming" (absolute support) or "excluding" (absolute oppose) without a weight. This shall be supported directly by clinical evidence. The support aggregated from all the arguments of a candidate is called its net-support.
3) Statement (Patient Data Statement): A clinical expression such as a patient's presence of symptoms, signs, lab test results that may be judged as either true or false. This represents the circumstance under which an argument can apply.
A general process of key element elicitation for argumentation rules is given as below. The clinical guidelines for radiology decisions in Figure 3 are used. Some manual efforts from both clinical domain experts and knowledge engineers are required.
1) Decision candidate elicitation: the decision candidates are usually mentioned side by side in the guideline as alternative solutions to a given problem explicitly, e.g., mammogram and ultrasound can be considered as candidate imaging methods of radiology (Paragraph 3). The resulting main structure of RDF-based argumentation rules here is multiple "candidate" elements under a "decision" element, as seen in Part 1 of Figure 4.
2) Argument identification and grouping: The arguments need to be identified and grouped under decision candidates. Each argument can have a weight, a support type and a statement, e.g., all the indications for both imaging methods are of the "for" type of arguments (Paragraphs 1 and 2) whereas avoiding mammogram in certain situations (Paragraphs 2 and 3) are of the "against" type. Arguments are associated with differentiated weights as implied in the guideline, e.g., a weight of -400 indicates that the patient being pregnant is a quite strong argument against doing mammogram. The main structure here is multiple "argument" elements under a "candidate" element with various support types and weights, as seen in Part 2 of Figure 4.
3) Statement construction: An argument has a logic representation that needs to be evaluated to hold true to support or oppose a decision candidate. This logic representation is used for statement construction, e.g., a patient being of pregnancy (Paragraph 2) is an argument that opposes the decision candidate of mammogram. A statement may be joint by multiple parts linked by "and" and "or" (as we will see examples later). The main structure here is a "statement" with a standard triple structure, as seen in Part 3 of Figure 4.
In the imaging assessment of Triple Assessment, a typical argumentation rule is that: "a patient being pregnant" (Statement) is an Argument against (support type) "mammogram" (Candidate), as indicated by the "ACR Practice Guideline for the Performance of Diagnostic Mammography" [32] for the reason of the potential radiation risks to the fetus (clinical evidence). An argument glues together statements with a decision candidate. In addition to its XML format, a directed graphical representation of such an argumentation rule is shown in Figure 5. It has a layered RDF structure. Here, a rule's RDF triple is that a statement (subject), an argument against (predicate), and a decision candidate of mammogram (object). Within it, a statement can be further represented as a RDF triple of subject-predicate-object, whereas the subject and predicate elements can be both RDF resources. The actual statement's RDF triple here is that patient (subject), currently Pregnant (predicate), and true (object).
Two special kinds of clinical guidelines may be worth mentioning separately. One of them focuses on the increasingly important emphasis on reducing practices that are likely to have limited benefit and potential harm to patients and so recommending against overscreening, overdiagnosis, and overtreatment [34]. A search tool is suggested [35] to help with retrieving recommendations that are put together from over 60 medical societies, under the name of "Choosing Wisely campaign". We believe "what not to do" recommendations are complementary to "what to do" recommendations towards complete and helpful decision support. A typical scenario is a drug-drug interaction that causes side effect, and the existence of simultaneous prescribing should be checked against to exclude improper treatment. Generically, negative relationships should be represented between symptoms, signs or other conditions, and certain screening or treatment actions that should be avoided. Using our existing scheme, such can be represented as arguments marked with "excluding" in the support-type tag. While a recommendation is to be suggested from the given decision candidates, the ones with "excluding" types of arguments shall definitely not be considered (regarded as with a weight of negative infinite). One example retrieved using the search tool says: "Don't routinely use breast MRI for breast cancer screening in average risk women." (American Cancer Society guidelines [36]), distinguished in its support type and represented in Figure 6. Another one says: "Don't routinely recommend follow-up mammograms more often than annually for women who have had radiotherapy following breast conserving surgery." (American Society of Clinical Oncology guideline [37]). These are important rules to be included in delivering proper decision support. The other type of arguments, in contrast, has "confirming" in their support-type tag. The decision candidates with such arguments should definitely be recommended (regarded as with a weight of positive infinite).
Built on the basis of defeasible reasoning, our argumentation scheme can yet be extended to accommodate Toulmin's Rebuttal element, with the addition of an attack relations among arguments. Arguments are interactive rather than independent, whereas an argument can be attacked by another argument (a rebuttal) and defeated which makes it un-justified (to support its original candidate). An attacking relationship can be equally regarded as an exception of the original argument while an additional condition is established. For example, the clinical guideline of "However, the potential risk of mammography is negligible and diagnostic mammography is not contraindicated if cancer is strongly suspected" (Paragraph 2 in Figure 3 [32]) makes such a relationship, shown in Figure 7.
An argument is justified if not defeated, and its original supporting or opposing strength to a candidate may be simply retained, or alternatively adjusted by the attacking power. In the latter case, an aggregating process of the overall strength of this very argument node is required. An argument can be weakened by its linked attacking argument, but which in turn may be attacked by yet another argument that weakened the original attacking effect, and this goes on iteratively. An "attack and weaken" relation between an argument pair is a preferred option set in our model by default, just like the relation between an argument and candidate pair, but a simpler "attack and defeat" model can be switched to if required.
A Rule Engine has been developed to support runtime parsing and execution of RDF-based argumentation rules. The algorithm used by the engine is presented in Figure 8. It takes a RDF model (line 1) and invokes underlying Jena functions for interpreting RDF model elements. This collects into various data structures the decision candidates, the arguments in support or against them, and the statement criteria that needs to be justified for these arguments (lines 2–7). A list of decision candidates together with all those justified arguments in support or against them is eventually returned (lines 8–10). The outcome will be available on the decision support interface on request.
In the algorithm, an argument judging facility of VERIFY-ARGUMENT is defined (line 8). This function takes in an argument of composite statement parts linked by keywords of "AND" and/or "OR". It is demonstrated in Figure 9 a mechanism of interpreting a generic argument structure on the left hand side and a concrete example on the right hand side. It says that an argument to support mammogram and with a weight of 100 is that the patient has been assessed as being at medium or high genetic risk and is over 30 years old. Briefly, the "OR" keywords serve as the splitting points to break a composite argument into separate parts (line 1 of the function). Each part is judged in an iterative manner and if any one of them is successfully judged (lines 3–11) then the whole argument is valid and it jumps out of the loop. The judging of each part is via further splitting them into multiple atomic statements linked only by the "AND" keyword (no more "OR" in between at this moment) and every single atomic statement must be judged as true (lines 4–9) for this part to hold true. A local EHR service can be invoked to match against the atomic statements (line 6).
Upon the completion of argument evaluation by the engine, the decision candidates along with the justified arguments supporting or opposing them are available. Thus, all decision candidates can have their overall weights calculated, via adding together the weights of arguments for them and taking away the weights of arguments against them. The argumentation process is followed by a recommendation process, governed by the recommendation scheme shown in the bottom part of Figure 2. A key element of the scheme is recommendation rules, which represent the strategy of choosing one or more preferred candidates among the alternatives. A strategy as such is often implicitly stated in guidelines and the definition of recommendation rules requires thorough understanding of the guidelines. These may vary one another depending upon the decision problems. One may be defined as: a preferred candidate is chosen as the one with the maximum net-support weight among all candidates by ranking (more arguments for it than others). Another one may be: any candidate with a net-support weight over zero should be chosen (at the very least some argument for them). Yet a third one may be: a preferred candidate is chosen as the first one from an ordered candidate list that has a net-support weight over zero (the one with some argument for them and with the highest priority). We choose to express recommendation rules in the W3C recommended rule language of SWRL, to accommodate generic recommendation strategies and towards a semantic integration with the argumentation.
In our case study, we understand from guidelines that an appropriate action for the radiology decision might be both "do a mammogram of both breasts" and "do an ultrasound of the affected area", or just one of them, or neither. The recommendation rules can be defined as: if the net-support weight of any of the first two options of mammogram and ultrasound is greater than or equal to 1, then that candidate is recommended (multi-selection possible). If neither of the two candidates is greater than or equal to 1, then a third option of "do neither" is recommended, as shown in Figure 10. The recommendation rules in SWRL can be regarded as a list of IF-THEN rules. They are represented using Ontology Web Language (OWL), edited by Protégé v5.2.0 and interpreted by an inference engine of Drools. A recommendation rule written in SWRL seeks to satisfy its antecedent part and if so its consequent part is applied, both expressed on the basis of ontology. It is at runtime that variables are instantiated with values, relationships are established, logic representations are satisfied, and rules are fired.
It is given in Table 1 some example classes and properties that constitute OWL ontology and support SWRL reference and recommendation. In this way, for example, the first SWRL rule representation can be interpreted as: IF the individual in the class of Candidate has name "mammogram", AND it has a weight greater than or equal to the reference value 1, THEN put the individual of mammogram into the class of Recommendation.
Name | Type | Description |
Candidate | Class | A class represents the decision candidates. |
Recommendation | Class | A class stores the recommended decision candidate. |
ultrasound | Individual | An individual as an instance of a Candidate with datatype constraint of "hasName" and "hasWeight". |
mammogram | Individual | An individual as an instance of a Candidate with datatype constraint of "hasName" and "hasWeight". |
doingNeither | Individual | An individual as an instance of a Candidate with datatype constraint of "hasName" and "hasWeight". |
hasWeight | Datatype property (Integer) | A property that represents the weight of a Candidate. |
hasName | Datatype property (String) | A property that represents the name of a Candidate. |
An ontology is built to support the definition of SWRL, with its major part shown in Figure 11. The elements of Candidate, Argument, and Statement in the argumentation scheme are present as the main line of class structure. In a side branch of Argumentation, Recommendation Rules encapsulate the decision strategy and make a certain Recommendation out from the Candidates available. Among the Candidates, human decision makers may choose one as a Commitment eventually, most likely but not necessarily the same as the Recommendation. While a concrete decision is demanded, class instances as individuals become available, e.g., ultrasound and mammogram are Candidates for the decision task of radiology. In the bottom layer, Statements need to be evaluated, e.g., "patient-age less-than 35", "patient currently-pregnant true", "patient lump true", etc. Typically these include patient symptoms, signs and many other terms and so standardized clinical ontology can be referred to. Such RDF resource elements may be retrieved from the existing EHRs in an inter-operable manner, and evidence sources may also be referred.
The main results are a prototype decision support system with integrated explanation. It is particularly important that the decision rationale behind the recommendation is made explicit if guidelines are to be adhered invariably. In contrast with the convention of providing suggestions only, we believe that why these are suggested should be available in addition to what, in the very decision contexts. This would allow decision makers to be convinced of the suggestions and help to reduce the chance of inappropriate decisions. Therefore, in our design of a prototype decision support system, three key components are present.
1) The main decision support interface guides the decision support process, from prompting users for clinical data collection until presenting the recommendation. The screenshots of the typical moments are shown in Figure 12(a), (b), respectively. In Figure 12(a), an automatically generated enquiry interface page is presented, and the data for enquiry are those necessary for executing the RDF-based argumentation rules. In Figure 12(b), an imaging decision on the use of ultrasound investigation is recommended, as indicated by a checkmark. The pros and cons of each candidate are presented and indicated by the plus and minus marks under the candidates. The candidates are also accompanied with their aggregated net-support values. These offer a simple but natural form of explanation.
The decision makers may, however, overturn the default recommendation, and a reason needs to be supplied. Such a process may proceed in several rounds, each of which has a particular set of data for collection followed by clinical, imaging, and pathology argumentation and recommendation. A decision path shall be selected step by step until all major decisions are finally made.
2) A context-sensitive viewer of the original clinical guidelines provides to decision makers the evidence on which basis the decision support is delivered. The appropriate parts of the guidelines in natural languages are displayed in the viewer while decision makers are performing corresponding tasks. The viewer is refreshed and the relevant parts are jumped to for reference while the decision context is changed. This explains, for example, why a clinical symptom is critical and needs to be inquired about for a patient or why a diagnosis is suggested, according to the guideline description.
3) A proof knowledge graph further explains the argumentation and recommendation rationale. Such front-end representation and explanation is in contrast with the back-end interpretation and execution, though both use the same set of argumentation knowledge. Each decision candidate can have its justified and non-justified arguments linked to them, and the comparison of their strengths shown visually. As the proof knowledge graph can be directly examined by decision makers in the decision context, the argumentation is made explicit rather than hidden away, and the recommendation rationale can be appreciated in a human-understandable manner. This is particularly useful in explaining situations whereas an arguable decision is recommended even though some arguments are against it, or a seemingly sound decision is not recommended even though some arguments support it. Ultimately, the human decision actions are less likely to be varied and strayed away from guidelines.
It is shown in Figure 13 a pair of proof knowledge graphs generated automatically within the decision support environment. This includes (a) a generic argumentation graph for a given decision support problem, and (b) a specific recommendation graph for a particular scenario. An argumentation graph is a fully connected graph, establishing all general relationships between concepts as indicated by argumentation rules. One type of connection is concerned with "argument" and "candidate" types of nodes. If Node A (a type of "argument", rectangle, in grey by default) supports/opposes Node C (a type of "candidate", oval, in blue), an edge Argc runs from A to C (a type of "argument-for" or "argument-against", with a strength value). Another type of connection is concerned with "candidate" and "decision" types of nodes. If Node C (a type of "candidate") is a candidate of Node D (a type of "decision", cycle, in red), an edge Cand runs from C to D (a type of "candidate-of"). A specific recommendation graph is a runtime instantiation and partial graph of the previous one. Only the nodes and relationships justified in the context of a specific patient are selected, indicating the establishment of clinical evidences and supporting the comparison of the relative strength of candidates. The graph is produced following the data collection process. The "argument" type of nodes is evaluated and those successfully judged are highlighted and filled in green. The edges that point them to the related "candidate" nodes are also highlighted to indicate their supporting or opposing conditions hold. All other nodes and edges of the same type are taken away. Also, all "candidate" type of nodes is marked with their aggregated weights. One of them is eventually recommended for the "decision" type of node, marked with an outer highlighted cycle indicating it is a "preferred candidate".
Our prototype has its main interface shown in Figure 14. Its three integrated parts include: the main decision support interface (left hand side), the guideline viewer (top right hand side), and the proof knowledge graph (bottom right hand side). At runtime, the decision makers are guided through the decision-making interface, referring simultaneously to the guideline text and the proof knowledge graph. At first, the graph is presented as a general graph of nodes and edges via parsing the RDF-based argumentation rules. Also, the appropriate guidelines as summarized in Figure 3 are loaded into the guideline viewer. This is via parsing the guideline source links as annotated in the "Evidence" node of the argumentation scheme, shown in Figure 5. As decision makers go through the decision process, the enquiry data are fulfilled and this leads, immediately, to their related arguments being established and relevant nodes highlighted in the graph. In Figure 14, it is shown that five argument nodes turn in green in correspondence to the enquiry data just being collected. Also, the proper guidelines are populated into the viewer, or the precise sections jumped to, as soon as the decision context is changed. This goes on until, finally, all recommendations are provided for this particular patient, an example recommendation page being shown in Figure 12(b). Each patient will, eventually, have a specific proof knowledge graph built to reflect her own situations though the same argumentation rationale applies. The graph can be explored by decision makers to make better sense of the recommendation presented on the interface prior to any final decision commitment. These three components of the system are rendered under synchronized coordination.
We carried out an empirical experiment to evaluate our approach. The experiment is organized in the following manner.
1) Firstly, we prepared the clinical decision environments. The prototype system developed for breast cancer, as described in Section 3, was used for comparison. It is empowered with the full clinical decision support capabilities with backend argumentation, recommendation at point of care, and context-sensitive guideline viewer and visualized proof knowledge graph for explanation. The ones for comparison are a conventional decision support system and human clinicians without any decision support. The conventional system was developed using PROforma, the same guidelines and sets of user interfaces were used, but it was limited in explanation facilities. The human users were supplied with a basic decision-making environment but no recommendation. The interfaces of the three environments are shown in Figure 15.
2) Secondly, we set out a number of 8 metrics, namely accuracy, variation, adherence, time, satisfaction, confidence, learning, and integration, for evaluation among the three decision support environments. Accordingly, 8 hypotheses were drawn upon from these. We expected clinicians using our prototype system would perform better (objective) or feel supported better (subjective) than other environments in the above metrics. This part is detailed in Section 4.1.
3) Thirdly, we prepared patient cases for running through decision-making, and recruited postgraduate medical college students as participants. They were randomly assigned to one of the three decision-making environments as a group. The arrangement of patient cases for each participant was designed in a way to enable an independent and fair assessment of different metrics. Four hypotheses on accuracy, variation, adherence, and time as objective metrics were directly measured using the data collected from the observation of decision-making processes of participants. Three hypotheses on satisfaction, confidence, and learning as subjective metrics were measured using questionnaires for decision makers immediately following the decision-making. The last hypothesis on integration was measured using separate questionnaires for the staff of the host IT department. This part is detailed in Section 4.2.
4) Lastly, the data was collected and analyzed in an attempt to confirm the 8 hypotheses. Two P-values were used, one as a statistical measurement that assumes the null hypothesis is correct between two decision support systems, and another between our decision support system and human clinicians. The previously defined hypotheses are considered as alternative hypotheses. The result is that the hypotheses on 6 metrics were confirmed between two decision support systems, and all 8 metrics between our decision support system and human clinicians. We analyzed and gave explanation on why there was no statistically significant difference in terms of the time spent on decisions and the satisfaction level of using the two systems. This part is detailed in Section 4.3.
Experiments were carried out to investigate various quality attributes of the prototype CDSS built with the systematic approach of argumentation, recommendation, and explanation (called ARE-CDSS). We compared ARE-CDSS with a conventional CDSS (called C-CDSS) developed using PROforma, as well as human clinicians (called HC) without any system-level support. Both CDSSs shared the same clinical guidelines, and guided decision makers in decision processes of collecting clinical data, recommending decision options in ranked lists, prompting clinical actions to commit, etc. Nevertheless, ARE-CDSS is capable of semantic linking with local environments and provides advanced explanation facilities. It has been suggested in literature [38,39] that metrics for evaluating decision support systems could be categorized under productivity, process, and perception. These have been extended to derive the precise metrics for our study, summarized in Table 2.
Category | Metric | Description |
Productivity | Accuracy | The accuracy of outcomes produced by decision makers. |
Variation | The variation of outcomes produced by decision makers between two or more similar patient cases (which should be consistent). | |
Process | Adherence | The adherence of decision-making to recommendations. |
Time | The time spent in making decisions. | |
Perception | Satisfaction | The satisfaction of decision makers towards the support received (or themselves if no support is available, the same principle applies below). |
Confidence | The confidence of decision makers in committing to the suggested recommendations. | |
Learning | The level of learning involved in recommendation and explanation during the decision-making processes and the insights decision makers can get from it. In other words, to what extent is the improved understanding from the current experience facilitating the development of skills useful for future decision-making? | |
Integration | The level of desire among hospital IT manager/staff in integrating the CDSS with existing hospital information systems. |
A total number of 8 hypotheses were drawn upon from Table 2, as follows:
Hypothesis 1, Accuracy: Clinicians using ARE-CDSS yield higher accuracy in decision outcomes than those using C-CDSS and HC.
Hypothesis 2, Variation: Clinicians using ARE-CDSS yield less variation in decision outcomes than those using C-CDSS and HC.
Hypothesis 3, Adherence: Clinicians using ARE-CDSS yield higher adherence to recommendations than those using C-CDSS.
Hypothesis 4, Time: Clinicians using ARE-CDSS spend less time than those using C-CDSS and HC.
Hypothesis 5, Satisfaction: Clinicians using ARE-CDSS are more satisfied than those using C-CDSS and HC.
Hypothesis 6, Confidence: Clinicians using ARE-CDSS are more confident than those using C-CDSS and HC.
Hypothesis 7, Learning: Clinicians using ARE-CDSS feel they have more opportunity to learn from it than those using C-CDSS or HC.
Hypothesis 8, Integration: IT managers and staff have a higher level of desire in integrating ARE-CDSS with existing hospital information systems than C-CDSS.
In conducting hypothesis tests, we were able to evaluate the association between various metrics with ARE-CDSS, C-CDSS, and HC, respectively. This provided insights into the value of our approach and the difference it might make, if any.
The experiments were designed as follows. Firstly, we prepared a set of 50 patient cases recorded in the past 5 years from a major national Grade-A tertiary hospital in the Wuhan city through our colleagues from the Breast & Thyroid Surgery Department. The selection of the patient cases was under the supervision and assistance from the Director of the department and the hospital's IT manager. A process of data anonymisation had been carried out to protect patient privacy and respect hospital regulations. The patient cases were coded P1 through P50, and the data were distributed across clinical scenarios so that a diversity of decisions were available.
Then, a number of 40 postgraduate medical college students were recruited to join the experiments, designated as participating subjects of S1 through S40. They had all been trained with background medical knowledge and up to one year of medical practice experience. They were assigned to one of the three clinical decision-making environments of ARE-CDSS, C-CDSS, or HC.
After that, each participating subject were given 10 out of the 50 patient cases as their tasks. The groups with facilitating CDSSs also received a 30 minutes tutorial on the software packages regarding their functions and operations. They all proceeded to decision-making upon the presenting of patient cases, while the HC group making decisions on their own, the C-CDSS group having basis recommendations and the ARE-CDSS group having additional proof knowledge graphs, etc. The decisions made by every participant were recorded, as well as the time spent on each patient case.
Finally, straight following the tasks, participants were asked to fill out questionnaires concerning their satisfaction, confidence, and learning opportunity involved in the tasks. The IT manager of the hospital and four working staff were invited to observe the entire process as mentioned above, designated as I1 through to I5. They were asked to fill out a separate questionnaire to express their desire of integrating these techniques.
Among the 40 participants, 3 failed to complete the entire processes and another 4 were dropped due to the insufficient time spending on the tasks. We considered one had not taken the decision-making tasks seriously if the time spent on any patient case is less than 10 minutes. The remaining 33 participants were randomly assigned to ARE-CDSS, C-CDSS, and HC, making 11 participants in each group. Statistical tests were carried out and no significant difference was found among the groups. A typical setting of patient cases for each participant is described in Table 3.
Patient case | P1 | P2 | P3 | P4 | P5 | P6 | P7 | P8 | P9 | P10e |
Uniqueness of decision paths & CDSS functionality | Ua, Onc | U, Offd | U, On | U, Off | U, On | = P1b, Off | = P2, On | = P3, Off | = P4, On | = P5, Off |
Note: aP1–5 demand running through unique decision paths, abbreviated by "U". bP6–10 share clinical characteristics with P1–5, hence running through equivalent decision paths. cThe CDSS functionality would be turned On for a patient case with explanation facilities, etc. dThe CDSS functionality would be turned Off for a patient case, temporarily. The decision maker would have to make decisions on her own, though using the same set of data and interfaces, i.e., The entire right part of components shown in Figure 14 would be unavailable, and the decision-making process shown in Figure 12(b) would be limited without the ranking and explanation functions. eA decision maker would work on the cases in an ascending order from P1 to P10. The above collective set is called a single patient case set. A total of 5 patient case sets were prepared, and they were randomly selected and assigned to S1 through to S33. |
The accuracy in Hypothesis 1 was measured as the total accurate decisions being made among the 8 patient cases of P1, P3 & P5–10 by each decision maker, as P2 & P4 would have their CDSS functionality turned off, and P6, P8 & P10 would have indications from previous similar cases.
The variation in Hypothesis 2 was measured as the total decision paths chosen by each decision maker that differ each other, unexpectedly, among the 5 pairs of P1–6, P2–7, P3–8, P4–9, and P5–10.
The adherence in Hypothesis 3 was measured as the total decisions being made in compliant with the recommendations, among P1, P3, P5, P7 and P9, while the CDSS functionality turned on.
The time in Hypothesis 4 was measured as the average time spent on each patient case.
The satisfaction, confidence, learning and integration in Hypotheses 5–8 was measured using questionnaires with a 5-point Likert Scale, whereas Hypotheses 5–7 were targeted to S1 through to S33, and Hypothesis 8 to I1 through to I5.
On gathering and analyzing the data on user decision-making and feedback, the hypotheses could be confirmed (or not) in accord with the results shown in Table 4. P-values were used to determine whether we should accept or reject our hypotheses. P-value1 is a statistical measurement that assumes the null hypothesis is correct between ARR-CDSS and C-CDSS, and P-value2 between ARR-CDSS and HC, while alternative hypotheses are defined in Hypotheses 1–8, respectively.
Hypothesis | ARE-CDSS | C-CDSS | HC | P-value1 | Confirm (α = 0.05) | P-value2 | Confirm (α = 0.05) | |||
Mean | S.D. | Mean | S.D. | Mean | S.D. | |||||
H1: Accuracy | 7.1818 | 1.0787 | 6.1818 | 1.4709 | 4.5455 | 2.1616 | 0.042 | Yes | 0.0009 | Yes |
H2: Variation | 0.6364 | 1.0269 | 1.6364 | 1.2863 | 3.3636 | 2.0136 | 0.0288 | Yes | 0.00035 | Yes |
H3: Adherence | 4.6364 | 0.6742 | 3.7273 | 1.1037 | / | / | 0.0152 | Yes | / | / |
H4: Time | 14.6091 | 1.4032 | 16.0455 | 2.6961 | 20.118 | 4.9562 | 0.0664 | No | 0.0010 | Yes |
H5: Satisfaction | 4.1818 | 0.7508 | 4.0909 | 0.5394 | 3.3636 | 0.9244 | 0.3738 | No | 0.0169 | Yes |
H6: Confidence | 4.7273 | 0.4671 | 4.1818 | 0.8739 | 2.7273 | 1.1037 | 0.0414 | Yes | 0.00001 | Yes |
H7: Learning | 4.8182 | 0.4045 | 3.2727 | 1.009 | 1.6364 | 0.809 | 0.00007 | Yes | < 0.00001 | Yes |
H8: Integration | 4.2 | 0.8367 | 2.6 | 1.1402 | / | / | 0.0176 | Yes | / | / |
As a result, null hypotheses were rejected in 6 out of 8 circumstances between ARR-CDSS and C-CDSS, and in all circumstances between ARR-CDSS and HC. In the former case, the hypothesis tests indicate that H1–3 & H6–8 were statistically significant. This revealed a stronger association of accuracy, variation, adherence, confidence, and learning with the new approach than conventional methods or situations whereas no decision support is available at all. It is convincing that the enhanced results in both decision outcomes and user experience were highly related with the integrated mechanism of argumentation, recommendation and explanation. This facilitated decision makers to better examine, exploit, and understand their options and decision rationale. The favorable attitude of IT manager and staff was also encouraging towards the deployment of the new technique. The optimal results align with our primitive research goal and suggest the proposed methods could be a substantial contribution to the current CDSS literature. The comparison of the mean values of the metrics is shown in Figure 16.
As for H4, the result indicates there is no statistically significant difference (P = 0.0664) between ARE-CDSS and C-CDSS in terms of the time spent on decisions, though the mean value of the former is less than the latter. We believe that two reasons contributed to this: 1) the decision time spent on each patient case was calculated as the total time from the start of presenting the case till the end of decisions made. This included the rendering of the components of additional proof knowledge graphs and the guideline viewer, inevitably with a delayed effect; 2) the explanation facilities had probably drawn too much interest to decision makers in their deep investigation and understanding of cases, and the extra time spent on self-learning processes counteracted a timely decision-making manner. Nevertheless, this is arguably a worthy trade-off in the full picture of clinical decision-making. As for H5, a short interview with the participants following the analysis of the questionnaires revealed that a couple of users prefer more concise and focused interface in decision support. Nevertheless, the average of the satisfaction level of ARE-CDSS is slightly higher than C-CDSS though there is no statistically significant difference between them (P = 0.3738).
A couple of follow-up thoughts are worthy of discussion. The result on H7 indicates a statistically significant difference (P < 0.05) both between ARE-CDSS and C-CDSS, and between ARE-CDSS and HC in terms of learning and better understanding about decision-making as a result of the experiment. The conclusion was drawn, though, from the data collected from questionnaires and subjective in some way. A question is then: could we possibly evaluate, in an objective and quantitative manner, the difference between groups on learning? One way of doing this might be the design of a test, using closely related decision-making scenarios and handing it out to participants both before and after the experiment. The paper-based test should include not just what options one would choose but also why. In this way, we could measure the improvement of each participant's knowledge about decision-making, and analyze the difference the experiment makes for each group of participants.
Another question is that: could the decision support facilities deprive decision makers of their own sense of decision-making, and even mislead them in worst scenarios? That might be a dangerous situation, because no system could ever possess complete or perfect knowledge. It would be helpful, in a future experiment, to mix a few wrong recommendations into the complete group of study, and observe whether the participants could pick up any or all of these and give appropriate reasons for rejecting the suggestions. This way, the system could also be self-learning and improve its own knowledgebase continuously. Suppose in certain decision-making contexts, an expert user suggests new options or optimal choices differently due to emerging new arguments. Upon the recurrence of the same situation among different users, the new knowledge should be considered for incorporation.
The contributions of this work are three-fold:
1) It provides an approach of applying Semantic Web-oriented knowledge representation languages and inference machinery to well-recognized argumentation theories and schemes to deliver clinical decision support suited in open and interoperable environments.
2) It contributes to researchers in the clinical decision support field a general means and a reference of Triple Assessment to build tools for other diseases. One can follow the practice described in the paper systematically, starting from the elicitation of argumentation and recommendation rules from clinical guidelines, until finally the construction of prototype systems for various purposes. Some components such as the inference engine and ontology can be reused. The design of eight metrics for assessment can also be reused for their own empirical evaluation.
3) It offers a practical method of integrating recommendation and explanation in delivering decision support. The context-aware, visualized proof knowledge graphs are specially designed and demonstrated in the prototype system. As decision makers are informed of not just what is recommended but also why within an integrated decision support environment, some suboptimal decisions will get criticized and abandoned which may have otherwise been selected by chance without such an explanation component. This makes the efforts invested in representing clinical decision knowledge more worthwhile. Once captured, such knowledge can be put into effective and confident reuse in practice, rather than disregarded in documents, locked in a few experts' head, or tragically, dismissed simply because of insufficient explanation.
In this paper, a systematic approach for argumentation, recommendation, and explanation in clinical decision support is proposed. A prototype system of triple assessment of breast cancer is developed for demonstration. In the approach, the argumentation scheme provides a generic and just enough structure towards problem-solving: raising the decision problem, eliciting the candidates, finding the arguments in relation with the candidates, and the actual statements that make part of the arguments. Though simple and intuitive, it has expressive power and puts the cognitive cost of applying them to the minimum. Yet, the RDF-based representation supports not only the modelling of the guidelines but also their automatic interpretation, until final semantic integration with SWRL-based recommendation rules.
In the future, we will investigate the extension of the approach spatially, temporally, and finally towards a hybrid paradigm of symbolic knowledge representation and reasoning combined with deep learning:
1) While two or more sets of independently developed clinical evidences across countries or organizations become available. As our argumentation scheme has been designed for human communities to contribute a wide range of inputs in the first place, various knowledge sources may well fit in and this offers an opportunity of more comprehensive recommendations. The same interpretation and explanation mechanisms apply. The aggregation of a wide variety of knowledge sources may be achieved in this approach via the matching and elicitation of RDF annotations of decision problems, candidates, arguments, etc. A focus of the study may be upon the merging of complementary or conflicting knowledge sources.
2) While the medicine advances as time goes by. Then new diagnosis or treatment options may become available for a given disease, or new arguments discovered for the existing options. Such new knowledge elements can be accommodated via the configuration of the RDF-based argumentation triple store. The recommendation will be generated in compliant with the new evidence with no further change to the system, as a generic interpretation engine has been put in place. Furthermore, we intend to enable decision makers from various organizations as end users to configure their own argumentation and recommendation rules flexibly. This will allow the evolution of the knowledge base and decision-making environment to reflect local knowledge and policies, etc. We will also look into the recommendations that may be discarded by clinicians during the running of the system, and these will be recorded and analyzed continuously to refine our knowledge base.
3) While we adopt the W3C endorsed standards such as RDF and SWRL for knowledge representation and inference, the method benefits from two perspectives evidently: the well-established national or international clinical guidelines are respected, and the decision support accompanied explanation is easily understandable to clinicians. At the same time, the work on clinical decision support using deep learning methods flourished, e.g., in predicting future clinical events [40] and personalized prescription of medication [41]. Such methods have the advantages of learning from large public medical datasets available such as MIMIC-III, using various kinds of algorithms. Nevertheless, evidence indicates that they also suffer a number of deficiencies, notably biased decision output for under-represented groups of population due to the underlying training data [42], and a lack of clinical-level reasoning and explanation for clinicians, among others. Given the strengths and weaknesses of both paradigms, we would hope to find a reconciliation of them in our future work, possibly via the supplement of a deep learning module or even deeper methodological integration. In doing so, our method would become even more powerful. Efforts would be made in the design of this hybrid paradigm that sustains the capability of guideline representation and being clinician-friendly, while makes the best use of big data and learning new knowledge and insights from it continuously.
The publication of this paper is funded by grants from National Natural Science Foundation of China (No. 61202101), and a visiting scholarship sponsored by the China Scholarship Council for a full year research collaboration with Oxford University. The paper is an outcome of continuous collaborative work between the co-authors. Sadly, Prof. John Fox has passed away when the paper is in publication. This piece of work is co-authored by him and devoted to him, in memory of his unique contribution to the clinical guideline representation language of PROforma in particular and clinical decision support research in the large.
The authors declare there is no conflict of interest.
[1] |
J. Y. Yang, L. Xiao, K. N. Li, Modelling clinical experience data as an evidence for patient-oriented decision support, BMC Med. Inf. Decis. Making, 20 (2020), 1-11. https://doi.org/10.1186/s12911-020-1121-4 doi: 10.1186/s12911-020-1121-4
![]() |
[2] |
G. Hripcsak, P. Ludemann, T. A. Pruor, O. B. Wigertz, P. B. Clayton, Rationale for the Arden Syntax, Comput. Biomed. Res., 27 (1994), 291-324. https://doi.org/10.1006/cbmr.1994.1023 doi: 10.1006/cbmr.1994.1023
![]() |
[3] | M. Peleg, A. A. Boxwala, O. Ogunyemi, Q. Zeng, S. Tu, R. Lacson, et al., GLIF3: The evolution of a guideline representation format, in Proceedings of the AMIA Symposium, (2000), 645-649. |
[4] |
D. R. Sutton, J. Fox, The syntax and semantics of the PROforma guideline modeling language, J. Am. Med. Inf. Assoc., 10 (2003), 433-443. https://doi.org/10.1197/jamia.m1264 doi: 10.1197/jamia.m1264
![]() |
[5] | L. Xiao, J. Fox, H. Zhu, An agent-oriented approach to support multidisciplinary care decisions, in Proceedings of the 3rd Eastern European Regional Conference on the Engineering of Computer Based Systems, (2013), 8-17. https://doi.org/10.1109/ECBS-EERC.2013.10 |
[6] | F. H. Eemeren, R. Grootendorst, R. H. Johnson, C. Plantin, C. A. Willard, Fundamentals of Argumentation Theory: A Handbook of Historical Backgrounds and Contemporary Developments, Routledge, 2013. https://doi.org/10.4324/9780203811306 |
[7] | J. Conklin, Chapter 4 IBIS: A tool for all reasons, in Dialogue Mapping: Building Shared Understanding of Wicked Problem, Wiley, 2005. |
[8] | H. W. J. Rittel, Second generation design methods, in Developments in Design Methodology (eds. N. Cross), Wiley & Sons, Chichester, UK, (1984), 317-327. |
[9] | J. Conklin, Dialogue Mapping, Wiley & Sons, Chichester, 2006. |
[10] |
J. Fox, D. Glasspool, D. Grecu, S. Modgil, M. South, V. Patkar, Argumentation-based inference and decision making-a medical perspective, IEEE Intell. Syst., 22 (2007), 34-41. https://doi.org/10.1109/MIS.2007.102 doi: 10.1109/MIS.2007.102
![]() |
[11] | M. Klein, P. Spada, R. Calabretta, Enabling deliberations in a political party using large-scale argumentation: A preliminary report, in Proceedings of the 10th International Conference on the Design of Cooperative Systems, (2012), 17. |
[12] | S. B. Shum, M. Sierhuis, J. Park, M. Brown, Software agents in support of human argument mapping, in Frontiers in Artificial Intelligence and Applications Series, IOS Press, Amsterdam, 216 (2010), 123-134. http://doi.org/10.3233/978-1-60750-619-5-123 |
[13] |
M. Klein, Enabling large-scale deliberation using attention-mediation metrics, Comput. Supported Collab. Work, 21 (2012), 449-473. https://doi.org/10.1007/s10606-012-9156-4 doi: 10.1007/s10606-012-9156-4
![]() |
[14] |
A. Bernstein, M. Klein, T. Malone, Programming the global brain, Commun. ACM, 55 (2012), 41-43. http://doi.org/10.1145/2160718.2160731 doi: 10.1145/2160718.2160731
![]() |
[15] | T. W. Malone, Superminds: The Surprising Power of People and Computers Thinking Together, Little, Brown Spark, 2018. |
[16] |
I. Rahwan, F. Zablith, C. Reed, Laying the foundations for a world wide argument web, Artif. Intell., 171 (2007), 897-921. https://doi.org/10.1016/j.artint.2007.04.015 doi: 10.1016/j.artint.2007.04.015
![]() |
[17] |
F. Bex, J. Lawrence, M. Snaith, C. Reed, Implementing the argument web, Commun. ACM, 56 (2013), 66-73. https://doi.org/10.1145/2500891 doi: 10.1145/2500891
![]() |
[18] | R. Duthie, J. Lawrence, C. Reed, J. Visser, D. Zografistou, Navigating arguments and hypotheses at scale, in Frontiers in Artificial Intelligence and Applications, IOS Press, 326 (2020), 459-460. https://doi.org/10.3233/FAIA200533 |
[19] |
C. Chesnevar, J. McGinnis, S. Modgil, I. Rahwan, C. Reed, G. Simari, et al., Towards an argument interchange format, Knowl. Eng. Rev., 21 (2006), 293-316. https://doi.org/10.1017/S0269888906001044 doi: 10.1017/S0269888906001044
![]() |
[20] | S. Hawke (W3C), Rule Interchange Format Working Group Charter, Available from: https://www.w3.org/2005/rules/wg/charter, last accessed in 2022/5. |
[21] | I. Rahwan, P. V. Sakeer, Towards representing and querying arguments on the semantic web, in Proceedings of COMMA 2006, (2006), 3-14. |
[22] |
L. Marco-Ruiz, C. Pedrinaci, J. A. Maldonado, L. Panziera, R. Chen, J. G. Bellika, Publication, discovery and interoperability of clinical decision support systems: A linked data approach, J. Biomed. Inf., 62 (2016), 243-264. https://doi.org/10.1016/j.jbi.2016.07.011 doi: 10.1016/j.jbi.2016.07.011
![]() |
[23] |
R. S. Gonçalves, S. W. Tu, C. I. Nyulas, M. J. Tierney, M. A. Musen, An ontology-driven tool for structured data acquisition using Web forms, J. Biomed. Semant., 8 (2017), 1-14. https://doi.org/10.1186/s13326-017-0133-1 doi: 10.1186/s13326-017-0133-1
![]() |
[24] |
F. Sadki, J. Bouaud, G. Guézennec, B. Séroussi, Semantically structured web form and data storage: A generic ontology-driven approach applied to breast cancer, Stud. Health Technol. Inf., 255 (2018), 205-209. https://doi.org/10.3233/978-1-61499-921-8-205 doi: 10.3233/978-1-61499-921-8-205
![]() |
[25] |
M. Martínez-Romero, J. M. Vázquez-Naya, J. Pereira, M. Pereira, A. Pazos, G. Baños, The iOSC3 system: Using ontologies and SWRL rules for intelligent supervision and care of patients with acute cardiac disorders, Comput. Math. Methods Med., (2013), 650671. https://doi.org/10.1155/2013/650671 doi: 10.1155/2013/650671
![]() |
[26] |
G. Fischer, A. C. Lemke, R. McCall, A. I. Morch, Making argumentation serve design, Hum. Comput. Interact., 6 (1991), 393-419. http://doi.org/10.1207/s15327051hci0603&4_7 doi: 10.1207/s15327051hci0603&4_7
![]() |
[27] |
V. Lully, P. Laublet, M. Stankovic, F. Radulovic, Enhancing explanations in recommender systems with knowledge graphs, Procedia Comput. Sci., 137 (2018), 211-222. https://doi.org/10.1016/j.procs.2018.09.020 doi: 10.1016/j.procs.2018.09.020
![]() |
[28] |
I. Segal, Y. Shahar, A distributed system for support and explanation of shared decision-making in the prenatal testing domain, J. Biomed. Inf., 42 (2009), 272-286. https://doi.org/10.1016/j.jbi.2008.09.004 doi: 10.1016/j.jbi.2008.09.004
![]() |
[29] | S. E. Toulmin, The Uses of Argument, Cambridge University Press, 2003. https://doi.org/10.1017/CBO9780511840005 |
[30] | The European Society of Breast Imaging (EUSOBI), Breast ultrasound: Recommendations for information to women and referring physicians by the European society of breast imaging, Insights Imaging, 9 (2018), 449-461. https://doi.org/10.1007%2Fs13244-018-0636-z |
[31] | The American College of Radiology (ACR), ACR Practice Parameter for the Performance of a Breast Ultrasound Examination, 2016. Available from: https://www.acr.org/-/media/ACR/Files/Practice-Parameters/us-breast.pdf?la=en. |
[32] | The American College of Radiology (ACR), ACR Practice Guideline for the Performance of Diagnostic Mammography, 2008 (Resolution 24). Available from: https://wiki.radiology.wisc.edu/images/a/a8/SOG_Outreach_ACRattachment.pdf. |
[33] |
Association of Breast Surgery at BASO, Royal College of Surgeons of England, Guidelines for the management of symptomatic breast disease, Eur. J. Surg. Oncol., 31 (2005), 1-21. https://doi.org/10.1016/j.ejso.2005.02.006 doi: 10.1016/j.ejso.2005.02.006
![]() |
[34] | J. Siwek, Getting medicine right: Overcoming the problem of overscreening, overdiagnosis, and overtreatment, Am. Fam. Phys., 91 (2015), 18-20. |
[35] | Choosing Wisely Search Tool Sponsored by American Family Physician, Available from: http://www.aafp.org/afp/recommendations/search.htm, last accessed in 2022/5. |
[36] |
D. Saslow, C. Boetes, W. Burke, S. Harms, M. O. Leach, C. D. Lehman, et al., American Cancer Society guidelines for breast screening with MRI as an adjunct to mammography, CA Cancer J. Clin., 57 (2007), 75-89. https://doi.org/10.3322/canjclin.57.2.75 doi: 10.3322/canjclin.57.2.75
![]() |
[37] |
J. L. Khatcheressian, Breast cancer follow-up and management after primary treatment: An American Society of Clinical Oncology clinical practice guideline update, J. Clin. Oncol., 31 (2013), 961-965. https://doi.org/10.1200/jco.2012.45.9859 doi: 10.1200/jco.2012.45.9859
![]() |
[38] | R. H. Sprague, E. Carlson, Building Effective Decision Support Systems, Prentice-Hall, 1982. |
[39] |
H. J. Watson, Revisiting ralph sprague's framework for developing decision support systems, Commun. Assoc. Inf. Syst., 42 (2018), 363-385. https://doi.org/10.17705/1CAIS.04213 doi: 10.17705/1CAIS.04213
![]() |
[40] |
Y. J. Ru, X. H. Qiu, X. Y. Tan, B. Chen, Y. B. Gao, Y. C. Jin, Sparse-attentive meta temporal point process for clinical decision support, Neurocomputing, 485 (2022), 114-123. https://doi.org/10.1016/j.neucom.2022.02.028 doi: 10.1016/j.neucom.2022.02.028
![]() |
[41] |
X. H. Qiu, X. Y. Tan, Q. Li, S. T. Chen, Y. J. Ru, Y. C. Jin, A latent batch-constrained deep reinforcement learning approach for precision dosing clinical decision support, Knowl. Based Syst., 237 (2022), 107689. https://doi.org/10.1016/j.knosys.2021.107689 doi: 10.1016/j.knosys.2021.107689
![]() |
[42] |
E. M. Cahan, T. Hernandez-Boussard, S. Thadaney-Israni, D. L. Rubin, Putting the data before the algorithm in big data addressing personalized healthcare, npj Digital Med., 2 (2019), 1-6. https://doi.org/10.1038/s41746-019-0157-2 doi: 10.1038/s41746-019-0157-2
![]() |
1. | Liang Xiao, Des Greer, Linked Argumentation Graphs for Multidisciplinary Decision Support, 2023, 11, 2227-9032, 585, 10.3390/healthcare11040585 | |
2. | Ziji Liu, Liang Xiao, Miaomiao He, Rujun Zhu, Hang Yang, Jianxia Chen, 2024, PICOAS: a clinical knowledge linking model for delivering up-to-date, interrelated, and personalized decision support, 979-8-3503-8622-6, 6589, 10.1109/BIBM62325.2024.10821913 |
Name | Type | Description |
Candidate | Class | A class represents the decision candidates. |
Recommendation | Class | A class stores the recommended decision candidate. |
ultrasound | Individual | An individual as an instance of a Candidate with datatype constraint of "hasName" and "hasWeight". |
mammogram | Individual | An individual as an instance of a Candidate with datatype constraint of "hasName" and "hasWeight". |
doingNeither | Individual | An individual as an instance of a Candidate with datatype constraint of "hasName" and "hasWeight". |
hasWeight | Datatype property (Integer) | A property that represents the weight of a Candidate. |
hasName | Datatype property (String) | A property that represents the name of a Candidate. |
Category | Metric | Description |
Productivity | Accuracy | The accuracy of outcomes produced by decision makers. |
Variation | The variation of outcomes produced by decision makers between two or more similar patient cases (which should be consistent). | |
Process | Adherence | The adherence of decision-making to recommendations. |
Time | The time spent in making decisions. | |
Perception | Satisfaction | The satisfaction of decision makers towards the support received (or themselves if no support is available, the same principle applies below). |
Confidence | The confidence of decision makers in committing to the suggested recommendations. | |
Learning | The level of learning involved in recommendation and explanation during the decision-making processes and the insights decision makers can get from it. In other words, to what extent is the improved understanding from the current experience facilitating the development of skills useful for future decision-making? | |
Integration | The level of desire among hospital IT manager/staff in integrating the CDSS with existing hospital information systems. |
Patient case | P1 | P2 | P3 | P4 | P5 | P6 | P7 | P8 | P9 | P10e |
Uniqueness of decision paths & CDSS functionality | Ua, Onc | U, Offd | U, On | U, Off | U, On | = P1b, Off | = P2, On | = P3, Off | = P4, On | = P5, Off |
Note: aP1–5 demand running through unique decision paths, abbreviated by "U". bP6–10 share clinical characteristics with P1–5, hence running through equivalent decision paths. cThe CDSS functionality would be turned On for a patient case with explanation facilities, etc. dThe CDSS functionality would be turned Off for a patient case, temporarily. The decision maker would have to make decisions on her own, though using the same set of data and interfaces, i.e., The entire right part of components shown in Figure 14 would be unavailable, and the decision-making process shown in Figure 12(b) would be limited without the ranking and explanation functions. eA decision maker would work on the cases in an ascending order from P1 to P10. The above collective set is called a single patient case set. A total of 5 patient case sets were prepared, and they were randomly selected and assigned to S1 through to S33. |
Hypothesis | ARE-CDSS | C-CDSS | HC | P-value1 | Confirm (α = 0.05) | P-value2 | Confirm (α = 0.05) | |||
Mean | S.D. | Mean | S.D. | Mean | S.D. | |||||
H1: Accuracy | 7.1818 | 1.0787 | 6.1818 | 1.4709 | 4.5455 | 2.1616 | 0.042 | Yes | 0.0009 | Yes |
H2: Variation | 0.6364 | 1.0269 | 1.6364 | 1.2863 | 3.3636 | 2.0136 | 0.0288 | Yes | 0.00035 | Yes |
H3: Adherence | 4.6364 | 0.6742 | 3.7273 | 1.1037 | / | / | 0.0152 | Yes | / | / |
H4: Time | 14.6091 | 1.4032 | 16.0455 | 2.6961 | 20.118 | 4.9562 | 0.0664 | No | 0.0010 | Yes |
H5: Satisfaction | 4.1818 | 0.7508 | 4.0909 | 0.5394 | 3.3636 | 0.9244 | 0.3738 | No | 0.0169 | Yes |
H6: Confidence | 4.7273 | 0.4671 | 4.1818 | 0.8739 | 2.7273 | 1.1037 | 0.0414 | Yes | 0.00001 | Yes |
H7: Learning | 4.8182 | 0.4045 | 3.2727 | 1.009 | 1.6364 | 0.809 | 0.00007 | Yes | < 0.00001 | Yes |
H8: Integration | 4.2 | 0.8367 | 2.6 | 1.1402 | / | / | 0.0176 | Yes | / | / |
Name | Type | Description |
Candidate | Class | A class represents the decision candidates. |
Recommendation | Class | A class stores the recommended decision candidate. |
ultrasound | Individual | An individual as an instance of a Candidate with datatype constraint of "hasName" and "hasWeight". |
mammogram | Individual | An individual as an instance of a Candidate with datatype constraint of "hasName" and "hasWeight". |
doingNeither | Individual | An individual as an instance of a Candidate with datatype constraint of "hasName" and "hasWeight". |
hasWeight | Datatype property (Integer) | A property that represents the weight of a Candidate. |
hasName | Datatype property (String) | A property that represents the name of a Candidate. |
Category | Metric | Description |
Productivity | Accuracy | The accuracy of outcomes produced by decision makers. |
Variation | The variation of outcomes produced by decision makers between two or more similar patient cases (which should be consistent). | |
Process | Adherence | The adherence of decision-making to recommendations. |
Time | The time spent in making decisions. | |
Perception | Satisfaction | The satisfaction of decision makers towards the support received (or themselves if no support is available, the same principle applies below). |
Confidence | The confidence of decision makers in committing to the suggested recommendations. | |
Learning | The level of learning involved in recommendation and explanation during the decision-making processes and the insights decision makers can get from it. In other words, to what extent is the improved understanding from the current experience facilitating the development of skills useful for future decision-making? | |
Integration | The level of desire among hospital IT manager/staff in integrating the CDSS with existing hospital information systems. |
Patient case | P1 | P2 | P3 | P4 | P5 | P6 | P7 | P8 | P9 | P10e |
Uniqueness of decision paths & CDSS functionality | Ua, Onc | U, Offd | U, On | U, Off | U, On | = P1b, Off | = P2, On | = P3, Off | = P4, On | = P5, Off |
Note: aP1–5 demand running through unique decision paths, abbreviated by "U". bP6–10 share clinical characteristics with P1–5, hence running through equivalent decision paths. cThe CDSS functionality would be turned On for a patient case with explanation facilities, etc. dThe CDSS functionality would be turned Off for a patient case, temporarily. The decision maker would have to make decisions on her own, though using the same set of data and interfaces, i.e., The entire right part of components shown in Figure 14 would be unavailable, and the decision-making process shown in Figure 12(b) would be limited without the ranking and explanation functions. eA decision maker would work on the cases in an ascending order from P1 to P10. The above collective set is called a single patient case set. A total of 5 patient case sets were prepared, and they were randomly selected and assigned to S1 through to S33. |
Hypothesis | ARE-CDSS | C-CDSS | HC | P-value1 | Confirm (α = 0.05) | P-value2 | Confirm (α = 0.05) | |||
Mean | S.D. | Mean | S.D. | Mean | S.D. | |||||
H1: Accuracy | 7.1818 | 1.0787 | 6.1818 | 1.4709 | 4.5455 | 2.1616 | 0.042 | Yes | 0.0009 | Yes |
H2: Variation | 0.6364 | 1.0269 | 1.6364 | 1.2863 | 3.3636 | 2.0136 | 0.0288 | Yes | 0.00035 | Yes |
H3: Adherence | 4.6364 | 0.6742 | 3.7273 | 1.1037 | / | / | 0.0152 | Yes | / | / |
H4: Time | 14.6091 | 1.4032 | 16.0455 | 2.6961 | 20.118 | 4.9562 | 0.0664 | No | 0.0010 | Yes |
H5: Satisfaction | 4.1818 | 0.7508 | 4.0909 | 0.5394 | 3.3636 | 0.9244 | 0.3738 | No | 0.0169 | Yes |
H6: Confidence | 4.7273 | 0.4671 | 4.1818 | 0.8739 | 2.7273 | 1.1037 | 0.0414 | Yes | 0.00001 | Yes |
H7: Learning | 4.8182 | 0.4045 | 3.2727 | 1.009 | 1.6364 | 0.809 | 0.00007 | Yes | < 0.00001 | Yes |
H8: Integration | 4.2 | 0.8367 | 2.6 | 1.1402 | / | / | 0.0176 | Yes | / | / |