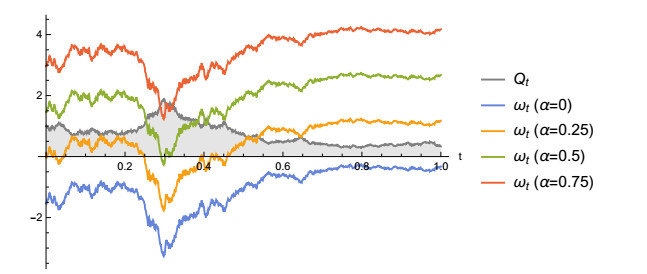
Concerning decisions for modern public transportation project, the lack of consensus between stakeholders and foreseeability of future transportation requirements might cause poor sustainability of the project. Unfortunately, many decision models give decision opinions without the test of the sustainability. Therefore, a dynamical Dijkstra simulation model is proposed to simulate the real traffic flows. In the model, the cost of the road connections is dynamically updated according to the change of the passenger flows. Then a combined decision support model using fuzzy AHP and dynamical Dijkstra simulation tests is designed. The combined model is capable of analyzing and creating consensus among different stakeholder participants in a transport development problem. The application of FAHP and dynamical Dijkstra ensures that the consensus creation is not only based on the FAHP decision making process but also on the response of the simulated execution of the decisions by dynamical Dijkstra. Thus, the decision makers by FAHP can firstly make their initial preferences in transportation planning, given the pairwise comparison matrices and generate the related weight for the traffic control parameters. And the dynamical Dijkstra simulations test the plan's setting and gives a response to iteratively adjust the FAHP matrices and parameters. The combined model is tested in different scenarios. And the results show that by the application of the proposed model, decision-makers can be more aware of the conflicts of interests among the involved groups, and they can pay more attention to possible violations causing by the change of traffic environment, including the citizen numbers, the construction cost, the roll cost, and etc., to get a more sustainable plan.
Citation: Xinlei Ma, Wen Chen, Zhan Gao, Tao Yang. Adaptive decision support model for sustainable transport system using fuzzy AHP and dynamical Dijkstra simulations[J]. Mathematical Biosciences and Engineering, 2022, 19(10): 9895-9914. doi: 10.3934/mbe.2022461
[1] | Yves Achdou, Ziad Kobeissi . Mean field games of controls: Finite difference approximations. Mathematics in Engineering, 2021, 3(3): 1-35. doi: 10.3934/mine.2021024 |
[2] | Benoît Perthame, Edouard Ribes, Delphine Salort . Career plans and wage structures: a mean field game approach. Mathematics in Engineering, 2019, 1(1): 38-54. doi: 10.3934/Mine.2018.1.38 |
[3] | Piermarco Cannarsa, Rossana Capuani, Pierre Cardaliaguet . C1;1-smoothness of constrained solutions in the calculus of variations with application to mean field games. Mathematics in Engineering, 2019, 1(1): 174-203. doi: 10.3934/Mine.2018.1.174 |
[4] | Pablo Blanc, Fernando Charro, Juan J. Manfredi, Julio D. Rossi . Games associated with products of eigenvalues of the Hessian. Mathematics in Engineering, 2023, 5(3): 1-26. doi: 10.3934/mine.2023066 |
[5] | Simone Paleari, Tiziano Penati . Hamiltonian lattice dynamics. Mathematics in Engineering, 2019, 1(4): 881-887. doi: 10.3934/mine.2019.4.881 |
[6] | Mario Pulvirenti . On the particle approximation to stationary solutions of the Boltzmann equation. Mathematics in Engineering, 2019, 1(4): 699-714. doi: 10.3934/mine.2019.4.699 |
[7] | Franco Flandoli, Eliseo Luongo . Heat diffusion in a channel under white noise modeling of turbulence. Mathematics in Engineering, 2022, 4(4): 1-21. doi: 10.3934/mine.2022034 |
[8] | Giacomo Canevari, Arghir Zarnescu . Polydispersity and surface energy strength in nematic colloids. Mathematics in Engineering, 2020, 2(2): 290-312. doi: 10.3934/mine.2020015 |
[9] | Zheming An, Nathaniel J. Merrill, Sean T. McQuade, Benedetto Piccoli . Equilibria and control of metabolic networks with enhancers and inhibitors. Mathematics in Engineering, 2019, 1(3): 648-671. doi: 10.3934/mine.2019.3.648 |
[10] | Jérôme Droniou, Jia Jia Qian . Two arbitrary-order constraint-preserving schemes for the Yang–Mills equations on polyhedral meshes. Mathematics in Engineering, 2024, 6(3): 468-493. doi: 10.3934/mine.2024019 |
Concerning decisions for modern public transportation project, the lack of consensus between stakeholders and foreseeability of future transportation requirements might cause poor sustainability of the project. Unfortunately, many decision models give decision opinions without the test of the sustainability. Therefore, a dynamical Dijkstra simulation model is proposed to simulate the real traffic flows. In the model, the cost of the road connections is dynamically updated according to the change of the passenger flows. Then a combined decision support model using fuzzy AHP and dynamical Dijkstra simulation tests is designed. The combined model is capable of analyzing and creating consensus among different stakeholder participants in a transport development problem. The application of FAHP and dynamical Dijkstra ensures that the consensus creation is not only based on the FAHP decision making process but also on the response of the simulated execution of the decisions by dynamical Dijkstra. Thus, the decision makers by FAHP can firstly make their initial preferences in transportation planning, given the pairwise comparison matrices and generate the related weight for the traffic control parameters. And the dynamical Dijkstra simulations test the plan's setting and gives a response to iteratively adjust the FAHP matrices and parameters. The combined model is tested in different scenarios. And the results show that by the application of the proposed model, decision-makers can be more aware of the conflicts of interests among the involved groups, and they can pay more attention to possible violations causing by the change of traffic environment, including the citizen numbers, the construction cost, the roll cost, and etc., to get a more sustainable plan.
The authors are honored to participate in this special issue and express their admiration to Professor Italo Capuzzo-Dolcetta for his mathematical work. The first author met Prof. Dolcetta for the first time at the end of his Ph.D. thesis more than 20 years ago during an extended visit to Roma. Prof. Dolcetta mentorship at that time influenced his career deeply and he is particularly grateful for this opportunity.
Mean-field games (MFG) is a tool to study the Nash equilibrium of infinite populations of rational agents. These agents select their actions based on their state and the statistical information about the population. Here, we study a price formation model for a commodity traded in a market under uncertain supply, which is a common noise shared by the agents. These agents are rational and aim to minimize the average trading cost by selecting their trading rate. The distribution of the agents solves a stochastic partial differential equation. Finally, a market-clearing condition characterizes the price.
We let (Ω,F,(Ft)0⩽t,P) be a complete filtered probability space such that (Ft)0⩽t is the standard filtration induced by t↦Wt, the common noise, which is a one-dimensional Brownian motion. We consider a commodity whose supply process is described by a stochastic differential equation; that is, we are given a drift bS:[0,T]×R2→R and volatility σS:[0,T]×R2→R+0, which are smooth functions, and the supply Qs is determined by the stochastic differential equation
dQs=bS(Qs,ϖs,s)ds+σS(Qs,ϖs,s)dWs in [0,T] | (1.1) |
with the initial condition ˉq. We would like to determine the drift bP:[0,T]×R2→R, the volatility σP:[0,T]×R2→R+0, and ˉw such that the price ϖs solves
dϖs=bP(Qs,ϖs,s)ds+σP(Qs,ϖs,s)dWs in [0,T] | (1.2) |
with initial condition ˉw and such that it ensures a market clearing condition. It may not be possible to find bP and σP in a feedback form. However, for linear dynamics, as we show here, we can solve quadratic models, which are of great interest in applications.
Let Xs be the quantity of the commodity held by an agent at time s for t⩽s⩽T. This agent trades this commodity, controlling its rate of change, v, thus
dXs=v(s)ds in [t,T]. | (1.3) |
At time t, an agent who holds x and observes q and w chooses a control process v, progressively measurable with respect to Ft, to minimize the expected cost functional
J(x,q,w,t;v)=E[∫TtL(Xs,v(s))+ϖsv(s)ds+Ψ(XT,QT,ϖT)], | (1.4) |
subject to the dynamics (1.1), (1.2), and (1.3) with initial condition Xt=x, and the expectation is taken w.r.t. Fr. The Lagrangian, L, takes into account costs such as market impact or storage, and the terminal cost Ψ stands for the terminal preferences of the agent.
This control problem determines a Hamilton-Jacobi equation addressed in Section 2.1. In turn, each agent selects an optimal control and uses it to adjust its holdings. Because the source of noise in Qt is common to all agents, the evolution of the probability distribution of agents is not deterministic. Instead, it is given by a stochastic transport equation derived in Section 2.2. Finally, the price is determined by a market-clearing condition that ensures that supply meets demand. We study this condition in Section 2.3.
Mathematically, the price model corresponds to the following problem.
Problem 1. Given a Hamiltonian, H:R2→R, H∈C∞, a commodity's supply initial value, ˉq∈R, supply drift, bS:R2×[0,T]→R, and supply volatility, σS:R2×[0,T]→R, a terminal cost, Ψ:R3→R, Ψ∈C∞(R3), and an initial distribution of agents, ˉm∈C∞c(R)∩P(R), find u:R3×[0,T]→R, μ∈C([0,T]×Ω;P(R3)), ˉw∈R, the price at t=0, the price drift bP:R2×[0,T]→R, and the price volatility σP:R2×[0,T]→R solving
{−ut+H(x,w+ux)=bSuq+bPuw+12(σS)2uqq+σSσPuqw+12(σP)2uwwdμt=((μ(σS)22)qq+(μσSσP)qw+(μ(σP)22)ww−div(μb))dt−div(μσ)dWt∫R3q+DpH(x,w+ux(x,q,w,t))μt(dx×dq×dw)=0,a.e.ω∈Ω,0⩽t⩽T, | (1.5) |
and the terminal-initial conditions
{u(x,q,w,T)=Ψ(x,q,w)μ0=ˉm×δˉq×δˉw, | (1.6) |
where b=(−DpH(x,w+ux),bS,bP), σ=(0,σS,σP), and the divergence is taken w.r.t. (x,q,w).
Given a solution to the preceding problem, we construct the supply and price processes
Qt=∫R3qμt(dx×dq×dw) |
and
ϖt=∫R3wμt(dx×dq×dw), |
which also solve
{dQt=bS(Qt,ϖt,t)dt+σS(Qt,ϖt,t)dWt in [0,T]dϖt=bP(Qt,ϖt,t)dt+σP(Qt,ϖt,t)dWt in [0,T] |
with initial conditions
{Q0=ˉqϖ0=ˉw | (1.7) |
and satisfy the market-clearing condition
Qt=∫R−DpH(x,ϖt+ux(x,Qt,ϖt,t))μt(dx). |
In [10], the authors presented a model where the supply for the commodity was a given deterministic function, and the balance condition between supply and demand gave rise to the price as a Lagrange multiplier. Price formation models were also studied by Markowich et al. [18], Caffarelli et al. [2], and Burger et al. [1]. The behavior of rational agents that control an electric load was considered in [16,17]. For example, turning on or off space heaters controls the electric load as was discussed in [13,14,15]. Previous authors addressed price formation when the demand is a given function of the price [12] or that the price is a function of the demand, see, for example [5,6,7,8,11]. An N-player version of an economic growth model was presented in [9].
Noise in the supply together with a balance condition is a central issue in price formation that could not be handled directly with the techniques in previous papers. A probabilistic approach of the common noise is discussed in Carmona et al. in [4]. Another approach is through the master equation, involving derivatives with respect to measures, which can be found in [3]. None of these references, however, addresses problems with integral constraints such as (1.7).
Our model corresponds to the one in [10] for the deterministic setting when we take the volatility for the supply to be 0. Here, we study the linear-quadratic case, that is, when the cost functional is quadratic, and the dynamics (1.1) and (1.2) are linear. In Section 3.2, we provide a constructive approach to get semi-explicit solutions of price models for linear dynamics and quadratic cost. This approach avoids the use of the master equation. The paper ends with a brief presentation of simulation results in Section 4.
In this section, we derive Problem 1 from the price model. We begin with standard tools of optimal control theory. Then, we derive the stochastic transport equation, and we end by introducing the market-clearing (balance) condition.
The value function for an agent who at time t holds an amount x of the commodity, whose instantaneous supply and price are q and w, is
u(x,q,w,t)=infvJ(x,q,w,t;v) | (2.1) |
where J is given by (1.4) and the infimum is taken over the set A((t,T]) of all functions v:[t,T]→R, progressively measurable w.r.t. (Fs)t⩽s⩽T. Consider the Hamiltonian, H, which is the Legendre transform of L; that is, for p∈R,
H(x,p)=supv∈R[−pv−L(x,v)]. | (2.2) |
Then, from standard stochastic optimal control theory, whenever L is strictly convex, if u is C2, it solves the Hamilton-Jacobi equation in R3×[0,T)
−ut+H(x,w+ux)−bPuw−bSuq−(σP)22uww−(σS)22uqq−σPσSuwq=0 | (2.3) |
with the terminal condition
u(x,q,w,T)=Ψ(x,q,w). | (2.4) |
Moreover, as the next verification theorem establishes, any C2 solution of (2.3) is the value function.
Theorem 2.1(Verification). Let ˜u:[0,T]×R3→R be a smooth solution of (2.3) with terminal condition (2.4). Let (X∗,Q,ϖ) solve (1.3), (1.1) and (1.2), where X∗ is driven by the (Ft)0⩽t-progressively measurable control
v∗(s):=−DpH(X∗s,ϖs+˜ux(X∗s,Qs,ϖs,s)). |
Then
1). v∗ is an optimal control for (2.1)
2). ˜u=u, the value function.
Theorem 2.1 provides an optimal feedback strategy. As usual in MFG, we assume that the agents are rational and, hence, choose to follow this optimal strategy. This behavior gives rise to a flow that transports the agents and induces a random measure that encodes their distribution. Here, we derive a stochastic PDE solved by this random measure. To this end, let u solve (2.3) and consider the random flow associated with the diffusion
{dXs=−DpH(Xs,ϖs+ux(Xs,Qs,ϖs,s))dsdQs=bS(Qs,ϖs,s)ds+σS(Qs,ϖs,s)dWsdϖs=bP(Qs,ϖs,s)ds+σP(Qs,ϖs,s)dWs | (2.5) |
with initial conditions
{X0=xQ0=ˉqϖ0=ˉw. |
That is, for a given realization ω∈Ω of the common noise, the flow maps the initial conditions (x,ˉq,ˉw) to the solution of (2.5) at time t, which we denote by (Xωt(x,ˉq,ˉw),Qωt(ˉq,ˉw),ϖωt(ˉq,ˉw)). Using this map, we define a measure-valued stochastic process μt as follows:
Definition 2.2. Let ω∈Ω denote a realization of the common noise W on 0⩽s⩽T. Given a measure ˉm∈P(R) and initial conditions ˉq,ˉw∈R take ˉμ∈P(R3) by ˉμ=ˉm×δˉq×δˉw and define a random measure μt by the mapping ω↦μωt∈P(R3), where μωt is characterized as follows:
for any bounded and continuous function ψ:R3→R
∫R3ψ(x,q,w)μωt(dx×dq×dw)=∫R3ψ(Xωt(x,q,w),Qωt(q,w),ϖωt(q,w))ˉμ(dx×dq×dw). |
Remark 2.3. Because ˉμ=ˉm×δˉq×δˉw, we have
∫R3ψ(Xωt(x,q,w),Qωt(q,w),ϖωt(q,w))ˉμ(dx×dq×dw)=∫Rψ(Xωt(x,ˉq,ˉw),Qωt(ˉq,ˉw),ϖωt(ˉq,ˉw))ˉm(dx). |
Moreover, due to the structure of (2.5),
μωt=(Xωt(x,ˉq,ˉw)#ˉm)×δQωt(ˉq,ˉw)×δϖωt(ˉq,ˉw). |
Definition 2.4. Let ˉμ∈P(R3) and write
b(x,q,w,s)=(−DpH(x,w+ux(x,q,w,s)),bS(q,w,s),bP(q,w,s)),σ(q,w,s)=(0,σS(q,w,s),σP(q,w,s)). |
A measure-valued stochastic process μ=μ(⋅,t)=μt(⋅) is a weak solution of the stochastic PDE
dμt=(−div(μb)+(μ(σS)22)qq+(μσSσP)qw+(μ(σP)22)ww)dt−div(μσ)dWt, | (2.6) |
with initial condition ˉμ if for any bounded smooth test function ψ:R3×[0,T]→R
∫R3ψ(x,q,w,t)μt(dx×dq×dw)=∫R3ψ(x,q,w,0)ˉμ(dx×dq×dw) | (2.7) |
+∫t0∫R3∂tψ+Dψ⋅b+12tr(σTσD2ψ)μs(dx×dq×dw)ds | (2.8) |
+∫t0∫R3Dψ⋅σμs(dx×dq×dw)dWs, | (2.9) |
where the arguments for b, σ and ψ are (x,q,w,s) and the differential operators D and D2 are taken w.r.t. the spatial variables x,q,w.
Theorem 2.5. Let ˉm∈P(R) and ˉq,ˉw∈R. The random measure from Definition 2.2 is a weak solution of the stochastic partial differential equation (2.6) with initial condition ˉμ=ˉm×δˉq×δˉw.
Proof. Let ψ:R3×[0,T]→R be a bounded smooth test function. Consider the stochastic process s↦∫R3ψ(x,q,w,s)μωs(dx×dq×dw). Let
(Xt(x,ˉq,ˉw),Qt(ˉq,ˉw),ϖt(ˉq,ˉw)) |
be the flow induced by (2.5). By the definition of μωt,
∫R3ψ(x,q,w,t)μωt(dx×dq×dw)−∫R3ψ(x,q,w,0)ˉμ(dx×dq×dw)=∫R[ψ(Xωt(x,ˉq,ˉw),Qωt(ˉq,ˉw),ϖωt(ˉq,ˉw),t)−ψ(x,ˉq,ˉw,0)]ˉm(dx). |
Then, applying Ito's formula to the stochastic process
s↦∫Rψ(Xs(x,ˉq,ˉw),Qs(ˉq,ˉw),ϖs(ˉq,ˉw),s)ˉm(dx), |
the preceding expression becomes
∫t0d(∫Rψ(Xs(x,ˉq,ˉw),Qs(ˉq,ˉw),ϖs(ˉq,ˉw),s)ˉm(dx))=∫t0∫R[Dtψ+Dψ⋅b+12tr(σTσD2ψ)]ˉm(dx)ds+∫t0∫RDψ⋅σˉm(dx)dWs=∫t0∫R3[Dtψ+Dψ⋅b+12tr(σTσD2ψ)]μs(dx×dq×dw)ds+∫t0∫R3Dψ⋅σμs(dx×dq×dw)dWs, |
where arguments of b, σ and the partial derivatives of ψ in the integral with respect to ˉm(dx) are (Xs(x,ˉq,ˉw),Qs(ˉq,ˉw),ϖs(ˉq,ˉw),s), and in the integral with respect to μt(dx×dq×dw) are (x,q,w,t). Therefore,
∫R3ψ(x,q,w,t)μωt(dx×dq×dw)−∫R3ψ(x,q,w,0)ˉμ(dx×dq×dw)=∫t0∫R3[Dtψ+Dψ⋅b+12tr(D2ψ:(σ,σ))]μωs(dx×dq×dw)ds+∫t0∫R3Dψ⋅σμωs(dx×dq×dw)dWs. |
Hence, (2.7) holds.
The balance condition requires the average trading rate to be equal to the supply. Because agents are rational and, thus, use their optimal strategy, this condition takes the form
Qt=∫R3−DpH(x,w+ux(x,q,w,t))μωt(dx×dq×dw), | (2.10) |
where μωt is given by Definition 2.2. Because Qt satisfies a stochastic differential equation, the previous can also be read in differential form as
bS(Qt,ϖt,t)dt+σS(Qt,ϖt,t)dWt=d∫R3−DpH(x,w+ux(x,q,w,t))μωt(dx×dq×dw). | (2.11) |
The former condition determines bP and σP. In general, bP and σP are only progressively measurable with respect to (Ft)0⩽t and not in feedback form. In this case, the Hamilton–Jacobi (2.3) must be replaced by either a stochastic partial differential equation or the problem must be modeled by the master equation. However, as we discuss next, in the linear-quadratic case, we can find bP and σP in feedback form.
Here, we consider a price model for linear dynamics and quadratic cost. The Hamilton-Jacobi equation admits quadratic solutions. Then, the balance equation determines the dynamics of the price, and the model is reduced to a first-order system of ODE.
Suppose that L(x,v)=c2v2 and, thus, H(x,p)=12cp2. Accordingly, the corresponding MFG model is
{−ut+12c(w+ux)2−bPuw−bSuq−12(σP)2uww−12(σS)2uqq−σPσSuwq=0dμt=((μ(σS)22)qq+(μσSσP)qw+(μ(σP)22)ww−div(μb))dt−div(μσ)dWtQt=−1cϖt+∫R−1cux(x,q,w,t)μωt(dx×dq×dw). | (3.1) |
Assume further that Ψ is quadratic; that is,
Ψ(x,q,w)=c0+c11x+c21q+c31w+c12x2+c22xq+c32xw+c42q2+c52qw+c62w2. |
Let
Πt=∫R3ux(x,q,w,t)μt(dx×dq×dw). |
The balance condition is Qt=−1c(ϖt+Πt). Furthermore, Definition 2.2 provides the identity
Πt=∫Rux(X∗t(x,ˉq,ˉw),Qt(ˉq,ˉw),ϖt(ˉq,ˉw),t)ˉm(dx). |
Lemma 3.1. Let (X∗,Q,ϖ) solve (1.3), (1.1) and (1.2) with v=v∗, the optimal control, and initial conditions ˉq,ˉw∈R. Let u∈C3(R3×[0,T]) solve the Hamilton-Jacobi equation (2.3). Then
dΠt=∫R(uxqσS+uxwσP)ˉm(dx)dWt, | (3.2) |
where the arguments for the partial derivatives of u are (X∗t(x,ˉq,ˉw),Qt(ˉq,ˉw),ϖt(ˉq,ˉw),t).
Proof. By Itô's formula, the process t↦ux(X∗t,Qt,ϖt,t) solves
d(ux(X∗t,Qt,ϖt,t))=(uxt+uxxv∗+uxqbS+uxwbP+uxqq12(σS)2+uxqwσSσP+uxww12(σP)2)dt++(uxqσS+uxwσP)dWt, | (3.3) |
with v∗(t)=−1c(ϖt+ux(X∗t,Qt,ϖt,t)). By differentiating the Hamilton-Jacobi equation, we get
−utx+1c(ϖt+ux)uxx−bPuwx−bSuqx−(σP)22uwwx−(σS)22uqqx−σPσSuwqx=0. |
Substituting the previous expression in (3.3), we have
d(∫Rux(X∗t(x,ˉq,ˉw),Qt(ˉq,ˉw),ϖt(ˉq,ˉw),t)ˉm(dx))=∫R(1c(ϖt+ux)uxx+uxxv∗)ˉm(dx)dt+∫R(uxqσS+uxwσP)ˉm(dx)dWt. |
The preceding identity simplifies to
∫R(uxqσS+uxwσP)ˉm(dx)dWt. |
Using Lemma 3.1, we have
−cdQt=∫R(uxqσS+uxwσP)ˉm(dx)dWt+dϖt; |
that is,
−cbSdt−cσSdWt=(σS∫Ruxqˉm(dx)+σP∫Ruxwˉm(dx))dWt+dϖt=bPdt+(σS∫Ruxqˉm(dx)+σP∫Ruxwˉm(dx)+σP)dWt. |
Thus,
bP=−cbS,σP=−σSc+∫Ruxqˉm(dx)1+∫Ruxwˉm(dx). | (3.4) |
If u is a second-degree polynomial with time-dependent coefficients, then
∫Ruxq(X∗t(x,ˉq,ˉw),Qt(ˉq,ˉw),ϖt(ˉq,ˉw),t)ˉm(dx) |
and
∫Ruxw(X∗t(x,ˉq,ˉw),Qt(ˉq,ˉw),ϖt(ˉq,ˉw),t)ˉm(dx) |
are deterministic functions of time. Accordingly, bP and σP are given in feedback form by (3.4), thus, consistent with the original assumption. Here, we investigate the linear-quadratic case that admits solutions of this form.
Now, we assume that the dynamics are affine; that is,
{bP(t,q,w)=bP0(t)+qbP1(t)+wbP2(t)bS(t,q,w)=bS0(t)+qbS1(t)+wbS2(t)σP(t,q,w)=σP0(t)+qσP1(t)+wσP2(t)σS(t,q,w)=σS0(t)+qσS1(t)+wσS2(t). | (3.5) |
Then, (3.4) gives
bP0=−cbS0,σP0=−σS0c+∫Ruxqˉm(dx)1+∫Ruxwˉm(dx)bP1=−cbS1,σP1=−σS1c+∫Ruxqˉm(dx)1+∫Ruxwˉm(dx)bP2=−cbS2,σP2=−σS2c+∫Ruxqˉm(dx)1+∫Ruxwˉm(dx). |
Because all the terms in the Hamilton-Jacobi equation are at most quadratic, we seek for solutions of the form
u(t,x,q,w)=a0(t)+a11(t)x+a21(t)q+a31(t)w+a12(t)x2+a22(t)xq+a32(t)xw+a42(t)q2+a52(t)qw+a62(t)w2, |
where aji:[0,T]→R. Therefore, the previous identities reduce to
bP0=−cbS0,σP0=−σS0c+a221+a32bP1=−cbS1,σP1=−σS1c+a221+a32bP2=−cbS2,σP2=−σS2c+a221+a32. | (3.6) |
Using (3.6) and grouping coefficients in the Hamilton-Jacobi PDE, we obtain the following ODE system
˙a12=2(a12)2c˙a22=c2a32bS1−ca22bS1+2a12a22c˙a32=c2a32bS2−ca22bS2+2a12+2a12a32c˙a11=c2a32bS0−ca22bS0+2a11a12c˙a42=ca52bS1−2a42bS1+a52(a22+c)(σS1)2a32+1−14(4a62(a22+c)2(σS1)2(a32+1)2+4a42(σS1)2)+(a22)22c˙a52=2ca62bS1+ca52bS2−a52bS1−2a42bS2−12(4a62(a22+c)2σS1σS2(a32+1)2+4a42σS1σS2)+2a52(a22+c)σS1σS2a32+1+a22(a32+1)c˙a62=2ca62bS2−a52bS2−14(4a62(a22+c)2(σS2)2(a32+1)2+4a42(σS2)2)+a52(a22+c)(σS2)2a32+1+(a32+1)22c˙a0=ca31bS0−a21bS0+a52(a22+c)(σS0)2a32+1−12(2a62(a22+c)2(σS0)2(a32+1)2+2a42(σS0)2)+(a11)22c˙a21=ca52bS0+ca31bS1−2a42bS0−a21bS1+2a52(a22+c)σS0σS1a32+1−12(4a62(a22+c)2σS0σS1(a32+1)2+4a42σS0σS1)+a11a22c˙a31=2ca62bS0+ca31bS2−a52bS0−a21bS2−12(4a62(a22+c)2σS0σS2(a32+1)2+4a42σS0σS2)+2a52(a22+c)σS0σS2a32+1+a11(a32+1)c, |
with terminal conditions
a0(T)=Ψ(0,0,0)=c0a11(T)=DxΨ(0,0,0)=c11a21(T)=DqΨ(0,0,0)=c21a31(T)=DwΨ(0,0,0)=c31a12(T)=12DxxΨ(0,0,0)=c12a22(T)=DxqΨ(0,0,0)=c22a32(T)=DxwΨ(0,0,0)=c32a42(T)=12DqqΨ(0,0,0)=c42a52(T)=DqwΨ(0,0,0)=c52a62(T)=12DwwΨ(0,0,0)=c62. |
While this system has a complex structure, it admits some simplifications. For example, the equation for a12 is independent of other terms and has the solution
a12(t)=cc12c+2c12(T−t). |
Moreover, we can determine a22 and a32 from the linear system
ddt[a22a32]=[−bS1+2ca12cbS1−bS2cbS2+2ca12][a22a32]+[02ca12]. |
Lemma 3.1 takes the form
dΠt=(a22(t)σS(Qt,ϖt,t)+a32(t)σP(Qt,ϖt,t))dWt. |
Therefore,
Πt=Π0+∫t0(a22(r)σS(Qr,ϖr,r)+a32(r)σP(Qr,ϖr,r))dWr |
where
Π0=a11(0)+2a12(0)∫Rxˉm(dx)+a22(0)ˉq+a32(0)ˉw. |
Replacing the above in the balance condition at the initial time, that is ˉw=−cˉq−Π0, we obtain the initial condition for the price
\begin{equation} \bar{w} = \tfrac{-1}{1+a_2^3(0)}\Big(a_1^1(0) + 2a_2^1(0) \int_{ {\mathbb{R}}}x\bar{m}(dx) + (a_2^2(0)+c) \bar{q}\Big). \end{equation} | (3.7) |
where a_1^1 can be obtained after solving for a_2^1 , a_2^2 and a_2^3 .
Now, we proceed with the price dynamics using the balance condition. Under linear dynamics, we have
\begin{align*} Q_t& = -\tfrac{1}{c} \left(\varpi_t+\Pi_0\right) \\ & -\tfrac{1}{c} \int_0^t a_2^2(r) \Big(\sigma_0^S(r) +Q_r \sigma _1^S(r)+\varpi_r \sigma _2^S(r) \Big) + a_2^3 (r)\Big(\sigma_0^P(r)+Q_r \sigma _1^P(r)+\varpi_r \sigma _2^P(r) \Big) dW_r. \end{align*} |
Thus, replacing the price coefficients for (3.6), we obtain
\begin{align*} d\varpi_t = &-c\left( b_0^S(t)+ b_1^S(t)Q_t+ b_2^S(t)\varpi_t\right) dt \\ &- \tfrac{c+a_2^2(t)}{1+a_2^3(t)} \left(\sigma_0^S(t)+\sigma_1^S(t) Q_t+\sigma_2^S(t) \varpi_t\right)dW_t, \\ dQ_t = &b^S dt + \sigma^S dW_t, \end{align*} |
which determines the dynamics for the price.
In this section, we consider the running cost corresponding to c = 1 ; that is,
L( {\bf v}) = \tfrac{1}{2} {\bf v}^2 |
and terminal cost at time T = 1
\Psi(x) = (x-\alpha)^2. |
We take \bar{m} to be a normal standard distribution; that is, with zero-mean and unit variance. We assume the dynamics for the normalized supply is mean-reverting
dQ_t = (1-Q_t) dt + Q_t dW_t, |
with initial condition \bar{q} = 1 . Therefore, the dynamics for the price becomes
d\varpi_t = -(1-Q_t) dt - \tfrac{1+a_2^2}{1+a_2^3} Q_t dW_t, |
with initial condition \bar{w} given by (3.7), and a_2^2 and a_2^3 solve
\begin{align*} \dot{a}_2^2 = & -a_2^3 + a_2^2 (1+2 a_2^1) \\ \dot{a}_2^3 = & 2 a_2^1(1+a_2^3), \end{align*} |
with terminal conditions a_2^2(1) = 0 and a_2^3(1) = 0 . We observe that the coefficient multiplying Q_t in the volatility of the price is now time-dependent.
For a fixed simulation of the supply, we compute the price for different values of \alpha . Agents begin with zero energy average. The results are displayed in Figure 1. As expected, the price is negatively correlated with the supply. Moreover, as the storage target increases, prices increase, which reflects the competition between agents who, on average, want to increase their storage.
The authors were partially supported by KAUST baseline funds and KAUST OSR-CRG2017-3452.
All authors declare no conflicts of interest in this paper.
[1] |
A. A. Rassafi, M. Vaziri, Sustainable transport indicators: definition and integration, Int. J. Environ. Sci. Technol., 2 (2005), 83–96. https://doi.org/10.1007/BF03325861 doi: 10.1007/BF03325861
![]() |
[2] |
T. A. Shiau, Evaluating transport infrastructure decisions under uncertainty, Transp. Plann. Technol., 37 (2014), 525–538. http://dx.doi.org/10.1080/03081060.2014.921405 doi: 10.1080/03081060.2014.921405
![]() |
[3] | K. Zhou, X. Peng, Z. Guo, Evaluation method of coupling between urban spatial structure and public transport system, in 2019 5th International Conference on Transportation Information and Safety (ICTIS), (2019), 723–729. http://dx.doi.org/10.1109/ICTIS.2019.8883745 |
[4] |
M. Das, A. Roy, S. Maity, S. Kar, S. Sengupta, Solving fuzzy dynamic ship routing and scheduling problem through new genetic algorithm, Decis. Making: Appl. Manage. Eng., 2021. https://doi.org/10.31181/dmame181221030d doi: 10.31181/dmame181221030d
![]() |
[5] |
Ö. N. Çam, H. K. Sezen, The formulation of a linear programming model for the vehicle routing problem in order to minimize idle time, Decis. Making: Appl. Manage. Eng., 3 (2020), 22–29. https://doi.org/10.31181/dmame2003132h doi: 10.31181/dmame2003132h
![]() |
[6] | O. Aslan, A. Yazici, Conflict-free route planning for autonomous transport vehicle, in 2020 28th Signal Processing and Communications Applications Conference (SIU), (2020), 1–4. https://doi.org/10.1109/SIU49456.2020.9302504 |
[7] | O. Surnin, A. Ivaschenko, P. Sitnikov, A. Suprun, A. Stolbova, O. Golovnin, Urban public transport digital planning based on an intelligent transportation system, in 2019 25th Conference of Open Innovations Association (FRUCT), (2019), 292–298. https://doi.org/10.23919/FRUCT48121.2019.8981507 |
[8] | I. Estalayo, E. Osaba, I. Laña, J. D. Ser, Deep recurrent neural networks and optimization meta-heuristics for green urban route planning with dynamic traffic estimates, in 2019 IEEE Intelligent Transportation Systems Conference (ITSC), (2019), 1336–1342. https://doi.org/10.1109/ITSC.2019.8916957 |
[9] |
S. Duleba, S. Moslem, Sustainable Urban transport development with stakeholder participation, an AHP-Kendall model: A case study for Mersin, Sustainability, 10 (2018), 36–47. http://doi.org/10.3390/su10103647 doi: 10.3390/su10103647
![]() |
[10] |
S. Duleba, S. Moslem, Examining Pareto optimality in analytic hierarchy process on real data: An application in public transport service development, Expert Syst., 116 (2019), 21–30. https://doi.org/10.1016/j.eswa.2018.08.049 doi: 10.1016/j.eswa.2018.08.049
![]() |
[11] |
M. Balaji, S. Santhanakrishnan, S. N. Dinesh, An application of analytic hierarchy process in vehicle routing problem, Period. Polytech. Transp. Eng., 47 (2019), 196–205. http://doi.org/10.3311/PPtr.10701 doi: 10.3311/PPtr.10701
![]() |
[12] |
S. Duleba, An AHP-ISM approach for considering public preferences in a public transport development decision, Transport, 6 (2019), 1–10. http://doi.org/10.3846/transport.2019.9080 doi: 10.3846/transport.2019.9080
![]() |
[13] |
H. Upadhyay, S. Juneja, A. Juneja, G. Dhiman, S. Kautish, Evaluation of ergonomics-related disorders in online education using fuzzy AHP, Comput. Intell. Neurosci., 2021 (2021), 1–11. https://doi.org/10.1155/2021/2214971 doi: 10.1155/2021/2214971
![]() |
[14] | O. Ghorbanzadeh, B. Feizizadeh, T. Blaschke, R. Khosravi, Spatially explicit sensitivity and uncertainty analysis for the landslide risk assessment of the gas pipeline networks, in Proceedings of the 21st AGILE conference on Geo-information science, (2018), 12–15. |
[15] |
M. M. De Brito, M. Evers, A. D. S. Almoradie, Participatory flood vulnerability assessment: A multi-criteria approach, Hydrol. Earth Syst. Sci., 22 (2018), 373–390. http://doi.org/10.5194/hess-22-373-2018 doi: 10.5194/hess-22-373-2018
![]() |
[16] |
H. Jin, M. Zhang, Y. Yuan, Analytic network process-based multi-criteria decision approach and sensitivity analysis for temporary facility layout planning in construction projects, Appl. Sci., 8 (2018), 24–34. https://doi.org/10.3390/app8122434 doi: 10.3390/app8122434
![]() |
[17] |
M. Hervás-Peralta, S. Poveda-Reyes, G. D. Molero, F. E. Santarremigia, J. P. Pastor-Ferrando, Improving the performance of dry and maritime ports by increasing knowledge about the most relevant functionalities of the terminal operating system (TOS), Sustainability, 11 (2019), 1–23. https://doi.org/10.3390/su11061648 doi: 10.3390/su11061648
![]() |
[18] |
O. Ghorbanzadeh, B. Feizizadeh, T. Blaschke, Multi-criteria risk evaluation by integrating an analytical network process approach into GIS-based sensitivity and uncertainty analyses, Geomat. Nat. Hazards Risk, 9 (2018), 127–151. https://doi.org/10.1080/19475705.2017.1413012 doi: 10.1080/19475705.2017.1413012
![]() |
[19] |
S. R. Meena, O. Ghorbanzadeh, T. Blaschke, A comparative study of statistics-based landslide susceptibility models: A case study of the region affected by the Gorkha earthquake in Nepal, ISPRS Int. J. Geo-Inf., 8 (2019). https://doi.org/10.3390/ijgi8020094 doi: 10.3390/ijgi8020094
![]() |
[20] |
A. Hawbani, E. Torbosh, X. Wang, P. Sincak, L. Zhao, A. Al-Dubai, Fuzzy-based distributed protocol for vehicle-to-vehicle communication, IEEE Trans. Fuzzy Syst., 29 (2021), 612–626. https://doi.org/10.1109/TFUZZ.2019.2957254 doi: 10.1109/TFUZZ.2019.2957254
![]() |
[21] |
S. Moslem, O. Ghorbanzadeh, T. Blaschke, S. Duleba, Analysing stakeholder consensus for a sustainable transport development decision by the fuzzy AHP and interval AHP, Sustainability, 11 (2019), 1–22. http://doi.org/10.3390/su11123271 doi: 10.3390/su11123271
![]() |
[22] |
V. Mohagheghi, S. M. Mousavi, M. Aghamohagheghi, B. Vahdani, A new approach of multi-criteria analysis for the evaluation and selection of sustainable transport investment projects under uncertainty: A case study, Int. J. Comput. Intell. Syst., 10 (2017), 605–626. https://doi.org/10.2991/ijcis.2017.10.1.41 doi: 10.2991/ijcis.2017.10.1.41
![]() |
[23] |
P. Ziemba, Selection of electric vehicles for the needs of sustainable transport under conditions of uncertainty—a comparative study on fuzzy MCDA Methods, Energies, 14 (2021), 7786. https://doi.org/10.3390/en14227786 doi: 10.3390/en14227786
![]() |
[24] |
B. Buran, M. Erçek, Public transportation business model evaluation with Spherical and Intuitionistic Fuzzy AHP and sensitivity analysis, Expert Syst. Appl., 204 (2022), 117519. https://doi.org/10.1016/j.eswa.2022.117519 doi: 10.1016/j.eswa.2022.117519
![]() |
[25] |
J. Wątróbski, K. Małecki, K. Kijewska, S. Iwan, A. Karczmarczyk, R. G. Thompson, Multi-criteria analysis of electric vans for city logistics, Sustainability, 9 (2017), 1453. https://doi.org/10.3390/su9081453 doi: 10.3390/su9081453
![]() |
[26] |
M. H. Ha, Z. Yang, M. W. Heo, A new hybrid decision making framework for prioritising port performance improvement strategies, Asian J. Shipp. Logist., 33 (2017), 105–116. https://doi.org/10.1016/j.ajsl.2017.09.001 doi: 10.1016/j.ajsl.2017.09.001
![]() |
[27] |
M. Yavuz, B. Oztaysi, S. C. Onar, C. Kahraman, Multi-criteria evaluation of alternative fuel vehicles via a hierarchical hesitant fuzzy linguistic model, Expert Syst., 42 (2015), 2835–2848. https://doi.org/10.1016/j.eswa.2014.11.010 doi: 10.1016/j.eswa.2014.11.010
![]() |
[28] |
U. R. Tuzkaya, Evaluating the environmental effects of transportation modes using an integrated methodology and an application, Int. J. Environ. Sci. Tech., 6 (2009), 277–290. http://doi.org/10.1007/BF03327632 doi: 10.1007/BF03327632
![]() |
[29] |
A. Awasthi, S. SChauhan, A hybrid approach integrating Affinity Diagram, AHP and fuzzy TOPSIS for sustainable city logistics planning, Appl. Math. Modell., 36 (2012), 573–584. https://doi.org/10.1016/j.apm.2011.07.033 doi: 10.1016/j.apm.2011.07.033
![]() |
[30] |
W. Pedrycz, Analytic hierarchy process (AHP) in group decision making and its optimization with an allocation of information granularity, IEEE Trans. Fuzzy Syst., 19 (2011), 527–539. https://doi.org/10.1109/TFUZZ.2011.2116029 doi: 10.1109/TFUZZ.2011.2116029
![]() |
[31] |
T. T. Nguyen, L. Gordon-Brown, Constrained fuzzy hierarchical analysis for portfolio selection under higher moments, IEEE Trans. Fuzzy Syst., 20 (2012), 666–682. https://doi.org/10.1109/TFUZZ.2011.2181520 doi: 10.1109/TFUZZ.2011.2181520
![]() |
[32] |
K. T. T. Bui, D. T. Bui, J. Zou, C. Van Doan, I. Revhaug, A novel hybrid artificial intelligent approach based on neural fuzzy inference model and particle swarm optimization for horizontal displacement modeling of hydropower dam, Neural Comput., 29 (2018), 1495–1506. https://doi.org/10.1007/s00521-016-2666-0 doi: 10.1007/s00521-016-2666-0
![]() |
1. | Masaaki Fujii, Akihiko Takahashi, Strong Convergence to the Mean Field Limit of a Finite Agent Equilibrium, 2022, 13, 1945-497X, 459, 10.1137/21M1441055 | |
2. | Masaaki Fujii, Akihiko Takahashi, Strong Convergence to the Mean-Field Limit of A Finite Agent Equilibrium, 2021, 1556-5068, 10.2139/ssrn.3905899 | |
3. | Diogo Gomes, Julian Gutierrez, Ricardo Ribeiro, A Random-Supply Mean Field Game Price Model, 2023, 14, 1945-497X, 188, 10.1137/21M1443923 | |
4. | Yuri Ashrafyan, Tigran Bakaryan, Diogo Gomes, Julian Gutierrez, 2022, The potential method for price-formation models, 978-1-6654-6761-2, 7565, 10.1109/CDC51059.2022.9992621 | |
5. | Diogo Gomes, Julian Gutierrez, Mathieu Laurière, Machine Learning Architectures for Price Formation Models, 2023, 88, 0095-4616, 10.1007/s00245-023-10002-8 | |
6. | Matt Barker, Pierre Degond, Ralf Martin, Mirabelle Muûls, A mean field game model of firm-level innovation, 2023, 33, 0218-2025, 929, 10.1142/S0218202523500203 | |
7. | Khaled Aljadhai, Majid Almarhoumi, Diogo Gomes, Melih Ucer, A mean-field game model of price formation with price-dependent agent behavior, 2024, 1982-6907, 10.1007/s40863-024-00465-0 | |
8. | Diogo Gomes, Julian Gutierrez, Mathieu Laurière, 2023, Machine Learning Architectures for Price Formation Models with Common Noise, 979-8-3503-0124-3, 4345, 10.1109/CDC49753.2023.10383244 | |
9. | Masaaki Fujii, Masashi Sekine, Mean-Field Equilibrium Price Formation With Exponential Utility, 2023, 1556-5068, 10.2139/ssrn.4420441 | |
10. | Yuri Ashrafyan, Diogo Gomes, A Fully-Discrete Semi-Lagrangian Scheme for a Price Formation MFG Model, 2025, 2153-0785, 10.1007/s13235-025-00620-y | |
11. | Masaaki Fujii, Masashi Sekine, Mean-field equilibrium price formation with exponential utility, 2024, 24, 0219-4937, 10.1142/S0219493725500017 | |
12. | Masaaki Fujii, Masashi Sekine, Mean Field Equilibrium Asset Pricing Model with Habit Formation, 2025, 1387-2834, 10.1007/s10690-024-09507-1 |