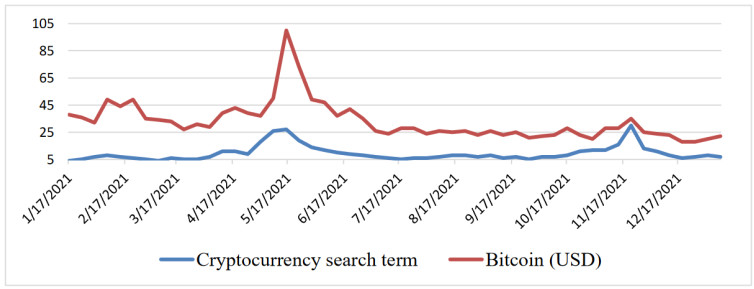
Soluble Fas ligand (sFasL, sCD95L) and its specific soluble binders, soluble Fas receptor (sFas, sCD95) and decoy receptor 3 (DcR3), have been investigated as possible clinical biomarkers in many serious diseases. The present review aimed to provide an overview of the current state of this medically promising research by extensively examining the relevant literature. The summarized results of the survey are presented after classification into six categories according to the type of targeted disease. To date, the studies have been mainly devoted to the diagnosis of disease severity states and prognosis of treatments about various types of cancers and autoimmune diseases represented by autoimmune lymphoproliferative syndrome and systemic lupus erythematosus, because these important life-threatening or intractable diseases were suggested to be most relevant to the impairment of apoptotic cell death-inducing systems, including the Fas receptor-mediated signaling system, and the mechanisms responsible for their onset. However, various more general inflammation-related diseases, including, but not limited to, other autoimmune and allergic diseases (e.g., rheumatoid arthritis and atopic asthma), infectious diseases (e.g., sepsis and chronic hepatitis), cardiovascular system-specific disorders (e.g., acute coronary syndromes and heart failure) as well as other diseases specific to the renal, hepatic, and respiratory systems, etc., have also been targeted as important fields of research. The data obtained so far demonstrated that sFas, sFasL, and DcR3 possess significant potential in the assessment of various disease states, which can contribute to the development of therapeutic interventions. Although further studies in various relevant fields are essential, it is expected that clinical translation of sFas, sFasL, and DcR3 into practical biomarkers will contribute to effective treatments of a wide variety of diseases.
Citation: Michiro Muraki. Soluble Fas ligand, soluble Fas receptor, and decoy receptor 3 as disease biomarkers for clinical applications: A review[J]. AIMS Medical Science, 2022, 9(2): 98-267. doi: 10.3934/medsci.2022009
[1] | Júlio Lobão, Luís Pacheco, Maria Beatriz Naia . Navigating the herd: The dynamics of investor behavior in the Brazilian stock market. Quantitative Finance and Economics, 2024, 8(3): 635-657. doi: 10.3934/QFE.2024024 |
[2] | Francisco Jareño, María De La O González, Pascual Belmonte . Asymmetric interdependencies between cryptocurrency and commodity markets: the COVID-19 pandemic impact. Quantitative Finance and Economics, 2022, 6(1): 83-112. doi: 10.3934/QFE.2022004 |
[3] | Francisco Jareño, María de la O González, José M. Almansa . Interest rate sensitivity of traditional, green, and stable cryptocurrencies: A comparative study across market conditions. Quantitative Finance and Economics, 2025, 9(1): 100-130. doi: 10.3934/QFE.2025004 |
[4] | Chiao Yi Chang, Fu Shuen Shie, Shu Ling Yang . The relationship between herding behavior and firm size before and after the elimination of short-sale price restrictions. Quantitative Finance and Economics, 2019, 3(3): 526-549. doi: 10.3934/QFE.2019.3.526 |
[5] | Sudeshna Ghosh . Asymmetric impact of COVID-19 induced uncertainty on inbound Chinese tourists in Australia: insights from nonlinear ARDL model. Quantitative Finance and Economics, 2020, 4(2): 343-364. doi: 10.3934/QFE.2020016 |
[6] | Samuel Asante Gyamerah . Modelling the volatility of Bitcoin returns using GARCH models. Quantitative Finance and Economics, 2019, 3(4): 739-753. doi: 10.3934/QFE.2019.4.739 |
[7] | Lennart Ante . Bitcoin transactions, information asymmetry and trading volume. Quantitative Finance and Economics, 2020, 4(3): 365-381. doi: 10.3934/QFE.2020017 |
[8] | Rubaiyat Ahsan Bhuiyan, Tanusree Chakravarty Mukherjee, Kazi Md Tarique, Changyong Zhang . Hedge asset for stock markets: Cryptocurrency, Cryptocurrency Volatility Index (CVI) or Commodity. Quantitative Finance and Economics, 2025, 9(1): 131-166. doi: 10.3934/QFE.2025005 |
[9] | Nilcan Mert, Mustafa Caner Timur . Bitcoin and money supply relationship: An analysis of selected country economies. Quantitative Finance and Economics, 2023, 7(2): 229-248. doi: 10.3934/QFE.2023012 |
[10] | Nitesha Dwarika . An innovative extended Bayesian analysis of the relationship between returns and different risk measures in South Africa. Quantitative Finance and Economics, 2022, 6(4): 570-603. doi: 10.3934/QFE.2022025 |
Soluble Fas ligand (sFasL, sCD95L) and its specific soluble binders, soluble Fas receptor (sFas, sCD95) and decoy receptor 3 (DcR3), have been investigated as possible clinical biomarkers in many serious diseases. The present review aimed to provide an overview of the current state of this medically promising research by extensively examining the relevant literature. The summarized results of the survey are presented after classification into six categories according to the type of targeted disease. To date, the studies have been mainly devoted to the diagnosis of disease severity states and prognosis of treatments about various types of cancers and autoimmune diseases represented by autoimmune lymphoproliferative syndrome and systemic lupus erythematosus, because these important life-threatening or intractable diseases were suggested to be most relevant to the impairment of apoptotic cell death-inducing systems, including the Fas receptor-mediated signaling system, and the mechanisms responsible for their onset. However, various more general inflammation-related diseases, including, but not limited to, other autoimmune and allergic diseases (e.g., rheumatoid arthritis and atopic asthma), infectious diseases (e.g., sepsis and chronic hepatitis), cardiovascular system-specific disorders (e.g., acute coronary syndromes and heart failure) as well as other diseases specific to the renal, hepatic, and respiratory systems, etc., have also been targeted as important fields of research. The data obtained so far demonstrated that sFas, sFasL, and DcR3 possess significant potential in the assessment of various disease states, which can contribute to the development of therapeutic interventions. Although further studies in various relevant fields are essential, it is expected that clinical translation of sFas, sFasL, and DcR3 into practical biomarkers will contribute to effective treatments of a wide variety of diseases.
Acute-on-chronic liver failure;
Acute coronary syndrome;
Alzheimer's disease;
Adenosine deaminase;
Autosomal dominant polycystic kidney disease;
Acute graft-versus-host disease;
Apnea-hypopnea index;
Allogeneic hematopoietic stem cell transplantation;
Acute kidney injury;
Alanine aminotransferase;
Autoimmune lymphoproliferative syndrome;
Age-related macular degeneration;
Acute myocardial infarction;
Acute physiology and chronic health evaluation;
Acute rejection;
Acute respiratory distress syndrome;
Ascites;
Bladder cancer;
Body-mass-index;
Coronary artery bypass grafting;
Coronary artery disease;
Cord blood;
Coronary collateral circulation;
Coronary heart disease;
Cirrhosis;
Chronic kidney disease;
Compensated liver cirrhosis accompanied with portal hypertension;
Chronic obstructive pulmonary disease;
SARS-CoV-2 virus;
Clinical remission;
C-reactive protein;
Cerebrospinal fluid;
Cardiovascular disease;
Decoy receptor 3;
Diabetic foot lesions;
Death-inducing signaling complex;
Diabetes mellitus;
Extracellular domain;
Estimated glomerular filtration rate;
Erythropoietin;
Erythropoietin-stimulating agent;
End-Stage renal disease;
Ebora virus disease;
Fas-associating protein with death domain;
Fas ligand;
Flare;
Graves' disease;
Graves' hyperthyroidism;
Gastrointestinal stromal carcinoma;
Graves' ophthalmopathy;
Hemoglobin;
Hepatitis-B virus;
Hepatocellular cancer;
Hepatitis-C virus;
Hemodialysis;
Hydrocephalus;
Heart failure;
Hemorrhagic fever with renal syndrome;
Hypoxic-ischemic encephalopathy;
Human immune-deficiency virus;
Head and neck cancer;
Hormone replacement therapy;
Hypertension;
Interferon;
Interleukin;
Intraparenchymal hemorrhage;
Ischemic stroke;
Intrauterine growth restriction;
Kawasaki disease;
Liver fibrosis;
Lupus nephritis;
Mild cognitive impairment;
Model for end-stage liver disease;
Metabolic syndrome;
Multiple sclerosis;
Nonalcoholic fatty liver disease;
Nonalcoholic steatohepatitis;
Non-hemorrhagic;
Nonagenarians;
Non-resolving subphenotype;
Oxygen-gas treatment;
Pleural effusion;
Post-hemorrhagic;
Pediatric kidney transplant recipients;
Plasma;
Postmenopausal syndrome;
Peripartum cardiography;
Prostate cancer;
Preserved renal function;
Pulmonary thromboembolism;
Periventricular leukomalacia;
Rheumatoid arthritis;
Renal cell cancer;
Relapsing-remitting MS;
Saliva;
Sickle cell disease;
Synovial fluid;
Soluble Fas receptor;
Soluble Fas ligand;
Systemic inflammatory response syndrome;
Stevens-Johnson syndrome;
Systemic lupus erythematosus;
Sequential organ failure assessment;
Serum;
Stomach cancer;
Tumor necrosis factor;
Sjögren's syndrome;
Subarachnoid hemorrhage;
Type-1 DM;
Type-2 DM;
Tau protein/amyloid β 1-42 ratio;
Tuberculosis;
Toxic epidermal necrolysis;
Thyroid stimulating hormone receptor antibodies;
Toxic epidermal necrolysis;
Peak oxygen consumption;
Vascular endothelial dysfunction;
Werner syndrome;
Percent body fat;
The area under the curve;
Regression coefficient;
Correlation analysis;
Concordance statistic;
Cutoff value;
Double determinant immunoassay;
Enzyme-linked immunosorbent assay;
Hazard ratio;
Interquartile range;
Liquid chromatography-electrospray ionization mass-spectrometry method;
Median;
Mean;
Multiplex array assay;
Not described;
Not significant;
Odds ratio;
Probability value;
Primer extension assay;
Pearson's correlation coefficient;
Spearman's correlation coefficient;
Determination coefficient;
(whole) Range;
Receiver operating characteristic curve;
Risk ratio;
Standard deviation;
Standard error of the mean;
Western-blotting method;
95% Confidential interval
The financial markets have witnessed a revolution in terms of the pervasiveness of fintech and blockchain technology. The introduction of cryptocurrency, a decentralised, encrypted, and digital currency based on blockchain technology, has caused major disruption in the financial markets. Cryptocurrency is a highly speculative instrument (Matthews, 2021) that is vulnerable to large price swings (Cheah and Fry, 2015; Katsiampa, 2017). Their price behaviour and market movements have piqued the interest of scholars and regulators as they question their value as an investment vehicle or the means of exchange (Velde, 2013). These digital currencies do not perform the duties of a traditional central bank-issued fiat money, lack the intrinsic value (Philippas et al., 2020) and are primarily used for speculation (Baur et al., 2018; Yermack, 2015). Since the introduction of Bitcoin (Nakamoto, 2008), cryptocurrency markets have grown exponentially despite volatility. This is demonstrated by the fact that well over 19000 cryptocurrencies that are trading in the market with a total market capitalization of USD 1.22 trillion (www. coinmarketcap.com, as of June 04, 2022) Although the instrument lack regulatory legitimacy, the entire ecosystem of cryptocurrency has flourished with the development of wallets, stable coins and miners and has the potential to disrupt the traditional payment models in the financial sector. Crypto markets are dominated by individual investors (Rubin et al., 2018) who lack knowledge of valuations and basic asset price drivers (Enoksen et al., 2020) and are influenced by the information published on social media and online forums such as Reddit, Facebook, and Twitter (Garcia and Schweitzer, 2015). The new-age investors interested in cryptocurrencies typically establish beliefs based on talks that lead to the sharing of impressions and future expectations, resulting in trade decisions that are not based on fundamentals, but rather on imitation and "crowd chasing". According to the studies, Bitcoin's valuation is influenced by social media sentiment (Mai et al., 2018) and user interest (Kristoufek, 2013), and it is argued that there may be a possible causal relationship between prices and interest-driven variables, as evidenced by Google Trends and Wikipedia views. This is supported by the recent report by Reddit where the word "crypto" garnered the most mentions in 2021 (The Outlook India, December 2021) and was discussed more than 6.6 million times during the year. Further, the total crypto market volume witnessed an increase of 2.07% to USD 105.26 billion (Outlook, 2021). Figure 1 illustrates a Google Trends graph showing the synchronized movement of user community interest in the search term cryptocurrency and the Bitcoin price.
The inadequate understanding of cryptocurrencies due to the lack of transparent and reputable information sources and the idiosyncratic and complicated character makes the market vulnerable to biases and irrational investment behaviour. How do investors price assets and make decisions in the absence of any reference points? Behavioural finance attempts to overcome this gap by investigating asset prices in the context of human behaviour. It tries to account for the participants' perspectives, feelings, and opinions on price changes. Given this theoretical framework and the potential impact of investor behaviour on cryptocurrency prices, this study investigates the presence of market-wide herding under various market regimes. Our research adds four novel contributions to the existing body of knowledge. First, instead of focusing solely on Bitcoin, it examines the 22 major cryptocurrencies, which account for 90% of the total market cap, yielding robust results. Second, we use quantile regression estimation rather than OLS. This ensures that the outcomes are not influenced by outliers or extreme values (Kumar, 2020; Philippas et al., 2020; Senarathne and Jianguo, 2020). Third, we broaden the research to look at herd behaviour during the once-in-a-lifetime Covid-19 outbreak. As a result, this paper adds to the body of literature on the impact of the pandemic on financial markets. Four, we decompose the dispersion to examine the bias for three market regimes: bull and bear markets, extreme market returns, and high volatility. This adequately captures the key market parameters that could influence investor behaviour.
Given the framework, the following research questions (RQ) are investigated in this study.
RQ1: Is there significant market-wide herd behaviour across different cryptocurrencies?
RQ2: Is the herd effect dependent on market asymmetries of return, extreme movements, and volatility?
RQ3: Does the COVID-19 pandemic have an effect on herding in cryptocurrency markets?
Our results provide evidence of significant market-wide herd behaviour. Further, herding exhibits asymmetry and is pronounced during bull periods, extreme down market returns and high volatility days. The results also yield that the sub-period of the Covid-19 outbreak intensified the herd phenomenon in the cryptocurrency market. We also highlight the implications of the empirical findings for investors and regulators. The rest of the study is structured as follows: Section 2 provides a review of the empirical literature on herding in the cryptocurrency market. Section 3 discusses research objectives, data, and methodology. Section 4 presents the empirical findings and the analysis. The last section concludes the study and explains the future scope of the research.
Humans are affected by emotions and cognitive biases in general, which influence their actions and choices, including financial and investment decisions. As a result, there is an incentive for imitation and herd behaviour to conform to others or get into the swim of things (Leibenstein, 1950). Cryptocurrencies are a new phenomenon, and the literature on price behaviour is still in its early stages. According to Giudici et al. (2020), cryptocurrency markets are marked by high uncertainty as a result of a lack of clarity on fundamental value and the opaqueness of technology, which adds to the volatility. Furthermore, they are unregulated and primarily attract non-institutional investor participation. These market conditions have the potential to amplify herding. According to the recent research on the price movement of cryptocurrencies, media coverage has a significant impact on Bitcoin valuation (Glaser et al., 2014), as it aids in the formation of beliefs and investor opinions. The contemporary literature concludes that user speculation is the primary price driver for Bitcoin (Fantazzini et al., 2016). The empirical research on herding has been divided into two categories: the first group investigates market-wide herd behaviour using price data (Christie, & Huang, 1995) and the second, examines herding at the participant level using individual datasets (Lakonishok et al., 1992; Wermers, 1999). Since user anonymity is a distinguishing feature of this market, obtaining detailed individual-level trade data is not possible (Kremer et al., 2013). Further, the most popular method to analyse individual data sets for herding is by Lakonishok et al. (1992) which suffers from certain limitations. The method employs the number of buyers and sellers in the stock and ignores the volume of transactions. During conditions of different trade volumes with an equal number of buyers and sellers, the results will be inaccurate (Bikhchandani and Sharma, 2000). Second, LSV (1992) does not recognise the cross-sectional temporal dependence in trading patterns of funds, as a result, does not inform if it is the same fund that herds over time (Puckett and Yan, 2008). The majority of quantitative research on crypto herding falls into the former category that uses the publicly available market price data. The measure of return dispersion has been used by Christie and Huang (1995) (cross-sectional standard deviation, CSSD), Chang et al. (2000) (cross-sectional absolute deviation, CSAD), and Hwang and Salmon (2004) (beta herding). The academic research provides mixed and inconclusive results on the existence of the herd phenomenon in the crypto market. This review first focuses on the studies that find significant herding in this market (Ballis and Drakos, 2020; Kaiser and Stöckl, 2020; Kallinterakis and Wang, 2019). Ballis and Drakos (2020) examine six currencies namely, Bitcoin, Ethereum, Litecoin, Monero, Dash, and Ripple from August 2015 to December 2018 and report herd behaviour that is more pronounced during up market conditions. Haryanto et al. (2019) show similar results for Bitcoin herding. Da Gama Silva et al. (2019) employ CSSD, CSAD, Hwang and Salmon's (2004) method and find the evidence of herd behaviour in the 50 most liquid and capitalised digital currencies. Kiaser and Stöckl (2020) attribute the persistence of herd behaviour to the irrational individual investors with non-professional backgrounds. Calderón (2018) by applying the Markov-Switching Regime technique to the top 100 cryptocurrencies finds that there are specific periods during which herding is present, for instance, market stress (Akinsomi, Coskun, and Gupta, 2018; Kumar 2020). Leclair (2018) also argues that herd behaviour is persistent, and shocks have a positive impact. Kallinterakis and Wang (2019) provide arguments in support of herding in the cryptocurrency market due to speculation, high volatility, and noise trading. Stavroyiannis and Babalos (2019) argue that investors find comfort in consensus and herd during periods of market turmoil, high volatility and stress. Similarly, Shrotryia and Kalra (2021) discover asymmetry in herd behaviour that is exacerbated during times of extreme volatility. In contrast to Kumar (2020), they conclude that bullish phases are associated with strong herd behaviour. Furthermore, COVID-19 periods amplify the herd hunch.
Next, we shift the focus to the academic research that yields inconclusive or conflicting findings for cryptocurrency herding (Amirat and Alwafi, 2020; Coskun et al., 2020; Vidal-Tomás et al., 2019; Yarovaya et al., 2021). Bouri et al. (2019) use the CSAD technique to study a sample of 14 prominent cryptocurrencies. The static model demonstrates anti-herding, whereas the time-varying method utilizing rolling window analysis demonstrates strong herding during sub-periods. Stavroyiannis and Babalos (2019) use the time-varying approach of beta herding and do not observe any significant evidence. This result is confirmed by Amirat and Alwafi (2020) for a sample of the top 20 mega cryptocurrencies using the static CSAD model. Youssef (2020) provides empirical evidence of the anti-herd phenomenon in the crypto market. Using high-frequency hourly data for the period of the Covid-19 outbreak, Yarovaya et al. (2021) investigate the four cryptocurrency markets-, i.e., USD, EUR, JPY, and KRW to conclude no major herding, however, the phenomenon continues to be reliant on up or down- market days. Coskun et al. (2020) report no herding during market asymmetry fluctuations using econometric techniques. Vidal-Tomás and Ibañez (2018) apply the Chang et al. (2000) metric for 65 digital currencies from 2015 to 2017. Although herding is exhibited during down markets and stress, the results validate rational asset pricing models that can explain the large dispersions. Similar asymmetry is found by Kumar (2020) using daily data for 100 major cryptos during bearish and high volatility days, but no evidence for bullish or low volatility days. The above review presents that despite the plethora of studies available, the results and findings are inconclusive and mixed. We propose the following hypotheses based on the review:
H1: The cryptocurrency market exhibits herd behaviour.
H2a: Herding in the cryptocurrency market is contingent upon asymmetries in return for rising and falling returns.
H2b Herding in the cryptocurrency market is contingent upon extreme market returns.
H2c: Herd behaviour is significant during high volatility periods in the cryptocurrency market.
H3: Herd behaviour is significant during the outbreak of the COVID-19 pandemic for the sub-period of January 2020 to December 2020.
1. To detect market-wide herding in the cryptocurrency market for the complete sample period.
2. To examine herd phenomenon under different market asymmetries of return, volatility and extreme return conditions.
3. To examine herding in the crypto market during the spread of the COVID-19 pandemic.
The study uses the daily adjusted closing prices (in USD) of a sample of the top 22 cryptocurrencies traded globally, which account for 90% of total market capitalization. Our study spans from January 1, 2017, through December 31, 2020. Cryptocurrencies only gained popularity around the year 2013, and since then, they have reached new highs in terms of price and volume. The time range is noteworthy since it includes the spike of period 2016–2017 and the subsequent fall, followed by a strong climb in 2019–2020 that continued even into the year 2020 amid the COVID-19 outbreak. The data is sourced from the database available at www.coinmarketcap.com since the trading activity in this database is characterised by the same underlying process as the exchange and therefore is appropriate to conduct research.
The present research examines herding using the relationship between return dispersion and market return. The dispersion was first coined by Christie and Huang (1995) and measured as CSSD which is defined as the proximity of market and individual asset return. As per the standard finance theories, periods of market stress are expected to increase dispersion as each individual security has a different sensitivity to the market. However, during herding, as investors suppress their individual beliefs, and follow the general market consensus, CSSD decreases and this relationship between CSSD and market return can be used to study herd behaviour. Equation (1) measures CSSD:
CSSDt=√N∑i=1(Ri,t−Rm,t)2N−1 | (1) |
The regression Equation is:
CSSDt=α+βLDLt+βUDUt+ϵt | (2) |
Here, Rt is the return on the cryptocurrency i or the equally-weighted average return of all individual cryptocurrencies at time t and 'n' is the number of cryptocurrencies in the market 'm' at time t. D is the dummy variable and takes the value of unity or zero for extreme up (U) or down (L) market returns. Equation (2) provides a linear relation between CSSD and market return and β is the regression coefficient. However, Chang et al. (2000) argue that the linearity does not hold true during large price movements and herding. Instead, the relationship is non-linearly increasing or even decreasing. Chang et al. (2000) measure dispersion as the cross-sectional absolute deviation (CSAD). Using the same rationale as above, during herd behaviour, all asset returns converge towards the market return and the dispersion reduces or increases at a decreasing pace in relation to the market return, rendering the connection non-linear. CSAD is calculated as follows:
CSADt=1NN∑i=1⃒Ri,t−Rm,t⃒ | (3) |
The regression Equation is:
CSADt=β0+β1⃒Rmt⃒+β2(R2mt)+∈t | (4) |
Significant and negative regression coefficient β2 implies herding. Further, the returns are calculated using the log difference to reduce skewness and kurtosis in the time series data (Urquhart, 2018).
Rit=ln(PtPt−1)∗100 | (5) |
Rmt=ln(CVtCVt−1)∗100 | (6) |
Pt is the cryptocurrency's closing price, and CVt is the market portfolio's closing value at time t. Market return (Rmt) can be computed as either a value-weighted return or an equally-weighted return. However, considering Bitcoin dominates the cryptocurrency market, adopting a value-weighted return may not be appropriate for the current study. This may skew the return observations, resulting in a Bitcoin-biased value-weighted market portfolio. As a result, the current analysis employs equally-weighted market return averages. The analysis of equity market herding by Tan et al. (2008) and Economou et al. (2011) finds that results employing value-weighted and equally-weighted averages of market return is comparable. This is further confirmed by Vidal-Tomás et al. (2019) and Kumar (2020) The majority of the extant literature uses the ordinary least squares (OLS) regression to estimate Equation (4) for equity markets. According to the research, OLS has limitations because it is a mean-based method that is easily influenced by outliers (Barnes and Hughes, 2002). Furthermore, it may not produce reliable findings when dealing with a non-normal dataset. The residual tests of Durbin-Watson and ARCH LM show that the sample data has autocorrelation and heteroskedasticity. The presence of both the above problems in addition to non-normal data is inconsistent with the assumptions of OLS. Further, since our analysis involves testing for market asymmetries, the data set is split and is not continuous. As a result, the Newey-West procedure to control for autocorrelation and heteroskedasticity is used. However, our results are robust across the different estimation methods1. The non-normal dataset also consists of extreme values. Barnes and Hughes (2002) argue that quantile regression is a more efficient and versatile estimator than OLS as it provides for analysing the impact of independent variables on dispersion over the entire distribution and is robust to the presence of outliers, providing more accurate and reliable results (Koenker and Hallock, 2001). For these reasons, this work uses quantile regression estimator to assess herd behaviour (Koenker and Bassett, 1978). This technique has been widely utilised in similar studies on equity markets (Ajaz and Kumar, 2018; Bharti and Kumar, 2019; Pochea et al., 2017; Yarovaya et al., 2021). If τ is the quantile, the regression Equation is as follows:
1 For brevity, the results for OLS with Newey West estimation is not reported here.
CSADt(τxt)=β0,τ+β1,τ|Rmt|+β2,τ(Rm)2+∈t,τ | (7) |
The hypotheses are tested for quantile values of 0.10, 0.25, 0.50, 0.75 and 0.90 (Stavroyiannis and Babalos, 2019).
We examine the herd phenomenon during bull and bear market phases. Equation (7) is reformulated for up and down-market movements to check for the asymmetries:
CSADt,τ=β0,τ+βup1,τ.|Rmt,τ|+βup2,τ.(Rmt,τ)2+∈t,τ,Rmt>0 | (8) |
CSADt,τ=β0,τ+βdown1,τ.|Rmt,τ|+βdown2,τ.(Rmt,τ)2+∈t,τ,Rmt≤0 | (9) |
Negative and significant β2up and β2down in Equation (8) and (9) imply herd behaviour during bull and bear markets respectively.
We examine if CSADt is significantly lower than the average during extreme market return periods. Christie and Huang (1995) and Chang et al. (2000) argue that extreme returns are not defined, therefore, we use the criteria of 5% to classify extreme up (down) returns as those which lie to the right (left) of the return distribution for 5%. Following dummy variable (D) regression Equations are estimated for extreme up and down-market returns:
CSADt,τ=β0,τ+βup1,τ.Dup.|Rmt,τ|+βup2,τ.Dup.(Rmt,τ)2+∈t,τ | (10) |
CSADt,τ=β0,τ+βdown1,τ.Ddown.|Rmt,τ|+βdown2,τ.Ddown.(Rmt,τ)2+∈t,τ | (11) |
Dup and Ddown are the dummy variables for up and down returns respectively and take a value of unity if Rmt lies to the extreme right or left of the distribution at 5 per cent level or zero otherwise. Significant and negative values of the regression coefficient β2up and β2down imply herd behaviour during extreme up and down markets respectively.
We use Equation (12) to examine herding during periods of high market volatility as it may raise investor fear (Pochea et al., 2017). Following is the regression Equation:
CSADt,τ=β0,τ+β1,τ.DHVOL.|Rmt,τ|+β2,τ,.(1−DHVOL).|Rmt,τ| |
+β3,τ.DHVOL.(Rmt,τ)2+β4,τ.(1−DHVOL).(Rmt,τ)2+∈t,τ | (12) |
Here, DHVOL is the dummy variable for high market volatility and takes the value of one for days characterized with high volatility, or zero otherwise. A negative and significant value of coefficient of nonlinear term, β3 implies herding during high volatility period. The conditional volatility of the cryptocurrency market is calculated using the GJR-GARCH (1, 1) model (Kumar, 2020). Figure 2 depicts market return clustering, as a result, the application of the GJR- GARCH (1, 1) model is relevant as it captures the stylized facts in time series data, like volatility clustering and asymmetric returns (Choi et al., 2012). The volatility is considered high on any day t if the value is more than the past 30-day moving average (Kumar, 2020; Tan et al., 2008).
Herding during the COVID-19 sub period is investigated for the period beginning January 1, 2020, and ending December 31, 2020 using Equation (6). This time spans from the start of the novel Coronavirus's spread, when the WHO declared the outbreak as public health emergency of international concern, through the conclusion of the year, when most global economies had lifted restrictions.
The descriptive statistics of CSADt and Rmt for the sample period are shown in Table 1. Panel A displays the statistics for the time preceding COVID-19. The selected sample's average daily market return is 0.0018 percent with a standard deviation of 0.0485 percent, while the average dispersion is 0.03 percent with a standard deviation of 0.02 percent. Panel B depicts the description for the COVID-19 pandemic sub-period. The average return over the period is greater at 0.0025 percent, while dispersion is lower at 0.024 percent, implying that dispersion decreased during the outbreak. Furthermore, the Augmented Dickey-Fuller test (ADF) significant values show that the series of Rmt and CSADt are stationary at the level for both sample periods. And, the significant Jarque—Bera coefficient verifies the non-normal distribution and emphasizes the utility of the quantile regression estimator.
Panel A-Pre-COVID 19 Period | Panel B-COVID-19 Sub-Period | ||||
Rmt | CSADt | Rmt | CSADt | ||
Mean | 0.0018 | 0.0321 | 0.0025 | 0.0242 | |
Median | 0.0024 | 0.0253 | 0.0062 | 0.0216 | |
Maximum | 0.1630 | 0.1661 | 0.1446 | 0.0948 | |
Minimum | −0.2822 | 0.0066 | −0.453597 | 0.006 | |
Std. Dev. | 0.0485 | 0.0219 | 0.0438 | 0.0112 | |
Skewness | −0.5974 | 1.9961 | −3.4336 | 2.2688 | |
Kurtosis | 6.2950 | 8.1602 | 35.9557 | 11.8496 | |
Jarque-Bera | 559.9892* | 1940.349* | 16904.15* | 1475.345* | |
ADF | −33.3920* | −3.3529* | −21.8388* | −9.0464* | |
Note: *significant at 1% level. Source: The Authors. |
Figure 3 provides the graphical representation of the relationship between equally-weighted market return (Rmt) and CSADt for the cryptocurrency market for the entire sample period. The relationship appears non-linear and shows that dispersion concentrates at a minimum level near zero for bull periods, whereas it increases for extreme positive returns.
Table 2 presents the quantile estimation results for (6) for the entire data period in Panel A. The coefficient β2 is negative and significant over the range of quantile values (τ = 0.25, 0.50, 0.75 and 0.90). This reveals that the cryptocurrency market exhibits decreasing dispersions with respect to market returns and therefore herds for the overall period. The study, therefore, accepts the first hypothesis (H1).
τ | Panel A | Panel B | |||||||||
Whole Period | Bull Phase | Bear Phase | |||||||||
β0 | β1 | β2 | β0 | β1 | β2 | β0 | β1 | β2 | |||
0.1 | 0.0102* (32.5938) |
0.1639* (14.1868) |
0.0017 (0.0633) |
0.0090* (11.7759) |
0.2871* (5.5961) |
−0.7986 (−1.3682) |
0.0104* (25.5667) |
0.1356* (10.268) |
0.0681** (2.3502) |
||
0.25 | 0.0126* (32.9239) |
0.1997* (14.8536) |
−0.0842* (−2.6724) |
0.0119* (17.1878) |
0.2969* (7.0583) |
−0.3648 (−0.9338) |
0.0125* (24.5231) |
0.1603* (10.199) |
0.0032 (0.0895) |
||
0.5 | 0.0171* (35.3812) |
0.2722* (14.3006) |
−0.2660* (−5.8396) |
0.0155* (16.5859) |
0.4538* (6.2022) |
−0.9169 (−1.1163) |
0.0179* (20.4726) |
0.1540* (3.5518) |
0.1175 (0.4156) |
||
0.75 | 0.0229* (25.4752) |
0.4399* (9.0004) |
−0.6635* (−6.3651) |
0.0198* (17.1239) |
0.7757* (9.0218) |
−1.8696** (−2.1908) |
0.0242* (21.9397) |
0.1660* (3.5836) |
0.1409 (0.5927) |
||
0.9 | 0.0315* (16.3718) |
0.7241* (7.9385) |
−1.3319* (−6.9239) |
0.0294* (12.1047) |
0.8935* (4.2319) |
−-0.692 (−0.3141) |
0.0378* (12.5923) |
0.2005*** (1.7132) |
−0.1509 (−0.3392) |
||
Note: Panel A: Presents the results of Equation (7) for the whole period and Panel B presents the results of (8) and (9) for bull and bear phases. The negative and significant value of β2 implies herd behaviour. *, **, *** indicates significance at 1%, 5% and 10% levels respectively and the value in parenthesis is the t-statistics. |
Next, using (8) and (9), we investigate for herding during asymmetrical market return movements and the results are provided in Panel B of table 2. The coefficient β2 is negative and significant for only one quantile value, implying herd behaviour during the bull phase, but not during the bear phase. The findings also confirm that the value of coefficient of non-linear term (β2) is lesser for all quantiles during bull phase compared to the whole period. This provides support that bull periods intensify herding. The results therefore confirm the presence of herding during asymmetrical market returns. The regression findings for (10) and (11) for extreme up and down-market returns are shown in panel A of table 3. The results reveal significantly negative β2 during extreme down returns for higher quantile values (τ = 0.75, 0.90). However, no evidence is found for up markets. This demonstrates considerable herding during extreme down markets. Therefore, the study accepts H2b.
τ | Panel A | Panel B | |||||||||
Extreme Up Market Returns | Extreme Down-Market Returns | High Volatility | |||||||||
β0 | β1 | β2 | β0 | β1 | β2 | β0 | β1 | β2 | |||
0.1 | 0.0102* (32.5938) |
0.1639* (14.1868) |
0.0017 (0.0633) |
0.0090* (11.7759) |
0.2871* (5.5961) |
−0.7986 (−1.3682) |
0.0104* (25.5667) |
0.1356* (10.268) |
0.0681** (2.3502) |
||
0.25 | 0.0126* (32.9239) |
0.1997* (14.8536) |
−0.0842* (−2.6724) |
0.0119* (17.1878) |
0.2969* (7.0583) |
−0.3648 (−0.9338) |
0.0125* (24.5231) |
0.1603* (10.199) |
0.0032 (0.0895) |
||
0.5 | 0.0171* (35.3812) |
0.2722* (14.3006) |
−0.2660* (−5.8396) |
0.0155* (16.5859) |
0.4538* (6.2022) |
−0.9169 (−1.1163) |
0.0179* (20.4726) |
0.1540* (3.5518) |
0.1175 (0.4156) |
||
0.75 | 0.0229* (25.4752) |
0.4399* (9.0004) |
−0.6635* (−6.3651) |
0.0198* (17.1239) |
0.7757* (9.0218) |
−1.8696** (−2.1908) |
0.0242* (21.9397) |
0.1660* (3.5836) |
0.1409 (0.5927) |
||
0.9 | 0.0315* (16.3718) |
0.7241* (7.9385) |
−1.3319* (−6.9239) |
0.0294* (12.1047) |
0.8935* (4.2319) |
−0.692 (−0.3141) |
0.0378* (12.5923) |
0.2005*** (1.7132) |
−0.1509 (−0.3392) |
||
Note: Panel A: Presents the results of Equation (10) and (11) for extreme up and down market returns and Panel B presents the estimation of (12) for high volatility periods. The negative and significant value of β2 implies herd behaviour for extreme up and down-market returns. Negative and significant β3 implies herding during high volatility. *, **, *** indicates significance at 1%, 5% and 10% level respectively and the value in parenthesis is the t-statistics. |
Panel B of table 3 also includes the empirical results of Equation (12) for high volatility periods. According to the regression results, the coefficient of the non-linear factor (β3) is negative and significant across all quantiles implying decreasing dispersion for high volatility days and evidence of herding. As a result, hypothesis H2c is accepted.
Table 4 shows the empirical estimations for the behaviour of CSADt during the sub-period of the spread of the novel Coronavirus. For the median and higher quantile values, the coefficient β2 is negative and significant. The excess value of kurtosis for the sub-period, as shown in table 1 (Panel B), also supports the claim that such exogeneous events engulf the market with panic and anxiety, driving herd phenomenon. As a result, H03 is accepted.
Τ | 0.1 | 0.25 | 0.5 | 0.75 | 0.9 |
β0 | 0.0108* (16.1144) |
0.0139* (20.5061) |
0.0170* (20.0784) |
0.0221* (22.8469) |
0.0274* (19.6571) |
β1 | 0.1454* (5.5300) |
0.1604* (6.1766) |
0.2192* (6.0090) |
0.2499* (7.1704) |
0.3144* (7.6693) |
β2 | 0.0442 (0.7841) |
−0.0041 (−0.0719) |
−0.1483** (−1.8757) |
−0.2411* (−3.1895) |
−0.4089* (−4.6463) |
Note: This table presents the results of Equation (6) as applied for the sub-period January 01, 2020, till December 31, 2020. Negative and significant value of β2 implies herd behaviour. *, **, *** indicates significance at 1%, 5% and 10% levels respectively and the value in parenthesis is the t-statistics. |
Positive shocks that increase the demand and price of cryptocurrency, cause more volatility than negative shocks (Baur and Dimpfl, 2018). In the absence of a transparent price discovery mechanism (Gerritsen et al., 2020; Kumar, 2020), investors resort to "pump and dump" culture and are subjected to herding owing to "FOMO" (fear of missing out) from the crowd. Additionally, fractional trading in cryptocurrencies has resulted in small ticket size investments by investors who lack adequate information and jump on the bandwagon during bull periods to make quick profits. This exacerbates short-term noise trading in the market, exposing it to the behavioural biases of herding and convergent trading (Fonseca et al., 2019; Gurdgiev, and O'Loughlin, 2020). The findings also show that during high market volatility, the interplay of fear and anxiety impacts herd behaviour significantly (Shaikh and Padhi, 2015; Youssef, 2020). Further, such periods destabilise the market, resulting in bubbles and biases (Kallinterakis and Wang, 2019; Papadamou et al., 2021; Shrotriya and Kalra, 2021). Next, our results provide credence to the caveats of behavioural finance as significant herding is witnessed during the sub period of the outbreak of COVID-19 virus (Lahmiri and Bekiros, 2020; Naeem et al., 2021). We provide two arguments in support of the results. First, exogenous events such as the COVID-19 pandemic, add to economic uncertainty and stock price volatility. Further, the global lockdowns resulted in a complete suspension of trade and supply networks disrupting financial markets. These institutional responses aggravate panic and anxiety resulting in "crowd chasing" behaviour as investors seek conformity. Second, the price of Bitcoin fell from USD 8000 in January 2020 to USD 4000 in March 2020 before skyrocketing to USD 28000 in December 2020 (www.coinmarketcap.com). Erratic price fluctuations add to informational inefficiency, resulting in behavioural convergent trends. Susana et al., (2020) reinforce this finding for considerable herd behaviour during the pandemic (Rubbaniy et al., 2021). However, Yarovaya et al. (2021) demonstrate opposing results of decreasing herding during the COVID 19 dissemination.
We investigated market-wide herding in the cryptocurrency market from January 1, 2017, through December 31, 2020, utilising a sample of the top 22 cryptocurrencies according market capitalization. Using the measure of dispersion, we examine herding in the cryptocurrency market for asymmetrical conditions- bull and bear phases, extreme up and down and high volatility. Furthermore, we also include the sub-period of the exogeneous event of the outbreak of the Covid-19 virus. The results reveal substantial evidence of herd phenomenon for the whole period. Further, herding is intensified during bull phases, extreme down markets and high volatility periods. With enormous trade volumes and strong investor activity, cryptocurrencies have significantly transformed the financial markets. In the absence of broader legal acceptability and regulatory uncertainty, these are inherently volatile and exposed to shocks and market stress. The evidence of herding has implications for regulators. First, this necessitates the development of sufficient risk management procedures and regulatory oversight including standardized global crypto guidelines for investor protection. This will allow for a more progressive market environment. Next, in the absence of any fundamental valuation model, we highlight the need of sticking to coin categories with a liquid market for investors. Criteria such as the potential and the problem it is addressing, or the entire supply of the currency can be the determinant in investment decisions, as opposed to socially motivated behaviour. Third, cryptocurrency investment is a "fad", particularly for a new age investor chasing the market trend to make quick profits. As demonstrated by this study, such irrationality is escalated during market asymmetries and stress situations. This necessitates prioritising investor education programmes and campaigns that guide through the risks with cryptocurrency transactions. Four, the findings lay the groundwork for portfolio managers to understand the effect of herd bias in the market and formulate appropriate investment strategies. Five, our study implies that due to the presence of behavioural biases, the standard asset valuation models might fail to explain the crypto prices. This calls for developing more reliable models that are better equipped to explain the price dynamics of cryptocurrencies. Given the success and disruption that cryptocurrencies have caused, it is imperative to assess their potential to channel their advantages for the economy. This research presents an interdisciplinary perspective on cryptocurrency markets and paves the way for future research.
This research did not receive any specific grant from funding agencies in the public, commercial, or not-for-profit sectors.
All authors declare no conflicts of interest in this paper.
[1] | McIlwain DR, Berger T, Mak TW (2013) Caspase functions in cell death and disease. Cold Spring Harb Perspect Biol 5: a008656. https://doi.org/10.1101/cshperspect.a008656 |
[2] | Nagata S (1999) Fas ligand-induced apoptosis. Annu Rev Genet 33: 29-55. https://doi.org/10.1146/annurev.genet.33.1.29 |
[3] | Pitti RM, Marsters SA, Lawrence DA, et al. (1998) Genomic amplification of a decoy receptor for Fas ligand in lung and colon cancer. Nature 396: 699-703. https://doi.org/10.1038/25387 |
[4] | Muraki M (2020) Sensitization to cell death induced by soluble Fas ligand and agonistic antibodies with exogenous agents: A review. AIMS Med Sci 7: 122-203. https://doi.org/10.3934/medsci.2020011 |
[5] | Cheng J, Zhou T, Liu C, et al. (1994) Protection from Fas-mediated apoptosis by a soluble form of the Fas molecule. Science 263: 1759-1762. https://doi.org/10.1126/science.7510905 |
[6] | Guégan JP, Legembre P (2018) Nonapoptotic functions of Fas/CD95 in the immune response. FEBS J 285: 809-827. https://doi.org/10.1111/febs.14292 |
[7] | Herrero R, Kajikawa O, Matute-Bello G, et al. (2011) The biological activity of FasL in human and mouse lungs is determined by the structure of its stalk region. J Clin Invest 121: 1174-1190. https://doi.org/10.1172/JCI43004 |
[8] | Malleter M, Tauzin S, Bessede A, et al. (2013) CD95L cell surface cleavage triggers a prometastatic signaling pathway in triple-negative breast cancer. Cancer Res 73: 6711-6721. https://doi.org/10.1158/0008-5472.CAN-13-1794 |
[9] | Holdenrieder S, Stieber P (2004) Apoptotic markers in cancer. Clin Biochem 37: 605-617. https://doi.org/10.1016/j.clinbiochem.2004.05.003 |
[10] | Audo R, Calmon-Hamaty F, Papon L, et al. (2014) Distinct effects of soluble and membrane-bound Fas ligand on fibroblast-like synoviocytes from rheumatoid arthritis patients. Arthritis Rheumatol 66: 3289-3299. https://doi.org/10.1002/art.38806 |
[11] | Hsieh SL, Lin WW (2017) Decoy receptor 3: an endogenous immunomodulator in cancer growth and inflammatory reactions. J Biomed Sci 24: 39. https://doi.org/10.1186/s12929-017-0347-7 |
[12] | Miwa K, Asano M, Horai R, et al. (1998) Caspase1-independent IL-1beta release and inflammation induced by the apoptosis inducer Fas ligand. Nat Med 4: 1287-1297. https://doi.org/10.1038/3276 |
[13] | Kurma K, Boizard-Moracchini A, Galli G, et al. (2021) Soluble CD95L in cancers and chronic inflammatory disorders, a new therapeutic target?. Biochim Biophys Acta Rev Cancer 1876: 188596. https://doi.org/10.1016/j.bbcan.2021.188596 |
[14] | Ueno T, Toi M, Tominaga T (1999) Circulating soluble Fas concentration in breast cancer patients. Clin Cancer Res 5: 3529-3533. |
[15] | Hara T, Tsurumi H, Takemura M, et al. (2000) Serum-soluble Fas level determines clinical symptoms and outcome of patients with aggressive non-Hodgkin's lymphoma. Am J Hematol 64: 257-261. https://doi.org/10.1002/1096-8652(200008)64:4<257::AID-AJH4>3.0.CO;2-2 |
[16] | Konno R, Takao T, Sato S, et al. (2000) Serum soluble Fas level as a prognostic factor in patients with gynecological malignancies. Clin Cancer Res 6: 3576-3580. |
[17] | Tsutsumi S, Kuwano H, Shimura T, et al. (2000) Circulating soluble Fas ligand in patients with gastric carcinoma. Cancer 89: 2560-2564. https://doi.org/10.1002/1097-0142(20001215)89:12<2560::AID-CNCR7>3.0.CO;2-Q |
[18] | Mizutani Y, Hongo F, Sato N, et al. (2001) Significance of serum soluble Fas ligand in patients with bladder carcinoma. Cancer 92: 287-293. https://doi.org/10.1002/1097-0142(20010715)92:2<287::AID-CNCR1321>3.0.CO;2-4 |
[19] | Ugurel S, Rappl G, Tilgen W, et al. (2001) Increased soluble CD95 (sFas/CD95) serum level correlates with poor prognosis in melanoma patients. Clin Cancer Res 7: 1282-1286. |
[20] | Hoffmann TK, Dworacki G, Tsukihiro T, et al. (2002) Spontaneous apoptosis of circulating T lymphocytes in patients with head and neck and its clinical importance. Clin Cancer Res 8: 2553-2562. |
[21] | Akhmedkhanov A, Lundin E, Guller S, et al. (2003) Circulating soluble Fas levels and risk of ovarian cancer. BMC Cancer 3: 33. https://doi.org/10.1186/1471-2407-3-33 |
[22] | Sheen-Chen SM, Chen HS, Eng HL, et al. (2003) Circulating soluble Fas in patients with breast cancer. World J Surg 27: 10-13. https://doi.org/10.1007/s00268-002-6378-5 |
[23] | Wu Y, Han B, Sheng H, et al. (2003) Clinical significance of detecting elevated serum DcR3/TR6/M68 in malignant tumor patients. Int J Cancer 105: 724-732. https://doi.org/10.1002/ijc.11138 |
[24] | Erdoğan B, Uzaslan E, Budak F, et al. (2005) The evaluation of soluble Fas and soluble Fas ligand levels of bronchoalveolar lavage fluid in lung cancer patients. Tuberk Toraks 53: 127-131. |
[25] | Nadal C, Maurel J, Gallego R, et al. (2005) Fas/Fas ligand ratio: a marker of oxaliplatin-based intrinsic and acquired resistance in advanced colorectal cancer. Clin Cancer Res 11: 4770-4774. https://doi.org/10.1158/1078-0432.CCR-04-2119 |
[26] | Shimizu M, Kondo M, Ito Y, et al. (2005) Soluble Fas and Fas ligand new information on metastasis and response to chemotherapy in SCLC patients. Cancer Detect Prev 29: 175-180. https://doi.org/10.1016/j.cdp.2004.09.001 |
[27] | Yatsuya H, Toyoshima H, Tamakoshi K, et al. (2005) Serum levels of insulin-like growth factor I, II, and binding protein 3, transforming growth factor β-1, soluble Fas ligand and superoxide dismutase activity in stomach cancer cases and their controls in the JACC study. J Epidemiol 15: S120-S125. https://doi.org/10.2188/jea.15.S120 |
[28] | Sonoda K, Miyamoto S, Hirakawa T, et al. (2006) Clinical significance of RCAS1 as a biomarker of uterine cancer. Gynecol Oncol 103: 924-931. https://doi.org/10.1016/j.ygyno.2006.05.047 |
[29] | Svatek RS, Herman MP, Lotan Y, et al. (2006) Soluble Fas-a promising novel urinary marker for the detection of recurrent superficial bladder cancer. Cancer 106: 1701-1707. https://doi.org/10.1002/cncr.21795 |
[30] | Baldini E, Ulisse S, Marchioni E, et al. (2007) Expression of Fas and Fas ligand in human testicular germ cell tumours. Int J Andorol 32: 123-130. https://doi.org/10.1111/j.1365-2605.2007.00823.x |
[31] | Simon I, Liu Y, Krall KL, et al. (2007) Evaluation of the novel serum markers B7-H4, spondin 2, and DcR3 for diagnosis and early detection of ovarian cancer. Gynecol Oncol 106: 112-118. https://doi.org/10.1016/j.ygyno.2007.03.007 |
[32] | Aguilar-Lemarroy A, Romero-Ramos JE, Olimon-Andalon V, et al. (2008) Apoptosis induction in Jurkat cells and sCD95 levels in women's sera are related with the risk of developing cervical cancer. BMC Cancer 8: 99. https://doi.org/10.1186/1471-2407-8-99 |
[33] | Chaudhry P, Srinvasan R, Patel FD, et al. (2008) Serum soluble Fas levels and prediction of response to platinum-based chemotherapy in epithelial ovarian cancer. Int J Cancer 122: 1716-1721. https://doi.org/10.1002/ijc.23213 |
[34] | Connor JP, Felder M (2008) Ascites from epithelial ovarian cancer contain high levels of functional decoy receptor 3 (DcR3) and is associated with platinum resistance. Gynecol Oncol 111: 330-335. https://doi.org/10.1016/j.ygyno.2008.07.012 |
[35] | Kilic A, Schubert MJ, Luketich JD, et al. (2008) Use of novel autoantibody and cancer-related protein arrays for the detection of esophageal adenocarcinoma in serum. J Thorac Cardiovasc Surg 136: 199-204. https://doi.org/10.1016/j.jtcvs.2008.01.012 |
[36] | Macher-Goeppinger S, Aulmann S, Wagener N, et al. (2008) Decoy receptor 3 is a prognostic factor in renal cell cancer. Neoplasia 10: 1049-1056. https://doi.org/10.1593/neo.08626 |
[37] | Nolen BM, Marks JR, Ta'san S, et al. (2008) Serum biomarker profiles and response to neoadjuvant chemotherapy for locally advanced breast cancer. Breast Cancer Res 10: R45. https://doi.org/10.1186/bcr2096 |
[38] | Tamakoshi A, Nakachi K, Ito Y, et al. (2008) Soluble Fas level and cancer mortality: Findings from a nested case-control study within a large-scale prospective study. Int J Cancer 123: 1913-1916. https://doi.org/10.1002/ijc.23731 |
[39] | Anderson GL, McIntosh M, Wu L, et al. (2010) Assessing lead time of selected ovarian cancer biomarkers: A nested case-control study. J Natl Cancer Inst 102: 26-38. https://doi.org/10.1093/jnci/djp438 |
[40] | Goon PKY, Lip GYH, Stonelake PS, et al. (2009) Circulating endothelial cells and circulating progenitor cells in breast cancer: Relationship to endothelial damage/dysfunction/apoptosis, clinicopathologic factors, and the Nottingham prognostic index. Neoplasia 11: 771-779. https://doi.org/10.1593/neo.09490 |
[41] | Kavathia N, Jain A, Walston J, et al. (2009) Serum markers of apoptosis decrease with age and cancer stage. Aging 1: 652-663. https://doi.org/10.18632/aging.100069 |
[42] | Vysotskii MM, Digaeva MA, Kushlinskii NE, et al. (2009) Serum sFas, leptin, and VEGF in patients with ovarian cancer and benign tumors. Bull Exp Biol Med 148: 810-814. https://doi.org/10.1007/s10517-010-0823-5 |
[43] | Boroumand-Noughabi S, Sima HR, Ghaffarzadehgan K, et al. (2010) Soluble Fas might serve as a diagnostic tool for gastric adenocarcinoma. BMC Cancer 10: 275. https://doi.org/10.1186/1471-2407-10-275 |
[44] | Habibagahi M, Jaberipour M, Fattahi MJ, et al. (2010) High concentration of serum soluble Fas in patients with head and neck carcinoma: A comparative study before and after surgical removal of tumor. Middle East J Cancer 1: 21-26. |
[45] | Nolen B, Velikokhatnaya L, Marrangoni A, et al. (2010) Serum biomarker panels for the discrimination of benign from malignant cases in patients with an adnexal mass. Gynecol Oncol 117: 440-445. https://doi.org/10.1016/j.ygyno.2010.02.005 |
[46] | Ulukaya E, Acilan C, Yilmaz M, et al. (2010) sFas levels increase in response to cisplatin-based chemotherapy in lung cancer patients. Cell Biochem Funct 28: 565-570. https://doi.org/10.1002/cbf.1689 |
[47] | Yang M, Chen G, Dang Y, et al. (2010) Significance of decoy receptor 3 in sera of hepatocellular carcinoma patients. Upsala J Med Sci 115: 232-237. https://doi.org/10.3109/03009734.2010.516410 |
[48] | Zekri AN, El-Din HMA, Bahnassy AA, et al. (2010) Serum levels of soluble Fas, soluble tumor necrosis factor-receptor II, interleukin-2 receptor and interleukin-8 as early predictors of carcinoma in Egyptian patients with hepatitis C virus genotype-4. Comp Hepatol 9: 1. https://doi.org/10.1186/1476-5926-9-1 |
[49] | Hoogwater FJH, Snoeren N, Nijkamp MW, et al. (2011) Circulating CD95-ligand as a potential prognostic marker for recurrence in patients with synchronous colorectal liver metastases. Anticancer Res 31: 4507-4512. |
[50] | Fersching DMI, Nagel D, Siegele B, et al. (2012) Apoptosis-related biomarkers sFas, MIF, ICAM-1 and PAI-1 in serum of breast cancer patients undergoing neoadjuvant chemotherapy. Anticancer Res 32: 2047-2058. |
[51] | Hammam O, Mahmoud O, Zahran M, et al. (2012) The role of Fas/Fas ligand system in the pathogenesis of liver cirrhosis and hepatocellular carcinoma. Hepat Mon 12: e6132. https://doi.org/10.5812/hepatmon.6132 |
[52] | Izbicka E, Streeper RT, Michalek JE, et al. (2012) Plasma biomarkers distinguish non-small cell lung cancer from asthma and differ in men and women. Cancer Genomics Proteomics 9: 27-35. |
[53] | Eissa S, Swellam M, Abdel-Malak C, et al. (2013) The clinical relevance of urinary soluble fas (sFas) for diagnosis of bilharzial bladder cancer. Asian Biomed 7: 761-767. https://doi.org/10.5372/1905-7415.0706.238 |
[54] | Owonikoko TK, Hossain MS, Bhimani C, et al. (2013) Soluble Fas ligand as a biomarker of disease recurrence in differentiated thyroid cancer. Cancer 119: 1503-1511. https://doi.org/10.1002/cncr.27937 |
[55] | Elbedewy TA, El-Feky S, El Sheikh MA, et al. (2014) Serum levels of soluble Fas and soluble Fas ligand as markers for hepatocellular carcinoma in hepatitis C virus patients. Int J Adv Res 2: 911-919. |
[56] | Xu Y, Zhang L, Sun S, et al. (2014) CC Chemokine ligand 18 and IGF-binding protein 6 as potential serum biomarkers for prostate cancer. Tohoku J Exp Med 233: 25-31. https://doi.org/10.1620/tjem.233.25 |
[57] | Yeh CN, Chung WH, Su SC, et al. (2014) Fas/Fas ligand mediates keratinocyte death in sunitinib-induced hand-foot skin reaction. J Invest Dermatol 134: 2768-2775. https://doi.org/10.1038/jid.2014.218 |
[58] | Kadam CY, Abhang SA (2015) Serum levels of soluble Fas ligand, granzyme B and cytochrome c during adjuvant chemotherapy of breast cancer. Clin Chim Acta 438: 98-102. https://doi.org/10.1016/j.cca.2014.08.012 |
[59] | Kouloubnis A, Sofroniadou S, Panoulas VF, et al. (2015) The role of TNF-α, Fas/Fas ligand system and NT-proBNP in the early detection of asymptomatic left ventricular dysfunction in cancer patients treated with anthracyclines. IJC Heart Vasc 6: 85-90. https://doi.org/10.1016/j.ijcha.2015.01.002 |
[60] | Lancrajan I, Schneider-Stock R, Naschberger E, et al. (2015) Absolute quantification of DcR3 and GDF-15 from human serum by LC-ESI MS. J Cell Mol Med 19: 1656-1671. https://doi.org/10.1111/jcmm.12540 |
[61] | Bamias G, Gizis M, Delladetsima I, et al. (2016) Elevated serum levels of the antiapoptotic protein decoy-receptor 3 are associated with advanced liver disease. Can J Gastroenterol Hepatol 2016: 2637010. https://doi.org/10.1155/2016/2637010 |
[62] | Chang SW, Wang BC, Gong XG, et al. (2016) Serum decoy receptor 3 is a diagnostic and prognostic biomarker in patients with colorectal cancer. Int J Clin Exp Pathol 9: 7488-7492. |
[63] | Dressen K, Hermann N, Manekeller S, et al. (2017) Diagnostic performance of a novel multiplex immunoassay in colorectal cancer. Anticancer Res 37: 2477-2486. https://doi.org/10.21873/anticanres.11588 |
[64] | Hermann N, Dressen K, Schroeder L, et al. (2017) Diagnostic relevance of a novel multiplex immunoassay panel in breast cancer. Tumor Biol 2017: 1-11. https://doi.org/10.1177/1010428317711381 |
[65] | Johdi NA, Mazlan L, Sagap I, et al. (2017) Profiling of cytokines, chemokines and other soluble proteins as a potential biomarker in colorectal cancer and polyps. Cytokine 99: 35-42. https://doi.org/10.1016/j.cyto.2017.06.015 |
[66] | Li J, Xie N, Yuan J, et al. (2017) DcR3 combined with hematological traits serves as a valuable biomarker for the diagnosis of cancer metastasis. Oncotarget 8: 107612-107620. https://doi.org/10.18632/oncotarget.22544 |
[67] | Korczyński P, Mierzejewski M, Krenke R, et al. (2018) Cancer ratio and other new parameters for differentiation between malignant and nonmalignant pleural effusions. Pol Arch Intern Med 128: 354-361. https://doi.org/10.20452/pamw.4278 |
[68] | Ku SC, Ho PS, Tseng YT, et al. (2018) Benzodiazepine-associated carcinogenesis: Focus on lorazepam-associated cancer biomarker changes in overweight individuals. Psychiatry Invest 15: 900-906. https://doi.org/10.30773/pi.2018.05.02.1 |
[69] | Singh RK, Meena RN, Tiwary SK, et al. (2018) Fas ligand as a circulating apoptosis marker in carcinoma breast. World J Surg Res 7: 7-14. |
[70] | Łaniewski P, Cui H, Roe DJ, et al. (2019) Features of the cervicovaginal microenvironment drive cancer biomarker signatures in patients across cervical carcinogenesis. Sci Rep 9: 7333. https://doi.org/10.1038/s41598-019-43849-5 |
[71] | Wei Y, Chen X, Yang J, et al. (2019) DcR3 promotes proliferation and invasion of pancreatic cancer via a DcR3/STAT1/IRF1 feedback loop. Am J Cancer Res 9: 2618-2633. |
[72] | Akhmaltdinova L, Sirota V, Zhumaliyeva V, et al. (2020) Inflammatory serum biomarkers in colorectal cancer in Kazakhstan population. Int J Inflam 2020: 9476326. https://doi.org/10.1155/2020/9476326 |
[73] | Ali AS, Perren A, Lindskog C, et al. (2020) Candidate protein biomarkers in pancreatic neuroendocrine neoplasms grade 3. Sci Rep 10: 10639. https://doi.org/10.1038/s41598-020-67670-7 |
[74] | Chiu CT, Wang PW, Asare-Werehene M, et al. (2020) Circulating plasma gelsolin: A predictor of favorable clinical outcomes in head and neck cancer and sensitive biomarker for early disease diagnosis combined with soluble Fas ligand. Cancers 12: 1569. https://doi.org/10.3390/cancers12061569 |
[75] | Feng K, Liu Y, Zhao Y, et al. (2020) Efficacy and biomarker analysis of nivolumab plus gemcitabine and cisplatin in patients with unresectable or metastatic biliary tract cancers: results from a phase II study. J Immunother Cancer 8: e000367. https://doi.org/10.1136/jitc-2019-000367 |
[76] | Honma N, Inoue T, Tsuchiya N, et al. (2020) Prognostic value of plasminogen activator inhibitor-1 in biomarker exploration using multiplex immunoassay in patients with axitinib. Health Sci Rep 3: e197. https://doi.org/10.1002/hsr2.197 |
[77] | Ghonaim E, El-Haggar S, Gohar S (2021) Possible protective effect of pantoprazole against cisplatin-induced nephrotoxicity in head and neck cancer patients: a randomized controlled trial. Med Oncol 38: 108. https://doi.org/10.1007/s12032-021-01558-y |
[78] | López-Jornet P, Aznar C, Ceron J (2021) Salivary biomarkers in breast cancer: a cross-sectional study. Support Care Cancer 29: 889-896. https://doi.org/10.1007/s00520-020-05561-3 |
[79] | Jodo S, Kobayashi S, Kayagaki N, et al. (1997) Serum levels of soluble Fas/APO-1 (CD95) and its molecular structure in patients with systemic lupus erythematosus (SLE) and other autoimmune diseases. Clin Exp Immunol 107: 89-95. https://doi.org/10.1046/j.1365-2249.1997.d01-901.x |
[80] | Ohtsuka K, Hashimoto M (2000) Serum levels of soluble Fas in patients with Graves' ophthalmopathy. Br J Ophthalmol 84: 103-106. https://doi.org/10.1136/bjo.84.1.103 |
[81] | Wang CY, Zhong WB, Chang TC, et al. (2002) Circulating soluble Fas ligand correlates with disease activity in Graves' Hyperthyroidism. Metabolism 51: 769-773. https://doi.org/10.1053/meta.2002.32034 |
[82] | Boku S, Takahashi T, Watanabe K, et al. (2003) Serum soluble Fas as a seromarker of disease activity in chronic hepatitis C and the possible involvement of peripheral blood mononuclear cells in the production of serum soluble Fas. Acta Med Biol 51: 49-58. |
[83] | Kiraz S, Ertenli I, Öztürk MA, et al. (2003) Increased soluble Fas suggests delayed apoptosis in familial Mediterranean fever complicated with amyloidosis. J Rheumatol 30: 313-315. |
[84] | Silvestris F, Grinello D, Tucci M, et al. (2003) Enhancement of T cell apoptosis correlates with increased serum levels of soluble Fas (CD95/Apo-I) in active lupus. Lupus 12: 8-14. https://doi.org/10.1191/0961203303lu250oa |
[85] | Hayashi H, Maeda M, Murakami S, et al. (2009) Soluble interleukin-2 as an indicator of immunological disturbance found in silicosis patients. Int J Immunol Pharmacol 22: 53-62. https://doi.org/10.1177/039463200902200107 |
[86] | Magerus-Chatinet A, Stolzenberg MC, Loffredo MS, et al. (2009) FAS-L, IL-10, and double-negative CD4−CD8− TCR α/β+ T cells are reliable markers of autoimmune lymphoproliferative syndrome (ALPS) associated with FAS loss of function. Blood 113: 3027-3030. https://doi.org/10.1182/blood-2008-09-179630 |
[87] | Sahebari M, Hatef MR, Rezaieyazdi Z, et al. (2010) Correlation between serum levels of soluble Fas (CD95/Apo-1) with disease activity in systemic lupus erythematosus patients in Khorasan, Iran. Arch Iran Med 13: 135-142. https://doi.org/10.1007/s00296-010-1633-9 |
[88] | Ikonomidis I, Tzortzis S, Lekakis J, et al. (2011) Association of soluble apoptotic markers with impaired left ventricular deformation in patients with rheumatoid arthritis. Effects of inhibition of interleukin-1 activity by anakinra. Thromb Haemost 106: 959-967. https://doi.org/10.1160/TH11-02-0117 |
[89] | Bamias G, Stamatelopoulos K, Zampeli E, et al. (2013) Circulating levels of TNF-like cytokine 1A correlate with the progression of atheromatous lesions in patients with rheumatoid arthritis. Clin Immunol 147: 144-150. https://doi.org/10.1016/j.clim.2013.03.002 |
[90] | Moreno M, Sáenz-Cuesta M, Castilló J, et al. (2013) Circulating levels of soluble apoptosis-related molecules in patients with multiple sclerosis. J Neuroimmunol 263: 152-154. https://doi.org/10.1016/j.jneuroim.2013.07.013 |
[91] | Oka S, Furukawa H, Shimada K, et al. (2013) Serum biomarker analysis of collagen disease patients with acute-onset diffuse interstitial lung disease. BMC Immunol 14: 9. https://doi.org/10.1186/1471-2172-14-9 |
[92] | Rensing-Ehl A, Janda A, Lorenz MR, et al. (2013) Sequential decisions on Fas sequencing guided by biomarkers in patients with lymphoproliferation and autoimmune cytopenia. Heamatologica 98: 1948-1955. https://doi.org/10.3324/haematol.2012.081901 |
[93] | Roberts CA, Ayers L, Bateman EAL, et al. (2013) Investigation of common variable immunodeficiency patients and healthy individuals using autoimmune lymphoproliferative syndrome biomarkers. Hum Immunol 74: 1531-1535. https://doi.org/10.1016/j.humimm.2013.08.266 |
[94] | Bollain-y-Goytia JJ, Arellano-Rodríguez M, Torres-Del-Muro FJ, et al. (2014) Soluble Fas and the -670 polymorphism of Fas in lupus nephritis. Int J Nephrol 2014: 780406. https://doi.org/10.1155/2014/780406 |
[95] | Chen MH, Liu PC, Chang CW, et al. (2014) Decoy receptor 3 suppresses B cell functions and has a negative correlation with disease activity in rheumatoid arthritis. Clin Exp Rheumatol 32: 715-723. |
[96] | Munroe ME, Vista ES, Guthridge JM, et al. (2014) Proinflammatory adaptive cytokine and shed tumor necrosis factor receptor levels are elevated preceding systemic lupus erythematosus disease flare. Arthritis Rheumatol 66: 1888-1899. https://doi.org/10.1002/art.38573 |
[97] | Price S, Shaw PA, Seitz A, et al. (2014) Natural history of autoimmune lymphoproliferative syndrome associated with FAS gene mutations. Blood 123: 1989-1999. https://doi.org/10.1182/blood-2013-10-535393 |
[98] | Romano E, Terenzi R, Manetti M, et al. (2014) Disease activity improvement in rheumatoid arthritis treated with tumor necrosis factor-α inhibitors correlates with increased soluble Fas levels. J Rheumatol 41: 1961-1965. https://doi.org/10.3899/jrheum.131544 |
[99] | Liu J, Zhao Z, Zou Y, et al. (2015) The expression of death decoy receptor 3 was increased in the patients with primary Sjögren's syndrome. Clin Rheumatol 34: 879-885. https://doi.org/10.1007/s10067-014-2853-2 |
[100] | Zijuan X, Hui S, Ye T, et al. (2015) Serum and synovial fluid levels of tumor necrosis factor-like ligand 1A and decoy receptor 3 in rheumatoid arthritis. Cytokine 72: 185-189. https://doi.org/10.1016/j.cyto.2014.12.026 |
[101] | Elsaeed GSM, Elshamaa MF, Salah DM, et al. (2016) Fas-ligand and granzyme-b levels in children with nephrotic syndrome. Int J Pharma Clin Res 8: 1600-1604. |
[102] | Maruyama H, Hirayama K, Nagai M, et al. (2016) Serum decoy receptor 3 levels are associated with the disease activity of MPO-ANCA-associated renal vasculitis. Clin Rheumatol 35: 2469-2476. https://doi.org/10.1007/s10067-016-3321-y |
[103] | Chen MH, Kan HT, Liu CY, et al. (2017) Serum decoy receptor 3 is a biomarker for disease severity in nonatopic asthma patients. J Formos Med Assoc 116: 49-56. https://doi.org/10.1016/j.jfma.2016.01.007 |
[104] | Engmann J, Rüdrich U, Behrens G, et al. (2017) Increased activity and apoptosis of eosinophils in blister fluids, skin and peripheral blood of patients with bullous pemphigoid. Acta Derm Venereol 97: 464-471. https://doi.org/10.2340/00015555-2581 |
[105] | Ding YW, Pan SY, Xie W, et al. (2018) Elevated soluble Fas and FasL in cerebrospinal fluid and serum of patients with anti-N-methyl-D-aspartate receptor encephalitis. Front Neurol 9: 904. https://doi.org/10.3389/fneur.2018.00904 |
[106] | Liphaus BL, Sallum AEM, Aikawa NE, et al. (2018) Increased soluble cytoplasmic Bcl-2 protein serum levels and expression and decreased Fas expression in lymphocytes and monocytes in Juvenile dermatomyositis. J Rheumatol 45: 1577-1580. https://doi.org/10.3899/jrheum.171248 |
[107] | Wigren M, Svenungsson E, Mattisson IY, et al. (2018) Cardiovascular disease in systemic lupus erythematosus is associated with increased levels of biomarkers reflecting receptor-activated apoptosis. Atherosclerosis 270: 1-7. https://doi.org/10.1016/j.atherosclerosis.2018.01.022 |
[108] | Molnár E, Radwan N, Kovács G, et al. (2020) Key diagnostic markers for autoimmune lymphoproliferative syndrome with molecular genetic diagnosis. Blood 136: 1933-1945. https://doi.org/10.1182/blood.2020005486 |
[109] | Sremec J, Tomasović S, Sremec NT, et al. (2020) Elevated concentrations of soluble Fas and FasL in multiple sclerosis patients with antinuclear antibodies. J Clin Med 9: 3845. https://doi.org/10.3390/jcm9123845 |
[110] | Vincent FB, Kandane-Rathnayake R, Koelmeyer R, et al. (2020) Associations of serum soluble Fas and Fas ligand (FasL) with outcomes in systemic lupus erythematosus. Lupus Sci Med 7: e000375. https://doi.org/10.1136/lupus-2019-000375 |
[111] | Kamal A, Abdelmegeid AK, Gabr MAM, et al. (2021) Serum decoy receptor 3 (DcR3): a promising biomarker for atopic asthma in children. Immunol Res 69: 568-575. https://doi.org/10.1007/s12026-021-09218-z |
[112] | Kessel C, Fail N, Grom A, et al. (2021) Definition and validation of serum biomarkers for optimal differentiation of hyperferritinaemic cytokine storm conditions in children: a retrospective cohort study. Lancet Rheumatol 3: 563-573. https://doi.org/10.1016/S2665-9913(21)00115-6 |
[113] | Qi Y, Xu J, Lin Z, et al. (2021) The network of pro-inflammatory factors CD147, DcR3, and IL33 in the development of Kawasaki disease. J Inflamm Res 14: 6043-6053. https://doi.org/10.2147/JIR.S338763 |
[114] | Su KW, Chiu CY, Tsai MH, et al. (2021) Cord blood soluble Fas ligand linked to allergic rhinitis and lung function in seven-year-old children. J Microbiol Immunol Infect . (in press). https://doi.org/10.1016/j.jmii.2021.03.016 |
[115] | De Freitas I, Fernández-Somoza M, Essenfeld-Sekler E, et al. (2004) Serum levels of the apoptosis-associated molecules, tumor necrosis factor-α/tumor necrosis factor type-I receptor and Fas/FasL, in sepsis. Chest 125: 2238-2246. https://doi.org/10.1378/chest.125.6.2238 |
[116] | Paunel-Görgülü A, Flohé S, Scholz M, et al. (2011) Increased serum soluble Fas after major trauma is associated with delayed neutrophil apoptosis and development of sepsis. Crit Care 15: R20. https://doi.org/10.1186/cc9965 |
[117] | Hou YQ, Xu P, Zhang M, et al. (2012) Serum decoy receptor 3, a potential new biomarker for sepsis. Clin Chim Acta 413: 744-748. https://doi.org/10.1016/j.cca.2012.01.007 |
[118] | Huttunen R, Syrjänen J, Vuento R, et al. (2012) Apoptosis markers soluble Fas (sFas), Fas ligand (FasL) and sFas/sFasL ratio in patients with bacteremia: A prospective cohort study. J Infect 64: 276-281. https://doi.org/10.1016/j.jinf.2011.12.006 |
[119] | Kim S, Mi L, Zhang L (2012) Specific elevation of DcR3 in sera of sepsis patients and its potential role as a clinically important biomarker of sepsis. Diagn Microbiol Infect Dis 73: 312-317. https://doi.org/10.1016/j.diagmicrobio.2012.04.008 |
[120] | Liu YJ, Shao LH, Wang Q, et al. (2014) Predictive value of decoy receptor 3 in postoperative nosocomial bacterial meningitis. Int J Mol Sci 15: 19962-19970. https://doi.org/10.3390/ijms151119962 |
[121] | Liu YJ, Shao LH, Zhang J, et al. (2015) The combination of decoy receptor 3 and soluble triggering receptor expressed on myeloid cells-1 for the diagnosis of nosocomial bacterial meningitis. Ann Clin Microbiol Antimicrob 14: 17. https://doi.org/10.1186/s12941-015-0078-0 |
[122] | Chu CM, Kao KC, Huang SH, et al. (2016) Diagnostic value of apoptosis biomarkers in severe sepsis-A pilot study. Cell Mol Biol 62: 32-37. https://doi.org/10.14715/cmb/2016.62.11.6 |
[123] | Carcillo JA, Halstead ES, Hall MW, et al. (2017) Three hypothetical inflammation pathobiology phenotypes and pediatric sepsis-induced multiple organ failure outcome. Pediatr Crit Care Med 18: 513-523. https://doi.org/10.1097/PCC.0000000000001122 |
[124] | Mikacenic C, Price BL, Harju-Baker S, et al. (2017) A two-biomarker model predicts mortality in the critically ill with sepsis. Am J Respir Crit Care Med 196: 1004-1011. https://doi.org/10.1164/rccm.201611-2307OC |
[125] | Gao L, Yang B, Zhang H, et al. (2018) DcR3, a new biomarker for sepsis, correlates with infection severity and procalcitonin. Oncotarget 9: 10934-10944. https://doi.org/10.18632/oncotarget.23736 |
[126] | Garcia-Obregon S, Azkargorta M, Seijas I, et al. (2018) Identification of a panel of serum protein markers in early stage of sepsis and its validation in a cohort of patients. J Microbiol Immunol Infect 51: 465-472. https://doi.org/10.1016/j.jmii.2016.12.002 |
[127] | Hou Y, Liang D, Liu Y, et al. (2018) Up-regulation of DcR3 in microbial toxins-stimulated HUVECs involves NF-κB signaling. BMC Biochem 19: 13. https://doi.org/10.1186/s12858-018-0102-z |
[128] | Zhao JJ, Lou XL, Chen HW, et al. (2018) Diagnostic value of decoy receptor 3 combined with procalcitonin and soluble urokinase-type plasminogen activator receptor for sepsis. Cell Mol Biol Lett 23: 22. https://doi.org/10.1186/s11658-018-0087-z |
[129] | Thompson K, Connor J (2019) When cultures fail: Postmortem decoy receptor 3 (DcR3) as a marker of antemortem sepsis. Acad Forensic Pathol 9: 15-23. https://doi.org/10.1177/1925362119851075 |
[130] | Hassan MH, Yassin MM, Hassan YM, et al. (2020) Decoy receptor 3 as a biomarker for diagnosis of bacterial sepsis. Egypt J Med Microbiol 29: 153-162. https://doi.org/10.51429/EJMM29320 |
[131] | Medina LMP, Rath E, Jahagirdar S, et al. (2021) Discriminatory plasma biomarkers predict specific clinical phenotypes of necrotizing soft-tissue infections. J Clin Invest 131: e149523. https://doi.org/10.1172/JCI149523 |
[132] | Iio S, Hayashi N, Mita E, et al. (1998) Serum levels of soluble Fas antigen in chronic hepatitis C patients. J Hepatol 29: 517-523. https://doi.org/10.1016/S0168-8278(98)80145-1 |
[133] | Raghuraman S, Abraham P, Daniel HD, et al. (2005) Characterization of soluble FAS, FAS ligand and tumour necrosis factor-alpha in patients with chronic HCV infection. J Clin Virol 34: 63-70. https://doi.org/10.1016/j.jcv.2005.01.009 |
[134] | Torre F, Bellis L, Delfino A, et al. (2008) Peripheral blood serum markers for apoptosis and liver fibrosis: Are they trustworthy indicators of liver realness?. Digest Liver Dis 40: 441-445. https://doi.org/10.1016/j.dld.2007.10.027 |
[135] | Hou Y, Xu P, Lou X, et al. (2013) Serum decoy receptor 3 is a useful predictor for the active status of chronic hepatitis B in hepatitis B e antigen-negative patients. Tohoku J Exp Med 230: 227-232. https://doi.org/10.1620/tjem.230.227 |
[136] | Dong Y, Shi D, Li M, et al. (2015) Elevated serum levels of decoy receptor 3 are associated with disease severity in patients with hemorrhagic fever with renal syndrome. Intern Emerg Med 10: 567-573. https://doi.org/10.1007/s11739-015-1195-7 |
[137] | Lin YT, Yen CH, Chen HL, et al. (2015) The serologic decoy receptor 3 (DcR3) levels are associated with slower disease progression in HIV-1/AIDS patients. J Formos Med Assoc 114: 498-503. https://doi.org/10.1016/j.jfma.2013.01.007 |
[138] | Ikomey GM, Julius A, Jacobs GB, et al. (2016) Fas mediated (CD95L) peripheral T-cell apoptosis marker in monitoring HIV-1 disease progression in adults in Yaoundé, Cameroon. Int J Immunol 4: 1-5. https://doi.org/10.11648/j.iji.20160401.11 |
[139] | Ikomey G, Assoumou MCO, Atashili J, et al. (2016) The potentials of Fas receptors and ligands in monitoring HIV-1 disease in children in Yaoundé, Cameroon. J Int Assoc Prov AIDS Care 15: 418-422. https://doi.org/10.1177/2325957413488202 |
[140] | McElroy AK, Harmon JR, Flietstra TD, et al. (2016) Kinetic analysis of biomarkers in a cohort of US patients with Ebola virus disease. Clin Infect Dis 63: 460-467. https://doi.org/10.1093/cid/ciw334 |
[141] | Shin SY, Jeong SH, Sung PS, et al. (2016) Comparative analysis of liver injury-associated cytokines in acute hepatitis A and B. Yonsei Med J 57: 652-657. https://doi.org/10.3349/ymj.2016.57.3.652 |
[142] | Lou X, Hou Y, Cao H, et al. (2018) Clinical significance of decoy receptor 3 upregulation in patients with hepatitis B and liver fibrosis. Oncol Lett 16: 1147-1154. https://doi.org/10.3892/ol.2018.8762 |
[143] | Zhang Z, Dai X, Qi J, et al. (2018) Astragalus mongholicus (Fisch.) Bge improves peripheral Treg cell immunity imbalance in the children with viral myocarditis by reducing the levels of miR-146b and miR-155. Front Pediatr 6: 139. https://doi.org/10.3389/fped.2018.00139 |
[144] | Dambaya B, Nkenfou CN, Ambada G, et al. (2019) Differential expression of Fas receptors (CD95) and Fas ligands (CD95L) in HIV infected and exposed uninfected children in Cameroon versus unexposed children. Pan Afric Med J 34: 39. https://doi.org/10.11604/pamj.2019.34.39.15038 |
[145] | Liang DY, Sha S, Yi Q, et al. (2019) Hepatitis B X protein upregulates decoy receptor 3 expression via the PI3K/NF-κB pathway. Cell Signal 62: 109346. https://doi.org/10.1016/j.cellsig.2019.109346 |
[146] | Shata MTM, Abdel-hameed EA, Rouster SD, et al. (2019) HBV and HIV/HBV infected patients have distinct immune exhaustion and apoptotic serum biomarker profiles. Pathog Immun 4: 39-65. https://doi.org/10.20411/pai.v4i1.267 |
[147] | Mercedes R, Brown J, Minard C, et al. (2020) A liver biopsy validation pilot study of shear wave elastography, APRI, FIB-4, and novel serum biomarkers for liver fibrosis staging in children with chronic viral hepatitis. Glob Pediatr Health 6: 1-8. https://doi.org/10.1177/2333794X20938931 |
[148] | Abers MS, Delmonte OM, Ricotta EE, et al. (2021) An immune-based biomarker signature is associated with mortality in COVID-19 patients. JCI Insight 6: e144455. https://doi.org/10.1172/jci.insight.144455 |
[149] | El-Mesery M, El-Mowafy M, Youssef LF, et al. (2021) Serum soluble fibrinogen-like protein 2 represents a novel biomarker for differentiation between acute and chronic Egyptian hepatitis B virus-infected patients. J Interferon Cytokine Res 41: 52-59. https://doi.org/10.1089/jir.2020.0118 |
[150] | Kessel C, Vollenberg R, Masjosthusmann K, et al. (2021) Discrimination of COVID-19 from inflammation-induced cytokine storm syndromes using disease-related blood biomarkers. Arthritis Rheumatol 73: 1791-1799. https://doi.org/10.1002/art.41763 |
[151] | Armah HB, Wilson NO, Sarfo BY, et al. (2007) Cerebrospinal fluid and serum biomarkers of malaria mortality in Ghanaian children. Malar J 6: 147. https://doi.org/10.1186/1475-2875-6-147 |
[152] | Jain V, Armah HB, Tongren JE, et al. (2008) Plasma IP-10, apoptotic and angiogenic factors associated with fatal cerebral malaria in India. Malar J 7: 83. https://doi.org/10.1186/1475-2875-7-83 |
[153] | Wu SH, Li CT, Lin CH, et al. (2010) Soluble Fas ligand is another good diagnostic marker for tuberculous pleurisy. Diagn Microbiol Infect Dis 68: 395-400. https://doi.org/10.1016/j.diagmicrobio.2010.08.008 |
[154] | Shu CC, Wu MF, Hsu CL, et al. (2013) Apoptosis-associated biomarkers in tuberculosis: promising for diagnosis and prognosis prediction. BMC Infect Dis 13: 45. https://doi.org/10.1186/1471-2334-13-45 |
[155] | Goeijenbier M, Gasem MH, Meijers JCM, et al. (2015) Markers of endothelial cell activation and immune activation are increased in patients with severe leptospirosis and associated with disease severity. J Infect 71: 437-446. https://doi.org/10.1016/j.jinf.2015.05.016 |
[156] | Shu CC, Wang JY, Hsu CL, et al. (2015) Diagnostic role of inflammatory and anti-inflammatory cytokines and effector molecules of cytotoxic T lymphocytes in tuberculous pleural effusion. Respirology 20: 147-154. https://doi.org/10.1111/resp.12414 |
[157] | Korczynski P, Klimiuk J, Safianowska A, et al. (2019) Impact of age on the diagnostic yield of four different biomarkers of tuberculous pleural effusion. Tuberculosis 114: 24-29. https://doi.org/10.1016/j.tube.2018.11.004 |
[158] | Struck NS, Zimmermann M, Krumkamp R, et al. (2020) Cytokine profile distinguishes children with Plasmodium falciparum malaria from those with bacterial blood stream infections. J Infect Dis 221: 1098-1106. https://doi.org/10.1093/infdis/jiz587 |
[159] | Nishigaki K, Minatoguchi S, Seishima M, et al. (1997) Plasma Fas ligand, an inducer of apoptosis, and plasma soluble Fas, an inhibitor of apoptosis, in patients with chronic congestive heart failure. J Am Coll Cardiol 29: 1214-1220. https://doi.org/10.1016/S0735-1097(97)00055-7 |
[160] | Sliwa K, Skudickey D, Bergemann A, et al. (2000) Peripartum cardiomyopathy: Analysis of clinical outcome, left ventricular function, plasma levels of cytokines and Fas/APO-1. J Am Coll Cardiol 35: 701-705. https://doi.org/10.1016/S0735-1097(99)00624-5 |
[161] | Adamopoulos S, Parissis J, Karatzas D, et al. (2002) Physical training modulates proinflammatory cytokines and the soluble Fas/soluble Fas ligand system in patients with chronic heart failure. J Am Coll Cardiol 39: 653-663. https://doi.org/10.1016/S0735-1097(01)01795-8 |
[162] | Shimizu M, Fukuo K, Nagata S, et al. (2002) Increased plasma levels of the soluble form of Fas ligand in patients with acute myocardial infarction and unstable angina pectoris. J Am Coll Cardiol 39: 585-590. https://doi.org/10.1016/S0735-1097(01)01800-9 |
[163] | Blanco-Colio LM, Martín-Ventura JL, Sol JM, et al. (2004) Decreased circulating Fas ligand in patients with familial combined hyperlipidemia or carotid atherosclerosis. Normalization by atorvastatin. J Am Coll Cardiol 43: 1188-1194. https://doi.org/10.1016/j.jacc.2003.10.046 |
[164] | Van der Meer IM, Oei HHS, Hofman A, et al. (2006) Soluble Fas, a mediator of apoptosis, C-reactive protein, and coronary and extracoronary atherosclerosis. The Rotterdam coronary calcification study. Atherosclerosis 189: 464-469. https://doi.org/10.1016/j.atherosclerosis.2006.01.004 |
[165] | Blanco-Colio LM, Martín-Ventura JL, de Teresa E, et al. (2007) Increased soluble Fas plasma levels in subjects at high cardiovascular risk. Atorvastatin on inflammatory markers (AIM) study, a substudy of ACTFAST. Arterioscler Thromb Vasc Biol 27: 168-174. https://doi.org/10.1161/01.ATV.0000250616.26308.d7 |
[166] | Blanco-Colio LM, Martín-Ventura JL, Tuñón J, et al. (2008) Soluble Fas ligand plasma levels are associated with forearm reactive hyperemia in subjects with coronary artery disease. A novel biomarker of endothelial function?. Atherosclerosis 201: 407-412. https://doi.org/10.1016/j.atherosclerosis.2008.02.005 |
[167] | Boos CJ, Balakrishnan B, Blann AD, et al. (2008) The relationship of circulating endothelial cells to plasma indices of endothelial damage/dysfunction and apoptosis in acute coronary syndromes: Implications for prognosis. J Thromb Haemost 6: 1841-1850. https://doi.org/10.1111/j.1538-7836.2008.03148.x |
[168] | Lanfear DE, Hasan R, Gupta RC, et al. (2009) Short term effects of milrinone on biomarkers of necrosis, apoptosis, and inflammation in patients with severe heart failure. J Trans Med 7: 67. https://doi.org/10.1186/1479-5876-7-67 |
[169] | Cardinal H, Brophy JM, Bogaty P, et al. (2010) Usefulness of soluble Fas levels for improving diagnostic accuracy and prognosis for acute coronary syndromes. Am J Cardiol 105: 797-803. https://doi.org/10.1016/j.amjcard.2009.10.061 |
[170] | Oyama JI, Maeda T, Sasaki M, et al. (2010) Green tea catechins improve human forearm vascular function and have potent anti-inflammatory and anti-apoptotic effects in smokers. Intern Med 49: 2553-2559. https://doi.org/10.2169/internalmedicine.49.4048 |
[171] | Cross DS, McCarty CA, Hytopoulos E, et al. (2012) Improved coronary risk assessment among intermediate risk patients using a clinical and biomarker based algorithm developed and validated in two population cohorts. Curr Med Res Opin 28: 1819-1830. https://doi.org/10.1185/03007995.2012.742878 |
[172] | Fertin M, Bauters A, Pinet F, et al. (2012) Circulating levels of soluble Fas ligand and left ventricular remodeling after acute myocardial infarction (from the REVE-2 study). J Cardiol 60: 93-97. https://doi.org/10.1016/j.jjcc.2012.03.001 |
[173] | Kinugawa T, Kato M, Yamamoto K, et al. (2012) Proinflammatory cytokine activation is linked to apoptotic mediator; soluble Fas level in patients with chronic heart failure. Int Heart J 53: 182-186. https://doi.org/10.1536/ihj.53.182 |
[174] | Sahinarslan A, Boyaci B, Kocaman SA, et al. (2012) The relationship of serum soluble Fas ligand (sFasL) level with the extent of coronary artery disease. Int J Angiol 21: 29-34. https://doi.org/10.1055/s-0032-1306418 |
[175] | Nilsson L, Szymanowski A, Swahn E, et al. (2013) Soluble TNF receptors are associated with infarct size and ventricular dysfunction in ST-elevation myocardial infarction. Plos One 8: e55477. https://doi.org/10.1371/journal.pone.0055477 |
[176] | Osmancik P, Teringova E, Tousek P, et al. (2013) Prognostic value of TNF-related apoptosis inducing ligand (TRAIL) in acute coronary syndrome patients. Plos One 8: e53860. https://doi.org/10.1371/journal.pone.0053860 |
[177] | Shah NR, Bieniarz MC, Basra SS, et al. (2013) Serum biomarkers in severe refractory cardiogenic shock. J Am Coll Cardiol HF 1: 200-206. https://doi.org/10.1016/j.jchf.2013.03.002 |
[178] | Szymanowski A, Li W, Lundberg A, et al. (2014) Soluble Fas ligand is associated with natural killer cell dynamics in coronary artery disease. Atherosclerosis 233: 616-622. https://doi.org/10.1016/j.atherosclerosis.2014.01.030 |
[179] | Taneja AK, Gaze D, Coats AJS, et al. (2014) Effects of nebivolol on biomarkers in elderly patients with heart failure. Int J Cardiol 175: 253-260. https://doi.org/10.1016/j.ijcard.2014.05.018 |
[180] | Chang TY, Hsu CY, Huang PH, et al. (2015) Usefulness of circulating decoy receptor 3 in predicting coronary artery disease severity and future major adverse cardiovascular events in patients with multivessel coronary artery disease. Am J Cardiol 116: 1028-1033. https://doi.org/10.1016/j.amjcard.2015.06.041 |
[181] | Adly AA, Ismail EA, Andrawes NG, et al. (2016) Soluble Fas/FasL ratio as a marker of vasculopathy in children and adolescents with sickle cell disease. Cytokine 79: 52-58. https://doi.org/10.1016/j.cyto.2015.12.022 |
[182] | Iso H, Maruyama K, Eshak ES, et al. (2017) Blood soluble Fas levels and mortality from cardiovascular disease in middle-aged Japanese: The JACC study. Atherosclerosis 260: 97-101. https://doi.org/10.1016/j.atherosclerosis.2017.03.020 |
[183] | Mattisson IY, Björkbacka H, Wigren M, et al. (2017) Elevated markers of death receptor-activated apoptosis are associated with increased risk for development of diabetes and cardiovascular disease. EBioMedicine 26: 187-197. https://doi.org/10.1016/j.ebiom.2017.11.023 |
[184] | Yan Y, Song D, Liu L, et al. (2017) The relationship of plasma decoy receptor 3 and coronary collateral circulation in patients with coronary artery disease. Life Sci 189: 84-88. https://doi.org/10.1016/j.lfs.2017.09.025 |
[185] | Li XY, Hou HT, Chen HX, et al. (2018) Increased circulating levels of tumor necrosis factor-like cytokine IA and decoy receptor 3 correlate with SYNTAX score in patients undergoing coronary surgery. J Int Med Res 46: 5167-5175. https://doi.org/10.1177/0300060518793787 |
[186] | Chen X, Wang R, Chen W, et al. (2019) Decoy receptor-3 regulates inflammation and apoptosis via PI3K/AKT signaling pathway in coronary heart disease. Exp Ther Med 17: 2614-2622. https://doi.org/10.3892/etm.2019.7222 |
[187] | Miyoshi T, Hosoda H, Nakai M, et al. (2019) Maternal biomarkers for fetal heart failure in fetuses with congenital heart defects or arrhythmias. Am J Obstet Gynecol 220: 104.e1-15. https://doi.org/10.1016/j.ajog.2018.09.024 |
[188] | Younus M, Fan W, Harrington DS, et al. (2019) Usefulness of a coronary artery disease predictive algorithm to predict global risk for cardiovascular disease and acute coronary syndrome. Am J Cardiol 123: 769-775. https://doi.org/10.1016/j.amjcard.2018.11.044 |
[189] | Doustkami H, Avesta L, Babapour B, et al. (2021) Correlation of serum decoy receptor 3 and interleukin-6 with severity of coronary artery diseases in male acute myocardial infarction patients. Acta Biomed 92: e2021285. https://doi.org/10.23750/abm.v92i5.9711 |
[190] | Masse M, Hérbert MJ, Troyanov S, et al. (2002) Soluble Fas is a marker of peripheral arterial occlusive disease in haemodialysis patients. Nephrol Dial Transplant 17: 485-491. https://doi.org/10.1093/ndt/17.3.485 |
[191] | El-Agroudy AE, El-Baz A (2010) Soluble Fas: a useful marker of inflammation and cardiovascular diseases in uremic patients. Clin Exp Nephrol 14: 152-157. https://doi.org/10.1007/s10157-009-0261-8 |
[192] | Chang EP, Lin YS, Huang SC, et al. (2012) High serum DcR3 levels are associated with the occurrence of peritonitis in patients receiving chronic peritoneal dialysis. J Chin Med Assoc 75: 644-648. https://doi.org/10.1016/j.jcma.2012.09.009 |
[193] | Dounousi E, Koliousi E, Papagianni A, et al. (2012) Mononuclear leukocyte apoptosis and inflammatory markers in patients with chronic kidney disease. Am J Nephrol 36: 531-536. https://doi.org/10.1159/000345352 |
[194] | Hung SC, Hsu TW, Lin YP, et al. (2012) Decoy receptor 3, a novel inflammatory marker, and mortality in hemodialysis patients. Clin J Am Soc Nephrol 7: 1257-1265. https://doi.org/10.2215/CJN.08410811 |
[195] | Bartnicki P, Rysz J, Franczyk B, et al. (2016) Impact of continuous erythropoietin receptor activator on selected biomarkers of cardiovascular disease and left ventricle structure and function in chronic kidney disease. Oxid Med Cell Longev 2016: 9879615. https://doi.org/10.1155/2016/9879615 |
[196] | Bhatraju PK, Robinson-Cohen C, Mikacenic C, et al. (2017) Circulating levels of soluble Fas (sCD95) are associated with risk for development of a nonresolving acute kidney injury subphenotype. Critical Care 21: 217. https://doi.org/10.1186/s13054-017-1807-x |
[197] | Claro LM, Moreno-Amaral AN, Gadotti AC, et al. (2018) The impact of uremic toxicity induced inflammatory response on the cardiovascular burden in chronic kidney disease. Toxins 10: 384. https://doi.org/10.3390/toxins10100384 |
[198] | Raptis V, Bakogiannis C, Loutradis C, et al. (2018) Serum Fas ligand, serum myostatin and urine TGF-β1 are elevated in autosomal dominant polycystic kidney disease patients with impaired and preserved renal function. Kidney Blood Press Res 43: 744-754. https://doi.org/10.1159/000489911 |
[199] | Bhatraju PK, Zelnick LR, Katz R, et al. (2019) A prediction model for severe AKI in critically ill adults that incorporates clinical and biomarker data. Clin J Am Soc Nephrol 14: 506-514. https://doi.org/10.2215/CJN.04100318 |
[200] | Chiloff DM, de Almeida DC, Dalboni MA, et al. (2020) Soluble Fas affects erythropoiesis in vitro and acts as a potential predictor of erythropoiesis-stimulating agent therapy in patients with chronic kidney disease. Am J Physiol Renal Physiol 318: F861-F869. https://doi.org/10.1152/ajprenal.00433.2019 |
[201] | Abu-Rajab Tamimi TI, Elgouhari HM, Alkhouri N, et al. (2011) An apoptosis panel for nonalcoholic steatohepatitis diagnosis. J Hepatol 54: 1224-1229. https://doi.org/10.1016/j.jhep.2010.08.023 |
[202] | Fealy CE, Haus JM, Solomon TPJ, et al. (2012) Short-term exercise reduces markers of hepatocyte apoptosis in nonalcoholic fatty liver disease. J Appl Physiol 113: 1-6. https://doi.org/10.1152/japplphysiol.00127.2012 |
[203] | Page S, Birerdinc A, Estep M, et al. (2013) Knowledge-based identification of soluble biomarkers: Hepatic fibrosis in NAFLD as an example. Plos One 8: e56009. https://doi.org/10.1371/journal.pone.0056009 |
[204] | El Bassat H, Ziada DH, Hasby EA, et al. (2014) Apoptotic and anti-apoptotic seromarkers for assessment of disease severity of non-alcoholic steatohepatitis. Arab J Gastroenterol 15: 6-11. https://doi.org/10.1016/j.ajg.2014.01.009 |
[205] | Buck M, Garcia-Tsao G, Groszmann RJ, et al. (2014) Novel inflammatory biomarkers of portal pressure in compensated cirrhosis patients. Hepatology 59: 1052-1059. https://doi.org/10.1002/hep.26755 |
[206] | Alkhouri N, Alisi A, Okwu V, et al. (2015) Circulating soluble Fas and Fas ligand levels are elevated in children with nonalcoholic steatohepatitis. Dig Dis Sci 60: 2353-2359. https://doi.org/10.1007/s10620-015-3614-z |
[207] | Ajmera V, Perito ER, Bass NM, et al. (2017) Novel plasma biomarkers associated with liver disease severity in adults with nonalcoholic fatty liver disease. Hepatology 65: 65-77. https://doi.org/10.1002/hep.28776 |
[208] | Perito ER, Ajmera V, Bass NM, et al. (2017) Association between cytokines and liver histology in children with nonalcoholic fatty liver disease. Hepatol Comm 1: 609-622. https://doi.org/10.1002/hep4.1068 |
[209] | Lin S, Wu B, Lin Y, et al. (2019) Expression and clinical significance of decoy receptor 3 in acute-on-chronic liver failure. BioMed Res Int 2019: 9145736. https://doi.org/10.1155/2019/9145736 |
[210] | Yasuda N, Gotoh K, Minatoguchi S, et al. (1998) An increase of soluble Fas, an inhibitor of apoptosis, associated with progression of COPD. Respir Med 92: 993-999. https://doi.org/10.1016/S0954-6111(98)90343-2 |
[211] | Takabatake N, Arao T, Sata M, et al. (2005) Circulating levels of soluble Fas ligand in cachexic patients with COPD are higher than those in non-cachexic patients with COPD. Intern Med 44: 1137-1143. https://doi.org/10.2169/internalmedicine.44.1137 |
[212] | Chen CY, Yang KY, Chen MY, et al. (2009) Decoy receptor 3 levels in peripheral blood predict outcomes of acute respiratory distress syndrome. Am J Respir Crit Care Med 180: 751-760. https://doi.org/10.1164/rccm.200902-0222OC |
[213] | Carolan BJ, Hughes G, Morrow J, et al. (2014) The association of plasma biomarkers with computed tomography-assessed emphysema phenotypes. Respir Res 15: 127. https://doi.org/10.1186/s12931-014-0127-9 |
[214] | Stapleton RD, Suratt BT, Neff MJ, et al. (2019) Bronchoalveolar fluid and plasma inflammatory biomarkers in contemporary ARDS patients. Biomarkers 24: 352-359. https://doi.org/10.1080/1354750X.2019.1581840 |
[215] | Aydin H, Tekin YK, Korkmaz I, et al. (2020) Apoptosis biomarkers (APAF-1, sFas, sFas-L, and caspase-9), albumin, and fetuin-A levels in pulmonary thromboembolic patients. Disaster Emerg Med J 5: 1-6. https://doi.org/10.5603/DEMJ.a2020.0005 |
[216] | Ghobadi H, Hosseini N, Aslani MR (2020) Correlations between serum decoy receptors 3 and airflow limitation and quality of life in male patients with stable stage and acute exacerbation of COPD. Lung 198: 515-523. https://doi.org/10.1007/s00408-020-00348-z |
[217] | Felderhoff-Mueser U, Bührer C, Groneck P, et al. (2003) Soluble Fas (CD95/Apo-1), soluble Fas ligand, and activated caspase 3 in the cerebrospinal fluid of infants with posthemorrhagic and nonhemorrhagic hydrocephalus. Pediatr Res 54: 659-664. https://doi.org/10.1203/01.PDR.0000084114.83724.65 |
[218] | Sival DA, Felderhoff-Müser U, Schmitz T, et al. (2008) Neonatal high pressure hydrocephalus is associated with elevation of pro-inflammatory cytokines IL-18 and IFNγ in cerebrospinal fluid. Cerebrospinal Fluid Res 5: 21. https://doi.org/10.1186/1743-8454-5-21 |
[219] | Craig-Schapiro R, Kuhn M, Xiong C, et al. (2011) Multiplexed immunoassay panel identifies novel CSF biomarkers for Alzheimer's disease diagnosis and prognosis. Plos One 6: e18850. https://doi.org/10.1371/journal.pone.0018850 |
[220] | Leifsdottir K, Mehmet H, Eksborg S, et al. (2018) Fas-ligand and interleukin-6 in the cerebrospinal fluid are early predictors of hypoxic-ischemic encephalopathy and long-term outcomes after birth asphyxia in term infants. J Neuroinflammation 15: 223. https://doi.org/10.1186/s12974-018-1253-y |
[221] | Jiang W, Jin P, Wei W, et al. (2020) Apoptosis in cerebrospinal fluid as outcome predictors in severe traumatic brain injury. An observational study. Medicine 99: e20922. https://doi.org/10.1097/MD.0000000000020922 |
[222] | Niewczas MA, Ficociello LH, Johnson AC, et al. (2009) Serum concentrations of markers of TNFα and Fas-mediated pathways and renal function in nonproteinuric patients with type 1 diabetes. Clin J Am Soc Nephrol 4: 62-70. https://doi.org/10.2215/CJN.03010608 |
[223] | Hussian SK, Al-Karawi IN, Sahab KS (2013) Role of Fas/Fas ligand pathway in a sample of Iraqi diabetic foot patients. Diyala J Med 5: 95-107. |
[224] | Stoynev N, Kainov K, Kirilov G, et al. (2014) Serum levels of sFas and sFasL in subjects with type 2 diabetes-the impact of arterial hypertension. Cent Eur J Med 9: 704-708. https://doi.org/10.2478/s11536-013-0318-7 |
[225] | Midena E, Micera A, Frizziero L, et al. (2019) Sub-threshold micropulse laser treatment reduces inflammatory biomarkers in aqueous humour of diabetic patients with macular edema. Sci Rep 9: 10034. https://doi.org/10.1038/s41598-019-46515-y |
[226] | Abe R, Shimizu T, Shibaki A, et al. (2003) Toxic epidermal necrolysis and Stevens-Johnson syndrome are induced by soluble Fas ligand. Am J Pathol 162: 1515-1520. https://doi.org/10.1016/S0002-9440(10)64284-8 |
[227] | Stur K, Karlhofer FM, Stingl G, et al. (2007) Soluble FAS ligand: A discriminating feature between drug-induced skin eruptions and viral exanthemas. J Invest Dermatol 127: 802-807. https://doi.org/10.1038/sj.jid.5700648 |
[228] | Chung WH, Hung SI, Yang JY, et al. (2008) Granulysin is a key mediator for disseminated keratinocyte death in Stevens-Johnson syndrome and toxic epidermal necrolysis. Nat Med 14: 1343-1350. https://doi.org/10.1038/nm.1884 |
[229] | Murata J, Abe R, Shimizu H (2008) Increased soluble Fas ligand levels in patients with Stevens-Johnson syndrome and toxic epidermal necrolysis preceding skin detachment. J Allergy Clin Immunol 122: 992-1000. https://doi.org/10.1016/j.jaci.2008.06.013 |
[230] | Genç ŞÖ, Karakuş S, Çetin A, et al. (2019) Serum Bcl-2, caspase-9 and soluble FasL as perinatal markers in late preterm pregnancies with intrauterine growth restriction. Turk J Pediatr 61: 686-696. https://doi.org/10.24953/turkjped.2019.05.007 |
[231] | Yeh CC, Yang MJ, Lussier EC, et al. (2019) Low plasma levels of decoy receptor 3 (DcR3) in the third trimester of pregnancy with preeclampsia. Taiwan J Obstet Gynecol 58: 349-353. https://doi.org/10.1016/j.tjog.2019.03.011 |
[232] | Oyama JI, Yamamoto H, Maeda T, et al. (2012) Continuous positive airway pressure therapy improves vascular dysfunction and decreases oxidative stress in patients with the metabolic syndrome and obstructive sleep apnea syndrome. Clin Cardiol 35: 231-236. https://doi.org/10.1002/clc.21010 |
[233] | Chedraui P, Escobar GS, Pérez-López FR, et al. (2014) Angiogenesis, inflammation and endothelial function in postmenopausal women screened for the metabolic syndrome. Maturitas 77: 370-374. https://doi.org/10.1016/j.maturitas.2014.01.014 |
[234] | Alkhouri N, Kheirandish-Gozal L, Matloob A, et al. (2015) Evaluation of circulating markers of hepatic apoptosis and inflammation in obese children with and without obstructive sleep apnea. Sleep Med 16: 1031-1035. https://doi.org/10.1016/j.sleep.2015.05.002 |
[235] | Farey JE, Fisher OM, Levert-Mignon AJ, et al. (2017) Decreased levels of circulating cancer-associated protein biomarkers following bariatric surgery. Obes Surg 27: 578-585. https://doi.org/10.1007/s11695-016-2321-y |
[236] | Goto M (2008) Elevation of soluble Fas (APO-1, CD95) ligand in natural aging and Werner syndrome. BioSci Trend 2: 124-127. |
[237] | Jiang S, Moriarty-Craige SE, Li C, et al. (2008) Associations of plasma-soluble Fas ligand with aging and age-related macular degeneration. Invest Ophthalmol Vis Sci 49: 1345-1349. https://doi.org/10.1167/iovs.07-0308 |
[238] | Karaflou M, Kaparos G, Rizos D, et al. (2010) Estrogen plus progestin treatment: effect of different progestin components on serum markers of apoptosis in healthy postmenopausal women. Fertil Steril 94: 2399-2401. https://doi.org/10.1016/j.fertnstert.2010.04.010 |
[239] | Kämppä N, Mäkelä KM, Lyytikäinen LP, et al. (2013) Vascular cell adhesion molecule 1, soluble Fas and hepatocyte growth factor as predictors of mortality in nonagenarians: The vitality 90+ study. Exp Gerontrol 48: 1167-1172. https://doi.org/10.1016/j.exger.2013.07.009 |
[240] | Kangas R, Pöllänen E, Rippo MR, et al. (2014) Circulating miR-21, miR-146a and Fas ligand respond to postmenopausal estrogen-based hormone replacement therapy—a study with monozygotic twin pairs. Mech Ageing Dev 143–144: 1-8. https://doi.org/10.1016/j.mad.2014.11.001 |
[241] | Wu Q, Chen H, Fang J, et al. (2012) Elevated Fas/FasL system and endothelial cell microparticles are involved in endothelial damage in acute graft-versus-host disease: A clinical analysis. Leuk Res 36: 275-280. https://doi.org/10.1016/j.leukres.2011.08.005 |
[242] | Fadel FI, Elshamaa MF, Salah A, et al. (2016) Fas/Fas ligand pathways gene polymorphisms in pediatric renal allograft rejection. Transpl Immunol 37: 28-34. https://doi.org/10.1016/j.trim.2016.04.006 |
[243] | Meki ARAM, Hasan HA, El-Deen ZMM, et al. (2003) Dysregulation of apoptosis in scorpion envenomed children: its reflection on their outcome. Toxicon 42: 229-237. https://doi.org/10.1016/S0041-0101(03)00128-4 |
[244] | Tamakoshi A, Suzuki K, Lin Y, et al. (2009) Cigarette smoking and serum soluble Fas levels: Findings from the JACC study. Mutat Res 679: 79-83. https://doi.org/10.1016/j.mrgentox.2009.08.002 |
[245] | Muraki M (2018) Development of expression systems for the production of recombinant human Fas ligand extracellular domain derivatives using Pichia pastoris and preparation of the conjugates by site-specific chemical modifications: A review. AIMS Bioengineer 5: 39-62. https://doi.org/10.3934/bioeng.2018.1.39 |
[246] | Muraki M, Hirota K (2019) Site-specific biotin-group conjugate of human Fas ligand extracellular domain: preparation and characterization of cell-death-inducing activity. Curr Top Pep Prot Res 20: 17-24. |
[247] | Muraki M, Honda S (2010) Efficient production of human Fas receptor extracellular domain-human IgG1 heavy chain Fc domain fusion protein using baculovirus/silkworm expression system. Prot Expr Purif 73: 209-216. https://doi.org/10.1016/j.pep.2010.05.007 |
[248] | Connolly K, Cho YH, Duan R, et al. (2001) In vivo inhibition of Fas ligand-mediated killing by TR6, a Fas ligand decoy receptor. J Pharmacol Exp Ther 298: 25-33. |
1. | Zejun Li, Yuxiang Zhang, Yuting Bai, Xiaohui Xie, Lijun Zeng, IMC-MDA: Prediction of miRNA-disease association based on induction matrix completion, 2023, 20, 1551-0018, 10659, 10.3934/mbe.2023471 | |
2. | Wenjie Li, Zimei Huang, Do different stock indices volatility respond differently to Central bank digital currency signals?, 2023, 31, 2688-1594, 5573, 10.3934/era.2023283 | |
3. | Mohammad Osoolian, Ahmad Badri, Mahdi Karimi, Investigating the effect of return jumps on herd behavior and its asymmetry in the Tehran Stock Exchange Market, 2024, 8, 1027-8257, 25, 10.61186/ijf.2024.428599.1446 | |
4. | Mahdi Karimi, Mohammad Ahadzadeh, Macro Herding Behavior and Its Implications in Tehran Stock Exchange: An Analysis of Extreme Market Conditions, 2024, 8, 1027-8257, 98, 10.61186/ijf.2024.462197.1475 | |
5. | Sanjeev Gupta, Ashish Kumar, Quantile regression modelling of herd behaviour in the Indian equity market across sectors: implications on financial market dynamics spanning the COVID-19 epoch, 2025, 1746-5664, 10.1108/JM2-10-2024-0347 | |
6. | Nupur Soti, Ashish Kumar, Thematic Review and Discussion of Research on Herd Behavior in Capital Markets: Highlighting the Gaps and Proposing Future Research Avenues, 2025, 15, 2158-2440, 10.1177/21582440251319995 |
Panel A-Pre-COVID 19 Period | Panel B-COVID-19 Sub-Period | ||||
Rmt | CSADt | Rmt | CSADt | ||
Mean | 0.0018 | 0.0321 | 0.0025 | 0.0242 | |
Median | 0.0024 | 0.0253 | 0.0062 | 0.0216 | |
Maximum | 0.1630 | 0.1661 | 0.1446 | 0.0948 | |
Minimum | −0.2822 | 0.0066 | −0.453597 | 0.006 | |
Std. Dev. | 0.0485 | 0.0219 | 0.0438 | 0.0112 | |
Skewness | −0.5974 | 1.9961 | −3.4336 | 2.2688 | |
Kurtosis | 6.2950 | 8.1602 | 35.9557 | 11.8496 | |
Jarque-Bera | 559.9892* | 1940.349* | 16904.15* | 1475.345* | |
ADF | −33.3920* | −3.3529* | −21.8388* | −9.0464* | |
Note: *significant at 1% level. Source: The Authors. |
τ | Panel A | Panel B | |||||||||
Whole Period | Bull Phase | Bear Phase | |||||||||
β0 | β1 | β2 | β0 | β1 | β2 | β0 | β1 | β2 | |||
0.1 | 0.0102* (32.5938) |
0.1639* (14.1868) |
0.0017 (0.0633) |
0.0090* (11.7759) |
0.2871* (5.5961) |
−0.7986 (−1.3682) |
0.0104* (25.5667) |
0.1356* (10.268) |
0.0681** (2.3502) |
||
0.25 | 0.0126* (32.9239) |
0.1997* (14.8536) |
−0.0842* (−2.6724) |
0.0119* (17.1878) |
0.2969* (7.0583) |
−0.3648 (−0.9338) |
0.0125* (24.5231) |
0.1603* (10.199) |
0.0032 (0.0895) |
||
0.5 | 0.0171* (35.3812) |
0.2722* (14.3006) |
−0.2660* (−5.8396) |
0.0155* (16.5859) |
0.4538* (6.2022) |
−0.9169 (−1.1163) |
0.0179* (20.4726) |
0.1540* (3.5518) |
0.1175 (0.4156) |
||
0.75 | 0.0229* (25.4752) |
0.4399* (9.0004) |
−0.6635* (−6.3651) |
0.0198* (17.1239) |
0.7757* (9.0218) |
−1.8696** (−2.1908) |
0.0242* (21.9397) |
0.1660* (3.5836) |
0.1409 (0.5927) |
||
0.9 | 0.0315* (16.3718) |
0.7241* (7.9385) |
−1.3319* (−6.9239) |
0.0294* (12.1047) |
0.8935* (4.2319) |
−-0.692 (−0.3141) |
0.0378* (12.5923) |
0.2005*** (1.7132) |
−0.1509 (−0.3392) |
||
Note: Panel A: Presents the results of Equation (7) for the whole period and Panel B presents the results of (8) and (9) for bull and bear phases. The negative and significant value of β2 implies herd behaviour. *, **, *** indicates significance at 1%, 5% and 10% levels respectively and the value in parenthesis is the t-statistics. |
τ | Panel A | Panel B | |||||||||
Extreme Up Market Returns | Extreme Down-Market Returns | High Volatility | |||||||||
β0 | β1 | β2 | β0 | β1 | β2 | β0 | β1 | β2 | |||
0.1 | 0.0102* (32.5938) |
0.1639* (14.1868) |
0.0017 (0.0633) |
0.0090* (11.7759) |
0.2871* (5.5961) |
−0.7986 (−1.3682) |
0.0104* (25.5667) |
0.1356* (10.268) |
0.0681** (2.3502) |
||
0.25 | 0.0126* (32.9239) |
0.1997* (14.8536) |
−0.0842* (−2.6724) |
0.0119* (17.1878) |
0.2969* (7.0583) |
−0.3648 (−0.9338) |
0.0125* (24.5231) |
0.1603* (10.199) |
0.0032 (0.0895) |
||
0.5 | 0.0171* (35.3812) |
0.2722* (14.3006) |
−0.2660* (−5.8396) |
0.0155* (16.5859) |
0.4538* (6.2022) |
−0.9169 (−1.1163) |
0.0179* (20.4726) |
0.1540* (3.5518) |
0.1175 (0.4156) |
||
0.75 | 0.0229* (25.4752) |
0.4399* (9.0004) |
−0.6635* (−6.3651) |
0.0198* (17.1239) |
0.7757* (9.0218) |
−1.8696** (−2.1908) |
0.0242* (21.9397) |
0.1660* (3.5836) |
0.1409 (0.5927) |
||
0.9 | 0.0315* (16.3718) |
0.7241* (7.9385) |
−1.3319* (−6.9239) |
0.0294* (12.1047) |
0.8935* (4.2319) |
−0.692 (−0.3141) |
0.0378* (12.5923) |
0.2005*** (1.7132) |
−0.1509 (−0.3392) |
||
Note: Panel A: Presents the results of Equation (10) and (11) for extreme up and down market returns and Panel B presents the estimation of (12) for high volatility periods. The negative and significant value of β2 implies herd behaviour for extreme up and down-market returns. Negative and significant β3 implies herding during high volatility. *, **, *** indicates significance at 1%, 5% and 10% level respectively and the value in parenthesis is the t-statistics. |
Τ | 0.1 | 0.25 | 0.5 | 0.75 | 0.9 |
β0 | 0.0108* (16.1144) |
0.0139* (20.5061) |
0.0170* (20.0784) |
0.0221* (22.8469) |
0.0274* (19.6571) |
β1 | 0.1454* (5.5300) |
0.1604* (6.1766) |
0.2192* (6.0090) |
0.2499* (7.1704) |
0.3144* (7.6693) |
β2 | 0.0442 (0.7841) |
−0.0041 (−0.0719) |
−0.1483** (−1.8757) |
−0.2411* (−3.1895) |
−0.4089* (−4.6463) |
Note: This table presents the results of Equation (6) as applied for the sub-period January 01, 2020, till December 31, 2020. Negative and significant value of β2 implies herd behaviour. *, **, *** indicates significance at 1%, 5% and 10% levels respectively and the value in parenthesis is the t-statistics. |
Panel A-Pre-COVID 19 Period | Panel B-COVID-19 Sub-Period | ||||
Rmt | CSADt | Rmt | CSADt | ||
Mean | 0.0018 | 0.0321 | 0.0025 | 0.0242 | |
Median | 0.0024 | 0.0253 | 0.0062 | 0.0216 | |
Maximum | 0.1630 | 0.1661 | 0.1446 | 0.0948 | |
Minimum | −0.2822 | 0.0066 | −0.453597 | 0.006 | |
Std. Dev. | 0.0485 | 0.0219 | 0.0438 | 0.0112 | |
Skewness | −0.5974 | 1.9961 | −3.4336 | 2.2688 | |
Kurtosis | 6.2950 | 8.1602 | 35.9557 | 11.8496 | |
Jarque-Bera | 559.9892* | 1940.349* | 16904.15* | 1475.345* | |
ADF | −33.3920* | −3.3529* | −21.8388* | −9.0464* | |
Note: *significant at 1% level. Source: The Authors. |
τ | Panel A | Panel B | |||||||||
Whole Period | Bull Phase | Bear Phase | |||||||||
β0 | β1 | β2 | β0 | β1 | β2 | β0 | β1 | β2 | |||
0.1 | 0.0102* (32.5938) |
0.1639* (14.1868) |
0.0017 (0.0633) |
0.0090* (11.7759) |
0.2871* (5.5961) |
−0.7986 (−1.3682) |
0.0104* (25.5667) |
0.1356* (10.268) |
0.0681** (2.3502) |
||
0.25 | 0.0126* (32.9239) |
0.1997* (14.8536) |
−0.0842* (−2.6724) |
0.0119* (17.1878) |
0.2969* (7.0583) |
−0.3648 (−0.9338) |
0.0125* (24.5231) |
0.1603* (10.199) |
0.0032 (0.0895) |
||
0.5 | 0.0171* (35.3812) |
0.2722* (14.3006) |
−0.2660* (−5.8396) |
0.0155* (16.5859) |
0.4538* (6.2022) |
−0.9169 (−1.1163) |
0.0179* (20.4726) |
0.1540* (3.5518) |
0.1175 (0.4156) |
||
0.75 | 0.0229* (25.4752) |
0.4399* (9.0004) |
−0.6635* (−6.3651) |
0.0198* (17.1239) |
0.7757* (9.0218) |
−1.8696** (−2.1908) |
0.0242* (21.9397) |
0.1660* (3.5836) |
0.1409 (0.5927) |
||
0.9 | 0.0315* (16.3718) |
0.7241* (7.9385) |
−1.3319* (−6.9239) |
0.0294* (12.1047) |
0.8935* (4.2319) |
−-0.692 (−0.3141) |
0.0378* (12.5923) |
0.2005*** (1.7132) |
−0.1509 (−0.3392) |
||
Note: Panel A: Presents the results of Equation (7) for the whole period and Panel B presents the results of (8) and (9) for bull and bear phases. The negative and significant value of β2 implies herd behaviour. *, **, *** indicates significance at 1%, 5% and 10% levels respectively and the value in parenthesis is the t-statistics. |
τ | Panel A | Panel B | |||||||||
Extreme Up Market Returns | Extreme Down-Market Returns | High Volatility | |||||||||
β0 | β1 | β2 | β0 | β1 | β2 | β0 | β1 | β2 | |||
0.1 | 0.0102* (32.5938) |
0.1639* (14.1868) |
0.0017 (0.0633) |
0.0090* (11.7759) |
0.2871* (5.5961) |
−0.7986 (−1.3682) |
0.0104* (25.5667) |
0.1356* (10.268) |
0.0681** (2.3502) |
||
0.25 | 0.0126* (32.9239) |
0.1997* (14.8536) |
−0.0842* (−2.6724) |
0.0119* (17.1878) |
0.2969* (7.0583) |
−0.3648 (−0.9338) |
0.0125* (24.5231) |
0.1603* (10.199) |
0.0032 (0.0895) |
||
0.5 | 0.0171* (35.3812) |
0.2722* (14.3006) |
−0.2660* (−5.8396) |
0.0155* (16.5859) |
0.4538* (6.2022) |
−0.9169 (−1.1163) |
0.0179* (20.4726) |
0.1540* (3.5518) |
0.1175 (0.4156) |
||
0.75 | 0.0229* (25.4752) |
0.4399* (9.0004) |
−0.6635* (−6.3651) |
0.0198* (17.1239) |
0.7757* (9.0218) |
−1.8696** (−2.1908) |
0.0242* (21.9397) |
0.1660* (3.5836) |
0.1409 (0.5927) |
||
0.9 | 0.0315* (16.3718) |
0.7241* (7.9385) |
−1.3319* (−6.9239) |
0.0294* (12.1047) |
0.8935* (4.2319) |
−0.692 (−0.3141) |
0.0378* (12.5923) |
0.2005*** (1.7132) |
−0.1509 (−0.3392) |
||
Note: Panel A: Presents the results of Equation (10) and (11) for extreme up and down market returns and Panel B presents the estimation of (12) for high volatility periods. The negative and significant value of β2 implies herd behaviour for extreme up and down-market returns. Negative and significant β3 implies herding during high volatility. *, **, *** indicates significance at 1%, 5% and 10% level respectively and the value in parenthesis is the t-statistics. |
Τ | 0.1 | 0.25 | 0.5 | 0.75 | 0.9 |
β0 | 0.0108* (16.1144) |
0.0139* (20.5061) |
0.0170* (20.0784) |
0.0221* (22.8469) |
0.0274* (19.6571) |
β1 | 0.1454* (5.5300) |
0.1604* (6.1766) |
0.2192* (6.0090) |
0.2499* (7.1704) |
0.3144* (7.6693) |
β2 | 0.0442 (0.7841) |
−0.0041 (−0.0719) |
−0.1483** (−1.8757) |
−0.2411* (−3.1895) |
−0.4089* (−4.6463) |
Note: This table presents the results of Equation (6) as applied for the sub-period January 01, 2020, till December 31, 2020. Negative and significant value of β2 implies herd behaviour. *, **, *** indicates significance at 1%, 5% and 10% levels respectively and the value in parenthesis is the t-statistics. |