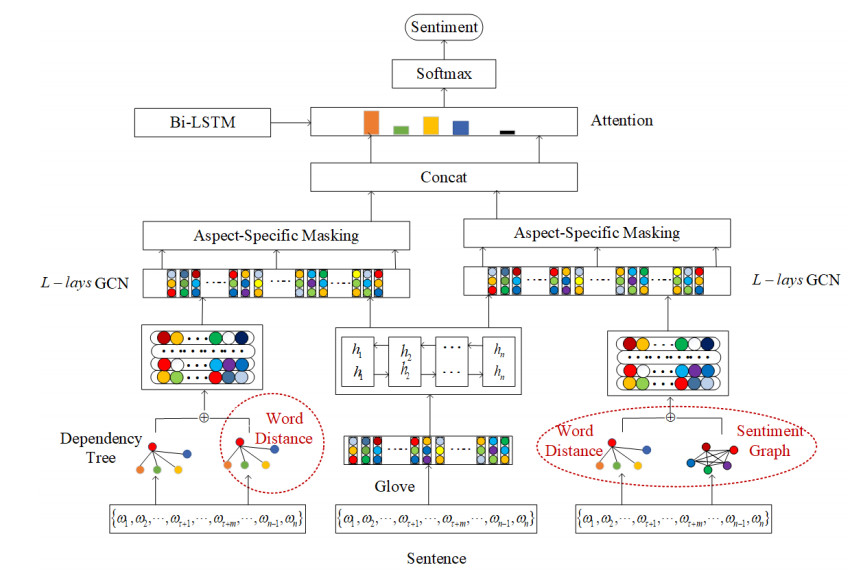
Citation: Lennart Ante. Bitcoin transactions, information asymmetry and trading volume[J]. Quantitative Finance and Economics, 2020, 4(3): 365-381. doi: 10.3934/QFE.2020017
[1] | Jinbao Song, Xiaoya Zhu . Research on public opinion guidance of converging media based on AHP and transmission dynamics. Mathematical Biosciences and Engineering, 2021, 18(5): 6857-6886. doi: 10.3934/mbe.2021341 |
[2] | Elodie Yedomonhan, Chénangnon Frédéric Tovissodé, Romain Glèlè Kakaï . Modeling the effects of Prophylactic behaviors on the spread of SARS-CoV-2 in West Africa. Mathematical Biosciences and Engineering, 2023, 20(7): 12955-12989. doi: 10.3934/mbe.2023578 |
[3] | Fulian Yin, Xueying Shao, Jianhong Wu . Nearcasting forwarding behaviors and information propagation in Chinese Sina-Microblog. Mathematical Biosciences and Engineering, 2019, 16(5): 5380-5394. doi: 10.3934/mbe.2019268 |
[4] | Xiaonan Chen, Suxia Zhang . An SEIR model for information propagation with a hot search effect in complex networks. Mathematical Biosciences and Engineering, 2023, 20(1): 1251-1273. doi: 10.3934/mbe.2023057 |
[5] | Fulian Yin, Jiahui Lv, Xiaojian Zhang, Xinyu Xia, Jianhong Wu . COVID-19 information propagation dynamics in the Chinese Sina-microblog. Mathematical Biosciences and Engineering, 2020, 17(3): 2676-2692. doi: 10.3934/mbe.2020146 |
[6] | Bochuan Du, Pu Tian . Factorization in molecular modeling and belief propagation algorithms. Mathematical Biosciences and Engineering, 2023, 20(12): 21147-21162. doi: 10.3934/mbe.2023935 |
[7] | Qiao Xiang, Tianhong Huang, Qin Zhang, Yufeng Li, Amr Tolba, Isack Bulugu . A novel sentiment analysis method based on multi-scale deep learning. Mathematical Biosciences and Engineering, 2023, 20(5): 8766-8781. doi: 10.3934/mbe.2023385 |
[8] | Wei Hong, Yiting Gu, Linhai Wu, Xujin Pu . Impact of online public opinion regarding the Japanese nuclear wastewater incident on stock market based on the SOR model. Mathematical Biosciences and Engineering, 2023, 20(5): 9305-9326. doi: 10.3934/mbe.2023408 |
[9] | Wenjie Yang, Qianqian Zheng, Jianwei Shen, Linan Guan . Bifurcation and pattern dynamics in the nutrient-plankton network. Mathematical Biosciences and Engineering, 2023, 20(12): 21337-21358. doi: 10.3934/mbe.2023944 |
[10] | Yunyuan Gao, Zhen Cao, Jia Liu, Jianhai Zhang . A novel dynamic brain network in arousal for brain states and emotion analysis. Mathematical Biosciences and Engineering, 2021, 18(6): 7440-7463. doi: 10.3934/mbe.2021368 |
As a significant branch in the field of natural language processing, sentiment analysis aims to extract the sentiment polarity from an input text. Unlike the chapter-level or sentence-level sentiment analysis, the aspect-level sentiment analysis can identify the fine-grain polarity of different aspects in a sentence [1,2,3]. For example, in the sentence: "While the lesson content is substantial, the teacher's speaking pace is excessively rapid!", the sentiment classification of "content" is positive and that of "speaking" is negative.
With the development of deep learning methods, the aspect-level sentiment analysis has achieved remarkable performance in the past few years. Wang et al. [4] proposed a long short-term memory (LSTM) network based on an attention mechanism to extract more information from different parts of a sentence. Ma et al. [5] proposed an interactive attention network to learn the attention weights of context and aspect words for good representations of target and context, respectively. However, the attention mechanism is not sufficient to capture the syntactic dependency between aspect words and context words. In order to solve this problem, a GCN based on dependency trees was proposed by Sun et al. [6]. Since, a variety of GCN variants are designed such as graph attention networks [7,8], multi-channel GCN [9], heterogenous GCNs [10] and dual GCNs [11,12,13,14,15] to capture more syntactic information and sematic information for accurate classification.
However, the existed works of advanced GCNs pay more attention on the sentiment knowledge information of individual words in a comment sentence and ignore the distance information between context words and aspect words. It is proven that the word distance is important for aspect-level sentiment analysis [16,17]. Therefore, it is significant to integrate word distance information into a graph network effectively facilitates the model in extracting dependency relationships between contextual words and specific aspects. Therefore, we first construct a conventional dependency graph for each sentence based on a dependency tree to capture the sentence's syntactic information. Then, through combining sentiment dependency relationships between contextual words and aspect words with distance relationships between words, all this information is fused into the dependency graph. Based on the syntactic dependency relationships and sentiment information of the sentence, a sentiment dependency graph for specific aspects is built. Moreover, considering the syntactic dependency relationships and distance information of the sentence, a distance-enhanced dependency graph for specific aspects is also constructed. Finally, the distance-enhanced and sentiment dependency graphs are input into a dual GCN model to obtain the graph representation of the comment sentence.
Therefore, we propose a word distance assisted dual graph convolutional network (DA2GCN) to characterize both sematic information and syntactic information well. The main contributions are as follows:
● We design a heterogenous dual-GCN to make good use of the word distance for characterizing the correlation of aspect words and context words. The word distance of a sentence is represented by a constructed matrix to establish the distance relationship between them. Then, the word distance information assists the dependency tree and sentiment graph to capture more information and are fed into two GCNs for further sentiment classification.
● We conduct a lot of experiments to verify the advances of our proposed DA2GCN on two self-collected Chinese dataset and five open-source English datasets. The comprehensive results and ablation study demonstrate that the proposed method DA2GCN can achieve higher accuracy and F1 with 1.69–1.81x training speedup over the latest dual-GCN work.
● We make the self-built Chinese datasets of MOOC and Douban, as well as the source code of DA2GCN publicly available on https://github.com/TJSL0715/DA2GCN under open-source licensing.
In this section, we introduce the typical deep learning methods and graph convolutional network assisted methods for aspect-level sentiment analysis.
Most aspect-level sentiment analysis depends on extracting the sentiment information of sentences from the context for identifying the sentiment polarity of a specific aspect. Tang et al. [18] proposed a target-based long short-term memory network to predict the sentiment polarity by modeling the relationship between aspect words and context, and selecting the most relevant parts in the context. Huang et al. [19] proposed an attention-over-attention neural network to learn the representation of aspect words and sentences through bidirectional LSTM (Bi-LSTM), and automatically focuses on important parts of the sentence using the attention mechanism. Zhao et al. [20] proposed a knowledge-enabled language representation model BERT, which can inject the domain-specific emotional knowledge into language representation for aspect-based sentiment analysis. Xiao et al. [21] proposed an enhanced aspect-level sentiment analysis method based on both BERT and multi-attention. Through the interactive attention mechanism of text and aspect words, it captures the correlation between aspect words and the entire text sentence, thereby improving the accuracy of ABSA. An et al. [10] proposed a heterogeneous aspect graph neural network to learn structural and semantic knowledge from inter-sentence relationships to improve the sentiment classification performance. Ma et al. [22] proposed an aspect-context dense connection model to merge the deep semantic information from different aspects and contexts. Yan et al. [23] proposed a sentiment knowledge-based bidirectional encoder representation from transformers. This model utilizes the BERT pre-trained model to encode emotional knowledge vocabulary and contextual words separately, which are subsequently employed for sentiment classification. Tian et al. [24] proposed an attention-based multi-level feature aggregation network, which considers both local and global information by applying attention to convolutional filters. This model uses a multi-level self-attention module to effectively learn the feature information between aspect words and context. However, these typical models cannot consider the words dependency well from a long-distance perspective well so that the sentiment classification is limited.
To address the long-distance correlation issue, GCNs are increasingly applied into the field of aspect-level sentiment analysis. GCN [25] can obtain the information of adjacent nodes, so that it can better capture the local information and global structure of an input text by constructing a graph of words dependency. Zhang et al. [26] extracted the grammatical information of the sentence by constructing a syntactic dependency tree generated by the corresponding grammatical information adjacency matrix and used GCN to learn the obtained grammatical information for excellent classification results. Wang et al. [27] proposed a multi-oriented heterogeneous graph convolution network, which can aggregate multi-faceted information of sentences into a graph, and uses a GCN to update and represent nodes jointly. However, using a single graph to characterize the multiple kinds of information is limited. Wu et al. [28] proposed a phrase dependency relational graph attention network, which aggregates directed dependency edges and phrase information. Phan et al. [29] proposed an aspect-level sentiment analysis of CNN Over BERT-GCN model. It uses BERT word embedding, Bi-LSTM to extract contextual features, GCN to extract grammatical information, and a CNN model is adopted on the feature vector to classify aspect-level emotions. Huang et al. [9] uses the multiple channels in subgraph structure in a novel scalable GCN for higher accuracy.
Dual graphs are proposed for a more effective aspect-level sentiment analysis. Among them, the combinations of dual GCN models based on syntax and semantics have achieved excellent results in emotion classification. Zhu et al. [11] proposed a global dependency and local dependency mixed GCN, which can make good use of both syntactic dependency structure and contextual information to mine the local structure information of sentences, and constructs a word-document graph through the entire corpus to reveal global dependency information between words. Zhu et al. [12] developed a text sequence graph and refined a dependency graph to uncover valuable structural insights. Two graph convolutional networks are used to effectively extract and enhance the understanding of this structural information. Wei et al. [13] proposed a new method GP-GCN, which aimed at to reduce noise by constructing a simplified global feature structure of text, and use the local structure and global features obtained by orthogonal feature projection for the final aspect-level sentiment classification. Jin et al. [30] proposed a knowledge-enhanced dual-channel graph neural network. The model integrates external emotional knowledge into both semantic and syntactic channels, and then utilizes a dynamic attention mechanism to fuse the diverse information from these channels. Wu et al. [14] fused two parallel graph convolutional networks to simultaneously learn different relationship features between sentences, and added a gate mechanism to GCN to filter the related noise during aggregating information. Although this method considers both the grammatical information and the sentiment information of the word, it does not consider the distance information between the aspect word and other words.
Inspired by these works, we also adopt a dual-GCN framework to capture more heterogeneous feature information. More importantly, unlike the existed work, our proposed DA2GCN exploits the word distance information to rebuild the grammatical dependency tree and syntactic sentiment knowledge and feed them into two GCNs for accurate and fast aspect-level sentiment analysis.
In this section, we detail the proposed method DA2GCN for accurate and fast aspect-level sentiment analysis.
The proposed DA2GCN consists of five parts: (1) the word embedding layer and Bi-LSTM layer, (2) dual graph convolution layers, (3) graph convolution fusion layer with aspect masking, (4) interactive attention layer, and (5) output layer as illustrated in Figure 1. Given a sentence S containing n words, S={ω1,ω2,⋯,ωτ+1,⋯,ωτ+m,⋯,ωn−1,ωn}, where {ωτ+1,⋯,ωτ+m} represents the aspect word or aspect phrase in the sentence. The input sentence proceeds to the five parts orderly and the sentiment classification result can be output finally.
This paper utilizes a 300-dimensional pre-trained word vector to transform the input sentence S into a word embedding matrix V∈R|n|×de, where de represents the dimensionality of the word embeddings. As the experiments in this paper involve datasets in both Chinese and English, we have utilized pre-trained word vectors from Chinese Wikipedia [31] and GloVe [32]. Subsequently, the word embedding matrix V is fed into a Bi-LSTM, resulting in the hidden layer state vector H={h1,h2,⋯,hτ+1,⋯,hτ+m,⋯,hn} for the sentence. Here, hi∈R2dh denotes the hidden state vector for the i word, and dh represents the output dimension of the unidirectional LSTM.
Typically, the aspect words and the sentiment words in a sentence are relatively close to each other. The distance between the sentiment words and the aspect words probably contains some important information. Therefore, we incorporate the distance between the aspect words and other words in a sentence into a sentiment classification model to exploring the key information. The distance between the aspect words and other words is shown in Figure 2. For example, "Substantial" is closer to "content" than "speaking", while its distance information is 2 and 4 for the content aspect and the speaking aspect, respectively.
Given a sentence S containing n words, S={ω1,ω2,⋯,ωτ+1,⋯,ωτ+m,⋯,ωn−1,ωn}, where {ωτ+1,⋯,ωτ+m} represents the aspect word or aspect phrase in the sentence. According to the distance between each word and a specific aspect word, an n×n diagonal matrix is constructed, where Dn=diag(y1,y2,⋯,yn) represents the distance between each word and the aspect word. Due to the excessive length of certain sentences leading to a significant disparity in distances between the aspect words and the other words, and considering that words closer to the aspect words carry greater weight in terms of information, the diagonal matrix can be modified by Eq (1):
D=diag(1−yi2∗ymax) | (1) |
Among them, 1≤i≤n, ymax refers to the maximum value from y1 to yn.
In order to utilize the sentiment information between the words in a given sentence, we use different polices for Chinese datasets and English datasets. As for a Chinese dataset, we use the Chinese sentiment word extreme value table from the Department of Chinese Language and Literature of Tsinghua University to build a sentiment knowledge module for Chinese dataset. English datasets are handled by the sentiment dictionary SenticNet [33] to build a sentiment knowledge module. When the word is positive, the extreme sentiment value is > 0; when the word is negative, the extreme sentiment value is < 0; when the word is neutral, the extreme sentiment value is = 0.
For any two words, ωi and ωj in the sentence S, their corresponding weight is calculated by Eq (2):
Sij=|TsNet(ωi)|+|TsNet(ωj)| | (2) |
Among them, TsNet(ωi)represents the weight of the word ωi in the sentiment dictionary. When the word ωi is not present in the sentiment polarity lexicon, TsNet(ωi)=0. Consequently, the sentiment information matrix E∈Rn×n for the sentence can be obtained.
In addition, the word distance matrix is combined with the sentiment knowledge matrix to rebuild a new sentiment knowledge matrix in Eq (3).
Ds=D+E | (3) |
A GCN is further used to extract the distance and the sentiment features, the adjacency matrix Ds of the new distanced assisted sentiment knowledge, along with the context representations H, which is generated by Bi-LSTM. The update procedure for each node in the grammatical GCN is as follows:
hli=∑nj=1 DsWlgl−1j | (4) |
hli=ReLU(hli/(di+1)+bl) | (5) |
gli=f(hli) | (6) |
where gl−1j∈R2dh is the hidden representation of the j-th node convolved in the l−1 layer graph, hli∈R2dh is the hidden representation of the i-th node convolved in the l-th layer graph, di=∑Ds is the degree of the node, Wl and bl represent the weight matrix and bias term of the l-th layer graph convolutional network, respectively, and f(·) is a position-aware transformation function.
This grammatical graph convolutional network, which is augmented with sentiment knowledge through layer-wise distances over L layers, can ultimately provide the following representation in Eq (7):
hLs={hL1,hL2,⋯,hLn} | (7) |
To leverage the word dependency relationships within a sentence, we employ the SpaCy [34] toolkit to construct a sentence dependency tree. Considering the positions of words in a dependency tree, an adjacency matrix G∈Rn×n of the sentence dependency tree can be derived, where n represents the number of words in the sentence. When Gij=1, it signifies a connection between the word i and the word j in the dependency tree. When Gij=0, it indicates the absence of a relationship between the word i and the word j in the dependency tree. Using the self-loop concept [25] to retain more information of word nodes, a self-loop operation is added to all the word nodes in the dependency tree, when i=j, Gij=1.
To take advantage of the relationship between different words in the dependency tree, this paper integrates the word distance information into a sentence's dependency tree. A new distance dependency tree can be constructed by considering both the dependency relationships between sentiment words and aspect words as well as the distance information between them. This approach takes into account both the syntactic dependencies and the spatial relationships between sentiment words and aspect words in Eq (8).
Dg=D+G | (8) |
Similar to the above grammatical GCN, a syntactic GCN is used to extract the distance information and the sentiment features, the adjacency matrix Dgof the distance-enhanced dependency tree, along with the context representations H, which is generated by Bi-LSTM. Each node is updated in the graph convolutional network as follows:
hli=∑nj=1 DgWlgl−1j | (9) |
hli=ReLU(hli/(di+1)+bl) | (10) |
gli=f(hli) | (11) |
where gl−1j∈R2dh is the hidden representation of the j-th node convolved in the l−1 layer graph, hli∈R2dh is the hidden representation of the i-th node convolved in the l-th layer graph, di=∑Dg is the degree of the node, Wl and bl represent the weight matrix and bias term of the l-th layer graph convolutional network, respectively, and f(·) is a position-aware transformation function.
This syntactic GCN is enhanced by layer-wise distance for the dependency tree over L layers, and gives the following information in Eq (12):
hLg={hL1,hL2,⋯,hLn} | (12) |
After the input sentence is processed by the two GCNs, we concatenate the outputs of two distinct graph convolutional networks to obtain a more comprehensive feature representation, denoted as hsg.
hsg=[hs;hg] | (13) |
To emphasize the feature information of the aspect words in a sentence, this proposed method DA2GCN masks the hidden state vectors of non-aspect words while keeping the state of aspect words unchanged in Eq (14):
hLt=0 | (14) |
where the condition should be satisfied 1≤t<τ+1,τ+m<t≤n
Then, the output of the aspect masking layer is obtained in Eq (15):
HLmask={0,⋯,hLτ+1,⋯,hLτ+m,⋯,0} | (15) |
The idea of the interactive attention layer is to learn the weights for interactions between different elements in the input, which enables a more effective capture of the interdependence among elements. Thus, in the interactive attention layer, our proposed model learns the correlations between aspect words and other words, which facilitates a more comprehensive understanding of the relationships and patterns in the input data.
The calculation of interactive attention weights is as follows:
βt=∑nt=1 hthLt=∑τ+mt=τ+1 hthLt | (16) |
αt=exp(βt)∑ni=1 exp(βi) | (17) |
r=∑nt=1 αtht | (18) |
where, ht refers to the output of the Bi-LSTM, hLt corresponds to the output of the aspect masking layer.
The output γ of the interactive attention layer is the input into a fully connected layer, so that the classification output is obtained through a softmax normalization layer.
p=softmax(Wpr+bp) | (19) |
where, Wpis the weight matrix, and bp is the bias term.
This paper uses the cross-entropy function and L2 regularization as loss function:
Loss=−∑Ci=1 yilog2pi+λ∥θ∥2 | (20) |
Here, C represents the number of sentence classifications, yi represents the real sentiment category of the sentence, pi represents the predicted sentence sentiment category, λ represents the weight of the L2 regularization term, and θ represents all trainable parameters.
In this section, we detailed the experimental configuration and results analysis to verify the advances of our proposed method DA2GCN.
In order to verify the effectiveness of the proposed DA2GCN in Chinese sentiment analysis, we collect an extensive range of review data from the Chinese University MOOC and Douban Book Review web pages, preprocess and annotate them. The joint dataset of MOOC and Douban is also used to evaluate the generalization of DA2GCN. Each dataset has three sentiment categories: Positive, negative, and neutral. Finally, the Chinese datasets are divided for training and test as Table 1 shows.
Dataset | Positive | Negative | Neutral | |||
Train | Test | Train | Test | Train | Test | |
MOOC | 2645 | 1065 | 580 | 283 | 275 | 152 |
Douban | 747 | 334 | 287 | 142 | 566 | 198 |
Joint dataset | 3392 | 1399 | 967 | 425 | 841 | 350 |
Moreover, we conduct the experiments on five public English datasets: one (Twitter) is originally built by Dong et al. [35] containing twitter posts, restaurants (Rest14), and laptops (Lap14) domain of SemEval 2014 task 4 [36], and restaurants (Rest15, Rest16) domain of SemEval 2015 task 12 [37] and SemEval 2016 task 5 [38]. The datasets configuration is detailed in Table 2.
Dataset | Positive | Negative | Neutral | |||
Train | Test | Train | Test | Train | Test | |
1561 | 173 | 1560 | 173 | 3127 | 346 | |
Lap14 | 994 | 341 | 870 | 128 | 464 | 169 |
Rest14 | 2164 | 728 | 807 | 196 | 637 | 196 |
Rest15 | 912 | 326 | 256 | 182 | 36 | 34 |
Rest16 | 1240 | 469 | 439 | 117 | 69 | 30 |
The typical classification criteria Accuracy and F1 score are used to measure the sentiment analysis results in this paper. The accuracy and F1 are defined as follows:
Accuracy=TP+TNTP+FP+TN+FN | (21) |
F1=2PRP+R | (22) |
TP represents the number of successfully predicted positive samples, FP represents the number of incorrectly predicted negative samples, TN represents the number of successfully predicted negative samples, and FN represents the number of incorrectly predicted positive samples. Accuracy represents the proportion of correctly classified samples among the total samples. P refers to the proportion of samples classified as positive among the true positive samples. R represents the proportion of correctly classified samples among the total samples. The F1 score is the weighted harmonic mean of precision and recall.
As for non-BERT based models, we use the Chinese Wikipedia 300-dimensional pre-trained word vector the Chinese datasets as the initial word embedding, and the GloVe vector to map each word to 300 dimensions for the English datasets in Table 3. The coefficient λ of L2 regularization item is 0.00001. The dimension of the hidden state vector is set to 300. The model parameters are optimized and updated using Adam with a learning rate of 0.001. If BERT is used for the word embedding to assist the aspect-level sentiment analysis, the word embedding dimension is configured to 768 for the pre-trained uncased BERT-base model. The corresponding learning rate is set to 0.00002.
Parameter | Value |
Embed_dim | 300/768 |
Batch_size | 32 |
Num_Epoch | 100 |
Learning_rate | 0.001/0.00002 |
GCN_layers | 2 |
Optimizer | adam |
l2reg | 0.00001 |
To evaluate the effectiveness of our proposed method, we compare it with a series of the state-of-the-art (SOTA)methods. Due to the limited number of studies on Chinese sentiment analysis, we select the following five SOTA methods for comparison:
● TD-LSTM [18]: A bidirectional LSTM model is proposed to automatically extract target information and perform sentiment analysis based on the correlation between aspect words and contextual words.
● ASCNN [26]: Bi-LSTM is combined with an undirected dependency tree for the sentence and CNN is used to extract information from syntactic dependency relationships.
● ASTCN [26]: GCN takes the place of CNN in the above ASCNN to extract syntactic information for a more accurate sentiment analysis.
● ASGCN [26]: Unlike ASTCN, GCN further exploits contextual features and syntactic information between words, which is combined with attention mechanisms for sentiment analysis.
● DSSGCN [15]: A dual-channel semantic learning graph convolutional network is proposed to extract semantic information obtained through cosine similarity and structural information acquired through co-occurrence words for sentiment analysis.
In order to further demonstrate the effectiveness of the proposed DA2GCN model, the following six GCN based SOTA methods are used on five English datasets:
● GL-GCN [11]: A local graph based on the syntactic information and sentence order, and a word-document global graph are used to construct two GCNs for the aspect-level sentiment analysis.
● SEDC-GCN [12]: The two graphs for text sequence enhanced dependency are constructed to characterize the more structural information, while a dual-channel graph encoder is designed to model them jointly.
● GP-GCN [13]: A dual-graph convolutional network is proposed using the global feature structure of the text, and the local dependency structure of the sentence for the aspect-level sentiment analysis.
● PFGGCN [14]: It integrates two parallel GCNs to learn the distinct relational features between sentences, and a gating mechanism to filter out the noise.
● TD-BERT [39]: A BERT model is used for assisting the aspect-level sentiment analysis.
● SK-GCN [40]: A new graph convolutional network model based on grammar and knowledge to make good use of both syntactic dependency trees and common-sense knowledge through dual GCNs for the aspect-level sentiment classification.
Chinese datasets results and analysis. It is observed that the proposed DA2GCN in this paper can achieve the best accuracy 78.54% and F1 67.21%, and outperforms the SOTA methods on the joint dataset in Figure 3. This primarily results from the unique characteristics of word distance consideration and dual GCNs in DA2GCN. It is interesting that DA2GCN achieves the highest accuracy, 1.41% and 1.11% higher accuracy, but a slightly lower F1 than DSSGCN for the two Chinese datasets of MOOC and Douban, respectively. Because the imbalance of the training datasets of three sentiment categories (positive, negative, and neutral) probably causes a higher accuracy and a bit lower F1 in DA2GCN. On the MOOC dataset, the DA2GCN performs a 2.04% higher accuracy and higher 2.38% F1 than ASGCN. Since the Douban data set mostly contains long and difficult sentences, the accuracy and f1 value of the model are both lower than the MOOC dataset. In comparison to the ASGCN model, our model exhibits an improvement of 2.22% in accuracy and 1.73% in F1 value. It can be shown that considering both sentiment knowledge information and distance information between words in our proposed DA2GCN is beneficial to improve the performance aspect-level sentiment analysis.
English dataset results and analysis. When GloVe is used for word embeddings on the English dataset, our proposed DA2GCN perform better than TD-LSTM, ASGCN, GL-GCN, and GP-GCN, but a bit worse than SEDC-GCN, FPGGCN, and DSSGCN as shown in Table 4. The primary reason is that the significant differences in the tokenization format between Chinese and English sentences in GloVe, while our proposed method is mainly customized for Chinese datasets. More importantly, when the popular BERT is used to enhance the word embeddings, our proposed model can achieve outstanding classification results on the Rest14, Rest15, and Rest16 datasets over the SOTA methods of SK-GCN, TD-BERT and GP-GCN. In a summary, our proposed model not only effectively enhances the sentiment classification performance on English datasets but also achieves the superior performance on Chinese datasets.
Embedding | Models | Twitter(%) | LAP14(%) | REST14(%) | REST15(%) | REST16(%) | |||||
Acc. | F1. | Acc. | F1. | Acc. | F1. | Acc. | F1. | Acc. | F1. | ||
Glove | TD-LSTM | 68.64 | 66.60 | 68.88 | 63.93 | 78.60 | 67.02 | 78.48 | 62.84 | 83.77 | 61.71 |
ASGCN | 72.15 | 70.40 | 75.55 | 71.05 | 80.77 | 72.02 | 79.89 | 61.89 | 88.99 | 67.48 | |
GL-GCN | 73.26 | 71.26 | 76.91 | 72.76 | 82.11 | 73.46 | 80.81 | 64.99 | 88.47 | 69.64 | |
GP-GCN | 71.67 | 69.45 | 73.90 | 68.67 | 80.89 | 70.90 | 79.89 | 61.78 | 83.90 | 64.67 | |
SEDC-GCN | 74.42 | 73.37 | 77.74 | 74.68 | 83.30 | 77.51 | 81.73 | 66.23 | 90.75 | 73.84 | |
PFGGCN | - | - | 78.06 | 74.52 | 83.78 | 76.55 | 82.15 | 66.73 | 90.92 | 75.26 | |
DSSGCN | 75.25 | 73.71 | 78.49 | 74.63 | 84.36 | 77.35 | 82.62 | 66.39 | 91.38 | 75.43 | |
DA2GCN | 72.83 | 71.17 | 76.96 | 73.68 | 83.30 | 76.52 | 80.26 | 64.57 | 88.47 | 70.75 | |
BERT | SK-GCN | 75.00 | 73.01 | 79.00 | 75.57 | 83.48 | 75.19 | 83.20 | 66.78 | 87.19 | 72.02 |
TD-BERT | 76.69 | 74.28 | 78.87 | 74.38 | 85.1 | 78.35 | - | - | - | - | |
GP-GCN | 75.90 | 73.90 | 79.90 | 75.89 | 83.89 | 75.09 | 83.90 | 66.89 | 87.78 | 72.89 | |
DA2GCN | 74.28 | 72.87 | 78.2 | 74.66 | 85.8 | 79.66 | 83.21 | 68.4 | 90.26 | 72.32 |
The model size and training time are also significant for evaluating the training speed. The comparative results of model size, training time and speedup are given in Figure 4(a)–(c), respectively.
The model size is measured by the number of parameters and our method has smaller parameters than the effective dual-GCN method DSSGCN. Therefore, the training speedup of our DA2GCN is up to 1.81x over DSSGCN. It is noted that the complex DSSGCN brings the higher performance on English datasets at the expense of a large model and long training time. Our DA2GCN can achieve the good tradeoff between accuracy and speed.
Compared with the similar-size models ASCNN, ASTCN and ASGCN, the training time of our DA2GCN is significantly reduced because of its fast convergence. In a summary, the proposed DA2GCN can be used for the fast and accurate aspect-level sentiment analysis using fewer parameters and training time.
To further examine the impacts of each component of DA2GCN on the sentiment classification performance, we conduct the ablation study as follows:
● R-DED: When the distance-enhanced dependency tree module is removed, and the model only considers the feature information of distance-enhanced sentiment knowledge. It employs a single graph convolutional network to extract features of distance-enhanced sentiment knowledge.
● R-DES: When the distance-enhanced sentiment knowledge module is removed, the model only considers the feature information of the distance-enhanced dependency tree. A single graph convolutional network is employed to extract features of the distance-enhanced dependency tree.
● R-SKM: When the sentiment knowledge information is removed, the model only considers the feature information of the dependency tree and word distance. It uses the dual graph convolutional networks, features of the word distance matrix, and the distance-enhanced dependency tree matrix are extracted separately.
● R-WDM: When the word distance information is removed, the model considers only the feature information of the dependency tree and sentiment knowledge. It uses the dual graph convolutional networks, features of the sentiment knowledge matrix, and the dependency tree adjacency matrix are extracted separately.
As is listed in Figure 5, the ablation study demonstrates that the complete DA2GCN model surpasses any variant lacking a single module in terms of classification performance. This confirms the importance of incorporating word distance and sentiment knowledge information into the model. When the word distance module is removed in R-DED, there is a noticeable decrease in accuracy and F1 score compared to the complete DA2GCN model, which highlights the significance of the word distance information in the accurate aspect-level sentiment analysis. In the case of the Douban dataset, the methods of R-DED and R-DES has little better F1 than our proposed method. Because the comments from Douban are mostly long sentences, the sentiment classification depends less distance information. Therefore, our DA2GCN achieves good performance on MOOC dataset and the joint dataset well.
In addition, we conducted a statistical analysis of the parameter count and training time for the models used in the ablation experiments on the MOOC dataset and Douban dataset, as detailed in Table 5. It is noteworthy that the table indicates an equivalent parameter count for each model. This is attributed to our exclusive modification of the values within the input parameter matrices, without altering the matrices' dimensions. Consequently, the total number of parameters in the models remains constant throughout this process. However, the training time varies among the models. This discrepancy arises because, despite the consistent parameter count, altering the initial values of the weights may influence the training and convergence processes, leading to differences in training times.
Model | MOOC | Douban | ||
Time(s) | Parameters(M) | Time(s) | Parameters(M) | |
R-DED | 123 | 7.2 | 266 | 5.6 |
R-DES | 153 | 7.2 | 244 | 5.6 |
R-SKM | 154 | 7.2 | 339 | 5.6 |
R-WDM | 164 | 7.2 | 414 | 5.6 |
DA2GCN | 146 | 7.2 | 365 | 5.6 |
The number of GCN layers decides how long the neighbor's information can be collected and is significant for GCN. More importantly, we use the effective dual GCNs. Therefore, the number of GCN layers in the two GCNs in our proposed DA2GCN is discussed. The homogeneous and heterogenous GCNs are both considered, where the same number or different values are set to search the optimal configuration.
The experimental results are illustrated in Figure 6, where the x-axis represents the number of layers in the dual graph convolution networks and the y-axis depicts the corresponding accuracy. The first digit signifies the layers for the word distance assisted grammatical GCN, while the second digit represents the layers for the word distance assisted syntactic GCN. It is noted that when the number of GCN layers are both set to 2, the highest accuracy is obtained. If there is only one layer in the two GCNs, the model has the lowest accuracy due to a smaller receptive field, failing to capture sufficient sentence feature information. On the other hand, as the number of layers increases for the two GCNs, the model becomes more complex and also introduces more noise to decrease the accuracy. Furthermore, an excessive number of network layers may lead to the overfitting so that the model's generalization is diminishing. Therefore, selecting an appropriate number of GCN layers is crucial for extracting effective feature information for higher accuracy.
In order to qualitatively demonstrate the improved performance of the proposed DA2GCN model in predicting aspect-based sentiment polarity, we visualize the attention weight through representative examples in Figure 7. It is observed that, in comparison to the R-WDM model (the model without the word distance module), the complete DA2GCN model pays more attention to crucial sentiment words and successfully extracts emotion features corresponding to specific aspects. This indicates that strengthening the word distance relationship based on specific aspects allows for a more accurate characterization of sentiment features, thereby enhancing the performance in the aspect-based sentiment analysis tasks.
The above experiments demonstrate that the proposed DA2GCN make good use of the dual GCN framework to consider the word distance information completely for rebuilding the grammatical knowledge and syntactic dependency tree and achieving higher performance of aspect-level sentiment analysis. More importantly, this approach not only works well on each single dataset from two Chinese datasets and five English datasets, but also has scalability and performance even larger datasets (joint MOOC and Doban up to more than 7000 comments). Furthermore, it is noted that DA2GCN has shorter training time than other dual-GCN methods because of the smaller number of parameters. Therefore, the lightweight DA2GCN can be used in the real-time applications. In summary, our proposed DA2GCN performs good scalability in the real-time applications.
Besides the aspect-level sentiment analysis, DA2GCN inspire other directions of natural language processing (NLP) such as spanning question answering, relation extraction, and machine reading comprehension to exploits diverse information in a dual-GCN framework for high performance text processing. Thus, the proposed method has good generalizability to other NLP applications.
However, it is noted that due to the significant differences in the tokenization formats between Chinese datasets and English datasets, our proposed method uses the Chinese customized tokenization format to extracts the distance information for all the datasets. Therefore, we will focus on the customized representation of English word distance for more accurate and fast aspect-level sentiment analysis in future work.
In this paper, a novel dual-GCN method DA2GCN is proposed to make good use of word distance information for rebuilding the grammatical knowledge and syntactic dependency tree so that the aspect-level sentiment classification can be improved well. The comprehensive results on two self-built Chinese datasets and five open-source English datasets demonstrate our DA2GCN can achieve higher accuracy and F1 over the SOTA methods. Moreover, DA2GCN has fewer parameters and consumes less training time than the latest dual-GCN methods. It is noted that due to the significant differences in the tokenization formats between Chinese datasets and English datasets, our proposed method uses the Chinese customized tokenization format to extracts the distance information for all the datasets. Consequently, DA2GCN can represent the distance information for Chinese words more effectively and performs better than English. Therefore, we will focus on the customized representation of English word distance for more accurate and fast aspect-level sentiment analysis in future work.
To further improve the performance of aspect-level sentiment classification, multimodal (text, video and audio) is increasingly attractive, unlike the pure textual data, the correlations between multimodal data are also more intricate. The complex hypergraph neural networks [41] can comprehensively depict complex higher-order data correlations using hyperedge convolution operations. In the future work, we will develop more effective hypergraph neural networks to characterize more heterogeneous information from multimodal and achieve higher performance of sentiment classification.
The authors declare that they have not used Artificial Intelligence (AI) tools in the creation of this article.
This research was funded by Shanghai Pujiang Program (Grant NO.21PJD026) and the Shanghai Association of Higher Education.
The authors declare that there is no conflict of interest.
[1] |
Aalborg HA, Molnár P, de Vries JE (2019) What can explain the price, volatility and trading volume of Bitcoin? Financ Res Lett 29: 255-265. doi: 10.1016/j.frl.2018.08.010
![]() |
[2] |
Admati AR, Pfeiderer P (1988) A Theory of Intraday Patterns: Volume and Price Variability. Rev Financ Stud 1: 3-40. doi: 10.1093/rfs/1.1.3
![]() |
[3] |
Ajinkya BB, Jain PC (1989) The behavior of daily stock market trading volume. J Account Econ 11: 331-359. doi: 10.1016/0165-4101(89)90018-9
![]() |
[4] | Alameda Research (2019) Investigation into the Legitimacy of Reported Cryptocurrency Exchange Volume. Available from: https://ftx.com/volume_report_paper.pdf |
[5] | Ante L (2020) A place next to Satoshi: scientific foundations of blockchain and cryptocurrency in business and economics. Scientometrics Available from: https://doi.org/10.1007/s11192-020-03492-8 |
[6] | Ante L, Fiedler I (2020) Market Reaction to Large Transfers on the Bitcoin Blockchain-Do Size and Motive Matter? Financ Res Lett. Available from: https://doi.org/10.1016/j.frl.2020.101619. |
[7] |
Bariviera AF (2017) The inefficiency of Bitcoin revisited: A dynamic approach. Econ Lett 161: 1-4. doi: 10.1016/j.econlet.2017.09.013
![]() |
[8] |
Baur DG, Cahill D, Godfrey K, et al. (2019) Bitcoin time-of-day, day-of-week and month-of-year effects in returns and trading volume. Financ Res Lett 31: 78-92. doi: 10.1016/j.frl.2019.04.023
![]() |
[9] |
Beaver WH (1968) The Information Content of Annual Earnings Announcements. J Account Res 6: 67-92. doi: 10.2307/2490070
![]() |
[10] | Bitfinex (2020) Bitfinex Security Features. Available from: https://support.bitfinex.com/hc/en-us/articles/213892469-Bitfinex-Security-Features. |
[11] |
Black F (1986) Noise. J Financ 41: 528-543. doi: 10.1111/j.1540-6261.1986.tb04513.x
![]() |
[12] |
Brown SJ, Warner JB (1985) Using daily stock returns. The case of event studies. J Financ Econ 14: 3-31. doi: 10.1016/0304-405X(85)90042-X
![]() |
[13] |
Campbell CJ, Wasley CE (1996) Measuring abnormal daily trading volume for samples of NYSE/ASE and NASDAQ securities using parametric and nonparametric test statistics. Rev Quant Financ Account 6: 309-326. doi: 10.1007/BF00245187
![]() |
[14] |
Caporale GM, Plastun A (2019) The day of the week effect in the cryptocurrency market. Financ Res Lett 31: 258-269. doi: 10.1016/j.frl.2018.11.012
![]() |
[15] |
Chae J (2005) Trading volume, information asymmetry, and timing information. J Financ 60: 413-442. doi: 10.1111/j.1540-6261.2005.00734.x
![]() |
[16] |
Ciaian P, Rajcaniova M, Kancs Artis (2016) The economics of BitCoin price formation. Appl Econ 48: 1799-1815. doi: 10.1080/00036846.2015.1109038
![]() |
[17] |
Cready WM, Ramanan R (1991) The power of tests employing log-transformed volume in detecting abnormal trading. J Account Econ 14: 203-214. doi: 10.1016/0165-4101(91)90005-9
![]() |
[18] | Decker C, Wattenhofer R (2016) Information propagation in the Bitcoin network, in: 13-Th IEEE International Conference on Peer-to-Peer Computing, 1-10. |
[19] |
Dorfleitner G, Lung C (2018) Cryptocurrencies from the perspective of euro investors: a re-examination of diversification benefits and a new day-of-the-week effect. J Asset Manage 19: 472-494. doi: 10.1057/s41260-018-0093-8
![]() |
[20] |
Easley D, Hvidkjaer S, O'Hara M (2002) Is information risk a determinant of asset returns? J Financ 57: 2185-2221. doi: 10.1111/1540-6261.00493
![]() |
[21] |
Fama EF (1970) Efficient Capital Markets: A Review of Theory and Empirical Work. J Financ 25: 383-417. doi: 10.2307/2325486
![]() |
[22] | Foster G, Olsen C, Shevlin T (1984) Earnings Releases, Anomalies, and the Behavior of Security Returns. Account Rev 59: 574-603. |
[23] | Fusaro T, Hougan M (2019) Bitwise Asset Management - Presentation to the U.S. Securities and Exchange Commission. Available from: https://www.sec.gov/comments/sr-nysearca-2019-01/srnysearca201901-5164833-183434.pdf. |
[24] | Gervais A, Ritzdorf H, Karame GO, et al. (2015) Tampering with the delivery of blocks and transactions in Bitcoin, in: Proceedings of the ACM Conference on Computer and Communications Security, 692-705. |
[25] |
Harris L (1986) Cross-Security Tests of the Mixture of Distributions. J Financ Quant Anal 21: 39-46. doi: 10.2307/2330989
![]() |
[26] |
Jain PC, Joh GH (1988) The Dependence between Hourly Prices and Trading Volume. J Financ Quant Anal 23: 269-283. doi: 10.2307/2331067
![]() |
[27] |
James C, Edmister RO (1983) The Relation Between Common Stock Returns Trading Activity and Market Value. J Financ 38: 1075-1086. doi: 10.1111/j.1540-6261.1983.tb02283.x
![]() |
[28] |
Kaiser L (2019) Seasonality in cryptocurrencies. Financ Res Lett 31: 232-238. doi: 10.1016/j.frl.2018.11.007
![]() |
[29] |
Karalevicius V (2018) Using sentiment analysis to predict interday Bitcoin price movements. J Risk Financ 19: 56-75. doi: 10.1108/JRF-06-2017-0092
![]() |
[30] |
Karpoff JM (1986) A Theory of Trading Volume. J Financ 41: 1069-1087. doi: 10.1111/j.1540-6261.1986.tb02531.x
![]() |
[31] |
Koutmos D (2018) Bitcoin returns and transaction activity. Econ Lett 167: 81-85. doi: 10.1016/j.econlet.2018.03.021
![]() |
[32] |
Kristoufek L (2018) On Bitcoin markets (in)efficiency and its evolution. Phys A Stat Mech Appl 503: 257-262. doi: 10.1016/j.physa.2018.02.161
![]() |
[33] |
Kyle AS (1985) Continuous Auctions and Insider Trading. Econometrica 53: 1315-1335. doi: 10.2307/1913210
![]() |
[34] |
Lakonishok J, Vermaelen T (1986) Tax-induced trading around ex-dividend days. J Financ Econ 16: 287-319. doi: 10.1016/0304-405X(86)90032-2
![]() |
[35] |
Li Z, Dong H, Huang Z, et al. (2018) Asymmetric Effects on Risks of Virtual Financial Assets (VFAs) in different regimes: A Case of Bitcoin. Quant Financ Econ 2: 860-883. doi: 10.3934/QFE.2018.4.860
![]() |
[36] |
Milgrom P, Stokey N (1982) Information, Trade and Common Knowledge. J Econ Theory 26: 17-27. doi: 10.1016/0022-0531(82)90046-1
![]() |
[37] |
Nadarajah S, Chu J (2017) On the inefficiency of Bitcoin. Econ Lett 150: 6-9. doi: 10.1016/j.econlet.2016.10.033
![]() |
[38] | Sapuric S, Kokkinaki A, Georgiou I (2020) The relationship between Bitcoin returns, volatility and volume: asymmetric GARCH modeling. J Enterp Inf Manage. |
[39] |
Vidal-Tomás D, Ibañez A (2018) Semi-strong efficiency of Bitcoin. Financ Res Lett 27: 259-265. doi: 10.1016/j.frl.2018.03.013
![]() |
[40] |
Wang JN, Liu HC, Hsu YT (2019) Time-of-day periodicities of trading volume and volatility in Bitcoin exchange: Does the stock market matter? Financ Res Lett, 1-8. doi: 10.1016/j.frl.2019.04.031
![]() |
[41] |
Wilcoxon F (1945) Individual Comparisons by Ranking Methods. Biometrics Bull 1: 80-83. doi: 10.2307/3001968
![]() |
1. | Benedikt V. Meylahn, Koen De Turck, Michel Mandjes, Trust in society: A stochastic compartmental model, 2025, 668, 03784371, 130563, 10.1016/j.physa.2025.130563 |
Dataset | Positive | Negative | Neutral | |||
Train | Test | Train | Test | Train | Test | |
MOOC | 2645 | 1065 | 580 | 283 | 275 | 152 |
Douban | 747 | 334 | 287 | 142 | 566 | 198 |
Joint dataset | 3392 | 1399 | 967 | 425 | 841 | 350 |
Dataset | Positive | Negative | Neutral | |||
Train | Test | Train | Test | Train | Test | |
1561 | 173 | 1560 | 173 | 3127 | 346 | |
Lap14 | 994 | 341 | 870 | 128 | 464 | 169 |
Rest14 | 2164 | 728 | 807 | 196 | 637 | 196 |
Rest15 | 912 | 326 | 256 | 182 | 36 | 34 |
Rest16 | 1240 | 469 | 439 | 117 | 69 | 30 |
Parameter | Value |
Embed_dim | 300/768 |
Batch_size | 32 |
Num_Epoch | 100 |
Learning_rate | 0.001/0.00002 |
GCN_layers | 2 |
Optimizer | adam |
l2reg | 0.00001 |
Embedding | Models | Twitter(%) | LAP14(%) | REST14(%) | REST15(%) | REST16(%) | |||||
Acc. | F1. | Acc. | F1. | Acc. | F1. | Acc. | F1. | Acc. | F1. | ||
Glove | TD-LSTM | 68.64 | 66.60 | 68.88 | 63.93 | 78.60 | 67.02 | 78.48 | 62.84 | 83.77 | 61.71 |
ASGCN | 72.15 | 70.40 | 75.55 | 71.05 | 80.77 | 72.02 | 79.89 | 61.89 | 88.99 | 67.48 | |
GL-GCN | 73.26 | 71.26 | 76.91 | 72.76 | 82.11 | 73.46 | 80.81 | 64.99 | 88.47 | 69.64 | |
GP-GCN | 71.67 | 69.45 | 73.90 | 68.67 | 80.89 | 70.90 | 79.89 | 61.78 | 83.90 | 64.67 | |
SEDC-GCN | 74.42 | 73.37 | 77.74 | 74.68 | 83.30 | 77.51 | 81.73 | 66.23 | 90.75 | 73.84 | |
PFGGCN | - | - | 78.06 | 74.52 | 83.78 | 76.55 | 82.15 | 66.73 | 90.92 | 75.26 | |
DSSGCN | 75.25 | 73.71 | 78.49 | 74.63 | 84.36 | 77.35 | 82.62 | 66.39 | 91.38 | 75.43 | |
DA2GCN | 72.83 | 71.17 | 76.96 | 73.68 | 83.30 | 76.52 | 80.26 | 64.57 | 88.47 | 70.75 | |
BERT | SK-GCN | 75.00 | 73.01 | 79.00 | 75.57 | 83.48 | 75.19 | 83.20 | 66.78 | 87.19 | 72.02 |
TD-BERT | 76.69 | 74.28 | 78.87 | 74.38 | 85.1 | 78.35 | - | - | - | - | |
GP-GCN | 75.90 | 73.90 | 79.90 | 75.89 | 83.89 | 75.09 | 83.90 | 66.89 | 87.78 | 72.89 | |
DA2GCN | 74.28 | 72.87 | 78.2 | 74.66 | 85.8 | 79.66 | 83.21 | 68.4 | 90.26 | 72.32 |
Model | MOOC | Douban | ||
Time(s) | Parameters(M) | Time(s) | Parameters(M) | |
R-DED | 123 | 7.2 | 266 | 5.6 |
R-DES | 153 | 7.2 | 244 | 5.6 |
R-SKM | 154 | 7.2 | 339 | 5.6 |
R-WDM | 164 | 7.2 | 414 | 5.6 |
DA2GCN | 146 | 7.2 | 365 | 5.6 |
Dataset | Positive | Negative | Neutral | |||
Train | Test | Train | Test | Train | Test | |
MOOC | 2645 | 1065 | 580 | 283 | 275 | 152 |
Douban | 747 | 334 | 287 | 142 | 566 | 198 |
Joint dataset | 3392 | 1399 | 967 | 425 | 841 | 350 |
Dataset | Positive | Negative | Neutral | |||
Train | Test | Train | Test | Train | Test | |
1561 | 173 | 1560 | 173 | 3127 | 346 | |
Lap14 | 994 | 341 | 870 | 128 | 464 | 169 |
Rest14 | 2164 | 728 | 807 | 196 | 637 | 196 |
Rest15 | 912 | 326 | 256 | 182 | 36 | 34 |
Rest16 | 1240 | 469 | 439 | 117 | 69 | 30 |
Parameter | Value |
Embed_dim | 300/768 |
Batch_size | 32 |
Num_Epoch | 100 |
Learning_rate | 0.001/0.00002 |
GCN_layers | 2 |
Optimizer | adam |
l2reg | 0.00001 |
Embedding | Models | Twitter(%) | LAP14(%) | REST14(%) | REST15(%) | REST16(%) | |||||
Acc. | F1. | Acc. | F1. | Acc. | F1. | Acc. | F1. | Acc. | F1. | ||
Glove | TD-LSTM | 68.64 | 66.60 | 68.88 | 63.93 | 78.60 | 67.02 | 78.48 | 62.84 | 83.77 | 61.71 |
ASGCN | 72.15 | 70.40 | 75.55 | 71.05 | 80.77 | 72.02 | 79.89 | 61.89 | 88.99 | 67.48 | |
GL-GCN | 73.26 | 71.26 | 76.91 | 72.76 | 82.11 | 73.46 | 80.81 | 64.99 | 88.47 | 69.64 | |
GP-GCN | 71.67 | 69.45 | 73.90 | 68.67 | 80.89 | 70.90 | 79.89 | 61.78 | 83.90 | 64.67 | |
SEDC-GCN | 74.42 | 73.37 | 77.74 | 74.68 | 83.30 | 77.51 | 81.73 | 66.23 | 90.75 | 73.84 | |
PFGGCN | - | - | 78.06 | 74.52 | 83.78 | 76.55 | 82.15 | 66.73 | 90.92 | 75.26 | |
DSSGCN | 75.25 | 73.71 | 78.49 | 74.63 | 84.36 | 77.35 | 82.62 | 66.39 | 91.38 | 75.43 | |
DA2GCN | 72.83 | 71.17 | 76.96 | 73.68 | 83.30 | 76.52 | 80.26 | 64.57 | 88.47 | 70.75 | |
BERT | SK-GCN | 75.00 | 73.01 | 79.00 | 75.57 | 83.48 | 75.19 | 83.20 | 66.78 | 87.19 | 72.02 |
TD-BERT | 76.69 | 74.28 | 78.87 | 74.38 | 85.1 | 78.35 | - | - | - | - | |
GP-GCN | 75.90 | 73.90 | 79.90 | 75.89 | 83.89 | 75.09 | 83.90 | 66.89 | 87.78 | 72.89 | |
DA2GCN | 74.28 | 72.87 | 78.2 | 74.66 | 85.8 | 79.66 | 83.21 | 68.4 | 90.26 | 72.32 |
Model | MOOC | Douban | ||
Time(s) | Parameters(M) | Time(s) | Parameters(M) | |
R-DED | 123 | 7.2 | 266 | 5.6 |
R-DES | 153 | 7.2 | 244 | 5.6 |
R-SKM | 154 | 7.2 | 339 | 5.6 |
R-WDM | 164 | 7.2 | 414 | 5.6 |
DA2GCN | 146 | 7.2 | 365 | 5.6 |