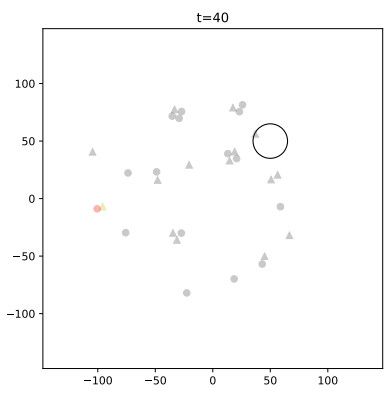
In the context of the theory of multi-agent systems, the shepherding problem refers to designing the dynamics of a herding agent, called a sheepdog, so that a given flock of agents, called sheep, is guided into a goal region. Although several effective methodologies and algorithms have been proposed in the last decade for the shepherding problem under various formulations, little research has been directed to the practically important case in which the flock contains sheep agents unresponsive to the sheepdog agent. To fill in this gap, we propose a sheepdog algorithm for guiding unresponsive sheep in this paper. In the algorithm, the sheepdog iteratively applies an existing shepherding algorithm, the farthest-agent targeting algorithm, while dynamically switching its destination. This procedure achieves the incremental growth of a controllable flock, which finally enables the sheepdog to guide the entire flock into the goal region. Furthermore, we illustrate by numerical simulations that the proposed algorithm can outperform the farthest-agent targeting algorithm.
Citation: Ryoto Himo, Masaki Ogura, Naoki Wakamiya. Iterative shepherding control for agents with heterogeneous responsivity[J]. Mathematical Biosciences and Engineering, 2022, 19(4): 3509-3525. doi: 10.3934/mbe.2022162
[1] | Peng Lu, Rong He, Dianhan Chen . Exploring S-shape curves and heterogeneity effects of rumor spreading in online collective actions. Mathematical Biosciences and Engineering, 2022, 19(3): 2355-2380. doi: 10.3934/mbe.2022109 |
[2] | Jun Zhai, Bilin Xu . Research on meme transmission based on individual heterogeneity. Mathematical Biosciences and Engineering, 2021, 18(5): 5176-5193. doi: 10.3934/mbe.2021263 |
[3] | Giulia Bertaglia, Lorenzo Pareschi, Giuseppe Toscani . Modelling contagious viral dynamics: a kinetic approach based on mutual utility. Mathematical Biosciences and Engineering, 2024, 21(3): 4241-4268. doi: 10.3934/mbe.2024187 |
[4] | Sai Zhang, Li Tang, Yan-Jun Liu . Formation deployment control of multi-agent systems modeled with PDE. Mathematical Biosciences and Engineering, 2022, 19(12): 13541-13559. doi: 10.3934/mbe.2022632 |
[5] | Xiaofeng Chen . Double-integrator consensus for a switching network without dwell time. Mathematical Biosciences and Engineering, 2023, 20(7): 11627-11643. doi: 10.3934/mbe.2023516 |
[6] | Yuhang Yao, Jiaxin Yuan, Tao Chen, Xiaole Yang, Hui Yang . Distributed convex optimization of bipartite containment control for high-order nonlinear uncertain multi-agent systems with state constraints. Mathematical Biosciences and Engineering, 2023, 20(9): 17296-17323. doi: 10.3934/mbe.2023770 |
[7] | Hebing Zhang, Xiaojing Zheng . Invariable distribution of co-evolutionary complex adaptive systems with agent's behavior and local topological configuration. Mathematical Biosciences and Engineering, 2024, 21(2): 3229-3261. doi: 10.3934/mbe.2024143 |
[8] | Jinxin Du, Lei Liu . Adaptive fuzzy fixed time formation control of state constrained nonlinear multi-agent systems against FDI attacks. Mathematical Biosciences and Engineering, 2024, 21(3): 4724-4741. doi: 10.3934/mbe.2024207 |
[9] | Meiqiao Wang, Wuquan Li . Distributed adaptive control for nonlinear multi-agent systems with nonlinear parametric uncertainties. Mathematical Biosciences and Engineering, 2023, 20(7): 12908-12922. doi: 10.3934/mbe.2023576 |
[10] | Ruiping Yuan, Jiangtao Dou, Juntao Li, Wei Wang, Yingfan Jiang . Multi-robot task allocation in e-commerce RMFS based on deep reinforcement learning. Mathematical Biosciences and Engineering, 2023, 20(2): 1903-1918. doi: 10.3934/mbe.2023087 |
In the context of the theory of multi-agent systems, the shepherding problem refers to designing the dynamics of a herding agent, called a sheepdog, so that a given flock of agents, called sheep, is guided into a goal region. Although several effective methodologies and algorithms have been proposed in the last decade for the shepherding problem under various formulations, little research has been directed to the practically important case in which the flock contains sheep agents unresponsive to the sheepdog agent. To fill in this gap, we propose a sheepdog algorithm for guiding unresponsive sheep in this paper. In the algorithm, the sheepdog iteratively applies an existing shepherding algorithm, the farthest-agent targeting algorithm, while dynamically switching its destination. This procedure achieves the incremental growth of a controllable flock, which finally enables the sheepdog to guide the entire flock into the goal region. Furthermore, we illustrate by numerical simulations that the proposed algorithm can outperform the farthest-agent targeting algorithm.
The control of artificial objects has been studied extensively in conjunction with the development of control engineering centered on feedback control [1]. Moreover, it has been widely applied to the control of transportation machinery [2], industrial processes [3], epidemic processes [4], and pest management [5]. On the contrary, controlling the swarm motion of non-artificial objects such as a herd of animals is not necessarily an easy task because of the difficulty of modeling animal dynamics, individual differences, and nonlinearities. Nevertheless, many research and development systems have already been proposed to control non-artificial objects due to their potential applications in various situations. Examples include guiding sheep with unmanned robots [6], guiding crowds during evacuations [7,8], developing a swarm of unmanned aircraft to keep birds away from runways to prevent bird strikes [9] and the collection of oil spilled from tankers [10].
In the context of multi-agent systems [11,12], the shepherding problem [13] refers to a problem of guiding a flock of agents from an initial location to an objective area by fewer external entities and have been widely studied due to its several potential applications such as robotic agents to herd sheep and crowd control. In the shepherding problem, a single or multiple sheepdogs guide a group of agents, called sheep, to a destination. The sheep move according to their own dynamics, and the sheepdog is required to have an advanced movement law to guide the flock to the destination. Following some early pioneering works (see, e.g., [14,15]) motivated by real sheepdogs and sheep, emerging attention has been paid to the shepherding problem in recent years (see the survey [13] and the references therein). For example, Vaughan et al. [16] proposed a movement law for a sheepdog to approach the center of gravity of a flock of sheep and conducted an actual experiment using a robot to guide a flock of ducks. Strömbom et al. [17] proposed a movement law that switches between two modes of collection and guidance and showed that the law could explain the behavior of a sheepdog on an actual farm. Tsunoda et al. [18] proposed a movement law that approaches the sheep farthest from the goal in the flock, and showed its superiority to the movement laws of Vaughan et al. and Strömbom et al. by simulation.
We can find a further increasing amount of research effort on the shepherding problem in the recent literature. As for the shepherding model, Yaxley et al. [19] examined the behavioral and physiological response of real sheep to drones for establishing a plausible mathematical model of the shepherding problem. Also, El-Fiqi et al. [20] presented a preliminary study for improving the shepherding model by Strömbom et al. [17]. In the context of robotics, Ordaz-Rivas et al. [21] proposed a shepherding algorithm taking into account the kinematics of the steering agent, and demonstrated its effectiveness with experiments. Furthermore, Song et al. [22] proposed a caging type shepherding algorithm and demonstrated its efficacy with experiments. We can also find research works on the shepherding problem in various directions. For example, Ko and Zuazua [23] investigated the shepherding problem for the flock of evaders trying to escape from the goal region, and proposed a feedback strategy stabilizing the direction of evaders. Nguyen et al. [24] have demonstrated the potential scalability in the perceptron-based design of shepherding algorithm. The simulations by Goel et al. [25] suggests the superiority of the guidance by the shepherding model over the one by a leader model. Campbell et al. [26] proposed various consensus-based shepherding algorithms and thoroughly compared their performances with numerical simulations.
Although most of the previous works on the shepherding problem assume that the agents (or sheep) are qualitatively the same, this homogeneity assumption does not necessarily hold in realistic scenarios [27,28]. Specifically, in the context of the control of non-artificial objects, it is not easy to ensure the homogeneity of agents to be controlled, unlike in the case of artificial objects [29]. Furthermore, as will be illustrated in this paper via simulations, the shepherding algorithms based on the homogeneity assumption do not necessarily perform well for heterogeneous flocks. This gap between the reality and the current results in the literature suggests the necessity of exploring the problem of shepherding flocks having heterogeneity.
In this paper, we first propose a mathematical model of a heterogeneous flock consisting of the following two types of agents; responsive agents (agents that respond well to the sheepdog agent) and unresponsive agents (agents that do not respond well to the sheepdog). This scenario is motivated by crowd evacuation in disasters, in which crowds are under panic and tend to follow other people without their individuality [30]. This scenario could also be found in the context of swarm robotics; a malfunction or a failure in its communication devices can make robots lose their ability to follow direction from an external sheepdog robot. However, it can be easily expected and confirmed that several existing algorithms for shepherding do not work well in this scenario.
Motivated by the aforementioned gap lying in the current literature, we then present an algorithm that can guide both responsive and unresponsive sheep by leveraging the attraction of unresponsive sheep to responsive sheep. Specifically, in the algorithm, the sheepdog iteratively applies an existing shepherding algorithm, the farthest-agent targeting algorithm, while dynamically switching its destination. This procedure achieves the incremental growth of a controllable flock, which finally enables the sheepdog to guide the entire flock into the goal region.
The effectiveness of the proposed algorithm is evaluated in comparison with existing methods by simulation. First, we consider a flock in which some sheep agents do not respond to the sheepdog agent at all. The total number of sheep, the number of unresponsive sheep, and the initial density of sheep placement are varied. We find that the proposed algorithm outperforms the farthest-agent targeting algorithm in success rates of shepherding. In addition, the time required for successful induction is compared by varying the degree to which the sheep that do not respond to the sheepdog respond. From this numerical simulation, we confirm the robustness of the proposed method with respect to the degree.
This paper is organized as follows. In Section 2, we describe the proposed model of the shepherding problem. In Section 3, we describe our proposed guidance method using the flock properties. In Section 4, we compare the proposed algorithm with existing methods by simulation. We conclude the paper and mention open research directions in Section 5.
In this section, we describe the proposed model of the shepherding problem. We first state the dynamical model of the agents called sheep in Subsection 2.1. Then, in Subsection 2.2, we describe the heterogeneity of the agents. After formulating the shepherding problem in Subsection 2.3, in Subsection 2.4 we discuss and illustrate the potential limitation of an existing shepherding algorithm called the farthest-agent targeting algorithm [18].
We consider the situation where there exist N agents called sheep and one herder agent called sheepdog. As in the literature [17,18]. The sheep and sheepdog are assumed to automatically and dynamically move on the two-dimensional plane R2 in discrete-time steps based on the well-known Boids model. For all i∈{1,…,N} and k≥0, we let xi(k)∈R2 denote the position of the ith sheep at time k. Then, the update law of the position of the sheep is described as
xi(k+1)=xi(k)+vi(k), | (2.1) |
where vi(k)∈R2 denotes the movement vector of the ith sheep at time k and is constructed as
vi(k)=Ks1v1i(k)+Ks2v2i(k)+Ks3v3i(k)+Ks4v4i(k), | (2.2) |
in which the vectors v1i(k), v2i(k), and v3i(k)∈R2 are the movement vectors corresponding to the separation, union, and alignment in the Boids model, respectively. Also, the vector v4i(k) represents the repulsion force applied from the sheepdog. Furthermore, the nonnegative numbers Ks1, Ks2, Ks3, and Ks4 are the constants for determining the weights of each of the vectors.
We assume that each sheep interacts with not all the sheep on the plane but with the sheep sufficiently close to itself. Specifically, we assume that the ith sheep receives forces from all the sheep located in an open disc Si(k)⊂R2 with center xi(k) and radius R>0. Let Ni(k) denote the set of indices of the sheep located in the region Si(k) at time k; i.e., let us define
Ni(k)={j∈{1,…,N}∖{i}∣xj(k)∈Si(k)}. | (2.3) |
Then, the movement vectors v1i(k), v2i(k), v3i(k), and v4i(k) in Eq (2.2) are defined as follows:
v1i(k)=−1|Ni(k)|∑j∈Ni(k)xj(k)−xi(k)‖xj(k)−xi(k)‖3, | (2.4) |
v2i(k)=1|Ni(k)|∑j∈Ni(k)vj(k−1)‖vj(k−1)‖, | (2.5) |
v3i(k)=1|Ni(k)|∑j∈Ni(k)xj(k)−xi(k)‖xj(k)−xi(k)‖, | (2.6) |
v4i(k)=xd(k)−xi(k)‖xd(k)−xi(k)‖3, | (2.7) |
where xd(k)∈R2 denotes the position of the sheepdog at time k, and ‖⋅‖ denotes the Euclidean norm of a vector. We remark that the equations in the defin itions (2.4)–(2.6) are ill-defined if the set Ni(k) is empty; to avoid this potential undecidablility, we let v1i(k)=v2i(k)=v3i(k)=0 when Ni(k) is empty.
We describe the heterogeneity within the sheep flock that we consider in this paper. Among various types of heterogeneity that we can introduce in the flock, in this paper, we focus on the responsiveness of sheep to the sheepdog, as we can consider the responsiveness to be one of the most critical factors for shepherding. Therefore, we consider the situation in which the sheep flock consists of the following two types of individuals: one is the sheep that respond well to the sheepdog (called responsive sheep), and the other is the sheep that do not respond well to the sheepdog (called unresponsive sheep).
We mathematically model the aforementioned situation in the following manner. Let us assume that there exist K≤N unresponsive sheep within the flock. We assign the indices 1,2,…,K to the unresponsive sheep without loss of generality. Therefore, the remaining N−K sheep are assumed to be responsive, and are indexed as K+1,…,N. It is supposed that both the responsive and unresponsive sheep follow the dynamics specified by Eqs (2.4)–(2.7). Their difference is encoded as the difference in the value of the coefficient Ks4 on the repulsion force v4i from the sheepdog. In this paper, we let this coefficient of unresponsive sheep be denoted by ˜K4s. Under this notation, the dynamics of an unresponsive sheep i is now described as
vi(k)=Ks1v1i(k)+Ks2v2i(k)+Ks3v3i(k)+˜Ks4v4i(k). | (2.8) |
The unresponsiveness is realized by setting the coefficient ˜Ks4 smaller than the one Ks4 of the responsive sheep. Hence, we assume the relationship
0≤˜Ks4<Ks4. | (2.9) |
In the extreme case of ˜Ks4=0 within the unresponsive sheep, an unresponsive sheep completely ignores the presence of the sheepdog. We call such an unresponsive sheep as a non-reacting sheep. We finally remark that the value of the coefficient Ks4 is assumed to be common within the responsive sheep, and so is ˜Ks4. In other words, we do not consider the heterogeneity within the flock of responsive and unresponsive sheep.
We can now state the problem studied in this paper. As briefly described in the beginning of Subsection 2.1, we assume that there exists one and only one sheepdog. Furthermore, we assume that the sheepdog knows 1) the global positions of all sheep at each time step and 2) the type of each of the sheep (i.e., responsive or unresponsive). We place these assumptions because the objective of this research is not in the real-world implementation of the algorithm but in clarifying the possibility of shepherding a heterogeneous flock of sheep with an appropriately designed shepherding algorithm. Therefore, we leave as a future work the investigation of the practicability of the algorithm to be proposed.
The objective of the sheepdog is set to be herding all the sheep into a goal region G⊂R2 within a specified time T>0. In this paper, we suppose that the goal region G is an open disk with center xg∈R2 and radius Rg>0; therefore,
G={x∈R2∣‖x−xg‖<Rg}. | (2.10) |
One natural candidate algorithm that could solve the above-stated shepherding problem in the heterogeneous setting would be the farthest-agent targeting algorithm proposed in [18]. This algorithm is a method of guiding a flock of sheep by approaching the sheep farthest from the destination and is numerically illustrated in [18] to be superior to other typical shepherding algorithms such as the target switching algorithm presented in [17].
Let us first briefly describe the detail of the farthest-agent targeting algorithm. In this algorithm the sheepdog focuses on the sheep farthest from the destination. First, the position of the sheepdog xd is updated from time k to time k+1 as in the following equation:
xd(k+1)=xd(k)+vd(k), | (2.11) |
where vd(k)∈R2 denotes the movement vector of the sheepdog and is constructed as
vd(k)=Kd1v1(k)+Kd2v2(k)+Kd3v3(k). | (2.12) |
On the right-hand side of this equation, Kd1, Kd2, and Kd3 are nonnegative constants. The movement vectors v1(k), v2(k), and v3(k) are constructed using the sheep farthest from the destination as follows. For each time k, let t(k)∈{1,…,N} denote the index of the sheep farthest from the destination; i.e., let us define
t(k)=argmax1≤i≤N‖xi(k)−xg‖. | (2.13) |
We remark that, if there exist multiple indices i maximizing the term ‖xi(k)−xg‖, then we take the smallest one. Then, the movement vectors v1(k), v2(k), and v3(k) in Eq (2.12) are defined by
v1(k)=xd(k)−xt(k)(k)‖xd(k)−xt(k)(k)‖, | (2.14) |
v2(k)=−xd(k)−xt(k)(k)‖xd(k)−xt(k)(k)‖3, | (2.15) |
v3(k)=xd(k)−xg‖xd(k)−xg‖, | (2.16) |
which represent the gravitational force from the sheep t(k) to the sheepdog, the repulsive force from the sheep t(k) to the sheepdog, and the repulsive force from the destination to the sheepdog, respectively.
Although the farthest-agent targeting algorithm is known for its superiority to some other algorithms including the one presented in [17] under homogeneous situations, the algorithm is not necessarily able to solve the heterogeneous shepherding problem stated in Subsection 2.3 effectively because the method does not assume the heterogeneity. The specific reason for this limitation is that, once an unresponsive sheep becomes the farthest agent, the algorithm would slow down because the sheepdog needs to herd the unresponsive sheep. This phenomenon can be fatal when the unresponsive and farthest sheep is non-reacting; in this case, we can easily expect that the algorithm becomes stuck in the middle. To confirm this qualitative argument, we perform the shepherding of a heterogeneous flock with the farthest-agent targeting algorithm. As is discussed, the algorithm becomes stuck in the middle as shown in Figure 1, where the sheepdog tries to chase a non-reacting sheep. From several numerical simulations with various settings, we have confirmed that this phenomenon is not an exception.
Motivated by the potential limitation of the farthest-agent targeting algorithm, we propose another algorithm for shepherding with potentially higher success rates and shorter shepherding times in the next section.
In this section, we describe the algorithm for solving the shepherding problem. We start by giving an overview of the proposed algorithm. The proposed algorithm can be divided into two stages, which we call operations a and b summarized as follows:
a. Collect all responsive sheep around an unresponsive sheep.
b. Guide all sheep to the goal area.
In operation a, an unresponsive sheep is set as the temporal destination, and the farthest-agent targeting algorithm is used to collect the responsive sheep around the temporal destination. This procedure creates a controllable flock consisting of all the responsive sheep and one unresponsive sheep. Although the unresponsive sheep does not well-react to the sheepdog, it still heads toward the center of the flock due to its union force Eq (2.6). Therefore, we expect that the entire flock can now be guided by the farthest-agent targeting algorithm.
The above process is repeated for the number of unresponsive sheep, i.e., K times. Thus, for each iteration of the process, one unresponsive sheep will be merged into the controllable flock, which leads to its incremental growth. Therefore, once the operation a is completed, the controllable flock would consist of all the responsive and unresponsive flock. Hence, applying the farthest-agent targeting algorithm to the controllable flock would allow the sheepdog to guide all the sheep into the goal region.
Algorithm 1: proposed algorithm |
procedure PROPOSED ALGORITHM Initialize: I←{K+1,…,N} n←argi∉Imin‖xg−xi‖ j←0 for k←1,2,…,T do if |xi−xn|<r for all i∈I then append n to I n←argi∉Imin‖xn−xi‖ j←j+1 end if if j≤K then Update(I, xn) else Update(U, xg) end if end for end procedure procedure Update G, x v1← attractive force from sheep i∈G which is farthest from x v2← repulsive force from sheep i∈G which is farthest from x v3← repulsive force from x vd←Kd1v1+Kd2v2+Kd3v3 xd←xd+vd end procedure |
Let us describe the details of the proposed shepherding algorithm. For each time k, we let I(k) denote the set of indices in the controllable flock. We initialize
I(0)={K+1,…,N}, | (3.1) |
i.e., we put all the responsive sheep into the set I(0). The objective of operation a is to expand I so that the controllable flock eventually contains all the sheep. To describe the operation, we prepare the variable n(k), which represents the index of the unresponsive sheep set as the temporal destination. We initialize the index as
n(0)=argmaxi∉I(0)‖xg−xi(0)‖, | (3.2) |
i.e., the sheepdog first tries to guide all the responsive sheep around the position of the unresponsive sheep closest to the goal region.
After the initialization, at each time k, the sheepdog tries to collect all the sheep indexed by I(k) to the temporal destination xn(k)(k). This collection is terminated when the following inequality is satisfied
‖xi(k)−xn(k)(k)‖<r, i∈I(k). | (3.3) |
Once this inequality is satisfied, we regard that the sheep n(k) is included into the controllable flock and update the set I(k) as
I(k+1)=I(k)∪{n(k)}. | (3.4) |
We also choose the new unresponsive sheep as the next temporal destination by
n(k+1)=argmaxi∉I(k)∪{n(k)}‖xn(k)(k)−xi(k)‖. | (3.5) |
We repeat this process until all the unresponsive sheep are included in the controllable flock. The feasibility of each step is supported by the effectiveness of the farthest-agent targeting algorithm applied to a flock of responsive sheep.
Once the index set I(k) becomes equal to the full index set {1,…,N}, we switch to operation b. In this operation, the sheepdog applies to the entire flock the farthest-agent targeting algorithm and tries to complete shepherding. Because unresponsive sheep are now all included in the controllable flock, we expect this operation to result in successful shepherding. For the sake of completeness, we summarize the proposed algorithm explained above in Algorithm 1, where for brevity, the dependence of the variables on the time step k is omitted.
We close this section by providing a short analysis of the time complexity of the proposed algorithm. Recall that operation a consists of K distinct processes, in which the number of unresponsive sheep not belonging to the controllable flock decreases from K to 0. For each m=1,…,K, we let ϕ(m) denote the number of time-steps required to merge the mth unresponsive sheep into the controllable flock. If we denote by ϕ(0) the number of time steps required for the operation b, then the total amount of the time steps required in the proposed algorithm equals ∑Km=0ϕ(m). If we let ˉϕ=max(ϕ(0),…,ϕ(K)), then we obtain a rough estimate O(Kˉϕ) of the amount of the time steps, indicating the (at least) linear dependence on K of the time complexity of the proposed algorithm. The evaluation of the time complexity of ˉϕ is not trivial and, therefore, is left as an open problem.
In this section, we illustrate the superiority of the proposed algorithm to the farthest-agent targeting algorithm via several numerical simulations.
In this subsection, we describe the relevant parameters of the simulations. As for the parameters of the sheep, we set Ks1=100, Ks2=0.5, Ks3=2, Ks4=500, and R=20. These values coincide with the ones used in the reference [18] except for Ks4, which we set to be one-tenth of [18]. The reason for setting a smaller value here in this simulation is to make the shepherding problem more challenging. As for the parameters of the sheepdog, we set Kd1=10, Kd2=200, and Kd3=4 as in the reference [18] but except for Kd3, which is now set to be half of [18] for the same reason.
We then describe the initial conditions of the simulations. In the simulations, the N sheep are randomly placed according to a uniform distribution in a region within a circle centered at the origin and having the radius Rinit>0. We define the density of the initial placement in the circle as
ρ=NR2initπ. | (4.1) |
The goal region is set to be the open disc having radius Rg=15 and center xg=(20,20). The position of the sheepdog is initialized as xd(0)=(−30,−50). Finally, we require that the shepherding task finishes within T=10000 discrete-time steps.
In order to illustrate the above parameters as well as the proposed algorithm, we here run the proposed algorithm for the case of N=10 sheep, K=4 non-reacting sheep (i.e., ˜Ks4=0), and density ρ=8×10−4. We show some snapshots in Figure 2. We can observe that the algorithm allows the sheepdog to grow the controllable flock one by one, and successfully guide all the sheep into the goal region G eventually, despite the presence of non-reacting sheep.
In this subsection, we thoroughly compare the performance of the proposed algorithm and the farthest-targeting algorithm in the scenario when the unresponsive sheep are non-reacting (i.e., ˜Ks4=0). For the comparison, we employ the following two performance indices:
● Shepherding success rate: The number of simulations of 100 different initial arrangements of sheep that resulted in guiding the flock to the destination by time T.
● Shepherding time: The time required to complete the simulation upon successful completion of the guidance.
We evaluate these two indices for various values of the total number N of the sheep, the number K of unresponsive sheep, and the initial density ρ of the sheep. Specifically, as for the total number of the sheep, we consider the following three scenarios: N=10, 30 and 50. As for the number of unresponsive sheep, we start from K=1 and increase it to K=N−1. Therefore, the trivially uncontrollable case of K=N is excluded. Finally, the initial density was chosen from ρ=6×10−4, 8×10−4, and 10×10−4. For the total of 2×3×87=522 scenarios, we randomly generate 100 different initial configurations and then perform both the proposed algorithm and the farthest-targeting algorithm. We then finally compute the success rate of shepherding.
In Figure 3, we illustrate how the success rate depends on the number of non-reacting sheep for all the possible pairs of (N,ρ). We observe that the proposed algorithm outperforms the farthest-agent targeting algorithm regardless of the number of non-reacting sheep for any of the scenarios N=10, 30, and 50. In the farthest-agent targeting algorithm, the success rate decreases almost linearly as K increases. Although we can observe a decrease in the proposed algorithm as well, its amount is relatively small except for large K. The decrease for large K could be attributed to the increased traveling distance of the sheepdog to collect unresponsive sheep, which could have caused the shortage of time for shepherding.
The results in Figure 3 suggest the robustness of the proposed algorithm against the increase in the number of unresponsive sheep. In order to quantify this robustness, let us introduce the following quantity:
η=max{ˉK∣r(K)≥r_forallK≤ˉK}, | (4.2) |
where r(K) denotes the success rate of shepherding with K unresponsive sheep, and r_>0 denotes the minimum required rate of success that needs to be specified by ourselves. Using the value r_=0.5, we compute the robustness index η for both the farthest-agent targeting and the proposed algorithms and show their values in Table 1. From Table 1, we quantitatively confirm the high robustness of the proposed algorithm compared with the farthest-agent targeting algorithm.
(a) N = 10 | |||
ρ=0.0006 | ρ=0.0008 | ρ=0.0010 | |
Farthest-agent targeting algorithm | 1 | 2 | 3 |
Proposed algorithm | 8 | 8 | 8 |
(b) N = 30 | |||
ρ=0.0006 | ρ=0.0008 | ρ=0.0010 | |
Farthest-agent targeting algorithm | 1 | 2 | 3 |
Proposed algorithm | 27 | 28 | 28 |
(c) N = 50 | |||
ρ=0.0006 | ρ=0.0008 | ρ=0.0010 | |
Farthest-agent targeting algorithm | 1 | 2 | 2 |
Proposed algorithm | 19 | 32 | 42 |
Remark 1. To ensure consistency with the original paper [18], we have used the farthest-agent targeting algorithm in its original form. Therefore, the sheepdog may choose as its target an unresponsive sheep. If we allow the algorithm to be modified to better deal with the heterogeneous situation studied in this paper, then one possible modification would be to enable the algorithm to choose only responsive sheep as the farthest agent and avoid choosing unresponsive sheep. However, we can infer that the modified algorithm still underperforms the proposed algorithm. For example, consider the situation in which an unresponsive sheep is the farthest agent and is initially far away enough to any responsive sheep as in Figure 1. Although we can expect that the proposed algorithm can deal with this situation, the unresponsive sheep will be neither driven by the sheepdog agent nor be attracted to any responsive sheep even with the modified algorithm. Further investigation of the performance of the modified algorithm is left for future research.
The under-performance of the farthest-agent targeting algorithm [18] in the previous simulations is within expectation because the algorithm is not designed under the consideration of non-reacting sheep. Therefore, in this subsection, we consider the scenario in which unresponsive sheep are not necessarily non-reacting. Therefore, we allow the coefficient ˜Ks4 to be strictly greater than 0 as in [18]. By performing this simulation, we aim to perform a more fair comparison of the two algorithms.
For simplicity, we fix the values of N and ρ to 30 and 8×10−4, respectively. The values of ˜Ks4 for the unresponsive sheep are set to be 50, 25, 5 and 0.5, while the coefficient Ks4 for the responsive sheep is still set to be 500 as in the previous simulations. Then, for all the possible pairs of ˜Ks4 and the two algorithms, we randomly generate 100 different initial configurations. For each configuration, we perform both the proposed algorithm and the farthest-targeting algorithm and obtain the total number of time steps τp and τc by the proposed and farthest-agent targeting algorithm, respectively. In the simulation, we restrict the number of unresponsive sheep K less than or equal to N/2=15.
We plot the pair (τc,τp) for each of the values of ˜Ks4 and show the results in Figure 4. Let us first focus on the case where the number of the unresponsive sheep is relatively small, ranging from one to three. In this case, there is in average no significant change in the time required for successful shepherding by the proposed and the farthest-agent targeting algorithms. Let us then move to the case where the number of unresponsive sheep is relatively large, ranging from 13 to (the maximum of) 15. In this case, for relatively larger ˜Ks4=50, both algorithms resulted in success, as most of the points do not lie on the threshold lines τc=10,000 and τp=10,000. We even observe the trend that τp is relatively larger than τc for more than half of the cases. This trend would be due to the iterative nature of the proposed algorithm; although the farthest-agent targeting algorithm tries to guide the entire flock of sheep from the beginning, the proposed algorithm gathers the unresponsive sheep one by one. This observation suggests that, in the case of mild unresponsiveness, the farthest-agent targeting algorithm can perform better than the proposed algorithm.
On the other hand, when ˜Ks4=0.5 and, therefore, the unresponsive sheep are nearly non-reacting, the farthest-agent targeting algorithm fails in most of the cases (i.e., τc=T=10,000 due to the termination at the final time) as we observed in the previous section. From this observation, we argue that the simulation results in the previous section for the case of ˜Ks4=0 qualitatively extends to the case of nonzero but small ˜Ks4=0. Specifically, the conclusion on the superiority of the proposed algorithm in the previous section for non-reacting sheep can extend to the case of unresponsive sheep.
In this paper, we have presented an algorithm for the shepherding problem in which the sheep flock contains both responsive and unresponsive individuals. In the algorithm, the sheepdog iteratively applies the farthest-agent targeting algorithm while dynamically switching its destination. This procedure achieves the incremental growth of the controllable flock, which finally enables the sheepdog to guide the entire flock into the goal region. The effectiveness of the proposed algorithm was first illustrated with the simulations for the case of non-reacting sheep and then further supported with the ones for the case of reacting but unresponsive sheep.
There are several research directions that should be further pursued. One such direction is theoretically analyzing the robustness of the proposed method, which was only empirically investigated in the paper. Another research direction is the comprehensive comparison of the proposed method with the algorithms in the literature. Although the superiority over the farthest-agent targeting method suggests the potential effectiveness of the proposed algorithm, performing such a comprehensive analysis is necessary to establish the efficacy of the proposed algorithm. Finally, performing statistical analysis of the simulation results would be helpful to obtain a deeper understanding of the performance of the proposed algorithm.
We thank Li Aiyi for help conducting simulations. This work was supported in part by JSPS KAKENHI Grant JP21H01352.
The authors declare that there is no conflict of interests regarding the publication of this paper.
[1] | T. Samad, A. M. Annaswamy, The Impact of Control Technology, IEEE Control Systems Society, 2011. |
[2] |
A. Sciarretta, L. Guzzella, Control of hybrid electric vehicles, IEEE Control Systems, 27 (2007), 60–70. https://doi.org/10.1109/MCS.2007.338280 doi: 10.1109/MCS.2007.338280
![]() |
[3] | k B. Bequette, Process Control: Modeling, Design, and Simulation, Pearson, 2002. |
[4] |
Z. Wang, Q. Zhang, X. Li, Markovian switching for near-optimal control of a stochastic SIV epidemic model, Math. Biosci. Eng., 16 (2019), 1348–1375. https://doi.org/10.3934/mbe.2019066 doi: 10.3934/mbe.2019066
![]() |
[5] |
Z. Shi, H. Cheng, Y. Liu, Y. Wang, Optimization of an integrated feedback control for a pest management predator-prey model, Math. Biosci. Eng., 16 (2019), 7963–7981. https://doi.org/10.3934/mbe.2019401 doi: 10.3934/mbe.2019401
![]() |
[6] | B. B. Erdene, O. E. Mandakh, Shepherding algorithm of multi-mobile robot system, in 2017 First IEEE International Conference on Robotic Computing, (2017), 358–361. https://doi.org/10.1109/IRC.2017.51 |
[7] | A. Garrell, A. Sanfeliu, F. Moreno-Noguer, Discrete time motion model for guiding people in urban areas using multiple robots, in 2009 IEEE/RSJ International Conference on Intelligent Robots and Systems, (2009), 486–491. https://doi.org/10.1109/IROS.2009.5354740 |
[8] | C. Vo, J. F. Harrison, J. M. Lien, Behavior-based motion planning for group control, in 2009 IEEE/RSJ International Conference on Intelligent Robots and Systems, (2009), 3768–3773. |
[9] | S. Gade, A. A. Paranjape, S. J. Chung, Herding a flock of birds approaching an airport using an unmanned aerial vehicle, in AIAA Guidance, Navigation, and Control Conference, (2015), 1540. https://doi.org/10.2514/6.2015-1540 |
[10] | E. M. H. Zahugi, M. M. Shanta, T. V. Prasad, Oil spill cleaning up using swarm of robots, in Advances in Computing and Information Technology (eds. N. Meghanathan, D. Nagamalai, N. Chaki), Springer, (2013), 215–224. https://doi.org/10.1007/978-3-642-31600-5_22 |
[11] | F. L. Lewis, H. Zhang, K. Hengster-Movric, A. Das, Cooperative Control of Multi-Agent Systems, Springer, 2014. https://doi.org/10.1007/978-1-4471-5574-4 |
[12] |
A. Belhadi, Y. Djenouri, G. Srivastava, J. C. W. Lin, Reinforcement learning multi-agent system for faults diagnosis of mircoservices in industrial settings, Computer Communications, 177 (2021), 213–219. https://doi.org/10.1016/j.comcom.2021.07.010 doi: 10.1016/j.comcom.2021.07.010
![]() |
[13] |
N. K. Long, K. Sammut, D. Sgarioto, M. Garratt, H. A. Abbass, A comprehensive review of shepherding as a bio-Inspired swarm-robotics guidance approach, IEEE Trans. Emerg. Top. Comput. Intel., 4 (2020), 523–537. https://doi.org/10.1109/TETCI.2020.2992778 doi: 10.1109/TETCI.2020.2992778
![]() |
[14] | G. M. Werner, M. G. Dyer, Evolution of herding behavior in artificial animals, in Second International Conference on From Animals to Animats 2: Simulation of Adaptive Behavior, (1993), 393–399. |
[15] | A. C. Schultz, J. J. Grefenstette, W. Adams, Robo-shepherd: Learning complex robotic behaviors, in International Symposium on Robotics and Automation, (1996), 763–768. |
[16] | R. Pfeifer, B. Blumberg, J. A. Meyer, S. W. Wilson, Robot Sheepdog Project achieves automatic flock control, in Fifth International Conference on Simulation of Adaptive Behavior, (1998), 489–493. |
[17] |
D. Strömbom, R. P. Mann, A. M. Wilson, S. Hailes, A. J. Morton, D. J. T. Sumpter, et al., Solving the shepherding problem: heuristics for herding autonomous, interacting agents, J. R. Soc. Interface, 11 (2014), 20140719. https://doi.org/10.1098/rsif.2014.0719 doi: 10.1098/rsif.2014.0719
![]() |
[18] |
Y. Tsunoda, Y. Sueoka, Y. Sato, K. Osuka, Analysis of local-camera-based shepherding navigation, Adv. Robotics, 32 (2018), 1217–1228. https://doi.org/10.1080/01691864.2018.1539410 doi: 10.1080/01691864.2018.1539410
![]() |
[19] |
K. J. Yaxley, K. F. Joiner, H. Abbass, Drone approach parameters leading to lower stress sheep flocking and movement: sky shepherding, Sci. Rep., 11 (2021), 7803. https://doi.org/10.1038/s41598-021-87453-y doi: 10.1038/s41598-021-87453-y
![]() |
[20] | H. E. Fiqi, B. Campbell, S. Elsayed, A. Perry, H. K. Singh, R. Hunjet, et al., A preliminary study towards an improved shepherding model, in Proceedings of the 2020 Genetic and Evolutionary Computation Conference Companion, USA, (2020), 75–76. https://doi.org/10.1145/3377929.3390067 |
[21] |
E. O. Rivas, A. Rodriguez-Liñan, L. Torres-Treviño, Flock of robots with self-cooperation for prey-predator task, J. Intell. Robotic Syst. Theory Appl., 101 (2021), 39. https://doi.org/10.1007/s10846-020-01283-0 doi: 10.1007/s10846-020-01283-0
![]() |
[22] |
H. Song, A. Varava, O. Kravchenko, D. Kragic, M. Y. Wang, F. T. Pokorny, et al., Herding by caging: a formation-based motion planning framework for guiding mobile agents, Auton. Robot., 45 (2021), 613–631. https://doi.org/10.1007/s10514-021-09975-8 doi: 10.1007/s10514-021-09975-8
![]() |
[23] |
D. Ko, E. Zuazua, Asymptotic behavior and control of a guidance by repulsion model, Math. Mod. Meth. Appl. Sci., 30 (2020), 765–804. https://doi.org/10.1142/S0218202520400047 doi: 10.1142/S0218202520400047
![]() |
[24] | T. Nguyen, J. Liu, H. Nguyen, K. Kasmarik, S. Anavatti, M. Garratt, et al., Perceptron-learning for scalable and transparent dynamic formation in swarm-on-swarm shepherding, in Proceedings of the International Joint Conference on Neural Networks, (2020), 1–8. |
[25] | R. Goel, J. Lewis, M. Goodrich, P. Sujit, Leader and predator based swarm steering for multiple tasks, in 2019 IEEE International Conference on Systems, Man and Cybernetics, (2019), 3791–3798. https://doi.org/10.1109/SMC.2019.8913942 |
[26] | B. Campbell, H. E. Fiqi, R. Hunjet, H. Abbass, Distributed multi-agent shepherding with consensus, in 12th International Conference on Swarm Intelligence, (2021), 168–181. https://doi.org/10.1007/978-3-030-78811-7_17 |
[27] |
A. Fujita, C. Feliciani, D. Yanagisawa, K. Nishinari, Traffic flow in a crowd of pedestrians walking at different speeds, Phys. Rev. E, 99 (2019), 062307. https://doi.org/10.1103/PhysRevE.99.062307 doi: 10.1103/PhysRevE.99.062307
![]() |
[28] |
M. Scatà, A. Di Stefano, P. Liò, A. La Corte, The impact of heterogeneity and awareness in modeling epidemic spreading on multiplex networks, Sci. Rep., 6 (2016), 37105. https://doi.org/10.1038/srep37105 doi: 10.1038/srep37105
![]() |
[29] |
T. Kamegawa, T. Akiyama, S. Sakai, K. Fujii, K. Une, E. Ou, et al., Development of a separable search-and-rescue robot composed of a mobile robot and a snake robot, Adv. Rob., 34 (2020), 132–139. https://doi.org/10.1080/01691864.2019.1691941 doi: 10.1080/01691864.2019.1691941
![]() |
[30] |
D. Helbing, A. Johansson, H. Z. Al-Abideen, Dynamics of crowd disasters: An empirical study, Phys. Rev. E, 75 (2007), 046109. https://doi.org/10.1103/PhysRevE.75.046109 doi: 10.1103/PhysRevE.75.046109
![]() |
1. | Anna Fujioka, Masaki Ogura, Naoki Wakamiya, Shepherding algorithm for heterogeneous flock with model-based discrimination, 2023, 37, 0169-1864, 99, 10.1080/01691864.2022.2133552 | |
2. | Adam J Hepworth, Aya Hussein, Darryn J Reid, Hussein A Abbass, Swarm analytics: Designing information markers to characterise swarm systems in shepherding contexts, 2022, 1059-7123, 105971232211370, 10.1177/10597123221137090 | |
3. | Anna Fujioka, Masaki Ogura, Naoki Wakamiya, 2022, Shepherding Algorithm Based on Variant Agent Detection for Heterogeneous Flock, 978-4-9077-6478-4, 87, 10.23919/SICE56594.2022.9905841 | |
4. | Sai Zhang, Li Tang, Yan-Jun Liu, Formation deployment control of multi-agent systems modeled with PDE, 2022, 19, 1551-0018, 13541, 10.3934/mbe.2022632 | |
5. | Adam J. Hepworth, Aya S. M. Hussein, Darryn J. Reid, Hussein A. Abbass, Contextually aware intelligent control agents for heterogeneous swarms, 2024, 18, 1935-3812, 275, 10.1007/s11721-024-00235-w | |
6. | Yusuke Tsunoda, Le Trong Nghia, Yuichiro Sueoka, Koichi Osuka, Experimental Analysis of Shepherding-Type Robot Navigation Utilizing Sound-Obstacle-Interaction, 2023, 35, 1883-8049, 957, 10.20965/jrm.2023.p0957 | |
7. | Wataru Imahayashi, Yusuke Tsunoda, Masaki Ogura, Route design in sheepdog system–traveling salesman problem formulation and evolutionary computation solution–, 2024, 38, 0169-1864, 632, 10.1080/01691864.2024.2321598 |
Algorithm 1: proposed algorithm |
procedure PROPOSED ALGORITHM Initialize: I←{K+1,…,N} n←argi∉Imin‖xg−xi‖ j←0 for k←1,2,…,T do if |xi−xn|<r for all i∈I then append n to I n←argi∉Imin‖xn−xi‖ j←j+1 end if if j≤K then Update(I, xn) else Update(U, xg) end if end for end procedure procedure Update G, x v1← attractive force from sheep i∈G which is farthest from x v2← repulsive force from sheep i∈G which is farthest from x v3← repulsive force from x vd←Kd1v1+Kd2v2+Kd3v3 xd←xd+vd end procedure |
(a) N = 10 | |||
ρ=0.0006 | ρ=0.0008 | ρ=0.0010 | |
Farthest-agent targeting algorithm | 1 | 2 | 3 |
Proposed algorithm | 8 | 8 | 8 |
(b) N = 30 | |||
ρ=0.0006 | ρ=0.0008 | ρ=0.0010 | |
Farthest-agent targeting algorithm | 1 | 2 | 3 |
Proposed algorithm | 27 | 28 | 28 |
(c) N = 50 | |||
ρ=0.0006 | ρ=0.0008 | ρ=0.0010 | |
Farthest-agent targeting algorithm | 1 | 2 | 2 |
Proposed algorithm | 19 | 32 | 42 |
Algorithm 1: proposed algorithm |
procedure PROPOSED ALGORITHM Initialize: I←{K+1,…,N} n←argi∉Imin‖xg−xi‖ j←0 for k←1,2,…,T do if |xi−xn|<r for all i∈I then append n to I n←argi∉Imin‖xn−xi‖ j←j+1 end if if j≤K then Update(I, xn) else Update(U, xg) end if end for end procedure procedure Update G, x v1← attractive force from sheep i∈G which is farthest from x v2← repulsive force from sheep i∈G which is farthest from x v3← repulsive force from x vd←Kd1v1+Kd2v2+Kd3v3 xd←xd+vd end procedure |
(a) N = 10 | |||
ρ=0.0006 | ρ=0.0008 | ρ=0.0010 | |
Farthest-agent targeting algorithm | 1 | 2 | 3 |
Proposed algorithm | 8 | 8 | 8 |
(b) N = 30 | |||
ρ=0.0006 | ρ=0.0008 | ρ=0.0010 | |
Farthest-agent targeting algorithm | 1 | 2 | 3 |
Proposed algorithm | 27 | 28 | 28 |
(c) N = 50 | |||
ρ=0.0006 | ρ=0.0008 | ρ=0.0010 | |
Farthest-agent targeting algorithm | 1 | 2 | 2 |
Proposed algorithm | 19 | 32 | 42 |