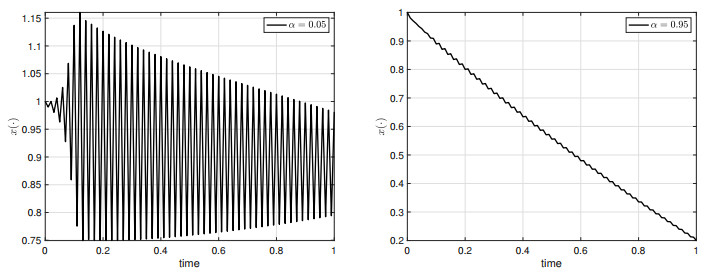
Researchers have proposed applying optimization techniques to improve performance of microstrip antennas (MSAs) in terms of bandwidth, radiation characteristics, polarization, directivity and size. The drawbacks of the conventional MSAs can be overcome by optimizing the antenna parameters while keeping a compact configuration. Applying a global optimizer is a better technique than using a local optimizer or a trial and error method for performance enhancement. This paper discusses genetic algorithm (GA) optimization of microstrip antennas presented by the antenna research community. The GA optimization procedure, antenna parameters optimized by using GA and the optimization objectives are presented by reviewing the literature. Further, evolution of GA in the field of MSAs and its significance are explored. Application of GA optimization to design broadband, multiband, high-directivity and miniature antennas is demonstrated with the support of several case studies giving an insight for further developments in the field.
Citation: Jeevani W. Jayasinghe. Application of Genetic Algorithm for Binary Optimization of Microstrip Antennas: A Review[J]. AIMS Electronics and Electrical Engineering, 2021, 5(4): 315-333. doi: 10.3934/electreng.2021016
[1] | Irshad Ahmad, Saeed Ahmad, Ghaus ur Rahman, Manuel De la Sen . Controllability of a generalized multi-pantograph system of non-integer order with state delay. AIMS Mathematics, 2023, 8(6): 13764-13784. doi: 10.3934/math.2023699 |
[2] | Sivaranjani Ramasamy, Thangavelu Senthilprabu, Kulandhaivel Karthikeyan, Palanisamy Geetha, Saowaluck Chasreechai, Thanin Sitthiwirattham . Existence, uniqueness and controllability results of nonlinear neutral implicit ABC fractional integro-differential equations with delay and impulses. AIMS Mathematics, 2025, 10(2): 4326-4354. doi: 10.3934/math.2025200 |
[3] | Hawsar HamaRashid, Hari Mohan Srivastava, Mudhafar Hama, Pshtiwan Othman Mohammed, Musawa Yahya Almusawa, Dumitru Baleanu . Novel algorithms to approximate the solution of nonlinear integro-differential equations of Volterra-Fredholm integro type. AIMS Mathematics, 2023, 8(6): 14572-14591. doi: 10.3934/math.2023745 |
[4] | Ahmed Morsy, Kottakkaran Sooppy Nisar, Chokkalingam Ravichandran, Chandran Anusha . Sequential fractional order Neutral functional Integro differential equations on time scales with Caputo fractional operator over Banach spaces. AIMS Mathematics, 2023, 8(3): 5934-5949. doi: 10.3934/math.2023299 |
[5] | Jie Yang, Guoping Chen . Existence of solutions for impulsive hybrid boundary value problems to fractional differential systems. AIMS Mathematics, 2021, 6(8): 8895-8911. doi: 10.3934/math.2021516 |
[6] | Yun Xin, Hao Wang . Positive periodic solution for third-order singular neutral differential equation with time-dependent delay. AIMS Mathematics, 2020, 5(6): 7234-7251. doi: 10.3934/math.2020462 |
[7] | M. Mallika Arjunan, Nabil Mlaiki, V. Kavitha, Thabet Abdeljawad . On fractional state-dependent delay integro-differential systems under the Mittag-Leffler kernel in Banach space. AIMS Mathematics, 2023, 8(1): 1384-1409. doi: 10.3934/math.2023070 |
[8] | Madeaha Alghanmi, Shahad Alqurayqiri . Existence results for a coupled system of nonlinear fractional functional differential equations with infinite delay and nonlocal integral boundary conditions. AIMS Mathematics, 2024, 9(6): 15040-15059. doi: 10.3934/math.2024729 |
[9] | Muhammad Nazam, Aftab Hussain, Asim Asiri . On a common fixed point theorem in vector-valued b-metric spaces: Its consequences and application. AIMS Mathematics, 2023, 8(11): 26021-26044. doi: 10.3934/math.20231326 |
[10] | Zhibo Cheng, Lisha Lv, Jie Liu . Positive periodic solution of first-order neutral differential equation with infinite distributed delay and applications. AIMS Mathematics, 2020, 5(6): 7372-7386. doi: 10.3934/math.2020472 |
Researchers have proposed applying optimization techniques to improve performance of microstrip antennas (MSAs) in terms of bandwidth, radiation characteristics, polarization, directivity and size. The drawbacks of the conventional MSAs can be overcome by optimizing the antenna parameters while keeping a compact configuration. Applying a global optimizer is a better technique than using a local optimizer or a trial and error method for performance enhancement. This paper discusses genetic algorithm (GA) optimization of microstrip antennas presented by the antenna research community. The GA optimization procedure, antenna parameters optimized by using GA and the optimization objectives are presented by reviewing the literature. Further, evolution of GA in the field of MSAs and its significance are explored. Application of GA optimization to design broadband, multiband, high-directivity and miniature antennas is demonstrated with the support of several case studies giving an insight for further developments in the field.
In this paper, we consider the minimization problem of
J(x0,u(⋅))=∫T0l(s,x(s),u(s))ds+h(x0,x(T)), | (1.1) |
subject to the Rn-valued integral-type state equation with α∈(0,1),
x(t)=x0+∫t0f(t,s,x(s),u(s))(t−s)1−αds,a.e.t∈[0,T], | (1.2) |
and the terminal state constraint
(x0,x(T))∈F⊂R2n. | (1.3) |
In (1.1)–(1.3), x(⋅)∈Rn is the state with the initial state x0∈Rn, and u(⋅)∈U is the control with U being the control space (see Section 2). Hence, we consider the following terminal state-constrained optimal control problem:
(P)infu(⋅)∈Up[0,T]J(x0,u(⋅)),subject to (1.2) and (1.3), |
where Up[0,T] is the set of admissible controls of (1.2). The problem aforementioned is referred to as (P) throughout this paper, and the precise problem statement of (P) is provided in Section 2. Note that the optimal control problems with and without state constraints capture various practical aspects of systems in science, biology, engineering, and economics [2,4,5,6,29,41,43].
The state equation in (1.2) is known as a class of Volterra integral equations. The main feature of Volterra integral equations is the effect of memories, which does not appear in ordinary (state) differential equations. In fact, Volterra integral equations of various kinds have been playing an important role in modeling and analyzing of practical physical, biological, engineering, and other phenomena that are governed by memory effects [7,17,19,32,34]. We note that one major distinction between (1.2) and other classical Volterra integral equations is that (1.2) has a kernel f(t,s,x,u)(t−s)1−α, which becomes singular at s=t. In fact, α∈(0,1) in the singular kernel f(t,s,x,u)(t−s)1−α of (1.2) determines the amount of the singularity, in which the large singular behavior occurs with small α∈(0,1). Hence, the singular kernel in (1.2) can be applied to various areas of science, engineering, finance and economics, in which certain extraordinary phenomena have to be described. For example, the model of fluid dynamics may have some singular phenomena, leading to the deformation of the corresponding dynamic equation [17,33,36].
The study of optimal control problems for various kinds of Volterra integral equations via the maximum principle have been studied extensively in the literature; see [3,12,13,18,20,22,23,30,35,39,40] and the references therein. Specifically, the first study on optimal control for Volterra integral equations (using the maximum principle) can be traced back to [40]. Several different formulations (with and without state constraints and/or delay) of optimal control for Volterra integral equations and their generalizations are reported in [3,7,12,18,20,30,35,39]. Some recent progress in different directions including stochastic frameworks can be found in [13,22,23,26,27,42]. We note that the above-mentioned existing works (with the exception of the stochastic case in [26,27,42]) considered the situation with nonsingular kernels only in Volterra integral equations, which corresponds to the case of f(t,s,x,u)=(t−s)1−αg(t,s,x,u) in (1.2). Hence, the problem settings in the earlier works can be viewed as a special case of (P).
Recently, (P) without the terminal state constraint in (1.3) was studied in [34]. Due to the presence of the singular kernel, the technical analysis including the maximum principle (without the terminal state constraint) in [34] should be different from that of the existing works mentioned above. In particular, the proof for the well-posedness and estimates of Volterra integral equations in [34, Theorem 3.1] requires a new type of Gronwall's inequality. Furthermore, the maximum principle without the terminal state constraint in [34, Theorem 4.3] needs a different duality and variational analysis via spike perturbation. More recently, the linear-quadratic optimal control problem (without the state constraint) for linear Volterra integral equations with singular kernels was studied in [28].
We note that the state equation in (1.2) is strongly related to classical state differential equations and (Caputo) fractional order differential equations. In particular, let DCα[x(⋅)] be the Caputo fractional derivative operator of order α∈(0,1) [32, Chapter 2.4]. Subsequently, by [32, Theorem 3.24 and Corollary 3.23], (1.2) becomes (Γ(⋅) denotes the gamma function)
DCα[x(⋅)](t)=f(t,x(t),u(t))⇔x(t)=x0+1Γ(α)∫t0f(s,x(s),u(s))(t−s)1−αds. | (1.4) |
In addition, when f(t,s,x,u)=(t−s)1−αg(s,x,u), (1.2) is specialized to
dx(t)dt=g(t,x(t),u(t))⇔x(t)=x0+∫t0g(s,x(s),u(s))ds. | (1.5) |
As (1.4) and (1.5) are special cases of our state equation in (1.2), the state equation in (1.2) is able to describe various types of differential equations including fractional and ordinary differential equations. We also mention that there are several different results on optimal control for fractional differential equations; see [1,8,14,25,31] and the references therein.
The aim of this paper is to obtain the Pontryagin maximum principle for (P). As noted above, since [34] did not consider the state-constrained control problem, (P) can be viewed as a generalization of [34] to the terminal state-constrained control problem. The main theoretical significances and technical difficulties are the proof of the maximum principle in Theorem 3.1, which is presented in Section 5. In particular, due to (i) the inherent complex nature of the Volterra integral equation with singular kernels, (ii) the presence of the terminal state constraint, and (iii) the generalized standing assumptions of f, our maximum principle and its detailed proof must be different from those provided in the existing literature (e.g., [12,18,22,23,30,34,35,39]). Note also that as our state equation in (1.2) includes the case of Caputo fractional differential equation in (1.4), the maximum principle in Theorem 3.1 also covers [8, Theorem 3.12]. However, as the variational analysis of this paper and that of [8] are different, the proof techniques including the characterization of the adjoint equation must be different. Indeed, the maximum principle of this paper is new in the optimal control problem context and its proof requires a different technique, compared with the existing literature.
The detailed statements of the main results of this paper are provided as follows:
● Regarding (ii), as mentioned above, the proof in Section 5 must be different from that of the unconstrained control problem in [34, Theorem 4.3]. Specifically, in contrast to [34, Theorem 4.3], due to the terminal state constraint in (1.3), we have to employ the Ekeland variational principle and the spike variation technique, together with the intrinsic properties of the distance function and the generalized Gronwall's inequality, to establish the duality analysis for Volterra-type forward variational and backward adjoint equations with singular kernels (see Lemma 5.2, Lemma 5.3 and Section 5.6). Note that this analysis leads to the desired necessary condition for optimality. Such a generalized proof of the maximum principle including the duality and variational analysis was not needed in [34, Theorem 4.3], as it did not consider the terminal state constraint in the corresponding optimal control problem. Note also that without the terminal state constraint, our maximum principle in Theorem 3.1 is reduced to the unconstrained case in [34, Theorem 4.3] (see Remark 3.1).
● As for (i), since our Volterra integral equation in (1.2) covers both singular and nonsingular kernels, the proof for the maximum principle in Section 5 should be different from that of the classical state-constrained maximum principle with the nonsingular kernel only (when f(t,s,x,u)=(t−s)1−αg(t,s,x,u) in (1.2)) studied in the existing literature (e.g., [12, Theorem 1], [22, Theorem 3.1] and [18,23,30,35,39]). In particular, due to the presence of the singular kernel in (1.2), we need a different proof for duality and variational analysis (see Lemma 3, Lemma 5.3 and Section 5.6). In addition, unlike the existing literature (e.g., [12, Theorem 1] and [22, Theorem 3.1]), our adjoint equation is obtained by the backward Volterra integral equation with the singular kernel, as a consequence of the new duality analysis with the singular kernel in Section 5.6 (see Theorem 3.1 and Remark 3.2).
● Concerning (iii), we mention that unlike the existing works for classical optimal control of Volterra integral equations with nonsingular kernels (e.g., [12,(4) and (5)], [22, page 3437 and (A3)], and [18,23,39]), our paper assumes neither the differentiability of (singular or nonsingular) kernels in (t,s,u) (time and control variables) nor the essentially boundedness of a class of admissible controls (see Remark 3.2). Hence, the detailed proof of the maximum principle provided in this paper must be different from those given in the existing literature.
As mentioned above, the main motivation of studying (P) is to provide the general maximum principle for terminal state-constrained optimal control problems of various classes of differential and integral type equations. Specifically, as mentioned above, (1.2) covers Volterra integral equations with singular and nonsingular kernels (see Remark 3.2), ordinary differential equations (see (1.5)), and Caputo-type fractional differential equations (see (1.4)). Hence, the terminal state-constrained optimal control problems for such kind of (differential and integral) state equations can be solved via the maximum principle of this paper (see Theorem 3.1). As stated above, the maximum principle for (P) has not been presented in the existing literature.
We mention that Volterra integral equations and terminal state-constrained optimal control problems can be applied to various practical applications in science, engineering, economics, and mathematical finance; see [2,4,5,6,7,17,19,29,32,34,41,43] and the references therein. In particular, Volterra integral equations can be used to study the so-called tautochrone problem in mechanical applications, the heat transfer problem in diffusion models, the shock wave problem, the renewal equation in the theory of industrial engineering, the investment problem in economics, and the problem of American option pricing [7,17,19,32,34]. The interacting biological population model can be described by Volterra integral equations [7]. Furthermore, as mentioned above, in a practical point of views, the singular kernel in (1.2) can be applied to those Volterra integral equations to study their singular and/or peculiar behavior. Hence, we can use the modeling framework of (1.2) for any differential and Volterra integral equations to capture the memory and/or singular effects, which can be observed in real world (e.g., the deformation of fluid dynamics due to singular phenomena [17,33,36], and the dependency of the current stock price on past investment strategies over a period of time [7,17]). We also note that the (terminal) state-constrained optimal control problems can be formulated for these examples (e.g., the Volterra-type renewal integral equation in industrial applications, the Volterra-type heat transfer integral equation in diffusion models, and the Volterra-type investment integral equation in economics). In fact, there are huge potential applications of optimal control problems, and their various different practical examples in science, engineering, biology, economics, and mathematical finance, including the optimal control for partial differential equations (PDEs), can be found in [2,4,5,6,29,41,43] and the references therein.
In view of the preceding discussion, we believe that the maximum principle of this paper broadens both the theoretical generality and the applicability of the domain of optimal control problems.
The rest of this paper is organized as follows. The problem statement of (P) is given in Section 2. The statement of the maximum principle for (P) is provided in Section 3. We study an example of (P) in Section 4. The proof of the maximum principle is given in Section 5. Finally, we conclude this paper in Section 6.
Let Rn be an n-dimensional Euclidean space, where ⟨x,y⟩:=x⊤y is the inner product and |x|:=⟨x,x⟩1/2 is the norm for x,y∈Rn. For A∈Rm×n, A⊤ denotes the transpose of A. Let [0,T] be a time interval such that T<∞. Let 1S(⋅) be the indicator function of any set S. In this paper, C≥0 denotes a generic constant, whose value is different from line to line. For any differentiable function f:Rn→Rl, let fx:Rn→Rl×n be the partial derivative of f with respect to x∈Rn. fx=[f⊤1,x⋯f⊤l,x]⊤ with fj,x∈R1×n, and when l=1, fx∈R1×n. For f:Rn×Rl→Rl, fx:Rn×Rl→Rl×n for x∈Rn, and fy:Rn×Rl→Rl×l for y∈Rl. For 1≤p<∞, define
● Lp([0,T];Rn): The space of functions ψ:[0,T]→Rn such that ψ is measurable and satisfies ‖ψ(⋅)‖Lp([0,T];Rn):=(∫T0|ψ(t)|pdt)1/p<∞;
● C([0,T];Rn): The space of functions ψ:[0,T]→Rn such that ψ is continuous and satisfies ‖ψ(⋅)‖∞:=supt∈[0,T]|ψ(t)|<∞.
Next, we state the precise problem statement of (P) provided in Section 1. In (1.2), α∈(0,1) is the parameter of the singularity, x(⋅)∈Rn is the state with the initial state x0∈Rn, and u(⋅)∈U is the control with U being the control space. In (1.2), f(t,s,x,u)(t−s)1−α denotes the singular kernel (with the singularity appearing at s=t), with f:[0,T]×[0,T]×Rn×U→Rn being a generator. Note that α∈(0,1) determines the level of singularity of (1.2); see Figure 1. In (1.2), f is dependent on two time parameters, t and s. While t is the outer time variable to determine the current time, s is the inner time variable describing the path or memory of (1.2) from 0 to t. In (1.1), l:[0,T]×Rn×U→R is the running cost, while h:Rn×Rn→R is the terminal cost.
Assumption 1. (i) (U,ρ) is a separable metric space. f and fx are continuous on [0,T]×[0,T] and Lipschitz continuous in (x,u)∈Rn×U with the Lipschitz constant K≥0. |f(t,s,0,u)|≤K for (t,s,u)∈[0,T]×[0,T]×U.
(ii) l and lx are continuous on [0,T] and Lipschitz continuous in (x,u)∈Rn×U with the Lipschitz constant K≥0. |l(t,0,u)|≤K for (t,u)∈[0,T]×U. h, hx0, and hx are Lipschitz continuous in both variables with the Lipschitz constant K≥0.
(iii) F is a nonempty closed convex subset of R2n.
For p>1α and u0∈U, we define the space of admissible controls for (1.2) as
Up[0,T]={u:[0,T]→U|u is measurable&ρ(u(⋅),u0)∈Lp([0,T];R+)}. |
Note that p>1α is needed for the well-posedness of (1.2), which is stated in the following lemma [34, Theorem 3.1 and Proposition 3.3]:
Lemma 2.1. Let (i) of Assumption 1 hold and p>1α. For any (x0,u(⋅))∈Rn×Up[0,T], (1.2) admits a unique solution in C([0,T];Rn), and there is a constant C≥0 such that ‖x(⋅)‖Lp([0,T];Rn)≤C(1+|x0|+‖ρ(u(⋅),u0)‖Lp([0,T];R+)).
Under Assumption 1, the main objective of this paper is to solve (P) via the Pontryagin maximum principle. Note that Assumption 1 is crucial for the well-posedness of the state equation in (1.2) by Lemma 2.1 as well as the maximum principle of (P). Assumptions similar to Assumption 1 have been used in various optimal control problems and their maximum principles; see [9,12,13,15,16,20,22,23,27,33,34,37,39,40,43] and the references therein. Note also that we do not need the differentiability of f in (t,s,u), which was assumed in the existing literature (e.g., [12,(4) and (5)], [22, page 3437 and (A3)], and [18,23,39]); see Remark 3.2.
We provide the statement of the maximum principles for (P). The proof is given in Section 5.
Theorem 3.1. Let Assumption 1 hold. Suppose that (¯u(⋅),¯x(⋅))∈Up[0,T]×C([0,T];Rn) is the optimal pair for (P), where ¯x(⋅)∈C([0,T];Rn) is the corresponding optimal state trajectory of (1.2). Then there exists a pair (λ,ξ), where λ∈R and ξ=(ξ1,ξ2)∈R2n, such that the following conditions hold:
● Nontriviality condition: (λ,ξ)≠0, where λ≥0 and ξ∈NF(¯x0,¯x(T)) with NF(x) being the normal cone to the convex set F defined in (5.1);
● Adjoint equation: p(⋅)∈Lp([0,T];Rn) is the unique solution to the following backward Volterra integral equation with the singular kernel:
p(t)=−λlx(t,¯x(t),¯u(t))⊤+∫Ttfx(r,t,¯x(t),¯u(t))⊤(r−t)1−αp(r)dr−1[0,T)(t)fx(T,t,¯x(t),¯u(t))⊤(T−t)1−α(λhx(¯x0,¯x(T))+ξ⊤2)⊤,a.e.t∈[0,T]; |
● Transversality conditions:
(i)0≤⟨ξ1,¯x0−y1⟩+⟨ξ2,¯x(T)−y2⟩,∀y=(y1,y2)∈F,(ii)∫T0p(t)dt=ξ1+ξ2+λhx0(¯x0,¯x(T))⊤+λhx(¯x0,¯x(T))⊤; |
● Hamiltonian-like maximum condition:
−λl(t,¯x(t),¯u(t))+∫Ttp(r)⊤f(r,t,¯x(t),¯u(t))(r−t)1−αdr−1[0,T)(t)(λhx(¯x0,¯x(T))+ξ⊤2)f(T,t,¯x(t),¯u(t))(T−t)1−α=maxu∈U{−λl(t,¯x(t),u)+∫Ttp(r)⊤f(r,t,¯x(t),u)(r−t)1−αdr−1[0,T)(t)(λhx(¯x0,¯x(T))+ξ⊤2)f(T,t,¯x(t),u)(T−t)1−α},a.e.t∈[0,T]. |
Remark 3.1. Without the terminal state constraint in (1.3), Theorem 3.1 holds with λ=1 and ξ=0. This is equivalent to [34, Theorem 4.3].
Remark 3.2. By taking f(t,s,x,u)=(t−s)1−αg(t,s,x,u) in Theorem 3.1, we obtain the maximum principle for the optimal control problems of classical Volterra integral equations with nonsingular kernels. Note that Theorem 3.1 is more general than the existing maximum principles for Volterra integral equations with nonsingular kernels (e.g., [12, Theorem 1], [22, Theorem 3.1] and [18,23,30,35,39]), where Theorem 3.1 assumes neither the differentiability of the corresponding kernel with respect to time and control variables nor the essential boundedness of a class of admissible controls (e.g., [12,(4) and (5)], [22, page 3437 and (A3)]). In addition, unlike the existing literature, the adjoint equation in Theorem 3.1 must be obtained in integral form, as a consequence of the new duality analysis with singular kernels in Section 5.6.
The motivation of this section is to demonstrate the applicability of Theorem 3.1 to the state-constrained linear-quadratic problem for Volterra integral equations with singular kernels. We consider the minimization of the objective functional of J(x0,u(⋅))=∫T0[x(s)+12u(s)2]ds subject to the R-valued Volterra integral equation with the singular kernel:
x(t)=x0+∫t0t2u(s)(t−s)1−αds,a.e. t∈[0,T]. | (4.1) |
For Case I, the terminal state constraint is given by (x0,x(T))∈F={x0=10,x(T)=10}⊂R2. Here, we may assume that U is an appropriate sufficiently large compact set satisfying Assumption 1. Note that this example is closely related to the (terminal) state-constrained linear-quadratic control problem, which can be applied to study the singular aspects of various applications in science, engineering, and economics.
Let T=3. By Theorem 3.1, the adjoint equation is p(t)=−λ. From the first transversality condition in (i), we have ξ∈R2. Then by the second transversality condition in (ii), ∫30p(t)dt=−3λ=ξ1+ξ2. Hence, we can choose λ=1 and ξ1=ξ2=−1.5. By the Hamiltonian-like maximum condition and the first-order optimality condition, the (candidate) optimal solution of Case I is as follows:
¯u(t)=∫3t−t2(r−t)1−αdr+t21.51[0,3)(t)(3−t)1−α,a.e. t∈[0,3]. | (4.2) |
We apply (4.2) to get the optimal state trajectory of (4.1). For Case I, the optimal state trajectory of (4.1) controlled by (4.2) when α=0.1 and α=0.5 is shown in Figure 2. Similar1y, when T=10, the optimal state trajectory is depicted in Figure 3.
We now consider Case II, where the (terminal) state constraint is generalized by
(x0,x(T))∈F={x0=10,x(T)∈[−10,10]}⊂R2. |
Similar to Case I, the adjoint equation is given by p(t)=−λ. The first transversality condition in (i) implies that ξ1∈R and ξ2=0. From the second transversality condition in (ii), it follows that ∫30p(t)dt=−3λ=ξ1. Hence, we can choose λ=1, ξ1=−3, and ξ2=0. Then analogous to (4.2), the (candidate) optimal solution of Case II is given by ¯u(t)=∫3t−t2(r−t)1−αdr, a.e. t∈[0,3]. Figure 4 depicts the optimal state trajectory (with α=0.1 and α=0.5) when T=3, and Figure 5 shows the case when T=10. Note that the numerical results of this section are obtained by the finite-difference method to approximate the solutions of (4.1) and (4.2).
Remark 4.1. The purpose of the examples in this section is to show an analytic method for applying Theorem 3.1. In the future research problem, a shooting-like method has to be developed to solve (P) numerically, which can be applied to several different complex situations of (P). This requires extending the classical approach (e.g., [11,16]) to the case of Volterra integral equations with singular kernels.
This section provides the proof of Theorem 3.1.
Recall that F is a nonempty closed convex subsets of R2n. Let dF:R2n→R+ be the standard Euclidean distance function to F defined by dF(x):=infy∈F|x−y| for x∈R2n. Note that dF(x)=0 when x∈F. Then it follows from the projection theorem [38, Theorem 2.10] that there exists a unique PF(x)∈F with PF(x):R2n→F⊂R2n, the projection of x∈R2n onto F, such that dF(x)=infy∈F|x−y|=|x−PF(x)|. By [38, Lemma 2.11], PF(x)∈F is the corresponding projection if and only if ⟨x−PF(x),y−PF(x)⟩≤0 for all y∈F, which leads to the characterization of PF(x). In view of [38, Definition 2.37], we have x−PF(x)∈NF(PF(x)) for x∈R2n, where NF(x) is the normal cone to the convex set F at a point x∈F defined by
NF(x):={y∈R2n|⟨y,y′−x⟩≤0,∀y′∈F}. | (5.1) |
Based on the distance function dF, (1.3) can be written by dF(¯x0,¯x(T))=0⇔(¯x0,¯x(T))∈F.
Lemma 5.1. [24, page 167] and [21, Proposition 2.5.4] The function dF(x)2 is Fréchet differentiable on R2n with the Fréchet differentiation of dF(x)2 at x∈R2n given by DdF(x)2(h)=2⟨x−PF(x),h⟩ for h∈R2n.
Recall that the pair (¯x(⋅),¯u(⋅))∈C([0,T];Rn)×Up[0,T] is the optimal pair of (P). Note that the pair (¯x0,¯x(T)) holds the terminal state constraint in (1.3). The optimal cost of (P) under (¯x(⋅),¯u(⋅)) can be written by J(¯x0,¯u(⋅)).
Recall the distance function dF in Section 5.1. For ϵ>0, we define the penalized objective functional as follows:
Jϵ(x0,u(⋅))=(([J(x0,u(⋅))−J(¯x0,¯u(⋅))+ϵ]+)2+dF(x0,x(T))2)12. | (5.2) |
We can easily observe that Jϵ(¯x0,¯u(⋅))=ϵ>0, i.e., (¯x0,¯u(⋅))∈Rn×Up[0,T] is the ϵ-optimal solution of (5.2). We define the Ekeland metric ˆd:(Rn×Up[0,T])×(Rn×Up[0,T])→R+:
ˆd((x0,u(⋅)),(˜x0,˜u(⋅))):=|x0−˜x0|+¯d(u(⋅),˜u(⋅)), | (5.3) |
where
¯d(u(⋅),˜u(⋅)):=|{t∈[0,T]|u(t)≠˜u(t)}|,∀u(⋅),˜u(⋅)∈Up[0,T]. | (5.4) |
Note that (Rn×Up[0,T],ˆd) is a complete metric space, and Jϵ(x0,u) in (5.2) is continuous on (Rn×Up[0,T],ˆd) [33, Proposition 3.10 and Corollary 4.2,Chapter 4].
In view of (5.2)–(5.4), we have
{Jϵ(x0,u(⋅))>0,∀(x0,u(⋅))∈Rn×Up[0,T],Jϵ(¯x0,¯u(⋅))=ϵ≤inf(x0,u(⋅))∈Rn×Up[0,T]Jϵ(x0,u(⋅))+ϵ. | (5.5) |
By the Ekeland variational principle [33, Corollary 2.2,Chapter 4], there exists a pair (xϵ0,uϵ)∈Rn×Up[0,T] such that
ˆd((xϵ0,uϵ(⋅)),(¯x0,¯u(⋅)))≤√ϵ, | (5.6) |
and for any (x0,u(⋅))∈Rn×Up[0,T],
{Jϵ(xϵ0,uϵ(⋅))≤Jϵ(¯x0,¯u(⋅))=ϵ,Jϵ(xϵ0,uϵ(⋅))≤Jϵ(x0,u(⋅))+√ϵˆd((xϵ0,uϵ(⋅)),(x0,u(⋅))). | (5.7) |
We write (xϵ(⋅),uϵ(⋅))∈C([0,T];Rn)×Up[0,T], where xϵ(⋅) is the state trajectory of (1.2) under (xϵ0,uϵ(⋅))∈Rn×Up[0,T].
The next step is to derive the necessary condition for (xϵ0,uϵ(⋅))∈Rn×Up[0,T]. To this end, we employ the spike variation technique, as U does not have any algebraic structure. Hence, standard (convex) variations cannot be used.
For δ∈(0,1), we define Eδ:={E∈[0,T]||E|=δT}, where |E| denotes the Lebesgue measure of E. For Eδ∈Eδ, we introduce the spike variation associated with uϵ:
uϵ,δ(s):={uϵ(s),s∈[0,T]∖Eδ,u(s),s∈Eδ, |
where u(⋅)∈Up[0,T]. Clearly, uϵ,δ(⋅)∈Up[0,T]. By definition of ¯d in (5.4),
¯d(uϵ,δ(⋅),uϵ(⋅))≤|Eδ|=δT. | (5.8) |
We also consider the variation of the initial state given by xϵ0+δa, where a∈Rn. Let xϵ,δ(⋅)∈C([0,T];Rn) be the state trajectory of (1.2) under (xϵ0+δa,uϵ,δ(⋅))∈Rn×Up[0,T]. By (5.7) and (5.8), it follows that
−√ϵ(|a|+T)≤1δ(Jϵ(xϵ0+δa,uϵ,δ(⋅))−Jϵ(xϵ0,uϵ(⋅))). | (5.9) |
We now study variational analysis of (5.9).
Lemma 5.2. The following result holds:
supt∈[0,T]|xϵ,δ(t)−xϵ(t)−δZϵ(t)|=o(δ), |
where Zϵ is the solution to the first variational equation related to the optimal pair (xϵ0,uϵ(⋅))∈Rn×Up[0,T] given by
Zϵ(t)=a+∫t0[fx(t,s,xϵ(s),uϵ(s))(t−s)1−αZϵ(s)ds+ˆf(t,s)(t−s)1−α]ds,a.e.t∈[0,T], |
with
ˆf(t,s):=f(t,s,xϵ(s),u(s))−f(t,s,xϵ(s),uϵ(s)). |
Proof. For δ∈(0,1), let Zϵ,δ(t):=xϵ,δ(t)−xϵ(t)δ, a.e. t∈[0,T], where based on the Taylor expansion, Zϵ,δ can be written as (note that fϵ,δx(t,s):=∫10fx(t,s,xϵ(s)+r(xϵ,δ(s)−xϵ(s)),uϵ,δ(s))dr)
Zϵ,δ(t)=a+∫t0(fϵ,δx(t,s)(t−s)1−αZϵ,δ(s)+1Eδ(s)δˆf(t,s)(t−s)1−α)ds,a.e.t∈[0,T]. |
Then we complete the proof by showing that limδ↓0supt∈[0,T]|Zϵ,δ(t)−Zϵ(t)|=0.
By Assumption 1, we have |ˆf(t,s)|≤4K+4K|xϵ(s)|+4Kρ(uϵ(s),u(s))=:˜ψ(s) and |fϵ,δx(t,s)|≤K. Based on Assumption 1, we can show that
|xϵ,δ(t)−xϵ(t)|≤b(t)+∫t0C(t−s)1−α|xϵ,δ(s)−xϵ(s)|ds, | (5.10) |
where b(t)=|δa|+∫t01Eδ(s)˜ψ(s)(t−s)1−αds. By [34, Lemma 2.3], it follows that
supt∈[0,T]|b(t)|≤|δa|+|∫t01Eδ(s)˜ψ(s)(t−s)1−αds|≤C(|δa|+|Eδ|). |
We apply the Gronwall's inequality in [34, Lemma 2.4] to get
supt∈[0,T]|xϵ,δ(t)−xϵ(t)|≤C(|δa|+|Eδ|)→0,as δ↓0 due to (5.8). | (5.11) |
From Lemma 2.1, Zϵ is a well-defined linear Volterra integral equation. Then with ˆb(t):=|a|+∫t0˜ψ(s)(t−s)1−αds and using a similar approach, we can show that
supt∈[0,T]|Zϵ(t)|≤C(|a|+‖˜ψ(⋅)‖Lp([0,T];R)). | (5.12) |
We obtain
Zϵ,δ(t)−Zϵ(t)=∫t0fϵ,δx(t,s)(t−s)1−α[Zϵ,δ(s)−Zϵ(s)]ds+∫t0(1Eδ(s)δ−1)ˆf(t,s)(t−s)1−αds+∫t0fϵ,δx(t,s)−fx(t,s,xϵ(s),uϵ(s))(t−s)1−αZϵ(s)ds. | (5.13) |
Notice that by Assumption 1, together with (5.11) and (5.12), it follows that as δ↓0,
sup(t,s)∈Δ|(fϵ,δx(t,s)−fx(t,s,xϵ(s),uϵ(s)))Zϵ(s)|≤C(|δa|+|Eδ|)→0. | (5.14) |
For convenience, let
b(1,1)(t):=∫t0|fϵ,δx(t,s)−fx(t,s,xϵ(s),uϵ(s))(t−s)1−αZϵ(s)|ds |
and
b(1,2)(t):=|∫t0(1Eδ(s)δ−1)ˆf(t,s)(t−s)1−αds|. |
By (5.14), limδ↓0supt∈[0,T]b(1,1)(t)=0. In addition, by invoking [34, Lemma 5.3.2], it follows that supt∈[0,T]b(1,2)(t)≤δ.. Then from (5.13), we have
|Zϵ,δ(t)−Zϵ(t)|≤b(1,1)(t)+δ+∫t0Zϵ,δ(s)−Zϵ(s)(t−s)1−αds. |
By applying [34, Lemma 2.4], it follows that
supt∈[0,T]|Zϵ,δ(t)−Zϵ(t)|≤C(supt∈[0,T]b(1,1)(t)+δ). |
Hence, we have
limδ↓0supt∈[0,T]|Zϵ,δ(t)−Zϵ(t)|=0. |
This completes the proof.
Based on the Taylor expansion,
1δ(J(xϵ0+δa,uϵ,δ(⋅))−J(xϵ0,uϵ(⋅)))=∫T0lϵ,δx(s)Zϵ,δ(s)ds+∫T01Eδδˆl(s)ds+hϵ,δx0(T)a+hϵ,δx(T)Zϵ,δ(T), |
where
ˆl(s):=l(s,xϵ(s),u(s))−l(s,xϵ(s),uϵ(s)), |
lϵ,δx(s):=∫10lx(s,xϵ(s)+r(xϵ,δ(s)−xϵ(s)),uϵ,δ(s))dr, |
hϵ,δx0(T):=∫10hx0(xϵ0+rδa,xϵ(T)+r(xϵ,δ(T)−xϵ(T)))dr, |
and
hϵ,δx(T):=∫10hx(xϵ0+rδa,xϵ(T)+r(xϵ,δ(T)−xϵ(T)))dr. |
Let us define
ˆZϵ(T)=∫T0lx(s,xϵ(s),uϵ(s))Zϵ(s)ds+∫T0ˆl(s)ds+hx0(xϵ0,xϵ(T))a+hx(xϵ0,xϵ(T))Zϵ(T). |
By Lemma 2.1, ˆZϵ is a well-defined Volterra integral equation. It then follows that
1δ(J(xϵ0+δa,uϵ,δ(⋅))−J(xϵ0,uϵ(⋅)))−ˆZϵ(T)=∫T0lϵ,δx(s)[Zϵ,δ(s)−Zϵ(s)]ds+∫T0[lϵ,δx(s)−lx(s,xϵ(s),uϵ(s))]Zϵ(s)ds+∫T0(1Eδδ−1)ˆl(s)ds+[hϵ,δx0(T)−hx0(xϵ0,xϵ(T))]a+hϵ,δx(T)[Zϵ,δ(T)−Zϵ(T)]+[hϵ,δx(T)−hx(xϵ0,xϵ(T))]Zϵ(T). |
Notice that limδ↓0supt∈[0,T]|Zϵ,δ(t)−Zϵ(t)|=0 by Lemma 5.2. By [33, Corollary 3.9,Chapter 4], it follows that supt∈[0,T]|∫t0(1δ1Eδ(s)−1)ˆl(s)ds|≤δ. Hence, similar to Lemma 5.2, we have
limδ↓0|1δ(J(xϵ0+δa,uϵ,δ)−J(xϵ0,uϵ))−ˆZϵ(T)|=0. | (5.15) |
From (5.9), we have
−√ϵ(|a|+T)≤1Jϵ(xϵ0+δa,uϵ,δ(⋅))+Jϵ(xϵ0,uϵ(⋅))×1δ(([J(xϵ0+δa,uϵ,δ(⋅))−J(¯x0,¯u(⋅))+ϵ]+)2−([J(xϵ0,uϵ(⋅))−J(¯x0,¯u(⋅))+ϵ]+)2+dF(xϵ0+δa,xϵ,δ(T))2−dF(xϵ0,xϵ(T))2). | (5.16) |
Let
λϵ:=[J(xϵ0,uϵ(⋅))−J(¯x0,¯u(⋅))+ϵ]+Jϵ(xϵ0,uϵ(⋅))≥0, | (5.17) |
For ξϵ∈R2n (ξϵ1∈Rn and ξϵ2∈Rn), let
ξϵ:=[ξϵ1ξϵ2]:={[xϵ0xϵ(T)]−PF(xϵ0,xϵ(T))Jϵ(xϵ0,uϵ(⋅))∈NF(PF(xϵ0,xϵ(T))),(xϵ0,xϵ(T))∉F,0∈NF(PF(xϵ0,xϵ(T))),(xϵ0,xϵ(T))∈F. | (5.18) |
Then from (5.15), (5.17), and (5.18), as δ↓0, (5.16) becomes
−√ϵ(|a|+T)≤λϵˆZϵ(T)+⟨ξϵ1,a⟩+⟨ξϵ2,Zϵ(T)⟩, | (5.19) |
where by (5.2), (5.17), and (5.18) (see also the discussion in Section 5.1),
|λϵ|2+|ξϵ|2=1. | (5.20) |
Lemma 5.3. For any (a,u(⋅))∈Rn×Up[0,T], the following results hold:
(i) limϵ↓0{|xϵ0−¯x0|+¯d(uϵ(⋅),¯u(⋅))}=0;
(ii) supt∈[0,T]|Zϵ(t)−Z(t)|=o(1),|ˆZϵ(T)−ˆZ(T)|=o(1), where
Z(t)=a+∫t0[fx(t,s,¯x(s),¯u(s))(t−s)1−αZ(s)ds+˜f(t,s)(t−s)1−α]ds,ˆZ(T)=∫T0[lx(s,¯x(s),¯u(s))Z(s)+˜l(s)]ds+¯hx0a+¯hxZ(T), |
with ˜f(t,s):=f(t,s,¯x(s),u(s))−f(t,s,¯x(s),¯u(s)), ˜l(s):=l(s,¯x(s),u(s))−l(s,¯x(s),¯u(s)), ¯hx0:=hx0(¯x0,¯x(T)), and ¯hx:=hx(¯x0,¯x(T)).
Proof. Part (i) is due to (5.6). The proof of (ii) is similar to Lemma 5.2.
We consider the limit of ϵ↓0. Instead of taking the limit with respect to ϵ↓0, let {ϵk} be the sequence of ϵ such that ϵk≥0 and ϵk↓0 as k→∞. We replace ϵ with ϵk. Then by (5.20), the sequences ({λϵk},{ξϵk}) are bounded for k≥0. From the standard compactness argument, we may extract a subsequence of {ϵk}, still denoted by {ϵk}, such that
({λϵk},{ξϵk})→(λ0,ξ0)=:(λ,ξ),as k→∞. | (5.21) |
By (5.17) and the property of the limiting normal cones [41, page 43],
λ≥0,ξ∈NF(PF(¯x0,¯x(T)))=NF(¯x0,¯x(T)). | (5.22) |
From (5.21) and (5.20), together with Lemma 5.3, as k→∞, it follows that
λϵkˆZϵk(T)≤λˆZ(T)+|ˆZϵk(T)−ˆZ(T)|+|λϵk−λ|ˆZ(T)→λˆZ(T),⟨ξϵk1,a⟩=⟨ξ1,a⟩+⟨ξϵk1,a⟩−⟨ξ1,a⟩→⟨ξ1,a⟩,⟨ξϵk2,Zϵk(T)⟩≤⟨ξ2,Z(T)⟩+|Zϵk(T)−Z(T)|+|ξϵk2−ξ2||Z(T)|→⟨ξ2,Z(T)⟩. |
Therefore, as k→∞, (5.19) becomes for any (a,u)∈Rn×Up[0,T],
0≤λˆZ(T)+⟨ξ1,a⟩+⟨ξ2,Z(T)⟩. | (5.23) |
When λ>0, the nontriviality condition in Theorem 3.1 holds. When λ=0, from (5.20)–(5.22), we must have ξ∈NF(¯x0,¯x(T)) and |ξϵk|→|ξ|=1. Hence, we have (λ,ξ)≠0, i.e., they cannot be zero simultaneously. This proves the nontriviality condition in Theorem 3.1.
Then by using the variational equations in Lemma 5.3, (5.23) becomes
0≤⟨ξ1+λ¯h⊤x0,a⟩+⟨ξ2+λ¯h⊤x,a⟩+∫T0λ˜l(s)ds+∫T0(λ¯hx+ξ⊤2)1[0,T)(s)fx(T,s,¯x(s),¯u(s))(T−s)1−αZ(s)ds+∫T0[λlx(s,¯x(s),¯u(s))Z(s)+(λ¯hx+ξ⊤2)1[0,T)(s)˜f(T,s)(T−s)1−α]ds. | (5.24) |
With the adjoint equation p(⋅)∈Lp([0,T];Rn) in Theorem 3.1, (5.24) becomes
0≤⟨ξ1+λ¯h⊤x0,a⟩+⟨ξ2+λ¯h⊤x,a⟩+∫T0λ˜l(s)ds+∫T0[−p(s)+∫Tsfx(r,s,¯x(s),¯u(s))⊤(r−s)1−αp(r)dr]⊤Z(s)ds+∫T0(λ¯hx+ξ⊤2)1[0,T)(s)˜f(T,s)(T−s)1−αds. | (5.25) |
In (5.25), the standard Fubini's formula and Lemma 5.3 lead to
∫T0[−p(s)+∫Tsfx(r,s,¯x(s),¯u(s))⊤(r−s)1−αp(r)dr]⊤Z(s)ds=∫T0−p(s)⊤[Z(s)−∫s0fx(s,r,¯x(r),¯u(r))(s−r)1−αZ(r)dr]ds=∫T0−p(s)⊤[a+∫s0˜f(s,r)(s−r)1−αdr]ds. |
Moreover, by definition of NF in (5.1), it follows that for any y=(y1,y2)∈F,
⟨ξ1,a⟩+⟨ξ2,a⟩≤⟨ξ1,¯x0−y1+a⟩+⟨ξ2,¯x(T)−y2+a⟩. |
Hence, (5.25) becomes for any (a,u)∈Rn×Up[0,T] and y=(y1,y2)∈F,
0≤⟨ξ1,¯x0−y1+a⟩+⟨ξ2,¯x(T)−y2+a⟩+λ¯hx0a+λ¯hxa−⟨∫T0p(s)ds,a⟩+∫T0−p(s)⊤∫s0˜f(s,r)(s−r)1−αdrds+∫T0(λ¯hx+ξ⊤2)1[0,T)(s)˜f(T,s)(T−s)1−αds+∫T0λ˜l(s)ds. | (5.26) |
We use (5.26) to prove the transversality conditions and the Hamiltonian-like maximum condition.
In (5.26), when u=¯u, for any y=(y1,y2)∈F, we have
0≤⟨ξ1,¯x0−y1+a⟩+⟨ξ2,¯x(T)−y2+a⟩+λ¯hx0a+λ¯hxa−⟨∫T0p(s)ds,a⟩. |
When (y1,y2)=(¯x0,¯x(T)), the above inequality holds for any a,−a∈Rn, which implies
∫T0p(s)ds=ξ1+ξ2+λ¯h⊤x0+λ¯h⊤x. | (5.27) |
Under (5.27), the above inequality becomes 0≤⟨ξ1,¯x0−y1⟩+⟨ξ2,¯x(T)−y2⟩ for any y=(y1,y2)∈F. This, together with (5.27), proves the transversality conditions in Theorem 3.1. In addition, as p(⋅)∈Lp([0,T];Rn), the nontriviality condition implies the nontriviality of the adjoint equation in Theorem 3.1.
We prove the Hamiltonian-like maximum condition in Theorem 3.1. Define
Λ(s,¯x(s),u):=−λl(s,¯x(s),u)+∫Tsp(r)⊤f(r,s,¯x(s),u)(r−s)1−αdr−1[0,T)(s)(λ¯hx+ξ⊤2)f(T,s,¯x(s),u)(T−s)1−α. |
When (y1,y2)=(¯x0,¯x(T)) and a=0 in (5.26), by the Fubini's formula, (5.26) can be written as
∫T0Λ(s,¯x(s),u(s))ds≤∫T0Λ(s,¯x(s),¯u(s))ds. | (5.28) |
As U is separable, there exists a countable dense set U0={ui,i≥1}⊂U. Moreover, there exists a measurable set Si⊂[0,T] such that |Si|=T and any t∈Si is the Lebesgue point of Λ(t,¯x(t),u(t)), i.e., it holds that limτ↓012τ∫t+τt−τΛ(s,¯x(s),u(s))ds=Λ(t,¯x(t),u(t)) [10, Theorem 5.6.2]. We fix ui∈U0. For any t∈Si, define
u(s):={¯u(s),s∈[0,T]∖(t−τ,t+τ),ui,s∈(t−τ,t+τ). |
Then (5.28) becomes
0≤limτ↓012τ∫t+τt−τ[Λ(s,¯x(s),¯u(s))−Λ(s,¯x(s),ui)]ds=Λ(t,¯x(t),¯u(t))−Λ(t,¯x(t),ui). |
This is implies that
Λ(t,¯x(t),ui)≤Λ(t,¯x(t),¯u(t)),∀ui∈U0,t∈∩i≥1Si. | (5.29) |
Since ∩i≥1Si=[0,T] by the fact that U0 is countable, Λ is continuous in u∈U, and U is separable, (5.29) implies the Hamiltonian-like maximum condition in Theorem 3.1. This completes the proof.
In this paper, we have studied the Pontryagin maximum principle for the optimal control problem of Volterra integral equations with singular kernels. The main technical difference in the proof compared with the existing literature is the variational and duality analysis. In fact, the variational analysis in our paper needs to handle the singular kernel, and the duality analysis requires to characterize the integral-type adjoint equation with the singular kernel to get the desired Hamiltonian-like maximization condition.
There are several important potential future research problems. One {direction} is to study (P) with additional generalized state constraints including the running state constraint. Another {direction} is to study the numerical aspects of solving (P) such as the shooting method and the Lagrangian collocation approach. Finally, one can extend (P) to the infinite-dimensional problem, which can be applied to studying optimal control of integral-type partial differential equations and integral-type systems with delays and singular kernels.
The authors declare they have not used Artificial Intelligence (AI) tools in the creation of this article.
This research was supported in part by the Korea Institute of Energy Technology Evaluation and Planning (KETEP) and the Ministry of Trade, Industry & Energy (MOTIE) of the Republic of Korea (RS-2023-00235742), in part by the National Research Foundation of Korea (NRF) Grant funded by the Ministry of Science and ICT, South Korea (NRF-2021R1A2C2094350) and in part by Institute of Information & communications Technology Planning & Evaluation (IITP) grant funded by the Korea government (MSIT) (No. 2020-0-01373, Artificial Intelligence Graduate School Program (Hanyang University)).
The author declares no conflict of interest in this paper.
[1] | Goudos SK, Kalialakis C, Mittra R (2016) Evolutionary algorithms applied to antennas and propagation: A review of state of the art. International Journal of Antennas and Propagation. |
[2] |
Hoorfar A (2007) Evolutionary programming in electromagnetic optimization: a review. IEEE T Antenn Propag 55: 523-537. doi: 10.1109/TAP.2007.891306
![]() |
[3] |
Rahmat-Samii Y, Kovitz JM, Rajagopalan H (2012) Nature-inspired optimization techniques in communication antenna designs. Proceedings of the IEEE 100: 2132-2144. doi: 10.1109/JPROC.2012.2188489
![]() |
[4] | Johnson JM, Rahmat-Samii Y (1997) Genetic algorithms in engineering electromagnetic. IEEE Antenn Propag M 39: 7-21. |
[5] |
Weile DS, Michielssen E (1997) Genetic algorithm optimization applied to electromagnetics: A review. IEEE T Antenn Propag 45: 343-353. doi: 10.1109/8.558650
![]() |
[6] | Haupt RL, Werner DH (2007) Genetic algorithms in electromagnetic. John Wiley & Sons. |
[7] |
Robinson J, Rahmat-Samii Y (2004) Particle swarm optimization in electromagnetic. IEEE T Antenn Propag 52: 397-407. doi: 10.1109/TAP.2004.823969
![]() |
[8] |
Karimkashi S, Kishk AA (2010) Invasive weed optimization and its features in electromagnetic. IEEE T Antenn Propag 58: 1269-1278. doi: 10.1109/TAP.2010.2041163
![]() |
[9] |
Rocca P, Oliveri G, Massa A (2011) Differential evolution as applied to electromagnetic. IEEE Antenn Propag M 53: 38-49. doi: 10.1109/MAP.2011.5773566
![]() |
[10] |
Villegas FJ, Cwik T, Rahmat-Samii Y, et al. (2004) A parallel electromagnetic genetic-algorithm optimization (EGO) application for patch antenna design. IEEE T Antenn Propag 52: 2424-2435. doi: 10.1109/TAP.2004.834071
![]() |
[11] | Jayasinghe JM, Uduwawala D (2015) A Novel Multiband Miniature Planar Inverted F Antenna Design for Bluetooth and WLAN Applications. Int J Antenn Propag. |
[12] | Sun SY (2009) Design of broadband microstrip antenna utilizing genetic algorithm. In International Symposium on Electromagnetic Compatibility. |
[13] | John M, Ammann M (2006) Design of a wide-band printed antenna using a genetic algorithm on an array of overlapping sub-patches. IEEE Int Workshop on Antenna Technol.: Small Antennas and Novel Metamaterials (IWAT2006). |
[14] | Mishra RG, Mishra R, Kuchhal P, et al. (2017) Optimization and analysis of high gain wideband microstrip patch antenna using genetic algorithm. International Journal of Engineering & Technology 7: 176-179. |
[15] | Jayasinghe JW (2014) Optimization of the performance of microstrip patch antennas and arrays by using genetic algorithms. Ph.D. Thesis, University of Peradeniya. |
[16] |
Soontornpipit P, Furse CM, Chung YC (2005) Miniaturized biocompatible microstrip antenna using genetic algorithm. IEEE T Antenn Propag 53: 1939-1945. doi: 10.1109/TAP.2005.848461
![]() |
[17] | Heidari AA, Dadgarnia A (2011) Design and optimization of a circularly polarized microstrip antenna for GPS applications using ANFIS and GA. General Assembly and Scientific Symposium, XXXth URSI, 1-4. IEEE. |
[18] | Gupta R, Gurjar S, Kumar A (2013) Using genetic algorithms reduction of rectangular microstrip patches. International Journal of Advanced Research in Computer and Communication Engineering 2: 1643-1646. |
[19] |
Jayasinghe JW, Anguera J, Uduwawala DN (2012) A simple design of multi band microstrip patch antennas robust to fabrication tolerances for GSM, UMTS, LTE, and Bluetooth applications by using genetic algorithm optimization. Progress In Electromagnetics Research M 27: 255-269. doi: 10.2528/PIERM12102705
![]() |
[20] | Jayasinghe JM, Anguera J, Uduwawala DN, et al. (2015) Nonuniform Overlapping Method in Designing Microstrip Patch Antennas Using Genetic Algorithm Optimization. Int J Antenn Propag. |
[21] |
Choo H, Hutani A, Trintinalia LC, et al. (2000) Shape optimisation of broadband microstrip antennas using genetic algorithm. Electron Lett 36: 2057-2058. doi: 10.1049/el:20001452
![]() |
[22] |
Griffiths LA, Furse C, Chung YC (2006) Broadband and multiband antenna design using the genetic algorithm to create amorphous shapes using ellipses. IEEE T Antenn Propag 54: 2776-2782. doi: 10.1109/TAP.2006.882154
![]() |
[23] | Polivka M, Drahovzal M, Rohan J, et al. (2006) Multiband patch antenna with perturbation elements generated by genetic algorithm. In 2006 First European Conference on Antennas and Propagation, 1-4. |
[24] | Xiao S, Wang BZ, Yang XS, et al. (2003) Reconfigurable microstrip antenna design based on genetic algorithm. IEEE Antennas and Propagatopm Society International Symposium 1: 407-410. |
[25] | Wyant A, Venkataraman J (2008) Optimization of Reduced Size Microstrip Patch with slots using a Genetic Algorithm. Dept. of Electrical Engineering, Rochester Institute of Technology. |
[26] |
Alatan L, Aksun MI, Leblebicioglu K, et al. (1999) Use of computationally efficient method of moments in the optimization of printed antennas. IEEE T Antenn Propag 47: 725-732. doi: 10.1109/8.768813
![]() |
[27] |
Kerkhoff AJ, Rogers RL, Ling H (2004) Design and analysis of planar monopole antennas using a genetic algorithm approach. IEEE T Antenn Propag 52: 2709-2718. doi: 10.1109/TAP.2004.834429
![]() |
[28] | Koziel S, Jayasinghe JW, Saraereh O, et al. (2017) Local Optimization of a Sierpinski Carpet Fractal Antenna, IEEE 12th International Conference on Industrial and Information Systems, 1-5. |
[29] | Behera BR (2017) Sierpinski Bow-Tie antenna with genetic algorithm. Engineering Science and Technology 20: 775-782. |
[30] | Adelpour Z, Mohajeri F, Sadeghi M (2010) Dual-frequency microstrip patch antenna with modified Koch fractal geometry based on genetic algorithm. 2010 Loughborough Antennas and Propagation Conference (LAPC), 401-404. |
[31] | Spence TG, Werner DH (2004) Genetically optimized fractile microstrip patch antennas. In IEEE Antennas and Propagation Society International Symposium 4: 4424-4427. |
[32] |
Ozgun O, Mutlu S, Aksun MI, et al. (2003) Design of dual-frequency probe-fed microstrip antennas with genetic optimization algorithm. IEEE T Antenn Propag 51: 1947-1954. doi: 10.1109/TAP.2003.814732
![]() |
[33] |
Namkung J, Hines EL, Green RJ, et al. (2007) Probe‐fed microstrip antenna feed point optimization using a genetic algorithm and the method of moments. Microw Opt Techn Lett 49: 325-329. doi: 10.1002/mop.22120
![]() |
[34] | Lu Q, Edward K, Sean D, et al. (2009) Optimum design of a probe fed dual frequency patch antenna using genetic algorithm. Proceedings of the ARMMS RF and Microwave Society. |
[35] |
Raychowdhury A, Gupta B, Bhattacharjee R (2000) Bandwidth improvement of microstrip antennas through a genetic‐algorithm‐based design of a feed network. Microw Opt Techn Lett 27: 273-275. doi: 10.1002/1098-2760(20001120)27:4<273::AID-MOP17>3.0.CO;2-8
![]() |
[36] | Chattoraj N, Roy GS (2006) Application of Genetic Algorithm to the Optimization of Microstrip Antennas with and without Superstrate. Mikrotalasna revija, 32-35. |
[37] |
Aljibouri B, Lim EG, Evans H, et al. (2000) Multiobjective genetic algorithm approach for a dual-feed circular polarised patch antenna design. Electron Lett 36: 1005-1006. doi: 10.1049/el:20000766
![]() |
[38] |
Raychowdhury A, Gupta B, Bhattacharjee R (2000) Bandwidth improvement of microstrip antennas through a genetic‐algorithm‐based design of a feed network. Microw Opt Techn Lett 27: 273-275. doi: 10.1002/1098-2760(20001120)27:4<273::AID-MOP17>3.0.CO;2-8
![]() |
[39] |
Mukherjee P, Gupta B (2005) Genetic algorithm-based design optimisation of aperture-coupled rectangular microstrip antenna. Defence Sci J 55: 487. doi: 10.14429/dsj.55.2011
![]() |
[40] |
Ding M, Jin R, Geng J (2007) Optimal design of ultra wideband antennas using a mixed model of 2‐D genetic algorithm and finite‐difference time‐domain. Microw Opt Techn Lett 49: 3177-3180. doi: 10.1002/mop.22928
![]() |
[41] |
Silva CRM, Lins HWC, Martins SR, et al. (2012) A multiobjective optimization of a UWB antenna using a self organizing genetic algorithm. Microw opt Tech let 54: 1824-1828. doi: 10.1002/mop.26945
![]() |
[42] |
Kim J, Yoon T, Kim J, et al. (2005) Design of an ultra wide-band printed monopole antenna using FDTD and genetic algorithm. IEEE Microw Wirel Co 15: 395-397. doi: 10.1109/LMWC.2005.850468
![]() |
[43] | Pourahmadazar J, Shirzad H, Ghobadi C, et al. (2010) Using a MAM and genetic algorithm to optimize UWB microstrip monopole antenna with FEM and HFSS. 5th International Symposium on Telecommunications (IST), 115-119. |
[44] |
John M, Ammann MJ (2007) Wideband printed monopole design using a genetic algorithm. IEEE Antenn Wirel Pr 6: 447-449. doi: 10.1109/LAWP.2007.891962
![]() |
[45] | Sun S, Yinghua LV, Zhang J (2010) The application of genetic algorithm optimization in broadband microstrip antenna design. Antennas and Propagation Society International Symposium (APSURSI), 1-4. |
[46] | Michael K, Kucharski AA (2006) Genetic algorithm optimization for broadband patch antenna design. International Conf. on Microwaves, Radar & Wireless Communications, 748-751. |
[47] | Sun S, Yinghua LV, Zhang J (2010) The application of genetic algorithm optimization in broadband microstrip antenna design. Antennas and Propagation Society International Symposium (APSURSI), 1-4. |
[48] |
Deshmukh AA, Kumar G (2005) Compact broadband U‐slot‐loaded rectangular microstrip antennas. Microw Opt Techn Lett 46: 556-559. doi: 10.1002/mop.21049
![]() |
[49] |
Johnson JM, Rahmat-Samii Y (1999) Genetic algorithms and method of moments (GA/MOM) for the design of integrated antennas. IEEE T Antenn Propag 47: 1606-1614. doi: 10.1109/8.805906
![]() |
[50] | Noor AOA (2015) An Automated Optimization Technique of a MSPA for Broadband Communication. Eng Tech J 33. |
[51] | Lech M, Mitchell A, Waterhouse R (2000) Optimization of broadband microstrip patch antennas. Asia-Pacific Microwave Conference, 711-714. |
[52] |
Lotfi AA, Kashani FH (2004) Bandwidth optimization of the E-shaped microstrip antenna using the genetic algorithm based on fuzzy decision making. Antennas and Propagation Society International Symposium 3: 2333-2336. doi: 10.1109/APS.2004.1331839
![]() |
[53] | Bulla G, de Salles AA, Vuong TP (2010) PIFA bandwidth optimization using genetic algorithm and capacitive feeding. IEEE International Conference on Wireless Information Technology and Systems (ICWITS), 1-4. |
[54] |
Nguyen TD, Duroc Y, Vuong TP (2011) Genetic algorithm for optimization of L-shaped PIFA antennas. Int J Microw Wirel T 3: 691-699. doi: 10.1017/S1759078711000985
![]() |
[55] | Chandran PP, Viswasom S (2014) Gain and bandwidth optimization of a novel microstrip patch antenna. Fourth International Conference on Advances in Computing and Communications (ICACC), 315-318. |
[56] |
Tseng LY, Han TY (2010) An evolutionary design method using genetic local search algorithm to obtain broad/dual-band characteristics for circular polarization slot antennas. IEEE T Antenn Propag 58: 1449-1456. doi: 10.1109/TAP.2010.2044312
![]() |
[57] |
Spence TG, Werner DH, Groff RD (2004) Genetic algorithm optimization of some novel broadband and multiband microstrip antennas. Antennas and Propagation Society International Symposium 4: 4408-4411. doi: 10.1109/APS.2004.1330329
![]() |
[58] |
Jayasinghe JW, Anguera J, Uduwawala DN, et al. (2017) A multipurpose genetically engineered microstrip patch antennas: Bandwidth, gain, and polarization. Microw Opt Techn Lett 59: 941-949. doi: 10.1002/mop.30439
![]() |
[59] |
Choo H, Ling H (2003) Design of broadband and dual-band microstrip antennas on a high-dielectric substrate using a genetic algorithm. IEE Proceedings-Microwaves, Antennas and Propagation 150: 137-142. doi: 10.1049/ip-map:20030291
![]() |
[60] | Fertas K, Kimouche H, Challal M, et al. (2015) Design and optimization of a CPW-fed tri-band patch antenna using genetic algorithms. ACES Journal 30: 754-759. |
[61] | Wakrim L, Ibnyaich S, Hassani M (2016) Novel Miniaturized Multiband and Wideband PIFA Antenna for Wireless Applications. 3rd International Conference on Automation, Control, Engineering and Computer Science, 3-6. |
[62] |
Choo H, Ling H ((2002) Design of multiband microstrip antennas using a genetic algorithm. IEEE Microw Wirel Co 12: 345-347. doi: 10.1109/LMWC.2002.803144
![]() |
[63] |
Ohira M, Deguchi H, Tsuji M, et al. (2004) Multiband single-layer frequency selective surface designed by combination of genetic algorithm and geometry-refinement technique. IEEE T Antenn Propag 52: 2925-2931. doi: 10.1109/TAP.2004.835289
![]() |
[64] |
Sathi V, Taherizadeh S, Lotfi A, et al. (2010) Optimisation of multi-frequency microstrip antenna using genetic algorithm coupled with method of moments. IET Microw Antennas P 4: 477-483. doi: 10.1049/iet-map.2009.0020
![]() |
[65] | Michael K, Kucharski AA (2006) Genetic algorithm optimization for Multiband patch antenna design. First European Conference on Antennas and Propagation, 1-4. |
[66] |
Werner DH, Werner PL, Church KH (2001) Genetically engineered multiband fractal antennas. Electron Lett 37: 1150-1151. doi: 10.1049/el:20010802
![]() |
[67] |
Ozgun O, Mutlu S, Aksun ML, et al. (2003) Design of dual-frequency probe-fed microstrip antennas with genetic optimization algorithm. IEEE T Antenn Propag 51: 1947-1954. doi: 10.1109/TAP.2003.814732
![]() |
[68] | Griffiths L, Chung YC, Furse C (2005) Integrated dual band GSM microstrip monopole using GA and FDTD. Antennas and Propagation Society International Symposium 4: 48-51. |
[69] | Lu Q, Korolkiewicz E, Danaher S, et al. (2009) Optimum design of a probe fed dual frequency patch antenna using genetic algorithm. |
[70] |
Villegas FJ, Cwik T, Rahmat-Samii Y, et al. (2002) Parallel genetic-algorithm optimization of a dual-band patch antenna for wireless communications. IEEE Antennas and Propagation Society International Symposium 1: 334-337. doi: 10.1109/APS.2002.1016316
![]() |
[71] | Castellana F, Bilotti F, Vegni L (2001) Automated dual band patch antenna design by a genetic algorithm based numerical code. IEEE Antennas and Propagation Society International Symposium 4: 696-699. |
[72] | Fertas K, Kimouche H, Challal M, et al. (2015) An optimized shaped antenna for multiband applications using Genetic Algorithm. 4th International Conference on Electrical Engineering (ICEE), 1-4. |
[73] |
Saxena NK, Khan MA, Pourush PKS, et al. (2011) GA optimization of cutoff frequency of magnetically biased microstrip circular patch antenna. AEU-Int J Electron C 65: 476-479. doi: 10.1016/j.aeue.2010.04.008
![]() |
[74] |
Herscovici N, Osorio MF, Peixeiro C (2002) Miniaturization of rectangular microstrip patches using genetic algorithms. IEEE Antenn Wirel Pr 1: 94-97. doi: 10.1109/LAWP.2002.805128
![]() |
[75] |
Lamsalli M, El Hamichi A, Boussouis M, et al. (2016) Genetic algorithm optimization for microstrip patch antenna miniaturization. Prog Electrom Res LE 60: 113-120. doi: 10.2528/PIERL16041907
![]() |
[76] |
Gupta VR, Gupta N (2006) Realization of a compact microstrip antenna: An optimization approach. Int J RF and Microw C E 16: 367-373. doi: 10.1002/mmce.20157
![]() |
[77] | Chattoraj N, Roy JS (2007) Application of Genetic Algorithm to the Optimization of Gain of Magnetized Ferrite Microstrip Antenna. Eng let 14. |
[78] |
Kim D, Ju J, Choi J (2012) A mobile communication base station antenna using a genetic algorithm based Fabry-Perot resonance optimization. IEEE T Antenn Propag 60: 1053-1058. doi: 10.1109/TAP.2011.2173108
![]() |
[79] |
Jayasinghe JW, Anguera J, Uduwawala DN (2013) A high-directivity microstrip patch antenna design by using genetic algorithm optimization. Progress In Electromagnetics Research C 37: 131-144. doi: 10.2528/PIERC13010805
![]() |
[80] | Chattoraj N, Roy JS (2006) The optimization of gain of patch antennas using Genetic Algorithm. ACTA TECHNICA CSAV 51: 279. |
[81] |
Zhu X, Shao W, Li JL, et al. (2012) Design and optimization of low RCS patch antennas based on a genetic algorithm. Progress In Electromagnetics Research 122: 327-339. doi: 10.2528/PIER11100703
![]() |
[82] | Mittra R (1997) Genetic algorithm: the last word for solving all of your design problems. Antennas and Propagation Society International Symposium 3: 1672-1675. |
[83] | Lu Q, Edward K, Sean D, et al. (2010) Optimum design of a probe fed dual frequency patch antenna using genetic algorithm. ARMMS RF and Microwave Society, 20-21. |
[84] |
Kim Y, Yun W, Yoon Y (1999) Dual-frequency and dual-polarisation wideband microstrip antenna. Electron Lett 35: 1399-1400. doi: 10.1049/el:19990995
![]() |
[85] |
Jayasinghe J, Anguera J, Uduwawala D (2015) On the behavior of several fitness functions for genetically optimized microstrip antennas. Int J Sci World 3: 53-58. doi: 10.14419/ijsw.v3i1.4132
![]() |
[86] |
Lee D, Lee S (2000) Design of a coaxially fed circularly polarized rectangular microstrip antenna using a genetic algorithm. Microw Opt Techn Lett 26: 288-291. doi: 10.1002/1098-2760(20000905)26:5<288::AID-MOP4>3.0.CO;2-U
![]() |
[87] | See CH, Abd-Alhameed RA, Zhou D, et al. (2006) A new design of circularly-polarised conical-beam microstrip patch antennas using a genetic algorithm. First European Conference on Antennas and Propagation, 1-4. |
[88] |
Zentner R, Sipus Z, Bartolic J (2001) Optimization synthesis of broadband circularly polarized microstrip antennas by hybrid genetic algorithm. Microw Opt Tech Lett 31: 197-201. doi: 10.1002/mop.1397
![]() |
[89] | Grimaccia F, Mussetta M, Pirinoli P, et al. (2006) Genetical swarm optimization (GSO): A class of population-based algorithms for antenna design. ICCE'06 First International Conference on Communications and Electronics, 467-471. |
[90] |
Günel T, Erer I (2002) Application of fuzzy genetic algorithm to the problem of synthesizing circular microstrip antenna elements with thick substrates. AEU-Int J Electron C 56: 215-217. doi: 10.1078/1434-8411-54100099
![]() |
[91] |
Zhang YJ, Gong SX, Wang X, et al. (2010) A hybrid genetic-algorithm space-mapping method for the optimization of broadband aperture-coupled asymmetrical U-shaped slot antennas. J Electromagnet Waves 24: 2139-2153. doi: 10.1163/156939310793699118
![]() |
[92] |
Pissoort D, Rogier H, Olyslager F, et al. (2003) Optimization of a microstrip antenna with a genetic algorithm for use as a ground penetrating radar. J Electromagnet Waves 17: 1197-1216. doi: 10.1163/156939303322519829
![]() |
[93] | Akila M, Anusha P, Sindhu M, et al. (2017) Examination of PSO, GA-PSO and ACO algorithms for the design optimization of printed antennas. IEEE Applied Electromagnetics Conference, 1-2. |
[94] | Mishra S, Chattopadyay S, Gangopadhyaya M (2015) A comparative study of DE, PSO and BFO for optimisation of Rectangular Microstrip Patch Antenna with inset feed parameter. International Conference and Workshop on Computing and Communication (IEMCON), 1-7. |
[95] |
Boada DFM, Sakomura ES, Nascimento DC (2021) Cavity model surrogate-based optimization for electrically thick circularly polarized rectangular microstrip antennas. AEU-Int J Electron C 131: 153597. doi: 10.1016/j.aeue.2020.153597
![]() |
[96] | Koziel S, Bekasiewicz A (2016) Multi-objective design of antennas using surrogate models. World Scientific. |
[97] |
Lee W, Jung M, Kim TH, et al. (2020) Patch antenna design using the GA and the binary method for dual-band applications. J Electromagnet Waves 34: 1691-1704. doi: 10.1080/09205071.2020.1781697
![]() |
[98] |
Awan WA, Zaidi A, Hussain M, et al. (2021) The Design of a Wideband Antenna with Notching Characteristics for Small Devices Using a Genetic Algorithm. Mathematics 9: 2113. doi: 10.3390/math9172113
![]() |
1. | Faïçal Ndaïrou, Delfim F. M. Torres, Pontryagin Maximum Principle for Incommensurate Fractional-Orders Optimal Control Problems, 2023, 11, 2227-7390, 4218, 10.3390/math11194218 | |
2. | Yuna Oh, Jun Moon, The infinite-dimensional Pontryagin maximum principle for optimal control problems of fractional evolution equations with endpoint state constraints, 2024, 9, 2473-6988, 6109, 10.3934/math.2024299 |