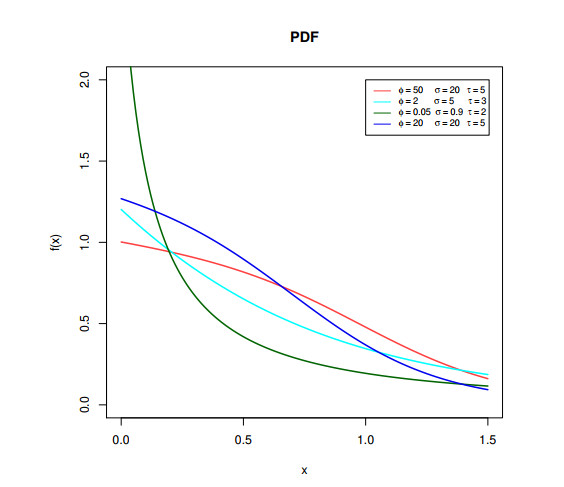
This paper introduces a new extension of the traditional Lomax distribution. The current distribution, which has one scale and two shape parameters, is known as logarithmic transformed Lomax distribution. The hazard rate function of the new distribution based on parameter values, making monotonically decreasing, upside-down and bathtub upside-down shaped. Some properties of the proposed distribution including mixture expansion, moments, entropies, probability weighted moments, order statistics, reversed failure rate and mean past life time are discussed. The distribution parameters are estimated using four frequentist methods: namely, maximum likelihood, least squares, weighted least squares, and maximum product of spacing. The efficiency of the different estimators is compared using a simulation analysis using mean square error. Finally, two real data sets for service times of Aircraft Windshield and survival times of a group of patients given chemotherapy medication are analyzed and compared to some other competing distributions. Based on the results of data analysis, the estimates of minimum and maximum values for the service times of Aircraft Windshield and the survival times of patients given chemotherapy medication are obtained.
Citation: Refah Alotaibi, Hassan Okasha, Hoda Rezk, Abdullah M. Almarashi, Mazen Nassar. On a new flexible Lomax distribution: statistical properties and estimation procedures with applications to engineering and medical data[J]. AIMS Mathematics, 2021, 6(12): 13976-13999. doi: 10.3934/math.2021808
[1] | Ehab M. Almetwally, Ahlam H. Tolba, Dina A. Ramadan . Bayesian and non-Bayesian estimations for a flexible reduced logarithmic-inverse Lomax distribution under progressive hybrid type-Ⅰ censored data with a head and neck cancer application. AIMS Mathematics, 2025, 10(4): 9171-9201. doi: 10.3934/math.2025422 |
[2] | Abdulhakim A. Al-Babtain, Rehan A. K. Sherwani, Ahmed Z. Afify, Khaoula Aidi, M. Arslan Nasir, Farrukh Jamal, Abdus Saboor . The extended Burr-R class: properties, applications and modified test for censored data. AIMS Mathematics, 2021, 6(3): 2912-2931. doi: 10.3934/math.2021176 |
[3] | Samah M. Ahmed, Abdelfattah Mustafa . Estimation of the coefficients of variation for inverse power Lomax distribution. AIMS Mathematics, 2024, 9(12): 33423-33441. doi: 10.3934/math.20241595 |
[4] | Refah Alotaibi, Hassan Okasha, Hoda Rezk, Abdullah M. Almarashi, Mazen Nassar . Correction: On a new flexible Lomax distribution: statistical properties and estimation procedures with applications to engineering and medical data. AIMS Mathematics, 2022, 7(5): 7419-7420. doi: 10.3934/math.2022414 |
[5] | Abdulhakim A. Al-Babtain, Ibrahim Elbatal, Christophe Chesneau, Mohammed Elgarhy . On a new modeling strategy: The logarithmically-exponential class of distributions. AIMS Mathematics, 2021, 6(7): 7845-7871. doi: 10.3934/math.2021456 |
[6] | Dawlah Alsulami . A new extension of the Rayleigh distribution: Properties, different methods of estimation, and an application to medical data. AIMS Mathematics, 2025, 10(4): 7636-7663. doi: 10.3934/math.2025350 |
[7] | Aisha Fayomi, Ehab M. Almetwally, Maha E. Qura . A novel bivariate Lomax-G family of distributions: Properties, inference, and applications to environmental, medical, and computer science data. AIMS Mathematics, 2023, 8(8): 17539-17584. doi: 10.3934/math.2023896 |
[8] | Ahmed Sedky Eldeeb, Muhammad Ahsan-ul-Haq, Mohamed S. Eliwa . A discrete Ramos-Louzada distribution for asymmetric and over-dispersed data with leptokurtic-shaped: Properties and various estimation techniques with inference. AIMS Mathematics, 2022, 7(2): 1726-1741. doi: 10.3934/math.2022099 |
[9] | Fiaz Ahmad Bhatti, G. G. Hamedani, Mashail M. Al Sobhi, Mustafa Ç. Korkmaz . On the Burr XII-Power Cauchy distribution: Properties and applications. AIMS Mathematics, 2021, 6(7): 7070-7092. doi: 10.3934/math.2021415 |
[10] | Naif Alotaibi, A. S. Al-Moisheer, Ibrahim Elbatal, Salem A. Alyami, Ahmed M. Gemeay, Ehab M. Almetwally . Bivariate step-stress accelerated life test for a new three-parameter model under progressive censored schemes with application in medical. AIMS Mathematics, 2024, 9(2): 3521-3558. doi: 10.3934/math.2024173 |
This paper introduces a new extension of the traditional Lomax distribution. The current distribution, which has one scale and two shape parameters, is known as logarithmic transformed Lomax distribution. The hazard rate function of the new distribution based on parameter values, making monotonically decreasing, upside-down and bathtub upside-down shaped. Some properties of the proposed distribution including mixture expansion, moments, entropies, probability weighted moments, order statistics, reversed failure rate and mean past life time are discussed. The distribution parameters are estimated using four frequentist methods: namely, maximum likelihood, least squares, weighted least squares, and maximum product of spacing. The efficiency of the different estimators is compared using a simulation analysis using mean square error. Finally, two real data sets for service times of Aircraft Windshield and survival times of a group of patients given chemotherapy medication are analyzed and compared to some other competing distributions. Based on the results of data analysis, the estimates of minimum and maximum values for the service times of Aircraft Windshield and the survival times of patients given chemotherapy medication are obtained.
The Lomax distribution was introduced to model business data by Lomax [1]. It has many applications in various fields such as income, wealth inequality, engineering, medical and biological sciences, lifetime and survival analysis. The Lomax distribution characterization can be defined in a variety of ways. It is a special case of the Pearson type VI distribution, as well as a combination of the exponential and gamma distributions. However, the Lomax distribution does not give a suitable modelling for data with non-monotone failure rates, such as the upside-down bathtub or bathtub then upside down failure rates, which are common in survival and biological studies. The need for extended forms of the Lomax model arises in many applied areas. The probability density function (PDF) for the Lomax distribution with shape parameter σ and scale parameter τ, is given by
g(x;σ,τ)=στσ(τ+x)−(σ+1),x>0,σ>0,τ>0, | (1.1) |
and the corresponding cumulative distribution function (CDF) is
G(x;σ,τ)=1−τσ(τ+x)−σ. | (1.2) |
The Lomax distribution is also known in the literature as the Pareto distribution of second kind. It has many applications in actuarial, biological studies, finance and insurance. It is also able to model the survival times of patients after a heart transplant (see Bain and Engelhardt [2]). Although the Lomax distribution has a wide range of applications, it cannot cover bathtub upside down failure formed data. Many authors suggested new forms of the standard Lomax distribution by adding shape parameter(s) to get more flexibility in the PDF and the hazard rate function to overcome this lack of flexibility. Among these studies, Marshall-Olkin extended Lomax distribution proposed by Ghitany et al. [3], exponentiated Lomax distribution introduced by Abdul-Moniem and Abdel-Hameed [4], beta Lomax distribution by Lemonte and Cordeiro [5]. Also, Cordeiro et al. [6] introduced gamma Lomax distribution, Tahir et al. [7] proposed Weibull Lomax distribution, Al-Zahrani [8] also proposed extended Poisson-Lomax distribution and transformed Lomax by Nassar et al. [9].
In this study, a new three parameter Lomax distribution which called logarithmic transformed Lomax (LTLo) distribution is introduced by adding a new shape parameter to the CDF in (1.2) based on the method proposed by Pappas et al. [10]. Pappas et al. [10] introduced a method for generating distributions by adding an extra shape parameter to provide a more flexibility to existing distributions. The proposed method is called Logarithmic Transformed (LT) method. The CDF and the corresponding PDF of the LT method are, respectively, given by
F(x;ϕ)=1−log[1−(1−ϕ)ˉG(x)]log(ϕ),ϕ>0,ϕ≠1 | (1.3) |
and
f(x;ϕ)=(ϕ−1)g(x)log(ϕ)[1−(1−ϕ)ˉG(x)], | (1.4) |
where ˉG(x)=1−G(x) and G(x) is the CDF of the baseline distribution. Many authors have used this approach to create new distributions by taking G(x) of any known distribution and applying the method. Al-Zahrani et al. [11] proposed a new extension of Weibull extension model and studied its properties. Dey et al. [12] studied some properties of this generator and obtained an expansion to the CDF and PDF in (1.3) and (1.4), respectively. They also introduced a generalization for generalized exponential distributions. Eltehiwy [13] introduced a logarithmic inverse Lindley distribution by taking the G(x) in (1.3) of the inverse Lindley distribution. We are motivated to propose the LTLo distribution because
1. It contains the Lomax distribution as a sub model.
2. It is suitable for modelling skewed data which may not be properly modelled by other competitive models.
3. It can model monotonically decreasing, upside down and bathtub then upside down hazard rates.
4. As presented in Section 2 that the LTLo distribution can be displayed as a mixture of the Lomax distribution.
5. The analysis of two real data sets show its superiority over other competing distributions namely, Kumaraswamy Lomax (KL), exponentiated Lomax (EL), beta Lomax (BL), gamma-Lomax (GL).
Another motivation to this paper is to see how different classical estimators of the LTLo distribution perform by considering various parameter values and different sample sizes and to draw a guideline for choosing the best estimation method in this case, which may be of deep interest to applied statisticians.
The rest of this article is organized as follows: The LTLo distribution is introduced in Section 2 as well as the expansion of its PDF and CDF. Some statistical properties are derived in Section 3. In Section 4, four methods of estimation are used to estimate the unknown parameters of the LTLo distribution. A simulation study is considered in Section 5 and in Section 6, two real data sets are analyzed. Finally, the paper is concluded in Section 7.
Let X be a random variable follows the Lomax distribution with PDF and CDF, respectively, given by (1.1) and (1.2), then the PDF of the LTLo distribution can be obtained from (1.4) as
f(x;ϕ,σ,τ)=(ϕ−1)στσ(τ+x)−(σ+1)log(ϕ)[1−(1−ϕ)τσ(τ+x)−σ],ϕ,σ,τ>0,ϕ≠1, | (2.1) |
and the corresponding CDF is
F(x;ϕ,σ,τ)=1−log[1−(1−ϕ)τσ(τ+x)−σ]log(ϕ). | (2.2) |
The survival and hazard rate functions of the LTLo distribution are, respectively, given by
S(x;ϕ,σ,τ)=log[1−(1−ϕ)τσ(τ+x)−σ]log(ϕ) | (2.3) |
and
h(x;ϕ,σ,τ)=(ϕ−1)στσ(τ+x)−(σ+1)[1−(1−ϕ)τσ(τ+x)−σ]log[1−(1−ϕ)τσ(τ+x)−σ]. | (2.4) |
Figure 1 depicts the different shapes of the PDF of the LTLo distribution given by (2.1) by considering different values to the scale and the shape parameters. The new distribution is able to model the right skewed data, as can be seen in Figure 1. Figure 2 also depicts the various shapes of its hazard rate function for various parameter values using τ=1. Figure 2 shows that the hazard rate function of the LTLo distribution can be decreasing, upside down, and bathtub then upside down shaped.
Now, an useful representation for the LTLo distribution can be obtained. Using the series representation in the form
(1−q)n−1=∞∑j=0(−1)j(n−1j)qj,if∣q∣<1,n>0 positive real non-integer. | (2.5) |
Applying (2.5) for the PDF in (2.1) gives
f(x)=∞∑k=0ϖkg(x;(k+1)σ,τ,ϕ). | (2.6) |
where g(x;(k+1)σ,τ,ϕ) denotes the PDF of the Lomax distribution with shape parameter (k+1)σ and scale parameter τ and
ϖk=(1−ϕ)k+1(k+1)logϕ. |
Different structural properties of the LTLo distribution can be determined using this representation. By integrating (1.1), the CDF of X is given by
F(x)=∞∑k=0ϖkG(x;(k+1)σ,τ,ϕ), | (2.7) |
where G(x) is the CDF of the Lomax distribution with scale parameter τ and shape parameter (k+1)σ.
In this section, some properties of the LTLo distribution are derived, specially quantile function, moments, incomplete moments, conditional moment, entropies and stress-strength.
For the LTLo distribution, the quantile function denoted by x=QLTLo(q) can be obtained by inverting (2.1) as follows
F−1(q)=QLTLo(u)=((1−ϕ)τσ1−ϕ1−q)1σ−τ. | (3.1) |
One can generate X by taking q as a uniform random variable in (0,1). The skewness and kurtosis measures can be obtained from (3.1) using a well-known relations.
The noncentral moment of the LTLo distribution can be obtained as
μ′r=E(Xr)=∫∞−∞xrf(x)dx=∞∑k=0ϖk∫∞0xrg(x;(k+1)σ,τ,ϕ)dx=∞∑k=0ϖk(k+1)στrB((k+1)σ−r,r+1),σ>r. |
where B(.,.) is the beta function. It is to be noted that the rth moment of X exists iff σ>r. In particular the first noncentral moment is the expected value E(X). Also, the variance of the distribution can be obtained as var(X)=E(X2)−[E(X)]2, where E(X2) is the second noncentral moments. Based on the first four noncentral moments the skewness and kurtosis can be obtained using the following relations
Skewness=μ′3−3μ′2μ+2μ3(μ′2−μ2)32andKurtosis=μ′4−4μ′3μ+6μ′2μ2−3μ4(μ′2−μ2)2. |
The following propositions are a description of three different types from moments such as incomplete moments, moment generating function (mgf) and conditional moment.
Prop 1. If X ∼ LTLo(ϕ,σ,τ), then the incomplete moments of X is given by
IX(t)=∞∑k=0ϖk(k+1)στtn+1(n+1)F2:1((k+1)σ+1;n+1;n+2;−tτ) | (3.2) |
where F2:1(.;.;.;.) is the Gauss hypergeometric function (see Gradshteyn and Ryzhik [14,Formula 9.10]).
The first incomplete moment, I1(t), follows from (3.2) with n=1. The main applications of I1(t) are the Bonferroni and Lorenz curves and the mean deviation.
Prop 2. If X ∼ LTLo, then the mgf of X is given by
MX(t)=∞∑m=0∞∑k=0ϖktmm!σ(k+1)τmB((k+1)σ−m,m+1),σ>m. |
Prop 3. If X ∼ LTLo(ϕ,σ,τ), then the conditional moment of X is given by
CX(t)=E(E(Xn|X>x)=ψn(x)1−F(x), |
where
ψn(x)=∞∑k=0ϖk∫∞xyng(y;(k+1)σ,τ)dy=∞∑k=0ϖk(k+1)στ(k+1)σ(σ(k+1)−n)x(k+1)σ−n×F2:1((k+1)σ−n;(k+1)σ+1;(k+1)σ−n;−τx),σ>n. |
The mean, variance, skewness and kurtosis of the LTLo distribution can be calculated using the expression of the noncentral moments. Figure 3 presents these values for various values of the parameters ϕ and σ and by considering the scale parameter τ to be one in all the cases. Figure 3 shows that the skewness of the LTLo distribution is always positive. It can be also noted that the mean, variance, skewness and kurtosis are non-monotonic as the parameters ranging from small to large.
Entropy is one of the most popular technique that used to measure the uncertainty associated with a random variable and it was originally used in physics. Measuring of entropy is very important role in many areas such as statistics, chemical and biological phenomenon. More entropy is referred to less information that found in a sample. The entropy of a random variable X with density function f(x) is a measure of its uncertainty. The Rˊenyi entropy (RE) introduced by Rˊenyi [15] is defined as
IR(δ)=11−δlog(∫fδ(x)dx),δ≥0,δ≠1. | (3.3) |
For the LTLo distribution with PDF given by (2.1), the RE can be written as
IR(δ)=11−δlog{(στσ(ϕ−1)logϕ)δ∫∞0((τ+x)−(σ+1)1−(1−ϕ)τσ(τ+x)−σ)δdx}=11−δlog{(σ(ϕ−1)logϕ)δ∞∑k=0Γ(k+δ)k!(1−ϕ)kτ(2σ+1)δ+1σ(k−δ)−δ−1},σ≠δ+1k−δ. |
Order statistics have a wide range of uses in statistics, including quality control monitoring, non parametric statistics and reliability. Let X1,…,Xn be a random sample of size n drawn from a continuous population then the PDF of the the sth order statistic is defined by
fX(s)(x)=f(x)B(s,n−s+1)n−s∑j=0(−1)j(n−sj)Fj+s−1(x), | (3.4) |
where B(.,.) is the beta function. Using (2.1) and (2.2), one can obtain
f(x)Fj+s−1(x)=(ϕ−1)∞∑k=0(−1)k(s+j−1k)στσ(τ+x)−(σ+1)ϕ+(ϕ−1)(1−τσ(τ+x)−σ)×[log(ϕ+(ϕ−1)(1−τσ(τ+x)−σ))]k[log(ϕ)]k+1. | (3.5) |
Substituting (3.5) in Eq (3.4), the PDF of X(s) for the LTLo distribution reduces to
fX(s)(x)=ϕ−1B(s,n−s+1)n−s∑j=0∞∑k=0(−1)k+j(n−sj)(s+j−1k)×jτσ(τ+x)−(σ+1)ϕ+(ϕ−1)(1−τσ(τ+x)−σ)[log(ϕ+(ϕ−1)(1−τσ(τ+x)−σ))]k[log(ϕ)]k+1. |
The k−th moment of X(s) can be obtained as
μk(s)=E(Xk(s))=∫∞−∞xkfX(s)(x)dx=ϕ−1B(s,n−s+1)n−s∑j=0∞∑k=0(−1)k+j(n−sj)(s+j−1k)×∫∞0aτσxk(τ+x)−(σ+1)ϕ+(ϕ−1)(1−τσ(τ+x)−σ)[log(ϕ+(ϕ−1)(1−τσ(τ+x)−σ))]k[log(ϕ)]k+1dx. | (3.6) |
A numerical integration technique can be used to obtain (3.6). Let k=1 and by setting s=1 and n, one can obtain the expected values of the first and last order statistics, respectively.
The (u,v)th probability weighted moments of X following the LTLo distribution is defined by
ρu,v=E{XuF(X)v}=∫∞−∞xuf(x)Fv(X)dx. | (3.7) |
Based on Eq (3.7), one can obtain
ρu,v=(ϕ−1)∞∑k=0(−1)k(vk)[log(ϕ)]k+1ξk, | (3.8) |
where
ξk=∫∞0στσxu(τ+x)−(σ+1)[log(ϕ+(ϕ−1)(1−τσ(τ+x)−σ))]−k[ϕ+(ϕ−1)(1−τσ(τ+x)−σ)]dx. | (3.9) |
The nth moment of the residual life can be calculated using a general formula (see Navarro et al. [16]).
Rn(t)=E((X−t)n|X>t)=11−F(t)∫∞t(x−t)nf(x)dx,n≥1. |
Based on Eq (2.1) and applying the binomial expansion of (x−t)n, one can obtain
Rn(t)=11−F(t)n∑m=0(−t)n−m(nm)∫∞txnf(x)dx=σ(ϕ−1)log(ϕ)(1−F(t))n∑m=0∞∑k=0(−1)n−m(nm)(1−ϕ)kτ(k+1)σ((k+1)σ−n)t(k+1)σ+m−2n×F2:1((k+1)σ−n;(k+1)σ+1;(k+1)σ−n;−τt). |
The nth moment of the reversed residual life via the general formula
mn(t)=E((t−X)n|X≤t)=1F(t)∫t0(t−x)nf(x)dx,n≥1. |
Based on Eq (2.1) and applying the binomial expansion of (t−x)n, one can obtain
mn(t)=1F(t)n∑m=0(−t)n−m(nm)∫t0xnf(x)dx=σ(ϕ−1)τlog(ϕ)F(t)n∑m=0∞∑k=0(−1)n−m(nm)(1−ϕ)kt2n−m+1n+1×F2:1((k+1)σ+1;n+1;n+2;−tτ). |
Let T≥0 be a continuous random variable with CDF F(t) and PDF f(t), then the reversed failure rate (RFR) is defined as
r(t)=limΔt→0P(T>t−Δt|T≤t)Δt=f(t)F(t). | (3.10) |
For the LTLo distribution, the RFR, say r(t), is obtained as
r(t)=(ϕ−1)στσ(τ+t)−(σ+1)[1−(1−ϕ)τσ(τ+t)−σ](log(ϕ)−log[1−(1−ϕ)τσ(τ+t)−σ]). |
The first moment of the mean past life time can be obtained via the general formula
S(t)=E((t−X)|X≤t)=1F(t)∫t0F(x)dx,x≥0. |
For the LTLo distribution, the mean past life time, S(t), is obtained as
S(t)=1F(t)∫t0(1−log[1−(1−ϕ)τσ(τ+x)−σ]log(ϕ))dx=1F(t)(t−1log(ϕ)∫t0log[1−(1−ϕ)τσ(τ+x)−σ]dx)=1F(t)(t−1log(ϕ)(−τ(σ−σF2:1(1,−1σ,−1+σσ,1−ϕ)+log(ϕ))+(t+τ)(σ−σF2:1(1,−1σ,−1+σσ,1−(1−ϕ)(τt+τ)σ)+log(1−(1−ϕ)(τt+τ)σ)))},τt>0. | (3.11) |
The strong mean past life time lies in the framework of the RFR function. It has many reliability properties that provide some applications in survival analysis and reliability. The strong mean past life time can be obtained via the general formula
S∗(t)=1F(t)∫t02xF(x)dx,x≥0. |
For the LTLo distribution, the strong mean past life time is derived as
S∗(t)=1F(t)∫t02x(1−log[1−(1−ϕ)τσ(τ+x)−σ]log(ϕ))dx=1F(t)(t2−2log(ϕ)∫t0xlog[1−(1−ϕ)τσ(τ+x)−σ]dx)=1F(t)(t2−12log(ϕ)(tσ(t−2τ)+στ2F2:1(1,−2σ,−2+σσ,(1−ϕ))−σ(t+τ)2F2:1(1,−2σ,−2+σσ,(1−ϕ)(τt+τ)σ)−4στ2F2:1(1,−1σ,−1+σσ,(1−ϕ))+4στ(t+τ)×F2:1(1,−1σ,−1+σσ,(1−ϕ)(τt+τ)σ)+2τ2log(ϕ)+2(t2−τ2)log(1−(1−ϕ)(τt+τ)σ)),τt>0. | (3.12) |
The stress-strength is the measure of the system efficiency which arises quite naturally in the mechanical reliability of a system. Practically, the system fails if and only if the used stress is more than its strength at any time. The concept of the stress-strength has gotten impressive consideration and commonly utilized in numerous engineering applications. Let Z1 and Z2 be two independent random variables with LTLo distributions, respectively. If Z1 represents "stress" and Z2 represents "strength, " then the stress-strength is given by
R=P(Z2>Z1)=∫∞0F1(z)dF2(z)=1−∫∞0ˉF1(z)dF2(z)=1+σ2τσ22(1−ϕ2)log(ϕ1)log(ϕ2)∫∞0(τ2+z)−σ2−1log[1−(1−ϕ1)τσ11(τ1+z)−σ1]1−(1−ϕ2)τσ22(τ2+z)−σ2dz. |
Using series expansion log(11−y)=∑∞k=1ykk, −1≤y<1 in the last equation, one can obtain
R=1−σ2τσ22(1−ϕ2)log(ϕ1)log(ϕ2)∞∑k=1τσ1k1(1−ϕ1)kk∫∞0(τ1+z)−σ1k(τ2+z)−σ2−11−(1−ϕ2)τσ22(τ2+z)−σ2dz=1−σ2τσ22(1−ϕ2)log(ϕ1)log(θ2)∞∑k=1τσ1k1(1−ϕ1)kk∫∞0(τ1+z)−σ1k(τ2+z)−σ2−1×(1−(1−ϕ2)τσ22(τ2+z)−σ2)−1dz. |
Using binomial series expansion in the last equation, one can obtain
R=1−σ2log(ϕ1)log(ϕ2)∞∑k=1∞∑j=0τσ1k1τσ2(j+1)2(1−ϕ1)k(1−ϕ2)j+1k×∫∞0(τ1+z)−σ1k(τ2+z)−σ2jdz=1−σ2log(ϕ1)log(θ2)∞∑k=1∞∑j=0∞∑i=0(−1)ikτ−i1τσ2(j+1)2(1−ϕ1)k(1−ϕ2)j+1×(σ1k+i−1i)∫∞0zi(τ2+z)−σ2jdz=1−σ2log(ϕ1)log(ϕ2)∞∑k=1∞∑j=0∞∑i=0(−1)ikτ−i1τσ2+i+12(1−ϕ1)k(1−ϕ2)j+1×(σ1k+i−1i)B(i+1,σ2j−i−1). |
In this section, four estimation methods are considered to estimates the unknown parameters, ϕ,σ and τ, of the the LTLo distribution.
To obtain the maximum likelihood estimates (MLEs), the log-likelihood function of the observations x1,⋯,xr of a random sample of size r from the PDF in (2.1) can be written as follows
L=rlog(ϕ−1)−rlog(σ)−rσlog(τ)−rlog(log(ϕ))−(σ+1)r∑j=1log(τ+xj)−r∑j=1log[1−(1−ϕ)τσ(τ+xj)−σ]. | (4.1) |
For the unknown parameters ϕ,σ and τ, the MLEs can be obtained by solving the following normal euations
∂L∂ϕ=rϕ−1−rϕlog(ϕ)−r∑j=1τσ(τ+xj)−σ1−(1−ϕ)τσ(τ+xj)−σ=0, |
∂L∂σ=rσ−rlog(τ)−r∑j=1log(τ+xj)+r∑j=1τσ(ϕ−1)[log(τ+xj)−log(τ)](τ+xj)σ[1−(1−ϕ)τσ(τ+xj)−σ]=0 |
and
∂L∂τ=rστ−(σ+1)r∑j=11τ+xj−r∑j=1στσ−1(ϕ−1)xj(τ+xj)σ+1[1−(1−ϕ)τσ(τ+xj)−σ]=0. |
To obtain the MLEs of ϕ,σ and τ, any numerical technique can be implemented for this purpose.
Let r denotes to the sample size and X(1)<X(2)<⋯<X(r) be the order statistics from the LTLo distribution, the least square estimators (LSEs) of the unknown parameters ϕ,σ and τ can be obtained by minimizing the following function
LS=r∑j=1[(1−log[1−(1−ϕ)τσ(τ+x(j))−σ]log(ϕ))−vj]2, |
with respect to unknown parameters ϕ,σ and τ, where vj=jn+1 or by solving
r∑j=1[(1−log[1−(1−ϕ)τσ(τ+x(j))−σ]log(ϕ))−vj]ψ1j=0, |
r∑j=1[(1−log[1−(1−ϕ)τσ(τ+x(j))−σ]log(ϕ))−vj]ψ2j=0 |
and
r∑j=1[(1−log[1−(1−ϕ)τσ(τ+x(j))−σ]log(ϕ))−vj]ψ3j=0, |
where
ψ1j=τσ(τ+x(j))−σ1−(1−ϕ)τσ(τ+x(j))−σ, | (4.2) |
ψ2j=τσ(ϕ−1)[log(τ+x(j))−log(τ)](τ+x(j))σ[1−(1−ϕ)τσ(τ+x(j))−σ] | (4.3) |
and
ψ3j=στσ−1(ϕ−1)x(j)(τ+x(j))σ+1[1−(1−ϕ)τσ(τ+x(j))−σ]. | (4.4) |
Similarly, one can obtain the weighted least square estimators (WLSEs) of the unknown parameters ϕ,σ and b, by minimizing
WLS=r∑j=1ci[(1−log[1−(1−ϕ)τσ(τ+x(j))−σ]log(ϕ))−vj]2, |
with respect to ϕ,σ and τ, where cj=(r+1)2(r+2)j(n−j+1) or by solving the following three nonlinear equations
r∑j=1cj[(1−log[1−(1−ϕ)τσ(τ+x(j))−σ]log(ϕ))−vj]ψ1j=0, |
r∑j=1cj[(1−log[1−(1−ϕ)τσ(τ+x(j))−σ]log(ϕ))−vj]ψ2j=0 |
and
r∑j=1cj[(1−log[1−(1−ϕ)τσ(τ+x(j))−σ]log(ϕ))−vj]ψ3j=0. |
where ψ1j,ψ2j and ψ3j are given by (4.2)–(4.4).
Let Λi(ϕ,a,τ) be the uniform spacings of a random sample obtained from the LTLo distribution which can be written as
Λj(ϕ,σ,τ)=log[1−(1−ϕ)τσ(τ+x(j−1))−σ]−log[1−(1−ϕ)τσ(τ+x(j))−σ]log(ϕ). |
Thus, the maximum product of spacing (MPS) estimators of ϕ,σ and τ can be obtained by maximizing the following function
MPS=1r+1r+1∑j=1logΛj(ϕ,σ,τ), |
with respect to ϕ,σ and τ. Another way to obtain these estimates is to solve the following three nonlinear equations
r+1∑j=1ψ1j−ψ1j−1Λj(ϕ,σ,τ)=0, |
r+1∑j=1ψ2j−ψ2j−1Λj(ϕ,σ,τ)=0 |
and
r+1∑j=1ψ3j−ψ3j−1Λj(ϕ,σ,τ)=0. |
where ψ1j,ψ2j and ψ3j are given by (4.2)–(4.4).
In this part, a simulation study is performed to evaluate which estimation method provides the most efficient estimates based on the minimal mean square error criterion, while taking into consideration various parameter values and sample sizes. The selected sample sizes are 20, 50,100,150,200 and 250, and the parameter values are (ϕ,σ,τ)=(2,2,2),(1.5,0.5,0.8) and (2.5,1.5,1). For each environment, the procedure is repeated 1000 times, and average estimates and average mean square errors (MSEs) are calculated. It is to be mentioned here that the different estimates are obtained using the quasi-Newton method and all computations in this section and the next section are implemented using MATHCAD program Ver.15. Also, all figures in this paper are developed using R programming language. Tables 1–3 summarize these values. From these tables it is observed that the various estimation methods give asymptotically unbiased estimates, meaning that as the sample size grows, the estimates appear to match the true parameter values. It should also be noted that as the sample size grows, the MSEs for all of the estimates decrease. Furthermore, the simulation results show that in the majority of circumstances, the LSEs have the smallest MSEs.
Estimates | MSE | ||||||||
n | Parameter | MLEs | LSEs | WLSEs | MPSEs | MLE | LSE | WLSE | MPSE |
20 | ϕ | 2.208 | 2.193 | 2.203 | 2.226 | 2.293 | 2.273 | 2.214 | 2.575 |
σ | 2.645 | 2.147 | 2.204 | 3.079 | 1.349 | 1.161 | 1.100 | 1.951 | |
τ | 3.175 | 2.540 | 2.628 | 3.439 | 2.526 | 1.749 | 1.853 | 2.726 | |
50 | ϕ | 2.013 | 2.069 | 2.142 | 1.965 | 1.843 | 1.860 | 1.904 | 1.854 |
σ | 2.473 | 2.141 | 2.174 | 2.802 | 0.807 | 0.757 | 0.694 | 1.212 | |
τ | 3.054 | 2.477 | 2.508 | 3.453 | 2.334 | 1.528 | 1.601 | 2.581 | |
100 | ϕ | 1.959 | 2.012 | 2.045 | 1.947 | 1.725 | 1.680 | 1.697 | 1.649 |
σ | 2.354 | 2.122 | 2.163 | 2.591 | 0.472 | 0.506 | 0.425 | 0.725 | |
bτ | 2.922 | 2.394 | 2.409 | 3.291 | 2.059 | 1.396 | 1.431 | 2.321 | |
150 | ϕ | 1.943 | 1.974 | 1.992 | 1.957 | 1.717 | 1.506 | 1.525 | 1.581 |
σ | 2.262 | 2.118 | 2.201 | 2.458 | 0.301 | 0.346 | 0.291 | 0.465 | |
τ | 2.829 | 2.317 | 2.346 | 3.209 | 1.866 | 1.213 | 1.317 | 2.416 | |
200 | ϕ | 1.953 | 1.981 | 1.941 | 1.921 | 1.693 | 1.368 | 1.462 | 1.438 |
σ | 2.237 | 2.175 | 2.184 | 2.325 | 0.245 | 0.279 | 0.225 | 0.381 | |
τ | 2.790 | 2.264 | 2.515 | 3.133 | 1.812 | 1.108 | 1.237 | 2.352 | |
250 | ϕ | 1.960 | 1.986 | 1.966 | 1.918 | 1.551 | 1.302 | 1.317 | 1.301 |
σ | 2.117 | 2.108 | 2.130 | 2.274 | 0.213 | 0.213 | 0.179 | 0.316 | |
τ | 2.315 | 2.221 | 2.363 | 2.700 | 1.673 | 1.076 | 1.178 | 2.037 |
Estimates | MSE | ||||||||
n | Parameter | MLEs | LSEs | WLSEs | MPSEs | MLE | LSE | WLSE | MPSE |
20 | ϕ | 2.154 | 1.853 | 1.805 | 2.737 | 2.509 | 2.011 | 2.083 | 2.993 |
σ | 0.596 | 0.519 | 0.517 | 0.702 | 0.076 | 0.072 | 0.063 | 0.130 | |
τ | 1.362 | 1.142 | 1.219 | 1.148 | 1.111 | 0.802 | 0.896 | 0.848 | |
50 | ϕ | 1.738 | 1.581 | 1.557 | 2.212 | 1.513 | 1.259 | 1.316 | 1.689 |
σ | 0.531 | 0.504 | 0.505 | 0.586 | 0.021 | 0.022 | 0.020 | 0.029 | |
τ | 1.249 | 1.137 | 1.182 | 1.052 | 0.686 | 0.524 | 0.578 | 0.448 | |
100 | ϕ | 1.590 | 1.490 | 1.483 | 1.912 | 1.109 | 0.955 | 1.015 | 1.131 |
σ | 0.504 | 0.491 | 0.492 | 0.537 | 0.009 | 0.010 | 0.009 | 0.010 | |
τ | 1.119 | 1.058 | 1.095 | 0.988 | 0.425 | 0.345 | 0.388 | 0.301 | |
150 | ϕ | 1.542 | 1.496 | 1.487 | 1.811 | 0.880 | 0.755 | 0.773 | 0.839 |
σ | 0.498 | 0.497 | 0.493 | 0.524 | 0.006 | 0.007 | 0.006 | 0.006 | |
τ | 1.038 | 0.984 | 1.007 | 0.928 | 0.288 | 0.226 | 0.246 | 0.199 | |
200 | ϕ | 1.480 | 1.497 | 1.494 | 1.674 | 0.653 | 0.609 | 0.614 | 0.610 |
σ | 0.497 | 0.498 | 0.491 | 0.517 | 0.004 | 0.005 | 0.004 | 0.004 | |
τ | 1.002 | 0.976 | 0.988 | 0.915 | 0.195 | 0.173 | 0.182 | 0.148 | |
250 | ϕ | 1.495 | 1.498 | 1.493 | 1.547 | 0.420 | 0.411 | 0.404 | 0.337 |
σ | 0.495 | 0.499 | 0.498 | 0.510 | 0.003 | 0.004 | 0.003 | 0.003 | |
τ | 0.931 | 0.928 | 0.933 | 0.885 | 0.093 | 0.096 | 0.097 | 0.075 |
Estimates | MSE | ||||||||
n | Parameter | MLEs | LSEs | WLSEs | MPSEs | MLE | LSE | WLSE | MPSE |
20 | ϕ | 2.080 | 2.131 | 1.664 | 2.118 | 2.862 | 2.233 | 2.182 | 3.265 |
σ | 2.193 | 1.799 | 1.758 | 2.617 | 1.245 | 0.834 | 0.842 | 1.887 | |
τ | 2.205 | 1.562 | 1.467 | 2.463 | 2.209 | 0.984 | 1.362 | 2.623 | |
50 | ϕ | 2.256 | 2.209 | 2.270 | 2.191 | 2.748 | 2.200 | 2.756 | 3.042 |
σ | 1.883 | 1.682 | 1.703 | 2.163 | 0.804 | 0.455 | 0.748 | 0.796 | |
τ | 2.050 | 1.406 | 1.431 | 2.291 | 1.778 | 0.876 | 1.575 | 2.333 | |
100 | ϕ | 2.357 | 2.378 | 2.342 | 2.354 | 2.266 | 2.071 | 2.356 | 2.468 |
σ | 1.744 | 1.675 | 1.695 | 2.542 | 0.587 | 0.369 | 0.510 | 0.555 | |
τ | 1.692 | 1.361 | 1.353 | 1.684 | 1.010 | 0.827 | 0.820 | 0.988 | |
150 | ϕ | 2.401 | 2.419 | 2.367 | 2.692 | 1.391 | 1.006 | 1.004 | 1.592 |
σ | 1.659 | 1.643 | 1.645 | 1.778 | 0.155 | 0.114 | 0.164 | 0.228 | |
τ | 1.404 | 1.261 | 1.272 | 1.582 | 0.363 | 0.225 | 0.225 | 0.524 | |
200 | ϕ | 2.421 | 2.442 | 2.433 | 2.398 | 0.941 | 0.810 | 0.810 | 0.995 |
σ | 1.598 | 1.539 | 1.564 | 1.658 | 0.063 | 0.060 | 0.078 | 0.083 | |
τ | 1.245 | 1.154 | 1.169 | 1.300 | 0.121 | 0.077 | 0.086 | 0.144 | |
250 | ϕ | 2.429 | 2.468 | 2.452 | 2.443 | 0.570 | 0.681 | 0.695 | 0.566 |
σ | 1.479 | 1.486 | 1.465 | 1.540 | 0.033 | 0.025 | 0.045 | 0.031 | |
τ | 1.074 | 1.022 | 1.054 | 1.094 | 0.025 | 0.020 | 0.021 | 0.027 |
Here, we provide an application to two real data sets to show the importance of our new distribution. The first data set (data set I) presents the service times of 63 Aircraft Windshield and measured in 1000 h. These data were considered by Murthy et al. [17]. The second data set (data set II) reported by Bekker et al. [18], which refers to the survival times (in years) of a group of patients given chemotherapy medication alone. The data set includes the survival times (in years) for 46 patients. The LTLo distribution is fitted to these data using the maximum likelihood method and compare its results with some competitive models namely, KL, EL, BL, GL and Lomax (L) distributions. These distributions have the following PDFs (for x>0):
KL:f(x;ϕ,α,σ,τ)=ϕαστ(1+xτ)−(σ+1)[1−(1+xτ)−σ]ϕ−1{1−[1−(1+xτ)−σ]ϕ}α−1. |
EL:f(x;ϕ,σ,τ)=ϕστ(1+xτ)−(σ+1)[1−(1+xτ)−σ]ϕ−1, |
BL:f(x;ϕ,α,σ,τ)=στB(ϕ,α)(1+xτ)−(ασ+1)[1−(1+xτ)−σ]ϕ−1, |
and
GL:f(x;ϕ,σ,τ)=στσΓ(ϕ)(τ+x)σ+1{−σlog(ττ+x)}ϕ−1. |
The estimates and the corresponding standard error of estimates of these models are presented in Table 4 for data set I and in Table 5 for data set II. The Kolmogorov-Smirnov (K-S) statistic and its p-value are used to compare the various models with the LTLo model. Tables 4 and 5 also show these statistics for data set I and data set II, respectively. Moreover, the goodness of fit statistics, Akaike information criterion (AIC), consistent Akaike information criterion (CAIC), Bayesian information criterion (BIC), Hannan-Quinn information criterion (HQIC), Anderson-Darling (A∗) and Cramer-von Mises (W∗) are also computed and displayed in Table 6 for the two real data sets. Based on these findings, one can conclude that the LTLo distribution perform better than the other competing models, and therefore it should be chosen as the best one. Also, the Q-Q plots for the two data sets are presented in Figure 4 which indicate that the LTLo distribution is a good model to fit these data. The plots of the histogram and the fitted PDF of the LTLo distribution are displayed in Figures 5(a) and 6(a) for data set I and data set II, respectively. On the other hand, the plots of the fitted and the empirical survival functions are displayed in Figures 5(b) and 6(b) for data set I and data set II, respectively. Overall, one can deduce that the LTLo distribution is an acceptable model to fit these data sets. Figure 7 displays the log-likelihood functions of ϕ,σ and τ for the two data sets, which indicate that the MLEs are unique. Since the L distribution can be obtained as a special case of the LTLo distribution. Therefore, the likelihood ratio test (LRT) can be considered to test the null hypothesis that the parameter ϕ tends to one. The results of the LRT are presented in Table 7 for the two data sets. The results of the LRT show that the LTLo distribution is more appropriate for the given data sets than the traditional L distribution.
Model | Estimates | K-S (p-value) | |||
LTLo(ϕ,σ,τ) | 814.15 (2249.9) | 156.240 (449.800) | 85.750 (265.21) | 0.0839 (0.7668) | |
KL(ϕ, α, σ, τ) | 2.5700 (4.7600) | 65.0600 (177.600) | 1.6700 (0.2600) | 60.57 (86.010) | 0.0988 (0.5707) |
EL(ϕ, σ, τ) | 1.9150 (0.3500) | 22971.15 (3209.5) | 32882 (162.230) | 0.1298 (0.2391) | |
BL(ϕ, α, σ, τ) | 4.9700 (50.530) | 169.570 (339.210) | 1.9300 (0.3200) | 31.26 (316.84) | 0.1237 (0.2904) |
GL(α, σ, τ) | 1.9100 (0.3200) | 35842.4 (6945.10) | 39197.6 (151.7) | 0.1227 (0.2993) | |
L(σ, τ) | 99269 (11863.5) | 207019.4 (301.20) | 0.1919 (0.0193) |
Model | Estimates | K-S (p-value) | |||
LTLo(ϕ,σ,τ) | 1.158 (1.256) | 136.941 (639.186) | 174.126 (857.591) | 0.0940 (0.8216) | |
KL(ϕ, α, σ, τ) | 1.054 (0.352) | 140.562 (25.321) | 47.377 (1.0253) | 7073.1 (352.5) | 0.1076 (0.6743) |
EL(ϕ, σ, τ) | 1.1340 (0.243) | 30.246 (4.222) | 36.276 (5.930) | 0.1079 (0.6324) | |
BL(ϕ, α, σ, τ) | 1.1194 (0.221) | 11.537 (8.468) | 4.1860 (5.700) | 56.945 (3.381) | 0.1095 (0.6144) |
GL(α, σ, τ) | 1.1525 (0.2423) | 17.185 (3.067) | 18.787 (4.044) | 0.1087 (0.6229) | |
L(σ, τ) | 132.7 (565.6) | 176.898 (759.2) | 0.1895 (0.0790) |
Model | Data I | Data II | ||||||||||
AIC | BIC | CAIC | HQIC | A∗ | W∗ | AIC | BIC | CAIC | HQIC | A∗ | W∗ | |
LTLo | 203.9 | 210.4 | 204.4 | 206.5 | 0.311 | 0.04 | 122.5 | 127.9 | 123.1 | 124.5 | 0.510 | 0.075 |
KL | 209.7 | 218.3 | 210.4 | 213.1 | 0.740 | 0.122 | 124.2 | 131.4 | 125.2 | 126.9 | 0.544 | 0.081 |
EL | 213.1 | 219.5 | 213.5 | 215.6 | 1.230 | 0.204 | 122.3 | 127.7 | 122.9 | 124.3 | 0.515 | 0.076 |
BL | 213.9 | 222.4 | 214.6 | 217.2 | 1.130 | 0.186 | 124.2 | 131.5 | 125.3 | 126.9 | 0.522 | 0.077 |
GL | 211.7 | 218.1 | 212.1 | 214.2 | 1.110 | 0.200 | 122.4 | 127.8 | 123.0 | 124.4 | 0.511 | 0.076 |
L | 222.6 | 226.9 | 223.0 | 224.3 | 1.130 | 0.190 | 237.2 | 241.5 | 237.4 | 238.9 | 1.624 | 0.206 |
Data | Test value | p-value |
I | 17.558 | 0.000 |
II | 114.70 | 0.000 |
The different estimation methods are used to calculate the estimates the LTLo model parameters for the two data sets. These estimates are obtained and presented in Tables 8 and 9 for the data set I and data set II, respectively. The K-S and its p-value are obtained for each method to show which method provides a good fit. The results in Tables 8 and 9 show that the LSEs has the smallest K-S distance with highest p-value. So, it is recommended to use the least square method to estimate the parameters of the LTLo distribution from the two real data sets. Now, the estimates displayed in Table 8 and the moments of the first and last order statistics can be used to estimate the minimum and the maximum of the service times of Aircraft Windshield in 1000 h on average for different sample sizes. These estimates are displayed in Figure 8 for data set I based on the different estimation methods. From Figure 8 and using the least square method one can expect that the minimum service time of Aircraft Windshield is 0.024 1000 h based on a sample of size 160 Aircraft Windshield. Similarly, it is expected to have 6.122 1000 h as a maximum service time of Aircraft Windshield. From data set II and using the same approach, the different estimates of the minimum and the maximum survival times (in years) are displayed in Figure 9 based on different sample sizes. Based on the least square method one can observe from Figure 9 that the expected minimum survival time is 0.0072 year based on a sample of 160 patients, while the expected maximum survival time is 7.109 year.
Model | Estimates (ϕ,σ,τ) | K-S (p-value) | ||
MLEs | 814.15 | 156.240 | 85.750 | 0.0839 (0.7668) |
LSEs | 858.79 | 154.470 | 87.170 | 0.0755 (0.8656) |
WLSEs | 916.34 | 156.880 | 87.390 | 0.0763 (0.8567) |
MPSEs | 814.95 | 154.192 | 82.686 | 0.0922 (0.6585) |
Model | Estimates (ϕ,σ,τ) | K-S (p-value) | ||
MLEs | 1.158 | 136.941 | 174.126 | 0.0940 (0.8216) |
LSEs | 0.362 | 128.573 | 244.201 | 0.0790 (0.9414) |
WLSEs | 0.960 | 134.956 | 188.283 | 0.0972 (0.7885) |
MPSEs | 0.982 | 136.635 | 176.550 | 0.0796 (0.9382) |
In this paper, a new extension of Lomax distribution which called logarithmic transformed Lomax distribution has been introduced. The proposed distribution contains one scale and two shape parameters. Its hazard rate function can be decreasing, upside down and bathtub then upside down shaped. The various hazard rate shapes candidate the distribution to use quite effectively in analyzing many life applications. The new statistical properties of the proposed distribution are discussed. Also, the unknown parameters of the proposed distribution are estimated using four different estimation methods. In terms of minimum mean square errors, the performance of the various estimators is compared using a simulation study. The least square estimates outperformed the other suggested approaches in a simulation analysis. Finally, two real data sets for service times of Aircraft Windshield and survival times of a group of patients given chemotherapy medication are examined to demonstrate its usefulness and superiority over some well-known distributions with three or more parameters. Finally, The proposed distribution is used to estimate the minimum and maximum values for the service times of Aircraft Windshield and the survival times of patients given chemotherapy medication. As a future work, one can estimate the entropy, stress-strength, Bonferroni and Lorenz curves of the logarithmic transformed Lomax distribution using complete or censored data.
The authors would like to express their thanks to the editor and anonymous referees for useful suggestions and valuable comments. This research was funded by the Deanship of Scientific Research at Princess Nourah bint Abdulrahman University through the Fast-track Research Funding Program.
The authors declare there is no conflict of interest in this paper.
[1] | K. S. Lomax, Business failures: Another example of the analysis of failure data, J. Am. Stat. Assoc., 49 (1954), 847–852. |
[2] | L. Bain, M. Engelhardt, Introduction to Probability and Mathematical Statistics, Duxbury Press, 1992. |
[3] | M. E. Ghitany, F. A. Al-Awadhi, L. A. Alkhalfan, Marshall-Olkin extended Lomax distribution and its application to censored data, Commun. Stat. Theory Methods, 36 (2007), 1855–1866. |
[4] | I. B. Abdul-Moniem, H. F. Abdel-Hameed, On exponentiated Lomax distribution, Int. J. Math. Arch., 3 (2012), 2144–2150. |
[5] | A. J. Lemonte, G. M. Cordeiro, An extended Lomax distribution, Statistics, 47 (2013), 800–816. |
[6] | G. M. Cordeiro, E. M. M. Ortega, D. C. C. da Cunha, The Exponentiated Generalized Class of Distributions, J. Data Sci., 11 (2013), 1–27. |
[7] | M. H. Tahir, G. M. Cordeiro, M. Mansoor, M. Zubair, The Weibull-Lomax distribution: properties and applications, Hacettepe J. Math. Stat., 44 (2015), 461–480. |
[8] | B. AL-Zahrani, An extended Poisson-Lomax distribution, Adv. Math. Sci. J., 4 (2015), 79–89. |
[9] | M. Nassar, S. Dey, D. Kumar, Logarithm transformed Lomax distribution with applications, Calcutta Stat. Assoc. Bull., 70 (2018), 122–135. |
[10] | V. Pappas, K. Adamidis, S. Loukas, A family of lifetime distributions, Int. J. Qual., Stat. Reliab., (2012), 1–6. |
[11] | B. Al-Zahrani, P. R. D. Marinho, A. A. Fattah, A. H. N. Ahmed, G. M. Cordeiro, The (P-A-L) extended Weibull distribution: a new generalization of the Weibull distribution, Hacettepe J. Math. Stat., 45 (2016), 851–868. |
[12] | S. Dey, M. Nassar, D. Kumar, Alpha logarithmic transformed family of distributions with applications, Ann. Data Sci., 4 (2017), 457–482. |
[13] | M. Eltehiwy, Logarithmic inverse Lindley distribution: Model, properties and applications, J. King Saud. Univ. Sci., 32 (2018), 136–144. |
[14] | I. S. Gradshteyn, I. M. Ryzhik, Tables of Integrals, Series and Products, Seventh Edition, Academic Press, Inc., 2007. |
[15] | A. Rényi, On measures of entropy and information, In: Berkeley Symposium on Mathematical Statistics and Probability, University of California Press, Berkeley, 1961,547–561. |
[16] | J. Navarro, M. Franco, J. M. Ruiz, Characterization through moments of the residual life and conditional spacing, Indian J. Stat., Ser. A, 60 (1998), 36–48. |
[17] | D. N. P. Murthy, M. Xie, R. Jiang, Weibull Models, John Wiley, New York, 2004. |
[18] | A. Bekker, J. Roux, P. Mostert, A generalization of the compound Rayleigh distribution: using a Bayesian methods on cancer survival times, Commun. Stat. Theory Methods, 29 (2000), 1419–1433. |
1. | Refah Alotaibi, Mazen Nassar, A New Exponential Distribution to Model Concrete Compressive Strength Data, 2022, 12, 2073-4352, 431, 10.3390/cryst12030431 | |
2. | Refah Alotaibi, Hassan Okasha, Hoda Rezk, Abdullah M. Almarashi, Mazen Nassar, Correction: On a new flexible Lomax distribution: statistical properties and estimation procedures with applications to engineering and medical data, 2022, 7, 2473-6988, 7419, 10.3934/math.2022414 | |
3. | Huda M. Alshanbari, Omalsad Hamood Odhah, Zubair Ahmad, Faridoon Khan, Abd Al-Aziz Hosni El-Bagoury, A New Probability Distribution: Model, Theory and Analyzing the Recovery Time Data, 2023, 12, 2075-1680, 477, 10.3390/axioms12050477 |
Estimates | MSE | ||||||||
n | Parameter | MLEs | LSEs | WLSEs | MPSEs | MLE | LSE | WLSE | MPSE |
20 | ϕ | 2.208 | 2.193 | 2.203 | 2.226 | 2.293 | 2.273 | 2.214 | 2.575 |
σ | 2.645 | 2.147 | 2.204 | 3.079 | 1.349 | 1.161 | 1.100 | 1.951 | |
τ | 3.175 | 2.540 | 2.628 | 3.439 | 2.526 | 1.749 | 1.853 | 2.726 | |
50 | ϕ | 2.013 | 2.069 | 2.142 | 1.965 | 1.843 | 1.860 | 1.904 | 1.854 |
σ | 2.473 | 2.141 | 2.174 | 2.802 | 0.807 | 0.757 | 0.694 | 1.212 | |
τ | 3.054 | 2.477 | 2.508 | 3.453 | 2.334 | 1.528 | 1.601 | 2.581 | |
100 | ϕ | 1.959 | 2.012 | 2.045 | 1.947 | 1.725 | 1.680 | 1.697 | 1.649 |
σ | 2.354 | 2.122 | 2.163 | 2.591 | 0.472 | 0.506 | 0.425 | 0.725 | |
bτ | 2.922 | 2.394 | 2.409 | 3.291 | 2.059 | 1.396 | 1.431 | 2.321 | |
150 | ϕ | 1.943 | 1.974 | 1.992 | 1.957 | 1.717 | 1.506 | 1.525 | 1.581 |
σ | 2.262 | 2.118 | 2.201 | 2.458 | 0.301 | 0.346 | 0.291 | 0.465 | |
τ | 2.829 | 2.317 | 2.346 | 3.209 | 1.866 | 1.213 | 1.317 | 2.416 | |
200 | ϕ | 1.953 | 1.981 | 1.941 | 1.921 | 1.693 | 1.368 | 1.462 | 1.438 |
σ | 2.237 | 2.175 | 2.184 | 2.325 | 0.245 | 0.279 | 0.225 | 0.381 | |
τ | 2.790 | 2.264 | 2.515 | 3.133 | 1.812 | 1.108 | 1.237 | 2.352 | |
250 | ϕ | 1.960 | 1.986 | 1.966 | 1.918 | 1.551 | 1.302 | 1.317 | 1.301 |
σ | 2.117 | 2.108 | 2.130 | 2.274 | 0.213 | 0.213 | 0.179 | 0.316 | |
τ | 2.315 | 2.221 | 2.363 | 2.700 | 1.673 | 1.076 | 1.178 | 2.037 |
Estimates | MSE | ||||||||
n | Parameter | MLEs | LSEs | WLSEs | MPSEs | MLE | LSE | WLSE | MPSE |
20 | ϕ | 2.154 | 1.853 | 1.805 | 2.737 | 2.509 | 2.011 | 2.083 | 2.993 |
σ | 0.596 | 0.519 | 0.517 | 0.702 | 0.076 | 0.072 | 0.063 | 0.130 | |
τ | 1.362 | 1.142 | 1.219 | 1.148 | 1.111 | 0.802 | 0.896 | 0.848 | |
50 | ϕ | 1.738 | 1.581 | 1.557 | 2.212 | 1.513 | 1.259 | 1.316 | 1.689 |
σ | 0.531 | 0.504 | 0.505 | 0.586 | 0.021 | 0.022 | 0.020 | 0.029 | |
τ | 1.249 | 1.137 | 1.182 | 1.052 | 0.686 | 0.524 | 0.578 | 0.448 | |
100 | ϕ | 1.590 | 1.490 | 1.483 | 1.912 | 1.109 | 0.955 | 1.015 | 1.131 |
σ | 0.504 | 0.491 | 0.492 | 0.537 | 0.009 | 0.010 | 0.009 | 0.010 | |
τ | 1.119 | 1.058 | 1.095 | 0.988 | 0.425 | 0.345 | 0.388 | 0.301 | |
150 | ϕ | 1.542 | 1.496 | 1.487 | 1.811 | 0.880 | 0.755 | 0.773 | 0.839 |
σ | 0.498 | 0.497 | 0.493 | 0.524 | 0.006 | 0.007 | 0.006 | 0.006 | |
τ | 1.038 | 0.984 | 1.007 | 0.928 | 0.288 | 0.226 | 0.246 | 0.199 | |
200 | ϕ | 1.480 | 1.497 | 1.494 | 1.674 | 0.653 | 0.609 | 0.614 | 0.610 |
σ | 0.497 | 0.498 | 0.491 | 0.517 | 0.004 | 0.005 | 0.004 | 0.004 | |
τ | 1.002 | 0.976 | 0.988 | 0.915 | 0.195 | 0.173 | 0.182 | 0.148 | |
250 | ϕ | 1.495 | 1.498 | 1.493 | 1.547 | 0.420 | 0.411 | 0.404 | 0.337 |
σ | 0.495 | 0.499 | 0.498 | 0.510 | 0.003 | 0.004 | 0.003 | 0.003 | |
τ | 0.931 | 0.928 | 0.933 | 0.885 | 0.093 | 0.096 | 0.097 | 0.075 |
Estimates | MSE | ||||||||
n | Parameter | MLEs | LSEs | WLSEs | MPSEs | MLE | LSE | WLSE | MPSE |
20 | ϕ | 2.080 | 2.131 | 1.664 | 2.118 | 2.862 | 2.233 | 2.182 | 3.265 |
σ | 2.193 | 1.799 | 1.758 | 2.617 | 1.245 | 0.834 | 0.842 | 1.887 | |
τ | 2.205 | 1.562 | 1.467 | 2.463 | 2.209 | 0.984 | 1.362 | 2.623 | |
50 | ϕ | 2.256 | 2.209 | 2.270 | 2.191 | 2.748 | 2.200 | 2.756 | 3.042 |
σ | 1.883 | 1.682 | 1.703 | 2.163 | 0.804 | 0.455 | 0.748 | 0.796 | |
τ | 2.050 | 1.406 | 1.431 | 2.291 | 1.778 | 0.876 | 1.575 | 2.333 | |
100 | ϕ | 2.357 | 2.378 | 2.342 | 2.354 | 2.266 | 2.071 | 2.356 | 2.468 |
σ | 1.744 | 1.675 | 1.695 | 2.542 | 0.587 | 0.369 | 0.510 | 0.555 | |
τ | 1.692 | 1.361 | 1.353 | 1.684 | 1.010 | 0.827 | 0.820 | 0.988 | |
150 | ϕ | 2.401 | 2.419 | 2.367 | 2.692 | 1.391 | 1.006 | 1.004 | 1.592 |
σ | 1.659 | 1.643 | 1.645 | 1.778 | 0.155 | 0.114 | 0.164 | 0.228 | |
τ | 1.404 | 1.261 | 1.272 | 1.582 | 0.363 | 0.225 | 0.225 | 0.524 | |
200 | ϕ | 2.421 | 2.442 | 2.433 | 2.398 | 0.941 | 0.810 | 0.810 | 0.995 |
σ | 1.598 | 1.539 | 1.564 | 1.658 | 0.063 | 0.060 | 0.078 | 0.083 | |
τ | 1.245 | 1.154 | 1.169 | 1.300 | 0.121 | 0.077 | 0.086 | 0.144 | |
250 | ϕ | 2.429 | 2.468 | 2.452 | 2.443 | 0.570 | 0.681 | 0.695 | 0.566 |
σ | 1.479 | 1.486 | 1.465 | 1.540 | 0.033 | 0.025 | 0.045 | 0.031 | |
τ | 1.074 | 1.022 | 1.054 | 1.094 | 0.025 | 0.020 | 0.021 | 0.027 |
Model | Estimates | K-S (p-value) | |||
LTLo(ϕ,σ,τ) | 814.15 (2249.9) | 156.240 (449.800) | 85.750 (265.21) | 0.0839 (0.7668) | |
KL(ϕ, α, σ, τ) | 2.5700 (4.7600) | 65.0600 (177.600) | 1.6700 (0.2600) | 60.57 (86.010) | 0.0988 (0.5707) |
EL(ϕ, σ, τ) | 1.9150 (0.3500) | 22971.15 (3209.5) | 32882 (162.230) | 0.1298 (0.2391) | |
BL(ϕ, α, σ, τ) | 4.9700 (50.530) | 169.570 (339.210) | 1.9300 (0.3200) | 31.26 (316.84) | 0.1237 (0.2904) |
GL(α, σ, τ) | 1.9100 (0.3200) | 35842.4 (6945.10) | 39197.6 (151.7) | 0.1227 (0.2993) | |
L(σ, τ) | 99269 (11863.5) | 207019.4 (301.20) | 0.1919 (0.0193) |
Model | Estimates | K-S (p-value) | |||
LTLo(ϕ,σ,τ) | 1.158 (1.256) | 136.941 (639.186) | 174.126 (857.591) | 0.0940 (0.8216) | |
KL(ϕ, α, σ, τ) | 1.054 (0.352) | 140.562 (25.321) | 47.377 (1.0253) | 7073.1 (352.5) | 0.1076 (0.6743) |
EL(ϕ, σ, τ) | 1.1340 (0.243) | 30.246 (4.222) | 36.276 (5.930) | 0.1079 (0.6324) | |
BL(ϕ, α, σ, τ) | 1.1194 (0.221) | 11.537 (8.468) | 4.1860 (5.700) | 56.945 (3.381) | 0.1095 (0.6144) |
GL(α, σ, τ) | 1.1525 (0.2423) | 17.185 (3.067) | 18.787 (4.044) | 0.1087 (0.6229) | |
L(σ, τ) | 132.7 (565.6) | 176.898 (759.2) | 0.1895 (0.0790) |
Model | Data I | Data II | ||||||||||
AIC | BIC | CAIC | HQIC | A∗ | W∗ | AIC | BIC | CAIC | HQIC | A∗ | W∗ | |
LTLo | 203.9 | 210.4 | 204.4 | 206.5 | 0.311 | 0.04 | 122.5 | 127.9 | 123.1 | 124.5 | 0.510 | 0.075 |
KL | 209.7 | 218.3 | 210.4 | 213.1 | 0.740 | 0.122 | 124.2 | 131.4 | 125.2 | 126.9 | 0.544 | 0.081 |
EL | 213.1 | 219.5 | 213.5 | 215.6 | 1.230 | 0.204 | 122.3 | 127.7 | 122.9 | 124.3 | 0.515 | 0.076 |
BL | 213.9 | 222.4 | 214.6 | 217.2 | 1.130 | 0.186 | 124.2 | 131.5 | 125.3 | 126.9 | 0.522 | 0.077 |
GL | 211.7 | 218.1 | 212.1 | 214.2 | 1.110 | 0.200 | 122.4 | 127.8 | 123.0 | 124.4 | 0.511 | 0.076 |
L | 222.6 | 226.9 | 223.0 | 224.3 | 1.130 | 0.190 | 237.2 | 241.5 | 237.4 | 238.9 | 1.624 | 0.206 |
Data | Test value | p-value |
I | 17.558 | 0.000 |
II | 114.70 | 0.000 |
Model | Estimates (ϕ,σ,τ) | K-S (p-value) | ||
MLEs | 814.15 | 156.240 | 85.750 | 0.0839 (0.7668) |
LSEs | 858.79 | 154.470 | 87.170 | 0.0755 (0.8656) |
WLSEs | 916.34 | 156.880 | 87.390 | 0.0763 (0.8567) |
MPSEs | 814.95 | 154.192 | 82.686 | 0.0922 (0.6585) |
Model | Estimates (ϕ,σ,τ) | K-S (p-value) | ||
MLEs | 1.158 | 136.941 | 174.126 | 0.0940 (0.8216) |
LSEs | 0.362 | 128.573 | 244.201 | 0.0790 (0.9414) |
WLSEs | 0.960 | 134.956 | 188.283 | 0.0972 (0.7885) |
MPSEs | 0.982 | 136.635 | 176.550 | 0.0796 (0.9382) |
Estimates | MSE | ||||||||
n | Parameter | MLEs | LSEs | WLSEs | MPSEs | MLE | LSE | WLSE | MPSE |
20 | ϕ | 2.208 | 2.193 | 2.203 | 2.226 | 2.293 | 2.273 | 2.214 | 2.575 |
σ | 2.645 | 2.147 | 2.204 | 3.079 | 1.349 | 1.161 | 1.100 | 1.951 | |
τ | 3.175 | 2.540 | 2.628 | 3.439 | 2.526 | 1.749 | 1.853 | 2.726 | |
50 | ϕ | 2.013 | 2.069 | 2.142 | 1.965 | 1.843 | 1.860 | 1.904 | 1.854 |
σ | 2.473 | 2.141 | 2.174 | 2.802 | 0.807 | 0.757 | 0.694 | 1.212 | |
τ | 3.054 | 2.477 | 2.508 | 3.453 | 2.334 | 1.528 | 1.601 | 2.581 | |
100 | ϕ | 1.959 | 2.012 | 2.045 | 1.947 | 1.725 | 1.680 | 1.697 | 1.649 |
σ | 2.354 | 2.122 | 2.163 | 2.591 | 0.472 | 0.506 | 0.425 | 0.725 | |
bτ | 2.922 | 2.394 | 2.409 | 3.291 | 2.059 | 1.396 | 1.431 | 2.321 | |
150 | ϕ | 1.943 | 1.974 | 1.992 | 1.957 | 1.717 | 1.506 | 1.525 | 1.581 |
σ | 2.262 | 2.118 | 2.201 | 2.458 | 0.301 | 0.346 | 0.291 | 0.465 | |
τ | 2.829 | 2.317 | 2.346 | 3.209 | 1.866 | 1.213 | 1.317 | 2.416 | |
200 | ϕ | 1.953 | 1.981 | 1.941 | 1.921 | 1.693 | 1.368 | 1.462 | 1.438 |
σ | 2.237 | 2.175 | 2.184 | 2.325 | 0.245 | 0.279 | 0.225 | 0.381 | |
τ | 2.790 | 2.264 | 2.515 | 3.133 | 1.812 | 1.108 | 1.237 | 2.352 | |
250 | ϕ | 1.960 | 1.986 | 1.966 | 1.918 | 1.551 | 1.302 | 1.317 | 1.301 |
σ | 2.117 | 2.108 | 2.130 | 2.274 | 0.213 | 0.213 | 0.179 | 0.316 | |
τ | 2.315 | 2.221 | 2.363 | 2.700 | 1.673 | 1.076 | 1.178 | 2.037 |
Estimates | MSE | ||||||||
n | Parameter | MLEs | LSEs | WLSEs | MPSEs | MLE | LSE | WLSE | MPSE |
20 | ϕ | 2.154 | 1.853 | 1.805 | 2.737 | 2.509 | 2.011 | 2.083 | 2.993 |
σ | 0.596 | 0.519 | 0.517 | 0.702 | 0.076 | 0.072 | 0.063 | 0.130 | |
τ | 1.362 | 1.142 | 1.219 | 1.148 | 1.111 | 0.802 | 0.896 | 0.848 | |
50 | ϕ | 1.738 | 1.581 | 1.557 | 2.212 | 1.513 | 1.259 | 1.316 | 1.689 |
σ | 0.531 | 0.504 | 0.505 | 0.586 | 0.021 | 0.022 | 0.020 | 0.029 | |
τ | 1.249 | 1.137 | 1.182 | 1.052 | 0.686 | 0.524 | 0.578 | 0.448 | |
100 | ϕ | 1.590 | 1.490 | 1.483 | 1.912 | 1.109 | 0.955 | 1.015 | 1.131 |
σ | 0.504 | 0.491 | 0.492 | 0.537 | 0.009 | 0.010 | 0.009 | 0.010 | |
τ | 1.119 | 1.058 | 1.095 | 0.988 | 0.425 | 0.345 | 0.388 | 0.301 | |
150 | ϕ | 1.542 | 1.496 | 1.487 | 1.811 | 0.880 | 0.755 | 0.773 | 0.839 |
σ | 0.498 | 0.497 | 0.493 | 0.524 | 0.006 | 0.007 | 0.006 | 0.006 | |
τ | 1.038 | 0.984 | 1.007 | 0.928 | 0.288 | 0.226 | 0.246 | 0.199 | |
200 | ϕ | 1.480 | 1.497 | 1.494 | 1.674 | 0.653 | 0.609 | 0.614 | 0.610 |
σ | 0.497 | 0.498 | 0.491 | 0.517 | 0.004 | 0.005 | 0.004 | 0.004 | |
τ | 1.002 | 0.976 | 0.988 | 0.915 | 0.195 | 0.173 | 0.182 | 0.148 | |
250 | ϕ | 1.495 | 1.498 | 1.493 | 1.547 | 0.420 | 0.411 | 0.404 | 0.337 |
σ | 0.495 | 0.499 | 0.498 | 0.510 | 0.003 | 0.004 | 0.003 | 0.003 | |
τ | 0.931 | 0.928 | 0.933 | 0.885 | 0.093 | 0.096 | 0.097 | 0.075 |
Estimates | MSE | ||||||||
n | Parameter | MLEs | LSEs | WLSEs | MPSEs | MLE | LSE | WLSE | MPSE |
20 | ϕ | 2.080 | 2.131 | 1.664 | 2.118 | 2.862 | 2.233 | 2.182 | 3.265 |
σ | 2.193 | 1.799 | 1.758 | 2.617 | 1.245 | 0.834 | 0.842 | 1.887 | |
τ | 2.205 | 1.562 | 1.467 | 2.463 | 2.209 | 0.984 | 1.362 | 2.623 | |
50 | ϕ | 2.256 | 2.209 | 2.270 | 2.191 | 2.748 | 2.200 | 2.756 | 3.042 |
σ | 1.883 | 1.682 | 1.703 | 2.163 | 0.804 | 0.455 | 0.748 | 0.796 | |
τ | 2.050 | 1.406 | 1.431 | 2.291 | 1.778 | 0.876 | 1.575 | 2.333 | |
100 | ϕ | 2.357 | 2.378 | 2.342 | 2.354 | 2.266 | 2.071 | 2.356 | 2.468 |
σ | 1.744 | 1.675 | 1.695 | 2.542 | 0.587 | 0.369 | 0.510 | 0.555 | |
τ | 1.692 | 1.361 | 1.353 | 1.684 | 1.010 | 0.827 | 0.820 | 0.988 | |
150 | ϕ | 2.401 | 2.419 | 2.367 | 2.692 | 1.391 | 1.006 | 1.004 | 1.592 |
σ | 1.659 | 1.643 | 1.645 | 1.778 | 0.155 | 0.114 | 0.164 | 0.228 | |
τ | 1.404 | 1.261 | 1.272 | 1.582 | 0.363 | 0.225 | 0.225 | 0.524 | |
200 | ϕ | 2.421 | 2.442 | 2.433 | 2.398 | 0.941 | 0.810 | 0.810 | 0.995 |
σ | 1.598 | 1.539 | 1.564 | 1.658 | 0.063 | 0.060 | 0.078 | 0.083 | |
τ | 1.245 | 1.154 | 1.169 | 1.300 | 0.121 | 0.077 | 0.086 | 0.144 | |
250 | ϕ | 2.429 | 2.468 | 2.452 | 2.443 | 0.570 | 0.681 | 0.695 | 0.566 |
σ | 1.479 | 1.486 | 1.465 | 1.540 | 0.033 | 0.025 | 0.045 | 0.031 | |
τ | 1.074 | 1.022 | 1.054 | 1.094 | 0.025 | 0.020 | 0.021 | 0.027 |
Model | Estimates | K-S (p-value) | |||
LTLo(ϕ,σ,τ) | 814.15 (2249.9) | 156.240 (449.800) | 85.750 (265.21) | 0.0839 (0.7668) | |
KL(ϕ, α, σ, τ) | 2.5700 (4.7600) | 65.0600 (177.600) | 1.6700 (0.2600) | 60.57 (86.010) | 0.0988 (0.5707) |
EL(ϕ, σ, τ) | 1.9150 (0.3500) | 22971.15 (3209.5) | 32882 (162.230) | 0.1298 (0.2391) | |
BL(ϕ, α, σ, τ) | 4.9700 (50.530) | 169.570 (339.210) | 1.9300 (0.3200) | 31.26 (316.84) | 0.1237 (0.2904) |
GL(α, σ, τ) | 1.9100 (0.3200) | 35842.4 (6945.10) | 39197.6 (151.7) | 0.1227 (0.2993) | |
L(σ, τ) | 99269 (11863.5) | 207019.4 (301.20) | 0.1919 (0.0193) |
Model | Estimates | K-S (p-value) | |||
LTLo(ϕ,σ,τ) | 1.158 (1.256) | 136.941 (639.186) | 174.126 (857.591) | 0.0940 (0.8216) | |
KL(ϕ, α, σ, τ) | 1.054 (0.352) | 140.562 (25.321) | 47.377 (1.0253) | 7073.1 (352.5) | 0.1076 (0.6743) |
EL(ϕ, σ, τ) | 1.1340 (0.243) | 30.246 (4.222) | 36.276 (5.930) | 0.1079 (0.6324) | |
BL(ϕ, α, σ, τ) | 1.1194 (0.221) | 11.537 (8.468) | 4.1860 (5.700) | 56.945 (3.381) | 0.1095 (0.6144) |
GL(α, σ, τ) | 1.1525 (0.2423) | 17.185 (3.067) | 18.787 (4.044) | 0.1087 (0.6229) | |
L(σ, τ) | 132.7 (565.6) | 176.898 (759.2) | 0.1895 (0.0790) |
Model | Data I | Data II | ||||||||||
AIC | BIC | CAIC | HQIC | A∗ | W∗ | AIC | BIC | CAIC | HQIC | A∗ | W∗ | |
LTLo | 203.9 | 210.4 | 204.4 | 206.5 | 0.311 | 0.04 | 122.5 | 127.9 | 123.1 | 124.5 | 0.510 | 0.075 |
KL | 209.7 | 218.3 | 210.4 | 213.1 | 0.740 | 0.122 | 124.2 | 131.4 | 125.2 | 126.9 | 0.544 | 0.081 |
EL | 213.1 | 219.5 | 213.5 | 215.6 | 1.230 | 0.204 | 122.3 | 127.7 | 122.9 | 124.3 | 0.515 | 0.076 |
BL | 213.9 | 222.4 | 214.6 | 217.2 | 1.130 | 0.186 | 124.2 | 131.5 | 125.3 | 126.9 | 0.522 | 0.077 |
GL | 211.7 | 218.1 | 212.1 | 214.2 | 1.110 | 0.200 | 122.4 | 127.8 | 123.0 | 124.4 | 0.511 | 0.076 |
L | 222.6 | 226.9 | 223.0 | 224.3 | 1.130 | 0.190 | 237.2 | 241.5 | 237.4 | 238.9 | 1.624 | 0.206 |
Data | Test value | p-value |
I | 17.558 | 0.000 |
II | 114.70 | 0.000 |
Model | Estimates (ϕ,σ,τ) | K-S (p-value) | ||
MLEs | 814.15 | 156.240 | 85.750 | 0.0839 (0.7668) |
LSEs | 858.79 | 154.470 | 87.170 | 0.0755 (0.8656) |
WLSEs | 916.34 | 156.880 | 87.390 | 0.0763 (0.8567) |
MPSEs | 814.95 | 154.192 | 82.686 | 0.0922 (0.6585) |
Model | Estimates (ϕ,σ,τ) | K-S (p-value) | ||
MLEs | 1.158 | 136.941 | 174.126 | 0.0940 (0.8216) |
LSEs | 0.362 | 128.573 | 244.201 | 0.0790 (0.9414) |
WLSEs | 0.960 | 134.956 | 188.283 | 0.0972 (0.7885) |
MPSEs | 0.982 | 136.635 | 176.550 | 0.0796 (0.9382) |