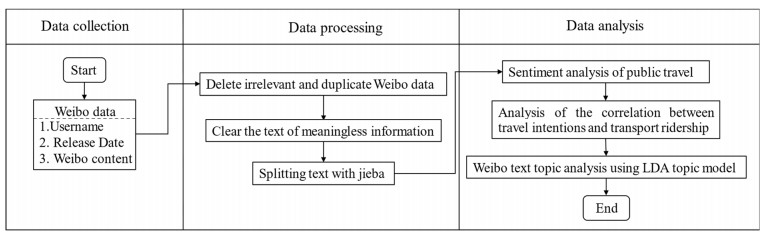
Citation: Xiaoyue Xie, Jian Shi. A distributed quantile estimation algorithm of heavy-tailed distribution with massive datasets[J]. Mathematical Biosciences and Engineering, 2021, 18(1): 214-230. doi: 10.3934/mbe.2021011
[1] | Gang Cheng, Changliang He . Analysis of bus travel characteristics and predictions of elderly passenger flow based on smart card data. Electronic Research Archive, 2022, 30(12): 4256-4276. doi: 10.3934/era.2022217 |
[2] | Peiqun Lin, Xuanyi Liu, Mingyang Pei, Pan Wu . Revealing the spatial variation in vehicle travel time with weather and driver travel frequency impacts: Findings from the Guangdong–Hong Kong–Macao Greater Bay Area, China. Electronic Research Archive, 2022, 30(10): 3711-3734. doi: 10.3934/era.2022190 |
[3] | Nuri Park, Junhan Cho, Juneyoung Park . Assessing crash severity of urban roads with data mining techniques using big data from in-vehicle dashcam. Electronic Research Archive, 2024, 32(1): 584-607. doi: 10.3934/era.2024029 |
[4] | Jiping Xing, Xiaohong Jiang, Yu Yuan, Wei Liu . Incorporating mobile phone data-based travel mobility analysis of metro ridership in aboveground and underground layers. Electronic Research Archive, 2024, 32(7): 4472-4494. doi: 10.3934/era.2024202 |
[5] | Jiping Xing, Yunchi Wu, Di Huang, Xin Liu . Transfer learning for robust urban network-wide traffic volume estimation with uncertain detector deployment scheme. Electronic Research Archive, 2023, 31(1): 207-228. doi: 10.3934/era.2023011 |
[6] | Yanling Dong, Xiaolan Zhou . Advancements in AI-driven multilingual comprehension for social robot interactions: An extensive review. Electronic Research Archive, 2023, 31(11): 6600-6633. doi: 10.3934/era.2023334 |
[7] | Yang Yang, Yun-Rui Yang, Xin-Jun Jiao . Traveling waves for a nonlocal dispersal SIR model equipped delay and generalized incidence. Electronic Research Archive, 2020, 28(1): 1-13. doi: 10.3934/era.2020001 |
[8] | Chuanyao Li, Yichao Lu, Yuqiang Wang, Gege Jiang . Congestion behavior and tolling strategies in a bottleneck model with exponential scheduling preference. Electronic Research Archive, 2023, 31(2): 1065-1088. doi: 10.3934/era.2023053 |
[9] | Shao-Xia Qiao, Li-Jun Du . Propagation dynamics of nonlocal dispersal equations with inhomogeneous bistable nonlinearity. Electronic Research Archive, 2021, 29(3): 2269-2291. doi: 10.3934/era.2020116 |
[10] | Cui-Ping Cheng, Ruo-Fan An . Global stability of traveling wave fronts in a two-dimensional lattice dynamical system with global interaction. Electronic Research Archive, 2021, 29(5): 3535-3550. doi: 10.3934/era.2021051 |
COVID-19 has severely impacted transport, with public transport passenger traffic in 36 central cities nationwide standing at 44.15 billion in 2020, down 33.7% year-on-year, according to the Ministry of Transport of the People's Republic of China. With COVID-19 under effective control in China, the national COVID-19 prevention and control have entered a normalized phase since April 29th, 2020 [1], i.e., it has entered the post-COVID-19 era [2]. However, the rebound of COVID-19 in local areas has caused ups and downs in the public's willingness to travel, resulting in fluctuations in the recovery of urban public transport. Therefore, studying the public's willingness to travel in the post-COVID-19 era provides data support for grasping public travel choices and responding to public health emergencies, and providing a reference for the development of urban public transportation in the post-COVID-19 era.
COVID-19 has devastated the entire world, and people's lifestyles, work styles, and travel behaviors are bound to change significantly. Travel willingness is an antecedent variable of travel behavior, which will have a significant effect on the final travel behavior, and therefore travel willingness is related to urban public transportation ridership [3,4,5]. Some studies have shown that the public's willingness to travel and public transport ridership dropped significantly during the COVID-19 outbreak [6,7,8]. Because the main disadvantage of public transportation is the small physical distance, some travelers who usually commute and shop by public transit are shifting their trips to private cars or micro-trips [9,10]. Additional studies have shown that if the development of COVID-19 gradually stabilizes and the safety and hygiene of the general public traveling can be ensured, the willingness of individuals to travel will rise, and public transportation ridership will rebound but still less willing to travel than before the outbreak [11].
Most existing studies have used questionnaire methods [12,13,14,15,16,17,18]to obtain data, and the same is valid for studies addressing travel willingness s in the post-COVID-19 era [19,20,21,22,23,24]. However, the high reliance on the questionnaire method may lead to several limitations, such as relatively low response rates, random bias in the data, and cumbersome data collection and processing [25].
In the wake of the COVID-19, social media platforms represented by Weibo have become the main channel for the public to exchange views and express opinions, promoting the social spread of personal concerns and emotions. As one of China's most influential social media platforms, Weibo is an effective and reliable data source with a wide range of users, many texts, and high timeliness of the content. The use of social text mining techniques to obtain information of interest or usefulness from Weibo data has been applied in the research on COVID-19. Cao et al. [26] explored the pattern of sentiment evolution by studying public sentiment in online discussions on Sina Weibo during the Wuhan city closure in January 2020. Hou et al. [27] conducted a public attention analysis based on Weibo texts during COVID-19 to understand public sentiment comprehensively. Viviani et al. [28] assessed the potential psychological vulnerability of residents during COVID-19 by analyzing the content of Weibo. Shao et al. [29] studied the relationship between public sentiment changes and agricultural price fluctuations during COVID-19. Ge et al. [30] used data from comments on public opinion under the road passenger transport policy during COVID-19 to conduct a mining analysis of residents' travel demand and concerns.
In summary, the established literature has examined the impact of the outbreak of COVID-19 on the public's willingness to travel. However, changes in the outbreak and targeted prevention and control measures have yet to be considered. The corresponding travel willingness of the people is dynamic and gradually leveling off, with a gradual return to the pre-COVID-19 period [31,32]. Although COVID-19 is now effectively under control, the duration of the COVID-19 and the regional risk level are still unclear, and residents' travel willingness and sentiments in the post-COVID-19 era need to be further studied. Traditional questionnaires cannot circumvent the subjectivity of researchers' judgments, and using big data to investigate changes in the public's travel willingness can compensate for the lack of research in this area to a certain extent. Therefore, this study uses web crawler technology to obtain raw text data from Weibo, analyses the changing trend of public travel willingness in the post-COVID-19 era through text mining, and investigates the correlation between urban public transport ridership and general travel willingness, as well as the influencing factors of travel willingness. The study provides data support and directional guidance for the recovery and stimulation of urban public transport in the post-COVID-19 era.
Compared to traditional survey methods, obtaining data through social media is efficient and inexpensive, while social media has become a popular channel for collecting public opinion due to its large volume of data and accessibility features [33]. However, existing studies need more effective methods to extract and interpret social media data. Therefore, this study constructs a comprehensive framework (see Figure 1) that enables efficient extraction and analysis of public travel willingness from Weibo.
The primary construction process consists of four steps: 1) collecting text posted during a specific period of time; 2) pre-processing the text to retain text that is meaningful for subsequent analysis; 3) labeling the data and training a machine learning model to extract text relevant to travel sentiment from the original Weibo dataset; and 4) using text mining techniques and quantitative metrics to present and interpret the data.
A total of 15,666 original tweets related to travel during the COVID-19 were collected, using "travel during the COVID-19", "going out during the COVID-19", "travel in the post-COVID-19 era" and "going out in the post-COVID-19 era" as keywords, from December 27th, 2019 to April 28th, 2020 and from April 29th, 2020 to October 31th, 2021, with a total of 15,666 original Weibo, extracting information on user ID, Weibo body and posting time.
Data pre-processing is carried out, first removing duplicate data and retaining the first occurrence of the text. Then remove text data that needs to be more confusingly formatted, missing content, and irrelevant. Finally, the text is cleaned of meaningless information, including special symbols, usernames, and emoticons. Jieba in Python was used to segment the text. To accurately analyze the public's travel sentiment, this study first merged the list of deactivated words from Baidu, Harbin Institute of Technology, and Sichuan University Machine Intelligence Laboratory, then removed some negative words, negative and positive feeling words, and finally kept expanding the list of non-indexed words based on the separation results until the word separation effect could meet the model requirements.
Naive Bayes can be used for Weibo text sentiment classification, a widely used classification algorithm in machine learning and data mining, with the advantages of a simple algorithm, high classification accuracy, and fast speed [34]. It first calculates the conditional probability that the sample to be judged belongs to each category based on Bayes' theorem and the assumption of conditional independence of attributes and then determines it as the category with the highest probability.
In statistics, Spearman's correlation is used to estimate the correlation between two variables [35], and it is applied to the analysis of both continuous and discrete variables. Let the binary sample $ \{\left({X}_{i}, {Y}_{i}\right), 1\le i\le n\} $ be from the samples of the overall $ X $, $ Y $, respectively, and let $ R\left({x}_{j}\right) $, $ R\left({y}_{j}\right) $ denote the rank of $ {x}_{j} $, $ {y}_{j} $ in $ \{\left({X}_{i}, {Y}_{i}\right), 1\le i\le n\} $, respectively, then the Spearman's rank correlation coefficient between $ X $ and $ Y $ is defined as:
$ r\left(X, Y\right) = 1-\frac{6{\sum }_{j = 1}^{n}{d}_{j}^{2}}{n({n}^{2}-1)} $ | (1) |
where, $ {d}_{j} = R\left({x}_{j}\right)-R\left({y}_{j}\right), j = 1, 2, \cdots, n $.
LDA (Latent Dirichlet allocation), or latent Dirichlet allocation, was originally proposed by Blei et al. and is one of the most widely used probabilistic topic models [36]. It is a Bayesian probabilistic model that contains a three-level structure of words, topics and documents, and the main purpose of LDA is to identify potential topics based on the word frequency of each document. It can therefore be considered as an unsupervised generative topic model that uses a specified corpus to estimate the topic probability distribution for each document $ \theta $ and the per-topic word $ \varphi $ probability distribution. It is assumed that each document is generated by the process shown in Figure 2, where K denotes the number of topics, D denotes the number of documents in the corpus, N denotes the number of words in the documents, and the white dashed circles, white solid circles and grey solid circles represent hyperparameters, latent variables, and observed variables respectively.
The development of the COVID-19 situation affects the public's willingness to travel, and the Weibo text reflects a certain extent, the fluctuation of the public's willingness to travel due to the change in the COVID-19 situation. This paper focuses on implementing a Naive Bayes sentiment propensity analysis using the SnowNLP library.
The study randomly selected and manually labeled 500 Weibo as the pre-annotated dataset. The pre-annotations contained four categories: 100 texts annotated as off-topic, 107 texts annotated with negative attitudes, 80 texts with neutral attitudes, and 113 texts expressing positive attitudes. The category of neutral attitudes refers to texts expressing indifferent opinions or stating objective facts. Table 1 shows examples of manually annotated texts in the annotated dataset. The annotated set was used as the training set to train the naive Bayesian classification model, and the training set was used to evaluate accuracy. The best-performing classifier is applied to the entire dataset by tuning the hyperparameters. The trained classifier was tested at 0.8275 by the model accuracy test, indicating a good classification result.
Authors | Research Subjects | Research Period | Research Methods |
Raymond et al. [12] (2021) | The impact of COVID-19 morbidity and mortality on travel willingness | During the COVID-19 | Multi-Cluster Analysis Method |
Yang et al. [13] (2021) | Influence of passenger perception of safety on willingness to travel by air | During the COVID-19 | Structural Equation Model (SEM) |
Shi et al. [14] (2021) | Factors and Mechanisms of Influence of the COVID-19 on Residents' Willingness to Travel for Leisure | During the COVID-19 | SEM |
Liang et al. [15] (2022) | Residents' willingness to travel under the COVID-19 | During the COVID-19 | SEM |
Mehrbakhsh et al. [16] (2022) | The influence of opinions in social media on travel decisions | During the COVID-19 | SEM |
Francene et al. [17] (2023) | Change in travel attitudes due to the COVID-19 | During the COVID-19 | SEM |
Jordan et al. [18] (2023) | Influence of college students' perceived risk of the COVID-19 on their willingness to travel | During the COVID-19 | SEM |
Zhang et al. [19] (2022) | People's willingness to travel to urban parks | Post-COVID-19 era | SEM |
Zhao et al. [20] (2022) | Residents' travel mentality | Post-COVID-19 era | Theory of Planned Behavior |
Sun et al. [21] (2022) | The effect of people's trust in destination risk regulation on their willingness to travel | Post-COVID-19 era | SEM |
Vikram et al. [22] (2022) | Impact of the COVID-19 on Commuters' Travel willingness in Delhi, India | Post-COVID-19 era | SEM |
Shalini et al. [23] (2022) | Influence of personality traits on willingness to travel | Post-COVID-19 era | Artificial Neural Networks |
Yao et al. [24] (2023) | How to stimulate people's willingness to travel | Post-COVID-19 era | SEM |
The results of the Weibo text sentiment classification during the COVID-19 (D) and post-COVID-19 era (P) are shown in Table 2, with the mean values of effective travel sentiment during the COVID-19 and post-COVID-19 era being -0.8197 and -0.0640 respectively. This data refers to the ordinary people's sentiment toward travel during the COVID-19 and post-COVID-19 era. The mean value of -0.8197 during the COVID-19 period means that people's sentiment toward travel is generally negative, probably due to the impact of COVID-19, which makes people reluctant to travel or feel the risk and inconvenience of travel. The mean value for the post-COVID-19 period is -0.0640, meaning that people's sentiment toward travel has gradually recovered, probably because COVID-19 is under control or people have gradually adapted to the changes in their lives brought about by COVID-19. This data can reflect the impact of COVID-19 on people's emotions and behavior.
Off-topic: as soon as we left the house, the wind was howling and the thunderstorm was in full force. | ||
Tangential to the theme: wash your hands regularly, get plenty of ventilation, get vaccinated and wear a mask when you go out! | ||
Positive: #GuangzhouOutbreak #Get out and protect yourself, whether you're in a medium-risk area or not! ~Believe Guangzhou will survive the second wave of the outbreak! | Neutral: #Foshan Nanhai District 1 site upgraded to medium risk #Wear a mask when you go out and try not to go out and gather together if not necessary | Negative: #Foshan Nanhai District 1 site upgraded to medium risk #dare not go out |
Figure 3 shows the general trend of the public's change in travel sentiment during the COVID-19 and post-COVID-19 era. During COVID-19, the public's emotions about traveling did not fluctuate much. However, they were in negative emotions most of the time because the government advocated "home quarantine to reduce unnecessary travel", and prolonged home stay would cause negative psychological reactions, such as anxiety and fear, which would affect the public's willingness to travel. After the outbreak, the social media platforms represented by microblogs showed an "explosion" of group communication and promoted the socialization of individual emotions, and the emotional experience caused by the outbreak spread to the population through mass communication.
In the post-COVID-19 era, the public's travel emotions fluctuated, with positive and negative emotions alternating, i.e., in the temporal dimension, the daily sentiment trend indicated that the public went through a stage of emotional turnover between fearful and nervous and smooth and confident. However, there is still some time in negative and negative emotions, indicating that the public's fear of infection for travel still exists, although it has improved significantly.
The positive emotional high-frequency words for public travel in the post-COVID-19 era are shown in Figure 4. The words safety, health, strict, service, and happy reflect the public's confidence in preventing and controlling COVID-19, their feeling of safety in the travel environment, and their expectation of travel service quality in the post-COVID-19 era. People pay more attention to the safety and health of travel, have higher requirements for the hygiene of transportation and places, and expect more stringent supervision and service guarantee. Words such as happy, pleasant, and comfortable indicate that the public also has higher expectations for travel experiences and wants to enjoy better services and travel experiences. Therefore the strength of prevention and control of COVID-19 and the degree of implementation are essential factors affecting residents' travel.
The positive emotional high-frequency words of public travel in the post-COVID-19 era are shown in Figure 5. Words such as mask, risk, isolation, and time reflect the public's concern about travel in the post-COVID-19 era, showing fear of infection, so the level of COVID-19 prevention and control in their area and the travel time of transportation become the public's concern about travel in the post-COVID-19 era.
To analyze the correlation between the public's willingness to travel and urban public transport ridership under COVID-19, this study replaced the public's willingness to travel with the daily positive and negative sentiment volumes obtained in Section 4.1. It used SPSS statistical software to conduct a Spearman correlation data analysis. Data on urban public transport ridership were obtained from the Ministry of Transport, and the current study included 670 days of data, i.e., from January 1th, 2020 to April 28th, 2020 and from April 29th, 2020 to October 31th, 2021.
Table 3 shows the Spearman correlation between public travel willingness and urban public transport ridership. The table shows that positive public sentiment is positively and significantly correlated with urban public transport ridership.
Category | Off-topic | Relevant to the topic | Total | ||||||||||
Periods | D | P | D | P | D | P | |||||||
Counts | 1136 | 2081 | 7608 | 4841 | 8744 | 6922 | |||||||
Percentage | 12.99% | 30.06% | 87.01% | 69.94% | 100% | 100% | |||||||
Category | Positive | Neutral | Negative | Total | |||||||||
Periods | D | P | D | P | D | P | D | P | |||||
Counts | 516 | 1888 | 340 | 755 | 6752 | 2198 | 7608 | 4841 | |||||
Percentage | 6.78% | 39.00% | 4.67% | 15.60% | 88.75% | 45.40% | 100% | 100% |
On the other hand, negative public sentiment is negatively correlated with urban public transport ridership, with both positive and negative sentiment having a relatively small effect on taxi ridership, which is related to the fact that taxis have a relatively safe riding environment and are an important mode of travel for the public in the COVID-19.
And as seen in Figure 6, the trend of urban public transport ridership is almost the same as the trend of the public's positive travel willingness, while it is the opposite of the trend of the public's negative travel willingness.
And it can be seen from Figure 6 that the trend of the change in urban public transportation passenger ridership is almost the same as the trend of the change in the public's positive willingness to travel. In contrast, it is the opposite of the trend of the change in the public's negative willingness to travel because there is a close correlation between the shift in public transportation passenger ridership and the difference in public willingness to travel. When the public's demand for travel increases, public transportation passenger ridership will increase accordingly, while when the public's demand for travel decreases, public transportation passenger ridership will decrease. Therefore, public transportation passenger ridership is almost the same as the public's positive willingness to travel because the public's positive willingness to travel will promote the increase of public transportation passenger ridership. On the contrary, negative public willingness to travel leads to a decrease in public transportation passenger ridership, so the trend of public transportation passenger ridership is precisely opposite to the trend of negative public willingness to travel.
Under the influence of COVID-19, travelers perceived a higher likelihood of being infected in public transportation than traveling by other means of transportation. As shown in the above study results, travelers who perceived a higher risk of infection in public transit were less likely to use it, and those who did not travel by public transportation chose private cars and cabs instead.
When modeling LDA, the number of topics varies dynamically with the data sample, so it is necessary first to determine the number of topics that makes the model optimal. The optimal number of topics, K, is selected using a commonly used evaluation criterion in statistical language models, the "confusion level" [37]. In general, the smaller the perplexity value, the higher the model's performance in generating documents and the better the model's generalizability. The value of K for the optimal number of topics can be obtained by calculating and analyzing the perplexity by taking different values for K, provided that the other parameters are determined.
COVID-19, the first phase of the theme analysis, runs from January 1th, 2020, to April 28th, 2020. In Figure 7(a) of the theme number confusion degree line, as the value of theme number K increases, the confusion degree gradually decreases, and when K is 6, there is a significant inflection point. Therefore, inflection point 6 is the optimal value of topic K. The number of topics generated during COVID-19 was also selected to be 6. To find popular topics from the six topics in Table 4, this study obtained the probability of each tweet belonging to a different topic based on the document-topic probability distribution to calculate each topic's intensity and intensity threshold.
Variables | Correlation coefficient | |
The Volume of positive emotions | Public transport ridership | 0.848** |
Rail ridership | 0.829** | |
Taxi ridership | 0.612** | |
The Volume of negative emotions | Public transport ridership | -0.754** |
Rail ridership | -0.765** | |
Taxi ridership | -0.526* | |
Note: **: At the 0.01 level (two-tailed), the correlation is significant; *: At the 0.05 level (two-tailed), the correlation is significant. |
Figure 8(a) shows the distribution of intensity values for each case, with the vertical axis indicating the topic intensity value and the horizontal line marking the topic intensity threshold (15%). Themes 2, 3, and 5 have topic intensity values above the threshold, i.e., travel prevention, life prevention, and travel elements are the top topics in the current situation. Therefore, people pay more attention to personal protection and the spatial distance and time of transportation when traveling, such as carrying good masks, wet and dry tissues, hand-free disinfectant water, etc., and avoiding direct contact with equipment in the car as much as possible, as well as avoiding talking with others.
Topic | Topic Name | LDA Keywords | ||
Periods | D | P | D | P |
1 | Action against the COVID-19 | COVID-19 prevention policy | Action; Ambulance; Meaning; Infected Area | Mask; Region; New crown; Prevention and control; Vaccine; High risk |
2 | Traveling against the COVID-19 | Travel Risks | Prevention and control; Taxis; Disinfection; Personnel; Vehicles; Masks | High Risk; Cases; Local Reports; Impact; Travel |
3 | Living with the COVID-19 | Life's Little Things | Masks; life; hand washing; public good; feeling; time | Life; time; time; video; things; home |
4 | Webcasts | Nucleic Acid Testing | Video; web page; COVID-19 prevention; links; campaign; live | nucleic acid; people; cases; region; prevention control; high risk |
5 | Travel Essentials | Prevention and Control Management | Public transport; Subway; Passengers; Operation; Control; Time | prevention and control; people; measures; management; people |
6 | COVID-19 Prevention and Control | Outbreak Prevention and Control | Cases; Patients; Masks; Neighborhoods; Home; Community | Streets; Communities; Neighborhoods; Areas; Vehicles; Prevention and Control |
7 | - | Travel and Tourism | Development; Tourism; Enterprises; Services; Markets; Attractions | |
8 | - | Travel for the Elderly | Transport; Sector; Services; elderly; Passenger transport; Area |
The second phase of the thematic analysis is the post-COVID-19 era: April 29th, 2020 to October 31th, 2021. In Figure 7(b) of the theme count-confusion line, the confusion level decreases as the value of theme count K increases, and there is a significant inflection point when K is 8. Therefore, the inflection point 8 is the optimal value for topic K. Therefore, the number of topics generated in the post-COVID-19 era was also selected to be 8. To find popular topics from the 8 topics in Table 4, this study obtained the probability of each tweet belonging to different topics based on the document-topic probability distribution to calculate the intensity and intensity threshold for each topic. Figure 8(b) shows the distribution of intensity values for each topic, with the vertical axis indicating the topic intensity value and the horizontal line indicating the topic intensity threshold (10%). Topic intensity values for Topics 1–4 are above the threshold, i.e., COVID-19 prevention policies, travel risks, life hacks, and nucleic acid testing are popular topics in the current context. The traveling public in the post-COVID-19 era is more concerned about the risk dynamics of the destination COVID-19 and the latest COVID-19 prevention policies and is also very worried about the travel use of nucleic acid testing certificates.
This paper analyzes the public's willingness to travel in the post-COVID-19 era based on Weibo social text data, combined with LDA thematic model analysis, to obtain the public group's emotional tendency, factors affecting the public's travel emotion, and the public's travel emotion fluctuation in the post-COVID-19 era through naive Bayesian, which provides a reference for analyzing the public's travel emotion change in the post-COVID-19 era and guiding the public to choose urban public transport travel with the following specific conclusions.
1) In the post-COVID-19 era, the public is more concerned about the level of COVID-19 control in their area and the waiting and in-transit transport times. The public's cheerful travel willingness is significantly and positively correlated with the ridership of public transport and rail transport, while negative travel willingness is negatively correlated with them.
2) COVID-19 prevention policies and travel risks are popular topics of public travel discussions in the post-COVID-19 era, and the public's fear of infection has significantly improved with the arrival of the post-COVID-19 era.
As the first country to be affected by COVID-19, it is of some theoretical and practical significance to study public travel willingness in the post-COVID-19 era in China. Because of the recurring uncertainty of the COVID-19, public travel has been dramatically affected. However, due to the suddenness of the COVID-19, there are few studies on the factors influencing travel in the post-COVID-19 era. Therefore, this paper analyzes the changing trend of residents' travel willingness and the correlation between urban public transportation ridership and travel willingness from the perspective of residents' travel willingness in the post-COVID-19 era by collecting social network data. This has good theoretical significance for exploring the factors of residents' travel willingness in the post-COVID-19 era and its influencing mechanism.
Previous studies have investigated the factors associated with travel willingness, such as individual personality traits and the morbidity and mortality rates of COVID-19 [12,23]. Compared with previous studies, this paper provides a long-term data analysis from the beginning of COVID-19 to China's entry into the post-COVID-19 era. It explores the essential factors influencing the public's willingness to travel and the correlation between their willingness to travel and urban public transportation ridership.
However, this study also has some limitations. Since this study uses Sina Weibo text data, it does not include the individual socioeconomic attributes of travelers, and in addition, the data source is relatively homogeneous and does not include data from Facebook and Twitter regarding travel willingness.
The research was funded by the Humanities and Social Science Fund Project of Ministry of Education (17YJCZH220); National Natural Science Foundation of China (61803057); Chongqing Jiaotong University Graduate Student Research Innovation Project Funding (2022S0037).
The authors declare there is no conflict of interest.
[1] |
H. Rootzén, R. W. Katz, Design life level: Quantifying risk in a changing climate. Water Resour. Res., 49 (2013), 5964-5972. doi: 10.1002/wrcr.20425
![]() |
[2] |
M. M. de Oliveira, N. F. Ebecken, J. L. de Oliveira, E. Gilleland, Generalized extreme wind speed distributions in south America over the Atlantic Ocean region, Theor. Appl. Climatol., 104 (2011), 377-385. doi: 10.1007/s00704-010-0350-3
![]() |
[3] |
R. Potocky, M. Stehlik, H. Waldl, On sums of claims and their applications in analysis of pension funds and insurance products, Prague Econ. Pap., 23 (2014), 349-370. doi: 10.18267/j.pep.488
![]() |
[4] |
P. Jordanova, Z. Fabian, P. Hermann, L. Střelec, A. Rivera, S. Girard, et al., Weak properties and robustness of t-hill estimators, Extremes, 19 (2016), 591-626. doi: 10.1007/s10687-016-0256-2
![]() |
[5] |
M. Stehlík, L. N. Soza, Z. Fabián, M. Jiřina, P. Jordanova, S. C. Arancibia, et al., On ecological aspects of dynamics for zero slope regression for water pollution in Chile, Stochastic Anal. Appl., 37 (2019), 574-601. doi: 10.1080/07362994.2019.1592692
![]() |
[6] |
J. Pickands, Statistical inference using extreme order statistics, Ann. Stat., 3 (1975), 119-131. doi: 10.1214/aos/1176343003
![]() |
[7] | J. Hosking, J. Wallis, Parameters and quantile estimation for the generalized pareto distribution, Technometrics, 29 (1998), 339-349. |
[8] |
S. Juarez, W. Schucany, Robust and efficient estimation for the generalized pareto distribution, Extremes, 7 (2004), 237-251. doi: 10.1007/s10687-005-6475-6
![]() |
[9] |
J. Zhang, Likelihood moment estimation for the generalized pareto distribution, Aust. N. Z. J. Stat., 49 (2007), 69-77. doi: 10.1111/j.1467-842X.2006.00464.x
![]() |
[10] |
J. Zhang, Improving on estimation for the generalized pareto distribution, Technometrics, 52 (2010), 335-339. doi: 10.1198/TECH.2010.09206
![]() |
[11] |
J. Zhang, M. Stephens, A new and efficient estimation method for the generalized pareto distribution, Technometrics, 51 (2009), 316-325. doi: 10.1198/tech.2009.08017
![]() |
[12] |
J. He, Z. Sheng, B. Wang, K. Yu, Point and exact interval estimation for the generalized Pareto distribution with small samples, Stats its interface, 7 (2014), 389-404. doi: 10.4310/SII.2014.v7.n3.a9
![]() |
[13] |
J. Song, S. Song, A quantile estimation for massive data with generalized Pareto distribution, Comput. Stat. Data Anal., 56 (2012), 143-150. doi: 10.1016/j.csda.2011.06.030
![]() |
[14] |
M. H. Park, J. H. T. Kim, Estimating extreme tail risk measures with generalized Pareto distribution, Comput. Stat. Data Anal., 98 (2016), 91-104. doi: 10.1016/j.csda.2015.12.008
![]() |
[15] |
S. Kang, J. Song, Parameter and quantile estimation for the generalized pareto distribution in peaks over threshold framework, J. Korean Stat. Soc., 46 (2017), 487-501. doi: 10.1016/j.jkss.2017.02.003
![]() |
[16] |
S. Boyd, N. Parikh, E. Chu, B. Peleato, J. Eckstein, Distributed optimization and statistical learning via the alternating direction method of multipliers, Found. Trends Mach. Learn., 3 (2010), 1-122. doi: 10.1561/2200000016
![]() |
[17] |
E. Chu, A. Keshavarz, S. Boyd, A distributed algorithm for fitting generalized additive models, Optim. Eng., 14 (2013), 213-224. doi: 10.1007/s11081-013-9215-9
![]() |
[18] |
X. Yuan, Alternating direction method for covariance selection models, J. Sci. Comput., 51 (2012), 261-273. doi: 10.1007/s10915-011-9507-1
![]() |
[19] |
Y. Gu, J. Fan, L. Kong, S. Ma, H. Zou, ADMM for high-dimensional sparse penalized quantile regression, Technometrics, 60 (2018), 319-331, doi: 10.1080/00401706.2017.1345703
![]() |
[20] | M. Hong, Z. Q. Luo, M. Razaviyayn, Convergence analysis of alternating direction method of multipliers for a family of non-convex problems, SIAM J. Optim., 26 (2014), 3836-3840. |
[21] |
B. He, X. Yuan, On the O(1/n) convergence rate of the douglas-rachford alternating direction method, SIAM J. Numer. Anal., 50 (2012), 700-709. doi: 10.1137/110836936
![]() |
[22] |
W. Deng, W. Yin, On the global and linear convergence of the generalized slternating direction method of multipliers, J. Sci. Comput., 66 (2016), 889-916. doi: 10.1007/s10915-015-0048-x
![]() |
[23] | J. Liu, S. J. Wright, C. Ré, V. Bittorf, S. Sridhar, An asynchronous parallel stochastic coordinate descent algorithm, J. Mach. Learn. Res., 16 (2013), 285-322. |
[24] |
H. R. Feyzmahdavian, A. Aytekin, M. Johansson, An asynchronous mini-batch algorithm for regularized stochastic optimization, IEEE Trans. Autom. Control, 61 (2016), 3740-3754. doi: 10.1109/TAC.2016.2525015
![]() |
[25] | A. McNeil, T. Saladin, The peaks over thresholds method for estimating high quantiles of loss distributions, Proc. 28th Int. ASTIN Colloq., (1997), 23-43. |
[26] | A. A. Balkema, L. de Haan, Residual life time at great age, Ann. Probab., 2 (2004), 792-804. |
[27] | P. Embrechts, C. Kluppelberg, T. Mikosch, Modelling Extremal Events for Insurance and Finance, Springer-Verlag, Berlin Heidelberg, 1997. |
[28] |
H. Zhu, A. Cano, G. Giannakis, Distributed consensus-based demodulation: Algorithms and error analysis, IEEE Trans. Wireless Commun., 9 (2010), 2044-2054. doi: 10.1109/TWC.2010.06.090890
![]() |
![]() |
![]() |
Authors | Research Subjects | Research Period | Research Methods |
Raymond et al. [12] (2021) | The impact of COVID-19 morbidity and mortality on travel willingness | During the COVID-19 | Multi-Cluster Analysis Method |
Yang et al. [13] (2021) | Influence of passenger perception of safety on willingness to travel by air | During the COVID-19 | Structural Equation Model (SEM) |
Shi et al. [14] (2021) | Factors and Mechanisms of Influence of the COVID-19 on Residents' Willingness to Travel for Leisure | During the COVID-19 | SEM |
Liang et al. [15] (2022) | Residents' willingness to travel under the COVID-19 | During the COVID-19 | SEM |
Mehrbakhsh et al. [16] (2022) | The influence of opinions in social media on travel decisions | During the COVID-19 | SEM |
Francene et al. [17] (2023) | Change in travel attitudes due to the COVID-19 | During the COVID-19 | SEM |
Jordan et al. [18] (2023) | Influence of college students' perceived risk of the COVID-19 on their willingness to travel | During the COVID-19 | SEM |
Zhang et al. [19] (2022) | People's willingness to travel to urban parks | Post-COVID-19 era | SEM |
Zhao et al. [20] (2022) | Residents' travel mentality | Post-COVID-19 era | Theory of Planned Behavior |
Sun et al. [21] (2022) | The effect of people's trust in destination risk regulation on their willingness to travel | Post-COVID-19 era | SEM |
Vikram et al. [22] (2022) | Impact of the COVID-19 on Commuters' Travel willingness in Delhi, India | Post-COVID-19 era | SEM |
Shalini et al. [23] (2022) | Influence of personality traits on willingness to travel | Post-COVID-19 era | Artificial Neural Networks |
Yao et al. [24] (2023) | How to stimulate people's willingness to travel | Post-COVID-19 era | SEM |
Off-topic: as soon as we left the house, the wind was howling and the thunderstorm was in full force. | ||
Tangential to the theme: wash your hands regularly, get plenty of ventilation, get vaccinated and wear a mask when you go out! | ||
Positive: #GuangzhouOutbreak #Get out and protect yourself, whether you're in a medium-risk area or not! ~Believe Guangzhou will survive the second wave of the outbreak! | Neutral: #Foshan Nanhai District 1 site upgraded to medium risk #Wear a mask when you go out and try not to go out and gather together if not necessary | Negative: #Foshan Nanhai District 1 site upgraded to medium risk #dare not go out |
Category | Off-topic | Relevant to the topic | Total | ||||||||||
Periods | D | P | D | P | D | P | |||||||
Counts | 1136 | 2081 | 7608 | 4841 | 8744 | 6922 | |||||||
Percentage | 12.99% | 30.06% | 87.01% | 69.94% | 100% | 100% | |||||||
Category | Positive | Neutral | Negative | Total | |||||||||
Periods | D | P | D | P | D | P | D | P | |||||
Counts | 516 | 1888 | 340 | 755 | 6752 | 2198 | 7608 | 4841 | |||||
Percentage | 6.78% | 39.00% | 4.67% | 15.60% | 88.75% | 45.40% | 100% | 100% |
Variables | Correlation coefficient | |
The Volume of positive emotions | Public transport ridership | 0.848** |
Rail ridership | 0.829** | |
Taxi ridership | 0.612** | |
The Volume of negative emotions | Public transport ridership | -0.754** |
Rail ridership | -0.765** | |
Taxi ridership | -0.526* | |
Note: **: At the 0.01 level (two-tailed), the correlation is significant; *: At the 0.05 level (two-tailed), the correlation is significant. |
Topic | Topic Name | LDA Keywords | ||
Periods | D | P | D | P |
1 | Action against the COVID-19 | COVID-19 prevention policy | Action; Ambulance; Meaning; Infected Area | Mask; Region; New crown; Prevention and control; Vaccine; High risk |
2 | Traveling against the COVID-19 | Travel Risks | Prevention and control; Taxis; Disinfection; Personnel; Vehicles; Masks | High Risk; Cases; Local Reports; Impact; Travel |
3 | Living with the COVID-19 | Life's Little Things | Masks; life; hand washing; public good; feeling; time | Life; time; time; video; things; home |
4 | Webcasts | Nucleic Acid Testing | Video; web page; COVID-19 prevention; links; campaign; live | nucleic acid; people; cases; region; prevention control; high risk |
5 | Travel Essentials | Prevention and Control Management | Public transport; Subway; Passengers; Operation; Control; Time | prevention and control; people; measures; management; people |
6 | COVID-19 Prevention and Control | Outbreak Prevention and Control | Cases; Patients; Masks; Neighborhoods; Home; Community | Streets; Communities; Neighborhoods; Areas; Vehicles; Prevention and Control |
7 | - | Travel and Tourism | Development; Tourism; Enterprises; Services; Markets; Attractions | |
8 | - | Travel for the Elderly | Transport; Sector; Services; elderly; Passenger transport; Area |
Authors | Research Subjects | Research Period | Research Methods |
Raymond et al. [12] (2021) | The impact of COVID-19 morbidity and mortality on travel willingness | During the COVID-19 | Multi-Cluster Analysis Method |
Yang et al. [13] (2021) | Influence of passenger perception of safety on willingness to travel by air | During the COVID-19 | Structural Equation Model (SEM) |
Shi et al. [14] (2021) | Factors and Mechanisms of Influence of the COVID-19 on Residents' Willingness to Travel for Leisure | During the COVID-19 | SEM |
Liang et al. [15] (2022) | Residents' willingness to travel under the COVID-19 | During the COVID-19 | SEM |
Mehrbakhsh et al. [16] (2022) | The influence of opinions in social media on travel decisions | During the COVID-19 | SEM |
Francene et al. [17] (2023) | Change in travel attitudes due to the COVID-19 | During the COVID-19 | SEM |
Jordan et al. [18] (2023) | Influence of college students' perceived risk of the COVID-19 on their willingness to travel | During the COVID-19 | SEM |
Zhang et al. [19] (2022) | People's willingness to travel to urban parks | Post-COVID-19 era | SEM |
Zhao et al. [20] (2022) | Residents' travel mentality | Post-COVID-19 era | Theory of Planned Behavior |
Sun et al. [21] (2022) | The effect of people's trust in destination risk regulation on their willingness to travel | Post-COVID-19 era | SEM |
Vikram et al. [22] (2022) | Impact of the COVID-19 on Commuters' Travel willingness in Delhi, India | Post-COVID-19 era | SEM |
Shalini et al. [23] (2022) | Influence of personality traits on willingness to travel | Post-COVID-19 era | Artificial Neural Networks |
Yao et al. [24] (2023) | How to stimulate people's willingness to travel | Post-COVID-19 era | SEM |
Off-topic: as soon as we left the house, the wind was howling and the thunderstorm was in full force. | ||
Tangential to the theme: wash your hands regularly, get plenty of ventilation, get vaccinated and wear a mask when you go out! | ||
Positive: #GuangzhouOutbreak #Get out and protect yourself, whether you're in a medium-risk area or not! ~Believe Guangzhou will survive the second wave of the outbreak! | Neutral: #Foshan Nanhai District 1 site upgraded to medium risk #Wear a mask when you go out and try not to go out and gather together if not necessary | Negative: #Foshan Nanhai District 1 site upgraded to medium risk #dare not go out |
Category | Off-topic | Relevant to the topic | Total | ||||||||||
Periods | D | P | D | P | D | P | |||||||
Counts | 1136 | 2081 | 7608 | 4841 | 8744 | 6922 | |||||||
Percentage | 12.99% | 30.06% | 87.01% | 69.94% | 100% | 100% | |||||||
Category | Positive | Neutral | Negative | Total | |||||||||
Periods | D | P | D | P | D | P | D | P | |||||
Counts | 516 | 1888 | 340 | 755 | 6752 | 2198 | 7608 | 4841 | |||||
Percentage | 6.78% | 39.00% | 4.67% | 15.60% | 88.75% | 45.40% | 100% | 100% |
Variables | Correlation coefficient | |
The Volume of positive emotions | Public transport ridership | 0.848** |
Rail ridership | 0.829** | |
Taxi ridership | 0.612** | |
The Volume of negative emotions | Public transport ridership | -0.754** |
Rail ridership | -0.765** | |
Taxi ridership | -0.526* | |
Note: **: At the 0.01 level (two-tailed), the correlation is significant; *: At the 0.05 level (two-tailed), the correlation is significant. |
Topic | Topic Name | LDA Keywords | ||
Periods | D | P | D | P |
1 | Action against the COVID-19 | COVID-19 prevention policy | Action; Ambulance; Meaning; Infected Area | Mask; Region; New crown; Prevention and control; Vaccine; High risk |
2 | Traveling against the COVID-19 | Travel Risks | Prevention and control; Taxis; Disinfection; Personnel; Vehicles; Masks | High Risk; Cases; Local Reports; Impact; Travel |
3 | Living with the COVID-19 | Life's Little Things | Masks; life; hand washing; public good; feeling; time | Life; time; time; video; things; home |
4 | Webcasts | Nucleic Acid Testing | Video; web page; COVID-19 prevention; links; campaign; live | nucleic acid; people; cases; region; prevention control; high risk |
5 | Travel Essentials | Prevention and Control Management | Public transport; Subway; Passengers; Operation; Control; Time | prevention and control; people; measures; management; people |
6 | COVID-19 Prevention and Control | Outbreak Prevention and Control | Cases; Patients; Masks; Neighborhoods; Home; Community | Streets; Communities; Neighborhoods; Areas; Vehicles; Prevention and Control |
7 | - | Travel and Tourism | Development; Tourism; Enterprises; Services; Markets; Attractions | |
8 | - | Travel for the Elderly | Transport; Sector; Services; elderly; Passenger transport; Area |