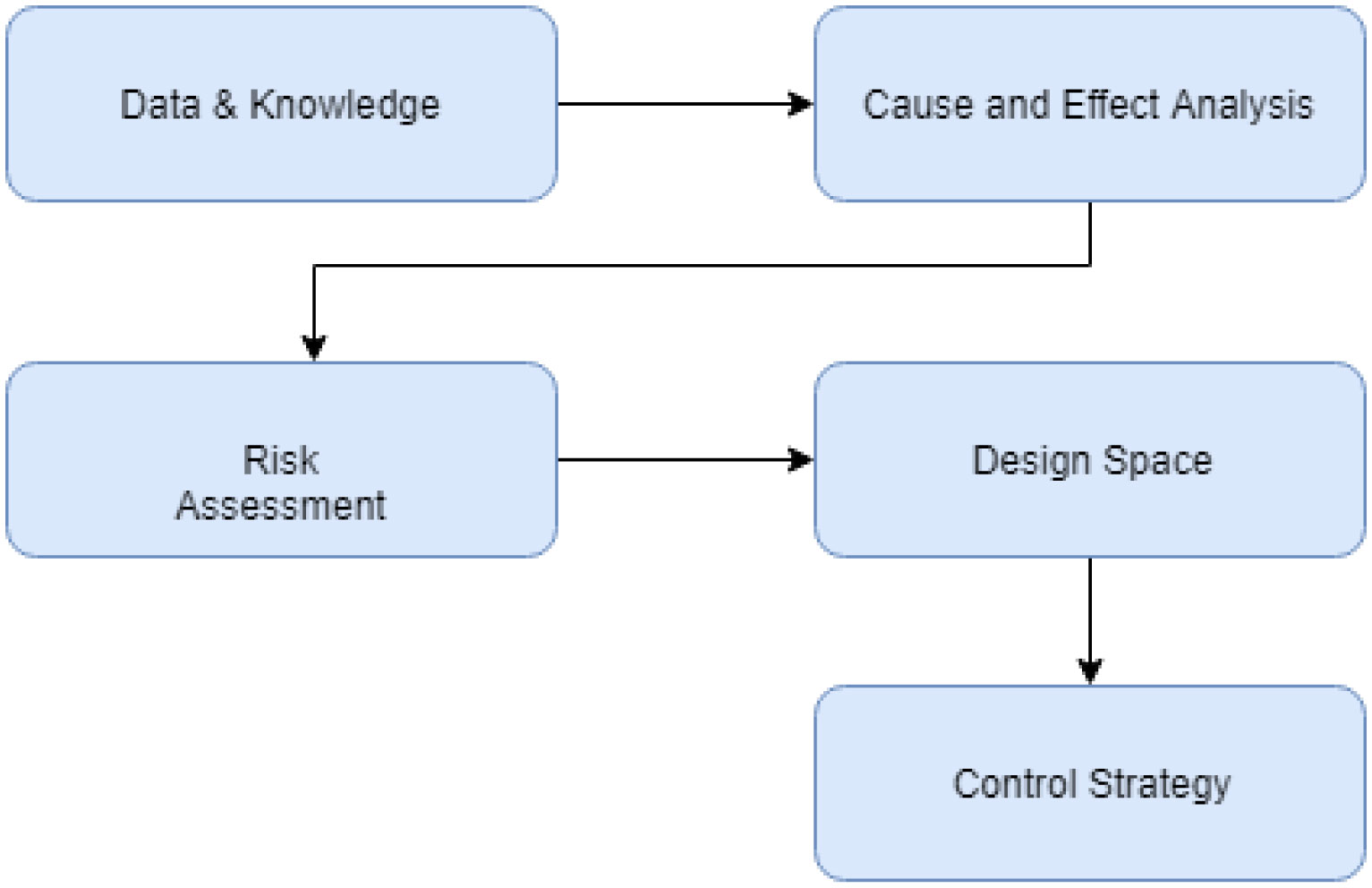
Citation: Daniel Borchert, Diego A. Suarez-Zuluaga, Yvonne E. Thomassen, Christoph Herwig. Risk assessment and integrated process modeling–an improved QbD approach for the development of the bioprocess control strategy[J]. AIMS Bioengineering, 2020, 7(4): 254-271. doi: 10.3934/bioeng.2020022
[1] | Sébastien Bernacchi, Simon Rittmann, Arne H. Seifert, Alexander Krajete, Christoph Herwig . Experimental methods for screening parameters influencing the growth to product yield (Y(x/CH4)) of a biological methane production (BMP) process performed with Methanothermobacter marburgensis. AIMS Bioengineering, 2014, 1(2): 72-87. doi: 10.3934/bioeng.2014.2.72 |
[2] | Ruming Li, Brian Fristensky, Guixue Wang . Sequence data analysis and preprocessing for oligo probe design in microbial genomes. AIMS Bioengineering, 2017, 4(1): 28-45. doi: 10.3934/bioeng.2017.1.28 |
[3] | Shital Hajare, Rajendra Rewatkar, K.T.V. Reddy . Design of an iterative method for enhanced early prediction of acute coronary syndrome using XAI analysis. AIMS Bioengineering, 2024, 11(3): 301-322. doi: 10.3934/bioeng.2024016 |
[4] | George H McArthur IV, Pooja P Nanjannavar, Emily H Miller, Stephen S Fong . Integrative metabolic engineering. AIMS Bioengineering, 2015, 2(3): 93-103. doi: 10.3934/bioeng.2015.3.93 |
[5] | Meher Langote, Saniya Saratkar, Praveen Kumar, Prateek Verma, Chetan Puri, Swapnil Gundewar, Palash Gourshettiwar . Human–computer interaction in healthcare: Comprehensive review. AIMS Bioengineering, 2024, 11(3): 343-390. doi: 10.3934/bioeng.2024018 |
[6] | Moawiah M Naffaa . Innovative therapeutic strategies for traumatic brain injury: integrating regenerative medicine, biomaterials, and neuroengineering. AIMS Bioengineering, 2025, 12(1): 90-144. doi: 10.3934/bioeng.2025005 |
[7] | Sébastien Bernacchi, Michaela Weissgram, Walter Wukovits, Christoph Herwig . Process efficiency simulation for key process parameters in biological methanogenesis. AIMS Bioengineering, 2014, 1(1): 53-71. doi: 10.3934/bioeng.2014.1.53 |
[8] | Jéssica de Oliveira Rossi, Gabriel Tognon Rossi, Maria Eduarda Côrtes Camargo, Rogerio Leone Buchaim, Daniela Vieira Buchaim . Effects of the association between hydroxyapatite and photobiomodulation on bone regeneration. AIMS Bioengineering, 2023, 10(4): 466-490. doi: 10.3934/bioeng.2023027 |
[9] | Atta Ullah, Hamzah Sakidin, Shehza Gul, Kamal Shah, Yaman Hamed, Maggie Aphane, Thabet Abdeljawad . Sensitivity analysis-based control strategies of a mathematical model for reducing marijuana smoking. AIMS Bioengineering, 2023, 10(4): 491-510. doi: 10.3934/bioeng.2023028 |
[10] | Sumaiya Noor, Salman A. AlQahtani, Salman Khan . Chronic liver disease detection using ranking and projection-based feature optimization with deep learning. AIMS Bioengineering, 2025, 12(1): 50-68. doi: 10.3934/bioeng.2025003 |
Thank you for your cooperation. There are three process validation stages suggested by the Food and Drugs Administration (FDA) [1]:
Stage 1 aims to identify the relations of the process parameters (PP)s, operated within a particular range, to the critical quality attributes (CQAs) [2] by using process knowledge and experimental data. As suggested by the international conference of harmonization (ICH) Q9 guideline [3], it is current good practice to conduct a risk assessment (RA) before experiments are planned. Since such a procedure is common practice in process development, it should still be used in process validation to ensure the traceability of the conducted experiments. Within the Q9 guideline, the RA target is “the identification of hazards and the analysis and evaluation of risks associated with exposure to those hazards” [3]. Hence, the impact of each PP on each CQA is evaluated within that approach. The information required to perform a RA [3] consists of historical data, process expert's knowledge and literature. Once the PPs with the biggest influence on the CQAs are determined via the RA, their influence is experimentally confirmed. In the pharmaceutical industry, this is often done via design of experiment (DoE) studies [4] of each unit operation. The exact amount of PPs investigated within a DoE, as well as the aim of the design, are the most crucial factors which must be carefully selected [5]. This unit operation isolated view of the process is often reported as contra-productive because they often interactively influence each other [6]. Therefore, it is recommended to change the process characterization from a unit operation oriented perspective to a holistic one. This holistic process evaluation or the reduction of separate unit operation can either be done by a Life Cycle assessment [7] or by continuous processing [8]–[10], perfusion [7],[9] or QbD [11]. All of these techniques have the purpose to reduce the overall experimental costs.
Next to characterizing the process, the “Stage 1” goal is to identify an appropriate process control strategy. This strategy identifies the necessary proven acceptable ranges (PARs) for the critical PPs (CPPs) to achieve the required product quality [12]. This is best done by using a QbD approach [13]. Figure 1 displays the QbD approach as proposed by the CMC Biotech working group [13] used for “Stage 1”. Afterward, Stage 2 will be pursued to prove the chosen control strategy, while finally, Stage 3 is used to monitor and continuously improve the process. Within this work, the procedure, methods and workflow suitable for Stage 1 will be discussed in more detail. Stage 2 and 3 are not further considered within this work.
Figure 1 represents the five major parts of the QbD approach for Stage 1. Data & Knowledge represent the need for data and process knowledge and act as the start of the approach. Cause and Effect Analysis symbolizes the need for statistical analysis approaches to identify the impact of PPs on CQAs. Risk Assessment is the evaluation tool used to estimate the PP effect on the CQA based on statistical and process knowledge. Design Space represents the identified operation range of the CPPs within the process. Finally, Control Strategy serves the aim of the assessment and indicates the required PP control action (with specified PARs) to produces the required CQA quality. Although this approach has shown good results in the last years [13], it requires much time and costs to fulfill each step. This is mainly because the approach has been focused on each unit operation independently.
Moreover, this per unit operation approach bears a significant risk, which is that an isolated establishment of the control strategy may fail because the interactions between the unit operations may not be taken into account [11].
In order to avoid the above mentioned issues, we identified improvement capabilities using basic data science approaches. Within this work, an improved QbD approach for process characterization, Stage 1 [1], is shown. The suggested approach focuses on improvements in the steps Cause and Effect Analysis and Risk Assessment and how the holistic interactive process impacts these two procedures. These improvements result in reduced experimental effort and in the development of an integrated process control strategy. The herein presented QbD workflow is based on a novel combination of RA and integrated process modeling (IPM). We demonstrate it via a case study and show that the approach can be applied to a biopharmaceutical process anticipated to become a platform process. In this case study, we were able to reduce the number of factors considered within a DoE significantly, from at least eight to three.
The dataset used for the herein conducted case study was provided by the Institute for Translational Vaccinology (Intravacc). The data represent development studies for an inactivated polio vaccine platform process. Sabin poliovirus type 2 was produced with an animal component free media on Vero cells (USP phase). Further, five downstream processing (DSP) unit operations were conducted to purify the virus. The key performance indicator (KPI) and the impurities were determined at the end of the USP and at each DSP unit operation.
Within the case study, two commercially available software tools were used to perform the cause and effect analysis. SIMCA version 13.0.3.0 (Umetrics AB, Umea, Sweden) was used for raw data analysis (RDA). To perform feature based analysis (FBA), inCyght® Web version 2020.03 (Exputec GmbH, Vienna, Austria) was used. The required uni- and multivariate statistical analysis tools were already implemented in the software. The data were preprocessed with inCyght® and MS Excel 2016 (Microsoft, Redmond, WA) before data analysis with SIMCA. Python 3.7 (Python Software Foundation [14]) was used for the RA simulation and plotting.
This section is presented in two parts. First, a comparison of the current QbD (cQbD) with the improved (new) QbD (nQbD) approach is given. Second, the results of the analysis for a case study based on the nQbD approach are described.
The cQbD approach is well established and often used in industry. In parallel, data science applications increased in importance within the past years [15]. They are used within the cQbD approach to increase process knowledge and to save time and costs. The nQbD approach applies a combination of existing and novel data science and analysis methods, resulting in an improved QbD approach. Figure 2 summarizes both approaches side by side.
The basic concept of the QbD approach, as shown in Figure 1, has not been changed with the suggested approach (Figure 2A). Within Figure 2, if a particular strategy differs, a separate box for each approach is displayed. The first evaluation of Figure 2B indicates that the input and output of both approaches are similar, while in the activity section, differences are observed. Within this section, the entire workflow and in more detail, the differences between the current and the new QbD approaches will be explained below.
At the beginning of the analysis process, knowledge and historical data are often available. We assume that the key performance indicators of the process, often called the responses, are already defined according to the ICH Q8 guideline [16]. The more is known about the process, the better and faster the analysis approach will be. Therefore, comprehensive process knowledge is crucial for the following steps. In former times, it was often reported [17] that just one data format was used for data analysis since it was often not possible to merge all available data types. This reduced data gathering results in a significant loss of information and thus process knowledge. The holistic data storage and analysis of all available data types are often very complex and time-intensive. Many different data storage and analysis tools are currently available; its benefits and appropriate analysis tools were recently reviewed by Steinwandter et al. [15].
At the beginning of the workflow, it is crucial to identify the reason for the response variance. This is done via a cause and effect analysis (often also called root cause analysis [18],[19]).
cQbD Approach. Currently, two approaches are commonly followed to perform the cause and effect analysis. Raw data analysis (RDA) [20] is currently the most prominent tool used in industry. While the feature-based approach (FBA) has increased in importance within recent years [21],[22]. Nevertheless, independent of the approach, the workflow to identify influencing parameters effecting a CQA can be done within seven steps (Figure 3).
The RDA and FBA approaches differ primarily in step 3 (Data Unfolding). While the RDA analysis uses all available data holistically, the FBA uses reasonable information mined from the process data [18]. Furthermore, both approaches use just a particular data format [17],[20]–[22], time series data for RDA and features for FBA. The remaining data are often not included in the analysis. Since not all available data are considered in data analysis, a loss of process knowledge by using each approach individually is assumed. Independent of the approach, the multivariate model generation and result interpretation might be hard for bioprocess engineers. Hence, they rely on statisticians to do such an analysis. On the other hand, statisticians are often not trained in the context of biopharmaceutical production, which can result in a multivariate data analysis (MVDA), which is not useful to apply. This problem regularly results in the bioprocess expert using software tools to apply and understand data analysis algorithms and their results.
nQbD Approach. Even when process scientists possess both process and statistical knowledge, the gap of using all available data for data analysis still exists. In this contribution, a comprehensive data evaluation workflow that enables the use of all data holistically and the mining of reasonable and adequate information [18] is presented. As shown in Borchert et al. [18], a combination of RDA and FBA is the crucial element of the analysis approach. The workflow is based on three elements:
1. Hypotheses generation
2. Process knowledge
3. Hypotheses testing
The RDA covers elements 1 and 2. Generally, the variance in the CQA is detected holistically, but the real causing factor needs to be further investigated. The first hypotheses can be established during data analysis. By subsequently applying the RDA, the hypotheses can be verified, or new ones can be generated. Based on that outcome, the FBA can be used to test the generated hypotheses (Element 3) via reasonable information mining from the previously investigated time series data. The mined information will be evaluated by applying standard uni- and multivariate data analysis approaches. A final multivariate regression model ends up in the identified root cause, which is often displayed using an Ishikawa (Fishbone) diagram.
This unique analysis capability by using all available data was already applied to a vaccine production process using measurements from the upstream unit operation and is shown to be useful for [19]:
Consequently, applying this approach allows us to learn more from the data. This means that a stable basis for the next step in process characterization is established.
The next step in the QbD approach is risk evaluation. Within an RA, usually, three factors [3] are often estimated for each PP on each CQA.
Usually, a team of process experts rates each factor, within the predefined PP ranges, based on their process knowledge and experience.
cQbD Approach. The standard approach evaluates a RA using the Risk Priority Number (RPN). This method multiplies the S, O and D values to obtain the RPN. Since it is often the case that the three factors are ranked from one to ten, the RPN can, therefore, range from 1–1000. Consequently, each PP has an RPN and can be sorted based on it. The higher the RPN, the higher the PP is considered to (negatively) impact the CQA when operating outside the PP target range. Usually an arbitrary RPN number is defined as the threshold to determine if the PP is criticality affecting the CQA.
This approach is prevalent in the industry. However, from a mathematical point, it is not appropriate. The reasons for this are [23]:
In the past years, several approaches were developed to tune the RPN approach [24]. These tools are mainly developed in order to avoid the current pitfalls. Those multi-attribute decision approaches are based on sophisticated algorithms like grey relation [25] or the order of preference by the similarity of the ideal solution [26]. Such tools are handy for their benefit but are often hard to interpret for biopharmaceutical process experts.
nQbD Approach. This approach translates the S estimation of the RA to model effect size and to use the O estimation to predict the operating parameter within a defined judged range. A simple linearization is used for the model size definition and standard truncate and selection methods are used to estimate the parameter distribution within the PP judged range [27]. In addition to the conventional RA, a new estimation needs to be entered into the RA, the critical delta of CQA [%] [27]. Like for S and O, this needs to be done for each unit operation and CQA. This value can be gathered from process knowledge, experimental data, or literature.
Beyond this novel S and O interpretation, this implements “integrated process modeling” (IPM) [28] into it. This enables a holistic process assessment of the CQA for all unit operations. Figure 4 shows a three unit operations example.
Figure 4 shows the tool based on three-unit operations. The holistic simulation, starting from the first until the last unit operation, is further referred to as a cycle. In order to simulate the process reproducibility as close as possible to the real one, a Monte Carlo simulation [29] was applied. The method simulated 1000 such cycles [28], including all PP for each CQA independently. In order to evaluate the PP critically onto the process, this simulation has to be repeated by excluding one PP from the simulation, until each PP has been removed once. After that, the resulting CQA distribution at the last unit operation results in the CQA reduction per PP.
This result allows a quantitative statement and sorting of PPs accordingly. PPs criticality can be identified by evaluating their simulated impact on the quality attribute. And, it can now be estimated based on the impact not in a single unit operation but on the whole process. Furthermore, this approach can avoid all incorrectness of the RPN approach:
The third factor “D” can be included after the simulation. Since factor D is often described as the ability to recognize a failure before it causes damage [3], a final re-sorting step can be applied. Therefore, the entire PP evaluation is split into equal blocks. These blocks are then further sorted by its D evaluation. This re-sorting can cause that PPs with a lower impact on the process appear as more critical than before although the S and O linearization shows less quantitative impact [27].
This method implements a novel rational decision-making tool, which avoids all of the known disadvantages of the RPN approach. Moreover, it enables a quantitative evaluation of the whole process.
The last step in the activity section is the design space evaluation. The design space is used to describe the relationship between the input parameters of the process and the CQA [16]. According to the ICH Q8 (R2) guideline, the design space is defined as the multivariate combination and interaction of input variables and process parameters that have been demonstrated to provide assurance of quality. The design space can be a sophisticated mathematical operation or a combination of factors of the multivariate model [16]. Beyond its interpretation, the most important fact is that if the process is operated in that space, the CQA will meet the defined product quality.
The prior conducted MVDA is used to increase process knowledge and the RA results in the first hypotheses of PP effects to the CQA. The evaluation of such hypotheses is commonly done using a DoE approach, since targeted experiments will be conducted. The appropriate design is often very sophisticated since many factors have to be considered. Nevertheless, a DoE will be often conducted for a particular purpose; the three primary reasons for a DoE are:
1. Screening—Identify the most influential factors and their appropriate ranges
2. Optimization—Identify the process optimum
3. Robustness testing—Proof that the process is under control
Independent of the aim of the DoE, experiments must be conducted with a particular objective. After defining it, the next and most crucial step for DoE design is the factor selection. Depending on the number of factors that should be investigated in the experimental design, the number of required experiments can increase drastically and should be selected carefully. The number of factors, which will be considered in a DoE, are often taken from prior RA evaluation.
After DoE experiments, MVDA is conducted to identify the relations between the changes in the investigated factors on the CQA. In that case, regression analysis is often conducted [4]. The analysis procedure in this stage of the process is similar for cQbD and nQbD and therefore not further discussed in this work
cQbD Approach. The Fishbone diagram, from a prior root cause analysis, is one strategy used to identify the number of factors that have to be considered within a DoE [30]–[32]. Another strategy, and the most prominent, is the use of a RA [31].
Both approaches, Fishbone and RA, often result in many factors that must be included within DoE study. Such DoEs are costly and time consuming. Since these are critical factors within process assessment, the current design space assessment approach needs to be optimized.
The knowledge gathered from DoE can be used for control strategy definition or can be reassessed in the cause and effect analysis (Figure 2). This recycling step can occur multiple times (see Figure 2B, arrow x) so enough process knowledge is gathered and sufficient information for defining the control strategy is available. Hence, many experiments might be required to fulfill the aim.
nQbD Approach. Using the quantitative RA, it is feasible to:
Since the number of factors that are considered in the DoE can be optimized, the power of the model can increase drastically with fewer experiments. This reduction in experimental effort results in specific process knowledge, which can fill existing knowledge gaps and thus reduces the amount of knowledge recycling (Figure 2B, arrow y).
Furthermore, the herein developed RA evaluation approach can be used to simulate the entire process. This new evaluation tool enables a holistic process assessment leading to one holistic control strategy based on fast and reasonable design space evaluation.
The QbD approach aims to identify the control strategy of the process in scope. A control strategy is according to the ICH Q11 [33] guideline defined as a planned set of controls, derived from current produce and process understanding, that assures process performance and product quality (ICH Q10). Furthermore, it is mentioned within that guideline that, a control strategy should ensure that each drug substance CQA is within the appropriate range, limit, or distribution to assure drug substance quality. The control strategy assures that the CQA meets drug substance specifications over the entire process.
The essential element of the QbD approach, the process knowledge, will be used within all stages of the process lifecycle approach. Thus, it makes it crucial not only for process characterization but also for the entire product lifecycle.
cQbD Approach. According to the ICH Q11 recommendation, a CQA can have several control strategies over the process. This recommendation often results in many independent control strategies at a particular unit operation. Such independent control strategies are used to assess the CQA quality over the process. Furthermore, they are used to take counter measurements in case of any abnormalities. Nevertheless, such independent strategies are hard to maintain and time-consuming for the process operators and should be avoided in the future.
nQbD Approach. Since our approach lays the basis for one holistic process control strategy, it could be possible to save experimental effort and time in the future. This significantly reduces the number of control strategies from many different to one holistic. Nevertheless, this needs to be assessed in further studies, which is out of the scope of this contribution.
A case study was conducted to prove the applicability and to demonstrate the potential of the nQbD approach. Each step within the activity part, as shown in Figure 2B, was separately considered and core results are present here.
In order to follow the herein suggested nQbD approach, we consider two variables from the second DSP unit operation in more detail and track their progress through the entire case study. These variables are the transmembrane pressure (TMP) and the concentration factor (CF) of that unit operation, further referred to as UO2_TMP and UO2_CF, respectively.
All five DSP unit operations were investigated independently based on the workflow from Borchert et al. [18]. Finally, it was possible to get a significant multivariate model for each unit operation and the effects on the KPI and impurities could be identified. Figure 5 shows the resulting Ishikawa diagram for the second DSP unit operation.
Considering Figure 5, eight PP of unit operation 2 have a significant impact on the KPI and are considered as CPP. It can be seen that the parameter UO2_TMP shows the most significant impact on the KPI. The other parameters, as well as UO2_CF, show significant impact, but less than UO2_TMP and have to be considered as essential parameters in the next steps of analysis. This result visualization provides a first detailed understanding of each unit operation. Such information allows it to increase process knowledge and to evaluate the next step more accurately.
Based on the existing data and the previously gathered process knowledge, it was possible to evaluate the critical delta for each unit operation and CQA which would be loss (for KPI) or increase (for Impurity). This maximum change in CQA, which is still acceptable for the process, can be estimated based on process knowledge or calculated based on real data. In case of considering real data, the mean of the existing CQA measurement is taken and then three standard deviations from the mean can be considered as acceptable. This acceptable limit depends mainly on the investigated process. In our case, to calculate the acceptable change of the CQA at DS (DSCQA), the mean value was taken and compared with the lowest acceptable boundary of the CQA, according to the process regulation. Table 1 shows the result of this evaluation conducted for the KPI, Impurity 1 and Impurity 2. Detail measurements used for the calculations are not shown due to confidential reason and will be published separately.
Unit operation | Acceptable ∆CQA [%] KPI | Acceptable ∆CQA [%] Impurity 1 | Acceptable ∆CQA [%] Impurity 2 |
USP | 10 | 5 | 7 |
DSP UO1 | 10 | 20 | 20 |
DSP UO2 | 11 | 5* | 5* |
DSP UO3 | 7 | 22 | 20 |
DSP UO4 | 8 | 7 | 5 |
DSP UO5 | 4 | 2 | 2 |
Drug substance | 2.5 | 10 | 5 |
Based on the performed RA and the evaluation shown in Table 1, the IPM simulation could be conducted as suggested by Borchert et al. [27]. Based on that result, PPs can be stated as critical if a particular PP exceeds the DSCQA acceptable limit. It is often the case that the investigated KPI should remain as high as possible at the end of the process and that the impurity concentration should be minimized. Both aspects can be considered within the conduced simulation since relative values of the CQAs are considered in this approach.
The new suggested RA evaluation tool provides a more reasonable result interpretation as the standard used RPN approach [27]. Based on it, an overview of the quantitative contribution assessment is shown in Figure 6. The plot shows the estimated CQA reduction for each PP assessed within the investigated RA.
Figure 6 demonstrates that three parameters from two different unit operations can be seen as potentially critical for the entire process, since these variables are above the acceptable delta CQA [%], compare Table 1. Two out of three factors are, UO2_TMP and UO2_Var 3, since they have a higher delta CQA as accepted in the KPI assessment, and one, final UO3_Var5, should be included since delta CQA is higher as the acceptable limit for Impurity 1 and 2 assessment. Hence, those would be the PP selected for a follow-up DoE evaluation.
With the conduced case study, it was possible to identify the critical PPs from the entire process by using a rational PP evaluation. Furthermore, it was shown, based on the two tracked variables UO2_TMP and UO2_CF, such a result would not to be feasible when unit operations were assessed just individually. It has to be considered that PPs affect the entire process and not just the performance of the particular unit operations, as shown beginning of the analysis (Figure 5). Furthermore, it was shown that a critical CQA assessment, as shown in Table 1, is necessary to conduct a holistic process assessment. This awareness is crucial when the number of experiments should be significantly reduced.
Prior QbD studies resulted in eight crucial factors for DoE for the KPI (Figure 5) and no crucial factors for the selected impurities. The reduction of factors for a subsequent DoE study can reduce the experimental effort and can increase the power of the DoE. Details result cannot be shown for confidential reason at the moment and will be published separately.
This work aimed to demonstrate possible improvements within the QbD approach, which finally affect the process control strategy. Standard data science tools are combined to accelerate time to market by reducing experimental effort.
We established an improved QbD approach by using simple statistical tools and by combing existing approaches used for root cause analysis (RCA) [18]. Beyond the demonstration of new process knowledge gathering techniques [18],[19], a novel RA evaluation approach was developed [27] and demonstrated. The new approach allows that the current RA approach, the RPN approach, is no longer necessary since a more realistic PP criticality estimation is now present.
The primary benefit of this work is that a roadmap, beyond the appropriate use of data science tools, was established to:
The herein developed improvements of the QbD approach for control strategy can, therefore, be summarized as:
Within this contribution, a case study with DSP data was conducted to prove the suggested workflow improvements. It was shown that several improvements could be shown:
These presented achievements were feasible using the improved QbD approach. Based on that result, reduced time to market is assumed since meaningful impacts on process CQA can be identified and fewer experiments are needed with the DoE study. This reduction has an impact on the patients since drugs can be developed more efficiently concerning time to market.
For a comprehensive workflow elaboration, it should be pointed out that the limitation of the presented approach can be summarized as:
Although there are some limitations, we assume that modern Manufacturing Science and Technology (MSAT) Departments, as well as CMC Groups, can apply the herein presented approach. This is because process knowledge, skillset and rational thinking are usually available within the teams evaluating the process characterization.
We prove with the conducted case study, that:
As a whole, this work suggests a nQbD approach for process characterization purposes. The cQbD approach is commonly used and well established in the biopharmaceutical industry and has, therefore, a significant potential in its use. The correct interpretation of MVDA is crucial for further process characterization cycles. In order to accelerate and to facilitate the interpretation routine, reasonable data mining and a good process understanding are essential. It was shown that these steps need to be conducted already at the beginning of the data analysis and are crucial for the remaining analysis workflow. One solution for data mining procedures and process knowledge gathering is presented in this work. It shows that already simple steps with basic statistical methods can be applied to fulfill this aim, as long as this knowledge can be transferred to risk assessment. This transfer further facilitates the holistic process assessment by conducting the suggested linearization of Severity and Occurrence estimations to comprehensively assess the process design space leading to a new control strategy evaluation.
The scope if the work was to shown simple improvements on existing data science methods within QbD. Although machine learning or artificial intelligence algorithms may result in other results when included in the QbD, it is often difficult for process experts to follow such black box model approaches if they have not sufficient statistical or mathematical background. Moreover, interpretation of the relation between the variables might be difficult when using these algorithms.
Within a next step in the research field, we would suggest to include mechanistic model for data generation. Such models can be used to simulate unit operation where process data are missing or how additional unit operation within the process can affect the process holistically.
Concluding, it was shown that it is possible to improve the current and commonly used QbD approach with simple adjustments to decrease the overall process characterization efforts and thereby potentially accelerate time to market.
[1] | FDA, Guidance for Industry, 2011. Available from: https://www.fda.gov/downloads/drugs/guidances/ucm070336.pdf. |
[2] | Katz P, Campbell C (2012) FDA 2011 process validation guidance: process validation revisited. J GXP Compliance 16: 18-29. |
[3] | Guideline IHT (2005) Quality risk management Q9. |
[4] |
Politis NS, Colombo P, Colombo G, et al. (2017) Design of experiments (DoE) in pharmaceutical development. Drug Dev Ind Pharm 43: 889-901. doi: 10.1080/03639045.2017.1291672
![]() |
[5] |
Burdick RK, LeBlond DJ, Pfahler LB, et al. (2017) Process Design: Stage 1 of the FDA process validation guidance. Statistical Applications for Chemistry, Manufacturing and Controls (CMC) in the Pharmaceutical Industry Cham: Springer International Publishing, 115-154. doi: 10.1007/978-3-319-50186-4_3
![]() |
[6] | Doran PM (2013) Bioprocess Engineering Principles Boston: Elsevier. |
[7] |
Bunnak P, Allmendinger R, Ramasamy SV, et al. (2016) Life-cycle and cost of goods assessment of fed-batch and perfusion-based manufacturing processes for mAbs. Biotechnol Prog 32: 1324-1335. doi: 10.1002/btpr.2323
![]() |
[8] |
Diab S, Gerogiorgis DI (2018) Process modelling, simulation and technoeconomic evaluation of crystallisation antisolvents for the continuous pharmaceutical manufacturing of rufinamide. Comput Chem Eng 111: 102-114. doi: 10.1016/j.compchemeng.2017.12.014
![]() |
[9] |
Langer ES, Rader RA (2014) Continuous bioprocessing and perfusion: wider adoption coming as bioprocessing matures. BioProcessing J 13: 43-49. doi: 10.12665/J131.Langer
![]() |
[10] |
Fisher AC, Kamga MH, Agarabi C, et al. (2019) The current scientific and regulatory landscape in advancing integrated continuous biopharmaceutical manufacturing. Trends Biotechnol 37: 253-267. doi: 10.1016/j.tibtech.2018.08.008
![]() |
[11] | Herwig C, Glassey J, Kockmann N, et al. (2017) Better by Design: Qualtiy by design must be viewed as an opportunity not as a regulatory burden. The Chemical Engineer 915: 41-43. |
[12] |
Herwig C, Garcia-Aponte OF, Golabgir A, et al. (2015) Knowledge management in the QbD paradigm: manufacturing of biotech therapeutics. Trends Biotechnol 33: 381-387. doi: 10.1016/j.tibtech.2015.04.004
![]() |
[13] | CMC Biotech Working Group (2009) A-Mab: a Case Study in Bioprocess Development. |
[14] | Welcome to Python.org. Available from: https://www.python.org/. |
[15] |
Steinwandter V, Borchert D, Herwig C (2019) Data science tools and applications on the way to Pharma 4.0. Drug discov today 24: 1795-1805. doi: 10.1016/j.drudis.2019.06.005
![]() |
[16] | Guideline IHT (2009) Pharmaceutical Development Q8 (R2). |
[17] |
Thomassen YE, Van Sprang ENM, Van der Pol LA, et al. (2010) Multivariate data analysis on historical IPV production data for better process understanding and future improvements. Biotechnol Bioeng 107: 96-104. doi: 10.1002/bit.22788
![]() |
[18] |
Borchert D, Suarez-Zuluaga DA, Sagmeister P, et al. (2019) Comparison of data science workflows for root cause analysis of bioprocesses. Bioproc Biosyst Eng 42: 245-256. doi: 10.1007/s00449-018-2029-6
![]() |
[19] |
Suarez-Zuluaga DA, Borchert D, Driessen NN, et al. (2019) Accelerating bioprocess development by analysis of all available data: A USP case study. Vaccine 37: 7081-7089. doi: 10.1016/j.vaccine.2019.07.026
![]() |
[20] |
Kirdar AO, Green KD, Rathore AS (2008) Application of multivariate data analysis for identification and successful resolution of a root cause for a bioprocessing application. Biotechnol Prog 24: 720-726. doi: 10.1021/bp0704384
![]() |
[21] |
Sagmeister P, Wechselberger P, Herwig C (2012) Information processing: rate-based investigation of cell physiological changes along design space development. PDA J Pharm Sci Technol 66: 526-541. doi: 10.5731/pdajpst.2012.00889
![]() |
[22] |
Golabgir A, Gutierrez JM, Hefzi H, et al. (2016) Quantitative feature extraction from the Chinese hamster ovary bioprocess bibliome using a novel meta-analysis workflow. Biotechnol Adv 34: 621-633. doi: 10.1016/j.biotechadv.2016.02.011
![]() |
[23] |
Bowles JB (2003) An assessment of RPN prioritization in a failure modes effects and criticality analysis. The 2003 Proceedings Annual Reliability and Maintainability Symposium IEEE, 380-386. doi: 10.1109/RAMS.2003.1182019
![]() |
[24] | Sharma KD, Srivastava S (2018) Failure mode and effect analysis (FMEA) implementation: a literature review. J Adv Res Aeronaut Space Sci 5: 1-17. |
[25] |
Sharma RK, Kumar D, Kumar P (2007) Modeling system behavior for risk and reliability analysis using KBARM. Qual Reliab Eng Int 23: 973-998. doi: 10.1002/qre.849
![]() |
[26] |
Braglia M, Frosolini M, Montanari R (2003) Fuzzy TOPSIS approach for failure mode, effects and criticality analysis. Qual Reliab Eng Int 19: 425-443. doi: 10.1002/qre.528
![]() |
[27] |
Borchert D, Zahel T, Thomassen YE, et al. (2019) Quantitative CPP evaluation from risk assessment using integrated process modeling. Bioengineering 6: 114. doi: 10.3390/bioengineering6040114
![]() |
[28] |
Zahel T, Hauer S, Mueller EM, et al. (2017) Integrated process modeling—a process validation life cycle companion. Bioengineering 4: 86. doi: 10.3390/bioengineering4040086
![]() |
[29] |
Bonate PL (2001) A brief introduction to Monte Carlo simulation. Clin Pharmacokinet 40: 15-22. doi: 10.2165/00003088-200140010-00002
![]() |
[30] |
Wechselberger P, Sagmeister P, Engelking H, et al. (2012) Efficient feeding profile optimization for recombinant protein production using physiological information. Bioprocess Biosyst Eng 35: 1637-1649. doi: 10.1007/s00449-012-0754-9
![]() |
[31] |
Abu-Absi SF, Yang LY, Thompson P, et al. (2010) Defining process design space for monoclonal antibody cell culture. Biotechnol Bioeng 106: 894-905. doi: 10.1002/bit.22764
![]() |
[32] |
Liu H, Ricart B, Stanton C, et al. (2019) Design space determination and process optimization in at-scale continuous twin screw wet granulation. Comput Chem Eng 125: 271-286. doi: 10.1016/j.compchemeng.2019.03.026
![]() |
[33] | Guideline IHT (2011) Development and manufacture of drug substances (chemical entities and biotechnological/biological entities) Q11. |
1. | Sang-Ho Lee, Jin-Ki Kim, Jun-Pil Jee, Dong-Jin Jang, Young-Joon Park, Joo-Eun Kim, Quality by Design (QbD) application for the pharmaceutical development process, 2022, 52, 2093-5552, 649, 10.1007/s40005-022-00575-x | |
2. | Rüdiger W. Maschke, Barbara Pretzner, Gernot T. John, Christoph Herwig, Dieter Eibl, Improved Time Resolved KPI and Strain Characterization of Multiple Hosts in Shake Flasks Using Advanced Online Analytics and Data Science, 2022, 9, 2306-5354, 339, 10.3390/bioengineering9080339 |
Unit operation | Acceptable ∆CQA [%] KPI | Acceptable ∆CQA [%] Impurity 1 | Acceptable ∆CQA [%] Impurity 2 |
USP | 10 | 5 | 7 |
DSP UO1 | 10 | 20 | 20 |
DSP UO2 | 11 | 5* | 5* |
DSP UO3 | 7 | 22 | 20 |
DSP UO4 | 8 | 7 | 5 |
DSP UO5 | 4 | 2 | 2 |
Drug substance | 2.5 | 10 | 5 |