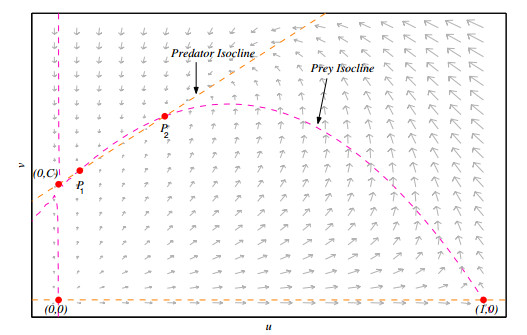
Successfully integrating newcomers into native communities has become a key issue for policy makers, as the growing number of migrants has brought cultural diversity, new skills, but also, societal tensions to receiving countries. We develop an agent-based network model to study interacting "hosts" and "guests" and to identify the conditions under which cooperative/integrated or uncooperative/segregated societies arise. Players are assumed to seek socioeconomic prosperity through game theoretic rules that shift network links, and cultural acceptance through opinion dynamics. We find that the main predictor of integration under given initial conditions is the timescale associated with cultural adjustment relative to social link remodeling, for both guests and hosts. Fast cultural adjustment results in cooperation and the establishment of host-guest connections that are sustained over long times. Conversely, fast social link remodeling leads to the irreversible formation of isolated enclaves, as migrants and natives optimize their socioeconomic gains through in-group connections. We discuss how migrant population sizes and increasing socioeconomic rewards for host-guest interactions, through governmental incentives or by admitting migrants with highly desirable skills, may affect the overall immigrant experience.
Citation: Yao-Li Chuang, Tom Chou, Maria R. D'Orsogna. A network model of immigration: Enclave formation vs. cultural integration[J]. Networks and Heterogeneous Media, 2019, 14(1): 53-77. doi: 10.3934/nhm.2019004
[1] | Yin Yang, Aiguo Xiao . Dissipativity and contractivity of the second-order averaged L1 method for fractional Volterra functional differential equations. Networks and Heterogeneous Media, 2023, 18(2): 753-774. doi: 10.3934/nhm.2023032 |
[2] | Ling Zhang, Xuewen Tan, Jia Li, Fan Yang . Dynamic analysis and optimal control of leptospirosis based on Caputo fractional derivative. Networks and Heterogeneous Media, 2024, 19(3): 1262-1285. doi: 10.3934/nhm.2024054 |
[3] | Verónica Anaya, Mostafa Bendahmane, David Mora, Ricardo Ruiz Baier . On a vorticity-based formulation for reaction-diffusion-Brinkman systems. Networks and Heterogeneous Media, 2018, 13(1): 69-94. doi: 10.3934/nhm.2018004 |
[4] | Liuchao Xiao, Wenbo Li, Leilei Wei, Xindong Zhang . A fully discrete local discontinuous Galerkin method for variable-order fourth-order equation with Caputo-Fabrizio derivative based on generalized numerical fluxes. Networks and Heterogeneous Media, 2023, 18(2): 532-546. doi: 10.3934/nhm.2023022 |
[5] | Chenchen Li, Chunyan Zhang, Lichao Feng, Zhihui Wu . Note on prescribed-time stability of impulsive piecewise-smooth differential systems and application in networks. Networks and Heterogeneous Media, 2024, 19(3): 970-991. doi: 10.3934/nhm.2024043 |
[6] | Vincent Renault, Michèle Thieullen, Emmanuel Trélat . Optimal control of infinite-dimensional piecewise deterministic Markov processes and application to the control of neuronal dynamics via Optogenetics. Networks and Heterogeneous Media, 2017, 12(3): 417-459. doi: 10.3934/nhm.2017019 |
[7] | Rossella Della Marca, Nadia Loy, Andrea Tosin . An SIR–like kinetic model tracking individuals' viral load. Networks and Heterogeneous Media, 2022, 17(3): 467-494. doi: 10.3934/nhm.2022017 |
[8] | Dag Lukkassen, Annette Meidell, Peter Wall . On the conjugate of periodic piecewise harmonic functions. Networks and Heterogeneous Media, 2008, 3(3): 633-646. doi: 10.3934/nhm.2008.3.633 |
[9] | Simone Göttlich, Stephan Martin, Thorsten Sickenberger . Time-continuous production networks with random breakdowns. Networks and Heterogeneous Media, 2011, 6(4): 695-714. doi: 10.3934/nhm.2011.6.695 |
[10] | Michael Burger, Simone Göttlich, Thomas Jung . Derivation of second order traffic flow models with time delays. Networks and Heterogeneous Media, 2019, 14(2): 265-288. doi: 10.3934/nhm.2019011 |
Successfully integrating newcomers into native communities has become a key issue for policy makers, as the growing number of migrants has brought cultural diversity, new skills, but also, societal tensions to receiving countries. We develop an agent-based network model to study interacting "hosts" and "guests" and to identify the conditions under which cooperative/integrated or uncooperative/segregated societies arise. Players are assumed to seek socioeconomic prosperity through game theoretic rules that shift network links, and cultural acceptance through opinion dynamics. We find that the main predictor of integration under given initial conditions is the timescale associated with cultural adjustment relative to social link remodeling, for both guests and hosts. Fast cultural adjustment results in cooperation and the establishment of host-guest connections that are sustained over long times. Conversely, fast social link remodeling leads to the irreversible formation of isolated enclaves, as migrants and natives optimize their socioeconomic gains through in-group connections. We discuss how migrant population sizes and increasing socioeconomic rewards for host-guest interactions, through governmental incentives or by admitting migrants with highly desirable skills, may affect the overall immigrant experience.
In recent years, Leslie-Gower type predator-prey models appear in various fields of the Mathematical Ecology, which have been proposed and studied extensively due to their increasing importance [1,2]. Among the most widely used mathematical predation models of this type, the Holling-Tanner model [3] (or May-Holling-Tanner [4,5]) plays a special role in view of the interesting dynamics it possesses [5]. It was proposed by James T. Tanner in 1975 [6] and based on the Leslie-Gower scheme [7], which was raised by the ecologist Patrick H. Leslie in 1948 [8].
These models are described by an autonomous bidimensional differential equation system characterized by the following aspects:
ⅰ) The prey-dependent functional response or predator consumption rate (depending only on the prey population) is hyperbolic, a particular form of Holling type Ⅱ [9,10]. It is described by the function $ h\left(x\right) = \frac{qx}{x+a} $, where $ x = x\left(t\right) $ is the prey population size. This function is known as the Michaelis-Menten function in Biochemical Kinetic.
ⅱ) The equation for predators is a logistic-type growth function [12,11,10]. So, the conventional environmental carrying capacity for predators $ K_{y} $ is expressed by a function of the available prey quantity [10]; in the seminal paper of P. H. Leslie [8], $ K_{y} $ is assumed proportional to prey abundance, i.e., $ K_{y} = K(x) = nx $. Implicitly, this formulation presupposes that the predator is a specialist.
In the logistic predator model, the quotient $ \frac{y}{nx} $, called the Leslie-Gower term, measures the loss in the predator population due to rarity (per capita $ \frac{y}{x} $) of its favorite food [13], where $ y = y\left(t\right) $ the predator population size. The importance of the Holling-Tanner model is highlighted by J. B. Collings in 1997 [14], who assured that it provides a way to avoid the biological control paradox wherein classical prey-dependent exploitation models generally do not allow for a pest (the prey) equilibrium density that is both low and stable [14].
The May-Holling-Tanner model [15,5,10] is studied partially in [3] and in the Murray's book [16]; it has been used in [17] to investigate numerically the dynamics of a predator-prey system for a pest in fruit-bearing trees, under the hypothesis that the parameters depend on the temperature; it has also shown its efficacy for describing the real ecological systems like mite/spider mite, canadian lynx/ snowshoe hare, sparrow/sparrow hawk and more [15].
In the paper by Saez and González-Olivares (1999) [5], its bifurcation diagram is described, establishing the existence of two limit cycles, surrounding the unique positive equilibrium point. Moreover, it is demonstrated that locally asymptotic stability of that equilibrium point does not imply global stability in this predator-prey model. This result implies the coexistence of a stable equilibrium and persistent oscillations [5]. Nevertheless, some authors have achieved conditions under which local stability of a positive equilibrium point implies its global stability [18].
The interesting phenomenon that local and global stability are not equivalent has also been shown in Leslie-Gower type models considering other mathematical form to describe the consumption function, as is shown in [12,19,4,20], where a non-monotonic functional response is assumed.
But the Leslie-Gower type models may present anomalies in its predictions, because it predicts that even in very low prey population density, when the consumption rate per predator is almost zero, predator population might increase, yet if the predator/prey ratio is very small [10]. Even so, it has been used to describe the interaction of certain populations [14,21].
Nonetheless, in the case of severe scarcity, some predator species can switch over to other available food, although its populational growth could be limited by the fact that its most favorite food is not available in abundance. This ability can be modelled by adding a positive constant $ c $ to the environmental carrying capacity for predators [22,13]. Thus, the $ c > 0 $ indicates the quantity of alternative aliment available for the predators.
Then, we have that environmental carrying capacity for predators $ K(x) = nx+c $; in that case, it is said that the model is represented by a Leslie-Gower scheme and it is also known as modified Leslie-Gower model [23,24]; if $ x = 0 $, then $ K(0) = c $, concluding that the predator is generalist since it choices an alternative food to avoid its extinction.
At the approach of the Leslie-Gower type predator-prey model [25] (with $ c = 0 $, considering implicitly a specialist predator), is assumed that a reduction in a predator population has a reciprocal relationship with per capita availability of its favorite food [13].
When $ c > 0 $, the modified May-Holling-Tanner model here analyzed has not these abnormalities and it enhances the predictions about the interactions. This model was proposed in [13], but focused on demonstrating the global stability of a unique positive equilibrium point.
On the other hand, one of the main elements of the predator-prey relationship is the predator functional response or consumption function, which refers to the change in attacked prey density per unit of time per predator when the prey population size changes [9]. In many predator-prey models is assumed that the functional response grows monotonic, being the inherent assumption the more prey in the environment, the better for the predators [10].
We will consider that the predator consumption function is prey-dependent and expressed by the hyperbolic function $ h(x) = \ \frac{q\ x}{x\ +\ a} $ [26,27] a particular case of a Holling type Ⅱ functional response [9]. The parameter $ a $ is a abruptness measure of the functional response [28]. If $ a\rightarrow 0 $, the curve grows quickly, while if $ a\rightarrow K $, the curve grows slowly, that is, a bigger amount of prey is needed to obtain $ \frac{q}{2} $.
The behavior of the system will be described according to the obtained constraints on the parameter values and classifying the different and rich dynamics resulting that have not been exposed on earlier works.
Although there are various works in which the model here proposed has been partially analyzed [23,24,1,29,30,2], new and novel properties of the model are here established such as: the existence of different class of bifurcations (Bogdanov-Takens, generalized Hopf, homoclinic and heteroclinic bifurcations); the existence up to two positive equilibrium points (see Figure 1), depending on the relative positions of isoclines, being one of them always a saddle point; the existence of at least one limit cycle encircling a stable positive equilibrium point; a separatrix curve in the phase plane which divides the behavior of the trajectories, that implies the existence of solutions nearest to that separatrix having different $ \omega -limit $, i.e., they are highly sensitives to the initial conditions [4,31].
Furthermore, using the computation of Lyapunov numbers (or quantities) [32,33], we were able to demonstrate the existence of two limit cycles, when a weak (or fine) focus occurs, being the innermost unstable and the outermost stable, showing examples to illustrate this property for different obtained cases.
Hence, our analysis allows to extend the properties of the model proposed in a highly cited article [13] and we complement the outcomes of the Holling-Tanner model established in [5], showing the existence of the phenomenon of multistability, when it exists: a local attractor positive equilibrium, a stable limit cycle and a stable equilibrium point over the $ y-axis $.
The rest of the paper is organized as follows: In the next Section, the modified May-Holling-Tanner model is presented; in Section 3, the main properties of the model are proved; in Section 4 we discuss the obtained results, giving the ecological meanings of them. To reinforce these results, some numerical simulations are shown in the last section.
The modified May-Holling-Tanner model [4,5] to be analyzed, considering a generalist predator, is described by the following autonomous bidimensional differential equation system of Kolmogorov type [34] :
$ Xμ:{dxdt=(r(1−xK)−qyx+a)xdydt=s(1−ynx+c)y $
|
(2.1) |
where $ x = x(t) $ and $ y = y(t) $ indicate the prey and predator population sizes respectively, for $ t\geq 0 $, measured as the number of individuals, biomass, density by unit of area or volume. The parameters are all positives, i. e., $ \mu = (r, K, q, a, s, n, c)\in \mathbb{R}{}{}_{+}^{7} $ and for ecological reasons $ a < K $; the parameters have their meanings are given in the following table:
Parameters | Meanings |
$ r $ | intrinsic prey growth rate or biotic potential |
$ K $ | prey environmental carrying capacity |
$ q $ | maximum number of prey that can be eaten by a predator in each time unit |
$ a $ | amount of prey to achieve one-half of the maximum rate $ q $ |
$ s $ | intrinsic predator growth rate |
$ n $ | measure of the food quality |
$ c $ | amount of alternative food available for predators |
The parameter $ n $ indicates also how the predators turn eaten prey into new predator births, and $ c $ is expresses that the predator is generalist, i.e., if it does not exist available prey, it switch to an alternative food. We note if $ c = 0 $ the May-Holling-Tanner model is obtained which is not defined in $ x = 0 $ and whose dynamics was described in [5].
In system $ \left(2.1\right) $, the growth population rate of predators, expressed by $ \frac{dy}{dt} $, becomes larger when $ c $ increases. This is in accordance with the ecological fact that if the predator is more capable of changing from its favorite prey to other food options, it can survive more easily when the prey is lacking severely [13].
As system $ \left(2.1\right) $ is of Kolmogorov type, the coordinates axis are invariable sets and the model is defined at
$ \Omega = \left\{ \left( x,y\right) \in \mathbb{R}^{2}/\ x\geq 0,\ y\geq 0\right\} = \mathbb{R}_{0}^{+}\times \mathbb{R}_{0}^{+} . $ |
The equilibrium points of system $ \left(2.1\right) $ or vector field $ X_{\mu } $ are $ (K, 0) $, $ (0, 0) $, $ (0, c) $ and $ (x_{e}, y_{e}) $, where $ x_{e} $ and $ y_{e} $ satisfy the equation of the isoclines $ y = nx+c $ and $ y = \frac{r}{q}\left(1-\frac{x}{K}\right) \left(x+a\right) $. Clearly, $ (x_{e}, y_{e}) $ can be a positive equilibrium point (equilibrium at the interior of the first quadrant) or cannot exists there, depending on the sign of factor $ 1-\frac{x}{K} $.
System $ \left(2.1\right) $ has been used to study non-autonomous versions by incorporating delay [29] or impulses [35,2] as well as autonomous model considering partial derivatives [36]. In spite of these recent studies, the dynamics of the system $ \left(2.1\right) $ have not fully analyzed and some obtained results are not true or well established.
In this paper, we show that local and global stabilities are not equivalent to this class of predator-prey models. The existence of subsets on the parameter space is proved for which there exist a bifurcation manifold of semistable limit cycles and an open manifold, where a positive equilibrium point is locally stable and surrounded by at least two limit cycles.
In order to simplify the calculations, it is convenient to reduce system $ \left(2.1\right) $ to a normal form; so, we follow the methodology used in [37,20,11,5], making a change of variable and a time rescaling, by means of a diffeomorphism [32]. So we have the following:
Proposition 1. (Topological equivalent system)
System $ \left(2.1\right) $ is topologically equivalent to the polynomial system given by
$ Yη(u,v):{dudτ=((1−u)(u + A)−Qv)u(u+C)=M(u,v)dvdτ=S(u+C−v)(u+A) v=N(u,v) $
|
(2.2) |
where $ \eta = \left(A, S, C, Q\right) \in $ $ \left] 0, 1\right[ \times \mathbb{R} _{+}^{3} $ with $ A = \frac{a}{K} < 1 $, $ \ S = \frac{s}{r} $, $ C = \frac{c}{Kn} $ and $ Q = \frac{nq}{r} $.
Moreover, system $ \left(2.2\right) $ is defined on the set $ \bar{\Omega} = \left\{ \left(u, v\right) \in \mathbb{R} ^{2}:0\leq u\mathit{\text{, }}0\leq v\right\} $.
Proof. Let $ x = Ku $ and $ y = $ $ nKv $; substituting into the system $ \left(2.1\right) $, simplifying and factoring, we obtain
$
U_{\mu }\left( x,y\right) :\left\{ dudt=r((1−u)−qu+aKnrv)udvdt=s(1−vu+cnK)v
\right.
$
|
Now, using the time rescaling given by $ \tau = \frac{r}{\left(u+\frac{a}{K} \right) \left(u+\frac{c}{nK}\right) }t $;
then,
$ \frac{du}{dt} = \frac{du}{d\tau }\frac{d\tau }{dt} = \frac{du}{d\tau } \frac{r}{\left(u+\frac{a}{K}\right) \left(u+\frac{c}{nK}\right) } \; {\rm{and}} \; \frac{dv}{dt} = \frac{dv}{d\tau }\frac{d\tau }{dt} = \frac{dv}{dt}\frac{r}{ \left(u+\frac{a}{K}\right) \left(u+\frac{c}{nK}\right) }. $ |
Rearranging and simplifying
$
V_{\mu }\left(x, y\right) :\left\{ dudt=((1−u)(u+aK)−qnrv)udvdt=sr(u+cnK−v)v(u+aK)
\right.
$
|
Making the above indicated substitution, system $ \left(2.2\right) $ is obtained.
Remark 2. 1. We have constructed the diffeomorphism$ \ $ $ \varphi :\bar{\Omega}\times \mathbb{R}\longrightarrow \Omega \times \mathbb{R} $, so that
$ \varphi (u,v,\tau ) = \left( Ku,nKv,\frac{1}{r}\left( u+\frac{c}{nK}\right) \left( u\ +\ \frac{a}{K}\right) \tau \right) = (x,y,t). $ |
The Jacobian matrix of $ \varphi $ is
$
D\varphi \left( u,v,\tau \right) = \left( K000nK01Knr(c+an+2Knu)τ01r(u+cnK)(u + aK)
\right)
$
|
and we have that $ \det D\varphi (u, v, \tau)\; = \frac{nK^{2}}{r}\left(u+\frac{ c}{nK}\right) \left(u\ +\ \frac{a}{K}\right) > 0 $.
Then, the diffeomorphism $ \varphi $ is a smooth change of variables with a rescaling of the time preserving the time orientation; thus, the vector field $ X_{\mu }\left(x, y\right) $, is topologically equivalent to the vector field $ Y_{\eta }\; = \; \varphi \circ X_{\mu } $ with $ Y_{\eta }\left(u, v\right) = M(u, v) \frac{\partial }{\partial u}+N(u, v) \frac{\partial }{ \partial v} $ and the associated differential equation system is given by the polynomial system of fourth degree of Kolmogorov type [34].
2. With this parameterization and time rescaling we have obtained a representative system with the least quantity of parameters possible; system $ \left(2.2\right) $ describes the dynamical behaviors of all those systems topologically equivalent to the system $ \left(2.1\right) $. Therefore, more important than knowing the influence of a specific parameter in the dynamical behavior of the system $ \left(2.1\right) $, it may be best to know the relationships between some of them, which also permits a simple description of the system properties.
The equilibrium points of system $ \left(2.2\right) $ or singularities of vector field $ Y_{\eta } $ are $ (1, 0) $, $ (0, 0) $, $ (0, C) $ and the points $ \left(u_{e}, v_{e}\right) $ which lie over the curves:
$ v = \frac{1}{Q}\left( 1-u\right) (u\ +\ A) \; {\rm{and}} \; v = u+C . $ |
Then, the abscissa $ u $ of the positive equilibrium points is a solution of the second-degree equation:
$ u2−(1−A−Q)u+(CQ−A)=0, $
|
(2.3) |
Considering the Descartes' Rule of Signs and according to the sign of the factors $ B_{1} = 1-A-Q $ and $ B_{0} = CQ-A $ and $ \Delta \left(\eta \right) = \left(1-A-Q\right) ^{2}-4\left(CQ-A\right) $, the equation $ \left(2.3\right) $ has two, one or none positive roots. In the following, we describe the diverse cases existing for the equation $ \left(2.3 \right) $.
1) Assuming $ B_{1} = 1-A-Q > 0 $, $ B_{0} = $ $ CQ-A > 0 $ and
1.1 $ \Delta \left(\eta \right) > 0 $, the solutions are:
$ u1=12(1−A−Q−√Δ(η)) and u2=12(1−A−Q+√Δ(η)), $
|
(2.4) |
1.2 $ \Delta \left(\eta \right) = 0 $, the solution is $ u^{\ast } = \frac{1}{2} \left(1-A-Q\right) $,
1.3 $ \Delta \left(\eta \right) < 0 $, there is no positive solution.
2) The solutions are $ u_{1} < 0 < u_{2} $, if and only if,
2.1)$ \qquad 1-A-Q > 0 $ and $ CQ-A < 0 $, or else,
2.2)$ \qquad 1-A-Q < 0 $ and $ CQ-A < 0 $.
3) If $ 1-A-Q > 0 $ and $ CQ-A = 0 $, there are two solutions
$ \qquad u_{1} = 0 $ and $ u_{2} = \ G = 1-A-Q = 1-A-\frac{A}{C} > 0 $.
4) If $ 1-A-Q = 0 $ and $ CQ-A < 0 $, we have two solutions, $ u_{1} < 0 < u_{2} $.
5) Moreover, equation $ \left(2.3\right) $ does not have real roots, if and only if,
5.1 $ 1-A-Q = 0 $ and $ CQ-A > 0 $, or
5.2 $ 1-A-Q < 0 $ and $ CQ-A > 0 $.
According to the above analysis of equation $ \left(2.3\right) $ we have:
1. Assuming $ B_{1} = 1-A-Q > 0 $ and $ B_{0} = CQ-A > 0 $, then, there exists three possibilities for system $ \left(2.2\right) $:
1.1. There are two equilibrium points at interior of the first quadrant, if and only if, $ C < \frac{1}{4Q}\left(4A+\left(1-A-Q\right) ^{2}\right) $, which are $ P_{1} = \left(u_{1}, u_{1}+C\right) $ and $ P_{2} = \left(u_{2}, u_{2}+C\right) $ with $ 0 < u_{1} < u_{2} < 1 $.
We note that the coordinates of the points $ P_{1} $ and $ P_{2} $ do not depend on the parameter $ S $.
1.2. There is a unique equilibrium point at interior of the first quadrant, if and only if, $ \Delta \left(\eta \right) = 0 $. In this case, the points $ P_{1} $ and $ P_{2} $ coincide, i.e.,
$ \left( u_{1},u_{1}+C\right) = \left( u_{2},u_{2}+C\right) = \left( E,E+C\right) $ |
with $ E = \frac{1-A-Q}{2} $ and $ C = \frac{1}{4Q}\left(4A+\left(1-A-Q\right) ^{2}\right) $.
1.3. For $ 1-A-Q > 0 $ and $ CQ-A = 0 $, there are not equilibrium points at interior of the first quadrant, if and only if, $ C > \frac{1}{4Q}\left(4A+\left(1-A-Q\right) ^{2}\right) $.
The case 1.1 is shown in the Figure 1.
2. In this case, the unique equilibrium point at interior of the first quadrant is, $ P_{2} = \left(u_{2}, u_{2}+C\right) = \left(L, L+C\right) $
with $ L = \frac{1}{2}\left(1-A-Q+\sqrt{\Delta \left(\eta \right) }\right) $. According to the relation between $ C $ and $ L $, the point $ P_{1} $ lies in the second or the third quadrant.
3. Clearly, the point $ P_{1} $ coincides with $ \left(0, C\right) $. Then, $ \left(\frac{C-A-AC}{C}, \frac{\left(C-A\right) \left(C+1\right) }{C} \right) $ is the unique equilibrium point at interior of the first quadrant.
4. For $ 1-A = Q $, the unique equilibrium point at interior of the first quadrant is $ P_{2} = \left(F, F+C\right) $ with $ F = \sqrt{A-C\left(1-A\right) } $, and $ A-C\left(1-A\right) > 0 $. So, $ C < \frac{A}{1-A} $. Moreover, the point $ P_{1} = \left(-F, -F+C\right) $ lies in the second or the third quadrant.
5. There are not equilibrium points at interior of the first quadrant, if and only if,
5.1. $ 1-A-Q = 0 $ and $ CQ-A\geq 0 $, or
5.2. $ 1-A-Q < 0 $ and $ CQ-A\geq 0 $.
The above classification 1-5 implies the study of different cases in this family of systems, according to the quantity of the equilibrium points and the relations between the parameters $ A $, $ C $ and $ Q $. We note that $ A $ is the intercept of the prey isocline with the $ v-axis $; then, the relative position among $ C $ and $ A $ over this axis, influences the quantity of positive equilibrium points and the nature of these equilibriums.
In short, we summarize the different cases to study in the following table (Table 2).
Case | sg$\left(B_{1}\right) $ | sg$\left(B_{0}\right) $ | sg$\left(\Delta \left(\eta \right) \right) $ | Number of Positive equilibrium |
1.1 | $+$ | $+$ | $+$ | $2$ |
1.2 | $+$ | $+$ | $0$ | $1$ |
1.3 | $+$ | $+$ | $-$ | $0$ |
2.1 | $+$ | $-$ | $+$ | $1$ |
2.2 | $-$ | $+$ | $+$ | $1$ |
3 | $+$ | $0$ | $+$ | $1$ |
4 | $0$ | $-$ | $+$ | $1$ |
5.1 | $0$ | $+$ | $-$ | $0$ |
5.2 | $-$ | $+$ | $-$ | $0$ |
We note system $ \left(2.1\right) $ has a significant difference with May-Holling-Tanner model (when $ C = 0 $), respect to the quantity of equilibrium points [5], since system $ \left(2.1\right) $ can have up to two positive equilibrium points, apart from of the new equilibrium $ \left(0, C\right) $ (see Figure 1). Meanwhile, in the May-Holling-Tanner model [3,5] there exists a unique positive equilibrium point (the point $ P_{1} $ lies in the third quadrant); nevertheless, other dynamical differences between both models will be established.
To determine the local nature of the equilibrium points we will use the Jacobian matrix of system $ \left(2.2\right) $ which is:
$ DYη(u,v)=(DYη(u,v)11−Qu(u+C)Sv(A+C+2u−v)S(A+u)(C+u−2v)) $
|
with
$ DY_{\eta }\left(u, v\right)_{11} = -4u^{3}+3\left(1-C-A\right) u^{2}+2\left(\left(A+C\left(1-A\right) -Qv\right) \right) u+C\left(A-Qv\right) . $ |
For system $ \left(2.2\right) $ we have the following general properties:
Lemma 3. (Existence of positevely invariant region)
The set $ \tilde{\Gamma} = \left\{ \left(u, v\right) \in \bar{\Omega}/\ 0\leq u\leq 1, \; v\geq 0\right\} $ is a region positevely invariant.
Proof. Clearly the $ u-axis $ and the $ v-axis $ are invariant sets because the system is a Kolmogorov type. If $ u = 1 $, we have
$ \frac{du}{d\tau } = -Qv\left( 1+C\right) \lt 0 $ |
and whatever it is the sign of
$ \frac{dv}{d\tau } = S\left( 1+A\right) \left( \ 1+C-\ v\right) \ v $ |
the trajectories enter and remain in the region $ \tilde{\Gamma} $.
Lemma 4. (Boundedness of solutions)
The solutions are bounded
Proof. See [13] or else, applying the Poincaré compactification and the directional blowing-up method [32,33], using the change of variables $ X = rw $ and $ Y = w $ and the time rescaling given by $ \zeta = w^{2}T $; after doing a large algebraic work ([38,39]) a new system is obtained, in which the point $ \left(0, \infty \right) $ is a non-hyperbolic saddle point.
Lemma 5. For all $ \eta = (A, S, C, Q)\in ]0, 1[\times \mathbb{R}_{+}^{3} $
1. The equilibrium $ \left(1, 0\right) $ is a saddle point.
2. The equilibrium $ \left(0, 0\right) $ is a repeller point.
Proof. Evaluating the Jacobian matrix in each point is immediate that
1) $ \det DY_{\eta }\left(1, 0\right) = -S\left(A+1\right) ^{2}\left(1+C\right) ^{2} < 0 $.
Therefore, the equilibrium $ \left(1, 0\right) $ is saddle point.
2) $ \det DY_{\eta }(0, 0) = A^{2}C^{2}S > 0 $ and tr$ DY_{\eta }(0, 0) = AC\left(1+S\right) > 0 $.
Then, the equilibrium $ \left(0, 0\right) $ is a repeller point.
Lemma 6. The equilibrium $ \left(0, C\right) $ is
i) a saddle point, if and only if, $ CQ-A < 0 $.
ii) an attractor point, if and only if, $ CQ-A > 0 $.
iii) a non hyperbolic attractor point, if and only if, $ CQ-A = 0 $.
Proof. It is immediate, since evaluating the Jacobian matrix in the point $ \left(0, C\right) $ we obtain
$ {\rm{det}} DY_{\eta }\left(0, C\right) = AC^{2}S\left(CQ-A\right) \; {\rm{and}} $ |
$ {\rm{tr}} DY_{\eta }\left(0, C\right) = C\left(A-AS-CQ\right) . $ |
Therefore, the point $ \left(0, C\right) $ is
ⅰ) a saddle point, if and only if, $ CQ-A < 0 $, because $ \det DY_{\eta }\left(0, C\right) < 0 $.
ⅱ) an attractor point, if and only if, $ CQ-A > 0 $, since $ \det DY_{\eta }\left(0, C\right) > 0 $ and tr$ DY_{\eta }\left(0, C\right) < 0 $.
ⅲ) If$ \ CQ-A = 0 $, then, we obtain that $ \det DY_{\eta }\left(0, C\right) = 0 $, tr$ DY_{\eta }\left(0, C\right) < 0 $ and the Jacobian matrix has an eigenvalue zero.
Remark 7. 1. These above results confirm the fact that the predator population is generalist; then, its extinction is avoided.
When the favorite prey is scarse($ u = 0 $), the predators attain their environmental carrying capacity $ C $.
2. Let us $ W^{u}\left(1, 0\right) $, the unstable manifold of the hyperbolic saddle point $ \left(1, 0\right) $, and $ \bar{\Sigma} = W^{s}\left(0, C\right) $, the stable manifold of the saddle point $ \left(0, C\right) $ (hypebolic or not).
Then, the relative position of both manifold determines a heteroclinic curve, when $ W^{u}\left(1, 0\right) \cap \bar{\Sigma}\neq \phi $.
3. We note that the positive equilibria lie in the region
$ ˉΛ={(u,v)∈ˉΓ / 0≤u≤1,0≤v≤vΣ,suchthat(u,vΣ)∈ˉΣ}. $
|
In the following, we consider only the case 1, in which there exists two positive equilibrium point at interior of the first quadrant, they collapse or they do not exist there. In this case, the point $ \left(0, C\right) $ is a local attractor.
The positive singularities must fulfill the equation of the predator isocline $ v = u+C $ and the prey isocline $ \left(1-u\right) \left(u\ +\ A\right) -Qv = 0 $; so. we obtain
$
DY_{\eta }\left(u, u+C\right) = \left(C+u\right) \left(−u(A+2u−1)−QuS(A+u)−S(A+u)
\right) .
$
|
Thus,
$ {\rm{det}} DY_{\eta }\left( u,u+C\right) = S\left( C+u\right) ^{2}\left( A+u\right) u\left( A+Q+2u-1\right) {\rm{and}} $ |
$ {\rm{tr}} DY_{\eta }\left( u,u+C\right) = \left( C+u\right) \left( u\left( 1-2u-A\right) -S\left( A+u\right) \right) $ |
Remark 8. Remembering $ B_{1} = 1-A-Q > 0 $, the sign of det$ DY_{\eta }\left(u, u+C\right) $ depends on the factor $ 2u-\left(1-A-Q\right) $.
At once, the sign of tr$ DY_{\eta }\left(u, u+C\right) $ depends on the sign of
$ T\left(u, A, S\right) = u\left(1-2u-A\right) -S\left(A+u\right) $.
We have,
a) If $ u > \frac{1-A-Q}{2} $ implies that det$ DY_{\lambda }\left(u, u+C\right) > 0 $ and the nature of singularity depends on the sign of the tr$ DY_{\lambda }(u, u+C) $.
b) If $ u < \frac{1-A-Q}{2} $, then det$ DY_{\lambda }\left(u, u+C\right) < 0 $ and $ \left(u, u+C\right) $ is a saddle point.
c) If $ u = \frac{1-A-Q}{2} $, then the two equilibrium points coincide.
Theorem 9. Nature of the first positive equilibrium
The equilibrium point $ P_{1} = \left(u_{1}, u_{1}+C\right) $ is a saddle point.
Proof. As det$ DY_{\eta }\left(u_{1}, u_{1}+C\right) = Su_{1}\left(C+u_{1}\right) ^{2}\left(A+u\right) \left(2u_{1}-\left(1-A-Q\right) \right) $, then
$ u_{1}-\frac{1-A-Q}{2} = \frac{1}{2}\left( 1-A-Q-\sqrt{\Delta \left( \eta \right) }\right) -\frac{1-A-Q}{2} = -\frac{1}{2}\sqrt{\Delta \eta } \lt 0 $ |
Therefore, the equilibrium $ \left(u_{1}, u_{1}+C\right) $ is a saddle point.
We note that the point $ \left(u_{1}, u_{1}+C\right) $ is not in the interior of the first quadrant, if and only if, $ CQ-A < 0 $, and it coincides with $ \left(0, C\right) $, if and only if, $ CQ-A = 0 $.
Remark 10. Existence of a separatrix curve
Let $ W_{+}^{s}\left(P_{1}\right) $ be the superior stable manifold of $ P_{1} = \left(u_{1}, u_{1}+C\right) $; it originates a separatrix curve $ \bar{\Sigma} $, in the phase plane, whose $ \alpha -limit $ can stay out or inside $ \bar{\Gamma} $. Any solutions having initial conditions above this separatrix has the point $ \left(0, C\right) $ as its $ \omega -limit $.
Theorem 11. (Existence of a heteroclinic curve)
A subset of parameter exists for which a heteroclinic curve joining the equilibrium points $ \left(1, 0\right) $ and $ \left(u_{1}, u_{1}+C\right) $.
Proof. Let $ W^{u}\left(1, 0\right) $ the unstable manifold of the saddle point $ \left(1, 0\right) $ and $ W_{+}^{s}\left(P_{1}\right) $ the superior stable manifold of $ P_{1} = \left(u_{1}, u_{1}+C\right) $. It is clear that the curve determined by the unstable manifold $ W^{u}\left(1, 0\right) $ remains at $ \bar{\Gamma} $ by Lemma 3 and its $ \omega -limit $ can be the point $ P_{2} = \left(u_{2}, u_{2}+C\right) $ or a stable limit cycle surrounding that point.
Assuming that the $ \alpha -limit $ of $ W_{+}^{s}\left(P_{1}\right) $ is out of $ \bar{\Gamma} $, then the curve $ \bar{\Sigma} $, is above the curve determined by $ W^{u}\left(1, 0\right) $. If the $ \alpha -limit $ of $ W_{+}^{s}\left(P_{1}\right) $ is inside of $ \bar{\Gamma} $, then the curve $ \bar{\Sigma} $ is below the curve determined by $ W^{u}\left(1, 0\right) $.
Then, by the Existence and Uniqueness Theorem of solutions [32], there exists a subset on the parameter space for which the two manifolds coincide, forming a heteroclinic curve.
Remark 12. The nature of the equilibrium $ P_{2} = \left(u_{2}, u_{2}+C\right) $ depends of the relation between $ v_{u} $ and $ v_{s} $, the ordinate of the points $ \left(u^{\ast }, v_{u}\right) \in W^{u}\left(1, 0\right) $ and $ \left(u^{\ast }, v_{s}\right) \in \bar{\Sigma} $, respectively.
Theorem 13. (Nature of the second positive equilibrium)
Let us $ u = u^{\ast } $, any value of $ u $ such that $ u_{1} < u^{\ast } < 1 $.
Considering $ \left(u^{\ast }, v^{s}\right) \in W^{s}\left( u_{1}, v_{1}\right) = \bar{\Sigma} $ and $ \left(u^{\ast }, v^{u}\right) \in W^{s}\left(1, 0\right) $, and
a) Assuming $ v^{u} < v^{s} $, we have:
The equilibrium point $ P_{2} = \left(u_{2}, u_{2}+C\right) $ is
a1) an attractor point, if and only if, $ S > \frac{u_{2}\left( 1-2u_{2}-A\right) }{u_{2}+A} $,
a2) a repeller, if and only if, $ S < \frac{u_{2}\left(1-2u_{2}-A\right) }{ u_{2}+A} $; furthermore, there exists, at least a limit cycle surrounding this equilibrium point.
a3) a weak focus, if and only if, $ \ S = \frac{u_{2}\left(1-2u_{2}-A\right) }{u_{2}+A} $ and a Hopf bifurcation occurs.
b) Assuming $ v^{u} > v^{s} $, it has that: The equilibrium $ P_{2} = \left(u_{2}, u_{2}+C\right) $ is
b1) an attractor surrounded by a unstable limit cycle, if and only if, $ S > \frac{u_{2}\left( 1-2u_{2}-A\right) }{u_{2}+A} $,
b2) a repeller (node or focus) and the trajectories have the point $ \left(0, u_{2}+C\right) $ as their $ \omega -limit $, being this point an almost globally stable equilibrium [45,43].
Proof. a) As the Jacobian matrix is
$
DY_{\eta }(u_{2},u_{2}+C) = \left( u_{2}+C\right) \left( −(A+2u2−1)u2−Qu2S(u2+A)−S(u2+A)
\right)
$
|
then,
det$ DY_{\eta }(u_{2}, u_{2}+C) = S\left(u_{2}+C\right) ^{2}\left(u_{2}+A\right) \left(2u_{2}-M\right) u_{2} > 0 $.
Since $ 2u_{2}-\left(1-A-Q\right) = 1-A-Q+\sqrt{\Delta \left(\eta \right) } -\left(1-A-Q\right) = \sqrt{\Delta \left(\eta \right) } > 0 $
the nature of $ \left(u_{2}, u_{2}+C\right) $ depends on the sign of
$ {\rm{tr}} DY_{\eta }(u_{2},u_{2}+C) = \left( C+u_{2}\right) \left( u_{2}\left( 1-2u_{2}-A\right) -S\left( u_{2}+A\right) \right) $ |
i.e., the sign depends on the factor
$ T\left(u_{2}, A, S\right) = u_{2}\left(1-2u_{2}-A\right) -S\left(u_{2}+A\right) . $ |
We have,
a1) tr$ DY_{\eta }\left(u_{2}, u_{2}+C\right) < 0 $, if and only if, $ S > \frac{ u_{2}\left(1-2u_{2}-A\right) }{u_{2}+A} $; therefore, the point $ \left(u_{2}, u_{2}+C\right) $ is an attractor.
a2) tr$ DY_{\eta }\left(u_{2}, u_{2}+C\right) > 0 $, if and only if, $ S < \frac{ u_{2}\left(1-2u_{2}-A\right) }{u_{2}+A} $; then, the point $ \left(u_{2}, u_{2}+C\right) $ is a repeller.
As the trace changes sign, a Hopf bifurcation occurs [32] at the equilibrium point $ \left(u_{2}, u_{2}+C\right) $; then, the point $ \left(u_{2}, u_{2}+C\right) $ is surrounded by a stable limit cycle.
Furthermore, the transversality condition [32] is verified, since we have that
$ \frac{\partial }{\partial S}\left( \text{tr}DY_{\eta }(u_{2},u_{2}+C)\right) = -\left( u_{2}+A\right) $ |
a3) tr$ DY_{\eta }\left(u_{2}, u_{2}+C\right) = 0 $, if and only if, $ S = \frac{ u_{2}\left(1-2u_{2}-A\right) }{u_{2}+A} $; thus, the point $ \left(u_{2}, u_{2}+C\right) $ is a weak focus, whose weakness must be determined.
b) When $ v^{u} > v^{s} $, the $ \alpha-limit $ of the $ W^{s}\left(u_{1}, v_{1}\right) $ can be the repeller equilibrium $ P_{2} = \left(u_{2}, u_{2}+C\right) $ or an unstable limit cycle surrounding the point $ P_{2} $, when this is an attractor equilibrium.
In the first situation, the $ W_{+}^{u}\left(P_{1}\right) $ must coincide with the $ W_{+}^{u}\left(1, 0 \right) $, since the Existence and Uniqueness Theorem applies in the region $ \bar{\Lambda} $, or its $ \omega -limit $ is the equilibrium $ \left(0, C\right) $.
Assuming the existence of the an unstable limit cycle surrrounding the point $ P_{2} $, therefore this point must be an attractor equilibrium. Then, when the parameters change, that limit cycle coincides with the $ W_{+}^{s}\left(P_{1}\right) $, and after is broken. Thus, all the trajectories, except the point $ P_{2} $, tends to the equilibrium $ \left(0, C\right) $, which is an almost global attractor [45,43].
Remark 14. The equilibrium $ P_{2} = \left(u_{2}, u_{2}+C\right) $ can be node or focus depending of the quantity
$ H = \left( \text{tr}DY_{\eta }(u_{2},u_{2}+C)\right) ^{2}-4\left( \det DY_{\eta }(u_{2},u_{2}+C)\right) . $ |
After a few algebraic manipulations it has that the sign of $ H $ depends on the factor
$ H_{1} = \left(A+u_{2}\right) ^{2}S^{2}+ 2u_{2}\left(A+u_{2}\right) \left(A+2\left(1-A-Q\right) -2u_{2}-1\right) S+ u_{2}^{2}\left(A+2u_{2}-1\right) ^{2} $
As it is well-known, the point $ \left(u_{2}, u_{2}+C\right) $ is a focus, if and only if, $ H_{1} < 0 $; it is a node, if and only if, $ H_{1} > 0 $.
Theorem 15. (Existence of homoclinic curve)
There are conditions on the parameter values for which:
a) It exists a homoclinic curve determined by the stable and unstable manifold of point $ P_{1} = \left(u_{1}, u_{1}+C\right) $.
b) It exists a non-infinitesimal limit cycle that bifurcates from the homoclinic [41,44] surrounding the point $ P_{2} = \left( u_{2}, u_{2}+C\right) $.
Proof. We note that if the point $ \left(u, v\right) \in \overline{P_{1}P_{2}} $, then $ \frac{du}{dt} > 0 $; clearly, the direction of the vector field at the point lying in the straight line $ v = u+C $ is to the right, since
$ \frac{du}{dt} = \left(\ \left(1-u\right) \left(u\ +\ A\right) -Q\left(u+C\right) \right) u\left(u+C\right) \gt 0 $ and $ \frac{dv}{dt} = 0. $ |
Considering $ W_{+}^{s}\left(P_{1}\right) $ and $ W_{+}^{u}\left(P_{1}\right) $, the superior stable manifolds and the right unstable manifolds of $ P_{1} $, we have:
a) As $ \bar{\Gamma} $ is an invariant region, the orbits cannot cross the straight line $ u = 1 $ towards the right. The trajectory determined by the right unstable manifold $ W_{+}^{u}\left(P_{1}\right) $ cannot cut or cross the trajectory determined by the superior stable manifold $ W_{+}^{s}\left(P_{1}\right) $, by Theorem of existence and uniqueness (see Figure 2).
Moreover, the $ \alpha -limit $ of the $ W_{+}^{s}\left(P_{1}\right) $ can lie at the point $ \left(1, 0\right) $ by lemma 3 or at infinity in the direction of $ u-axis $.
On the other hand, the $ \omega -limit $ of the right unstable manifold $ W_{+}^{u}\left(P_{1}\right) $ must be:
ⅰ) the point $ P_{2} $, when this is an attractor;
ⅱ) a stable limit cycle, if $ P_{2} $ is a repeller.
ⅲ) the point $ \left(0, C\right) $.
Then, there is a subset on the parameter space for which $ W_{+}^{u}\left(P_{1}\right) $ intersects with $ W_{+}^{s}\left(P_{1}\right) $ and a homoclinic curve is obtained. In this case, the same point $ P_{1} $ is the $ \omega -limit $ of the right unstable manifold $ W_{+}^{u}\left(P_{1}\right) $.
b) When the point $ P_{2} = \left(u_{2}, u_{2}+C\right) $ is an attractor and the $ \omega -limit $ of the right unstable manifold $ W_{+}^{u}\left(P_{1}\right) $ is the point $ \left(0, C\right) $, there exists an unstable limit cycle dividing the behavior of trajectories in the neighborhood of $ P_{2} = \left(u_{2}, u_{2}+C\right) $, which is the frontier of the basin of attraction of that point.
In Figure 2 we show the relative position of the upper stable manifold $ W_{+}^{s}\left(P_{1}\right) $ and the right unstable manifold $ W_{+}^{u}\left(P_{1}\right) $, meanwhile in Figure 3 the homoclinic curve is shown.
Remark 16. An interesting aspect happens when the homoclinic curve is broken, since a non-infinitesimal limit cycle is generated [41]. To determine the nature (stability) of this non-infinitesimal limit cycle, we will consider $ R $, the absolute value of the ratio between the negative and positive eigenvalues of the Jacobian matrix evaluated in the saddle point $ P_{1} = \left(u_{1}, u_{1}+C\right) $, denoted by $ \lambda ^{-} $ and $ \lambda ^{+} $, respectively, i.e., $ R = \left\vert \frac{\lambda ^{-}}{\lambda ^{+}}\right\vert $.
Considering a little modification of the criterion described in [26] it has that if $ R > 1 $, the non-infinitersimal limit cycle generated by homoclinic bifurcation is stable (orbitally); if $ R < 1 $, this limit cycle is unstable and if $ R = 1 $ the limit cycle is neutrally stable [26]. So, $ R $ depends on the sign of the difference $ \nabla = \left\vert \lambda ^{-}\right\vert -\lambda ^{+} $.
We know that
$ {\rm{ det}} DY_{\eta }\left( u_{1},u_{1}+C\right) = Su_{1}\left( C+u_{1}\right) ^{2}\left( A+u\right) \left( 2u_{1}-\left( 1-A-Q\right) \right) \; {\rm{ and}} $ |
$ {\rm{tr}} DY_{\eta }\left( u_{1},u_{1}+C\right) = \left( C+u_{1}\right) \left( u_{1}\left( 1-2u_{1}-A\right) -S\left( A+u_{1}\right) \right) $ |
Then, the eigenvalues of the Jacobian matrix evaluated on $ P_{1} $ are:
$ \lambda ^{-} = \frac{1}{2}\left( C+u_{1}\right) \left( \text{tr} DY_{\eta }\left( u_{1},u_{1}+C\right) -\sqrt{\Delta \left( \rho \right) } \right) $ |
$ \lambda ^{+} = \frac{1}{2}\left( C+u_{1}\right) \left( \text{tr} DY_{\eta }\left( u_{1},u_{1}+C\right) +\sqrt{\Delta \left( \rho \right) } \right) $ |
with $ \lambda ^{-} < 0 < \lambda ^{+} $ and
$\Delta \left( \rho \right) = \left( \text{tr}DY_{\eta }\left( u_{1},u_{1}+C\right) \right) ^{2}-4 $det$ DY_{\eta }\left( u_{1},u_{1}+C\right) $ |
We have the following:
Theorem 17. (Stability of the non-infinitesimal limit cycle)
The non-infinitesimal limit cycle is:
a) stable, if and only if, $ T\left(u_{1}, A.S\right) > 0 $, i.e., $ S < \frac{ u_{1}\left(1-2u_{1}-A\right) }{A+u_{1}} $.
b) unstable, if and only if, $ T\left(u_{1}, A.S\right) < 0 $, i.e., $ S > \frac{ u_{1}\left(1-2u_{1}-A\right) }{A+u_{1}} $.
c) neutrally stable, if and only if, $ T\left(u_{1}, A.S\right) = 0 $, i.e., $ S = \frac{u_{1}\left(1-2u_{1}-A\right) }{A+u_{1}} $.
Proof. Clearly, $ \nabla = $ $ \left\vert \lambda ^{-}\right\vert -\lambda ^{+} = $ $ \left(C+u_{1}\right) $tr$ DY_{\eta }\left(u_{1}, u_{1}+C\right) = 0 $.
By remark the sign of tr$ DY_{\eta }\left(u_{1}, u_{1}+C\right) $ depends on the sign of the factor
$ T\left( u_{1},A.S,\right) = u_{1}\left( 1-2u_{1}-A\right) -S\left( A+u_{1}\right) . $ |
Considering $ R = 1 $, we have $ S = \frac{u_{1}\left(1-2u_{1}-A\right) }{A+u_{1}} $ and the non-infinitesimal limit cycle is neutrally stable.
Then, the other possibles cases a) and b) are obtained with $ R > 1 $ and $ R < 1 $, respectively.
Remark 18. 1. The breaking of the homoclinic curve determined by the intersection of the upper stable manifold and the unstable right manifold of the saddle point $ P_{1} = \left(u_{1}, u_{1}+C\right) $, i.e., $ W_{+}^{s}\left(P_{1}\right) \cap W_{+}^{u}\left(P_{1}\right) $, generates a non-infinitesimal limit cycle (originating a homoclinic bifurcation), which could coincide with other limit cycle obtained via Hopf bifurcation (infinitesimal limit cycle), when $ P_{2} = \left(u_{2}, u_{2}+C\right) $ is a center-focus.
2. The non-infinitesimal limit cycle increases until concide with $ W_{+}^{s}\left(P_{1}\right) $; then is broken, and the point $ P_{2} $ becomes to a repeller focus or node; so, the point $ \left(0, C\right) $ an almost global attractor [45,43].
In the next Theorem we determine the weakness of the focus $ P_{2} = \left(u_{2}, u_{2}+C\right) $, i. e., the number of the limit cycles bifurcating of a weak (fine) focus [32,33]; for this we will use the calculations of the Lyapunov numbers (or quantities) [32,44].
Theorem 19. (Order of the weak focus)
The singularity $ P_{2} = \left(u_{2}, u_{2}+C\right) $ of vector field $ Y_{\eta } $ is at least a two order weak focus, if and only if, $ S = \frac{ L\left(1-2L-A\right) }{A+L} $, with $ u_{2} = L = \frac{1}{2}\left(1-A-Q+\sqrt{ \Delta \left(\eta \right) }\right) $ and $ \eta _{2} $ can change of sign.
Proof. As $ S = \frac{L\left(1-2L-A\right) }{A+L} $ and $ Q = \frac{1}{L+C}\left(1-L\right) \left(L+A\right) $, system $ \left(2.2\right) $ can be expressed by
$ Zν(u,v):{dudτ=( (1−u)(u+A) −(1−L)(L+A)L+Cv) u(u+C)dvdτ=L(1−2L−A)A+L(u +C−v )(u+A) v $
|
where $ \nu = \left(A, L, C\right) \in \left(\left] 0, 1\right[ \right) ^{2}\times \mathbb{R} ^{+} $.
Setting $ u = U+L $ and $ v = V+C+L $, then the new system translated to origin of coordinates system is
$ Zν(U,V):{dUdτ=((1−U−L)(U+L+A)−(1−L)(L+A)L+C(V+C+L))(U+L)(U+L+C)dVdτ=L(1−2L−A)A+L(U−V)(U+L+A)(V+C+L) $
|
The Jacobian matrix of the vector field $ Z_{\nu } $ at the point $ \left(0, 0\right) $ is
$ DZ_{\nu }(0, 0) = \left(L(1−2L−A)(C+L)−(1−L)(A+L)LL(1−2L−A)(C+L)−L(1−2L−A)(C+L)
the same that $ DY_{\eta }\left(L, L+C\right) $. Denoting
$ W^{2} = $ det$ DZ_{\nu }(0,0) = L^{2}\left( C+L\right) \left( 1-2L-A\right) \left( A-C\left( 1-2L-A\right) +L^{2}\right) , $ |
it has
$ L\left( C+L\right) \left( 1-2L-A\right) = \frac{W^{2}}{L\left( A-C+AC+2CL+L^{2}\right) } ; $ |
the first Lyapunov quantity [32] is
$ \eta _{1} = $ tr$ DY_{\eta }\left( L,L+C\right) = {\rm{tr}} DZ_{\nu }(0,0) = \alpha = 0 . $ |
The Jordan matrix associated [3] to vector field $ Z_{\nu } $ is
$
J = \left( 0−WW0
\right) .
$
|
Then, the matrix change of basis [3] is given by
$
M = \left( Z11−α−βZ210
\right) = \left( L(1−2L−A)(C+L)−WL(1−2L−A)(C+L)0
\right) .
$
|
Now consider the change of variables given by
$
\left( UV
\right) = \left( L(1−2L−A)(C+L)−WL(1−2L−A)(C+L)0
\right) \left( xy
\right)
$
|
that is,
$ U = L\left( 1-2L-A\right) \left( C+L\right) x-Wy $ |
$ V = L\left( 1-2L-A\right) \left( C+L\right) x $ |
or
$ x = $ $ \frac{1}{L\left( 1-2L-A\right) \left( C+L\right) }V $ |
$ y = \frac{-U+V}{W}. $ |
Then the new system is
$
\tilde{Z}_{\nu }:\left\{ dxdτ=1L(1−2L−A)(C+L)dVdτdydτ=−1WdUdτ+1WdVdτ
\right.
$
|
After a large algebraic calculations and by means of a time rescaling given by $ \gamma = W\tau $, we obtain the normal form $ \tilde{Z}_{\nu } $ [32] to vector field $ Z_{\nu } $ given by
$
\begin{array}{lcl} \begin{array}{l} \frac{dx}{d\gamma } \\ \\ \end{array} & = & −y−H(A+C+2L)A+Lxy+WA+Ly2−H2(C+L)A+Lx2y+WHA+Lxy2 \\ \frac{dy}{d\gamma } & = & x+H2(C+L)(AC2+7CL2+3C2L+AC+2AL−2CL−C2+3L3+2ACL)W2x2−b11xy+A2C+6AL2+2A2L+CL2−AC−2AL−L2+3L3+3ACL(A+L)y2+H3(C+L)2(A−C+AC+5CL+C2+3L2)W2x3−b21x2y+b12xy2−W(A+C+4L−1)y3+H4(C+L)4W2x4−4H3(C+L)3Wx3y+6H2(C+L)2x2y2−4WH(C+L)xy3+W2y4
\end{array}
$
|
with
$ H = L\left( 1-2L-A\right) $ |
$ b_{11} = \frac{H\left( 4L^{4}+\left( 6A+9C\right) L^{3}+\left( 4C^{2}+\left( 15A-2\right) C+A\left( A+1\right) \right) L^{2}+\left( \left( 7A-1\right) C^{2}+ A\left( 4A-3\right) C+2A^{2}\right) L+AC\left( A-2C+2AC\right) \right) }{W\left( A+L\right) } , $ |
$ b_{21} = \frac{H^{2}\left( C+L\right) \left( 3AC^{2}+3A^{2}C+10AL^{2}+A^{2}L+13CL^{2}+3C^{2}L-3AC+AL-2CL+2A^{2}+8L^{3}+17ACL\right) }{W\left( A+L\right) } $ |
$ b_{12} = \frac{H\left( 3AC^{2}+3A^{2}C+12AL^{2}+2A^{2}L+13CL^{2}+3C^{2}L-3AC-AL-2CL+A^{2}-L^{2}+9L^{3}+17ACL\right) }{A+L} $ |
Using the Mathematica package [46] for the symbolic calculus, we obtain that the second Lyapunov quantity [32] is given by
$ \eta _{2} = \frac{L^{2}H^{2}\left( C+L\right) ^{2}}{8\left( A+L\right) ^{2}W^{3}}f_{1}\left( A,C,L\right) $ |
with $ f_{1}\left(A, C, L\right) = f_{10}\left(A, L\right) +Cf_{11}\left(A, L\right) +C^{2}f_{12}\left(A, L\right) +C^{3}f_{13}\left(A, L\right) $
where
$ f_{10}\left( A,L\right) = -8L^{7}+ 4\left( 1-2A\right) L^{6}-A\left( A+11\right) L^{5}+ A\left( A^{2}-8A+5\right) L^{4}+\\ A^{2}\left( 7A-34\right) L^{3}+A^{2}\left( 7A^{2}+4\right) L^{2}+A^{3}\left( A^{2}-10A+3\right) L+A^{4}\left( 1-A\right). $ |
$ f_{11}\left( A,L\right) = -36L^{6}+16\left( 2-3A\right) L^{5}-\left( 13A^{2}+15A+6\right) L^{4}+A\left( 11A^{2}-64A+37\right) L^{3}\\+A\left( 7A^{3}-46A^{2}+39A-8\right) L^{2}+A^{2}\left( 1-A\right) \left( 11A-A^{2}-4\right) L+\left( A-A^{2}-2\right) A^{3} . $ |
$ f_{12}\left( A,L\right) = -50L^{5}+22\left( 3-4A\right) L^{4}+\left( 75A-61A^{2}-28\right) L^{3}+ \left( 33A^{2}-19A^{3}-12A+4\right) L^{2}+\\ A\left( 1-A\right) \left( -4A+2A^{2}-1\right) L+A^{2}\left( 1-A\right) . $ |
$ f_{13}\left( A,L\right) = \left( -16L^{3}+24\left( 1-A\right) L^{2}-12\left( 1-A\right) ^{2}L+ 2\left( 1-A\right) ^{3}\right) L. $ |
Because to the difficulty in deciding whether a change of sign occurs in the factor $ f_{1}\left(A, C, L\right) $, a numerical evaluation will be made for the factor $ f_{10}\left(A, L\right) $, considering it is most influential when $ C $ tends to $ 0 $.
Choosing $ A = 0.1 $ we have
$ f_{10}\left( 0.1,L\right) = -\frac{1}{100\,000}f_{101}\left( L\right) $ |
with
$ f_{101}\left( L\right) = -201.0L-4070.0L^{2}+33300.0L^{3}-42100.0L^{4}+1.\, 11\times 10^{5}L^{5}-3.\, 2\times 10^{5}L^{6}+8.0\times\\ 10^{5}L^{7}-9.0 $ |
and choosing $ L = 0.175 $ it has,
$ f_{101}\left( L\right) = $ $ -16.\, 788 , $ |
then,
$ f_{10}\left( 0.1,0.175\right) = -\frac{1}{100\,000}\left( -39.\, 84\right) \gt 0 . $ |
Analogously, choosing $ A = 0.1 $ and $ L = 0.2 $, we have
$ f_{10}\left( 0.1,0.2\right) = -\frac{1}{100\,000} f_{101}\left( 0.2\right) = -\frac{1}{100\,000}\left( 12.\, 32\right) \lt 0 . $ |
So, $ \eta _{2}\ $change the sign depending of the above relations, existing at least, two limit cycles when $ \eta _{2} = 0 $.
Then, the sign of $ \eta _{3} $ must be obtained to prove the existence of exactly two limit cycles.
Remark 20. 1. As we have seen, the computation of weakness of the focus $ P_{2} = \left(L, L+C\right) $ requires the fulfilment of two strict relationships between the parameters of the model; a little deviation in one of them causes the condition for the existence of one or more limit cycles. So, these relations determine a subset of measure non-zero for the existence of two limit cycles.
2. This is ecologically important, inasmuch as in reality none of the equalities given in the above Theorem will be possible to maintain for a long time; thus, any tiny change in some of the involved parameters will imply inequality rather than equality; hence, we have a structurally unstable system.
3. We note that the proof of the above theorem does not depend on the sign of $ a_{0} = CQ-A $.
Case 1.2 By considering $ \Delta \left(\eta \right) = \left(1-A-Q\right) ^{2}-4\left(CQ-A\right) = 0 $, the points $ P_{1} = \left(u_{1}, u_{1}+C\right) $ and $ P_{2} = \left(u_{2}, u_{2}+C\right) $ are coincident.
So, $ u_{1} = u_{2} = E = \frac{1-A-Q}{2} $, if and only if, $ C = \frac{\left(1-A-Q\right) ^{2}+4A}{4Q} $. The point $ \left(u_{2}, u_{2}+C\right) $ lies in the first quadrant, if and only if, $ 1 > A+Q $.
Theorem 21. (Collapse of the positive equilibria)
The equilibrium point $ \left(E, E+C\right) $ with $ E = \frac{1-A-Q}{2} $, is:
i) a saddle-node attractor, if and only if, $ \ S > \frac{Q\left( 1-A-Q\right) }{A-Q+1} $,
ii) a saddle-node repeller, if and only if, $ \ S < \frac{Q\left( 1-A-Q\right) }{A-Q+1} $,
iii) a cusp point, if and only if, $ \ S = \frac{Q\left( 1-A-Q\right) }{A-Q+1} $.
Proof. The Jacobian matrix is
$
DY_{\eta }\left( E,E+C\right) = \left( E(E+C)(1−2E−A)−QE(E+C)S(E+A)(E+C)−S(E+A)(E+C)
\right) .
$
|
Then, det$ DY_{\eta }\left(E, E+C\right) = $ $ 0 $, and
$ {\rm{tr}} DY_{\eta }\left( E,E+C\right) = \left( C+E\right) \left( \left( -A-E\right) S+\left( E-AE-2E^{2}\right) \right) $ |
which depends on the sign of factor
$ T_{1}\left( A,Q,S\right) = -\left( \left( A-Q+1\right) S+Q\left( A+Q-1\right) \right) $ |
Then, the point $ \left(E, E+C\right) $ is
ⅰ) a saddle-node attractor, if and only if, $ S > \frac{Q\left(1-A-Q\right) }{A-Q+1} $,
ⅱ) a saddle-node repeller, if and only if, $ S < \frac{Q\left(1-A-Q\right) }{A-Q+1} $,
c) If $ S = \frac{Q\left(1-A-Q\right) }{A-Q+1} $, the Jacobian matrix is
$
DY_{\eta }\left( E,E+C\right) = \frac{1}{4}Q\left( A+Q-1\right) \left( A-2C+Q-1\right) \left( 1−11−1
\right) ,
$
|
whose Jordan form matrix is $ J = \left(0100
In this case, the point $ \left(0, C\right) $ is an attractor almost globally asymptotically stable [45,43], since an unique trajectory exists in the phase plane having the point $ \left(E, E+C\right) $ as its $ \omega -limit $.
Case 1.3 If $ B_{1} = 1-A-Q > 0 $ and $ B_{2} = CQ-A > 0 $ and $ \Delta \left(\eta \right) < 0 $, there were not exist positive equilibrium points.
Theorem 22. (Non-existence of positive equilibria)
When there exists no positive equilibria, the point $ \left(0, C\right) $ is globally asymptotically stable.
Proof. By lemma $ 5, $ we know the solutions are bounded; by lemma 3, $ \bar{\Gamma} $ is invariant region. As it was stated before, the equilibrium $ \left(1, 0\right) $ is a saddle point, then the Poincaré-Bendixon Theorem applies and the unique $ \omega -limit $ of the trajectories is the point $ \left(0, C\right) $.
In order to reinforce the obtained results, we show some numerical simulations (Figures 4–9), considering only the case 1, i.e., $ B_{1} = 1+A-Q > 0 $, $ B_{0} = CQ-A > 0 $ and $ \Delta > 0 $, being in this case the point $ \left(0, C\right) $ always attractor (local or global).
In the following picture (Figure 10) the bifurcation diagram of system $ \left(2.2\right) $ is shown.
Considering $ A $ and $ Q $ fixed it was created with the numerical bifurcation package MaTCont [40] showing that the bifurcation curves divide the $ \left(C, S\right) $-parameter space into five parts. Modifying the parameter $ C $ impacts the number of positive equilibrium points of system $ \left(2.2\right) $.
The modification of the parameter $ S $ changes the stability of the positive equilibrium point $ P_{2} $ of system $ \left(2.2\right) $, while the other equilibrium points do not change their behaviour.
There are no positive equilibrium points in system $ \left(2.2\right) $ when the parameters $ C $ and $ S $ are located in the red area where $ \Delta < 0 $. In this case, the origin is a global attractor.
For $ C = C^{\ast } $, which is the saddle-node curve $ SN $, the equilibrium points $ P_{1} $ and $ P_{2} $ collapse since $ \Delta = 0 $. So that, system $ \left(2.2\right) $ experiences a Bogdanov-Takens bifurcation. When the parameters $ C $ and $ S $ are located in the green, grey or blue area, system $ \left(2.2\right) $ has two equilibrium points $ P_{1} $ and $ P_{2} $.
The equilibrium point $ P_{1} $ is always a saddle point, while $ P_{2} $ can be unstable (grey area) or stable (blue area). For $ C $ and $ S $ in the green area the stable equilibrium point $ P_{2} $ can be surrounded by an unstable limit cycle (green Region IV(1)) or surrounded by two limit cycle (green Region IV(2)).
In this work, a modified May-Holling-Tanner predator-prey model was studied, particularly a modified Leslie-Gower model [13,25,5], considering that predators can eat other prey in the case of severe scarcity of its most favorite food. This situation was taken into account by adding a positive constant $ c $ in the function $ K_{y} $ representing the environmental carrying capacity for predators. This implies the existence of a new equilibrium point $ \left(0, c\right) $ in the $ y-axis $.
By means of a diffeomorphism, we analyzed a topologically equivalent system depending only on four parameters. It was shown that the model has a rich dynamic since this model can exhibit various kinds of bifurcations (e.g. saddle-node, Hopf-Andronov, Bogdanov-Takens, homoclinic, Hopf multiple bifurcations) as likewise infinitesimal and non-infinitesimal limit cycles, generated by Hopf and homoclinic bifurcation, respectively.
Conditions for the existence of equilibrium points and their nature were established. We proved that the equilibrium point $ \left(0, 0\right) $ is always a repeller for all parameter values, which means that there is no extinction of both populations simultaneously; moreover, $ \left(1, 0\right) $ is a saddle point, implying that the predator population can go to depletion, meanwhile the prey attains its maximum population size in the common environmental.
Also, a wide subset of the parameter values was determined, for which there exist two positive equilibrium points $ P_{1} = \left(u_{1}, u_{1}+C\right) $ and $ P_{2} = \left(u_{2}, u_{2}+C\right) $, being the first of them always a saddle point. The other equilibrium can be an attractor, a repeller or a weak focus, depending on the sign of the trace of its Jacobian matrix. Furthermore, both equilibrium points can collapse, obtaining a cusp point, i.e., Bogdanov-Takens bifurcation or codimension 2 bifurcation [47].
When two equilibrium points exist at the interior of the first quadrant in the system $ \left(2.2\right) $, the singularity $ (0, C) $ is an attractor and the stable manifold $ W^{s}\left(P_{1}\right) $ of $ P_{1} $ determines a separatrix curve which divides the phase plane into two regions. The trajectories having initial conditions above this curve have the point $ \left(0, C\right) $ as their $ \omega -limit $, meanwhile, those that lie below the separatrix can have a positive equilibrium point or a stable limit cycle as their $ \omega -limit $. This implies that there exists a great possibility for the prey population to go to extinction, although the ratio prey-predator is high (many prey and little predators).
We also prove the existence of a homoclinic curve determined by the stable and unstable manifolds of the positive saddle point $ P_{1} $, encircling the second positive equilibrium point $ P_{2} $; when it breaks up it originates a non-infinitesimal limit cycle.
The dynamics of the studied model, in which the predators have an alternative food to low densities of prey, differs from the May-Holling-Tanner model [3,5], since:
ⅰ) System $ \left(2.1\right) $ can have one, two or none positive equilibrium points at the interior of the first quadrant with a more varied dynamic; whereas, the May-Holling-Tanner model has a unique positive equilibrium point, which can never be a cusp point, then there no exists Bogdanov-Takens bifurcation for this model.
ⅱ) In system $ \left(2.1\right) $ there is a parameter constraint for which a homoclinic curve exists, something that does not appear in the May-Holling-Tanner model.
ⅲ) Each model has a separatrix curve dividing the behavior of the trajectories, which are originated by, the non-hyperbolic saddle point $ \left(0, 0\right) $, in the May-Holling-Tanner model, and in the modified model is created by the hyperbolic attractor point $ \left(0, C\right) $.
ⅳ) Both models have in common the existence of triple or bi-stability since two limit cycles can bifurcate of a weak focus, surrounding an attractor positive equilibrium point, being the innermost unstable (frontier of the attraction basin) and the outermost stable.
But, in the model here analyzed, this situation also can appear when exist two positive equilibria (see Figure 7) or when exists a unique equilibrium at the interior of the first quadrant (For instance, when $ A = 0.2 $, $ C = 0.4 $, $ Q = 0.5 $ and $ S = 0.12005 $, i.e., $ CQ-A = 0 $, when $ A = 0.2000001 $, $ C = 0.39999925 $, $ Q = 0.5 $ and $ S = 0.12005 $, i.e., $ CQ-A < 0 $). In the Holling-Tanner model, the two limit cycles appear when there is one positive equilibrium point.
The triple-stability phenomenon exists in the system $ \left(2.2 \right) $ when simultaneously are stable: (1) the point $ \left(0, C\right) $; (2) the positive equilibrium $ P_{2} $; and (3) a limit cycle, for a determined set of parameters; then, for these parameter values, both populations can coexist, oscillate around specific population sizes or prey population can be depleted and the predators survive as an alternative food.
It can conclude that for certain parameters values in the system $ \left(2.2\right) $, there exists self-regulation since the species can coexist experimenting oscillations of their population sizes surrounded a fix point, or else, the population sizes can tend to that fix point. But, depending on the ratio prey/predator the prey population can go to extinction for the same parameters values.
Moreover, system $ \left(2.2\right) $ is sensitive to disturbances of the parameter values, since there exist changes of the basin of attraction of $ P_{2} $ as it is shown in Figures 4–8.
The self-regulation depends mainly on the parameter $ S = $ $ \frac{s}{r} $. This implies that increasing the intrinsic predator growth rate $ s $ or decreasing the intrinsic prey growth rate $ r $, the possibility of oscillations of the population sizes increase. Similar statements can be derived for other parameters values of the system $ \left(2.2\right) $.
The complex dynamic of the analyzed model is a prominent issue to be considered by the ecologists and agencies responsible for conservation and management of renewable resources, as the open access fisheries.
This concern must be especially with those populations more sensitive to disturbances of the environment, considering that for given initial condition the dynamic of the model predicts the long term persistence of the populations, or else, the extinction of one of them.
On the other hand, the system $ \left(2.1\right) $ could have a behavior nearest to the model studied in [22], where $ c = 0 $, or with the model analyzed in [37], since the Allee effect implies a closer dynamic, due to the existence of two positive equilibria.
In short, in this article, we extend the dynamical properties of the model proposed in [13] and the partial results obtained in previous papers [23,24,1,29,2]; we also complement the outcomes obtained in [5] for the May-Holling-Tanner model, showing that the modified model has interesting and rich mathematical dynamics, describing different possible ecological behaviors.
This work was partially financed by DIEA PUCV 124.720/2012.
All authors declare no conflicts of interest in this paper.
[1] |
The dissemination of culture: A model with local convergence and global polarization. The Journal of Conflict Resolution (1997) 41: 203-226. ![]() |
[2] |
P. Barron, K. Kaiser and M. Pradhan, Local Conflict in Indonesia: Measuring Incidence and Identifying Patterns, World Bank Policy Research Paper 3384, 2004. |
[3] |
Acculturation and adaptation in a new society. International Migration Quarterly Review (1992) 30: 69-85. ![]() |
[4] | Living successfully in two cultures. International Journal of Intercultural Relations (2005) 29: 697-712. |
[5] | Comparative studies of acculturative stress. The International Migration Review (1987) 21: 491-511. |
[6] |
P. Boyle, K. Halfacree and V. Robinson, Exploring contemporary migration, 2nd edition, Pearson Education Limited, London and New York, 2013. |
[7] | (2003) The Age of Migration: International Population Movements in the Modern World. New York: The Guilford Press. |
[8] |
Foreword: The world economy and contemporary migration. The International Migration Review (1979) 13: 204-212. ![]() |
[9] |
Y.-S. Chiang, Cooperation could evolve in complex networks when activated conditionally on network characteristics, Journal of Artificial Societies and Social Simulation, 16 (2013), 6. |
[10] |
A bistable belief dynamics model for radicalization within sectarian conflict. Quarterly of Applied Mathematics (2017) 75: 19-37. ![]() |
[11] |
The role of social structure in the maintenance of cooperative regimes. Rationality and Society (2001) 13: 5-32. ![]() |
[12] |
M. H. Crawford and B. C. Campbell (eds.), Causes and Consequences of Human Migration, Cambridge University Press, Cambridge, UK, 2012. |
[13] | Determinants of recent immigrants'location choices: Quasi-experimental evidence. Journal of Population Economics (2009) 22: 145-174. |
[14] |
Mixing beliefs among interacting agents. Advances in Complex Systems (2000) 3: 87-98. ![]() |
[15] | Reaching a consensus. Journal of the American Statistical Association (1974) 69: 118-121. |
[16] |
Co-evolution of behaviour and social network structure promotes human cooperation. Ecology Letters (2011) 14: 546-551. ![]() |
[17] |
K. Felijakowski and R. Kosinski, Bounded confidence model in complex networks, International Journal of Modern Physics C, 24 (2013), 1350049, 12pp. |
[18] |
Opinion formation and self-organization in a social network in an intelligent agent system. ACTA Physica Polonica B (2014) 45: 2123-2134. ![]() |
[19] |
Ethnic preferences, social distance dynamics, and residential segregation: Theoretical explorations using simulation analysis. The Journal of Mathematical Sociology (2006) 30: 185-273. ![]() |
[20] | Choice shift and group polarization. American Sociological Review (1999) 64: 856-875. |
[21] |
A. Gabel, P. L. Krapivsky and S. Redner, Highly dispersed networks by enhanced redirection, Physical Review E, 88 (2013), 050802(R). |
[22] |
S. Galam, Heterogeneous beliefs, segregation, and extremism in the making of public opinions, Physical Review E, 71 (2005), 046123. |
[23] |
Stubbornness as an unfortunate key to win a public debate: An illustration from sociophysics. Society (2016) 15: 117-130. ![]() |
[24] |
S. Galam and M. A. Javarone, Modeling radicalization phenomena in heterogeneous populations, PLOS One, 11 (2016), e0155407. |
[25] |
Naíve learning in social networks and the wisdom of crowds. American Economic Journal: Microeconomics (2010) 2: 112-149. ![]() |
[26] |
D. Hales, Cooperation without memory or space: Tags, groups and the Prisoner's Dilemma, in Multi-Agent-Based Simulation. (eds. S. Moss and P. Davidsson), Springer, Berlin/Heidelberg, 2000, 157–166. |
[27] |
The evolution of ethnocentrism. Journal of Conflict Resolution (2006) 50: 926-936. ![]() |
[28] |
A dynamical systems model of unorganized segregation. The Journal of Mathematical Sociology (2018) 42: 113-127. ![]() |
[29] |
Emergence of segregation in evolving social networks. PNAS (2011) 108: 8605-8610. ![]() |
[30] | (2004) Becoming Europe: Immigration Integration And The Welfare State. Pittsburgh, PA: University of Pittsburgh Press. |
[31] |
M. A. Javarone, A. E. Atzeni and S. Galam, Emergence of cooperation in the Prisoner's Dilemma driven by conformity, in Applications of Evolutionary Computation. EvoApplications 2015. Lecture Notes in Computer Science (eds. A. Mora and G. Squillero), vol. 9028, Springer, Cham, 2015, 155–163. |
[32] |
W. Kandel and J. Cromartie, New patterns of Hispanic settlement in rural America, Technical Report 99, United States Department of Agriculture, 2004. |
[33] |
T. B. Klos, Decentralized interaction and co-adaptation in the repeated prisoners dilemma, Computational and Mathematical Organization Theory, 5 (1999), 147165. |
[34] |
Trade-offs between equality and difference: Immigrant integration, multiculturalism and the welfare state in cross-national perspective. Journal of Ethnic and Migration Studies (2010) 36: 1-26. ![]() |
[35] |
U. Krause, A discrete nonlinear and non-autonomous model of consensus formation, in Communications in Difference Equations (eds. S. Elaydi, G. Ladas, J. Popenda and J. Rakowski), Amsterdam: Gordon and Breach, 2000, 227–236. |
[36] |
Emerging patterns of Hispanic residential segregation: Lessons from rural and small–town America. Rural Sociology (2016) 81: 483-518. ![]() |
[37] |
Residential segregation in new Hispanic destinations: Cities, suburbs, and rural communities compared. Social Science Research (2010) 39: 215-230. ![]() |
[38] | The evolution of altruism in spatially structured populations. Journal of Artificial Societies and Social Simulations (2007) 10: 1-13. |
[39] |
From private attitude to public opinion: A dynamic theory of social impact. Psychological Review (1990) 97: 362-376. ![]() |
[40] |
Patterns of intergroup contact in public spaces: Micro-ecology of segregation in Australian communities. Societies (2014) 4: 30-44. ![]() |
[41] |
R. Riolo, The Effects of Tag-Mediated Selection of Partners in Evolving Populations Playing the Iterated Prisoner's Dilemma, Technical report, Santa Fe Institute, Santa Fe, NM, 1997. |
[42] |
Evolution of cooperation without reciprocity. Nature (2001) 414: 441-443. ![]() |
[43] |
F. C. Santos, J. M. Pacheco and T. Lenaerts, Cooperation prevails when individuals adjust their social ties, PLoS Computational Biology, 2 (2006), e140. |
[44] | Community segregation and the costs of ethnic subordination. Social Forces (1981) 59: 649-666. |
[45] |
UK dispersal policy and onward migration: Mapping the current state of knowledge. Journal of Refugee Studies (2012) 25: 25-49. ![]() |
[46] |
A. Szolnoki and M. Perc, Competition of tolerant strategies in the spatial public goods game, New Journal of Physics, 18 (2016), 083021. |
[47] |
UNHCR, Global trends: Forced displacement in 2017, Technical report, The UN Refugee Agency, The United Nations, 2018, http://www.unhcr.org/5b27be547.pdf. |
[48] | Heterogeneous investments promote cooperation in evolutionary public goods games. Physica A (2018) 502: 570-575. |
[49] | Collective dynamics of 'small-world' networks. Nature (1998) 393: 440-442. |
[50] |
Meet, discuss, and segregate. Complexity (2002) 7: 55-63. ![]() |
[51] |
Long-term effects of neighbourhood deprivation on diabetes risk: Quasi-experimental evidence from a refugee dispersal policy in Sweden. The Lancet Diabetes and Endocrinology (2016) 4: 517-524. ![]() |
1. | Weiyi Xu, Hu Wang, Zhenzhen Lu, Guojian Ren, Yongguang Yu, A fractional-order two-strain SVIR model with stability analysis, 2024, 91, 05779073, 674, 10.1016/j.cjph.2024.08.006 | |
2. | Kamal Shah, Thabet Abdeljawad, Bahaaeldin Abdalla, Manel Hleili, Study of a class of fractional-order evolution hybrid differential equations using a modified Mittag-Leffler-type derivative, 2024, 2024, 1687-2770, 10.1186/s13661-024-01886-8 | |
3. | Elkhateeb S. Aly, Mohammed A. Almalahi, Khaled A. Aldwoah, Kamal Shah, Criteria of existence and stability of an n-coupled system of generalized Sturm-Liouville equations with a modified ABC fractional derivative and an application to the SEIR influenza epidemic model, 2024, 9, 2473-6988, 14228, 10.3934/math.2024691 | |
4. | Yasir A. Madani, Mohammed A. Almalahi, Osman Osman, Blgys Muflh, Khaled Aldwoah, Khidir Shaib Mohamed, Nidal Eljaneid, Analysis of an Acute Diarrhea Piecewise Modified ABC Fractional Model: Optimal Control, Stability and Simulation, 2025, 9, 2504-3110, 68, 10.3390/fractalfract9020068 | |
5. | Jyoti Mishra, Modeling for the Menstrual Cycle with Non-Local Operators, 2025, 26668181, 101128, 10.1016/j.padiff.2025.101128 |
Parameters | Meanings |
$ r $ | intrinsic prey growth rate or biotic potential |
$ K $ | prey environmental carrying capacity |
$ q $ | maximum number of prey that can be eaten by a predator in each time unit |
$ a $ | amount of prey to achieve one-half of the maximum rate $ q $ |
$ s $ | intrinsic predator growth rate |
$ n $ | measure of the food quality |
$ c $ | amount of alternative food available for predators |
Case | sg$\left(B_{1}\right) $ | sg$\left(B_{0}\right) $ | sg$\left(\Delta \left(\eta \right) \right) $ | Number of Positive equilibrium |
1.1 | $+$ | $+$ | $+$ | $2$ |
1.2 | $+$ | $+$ | $0$ | $1$ |
1.3 | $+$ | $+$ | $-$ | $0$ |
2.1 | $+$ | $-$ | $+$ | $1$ |
2.2 | $-$ | $+$ | $+$ | $1$ |
3 | $+$ | $0$ | $+$ | $1$ |
4 | $0$ | $-$ | $+$ | $1$ |
5.1 | $0$ | $+$ | $-$ | $0$ |
5.2 | $-$ | $+$ | $-$ | $0$ |
Parameters | Meanings |
$ r $ | intrinsic prey growth rate or biotic potential |
$ K $ | prey environmental carrying capacity |
$ q $ | maximum number of prey that can be eaten by a predator in each time unit |
$ a $ | amount of prey to achieve one-half of the maximum rate $ q $ |
$ s $ | intrinsic predator growth rate |
$ n $ | measure of the food quality |
$ c $ | amount of alternative food available for predators |
Case | sg$\left(B_{1}\right) $ | sg$\left(B_{0}\right) $ | sg$\left(\Delta \left(\eta \right) \right) $ | Number of Positive equilibrium |
1.1 | $+$ | $+$ | $+$ | $2$ |
1.2 | $+$ | $+$ | $0$ | $1$ |
1.3 | $+$ | $+$ | $-$ | $0$ |
2.1 | $+$ | $-$ | $+$ | $1$ |
2.2 | $-$ | $+$ | $+$ | $1$ |
3 | $+$ | $0$ | $+$ | $1$ |
4 | $0$ | $-$ | $+$ | $1$ |
5.1 | $0$ | $+$ | $-$ | $0$ |
5.2 | $-$ | $+$ | $-$ | $0$ |
Simulated network dynamics leading to (a) complete segregation, and (b) integration between guest (red) and host (blue) populations. Shading of node colors represents the degree of hostility
Model diagram. Each node
Dynamics of the average utility per node
Dynamics of the integration index
Dynamics of the integration index
Dynamics of the integration index
Integration index at steady state. In panel (a)
Time
Integration index at steady state.