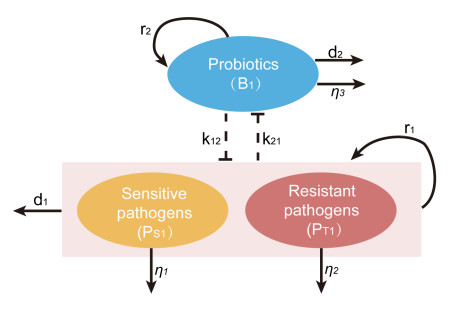
Citation: Avner Friedman, Mirosław Lachowicz, Urszula Ledzewicz, Monika Joanna Piotrowska, Zuzanna Szymanska. Preface[J]. Mathematical Biosciences and Engineering, 2017, 14(1): i-i. doi: 10.3934/mbe.201701i
[1] | Robert E. Beardmore, Rafael Peña-Miller . Rotating antibiotics selects optimally against antibiotic resistance, in theory. Mathematical Biosciences and Engineering, 2010, 7(3): 527-552. doi: 10.3934/mbe.2010.7.527 |
[2] | Jing Jia, Yanfeng Zhao, Zhong Zhao, Bing Liu, Xinyu Song, Yuanxian Hui . Dynamics of a within-host drug resistance model with impulsive state feedback control. Mathematical Biosciences and Engineering, 2023, 20(2): 2219-2231. doi: 10.3934/mbe.2023103 |
[3] | Robert E. Beardmore, Rafael Peña-Miller . Antibiotic cycling versus mixing: The difficulty of using mathematical models to definitively quantify their relative merits. Mathematical Biosciences and Engineering, 2010, 7(4): 923-933. doi: 10.3934/mbe.2010.7.923 |
[4] | Xiaodan Sun, Yanni Xiao, Zhihang Peng . Modelling HIV superinfection among men who have sex with men. Mathematical Biosciences and Engineering, 2016, 13(1): 171-191. doi: 10.3934/mbe.2016.13.171 |
[5] | Timothy C. Reluga, Jan Medlock . Resistance mechanisms matter in SIR models. Mathematical Biosciences and Engineering, 2007, 4(3): 553-563. doi: 10.3934/mbe.2007.4.553 |
[6] | Avner Friedman, Najat Ziyadi, Khalid Boushaba . A model of drug resistance with infection by health care workers. Mathematical Biosciences and Engineering, 2010, 7(4): 779-792. doi: 10.3934/mbe.2010.7.779 |
[7] | Silvia Martorano Raimundo, Hyun Mo Yang, Ezio Venturino . Theoretical assessment of the relative incidences of sensitive andresistant tuberculosis epidemic in presence of drug treatment. Mathematical Biosciences and Engineering, 2014, 11(4): 971-993. doi: 10.3934/mbe.2014.11.971 |
[8] | Michele L. Joyner, Cammey C. Manning, Brandi N. Canter . Modeling the effects of introducing a new antibiotic in a hospital setting: A case study. Mathematical Biosciences and Engineering, 2012, 9(3): 601-625. doi: 10.3934/mbe.2012.9.601 |
[9] | Awatif Jahman Alqarni, Azmin Sham Rambely, Sana Abdulkream Alharbi, Ishak Hashim . Dynamic behavior and stabilization of brain cell reconstitution after stroke under the proliferation and differentiation processes for stem cells. Mathematical Biosciences and Engineering, 2021, 18(5): 6288-6304. doi: 10.3934/mbe.2021314 |
[10] | Tianqi Song, Chuncheng Wang, Boping Tian . Mathematical models for within-host competition of malaria parasites. Mathematical Biosciences and Engineering, 2019, 16(6): 6623-6653. doi: 10.3934/mbe.2019330 |
Drug-resistant infection caused by over use of antibiotic is one of the most serious health problems of the 21st century. In the United Sates in 2005, a data survey showed that the total number of deaths caused by methicillin-resistant Staphylococcus.aureus invasion exceeded that caused by HIV/AIDS in the same year. A recent estimate suggested that at least 700,000 patients worldwide die from antibiotic resistance every year. These alarming situations highlight the need to explore the formation mechanism of antibiotic resistance to control the incidence of resistance.
As a standard treatment for various diseases, antibiotics have been widely used in the clinic due to their convenience and quick action. Standard antibiotic treatment guidelines aim to maximize treatment efficacy and minimize side effects, but they do not give enough consideration to the long-term impact, such as the emergence of antibiotic resistance. In fact, an increasing-number of studies have demonstrated that antibiotic use can alter the composition of the gut microbiota [1,2,3]. Some studies have shown that the microbiota composition in patients treated with antibiotics cannot return to the pretreatment state [4,5,6]. Additionally, a growing body of literature has suggested that antibiotic-resistant pathogens are enriched in the intestinal microbiota of patients with high antibiotic usage [7]. More generally, it has been demonstrated that altering the composition of the microbiota will interfere with some of the human-microbe interactions that are integral to human physiology. However, the fundamental role of the microflora composition has not been fully explored in the development of antibiotic resistance.
The harmony of the gut microbiota ecosystem is crucial to host health. As the dominant microorganisms in the whole intestinal flora, probiotics are very important in maintaining the balance of the gut microbiota. Several studies of intestinal flora have demonstrated that beneficial bacteria play an essential role in intestinal health. For example, beneficial bacteria can inhibit the reproduction and growth of pathogens or prevent the invasion of foreign pathogens by secreting bacteriocins or activating the host's immune defense system [8,9,10]. Altogether, exploring the mechanism of antibiotic resistance must take into account the role of probiotics.
FMT (short for Fecal microbiota transplantation) as a new therapy is one of the main treatments against drug-resistant infections. FMT is a therapeutic regimen of infusion of fecal preparation from a healthy donor into a patient to cure a certain disease [11]. The gut microbiota in healthy donors is dominated by a large number of probiotics. Therefore, FMT is a feasible strategy to resist drug resistance. Recently, FMT has gradually been adopted and has achieved considerable success in curing antibiotic-resistant infections [12,13,14,15,16]. For instance, many studies have shown that FMT restored both the gut microbiota composition and function in patients who suffered from recurrent Clostridium difficile-associated diarrhea [17,18,19]. Some studies have shown that the worldwide mean cure rates of FMT for diarrhea are approximately 91% [20]. Increasing use as a first-line treatment suggests that FMT combined with antibiotic treatment can be effective in treating infections caused by "superbugs" [12,13]. Therefore, understanding the effective mechanism of FMT is another important issue.
Mathematical modeling is a powerful tool to interpret the development of antibiotic resistance. Some studies have used dynamic systems approaches to explore the growth patterns of microbial species, species-species interactions, and susceptibilities of the intestinal microbiota to antibiotics [21,22,23]. Other researchers have proposed multi-stability or the existence of strongly interacting species to explain the microbial compositional shift caused by antibiotic treatments [24,25]. However, most quantitative studies of the intestinal microbiota are limited to the classification of microorganisms into antibiotic-sensitive and antibiotic-resistant bacterial groups, and the critical effects of probiotics are absent [26,27,28,29]. Additionally, the theoretical analysis of fecal bacteria transplantation is sparse.
Unlike previous studies, we focused on the case where gene sequences of sensitive and resistant pathogens differ at only one site or a few sites in the drug resistance determinant region. This situation has been confirmed by deep sequencing technology in many cases. For example, the difference between methicillin-resistant Staphylococcusaureus and non-resistant strains is that a site mutation occurs in the -10 consensus of the promoter [30]. Another example is that there was no significant difference in growth between NDM-1-expressing E.coli (which are resistant to all β-lactam antibiotics except monobactams) and their isogenic parental strains [31]. We also considered the role of probiotics in maintaining the balance of the gut microbiota. Therefore, we modeled the combined effects of inter-specifies interaction (probiotics and pathogens) and antibiotic therapy (or FMT combined with antibiotic treatment) to explore the mechanism of development of drug resistance and the mechanism of FMT. In our model, the intestinal microbiota was divided into the following three groups: probiotics, sensitive-pathogens and resistant-pathogens. Specifically, we wanted to explore the dynamic evolution of the core gut microbiota, to provide critical information for the optimal use of antibiotics to delay or even prevent the emergence of antibiotic resistance. By mathematical modeling and numerical simulations, we demonstrated the importance of probiotics and interspecies inhibition in the development of antibiotic resistance. This work clarified that the mechanism of FMT is essentially a transfer towards the healthy states in the phase space. In addition, we also suggested some strategies (i.e., early usage of FMT) to delay or prevent the emergence of drug resistance. These findings provide novel insights for understanding drug resistance and FMT.
We used the generalized Lotka-Volterra (L-V) equation to establish a time-dependent ecological dynamic model with external disturbances (antibiotics). The human intestinal microbiota is a complex and dynamic ecosystem of crucial importance to human health. In this study, we were primarily interested in the development of antibiotic-resistance which is caused by changes in the microbiota composition state. To achieve this goal, we classified the microbiota into three groups (probiotics, antibiotic-sensitive pathogens and antibiotic-resistant pathogens) to represent the response of commensal bacteria to antibiotic treatments.
Experimental studies have shown that microbial growth and reproduction will eventually saturate due to limited resources and space [32,33]. Therefore, we introduced a logistic equation to depict the growth pattern of bacteria under limitation of resources. Some studies on microbial interactions have shown that probiotics can effectively inhibit the growth of pathogens and the formation of pathogenic spores by secreting bacteriocins or short-chain fatty acids and so on; in turn, pathogens can also secrete toxins to eliminate probiotics [9,34,35,36]. Combining these existing experimental phenomena, we developed a model in which pathogens (consisting of antibiotic-sensitive pathogens and antibiotic-resistant pathogens) and probiotics (i.e., B1) inhibited each other, as shown in Figure 1. Our system further considered the competition for resources between sensitive pathogens and resistant pathogens with population sizes of PS1 and PT1, respectively. The ecological dynamic model assumes that three populations follow a set of ordinary differential equations:
{dPS1dt=r1(1−k1PS1+k2PT1K1)PS1−k12B21B21+a′2PS1−d1PS1−η1PS1dPT1dt=r1(1−k1PS1+k2PT1K1)PT1−k12B21B21+a′2PT1−d1PT1−η2PT1dB1dt=r2(1−B1K2)B1−k21(k1PS1+k2PT1)2(k1PS1+k2PT1)2+b′2B1−d2B1−η3B1 | (2.1) |
A key assumption of our model is that sensitive pathogens and resistant pathogens have differences in one site or very few sites in the gene coding sequence, which mainly affect the bactericidal activity of antibiotics. Actually, using deep sequencing technology, this situation has been confirmed in many cases [30,31]. For instance, the resistant strains have only one site mututaion from the non-resistant Staphylococcusaureus. The genetic determinant of resistance, mecA, is a gene of methicillin−resistantStaphylococcusaureus. DNA sequence of mecA from homologous resistant strains revealed that this resistance originated from a site mutation in the -10 consensus of the promoter, that is, thymine residues of sensitive strains (i.e., T at nt 1577) were replaced by adenine of resistant strains (i.e., A at nt 1577) [30]. Additionally, Stephan Gottig, et al. used a vivo infection model to study the growth kinetics of NDM-1-expressing E.coli and their isogenic controls [31]. The results showed that there was no significant difference in growth. Therefore, we assume that the relevant parameters of these two pathogens (sensitive and resistant) are identical except for antibiotic bactericidal rates (η1 and η2) and competition coefficients (k1 and k2). In our model, this assumption is the basis of explaining the mechanism of drug resistance.
Here, ri and di are the growth rate and the mortality rate of pathogens and probiotics (i=1,2), respectively. We introduce a logistic function to characterize the growth of probiotics and two types of pathogens. The term in brackets indicates that the growth of every species is limited by the maximum population size K1 (K2). k1 and k2 denote the inter-specific competition intensity of the two pathogen groups because they will compete for the same nutritional resources and space. The interspecific inhibitions between probiotics and pathogens, mediated through secretion (bacteriocin or toxins), are represented by increasing Hill functions. The inhibition coefficients k12 (pathogens by probiotics) and k21 (probiotics by pathogens) indicate the maximum inhibition intensity of bacteriocin and toxins, respectively. The parameter a′ (or b′) is probiotic (or pathogen) population that gives half of the maximum inhibition coefficient k12 (or k21). The last item in the equations describes the mortality rate caused by antibiotic intake. Many studies have shown that resistant pathogens can prevent the entry of antibiotics different ways, as follows: altering cell membrane permeability, changing protein structure in the body to inhibit binding, or secreting certain hydrolytic enzymes to break down antibiotics [37,38,39]. Therefore, we assumed that antibiotics have different effects in different species, i.e., the drug bactericidal rate ηj of species j ( = 1, 2 or 3) is different for different species (or ηj is the antibiotic-related death rate).
It is convenient to scale the variables and set the maximum capacity to unity (K1=K2=1). By introducing PS=PS1/K1;PT=PT1/K1;B=B1/K2; a=a′K2; and b=b′K1, we simplified the Eq (2.1) to obtain the following model:
{dPSdt=r1[1−(k1PS+k2PT)]PS−k12B2B2+a2PS−d1PS−η1PSdPTdt=r1[1−(k1PS+k2PT)]PT−k12B2B2+a2PT−d1PS−η2PTdBdt=r2(1−B)B−k21(k1PS+k2PT)2(k1PS+k2PT)2+b2B−d2B−η3B | (2.2) |
Different growth rates or mortality rates are used to characterize the different physiological stages. In the healthy stage, we set the drug bactericidal rate ηj as zeros in Eq (2.2), because there is no antibiotic involved. In the infection stage, increased r1 was used to describe the accumulation of the pathogens. For the antibiotic treatment stage, we utilized nonzero drug bactericidal rates, ηj (j=1, 2 or 3), to present the effects of antibiotics. For the FMT treatment stage, we introduced the survival rate coefficient k(0<k<1) to characterize the effect of fecal microbiota transplantation. For example, the microbiota composition state before FMT and that of donors are (PS,PT,B) and (PSh,PTh,Bh), respectively, and the instantaneous composition state after FMT becomes (PS+k∗PSh,PT+k∗PTh,B+k∗Bh). In the recovery stage, the same parameters as in the healthy stage were used. Table 1 summarizes the values of all the parameters used in the Eq (2.2) and the corresponding parameter values for different stages.
Symbols | Definition | Value (day−1) |
r1 | Growth rate of pathogens | 0.664 (without infection) |
0.664∗1.32 (with infection) | ||
r2 | Growth rate of probiotics | 0.718 |
d1 | Death rate of pathogens | 0.0501 |
d2 | Death rate of probiotics | 0.0751 |
k12 | Maximum inhibition coefficient of probiotics on pathogens | 0.76 |
k21 | Maximum inhibition coefficient of pathogens on probiotics | 0.84 |
k1 | Competitive coefficient of PT against PS | 0.5 |
k2 | Competitive coefficient of PS against PT | 0.5 |
a | Probiotics amount that yields 50% of k12 | 0.58 |
b | Pathogens amount that yields 50% of k21 | 0.58 |
η1 | Killing rate of antibiotic for PS | 0.086 (with antibiotic) |
η2 | Killing rate of antibiotic for PT | 0.0016 (with antibiotic) |
η3 | Killing rate of antibiotic for probiotics | 0.015 (with antibiotic) |
k | Survival rate of fecal microbiota | 0.5 |
We first characterized the host's complete physiological process of illness (red curve ①), drug treatment (sky blue curve ②), FMT (green curve ③) and recovery (blue curve ④) through time evolution curves of the total amount of pathogens (sensitive pathogens and resistant pathogens) (Figure 2A). Some studies have shown that each healthy individual harbors a stable gut microbiota [40]. Therefore, in our model, before the illness stage, the microbiota composition state was set in a healthy stable state, i.e., the amount of pathogens remained in a low level (-7 days to 0 days in Figure 2A). To depict the illness process, we used the overgrowth of pathogens, i.e., parameter r1 became 1.32∗r1. In the illness stage, the pathogen populations continued to increase. Then, antibiotic treatment started on the fourth day after the infection and lasted for 3 days. We defined a course of continuous-medication for 3 days as a standard treatment, because many clinical trials have confirmed that most common infectious diseases can be cured with 3 days of drug therapy. Here, we assumed that the antibiotic effects (non-zero ηj) were added to the system to eliminate pathogens in this stage. In the meantime, the reproductive rate of pathogens was also effectively suppressed and returned to the healthy level (r1). After drug treatment, the total amount of pathogens was significantly reduced to a lower level than that prior to treatment. From the 7th day, the system entered the self-repair stage and then fully recovered after a long time. In the recovery process, we set the drug bactericidal rate (ηj) to zero because the bactericidal activity of antibiotic also disappears after drug withdrawal.
Figure 2A shows the first sixteen complete illness-treatment processes of the same patient. It can be seen intuitively that the amount of pathogens after the first 15 antibiotic treatments can return to the pretreatment state. However, the amount of pathogens after the 16th treatment can stabilize to a higher level (Figure 2A).
To further explore why the same treatment can work for only the first 15 times on the same disease, we amplified the details of the first and 16th treatments (Figure 2B and C). After the first antibiotic treatment, the amount of pathogens rapidly decreased to a low level and reached a healthy stable state soon afterwards (Figure 2B). The patient accepted the same treatment after the 16th infection, and the amount of pathogens eventually returned to a high level (the illness stable state) (Figure 2C). The 16th treatment failure implies that the gut microbiota may have developed resistance or pseudo-resistance.
We then confirmed that the antibiotic lose efficacy in the 16th infection is due to the emergence of drug-resistance. Upon the 16th infection, we prolonged the duration of antibiotics treatment from 3 days to 4 months (the sky blue curve in Figure 2D), however, the amount of pathogens still remains at a high level (the illness stable state). The result shows that the amount of pathogens still rebounds to the illness state (the high stable state) even when the permanent treatment was given. Combining the data from the standard treatment and permanent treatment after the 16th illness, these results suggest that the gut microbiota has already developed permanent resistance.
We further studied the improved strategy of FMT (i.e., FMT with antibiotic treatment). The results demonstrate that the amount of pathogens returned to the healthy level in our model (Figure 2E). Therefore, this simulation also reproduced that drug-resistant infections can be eliminated by FMT in clinic. In the amplified details, the total pathogens decreased slightly after antibiotic intake, but instantly rose to higher levels after the inoculation of fecal bacteria and subsequently rapidly declined. These simulation results are consistent with experimental observations in mouse models by Chaysavanh and Jens et al [41].
Therefore, these simulation results confirmed that our model can be used to explore the mechanism of antibiotic-resistance and the effectiveness of FMT. By revealing the dynamic evolution of the gut microbiota, we could provide new ideas for how to reduce the risk of drug resistance in clinical treatments.
We first analyzed steady states to understand key features of this dynamic system, because the measurements of the gut microbiota composition in some studies typically represent steady state behaviors. Many experimental observations have indicated that the microbial ecosystem of each individual has a relatively stable and resilient community [4,5,42,43]. Unless a large perturbation (e.g., antibiotic intake) is introduced, the ecological system remains stable for months or even years. The steady state of ecological dynamics system Eq (2.2) are those positive real solutions that satisfy equations set Eq (2.2). Because sensitive and resistant pathogens in the ecological system Eq (2.2) share the same parameters in the absence of antibiotic intake, we can combine these two variables together to form a new variable P=k1∗PS+k2∗PT. Therefore, we recast system Eq (2.2) to an equivalent two-dimensional system Eq (3.1),
{dPdt=r1(1−P)P−k12B2B2+a2P−d1PdBdt=r2(1−B)B−k21P2P2+b2B−d2B | (3.1) |
in which P indicates the weighted total population of two pathogens.
Then, we focused on this equivalent 2-D system Eq (3.1) to study the steady states. Under some constraint conditions, the system has six steady states, named P0, P01, P30, P1, P2 and P3. Under the parameter values of Table 1, we can get two eigenvalues of the system at each equilibrium point approximately. By caculating the Jacobian matrix of Eq (3.1) at these steady states, we obtained their eigenvalues. The numerical results showed that steady states P1 and P3 are locally asymptotically stable (because eigenvalues at both equilibrium points are negative real roots), and the rest are unstable (Figure 3A). And the two eigenvalues at the equilibrium point P2 are one positive real root and one negative real root, respectively. Therefore, P2 is a saddle point. Here, we are particularly interested in three biologically meaningful steady states: a healthy stable state (P1) with probiotics dominant, a disease stable state (P3) with pathogens dominant and an unstable state (P2).
A one-dimensional stable manifold (m1) of the saddle point (P2) can divide the first quadrant of the phase plane into two regions (R1 and R2). The vector field in Figure 3 depicts the long-term behavior depending on the initial conditions. The trajectories from the initial points in R1 will converge towards the healthy stable state (P1), but the trajectories from R2 will ultimately reach the disease stable state (P3). Therefore, we call the region R1 as the self-healing area, and the region R2 as the non-self-healing area.
In fact, this stable manifold (m1) is also the boundary between the self-healing area and the non-self-healing area. After infection, the trajectory moved away from healthy stable state in our model. If the trajectory crossed this manifold m1 during the illness, it approached the disease stable state (P3) without treatment (the long blue curve in Figure 3C). In our model, we defined this disease as the non-self-healing disease in Figure 3C. In contrast, if the trajectory did not cross this manifold m1 during the illness, it returned to the healthy stable state even without treatment (blue curve in Figure 3B). The second case is the self-healing disease. Once the host infected with the non-self-healing disease, he had to be treated. The force of antibiotic treatment in our model pushes the trajectory back to above the critical manifold (e.g., the sky blue curve in Figure 3C).
Next, we analyzed the illness-recovery processes in the phase plane. In the absence of external disturbances, the gut microbiota stayed in the stable state P1 (in the R1 region) to maintain a functional balance in a healthy intestinal system. Once the host infected diseases, the amount of pathogens increased accordingly, resulting in a decrease in the probiotic amount. The orbit crossed the manifold m1 to the R2 region (red trajectory ① in Figure 4). In the first 15 illness-treatment processes, 3-day antibiotic intakes could push the trajectory across m1 and back to the R1 region (sky blue trajectory ② in Figure 4A). After withdrawing antibiotics, the orbits may automatically return to the healthy stable state P1 (blue trajectory ④ in Figure 4A), because the orbit started from the R1 region. However, in the 16th treatment process, 3-day antibiotic treatment could not push the trajectory back to the R1 region (trajectory ② in Figure 4B). Therefore, the orbit automatically went to the illness stable state P3 (trajectory ④ in Figure 4B), because it started from the R2 region. In the 16th, if we used long-term (even permanent) treatment, the trajectory still could not go back to the R1 region, and finally went to an illness state (trajectory ② in Figure 4C). If FMT combined with antibiotic (Figure 4D) was applied, the state was relocated to the R1 region (sky blue trajectory ②, and green trajectory ③), and then the orbit automatically returned to the healthy stable state P1 (trajectory ④). In summary, the system could reach a stable healthy state (P1) if and only if the microbiota composition state was transferred to the curable region (R1) by treatments. In this 2-D system, the microbial composition state always returned to the same state after repeated antibiotic perturbations (first 15 times). However, many studies have confirmed that the gut microbiota just returns to a stable state similar to that of the pretreatment state [4,5,10].
To test this phenomenon (i.e., the post-antibiotic state is similar to only the pre-treatment state), we further analyzed the dynamic behavior of a 3-D system. Instead of three isolated steady states P1−P3 in the 2-D system, there are three types of steady states (L1−L3) in the 3-D system (Figure 5). The 3-D phase space in Figure 5A depicts the long-term behavior depending on different initial values. The blue line segment L1 and red line segment L3 represent healthy equilibrium states and disease equilibrium states, respectively. The stable manifold (M1) of the unstable steady states in L2 is a 2-D surface in the 3-D phase space. The surface M1 divides the space into two domains (D1 and D2). These trajectories from the initial points in D1 converged towards stable healthy states in L1, but these trajectories from D2 ultimately reached a stable disease state in L3.
Furthermore, we reproduced the first 15 effective treatments (Figure 6A) and the outcomes of different treatments after the 16th illness (Figure 6B-D) in the 3-D phase space. For succinctness, only the first, 10th, 12th and 15th effective treatments are illustrated to show the illness-treatment-recovery process (Figure 6A). During the illness stage, the trajectory went away from the healthy state in L1, and passed the critical interface M1 to enter the D2 domain. Three-day antibiotic intakes could push the trajectory back to the D1 domain. After the withdrawal of antibiotics, the trajectory eventually returned to a healthy stable state in L1, but in a location slightly different from that pretreatment. Furthermore, the recovered healthy states gradually deteriorated (the amount of resistant pathogen increased) with repeating antibiotic treatments (Figure 6A).
During the 16th illness- treatment- recovery processes, the system eventually reached a disease stable state in L3, because the trajectory did not cross the critical interface (M1) after 3-day antibiotic (Figure 6B) or even permanent treatment (Figure 6C). However, after FMT combined with antibiotic treatment, the orbit reached a healthy stable state in L1 (Figure 6D). In fact, the state was relocated to the D1 domain by this combined strategy.
As shown in Figure 6A, the recovered healthy states gradually moved along L1 with repeated antibiotic treatments. From the perspective of pathogen composition, the amount of sensitive bacteria among pathogens gradually decreased, while the amount of resistant pathogens gradually increased with repeated antibiotic perturbations (Figure 6E). Antibiotic resistance emerges when the amount of resistant pathogens reaches a certain level. We used the ratio of resistant pathogens to sensitive pathogens in the healthy stable state to quantify the cumulative effects of repeating antibiotic disturbances. As shown in Figure 6F, this ratio (black dots) gradually increased, and finally passed a critical value (red dashed line) to achieve antibiotic-resistance.
These simulations show that every antibiotic treatment can change the location of the healthy stable state in L1, e.g., antibiotic treatment can permanently change the steady state ratio of resistant pathogens to sensitive pathogens. These results are consistent with the existing experimental observation that after antibiotic withdrawal, the change in the microbial composition state caused by antibiotics will persist for years or decades [4,5]. Repeating antibiotic treatments pushed the ratio towards the "bad" direction; that is, resistant pathogens in the post-recovery stable state increased in abundance. When the ratio of resistant pathogens to sensitive pathogens exceeds a threshold value, the antibiotic resistance of the gut microbiota emerges. This model affirmed that the successive shift of post-recovery stable states on L1 was essentially the change of the ratio (PT to PS).
In summary, every antibiotic treatment will permanently change the steady state ratio of sensitive pathogens to resistant pathogens. This small change is the foundation of development of antibiotic resistance. The effects of antibiotic intake will gradually accumulate. Due to the accumulation of the antibiotic effect, the ratio of sensitive pathogens to resistant pathogens continues to changing and eventually exceeds a certain value, inducing the development of antibiotic resistance.
In the above analysis, we confirmed that the ratio of sensitive pathogens to resistant pathogens is the root cause of antibiotic resistance. Therefore, we first studied how the initial pathogen composition influences the development of resistance. In Figure 7A, the x-axis stands for the initial ratio of sensitive pathogens to resistant pathogens in healthy individuals. The number of effective antibiotic treatments (before 3 days of antibiotic treatment failed) increased logarithmically with the initial ratio. Every antibiotic treatment increases the ratio of resistant pathogens to sensitive pathogens, until the ratio reaches a threshold to form the resistance. A higher initial ratio indicates a shorter distance to the threshold and fewer effective treatments before resistance than those for a lower initial ratio. Therefore, the initial pathogen composition structure is crucial in determining resistance.
In fact, probiotics also play an important role in the development of antibiotic resistance. Here, we further explored the risk of antibiotic resistance in different microenvironments of the intestinal microbiota. Usually, a higher level of beneficial bacteria means a better microenvironment because beneficial bacteria can inhibit the growth of pathogens. In our model, we used the growth rate of beneficial bacteria, which determines the beneficial bacteria amount in the healthy state, to describe the microenvironment of different hosts.
We simulated the total number of effective treatments in different microenvironments. The results show that the number of antibiotic treatments increased with an increasing reproduction rate (r2) in a small window between the gray region and the green region (Figure 7B). When the reproduction rate of probiotics was relatively small, antibiotic resistance emerged in the first treatment stage (the gray region in Figure 7B). However, when the reproduction rate of probiotics was high, 3-day antibiotic treatment remained effective and resistance never emerged (the green region in Figure 7B).
In addition to the amount of beneficial bacteria, the composition of beneficial bacteria may also be different in different hosts. In our model, we assumed that different compositions of beneficial bacteria can alter the maximum inhibition coefficient of probiotics to pathogens. Then, we explored the number of antibiotic treatments with different inhibition coefficients. Similarly, we also found that increasing the inhibition intensity of probiotics to pathogens could prolong the number of antibiotic treatments to varying degrees (Figure 7C).
Taken together, these simulations show that beneficial bacteria are indispensable in the intestinal flora composition. Enhancing the level or optimizing the endogenous composition of beneficial bacteria can effectively delay or even prevent (depending on the parameters) the development of antibiotic resistance.
Moreover, excessive administration (longer medication duration) of antibiotics had an important effect on the development of antibiotic resistance (Figure 7D). The x-axis represents the duration of antibiotic administration in a course of treatment. In contrast to the initial ratio of pathogens, the number of antibiotic treatments decreased logarithmically with the duration of antibiotic administration. Compared with standard treatment, prolonged antibiotic treatment could accelerate the change in the ratio of sensitive pathogens to resistant pathogens. In other words, excessive administration of antibiotics could promote the development of antibiotic resistance. Therefore, following the medication guidelines or the doctor's advice is very important.
Next, from the perspective of drugs, we explored their effects on antibiotic resistance. Since the application of penicillin in the clinic in 1940, the number of antibiotics has reached several thousand, and there are several hundred commonly used antibiotics in clinical practice. The gut microbiota has different responses to these antibiotics. Therefore, we studied the effect of different antibiotics, which corresponds to different parameter values of η1, η2 and η3 in our model.
Here, we explored the risk of antibiotic resistance from two aspects. The first aspect is that of the bactericidal rates against resistant pathogens. Here, we fixed the bactericidal rate of antibiotics against sensitive pathogens (η2=0.086) and probiotics (η3=0.015) and tested the resistance risk of different antibiotics (with different bactericidal rates for resistant pathogens, i.e., η2).
Generally, extending the antibiotic medication time may cure the illness. In our model, resistance meant that the infection cannot be cured even under endless antibiotic treatment. Therefore, we observed the medication time (may be finite or infinite days) of achieving an effective treatment to determine whether antibiotic resistance emerged.
Under the conditions of Figure 8A (η2=0.016), the medication time, T, was three days to achieve an effective treatment in the first eighteen infection-treatment processes. In the nineteenth treatment, the required medication time to achieve an effective treatment was prolonged to 4 days. In the twentieth infection, the required medication time was further extended to 9 days (Figure 8A). Furthermore, the antibiotic resistance emerged in the twenty-first treatment stage. We call this type of antibiotic resistance response incurable resistance because treatment failed even with permanent medication.
Under a different parameter value, η2=0.044 (Figure 8B), the required medication time was gradually extended as the infection time increased. Starting from the forty-first infection, the system could always return to a healthy stable state after a 5-day treatment. Therefore, the gut microbiota did not develop incurable resistance to this antibiotic (η2=0.044). Here, we call this type of antibiotic resistance responses a curable antibiotic resistance response or pseudorandom resistance.
In our model, we defined the culminating medication time as the required maximum medication time for a specific antibiotic. Hence, the culminating medication time for specific antibiotic (η2=0.016) was infinity and the culminating medication time was 5 when η2=0.044.
These simulations (Figure 8A and 8B) implied that the bactericidal rate (η2) may be a key factor determining the risk of antibiotic resistance. The culminating medication time can be used an effective indicator to measure the resistance of antibiotics. We further studied the culminating medication time for different antibiotics (i.e., different η2 values) (Figure 8C). Simulation result showed that a critical threshold (black dashed line) of the bactericidal rate (η2) did exist. When η2 exceeded the critical threshold, the culminating medication time was finite and was a decreasing function of η2 (red polygonal line). When η2 was below the critical threshold, the culminating medication time was infinite (gray region). This meant that the incurable antibiotic resistance is bound to occur.
The second aspect is that of bactericidal rates against beneficial bacteria (i.e., different η3 values). Therefore, we fixed the bactericidal rate of antibiotics against sensitive pathogens (η1=0.086) and resistant pathogens (η2=0.03) and explored the effect of different η3 values on antibiotic resistance. Similarly, our simulations presented two different types of antibiotic resistance responses.
Under the conditions of Figure 8D (η3=0.008), the medication time was three days to achieve an effective treatment in the first twenty-five infection-treatment processes. Beginning with the twenty-sixth treatment, the required medication time began to increase gradually from 4 days to 7 days. The culminating medication time was 7 days. In contrast, under the conditions of Figure 8E (η3=0.044), the culminating medication time was infinity.
We also explored the culminating medication time for different bactericidal rates against beneficial bacteria (i.e., η3). Simulation result showed that the critical threshold (black dashed curve in Figure 8F) of η3 existed. When η3 was below the critical threshold, the culminating medication time was finite and was an increasing function of η3 (red polygonal line). When η3 exceeded the critical threshold, the culminating medication time was infinite (gray region).
Altogether, a good antibiotic should have a stronger bactericidal rate against resistant pathogens and a weaker bactericidal rate against probiotics. Therefore, our model can also be recommended for optimizing the design of pharmaceutical compositions so that antibiotics have precise lethality.
In recent decades, clinical practices and mouse models have proven that a burgeoning therapeutic strategy (i.e., FMT) can effectively treat infections caused by resistant pathogens [12,13,14]. However, the fundamental mechanism of FMT in reshaping the gut microbiota has not been fully elucidated and there also remains a knowledge gap on how to improve FMT efficiency.
To determine the benefits of FMT, we compared the therapeutic efficacy of two different strategies (i.e., permanent antibiotic treatment and FMT combined with antibiotic treatment) in the P-B plane. Here, the horizontal axis P is the total amount of resistant pathogens and sensitive pathogens (i.e., P=k1∗PS+k2∗PT) and the vertical axis B is the amount of the beneficial bacteria. Before the 16th infection, the microflora composition remained in the healthy stable state P1 (blue dot). After infection, the population of pathogens rapidly reproduced and grew in size, resulting in a decrease in the amount of non-pathogens. The orbit crossed the manifold m1 to enter the R2 region (red trajectory ① in Figure 9). In the pure antibiotic treatment, permanent antibiotic therapy was used to eliminate the infection (Figure 9A). The trajectory eventually stabilized to a disease state because this treatment failed to push the trajectory (sky blue trajectory ② in Figure 9A) back into the R1 region. This result implied that the 16th infection was a resistant infection.
Furthermore, we tested the efficacy of FMT combined with antibiotic therapy in eliminating the 16th infection. We assumed that the donor fecal microbiota has a healthy stable state (denoted as (PSh,PTh,Bh) corresponding to (Ph,Bh) in the 2-D system). This assumption is because most healthy hosts have a similar "core microbiota". In addition, some studies have pointed out that many factors (such as bowel preparation for the procedure, fresh and frozen material, administration, methods of microbiota preparation and the source of fecal microbiota) may affect the efficiency of FMT [14,44,45,46,47,48]. To make our model more realistic, we introduced the survival rate k (0<k<1) to characterize the transplant survival rate of the donor fecal microbiota. The fecal bacteria injection transfers the transient state (P,B) to a new state (P,B) +k(Ph,Bh). Therefore, the original location of (P,B) is a decisive factor in determining whether the system state (P,B)+k(Ph,Bh) falls into the R1 region after FMT, when the colonization rate k is fixed. In addition, we defined the onset range (the purple pink region) of a single FMT which consists of many states in the phase plane. These states (P, B) within the onset range could be transferred to the R1 region by a single FMT. In Figure 9B, the treatment effect of FMT with antibiotic is shown: first, the trajectory of infection crossed the manifold m1 to enter the R2 region (red trajectory ①); second, the 3-day antibiotic pretreatment pushed the trajectory into the onset range (sky blue trajectory ②); third, FMT pulled the trajectory back to the R1 region (green trajectory ③); and finally, the trajectory (blue trajectory ④) stabilized to the healthy stable state P1.
Combining the above two therapeutic effects, it can be identified that the large number of probiotics from donors is a key factor affecting the therapeutic effect of FMT. The overwhelming advantage of probiotic to pathogen abundances ensured that the vector k (Ph,Bh) pointed roughly upward in the 2-D phase plane. Therefore, the upward- driven force caused by FMT facilitated the system state crossing the boundary m1 to enter the R1 region.
Antibiotic pretreatment in the combined therapy is also very important. Compared to FMT combined with antibiotic therapy, the 16th infection could not be eliminated by using FMT alone. The system transient state (Pd,Bd) after infection fell out of the onset range so that the trajectory of FMT (green trajectory ③ in Figure 9C) did not cross the manifold m1. Thus, the system finally stabilized to the disease stable state P3. However, 3-day antibiotic pretreatment could push the trajectory (sky blue trajectory ② in Figure 9B) into the onset range of FMT so that it successfully crossed the manifold m1 to enter the R1 region after a single FMT (green trajectory ③ in Figure 9B). Altogether, antibiotic pretreatment is also another key factor in determining the therapeutic efficacy of combined therapy (FMT with antibiotic) by facilitating the transition of the system state into the onset range.
Therefore, the mechanism of action of FMT combined with antibiotic is essentially a synergistic effect of antibiotic pretreatment and FMT. On the one hand, antibiotic pretreatment reduced the amount of whole microbiota (i.e., decreased P and B, respectively), thereby improving the effect of FMT. On the other hand, a large number of probiotics (in the fecal microbiota of donors) ensured that the direction of transplantation (i.e., (Ph,Bh)) pointed roughly upward in the 2-D phase plane. These simulation results are consistent with experimental phenomena; that is, antibiotic pretreatment promotes the successful colonization of the transplanted bacteria in the host [41,49].
In the above analysis, we confirmed that the use of FMT can effectively fight diseases that are resistant to antibiotics. In this section, we will explore the long-term effects of early usage of FMT (with antibiotic) on the formation of antibiotic resistance by examining the total number of treatments.
We first analyzed the total number of treatments for two different strategies, pure antibiotic treatment and FMT combined with antibiotic (Figure 10A) under a specific antibiotic and survival rate. In our previous analysis, FMT was used only after the system had revealed antibiotic resistance (Figure 4D or 6D). Here, FMT was used in advance, for example, after the first infection or second infection, to test the benefits of early application of FMT. In Figure 10A, the x-axis indicates the i-th infection from which the transplantation starts. For example, the 7-th infection indicates that the first 6 illnesses were treated with antibiotic; from the seventh infection, the host was treated with FMT combined with antibiotic. The y-axis is the total number of effective treatments. Additionally, we used the orange and blue bars to distinguish FMT combined with antibiotic treatment and antibiotic treatment, respectively. If FMT combined with antibiotic was used from the first illness, the treatment strategy could be used up to 35 times (the first bar). If FMT combined with antibiotic was used after using antibiotic 5 times, this strategy could be used only 24 times (the 6-th blue bar). Since the sixteenth infection, the standard antibiotic treatment was no longer effective (gray area). However, usage of FMT combined with antibiotic still could achieve 6 effective treatments (the 16-th orange bar). These simulation results indicate that early usage of FMT can delay the development of antibiotic resistance.
The above results are based on parameters in Table 1. These parameters, especially the bactericidal rate of antibiotics and the survival rate (i.e., ηj and k) may vary in the real environment. Therefore, the effect of early usage of FMT combined with antibiotic requires further study in a broad parameter space. Simulation results (different η2 and k values) show different typical cases. Under some parameters, the systems eventually exhibited resistance to FMT combined with antibiotic (Case A). However, under other parameters, the systems never exhibited resistance to FMT (with antibiotic) (Case B). In some situations, use of FMT combined with antibiotic could rescue the treatment after antibiotic resistance emergence (Case Ⅱ), but in other situations even FMT combined with antibiotic failed (Case Ⅰ). In all these typical cases (Case A-Ⅰ in Figure 10B, Case A-Ⅱ in Figure 10C, Case B-Ⅰ in Figure 10D and Case B-Ⅱ in Figure 10E), early usage of FMT could extend the number of effective treatments, compared with those of FMT combined with antibiotic use after resistance emergence.
For Case A-Ⅰ, after 16 treatments with antibiotic, antibiotic resistance emerged (gray region in Figure 10B) under certain parameters. If FMT combined with antibiotic was used first after resistance emergence, the treatment could not be rescued (the 17-th bar). However, if FMT combined with antibiotic was used beginning at the sixteenth infection, it could be used 10 times (the 16-th orange bar). If the combined treatment was used beginning at the first infection, the total number of effective treatments was 35 (the 1-st bar). The total number of effective treatments will be prolonged only if FMT is used in advance.
For Case A-Ⅱ, after 15 treatments with antibiotic, antibiotic resistance emerged (gray region in Figure 10C) under certain parameters. Unlike Case A-I, if FMT combined with antibiotic pretreatment was first used after resistance emergence, the treatment could be rescued, and up to 30 effective treatments were achieved (the 16-th orange bar). If FMT combined with antibiotic was first used beginning at the eleventh infection, it could be used 41 times (the 11-th orange bar). If the combined treatment was used from the first infection, the total number of effective treatments was 58 (the 1-st bar). Regardless of whether early FMT (with antibiotic) was employed, it always extended the number of effective treatments.
For Case B-Ⅰ, antibiotic resistance emerged (gray region in Figure 10D) after 16 treatments with antibiotic. If FMT combined with antibiotic pretreatment was first used after resistance emerged, the treatment could not be rescued (the 17-th bar). However, if the combined treatment was used in advance, the treatment was permanently effective (for example, the 16-th orange bar).
For Case B-Ⅱ, after 18 antibiotic treatments, antibiotic resistance emerged (gray region in Figure 10E). If FMT combined with antibiotic pretreatment was first used after resistance emergence, the treatment could be rescued. Moreover, the treatment was permanently effective (the 19-th orange bar).
All of the above four typical cases indicated that the early use of FMT combined with antibiotic pretreatment can significantly delay antibiotic resistance emergence (Figure 10A-E). Furthermore, in some cases, early use of the combined therapy could even permanently prevent the development of antibiotic resistance (Figure 10D-E). These results suggest that early usage of FMT combined with antibiotic could be recommended for clinical treatment.
In our model, we used FMT combined with 3-day antibiotic pretreatment to simulate the clinical recovery treatment protocol, antibiotic pretreatment combined with a single FMT. In clinical trials, feces dilutions by the donor were infused into the patient through a nasoduodenal tube (2 to 3 minutes per 50 ml) and the tube was removed after 30 minutes [15]. A single FMT in clinical treatment was usually used to treat patients with Clostridiumdifficile infection or refractory intestinal infection. However, some diseases require multiple FMTs, such as Crohn's disease or ulcerative colitis [50,51,52].
Next, we clarified whether multiple FMTs are superior to a single FMT. In fact, the onset range of multiple FMTs directly reflects the corresponding curable range. Therefore, we analyzed the variety of onset ranges in the phase plane (P - B). Here, the onset range consisted of those states that can be transferred to the R1 region by multiple FMTs. A single FMT pushed the transient state (P, B) in the R2 region up to a new state (P,B)+k(Ph,Bh) along the vector (Ph,Bh) (green arrow in Figure 11A). Therefore, the critical curve of a single FMT (red curve) is obtained directly by moving the manifold m1 downward by k(Ph,Bh). The upper left region (red) surrounded by the boundary and manifold (m1) is the onset range of a single FMT. Here, we assumed that the second FMT was performed on the second day after the first FMT. The corresponding boundary (two FMTs) was not simply obtained by moving the boundary (the first FMT). Therefore, we simulated the process to obtain the onset range of two FMTs (green). Generally, the onset range of two FMTs was bigger than that of a single FMT. Similarly, the onset range of n-FMT was larger than that of n-1 FMTs. This implies that multiple FMTs are better than a single FMT (Figure 11).
Since the onset range for every FMT is increasing, whether the corresponding onset range of infinite FMTs can cover the whole non-self-healing area (R2) remains to be further explored. In fact, there may be different cases. As shown in Figure 11A, the onset range of finite FMTs can fully cover the whole region of R2. However, under other parameters, the onset range of infinite FMTs can cover only a local region of R2 (Figure 11B). Further observation showed that the increase in the onset range (each FMT) gradually decreased in the second case, while in the first case, the opposite was true.
Altogether, these results show that multiple FMTs have a wider therapeutic range than a single FMT. Therefore, multiple FMTs can be recommended for the treatment of some serious infections or infections caused by "superbugs".
In the above analysis, fecal microbiota from donors with no history of medication was used for the microbiota of transplantation. However, in the real life, donors with no history of medication are quite rare. The fecal material may come from different donors, i.e., the composition of pathogens or probiotics is different. The transplant microbiota may also come from the same donor, i.e., the composition of pathogens and probiotics are the same but the composition of resistant pathogens and sensitive pathogens is different. In this section, we will explore the second case, in which a different composition of pathogens is caused by repeating antibiotic treatment.
Next, we analyzed the corresponding total number of treatments with FMT combined with antibiotic pretreatment (in the second case) in Figure 12A. Here, the x-axis and z-axis represent the i-th infection and the total number of FMT combined with antibiotic treatments, respectively. For instance, the 6-th bars indicate that patients were treated with the combined therapy from the sixth infection. From the x-z plane, it can be seen that the sooner FMT is used, the greater the number of FMT treatments is. The y-axis represents the number of times the donors have taken medicine. For example, 5-D denotes fecal material from donors that have taken medicine five times. From the y-z plane, we can see that the fewer number of times the donors take medicine (in Figure 12A y-axis), the greater the number of FMT treatments is. Furthermore, we explored the corresponding total number of treatments for early use of FMT combined with antibiotic in comparison with the standard antibiotic treatment. Here, the blue bar indicates the 3-day antibiotic treatment; the orange bar indicates FMT (with antibiotic) treatment. Figure 12B presents the use of FMT (with antibiotic) beginning at the first infection. Surprisingly, when fecal microbiota donors have taken medications less than 13 times, the total number of treatments was the same, despite the use of different fecal microbiota. However, when fecal microbiota donors have taken medicine 13 times or more, the total number of treatments decreased dramatically. We observed similar phenomena even when FMT (with antibiotic) treatments were not used until the 10th (Figure 12C) or even 16th infection (Figure 12D). These simulation results show that early use of FMT (with antibiotic) may accelerate the emergence of antibiotic resistance when fecal microbiota donors have taken medicine several times. This finding may be the reason why screening of donors should first exclude donors with a history of serious illness.
In brief, we confirmed that fecal material should come from donors who have taken fewer medicines. Clinically, the screening criteria should be strictly followed and further combined with "the exclusive method" to obtain the healthiest donors.
We established a dynamic microbiota model to describe the comprehensive effects of antibiotic therapy, interspecies inhibition and resource competition on the balance of the intestinal flora. The development of antibiotic resistance was revealed by simulating multiple complete illness - treatment - recovery processes. That is, repeated antibiotic intake promoted the proportion of resistant pathogens and sensitive pathogens in the healthy state to exceed a specific threshold, which led to the emergence of drug resistance.
We believe that the proposed resistance mechanism is also widely applicable in the treatment of other diseases. Drug resistance is commonly seen in various clinical treatments. For example, the first-line treatment of metastatic prostate cancer uses androgen deprivation therapy (ADT), but nearly all men progress to a resistant stage. In metastatic prostate cancer, there are three competing phenotypes: (ⅰ) T+ cells are sensitive to ADT; (ⅱ) TP (testosterone producing) cells are sensitive to ADT but also helps T+ cells; and (ⅲ) T− cells are resistant to ADT [53,54]. This kind of competing structure is similar to our sensitive pathogens / probitcs / resistant pathogens framework. Thus, the mechanism of drug resistance here may be similar to that proposed by us.
Furthermore, our model confirmed that antibiotic resistant infections could be cured based on FMT combined with antibiotic. In particular, the numerical simulation predictions also showed that early usage of FMT can delay or prevent the development of antibiotic resistance. This finding implied that multiple FMTs or early use of FMT may be implemented instead of antibiotic therapy alone to prevent the emergence of antibiotic resistance in the future. Therefore, we should strive to improve fecal microbiota transplantation so that it can be widely used in the clinic. Specifically, the screening criteria for fecal bacteria and transplantation techniques need to be improved. Similarly, in the treatment of cancer, some scholars have found that adaptive therapy can delay the emergence of drug resistance more effectively than those of the standard cure or continuous MTD treatment [53]. Therefore, adaptive therapy may also be an effective treatment to delay the development of antibiotic resistance. Besides, some investigations have shown that the prevalence of resistant microbiota decreased markedly following a reduction in specific antibiotic use in agricultural areas. Hence, we need to standardize and strictly limit the use of antibiotics to reduce the overall outbreak of antibiotic resistance.
The main purpose of this study is to qualitatively explain how drug-resistance emerges upon the accumulation of the effect of antibiotics. Although our mathematical model is rather simple, we maintain the most critical reactions among different intestinal flora in the mathematical model, which is the most essential reason for the mechanism of the emergence of drug-resistance. The mathematical model is still somewhat limited because it is difficult to determine all the real parameter values from experimental data or other literature. Therefore, we evaluate the relative value of some parameters based on the clinical data. For example, the killing rate of antibiotic for sensitive bacteria is larger than other bacteria [55]. Other parameters are determined according to the time scale of the clinical observations and some biological phenomena [56,57,58]. Regarding to the other limitation of this study, the main approach to reveal the mechanism of drug-resistance is numerical simulations with fixed parameter values. In fact, we also intended to perform some analytical studies upon system (2.2) and system (3.1). However, due to the complexity of the functions in the systems, we can't determine theoretically if all these sufficient conditions can be satisfied at the same time. Therefore, to analysis the stability, we will use piecewise linear system to approximate system (2.2) and system (3.1) in the future work.
This work is supported by the National Natural Science Foundation of China (Grant No. 11671417 and 11701405), the Jiangsu Province Science Foundation for Youths (Grant No. BK20170328), and the Priority Academic Program of Jiangsu Higher Education Institutions (Grant No. 5832004317).
All authors declare no conflicts of interest in this paper in this section.
1. | Shaillay Kumar Dogra, Joel Doré, Sami Damak, Gut Microbiota Resilience: Definition, Link to Health and Strategies for Intervention, 2020, 11, 1664-302X, 10.3389/fmicb.2020.572921 | |
2. | Lei Chen, Suresh Kumar, Hongyan Wu, A review of current antibiotic resistance and promising antibiotics with novel modes of action to combat antibiotic resistance, 2023, 205, 0302-8933, 10.1007/s00203-023-03699-2 |
Symbols | Definition | Value (day−1) |
r1 | Growth rate of pathogens | 0.664 (without infection) |
0.664∗1.32 (with infection) | ||
r2 | Growth rate of probiotics | 0.718 |
d1 | Death rate of pathogens | 0.0501 |
d2 | Death rate of probiotics | 0.0751 |
k12 | Maximum inhibition coefficient of probiotics on pathogens | 0.76 |
k21 | Maximum inhibition coefficient of pathogens on probiotics | 0.84 |
k1 | Competitive coefficient of PT against PS | 0.5 |
k2 | Competitive coefficient of PS against PT | 0.5 |
a | Probiotics amount that yields 50% of k12 | 0.58 |
b | Pathogens amount that yields 50% of k21 | 0.58 |
η1 | Killing rate of antibiotic for PS | 0.086 (with antibiotic) |
η2 | Killing rate of antibiotic for PT | 0.0016 (with antibiotic) |
η3 | Killing rate of antibiotic for probiotics | 0.015 (with antibiotic) |
k | Survival rate of fecal microbiota | 0.5 |
Symbols | Definition | Value (day−1) |
r1 | Growth rate of pathogens | 0.664 (without infection) |
0.664∗1.32 (with infection) | ||
r2 | Growth rate of probiotics | 0.718 |
d1 | Death rate of pathogens | 0.0501 |
d2 | Death rate of probiotics | 0.0751 |
k12 | Maximum inhibition coefficient of probiotics on pathogens | 0.76 |
k21 | Maximum inhibition coefficient of pathogens on probiotics | 0.84 |
k1 | Competitive coefficient of PT against PS | 0.5 |
k2 | Competitive coefficient of PS against PT | 0.5 |
a | Probiotics amount that yields 50% of k12 | 0.58 |
b | Pathogens amount that yields 50% of k21 | 0.58 |
η1 | Killing rate of antibiotic for PS | 0.086 (with antibiotic) |
η2 | Killing rate of antibiotic for PT | 0.0016 (with antibiotic) |
η3 | Killing rate of antibiotic for probiotics | 0.015 (with antibiotic) |
k | Survival rate of fecal microbiota | 0.5 |