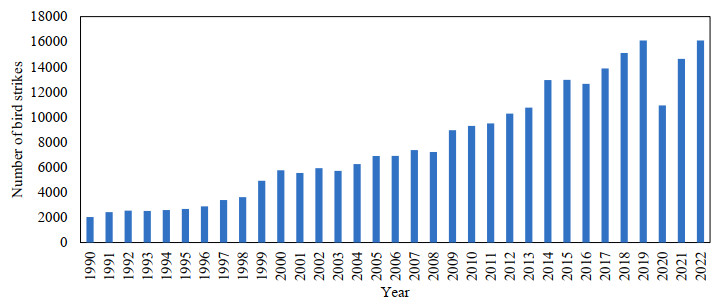
Citation: Wenchao Cai, Yadong Zhou. Preventing aircraft from wildlife strikes using trajectory planning based on the enhanced artificial potential field approach[J]. Metascience in Aerospace, 2024, 1(2): 219-245. doi: 10.3934/mina.2024010
[1] | James H. Page, Lorenzo Dorbolò, Marco Pretto, Alessandro Zanon, Pietro Giannattasio, Michele De Gennaro . Sensitivity analysis of mixed analysis-synthesis flight profile reconstruction. Metascience in Aerospace, 2024, 1(4): 401-415. doi: 10.3934/mina.2024019 |
[2] | Jiaqing Kou, Tianbai Xiao . Artificial intelligence and machine learning in aerodynamics. Metascience in Aerospace, 2024, 1(2): 190-218. doi: 10.3934/mina.2024009 |
[3] | O.N. Korsun, Om Moung Htang . The practical rules for aircraft parameters identification based on flight test data. Metascience in Aerospace, 2024, 1(1): 53-65. doi: 10.3934/mina.2024003 |
[4] | Igor V. Bezmenov . Trend detection in time series of measurement data in solving problems in space geodynamics and other research areas. Metascience in Aerospace, 2024, 1(3): 268-291. doi: 10.3934/mina.2024012 |
[5] | Hussein Kokash, G. Gilou Agbaglah . A two- to three-dimensional wake transition mechanism induced by the angle of attack of a NACA0012 airfoil. Metascience in Aerospace, 2024, 1(3): 329-345. doi: 10.3934/mina.2024015 |
[6] | Yuan Hu, Zhihao Jiang, Xintai Yuan, Xifan Hua, Wei Liu . Isometric mapping algorithm based GNSS-R sea ice detection. Metascience in Aerospace, 2024, 1(1): 38-52. doi: 10.3934/mina.2024002 |
[7] | Igor V. Bezmenov . Fast algorithm for cleaning highly noisy measurement data from outliers, based on the search for the optimal solution with the minimum number of rejected measurement data. Metascience in Aerospace, 2024, 1(1): 110-129. doi: 10.3934/mina.2024005 |
[8] | Pasynok Sergey . Cumulative STF coefficients evaluation and validation. Metascience in Aerospace, 2024, 1(4): 371-378. doi: 10.3934/mina.2024017 |
[9] | Jubilee Prasad Rao, Sai Naveen Chimata . Machine learning-based surrogates for eVTOL performance prediction and design optimization. Metascience in Aerospace, 2024, 1(3): 246-267. doi: 10.3934/mina.2024011 |
[10] | Victor Cánepa, Pablo Servidia . Design and evaluation of INS/GNSS loose and tight coupling applied to launch vehicle integrated navigation. Metascience in Aerospace, 2024, 1(1): 66-109. doi: 10.3934/mina.2024004 |
Bird collisions have been a serious issue for airplanes since the first recorded flight in 1912 [1]. Bird collisions predominantly occur during aircraft takeoffs and landings [2], posing threats to flight safety and the lives of both passengers and birds. Additionally, these collisions result in significant financial losses, amounting to millions of dollars for airline companies and the aviation sector annually.
To illustrate the impact of these collisions on the aviation sector, several nonfatal and fatal aviation accidents attributed to bird strikes have been documented [1]. For instance, historical records reveal instances such as Orville Wright's diary entry in 1905, detailing a nonfatal bird collision with his aircraft, the Wright Flyer III, while flying over a cornfield near Dayton, Ohio, USA [3]. Another incident occurred on April 3, 1912, marking the first bird collision resulting in a human fatality. This tragic event involved Calbraith Rodgers' airplane colliding with a gull while flying along the beach in southern California [4]. Furthermore, there have been three incidents highlighting the catastrophic nature of bird collisions during flights. In one instance, US Airways Flight 1549, an Airbus A320 carrying 155 passengers, struck a flock of geese shortly after takeoff from New York City to Charlotte, North Carolina, on January 15, 2009, causing all engines to lose power. The skilled pilots were forced to execute an emergency landing on the Hudson River in Midtown Manhattan due to their low altitude, saving all passengers and crew [5]. Another tragic accident occurred on August 28, 2012, when a Dornier 228 aircraft collided with a black kite during takeoff from Kathmandu, Nepal, resulting in the death of 16 passengers and 3 crew members [6]. Similarly, Ural Airlines Flight 178, an Airbus A321 carrying 226 passengers and 7 crew members, was forced to make an emergency landing in a cornfield 3 miles from Zhukovsky International Airport in Moscow, Russia, on August 15, 2019. The aircraft's engines were damaged by a flock of gulls during takeoff, causing a loss of thrust and preventing the flight from continuing [7].
Bird collisions with aircraft are a major problem that leads to considerable material damage each year. The major financial costs are attributed to repairs, renewal of faulty aircraft parts, and flight delays or cancelations. For example, the damages and the postponements hit approximately US$1.2 billion annually [8]. The total aviation losses due to bird collisions from 1990 to 2022 in the U.S. have been estimated at US$1,063,400,000. Figure 1 illustrates tens of thousands of bird collisions involving civil airplanes reported by pilots, airports, and airline companies in the U.S. to the Federal Aviation Administration's wildlife strike database [9]. However, due to incomplete reporting, these figures should be viewed as conservative estimates [10,11,12,13]. The upward trend signifies a concerning increase in wildlife strikes, attributed to various factors such as the growth of bird populations, increased air traffic, the use of quieter aircraft, and higher aircraft movements [14]. Figure 1 shows that the number of wildlife strikes generally increased, except for 2020 when the COVID-19 pandemic significantly affected airliners. Although the absolute numbers decreased overall, bird strikes surged in many locations. In 2021, approximately 15,000 bird collisions with civil airplanes were reported in the U.S. [15].
Statistical analyses indicate that bird collisions are most likely to occur during takeoff and landing phases [16], while the frequency of such collisions tends to decrease when aircraft fly at higher altitudes. The probability of damage from bird collisions increases at greater altitudes, particularly when aircraft are flying at higher speeds. Furthermore, the severity of damages tends to escalate during landing, as the power of engines gradually decreases [17]. Given the projected rise in Advanced Air Mobility (AAM) operations in airspace below 400 meters, bird strikes pose a significant threat to these operations. Despite the safety and financial concerns associated with bird collisions, the AAM community has yet to take decisive action to lower the risk of bird strikes with emerging low-altitude AAM aircraft [15,18,19].
The study introduces a solution approach for addressing local minimum (LM) and unattainability issues by enhancing the conventional artificial potential field (APF) approach. This is achieved through modifications to the gravitational and repulsive field functions, along with the introduction of safety length and step size rules. To reduce the number of bird strike incidents, the study integrates artificial potential energy (APE) and morphological smoothing techniques to adjust the trajectory. Therefore, the proposed method combines both APE and morphological smoothing not only to reduce the severity of bird collisions but also to maintain the trajectory within a shorter timeframe.
The article contributes to the following aspects:
(1) The development of bird obstacle potential energy fields, target gravitational potential energy fields, and other obstacle potential energy fields. By superimposing the potential energy of individual elements, the total potential energy field (PEF) is determined. This PEF is then used to find the path with the minimum potential energy as the outcome of the artificial potential field (APF) planning process.
(2) Adjustment of the planned APF is achieved through manipulation of the gravitational coefficient (where a higher coefficient implies greater safety) and the repulsive coefficient (where a higher coefficient results in a more optimal distance). This adjustment aims to achieve an optimal trajectory that simultaneously considers both aircraft safety and route distance.
(3) Recognition of the limitation of the APF in incorporating flight radius constraints, which may result in trajectories that do not meet these constraints. To address this, morphological filtering is introduced to smooth trajectories, ultimately determining the final trajectory while taking into account the flight radius.
The rest of the article is outlined as follows: The literature review is presented in Section 2. Section 3 is allocated to the proposed method. Section 4 presents the simulation study. Section 5 presents a discussion of the conducted research. The conclusion is given in Section 6.
Ensuring the safety of aircraft and determining the optimal route distance presents a multi-objective optimization challenge. Aircraft frequently encounter groups of birds during their flight paths, necessitating the need to navigate around bird obstacles while minimizing deviations from the intended trajectory. Therefore, the proposed method integrates both APE and morphological smoothing techniques. This combined approach not only reduces the potential severity of bird collisions but also facilitates the maintenance of the trajectory within a shorter timeframe.
Previous studies have primarily focused on identifying birds with a high likelihood of colliding with aircraft in various positions [20] and verifying the parameters influencing the probability of bird collisions [21]. Factors such as material parameters, geometry, impact velocity, and the altitude of bird collisions can significantly affect the resulting deformation and damage mechanisms of the aircraft structure [22]. Compared to metallic materials, composites display more complex impact damage mechanisms, including matrix cracking, fiber fracture, and delamination [23]. The cost and the expected number of future collisions have also been forecasted [24]. While these studies have resulted in a better understanding of the characteristics, they do not directly contribute to reducing the likelihood of incidents [15]. However, the chances could be minimized by predicting future bird movements and trajectories using past and real-time data. Thus, surveillance sensors such as avian and weather radars can be employed for detection and tracking purposes. Subsequently, these forecasts could inform trajectory planning (TP) to prevent collisions. For example, a simple linear regression model was developed to predict bird movements [25], although its accuracy was insufficient for implementation in TP.
Previous studies on bird collisions have primarily focused on verifying the parameters that influence bird collisions and their effects on aircraft. These studies aim to hinder or minimize the probability of bird collisions with airplanes by predicting bird movements to maintain flight trajectories and implement deconfliction approaches [24,25,26]. However, a few studies have been conducted to develop forecasting algorithms for predicting birds' flight trajectories [27] and to utilize them in trajectory planning for strategic deconfliction between aircraft and birds [28]. These studies involve detecting birds with a high likelihood of collisions in various positions and verifying parameters that influence the likelihood of bird collisions, to estimate costs and forecast the future quantity of bird collisions in specific areas [28].
To reduce the impact of bird collisions, it is crucial to identify birds with a high likelihood of colliding with aircraft, verify their flight characteristics, and determine the key parameters that influence these collisions. An analysis of 79 bird species identified the top ten birds most commonly involved in collisions with airplanes in the U.S.: red-tailed hawks, Canadian geese, turkey vultures, pigeons, mourning doves, gulls, American kestrels, barn swallows, European starlings, and killdeers [29]. Several studies have investigated the essential parameters that increase the likelihood of serious bird collisions [24,25,26,30,31]. These parameters include altitude, time of day, location, environmental conditions, season, and aircraft characteristics. Factors such as aircraft size and engine type can significantly influence the likelihood of bird collisions. For instance, large aircraft with turbofan engines can ingest birds.
Some studies employed numerical methods to analyze and predict the extent of damages. A numerical approach was presented to forecast bird strikes that caused damage to aeronautical parts constructed from various composite and metallic materials [32]. The numerical methods commonly used for simulating the high-speed bird impacts on actual aeronautical frameworks were compared [33]. Three numerical methods were primarily investigated. These included the employment of rigid body components to model, the employment of Lagrange theory to consider bird deformation within the effect, and the adoption of the smooth particle hydrodynamic method for modeling the body of a bird.
To reduce the likelihood of bird collision through implementable tools, three approaches have been employed in practice or investigated in studies: bird habitat correction, bird behavior adjustment, and airplane trajectory correction. Habitat correction could prevent birds from roosting and feeding in undesirable regions, which could be attained by eliminating food resources, enveloping ponds, and eliminating nesting places via trimming vegetation [34]. Hazing approaches like pyrotechnics, long-domain acoustic equipment, and lasers could alert the birds' habits and prevent them from arriving in a danger zone. Besides, varying the aircraft's appearance, like employing colors in its construction, can change the bird's attraction and avoid bird collisions [35,36]. The effectiveness of bird control methods may vary depending on the specific bird species targeted. Therefore, it is essential to integrate various approaches and periodically alternate between them [37,38]. Employing a single approach consistently can lead to a decline in effectiveness over time as birds become accustomed to it. One approach involves using herding drones to disperse birds from specific areas [39]. However, accurate forecasting of bird movement is critical for the successful implementation of this method, as the herding drone needs to be positioned near the birds' movements to scatter them effectively [40].
A summary of the bird migration surveillance and alert systems employed in aviation to alleviate the likelihood of bird collisions through migration was presented in [41]. Avian radars, weather radars, and global positioning systems (GPS) were employed to monitor bird tracks in real time.
By acquiring real-time bird movement data using bird movement forecasting approaches, efficient frameworks can be established for avoiding bird strikes. Reference [20] reports a system involving pilots and controllers, utilizing bird movement estimates to assess flight departure delays. Simulations conducted in this study suggested that this approach could effectively reduce the incidence of bird collisions with aircraft. However, despite the implementation of a simple linear regression equation for predicting bird movements as outlined in [20], the results indicated that this method is insufficient for accurately devising safe flight planning strategies in real-time scenarios.
With a more precise prediction of bird movements, a range of collision-prevention strategies capable of managing non-cooperative aircraft, similar to those, could be employed to reduce bird strikes [42,43,44,45,46]. These deconfliction approaches conceptualize birds as non-cooperative aircraft or obstacles [15].
Trajectory planning (TP) has received significant attention from researchers as a crucial component of robotics. It involves orchestrating the movement of an object through an environment with obstacles, adhering to specific principles to enable the object to navigate from its initial position to its destination while avoiding collisions with obstacles along its path. With advancements in mobile robot technology, TP plays a pivotal role in enabling robots to autonomously navigate their trajectories. It finds widespread application across various domains, including everyday tasks such as GPS navigation, road planning through geographic information systems (GIS), and network navigation planning for urban roads [47]. Furthermore, TP facilitates the implementation of high-tech endeavors such as autonomous and collision-free actions of robots, obstacle avoidance and penetration flights of unmanned aerial vehicles, and radar searches to evade cruise missiles [48]. TP [49] aims to automatically compute a collision-free and safe trajectory, which can guide an object to its target position while optimizing its path through an obstacle-laden environment. Various TP approaches have been presented in the literature, such as the A * algorithm, RRT algorithm [50], and APF approach [51,52]. Bio-inspired approaches like the ant colony [53] and genetic algorithms [54] have been presented in this area.
TP is categorized into global and local, which can be employed for known and unknown environmental information, respectively [55]. Global TP approaches involve free space, raster, visual map, and A* algorithms [56]. Local PP approaches involve fuzzy logic, APF, and neural network approaches [57]. When compared with the other approaches, the APF approach requires a lower calculational load and is an easier-to-use model with broadly applicable implementations [58,59,60,61]. Nevertheless, since the APF approach can only be utilized for TP by employing the locally available environmental data, it could easily fall into the local minimum (LM). The LM issue occurs when the robot is at an equilibrium point in the environment while the combined force equals zero, thus making the robot unable to move. The target unattainability issue means that the robot could not attain the target destination since the repulsive force is more substantial than the gravitational one around the hurdle or target destination [62]. To resolve the mentioned issues, researchers, at home and abroad, have performed various studies. The LM issue was resolved by developing virtual traction points and raising fast functions to enhance the motion rate of the robot [63]. An APF approach employing expert knowledge was introduced to address the LM issue [60]. To deal with the LM issue, the immune algorithm was integrated with the APF approach [59]. Similarly, the firefly method was integrated with the APF approach to resolve the issue of target unattainability [64]. The LM issue was reduced by establishing virtual traction points, and it was also addressed by creating virtual hurdles near the minimum [65].
The article aims to address the LM and target unattainability issues by implementing modifications to the conventional APF approach, including adjustments to the gravitational and repulsive field functions, and introducing safety length and step size rules. MATLAB R2022b was utilized for simulating the configurations. The simulation results, both before and after the modifications, have been presented, demonstrating the efficient elimination of the LM issue associated with the conventional APF approach. Figure 2 illustrates the steps of the proposed method.
A virtual force field approach, referred to as VFF, was proposed [66], utilizing the APF to guide a robot, such as a rover, by constructing a VFF. For example, it is employed to prevent robotic arms from colliding with a table while grasping objects, proving effective in mobile applications where it can generate smooth trajectories for moving objects.
Rather than relying on graphical representations of planned space, this method utilizes the concept of electromagnetic potential from electromagnetic field theory, considering that different objects in the planned area possess distinct potential fields. Object movement is influenced by two forces: an attractive force pulling the object towards the target destination (TD), and a repulsive force pushing it away from obstacles and threats. The resultant velocity of the object is determined by the combined effect of these forces. However, while the APF approach offers the advantage of rapid planning, it may struggle to effectively address trajectory planning constraints and can encounter local minima (LM).
The fundamental concept behind applying APE to TP in aviation involves creating a conceptual environment around the aircraft, analogous to a gravitational field. Here, both the destination and bird obstacles exert respective "gravitational" and "repulsive" forces on the aircraft as it flies. Therefore, when the TP is executed using the APF approach, it is expected to result in smoother and safer trajectory adjustments.
The commonly utilized APF method is the gradient PFA, wherein the aircraft occupies a specific abstract potential energy within the potential field, and its negative gradient orientation dictates the abstract force's direction. This abstract then forcefully guides the aircraft around bird obstacles towards its intended destination. Figure 3 illustrates the forces acting on the aircraft within the potential field.
Let the aircraft's two-dimensional workspace be represented by W=[x,y]T. The potential field is constructed by applying gravitational and repulsive forces, acting together on the aircraft in the potential field structure. The subscripts o and a describe the hurdle and the TP, respectively, shown in Eq. (1)
U(W)=Ua(W)+Uo(W) | (1) |
where Ua(W) and Uo(W) describe the gravitational and repulsive fields, respectively. Thus, the aircraft's combined force in the APF is described in Eq. (2).
F=Fa+Fo | (2) |
where the gravitational force is denoted by Fa=−grad[Ua(W)] and the repulsive force is represented by Fo=−grad[Uo(W)]. The combined forces on the aircraft in 2D are expressed in Eq. (3).
∇U(W)=[∂U∂x∂U∂y] | (3) |
The TP's gravitational potential function on the aircraft is given in Eq. (4).
Ua(W)=12a(W−Wa)2 | (4) |
where a describes the gravitational gain factor, W describes the aircraft's current coordinates in 2D, Wa indicates the TP location, and (W−Wa) describes the relative length between the aircraft and TP. The corresponding gravitational force can be converted to Fa=−k(W−Wa).
The obstacle's repulsive potential function is described in Eq. (5).
Uo(W)={12β(1α−1α0)2ifα≤α00ifα>α0 | (5) |
where β, α0, and α denote the repulsion gain factors, the constant describing the influence of distance to the bird obstacle, and the shortest distance between the aircraft and the bird obstacle, respectively. The corresponding repulsive force can be described in Eq. (6).
F0=−grad[Uo(W)]={β(1α−1α0)1α2∂α∂Wifα≤α00ifα>α0 | (6) |
where ∂α∂W=[∂α∂x,∂α∂y]T.
As an aircraft faces multiple bird obstacles, the potential field iteration is utilized to find the multiple bird obstacles' RPF against the aircraft. Figure 4 illustrates a potential field superposition (PFS).
Figure 4 depicts the multiple bird obstacles' RPF against the aircraft. The red dotted curve shows the trajectory that the aircraft follows.
a. Establishment of the channel gravitational potential field (GPF)
The traditional APF fails to integrate specific operational parameters into establishing the GPF. In this study, the midpoint of the channel is designated as a line of symmetry, while the center of the aircraft's activity range e0 serves as the safety boundary for the aircraft's route. Thus, the potential field within this range, aligned with the direction of the aircraft's route, is reduced. Therefore, a 2D coordinate system is constructed based on the horizontal and vertical coordinates of the aircraft's movement on either side of the centerline of the channel, with the midpoint of the channel acting as the primary gravitational point. The gravitational force becomes stronger as the distance from the centerline increases. Eq. (7) presents the GPF function.
Umid=a(|X−X0|2+|Y−e0|) | (7) |
where a, X0, and e0 represent the PFE gain coefficient of the channel GPF, the horizontal coordinate of the aircraft that starts, and the maximum steering distance in the longitudinal region, respectively. Figure 5 shows the channel GPF obtained by employing Eq. 7.
b. Design of repulsion potential field for an obstacle
Considering that the obstacle's shape impacts the smoothness and coherence of the trajectory, an elliptical-like shape is adopted to form the RPF, enhancing the trajectory's smoothness and rationality. Assuming that the coordinates of the obstacle are denoted by (X0,Y0), the shape of the RPF is defined as an ellipse-like function given in Eq. (8).
(X−X0)2σ12+(Y−Y0)2σ22=1 | (8) |
The RPF function transforms the elliptic-like boundary into a 3D elliptic-like field expressed by a 2D normal distribution function given in Eq. (9).
Urep(X,Y)=(2πσ1σ2√1−ρ2)−1exp{−12(1−ρ2)[(X−X0)2σ12−2ρ(X−X0)(Y−Y0)σ1σ2+(Y−Y0)2σ22]} | (9) |
Let ρ=0, β=(2πσ1σ2)−1. Now, Eq. (9) is simplified to obtain Eq. (10).
Urep(X,Y)=β{exp(−12[(X−X0)2σ12+(Y−Y0)2σ22])−Up} | (10) |
where β and ρ denote the long gain coefficient of RPE and the correlation between transverse and longitudinal directions, respectively. σ1 and σ2 represent the parameters that control the shape of the avoidance path of the collision.
A very small positive number Up is added to the mathematical expression of the repulsive field to balance it. In the context of this work, the design parameters σ1 and σ2 will be combined to practically implement the process that prevents the aircraft from bird collision.
When evaluating pilots' steering behaviors for crash avoidance, many of the paths for avoiding collisions can be approximated as fifth-degree polynomial curves [67]. Such collision avoidance routes formed by fifth-degree polynomial curves ensure smooth and continuous displacement, velocity, and acceleration curves for the aircraft, fulfilling the necessary criteria. In this study, the RPF model is devised using a fifth-degree polynomial to depict a reference path. The starting position of the collision avoidance region in which the aircraft starts steering is denoted by (X1,Y1). The termination of collision avoidance and return to the main channel position is represented by (X1,Y2). The location of the topmost point is denoted by (X2,Y0). Then, the longitudinal distance traveled by the aircraft when steering to avoid the collision is represented by d=|Y2−Y0|=|Y0−Y1|. The lateral offset distance is represented by b=|X2−X1| to return the aircraft to the main flight path at (X1,Y1), At this time, the RPE field should be zero, which leads to the expression of the parameter σ2.
σ2=√d2/d2[−2In(Up)][−2In(Up)] | (11) |
Based on the condition that the RPE fields are equal at each point on the collision avoidance route, the expression for the parameter σ1 is obtained by
σ1=10bd3(Y−Y0+σ2)3−15bd4(Y−Y0+σ2)4+6bd5(Y−Y0+σ2)5√1−(Y−Y0)2σ22 | (12) |
Figures 6 and 7 show the 3D diagram of the RPF of the elliptical obstacle and the contour map of the potential field obtained from Eq. 10, respectively.
c. Construction of the channel boundary potential field
In addition to the bird threat, the risk of deviating from the trajectory significantly impacts flight safety. The trajectory boundary potential field (BPF) is formulated to prevent aircraft from veering beyond the trajectory boundary and encroaching into the flight path of other aircraft. In a two-way trajectory, the aircraft encounters the highest risk at the extreme left and right sides of the local trajectory, while facing the lowest risk factor when flying near the centerline. To account for varying levels of risk within the channel, the boundary RPF is devised using a function that reflects different degrees of risk. When aircraft on both sides of the channel face elevated risk levels, a rapidly changing trend of the exponential function is employed to establish the BPF. Conversely, when the aircraft is positioned in the middle of the channel, a more gradual trend of change is achieved by employing the triangular function to establish the boundary function. Accordingly, the potential field function of the channel boundary can be expressed in Eq. (13).
Uroad={γ1(e|x−xl|−1)x⩽L/L44γ2[sin(2(x−xl)L)]L/L44<x<3L/3L44γ1(e|x−xr|−1)x⩾3L/3L44 | (13) |
Figure 8 presents the RPF's 3D diagram at the channel boundary determined by Eq. 11.
d. Velocity repulsion potential energy field
The higher the aircraft's speed towards the bird obstacle, the greater the repulsive force produced by the bird obstacle on the aircraft. The velocity of the RPE field is shown in Figure 9.
e. The total potential energy field (PEF)
Figure 10 shows the superposition of the repulsion fields of all obstacles.
The whole PEF is the summation of the obstacle repulsion field, TP gravitational field, course boundary repulsion field, and velocity repulsion field, as shown in Figure 11.
The resultant trajectory with the lowest potential energy field (PEF), referred to as the PEF-TP result, is depicted in Figure 12.
The objective of morphological open and closed operations is to refine the optimal trajectory, ensuring its compliance with the radius constraint. Specific open and closed smoothing operations are employed to guarantee that both left and right turns adhere to the radius constraint.
a. Open and closed operations
When image processing is carried out, various combinations of dilation and erosion are utilized to acquire better images. Typically, an image undergoes a sequence of dilation or erosion procedures using either the same or different structural elements, wherein open and closed operations represent the two most common combinations of dilation and erosion.
The morphological opening operation of A by B is described as A∘B, and this operation is the result of the erosion of A by B followed by the dilation of the erosion by B: A∘B=(A⊖B)♁B.
Another expression for the open operation is A∘B=∪{(B)z|(B)z⊆A}.
Where U{⋅} refers to the union of all sets in the curly brackets, and the symbol C⊆D indicates that C is a subset of D. A simple geometric description of the formula is that A∘B indicates the concatenation of identically matched translations of B within A.
Figure 13 describes the mentioned interpretation. Figure 13(a) describes the set A and the disc-shaped structural element B.
Figure 13(b) presents some translations of B that match exactly within A. The merger of these translations is represented by the shaded area in Figure 13(c), resulting from the open operation. The white region denotes areas in A where the structural elements do not match precisely, thereby not contributing to the results of the open operation. The morphological open operation serves to remove object regions incapable of enclosing structural elements, refine the object's contours, sever narrow connections, and eliminate minor protrusions.
The morphological closure of A by B is described by A⋅B, which results from dilation followed by erosion A⋅B=(A♁B)⊖B.
Geometrically speaking, A⋅B describes the union of all translations of B without overlapping with A. Figure 13(d) shows some of the translations of B without any overlap of A. By performing the mentioned translation-merge operation, the shaded area presented in Figure 13(e) can be obtained, resulting from the closure operation. Like the open operation, the morphological closure operation smoothens the object's contours. However, unlike the open operation, the closed operation usually joins narrow gaps to construct elongated curved openings and fills holes smaller than the structural elements.
The imopen and imclose functions are utilized to implement the open and close operations. The two functions' syntax takes the form C = imopen (A, B) and C = imclose (A, B), where A describes a binary image, and B represents a matrix with element values of 0 and 1 that describes the structure elements. strel object SE could be utilized instead of B.
b. Illustration of the smoothing case of the morphological open operator
The morphological open operation principle involves sliding the structural element beneath the curve to be smoothed, progressing from left to right, thereby tracing the outer contour of the circle as the open operation progresses. The resulting trajectory represents the smoothed curve following the completion of the open operation, illustrated in Figure 14.
c. Illustration of the smoothing case of the morphological closed operator
The principle underlying the morphological closed operation involves rolling the structural element across the curve to be smoothed, moving from left to right. This process delineates the outer contour of the circle as the closed operation progresses. The resulting trajectory depicts the curve smoothed by the closed operation, as depicted in Figure 15.
The study has certain limitations. First, a comprehensive simulation study must be conducted extensively on various types of aircraft. Second, the use of a 2D normal distribution function to represent a 3D elliptical-like field necessitates validation through additional data analytics to determine its generalizability. Third, further research is required to validate the effectiveness of APE on aircraft, given that it is typically applied to slow-speed mobile robots.
Given the substantial speed disparity between birds and aircraft, with aircraft flying at 10 times the speed of birds, the speed of birds is considered negligible in comparison to fast-flying aircraft. Thus, the likelihood of birds altering their trajectory within a short timeframe is minimal when compared to aircraft. As a result, the altitude of the birds remains constant throughout the simulation process.
On the contrary, the disparity in size among different parts of the aircraft ranges only from a few meters to several tens of meters, whereas the distance between birds and aircraft, necessary to avoid collisions, typically spans several hundred meters or even several thousand meters for trajectory planning. The positional deviation of various aircraft components from the center of mass of the aircraft fuselage is inconsequential for birds. Therefore, the study primarily focuses on evading birds and swiftly maintaining trajectory planning, rather than specifically targeting any particular aircraft component. Therefore, the position of the aircraft fuselage's center of mass is treated as a point mass, with calculations based on the scores obtained by treating both aircraft and birds as point masses. This approach allows birds to be treated as points during simulation due to their small size. Subsequently, bird collision avoidance and trajectory planning are executed based on the relative positions of aircraft and birds.
An aircraft's trajectory planning to avoid bird collisions typically does not require pre-planning, as altitude adjustments can be made directly in three-dimensional space. However, in practice, aircraft altitude adjustments occur gradually and cannot be quickly raised to higher levels. For instance, it may take up to half an hour for an aircraft to transition from takeoff to cruising altitude. In addition, both ascent and descent times are relatively slow. Thus, there is insufficient time to avoid bird collisions and devise an alternate trajectory in a short timeframe. Therefore, the adjustment of aircraft altitude within a short period can be disregarded.
a. Definition of scenarios
Figure 16 shows the designed scenario.
Through a comprehensive review of literature and real-time cases, the parameters of the configured scenario constitute a fundamental procedure for overall planning. The control parameters for the APF include the gravitational coefficient set at 2000 to adjust the distance weight, and the repulsive coefficient set at 100 to adjust the safe distance weight. The starting position coordinates of the aircraft are (0, 0) km, while the target position coordinates are (10, 10) km. The radius of influence of the bird obstacle is established at 2 km. The flight speed of the aircraft is set to 720 km/h, with a mass of 20,000 kg. Furthermore, the turning radius limit of the aircraft is defined as 1.5 km.
The number of birds in the bird parameter configuration is set to 22, i.e., 22 obstacles and their respective locations in a coordinate position, and the unit grid length in the morphological parameter is set to 0.02 km. In the event of a change in the obstacle's position, it becomes essential to adjust the gravitational and repulsive coefficients of the artificial PEF accordingly to ensure its alignment with the target point.
b. The results of the APF plans
Figures 17, 18, and 19 depict the potential energy distribution results for the provided flight scenario.
The aircraft's starting point is set at coordinates (0, 0), while the target location coordinates are represented by a very dark blue region on the right side, corresponding to (10, 10). The intensity of the blue shading indicates the strength of the gravitational force, with deeper shades indicating greater force. Each group of birds is abstracted into an obstacle, with a relatively large number of birds forming a noticeable peak. The intensity of the yellow shading on the top of these peaks signifies the strength of the repulsive force, with a deeper shade of yellow indicating stronger repulsion. The proposed algorithm assists the aircraft in determining an optimal path to circumvent obstacles and reach its destination efficiently. By utilizing this algorithm, the aircraft not only avoids obstacles effectively but also endeavors to reach its destination via the shortest route possible. Hence, the requirements of the artificial PEF aid aircraft in achieving the shortest path while avoiding obstacles along the way.
c. Smoothing of morphological opening and closing operations
The cell grid's length is configured to be 0.02 km. The original scene, depicted as a gridded image, is illustrated in Figures 20 and 21.
Figure 20 describes the discretization of the trajectory. Figure 21 shows the morphological operational structure element where the radius of the structural element is defined as 1.5 km and 1.5/0.02 = 75, so the radius length of the discretized structure element includes 75 meshes and the diameter length of its structure element includes 150 meshes.
d. Final trajectory planning
Figure 22(a) illustrates the process of determining the optimal trajectory for the aircraft within the specified configuration, aiming to navigate the aircraft to avoid obstacles efficiently while reaching the endpoint via the shortest possible distance, which constitutes the primary objective of this work. The efficacy of the artificially constructed PEF is demonstrated. In Figure 22(b), a partially enlarged schematic representation of Figure 22(a) is provided.
The fundamental idea behind employing APE in aviation TP is to conceptualize the aircraft's surroundings as an abstract gravitational field, where the destination and bird obstacles exert opposing "gravitational" and "repulsive" forces on the aircraft in flight. Ultimately, TP using the APF approach is anticipated to yield trajectories that are smoother and more secure.
In this work, three energy fields are delineated: the bird obstacle potential, the target gravitational potential, and the other obstacle potential. By summing up the potential energy of individual elements, the total potential energy field (PEF) is superimposed to identify the path with the minimum PEF through APF planning. Subsequently, the planned APF is fine-tuned by adjusting the gravitational coefficient. A higher gravitational coefficient ensures a safer TP. Similarly, the repulsive coefficient is determined, with higher values leading to more optimal distances for achieving the desired trajectory. This adjustment facilitates the attainment of a safer trajectory for aircraft. However, since the APF cannot integrate the flight radius trajectory constraint, it may not always comply with the flight radius constraint. To address this limitation, the manuscript introduces morphological filtering to smooth the trajectory, ensuring the final trajectory adheres to the flight radius constraint.
The RPF function transforms the elliptic-like boundary into a 3D elliptic-like field expressed by a 2D normal distribution function whose design parameters, σ1 and σ2, are used to practically implement the process that prevents aircraft from bird collisions. To address the potential risk of deviating from the trajectory, which poses a threat to flight safety, the flight trajectory BPF is developed. This BPF serves to prevent aircraft from veering off the trajectory boundary and inadvertently entering the path of other aircraft. Morphological open and closed operations are also employed to further refine the optimal trajectory, ensuring adherence to the radius constraint. These distinct smoothing operations, both open and closed, are applied to guarantee that both left and right turns comply with the radius constraint. Furthermore, the morphological open operation serves to eliminate regions that cannot accommodate structural elements, smoothen the contours of the object, sever narrow connections, and eliminate minor protrusions. In conclusion, the study integrates artificial potential energy (APE) and morphological smoothing to adjust the trajectory while considering the flight radius constraint, with a particular focus on leveraging the morphological open operation.
To attain better outcomes pertaining to the conducted research, a) a comprehensive simulation study is required, b) data-based probability distributions should be explored for implementing 3D elliptic-like field functions, and c) further research is needed to verify the effectiveness of APE on aircraft since it is generally applied to slow-speed mobile robots.
This work introduces a solution methodology to address the challenges posed by the local minimum (LM) and target unattainability issues within the conventional APF approach. This is achieved by refining the gravitational and repulsive field functions and establishing safety length and step size rules. To reduce wildlife strike incidents, the study integrates APE and morphological smoothing techniques to adjust the trajectory. Therefore, this combined approach not only minimizes the adverse effects of bird collisions but also ensures trajectory maintenance within a shorter timeframe.
The proposed approach combines a method from mobile robot technology, namely APF (artificial potential fields), which are used to navigate paths while avoiding obstacles, with morphological smoothing techniques. This integrated approach is aimed at reducing the susceptibility of aircraft to bird collisions during trajectory planning.
A combination of APE and morphological smoothing techniques is thus employed to adjust the trajectory while adhering to the flight radius constraint. Furthermore, a flight trajectory boundary potential field (BPF) is established to prevent aircraft from deviating beyond the trajectory boundary and potentially intersecting with the paths of other aircraft. Therefore, this approach aims to achieve an optimal trajectory distance, resulting in smoother and more secure trajectory planning.
The research contributes to the prevention of wildlife strikes in the following ways:
1. Development of bird obstacle potential energy fields, target gravitational potential energy fields, and other obstacle potential energy fields. By considering the potential energy of individual elements and superimposing them to achieve the total PEF, the path with the minimum PEF is determined through APF planning.
2. Adjustment of the planned APF by modifying the gravitational coefficient (where higher values indicate increased safety) and the repulsive coefficient (where higher values indicate optimal distance). This adjustment aims to achieve the optimum trajectory considering both aircraft safety and route distance.
3. Introduction of morphological filtering to address the inability of APF to incorporate flight radius constraints. This filtering smoothens trajectories, ensuring the final trajectory complies with the flight radius constraint.
Future research must be directed to explore alternative empirical probability distributions derived from real datasets to enhance the data-driven nature of solutions.
This work was supported by the National Natural Science Foundation of China (52202442).
The authors declare they have not used Artificial Intelligence (AI) tools in the creation of this article.
The authors declare no conflict of interest.
[1] | Serious accident database. Database of fatalities and destroyed aircraft due to bird and other wildlife strikes, 1912 to present. Available from: https://avisure.com/serious-accident-database/. |
[2] | Skybrary. Bird Strike (2019) Available from: https://www.skybrary.aero/articles/bird-strike. |
[3] | Dolbeer RA (2013) The history of wildlife strikes and management at airports. In USDA National Wildlife Research Center-Staff Publications; Johns Hopkins University Press: Baltimore. |
[4] | Cleary EC, Dolbeer RA (2005) Wildlife hazard management at airports: a manual for airport personnel. In USDA National Wildlife Research Center-Staff Publications; Johns Hopkins University Press: Baltimore, 133. |
[5] | Simplifying. What Happened To The Airbus A320 That Landed On The Hudson? 2022. Available from: https://simpleflying.com/miracle-on-the-hudson-aicraft-fate/. |
[6] | News WA, Sita Air Dornier 228 Crashes in Nepal, 19 killed, 2012. Available from: https://worldairlinenews.com/2012/09/29/sita-air-dornier-228-crashes-in-nepal-19-killed/. |
[7] | Herald, A. Accident: Ural A321 at Moscow on Aug 15th, 2019, Bird Strike into Both Engines Forces Landing in Cornfield, 2019. Available from: http://avherald.com/h?article=4cb94927&opt=0. |
[8] | Allan JR (2000) The costs of bird strikes and bird strike prevention. In Human conflicts with wildlife: economic considerations; Johns Hopkins University Press: Baltimore, 18. |
[9] | Dolbeer RA, Begier MJ, Miller PR, et al. (2022) Wildlife Strikes to Civil Aircraft in the United States, 1990–2022. Tech. rep. United States. Department of Transportation. Federal Aviation Administration. |
[10] | UK Civil Aviation Authority. CAA Paper 2006/05: The Completeness and Accuracy of Birdstrike Reporting in the UK; CAA Report; UK Civil Aviation Authority: London, UK, 2006. |
[11] | Dolbeer RA. Trends in Reporting of Wildlife Strikes with Civil Aircraft and in Identification of Species Struck Under a Primarily Voluntary Reporting System, 1990–2013; Special Report Submitted to the Federal Aviation Administration; DigitalCommons@University of Nebraska–Lincoln: Lincoln, NE, USA, 2015. Available from: https://digitalcommons.unl.edu/cgi/viewcontent.cgi?article=1190&context=zoonoticspu. |
[12] | Dolbeer RA, Weller JR, Anderson AL, et al. Wildlife Strikes to Civil Aircraft in the United States 1990–2015; Federal Aviation Administration National Wildlife Strike Database, Serial Report Number 22; Federal Aviation Administration, U.S. Department of Agriculture: Washington, DC, USA, 2016. |
[13] | Pitlik TJ, Washburn BE (2012) Using bird strike information to direct effective management actions within airport environments. In Proceedings of the 25th Vertebrate Pest Conference, Monterey, CA, USA, 5–8. |
[14] | Systems RR, Three Reasons Why Bird Strikes on Aircraft Are on the Rise, 2021. Available from: https://www.robinradar.com/press/blog/3-reasons-why-bird-strikes-on-aircraft-are-on-the-rise. |
[15] | Sabziyan Varnousfaderani E, Shihab SAM (2023) Bird Movement Prediction Using Long Short-Term Memory Networks to Prevent Bird Strikes with Low Altitude Aircraft. AIAA AVIATION 2023 Forum, San Diego, California, USA, 4531. https://doi.org/10.2514/6.2023-4531 |
[16] | McKee J, Shaw P, Dekker A, et al. (2016) Approaches to wildlife management in aviation. In Angelici, M.F., Eds; Problematic Wildlife, Springer: Cham, Switzerland, 465–488. https://doi.org/10.1007/978-3-319-22246-2_22 |
[17] | Dolbeer RA (2011) Increasing trend of damaging bird strikes with aircraft outside the airport boundary: implications for mitigation measures. Hum-Wildl Interact 5: 235–248. |
[18] |
Sabziyan Varnousfaderani E, Shihab SAM, Dulia EF (2023) Deep-Dispatch: A Deep Reinforcement Learning-Based Vehicle Dispatch Algorithm for Advanced Air Mobility. J Air Transp 2024: 1–22. https://doi.org/10.2514/1.D0416 doi: 10.2514/1.D0416
![]() |
[19] | Avrenli KA, Dempsey BJ (2014) Statistical analysis of aircraft–bird strikes resulting in engine failure. Transp Res Rec 2449: 14–23. |
[20] |
Metz IC, Ellerbroek J, Mühlhausen T, et al. (2021) Analysis of Risk-Based Operational Bird Strike Prevention. Aerospace 8: 32. https://doi.org/10.3390/aerospace8020032 doi: 10.3390/aerospace8020032
![]() |
[21] |
Filiz E, Öner G, Ahmet U, et al. (2023) An investigation of bird strike cases in the aviation sector with a novel approach within the context of the principal-agent phenomenon: Bird strikes and insurance in the USA. Heliyon 9. https://doi.org/10.1016/j.heliyon.2023.e18115 doi: 10.1016/j.heliyon.2023.e18115
![]() |
[22] |
Zhou Y, Sun Y, Cai W (2019) Bird-striking damage of rotating laminates using SPH-CDM method. Aerosp Sci Technol 84: 265–272. https://doi.org/10.1016/j.ast.2018.10.009. doi: 10.1016/j.ast.2018.10.009
![]() |
[23] |
Zhou Y, Sun Y, Huang T, et al. (2019) SPH-FEM simulation of impacted composite laminates with different layups. Aerosp Sci Technol 95: 105469. https://doi.org/10.1016/j.ast.2019.105469 doi: 10.1016/j.ast.2019.105469
![]() |
[24] |
Metz IC, Mühlhausen T, Ellerbroek J, et al. (2018) Simulation Model to Calculate Bird-Aircraft Collisions and Near Misses in the Airport Vicinity. Aerospace 5: 112. https://doi.org/10.3390/aerospace5040112 doi: 10.3390/aerospace5040112
![]() |
[25] | Metz IC (2021) Air Traffic Control Advisory System for the Prevention of Bird Strikes. Level of Thesis, Delft University of Technology, Delft, The Netherlands. |
[26] |
Metz IC, Ellerbroek J, Mühlhausen T, et al. (2020) The bird strike challenge. Aerospace 7: 26. https://doi.org/10.4233/uuid:013fe685-755f-4a76-8428-53be5c67fa51 doi: 10.4233/uuid:013fe685-755f-4a76-8428-53be5c67fa51
![]() |
[27] |
Nicole LM, Keith AH, Christy AM, et al. (2021) Climate variability has idiosyncratic impacts on North American aerial insectivorous bird population trajectories. Biol Conserv 263: 109329. https://doi.org/10.1016/j.biocon.2021.109329. doi: 10.1016/j.biocon.2021.109329
![]() |
[28] |
Lopez-Lago M, Casado R, Bermudez A, et al. (2017) A predictive model for risk assessment on imminent bird strikes on airport areas. Aerosp Sci Technol 62: 19–30. https://doi.org/10.1016/j.ast.2016.11.020 doi: 10.1016/j.ast.2016.11.020
![]() |
[29] | ABC'S Bird Library, Bird Calls Blog. Available from: https://abcbirds.org/blog/frequent-colliders/. |
[30] | Mathaiyan V, Vijayanandh R, Jung DW (2021) Determination of Strong Factor in Bird Strike Analysis using Taguchis method for Aircraft Manufacturing guide. J Phys Conf Ser 1733: 012002. |
[31] | Shao Q, Zhou Y, Zhu P, et al. (2020) Key factors assessment on bird strike density distribution in airport habitats: Spatial heterogeneity and geographically weighted regression model. Sustainability 12: 7235. |
[32] | Smojver I, Ivančević D (2011) Bird strike damage analysis in aircraft structures using Abaqus/Explicit and coupled Eulerian-Lagrangian approach. Compos Sci Technol 71: 489–498. |
[33] | Riccio A, Cristiano R, Saputo S (2016) A brief introduction to the bird strike numerical simulation. Am J Eng Applied Sci 9: 946–950. |
[34] | Company TDB, Airport Bird Control Drone, 2020. Availabl from: https://www.thedronebird.com/safe-humane-and-effective-bird-control/applications/airports/. |
[35] | Bishop J, McKay H, Parrott D, et al. (2003) Review of international research literature regarding the effectiveness of auditory bird scaring techniques and potential alternatives. Food Rural Affairs, London, 1–53. |
[36] | Seamans TW, Gosser AL (2016) Bird dispersal techniques. Wildlife Damage Management Technical Series. |
[37] | Matyjasiak P (2008) Methods of bird control at airports, In Theoretical and applied aspects of modern ecology. J. Uchmański (ed.), Cardinal Stefan Wyszyński University Press, Warsaw, 171–203. |
[38] |
Blackwell BF, Bernhardt GE (2004) Efficacy of aircraft landing lights in stimulating avoidance behavior in birds. J Wildlife Manage 68: 725–732. https://doi.org/10.2193/0022-541X doi: 10.2193/0022-541X
![]() |
[39] | Vas E, Lescroe¨l A, Duriez O, et al. (2015) Approaching birds with drones: first experiments and ethical guidelines. Biol Lett 11: 20140754. http://dx.doi.org/10.1098/rsbl.2014.0754 |
[40] |
Paranjape AA, Chung SJ, Kim K, et al. (2018) Robotic herding of a flock of birds using an unmanned aerial vehicle. IEEE Trans Robot 34: 901–915. https://doi.org/10.1109/TRO.2018.2853610 doi: 10.1109/TRO.2018.2853610
![]() |
[41] |
Van Gasteren H, Krijgsveld KL, Klauke N, et al. (2019) Agroecology meets aviation safety: Early warning systems in Europe and the Middle East prevent collisions between birds and aircraft. Ecography 42: 899–911. https://doi.org/10.1111/ecog.04125 doi: 10.1111/ecog.04125
![]() |
[42] |
Zhao P, Erzberger H, Liu Y (2021) Multiple-aircraft-conflict resolution under uncertainties. J Guid Control Dyn 44: 2031–2049. https://doi.org/10.2514/1.G005825 doi: 10.2514/1.G005825
![]() |
[43] | Sislak D, Volf P, Komenda A, et al. (2007) Agent-Based Multi-Layer Collision Avoidance to Unmanned Aerial Vehicles. In 2007 International Conference on Integration of Knowledge Intensive Multi-Agent Systems, Waltham, MA, USA, 365–370. |
[44] | Sislak D, Rehak M, Pechoucek M, et al. (2007) Negotiation-Based Approach to Unmanned Aerial Vehicles. In IEEE Workshop on Distributed Intelligent Systems: Collective Intelligence and Its Applications (DIS'06), Prague, Czech Republic, 279–284. |
[45] |
Fasano G, Accardo D, Moccia A, et al. (2007) Multi-sensor-based fully autonomous non-cooperative collision avoidance system for unmanned air vehicles. J Aerosp Comput Inf Commun, 5: 338–360. https://doi.org/10.2514/1.35145 doi: 10.2514/1.35145
![]() |
[46] | Panchal IM, Riberios M, Armanni S (2022) Urban air traffic management for collision avoidance with noncooperative airspace users, 33rd Congress of the International Council of the Aeronautical Sciences (ICAS 2022), 6801–6818. |
[47] | Liu MH, Yang Y, Yue Q (2016) Auxiliary road planning and design based on city engine and ArcGIS. Stud Surv Mapp Sci 64–67. |
[48] | Duan HB, Shao S, Su BW (2010) New ideas for the development of unmanned combat aircraft control technology based on bionic intelligence. Chin Sci Tech Sci 40: 853–860. |
[49] | Liang XH, Mu YH, Wu BH (2020) Review of related algorithms of path planning. Value Eng 39: 295–299. |
[50] | Dong M, Chen TZ, Yang H (2019) Simulation of unmanned vehicle path planning based on improved RRT algorithm. Comput Simul 36: 96–100. |
[51] | Wang JJ, Chen LS, Sheng M (2020) Research on robot path planning based on improved artificial potential field method. Eng Agric Environ Food 58: 66–70. |
[52] | Yu ZZ, Yan JH, Zhao J (2011) Path planning of mobile robot based on improved artificial potential field method. J Harbin Inst Technol 43: 50–55. |
[53] | Wang GC, Wu GX, Zuo YB (2019) Research on trajectory planning of packaging robot based on improved ant colony algorithm. J Electron Meas Instrum 33: 94–100. |
[54] | Liu LF, Yang XF (2019) Efficient path solving based on a hybrid genetic algorithm. Comput Appl Eng 55: 244–249. |
[55] |
Madridano Á , Al-Kaff A, Martín D, et al. (2021) Trajectory planning for multi-robot systems: Methods and applications, Expert Syst Appl 173: 114660. https://doi.org/10.1016/j.eswa.2021.114660 doi: 10.1016/j.eswa.2021.114660
![]() |
[56] |
Rao J, Xiang C, Xi J, et al. (2023) Path planning for dual UAVs cooperative suspension transport based on artificial potential field-A* algorithm. Knowl-Based Syst 277: 110797. https://doi.org/10.1016/j.knosys.2023.110797 doi: 10.1016/j.knosys.2023.110797
![]() |
[57] |
Liu Y, Chen C, Wang Y, et al. (2024) A fast formation obstacle avoidance algorithm for clustered UAVs based on artificial potential field. Aerosp Sci Technol 147: 108974. https://doi.org/10.1016/j.ast.2024.108974 doi: 10.1016/j.ast.2024.108974
![]() |
[58] | Liang XX, Liu CY, Song XL, et al. (2018) Research on path planning of mobile robots based on improved artificial potential field method. Comput Simul 35: 291–294. |
[59] | Liu F (2008) Robot path planning based on artificial potential field and immune algorithm. Software Guide 7: 51–53. |
[60] | Han W, Sun KB (2018) Intelligent omnidirectional vehicle path planning based on fuzzy artificial potential field method. Comput Appl Eng 54: 105–109. |
[61] |
Wu Z, Dai J, Jiang B, et al. (2023) Robot path planning based on an artificial potential field with deterministic annealing. ISA T 138: 74–87. https://doi.org/10.1016/j.isatra.2023.02.018 doi: 10.1016/j.isatra.2023.02.018
![]() |
[62] |
Mbede J B, Huang X, Wang M, (2000). Fuzzy motion planning among dynamic obstacles using artificial potential fields for robot manipulators. Robot Auton Syst 32: 61–72. https://doi.org/10.1016/S0921-8890(00)00073-7 doi: 10.1016/S0921-8890(00)00073-7
![]() |
[63] | Ren Y, Zhao H (2020) Improved artificial potential field method for robot obstacle avoidance and path planning. Comput Simul 37: 360–364. |
[64] | Li L, Guo Y, Zhang X, et al. (2017) Firefly algorithm combined with artificial potential field method for robot path planning. J Inf Sci Eng 7: 8–10. |
[65] | Liang XX, Liu CY (2018) Research on improving the artificial potential field method for mobile robot path planning. Comput Simul 35: 291–294,361. |
[66] | Khatib O (1986) Real-time obstacle avoidance for manipulators and mobile robots. Int J Rob Re c5: 90–98. |
[67] | Yoshida H, Shinohara S, Nagai M (2008) Lane change steering maneuver using model predictive control theory. Vehicle Syst Dyn 46: 669–681. |