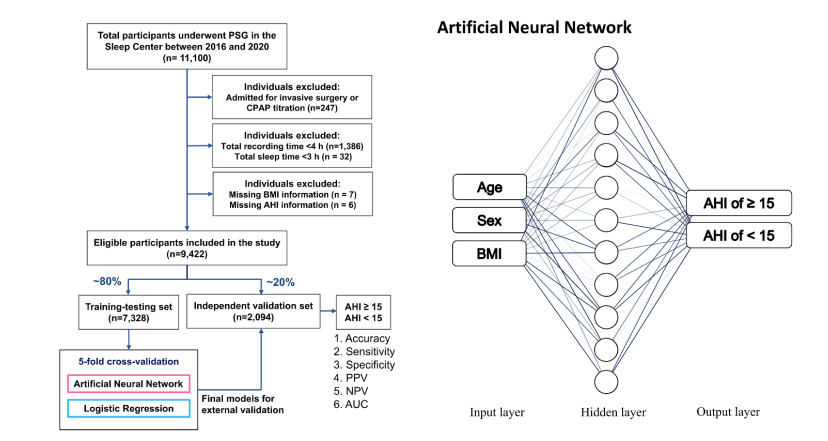
Staphylococcus aureus is one of the leading agents of nosocomial and community-acquired infections. In this study, we explored the genomic characterization of eight methicillin-resistant clinical isolates of S. aureus from Dhaka, Bangladesh. Notably, all strains were resistant to penicillin, cephalosporins, and monobactams, with partial susceptibility to meropenem and complete susceptibility to amikacin, vancomycin, and tigecycline antibiotics. The strains were found to have an average genome size of 2.73 Mbp and an average of 32.64% GC content. Multi-locus sequence typing analysis characterized the most predominant sequence type as ST361, which belongs to the clonal complex CC361. All isolates harbored the mecA gene, often linked to SCCmec_type IV variants. Multidrug resistance was attributed to efflux pumps NorA, NorC, SdrM, and LmrS alongside genes encoding beta-lactamase BlaZ and factors like ErmC and MepA. Additionally, virulence factors including adsA, sdrC, cap8D, harA, esaA, essC, isdB, geh, and lip were commonly identified. Furthermore, genes associated with heme uptake and clumping were present, highlighting their roles in S. aureus colonization and pathogenesis. Nine secondary metabolite biosynthetic gene clusters were found, of which six were common in all the strains. Numerous toxin-antitoxin systems were predicted, with ParE and ParB-like nuclease domains found to be the most prevalent toxin and antitoxin, respectively. Pan-genome analysis revealed 2007 core genes and 229 unique genes in the studied strains. Finally, the phylogenomic analysis showed that most Bangladeshi strains were grouped into two unique clades. This study provides a genomic and comparative insight into the multidrug resistance and pathogenicity of S. aureus strains, which will play a crucial role in the future antibiotic stewardship of Bangladesh.
Citation: Afia Anjum, Jarin Tabassum, Sohidul Islam, A. K. M. Imrul Hassan, Ishrat Jabeen, Sabbir R. Shuvo. Deciphering the genomic character of the multidrug-resistant Staphylococcus aureus from Dhaka, Bangladesh[J]. AIMS Microbiology, 2024, 10(4): 833-858. doi: 10.3934/microbiol.2024036
[1] | Abdulwahab Ali Almazroi . Survival prediction among heart patients using machine learning techniques. Mathematical Biosciences and Engineering, 2022, 19(1): 134-145. doi: 10.3934/mbe.2022007 |
[2] | Weidong Gao, Yibin Xu, Shengshu Li, Yujun Fu, Dongyang Zheng, Yingjia She . Obstructive sleep apnea syndrome detection based on ballistocardiogram via machine learning approach. Mathematical Biosciences and Engineering, 2019, 16(5): 5672-5686. doi: 10.3934/mbe.2019282 |
[3] | Lili Jiang, Sirong Chen, Yuanhui Wu, Da Zhou, Lihua Duan . Prediction of coronary heart disease in gout patients using machine learning models. Mathematical Biosciences and Engineering, 2023, 20(3): 4574-4591. doi: 10.3934/mbe.2023212 |
[4] | Jingchi Jiang, Xuehui Yu, Yi Lin, Yi Guan . PercolationDF: A percolation-based medical diagnosis framework. Mathematical Biosciences and Engineering, 2022, 19(6): 5832-5849. doi: 10.3934/mbe.2022273 |
[5] | Abigail Ferreira, Rui Lapa, Nuno Vale . A retrospective study comparing creatinine clearance estimation using different equations on a population-based cohort. Mathematical Biosciences and Engineering, 2021, 18(5): 5680-5691. doi: 10.3934/mbe.2021287 |
[6] | Atsushi Kawaguchi . Network-based diagnostic probability estimation from resting-state functional magnetic resonance imaging. Mathematical Biosciences and Engineering, 2023, 20(10): 17702-17725. doi: 10.3934/mbe.2023787 |
[7] | Michele L. Joyner, Cammey Cole Manning, Whitney Forbes, Valerie Bobola, William Frazier . Modeling Ertapenem: the impact of body mass index on distribution of the antibiotic in the body. Mathematical Biosciences and Engineering, 2019, 16(2): 713-726. doi: 10.3934/mbe.2019034 |
[8] | Wenzhu Song, Lixia Qiu, Jianbo Qing, Wenqiang Zhi, Zhijian Zha, Xueli Hu, Zhiqi Qin, Hao Gong, Yafeng Li . Using Bayesian network model with MMHC algorithm to detect risk factors for stroke. Mathematical Biosciences and Engineering, 2022, 19(12): 13660-13674. doi: 10.3934/mbe.2022637 |
[9] | Anastasia-Maria Leventi-Peetz, Kai Weber . Probabilistic machine learning for breast cancer classification. Mathematical Biosciences and Engineering, 2023, 20(1): 624-655. doi: 10.3934/mbe.2023029 |
[10] | Tingxi Wen, Hanxiao Wu, Yu Du, Chuanbo Huang . Faster R-CNN with improved anchor box for cell recognition. Mathematical Biosciences and Engineering, 2020, 17(6): 7772-7786. doi: 10.3934/mbe.2020395 |
Staphylococcus aureus is one of the leading agents of nosocomial and community-acquired infections. In this study, we explored the genomic characterization of eight methicillin-resistant clinical isolates of S. aureus from Dhaka, Bangladesh. Notably, all strains were resistant to penicillin, cephalosporins, and monobactams, with partial susceptibility to meropenem and complete susceptibility to amikacin, vancomycin, and tigecycline antibiotics. The strains were found to have an average genome size of 2.73 Mbp and an average of 32.64% GC content. Multi-locus sequence typing analysis characterized the most predominant sequence type as ST361, which belongs to the clonal complex CC361. All isolates harbored the mecA gene, often linked to SCCmec_type IV variants. Multidrug resistance was attributed to efflux pumps NorA, NorC, SdrM, and LmrS alongside genes encoding beta-lactamase BlaZ and factors like ErmC and MepA. Additionally, virulence factors including adsA, sdrC, cap8D, harA, esaA, essC, isdB, geh, and lip were commonly identified. Furthermore, genes associated with heme uptake and clumping were present, highlighting their roles in S. aureus colonization and pathogenesis. Nine secondary metabolite biosynthetic gene clusters were found, of which six were common in all the strains. Numerous toxin-antitoxin systems were predicted, with ParE and ParB-like nuclease domains found to be the most prevalent toxin and antitoxin, respectively. Pan-genome analysis revealed 2007 core genes and 229 unique genes in the studied strains. Finally, the phylogenomic analysis showed that most Bangladeshi strains were grouped into two unique clades. This study provides a genomic and comparative insight into the multidrug resistance and pathogenicity of S. aureus strains, which will play a crucial role in the future antibiotic stewardship of Bangladesh.
Obstructive sleep apnea (OSA) is featured by repeated upper airway narrowing or obstruction, causing a substantial reduction or cessation of airflow during sleep [1,2]. These disruptions in breathing lead to intermittent hypercapnia and hypoxemia, causing metabolic dysregulation, endothelial dysfunction, and systemic inflammatory responses [2,3]. These pathophysiologic changes have contributed to various major comorbidities [2,3,4,5]. OSA is a global health concern and economic burden, with estimates of the incidence of moderate or severe OSA reaching 6%-17% in the general adult population and up to 49% in the elderly population [4,6], with most of the cases being undiagnosed [7].
No single physical examination finding can be used to diagnose or exclusively account for OSA. The gold standard diagnostic test for OSA relies on in-lab all-night polysomnography (PSG) [7,8]. However, this method is costly, and patients require continuous sleep monitoring by medical staff [2]; thus, limited availability of PSG may cause delays in diagnosing OSA and an increased disease burden [7,9].
Several body measurements predispose patients to OSA. Body weight, body mass index (BMI), central body fat distribution, and large neck and waist circumferences are possible risk factors [2,6,10]. In addition, increased age and males are also at risk for OSA [2,6]. Despite the epidemiological reports demonstrating that age, sex, and BMI are associated with OSA severity, an integrated predicting model that uses only these three factors to assess OSA risk has not yet been developed.
Machine learning approaches have expanded the ability to design efficient predictive and diagnostic tools and have gained popularity in many areas of medical research and clinical applications [11,12]. Logistic regression (LR) is one of the computational methods for probing multivariate data to detect the independent effects of a variable after adjusting for the contributions of the others [13]. An artificial neural network (ANN) is a supervised learning algorithm with advantages in analyzing nonlinear data, enhancing data interpretation, and designing effective predictive and diagnostic models to assist different clinical tasks [11,14].
The present study investigated the clinical predictive validity of age, sex, and BMI for sleep apnea and applied LR and ANN algorithms to develop a risk-predicting model using these three parameters as predictors.
This retrospective observational study used clinical data from the medical records of participants who had undergone PSG at the Sleep Center of Shuang Ho Hospital, Taipei Medical University, Taiwan, between January 2015 and January 2020.
The inclusion criteria for this study were: (1) participants aged≥18 years and (2) who had undergone a full-night PSG. Participants were excluded if (1) they had received any OSA treatments or been admitted to the hospital for any invasive surgery for OSA, (2) they had undergone PSG for continuous positive airway pressure (CPAP) titration, (3) the total recording time was < 4 h [8], and (4) the total sleep time was < 3 h [15].
Before the PSG examination, a baseline screening questionnaire was used to assess each participant's basic information, medication, and surgical history. The participants were measured for height, weight, and BMI (kg/m2) at the time of registration.
All the participants underwent a full-night PSG recorded by qualified technicians. All the sleep signals were obtained using an Embla N7000 Recording System (Medcare Flaga, Reykjavik, Iceland) and Sandman Elite sleep diagnostic software (Natus Neurology Inc., Middleton, WI, USA).
Experienced sleep technologists scored all sleep apnea and hypopnea events following the American Academy of Sleep Medicine 2012 updated guideline [8,16]. Each participant's apnea-hypopnea index (AHI) was calculated according to the total number of apnea and hypopnea events divided by the total sleeping time. Hypopnea was defined as a 30-89% reduction in nasal airflow for at least 10s, with arousal or at least a decrease of 3% oxygen saturation. Apnea was defined as a more than 90% reduction in oral airflow for at least 10s with or without arousal or decreased saturation.
The severity of OSA was classified as none (AHI < 5), mild (AHI = 5-15), moderate (AHI = 15-30), and severe (AHI ≥ 30) [2,8]. To develop the predictive models for the risk of moderate-to-severe OSA, we divided the participants into none-and-mild (AHI < 15) and moderate-to-severe (AHI ≥ 15) OSA groups for further analysis.
Taipei Medical University-Joint Institutional Review Board (TMU-JIRB: N201911007) approved the protocol of this study. Waivers of informed consent were approved by the TMU-JIRB (TMU-JIRB: N201911007) for this retrospective study involving the secondary analysis of existing data.
All analyses were performed using STATISTICA software (TIBCO Software, Tulsa, OK, USA). Continuous data are presented as mean and standard deviation, whereas categorical variables are expressed as frequencies and percentages. We used Fisher's exact test to determine associations between two categorical variables and Pearson's chi-squared (X2) test to determine the statistical significance between the means of two continuous variables in the participants with none-to-mild and moderate-to-severe OSA. The one-way analysis of variance (ANOVA) was used to determine the statistical significance between the means of ≥ 3 independent groups. A two-tailed p-value less than 0.05 was considered statistically significant.
All the LR and ANN models were established and modeled using STATISTICA software. In the models, age and BMI were set as continuous variables, and sex was designated as a categorical variable. For our ANNs, the employed architecture was feed-forward, combined with a back-propagation algorithm with multilayer perceptron (MLP). A bias term was used for each neural layer in the MLP. We set the number of neurons in the hidden layer from 1 to 50. We initially partitioned the eligible PSG data into a training-testing set and an independent validation set, containing ~80% and ~20% of the data, respectively. The data from the training-testing set were used to determine the generalizability of the LR and ANN analyses using five-fold cross-validation (Figure 1A). All participants were stratified by age, sex, BMI, and OSA severity to maintain consistent proportions of demographic data across the training-testing and validation sets. We reported the mean AUCs for the training and testing sets of the LR and ANN models through five-fold cross-validation. The model with the best AUC in the five testing sets was identified to have the best predictive performance and used as the final model. The final models were then applied to the independent validation set, and we calculated the accuracy, sensitivity, specificity, positive predictive value (PPV), negative predictive value (NPV), and AUCs of the validation set derived from the confusion matrix [17] (Figure 1A). The classification threshold was set to 0.5 for all models.
During the study period, 11, 100 participants underwent PSG in the Sleep Center. Of these, 1, 665 participants were excluded from the baseline analyses for the following reasons: they had been admitted to the hospital for invasive surgery or CPAP titration (n = 247), the total recording time < 4h (n = 1, 386), the total sleep time < 3h (n = 32), missing BMI information (n = 7) or missing AHI information (n = 6) (Figure 1A). Overall, 9, 422 participants (2918 women and 6504 men, mean age: 49.2 ± 14.0 years) who had undergone PSG were enrolled in this study. Of these, 5, 920 (62.8%) had an AHI of ≥15, indicating moderate-to-severe OSA, and 3, 502 (37.2%) had an AHI < 15, indicating none-to-mild OSA.
A higher proportion of men have moderate-to-severe OSA than women (Table 1). Compared with participants with none-to-mild OSA, participants with moderate-to-severe OSA were older and had a higher BMI. In addition, participants with moderate-to-severe OSA had a shorter total sleep time, lower sleep efficiency, shorter rapid eye movement duration, and lower oxygen saturation during the PSG recording than those with none-to-mild OSA (Table 1).
Variables | Moderate-to-severe OSA (AHI ≥ 15) |
None-to-mild OSA (AHI < 15) |
p-value |
n | 5, 920 | 3, 502 | |
Age | 50.8 ± 13.5 | 46.6 ± 14.4 | < 0.0001 |
Female, n (%) | 1266 (21.4) | 1652 (47.2) | < 0.0001 |
Height (cm) | 167.2 ± 8.5 | 164.4 ± 8.9 | < 0.0001 |
Weight (kg) | 79.7±16.1 | 66.1 ± 13.1 | < 0.0001 |
BMI (kg/m2) | 28.4 ± 4.8 | 24.3 ± 3.8 | < 0.0001 |
Polysomnography | |||
Total recording time (min) | 366.6±11.9 | 367.8±13.4 | < 0.0001 |
Total sleep time (min) | 275.8±60.2 | 281.4±61.1 | < 0.0001 |
Sleep efficiency (%) | 75.2±16.4 | 76.5±16.5 | 0.0002 |
Non-REM sleep (min) | 240.6±51.7 | 242.2±51.3 | 0.133 |
REM sleep (min) | 35.2±22.5 | 39.1±23.7 | < 0.0001 |
AHI | 44.7±23.8 | 6.4±4.5 | < 0.0001 |
SO2 mean (%) | 94.5±2.6 | 96.6±1.3 | < 0.0001 |
Data are expressed as mean ± standard deviation. The p-value represents the comparison between the AHI ≥ 15 and AHI < 15 groups. |
A multivariate analysis was applied to adjust for age, sex, and BMI to determine the independent predictors of moderate-to-severe OSA. Table 2 presents the variables' crude and adjusted odds ratio (OR) values in the LR model. After adjustment for the associated variables, the regression analysis revealed that the female sex was associated with a 0.28-fold reduced risk of moderate-to-severe OSA (95% CI: 0.25-0.32; p < 0.0001), and every unit increase in age or BMI was associated with a 1.04-fold (95%CI: 1.04-1.05; p < 0.0001) or 1.27-fold (95%CI: 1.26-1.29; p < 0.0001) increase in the likelihood of moderate-to-severe OSA, respectively.
Unadjusted Model | Adjusted Model | |||
Variables | OR | 95% CI | OR | 95% CI |
Age | 1.02*† | 1.02-1.03 | 1.04*† | 1.04-1.05 |
Female | 0.30* | 0.28-0.33 | 0.28* | 0.25-0.32 |
BMI | 1.27*† | 1.25-1.28 | 1.27*† | 1.26-1.29 |
The odds ratio (OR) and 95% confidence intervals (CI) represent the association between the variables and the presence of moderate-to-severe OSA (AHI ≥ 15). *p < 0.0001; †Odds ratio of per unit changes. |
As mentioned, age, sex, and BMI were used as input parameters for developing the LR and ANN models for predicting moderate-to-severe OSA. As shown in Figure 1A, the 7328 participants in the training-testing group were randomly partitioned into training and testing sets for five-fold cross-validation. Table 3 showed that age, sex, BMI, and OSA severity distribution remained identical across the five testing sets and the independent validation sets.
Variable | Testing set 1 | Testing set 2 | Testing set 3 | Testing set 4 | Testing set 5 | Independent validation set | p-value |
n | 1466 | 1465 | 1466 | 1465 | 1466 | 2094 | |
AHI≥15, n (%) | 920 (62.8) | 919 (62.7) | 920 (62.8) | 919 (62.7) | 920 (62.8) | 1322 (63.1) | 1.000 |
Age | 49.0 ± 0.4 | 49.0 ± 0.4 | 49.9 ± 0.4 | 49.1 ± 0.4 | 49.0 ± 0.4 | 49.3 ± 0.3 | 0.503 |
Female, n (%) | 483 (33.0) | 438 (29.9) | 472 (32.2) | 445 (30.4) | 431 (29.4) | 649 (31.0) | 0.266 |
BMI (kg/m2) | 26.9 ± 4.9 | 26.9 ± 4.9 | 26.9 ± 5.0 | 26.9 ± 5.0 | 26.8 ± 4.8 | 26.9 ± 4.9 | 0.973 |
The p-value represents the multiple comparisons across the five testing and the independent validation sets. |
Following adequate training, the five testing sets that achieved the best prediction performance after five-fold cross-validation were ANN models with 3, 14, 25, 27, and 29 hidden neurons, respectively. The mean AUCs of the training sets were 0.808 ± 0.004 (Figure 2A) and 0.808 ± 0.015 for the testing sets (Figure 2B). Among the generated ANN models, the model containing 25 hidden neurons obtained the highest testing AUC and was selected as the final model. After being applied to the independent validation set, the ANN-based model achieved the accuracy, sensitivity, specificity, PPV, and NPV of 76.4%, 87.7%, 56.9%, 77.7%, 73.0%, with the AUC of 0.807, respectively. Results of the LR model revealed a mean AUC of 0.802 ± 0.004 for the training sets (Figure 2C) and 0.801 ± 0.015 for the testing sets (Figure 2D). After being validated to the independent validation set, the accuracy, sensitivity, specificity, PPV, and NPV were 76.3%, 87.5%, 57.0%, 77.7%, and 72. 7% with an AUC of 0.806 for the LR-based model. Figure 3 shows the ROC curve and AUC of the final ANN and LR models applied to the independent validation set.
The present study validated age, sex, and BMI as parameters for use in LR- and ANN models to estimate the risk of OSA in participants who had undergone PSG. The established LR- and ANN-based model integrating the three parameters achieved a notable prediction performance with an AUC of 0.806 and 0.807 for predicting moderate-to-severe OSA in the validation set. The relatively high AUC values indicated that the models could distinguish participants between groups of interest, namely, participants with none-to-mild and those with moderate-to-severe OSA. Patients with OSA are prone to various complications and require interventions [2,7]; our models may contribute to further understanding these three variables' fundamental role in assessing OSA risk.
OSA has been recognized as a global health and economic burden, and early identification and diagnosis of OSA is a crucial issue in preventive medicine [4,6,7]. Since standard diagnostic tests for OSA rely on expensive in-laboratory PSG, which can be challenging to schedule in high-demand situations, identifying crucial factors and developing a simple and reliable tool to estimate the risk of OSA is clinically relevant [7,9].
Anatomical and physiological differences in the oropharynx, larynx and upper respiratory tract, changes in respiratory physiology associated with different sleep structures, and differences in the distribution of adipose tissue have been suggested as reasons for sex differences in developing OSA [18]. Deterioration of the collapsibility of the pharyngeal airway and the sensitivity of the respiratory control system may contribute to the pathogenesis of OSA with aging [2,18]. Hormonal changes in menopausal women have been linked to an increased risk of OSA and a decreased sex difference in OSA with age [19,20]. The prevalence of OSA has been reported to increase 1.4 to 3.2-fold with each 10-year increment in age, and men had a 1.7 to 3.0-fold higher odds of developing OSA than women [2,6]. These findings support the rationale for using age and sex as parameters in a generic prediction model to predict OSA risk.
Obesity is a well-recognized risk factor for developing OSA, and a higher BMI is associated with greater severity of OSA for both sexes [19,21]. As already mentioned, anthropometric profiles, such as body weight, BMI, central body fat distribution, and neck and waist circumference, are predisposed to the risk of OSA [2,6,10]. In patients with OSA, occlusion of the nasal and oro-pharynx may occur during sleep when the tongue and epiglottis move backward and lean together. Individuals with obesity have excess soft tissue around the collapsible pharynx, making the narrow airway more prone to collapse [2,3,21]. In line with the findings of other studies, we observed that the participants with moderate-to-severe OSA had a higher BMI. Although other anthropometric profiles have been associated with OSA, neck and waist circumference were considered surrogates for BMI [4,21,22]. The study by Huxley et al. has also suggested that "measures of central obesity were superior to BMI as discriminators of cardiovascular risk factors, although the differences were small and unlikely to be of clinical relevance" [23]. Furthermore, the same study demonstrated that "combining BMI with any measure of central obesity did not improve the discriminatory capability of the individual measures" [23]. In this study, we proposed BMI as a simple, well-known, and readily available predictor of OSA for the prediction models.
Despite the epidemiological reports indicating that age, sex, and BMI are associated with OSA, an integrated predicting model using only these three attributes to assess OSA risk has not yet been developed. Advances in machine learning techniques have improved the ability of researchers and clinical applications to analyze massive amounts of data and develop appropriate diagnostic and predictive methods [24,25,26,27]. Several studies have applied machine learning algorithms to predict OSA risk. However, most of these studies have incorporated more complex parameters, such as sleep questionnaires [28,29,30], at-home PSG [15], cephalometric images [31], neck and waist circumference measurements [28,29,32,33], and facial-cervical measurements [28,32], into the predictive models.
In this context, our study used a large hospital-based sample of adults, allowing accurate comparison between none-to-mild and moderate-to-severe cases. The generated LR- and ANN-boosted models exhibited relatively high AUCs with adequate accuracy, sensitivity, PPV, and NPV, revealing the ability of these models to distinguish between the groups of interest, namely individuals with moderate-to-severe and none-to-mild OSA. Furthermore, our models used simple and easy-to-obtain parameters (age, sex, and BMI), which may provide a direction for developing predictive models under conditions of limited and constrained information.
Both LR and ANN are widely used models in biomedicine. This study's LR and ANN models achieved similar predictive validity in predicting OSA in patients undergoing PSG, which may be related to our data structure. ANN has the advantage of analyzing complex, multidimensional and nonlinear variables [34,35,36,37]. When the predictor-outcome relationships in the dataset are relatively simple and linear, LR using linear combinations of variables may achieve performance that approximates that of ANN [36,38]. This consideration was contextualized in the conclusion by Dreiseitl and Ohno-Machado [38] that "there is no single algorithm that performs better than all other algorithms on any given data set and application area." and also summarized by Sargent [34] that "both methods should continue to be used and explored in a complementary manner."
The present study has several limitations. First, in our cohort, the incidence of moderate-to-severe OSA was 62.8%, which is higher than the reported prevalence of OSA in the general population [4,19,20,39]. In clinical practice, patients referred for a PSG exam are usually expected to have OSA or another sleep disorder; therefore, the participant population in this study cannot be representative of the general population with a very low prevalence of OSA. Second, the data set used in this study was limited to a single center and included only Asian ethnic groups. Therefore, the trained model may not be generalizable beyond the institution or other ethnic groups [28,29]. Third, this retrospective observational study did not exclude undiagnosed medical conditions associated with OSA, such as neurological, cardiovascular, and pulmonary disorders, which may affect the model's predictive performance. Our model did not contain detailed anthropometric imaging or measurements, which may limit the model's ability to distinguish the disease-specific causes of OSA. Finally, the relatively low specificity for the models (57.0% and 56.9%) suggests a higher rate of false positives, meaning that a healthy person is more likely to receive an incorrect diagnosis of OSA using the proposed tool. As Jonas et al. [30] concluded, "There is uncertainty about the accuracy or clinical utility of all potential screening tools." a PSG to verify OSA severity and confirm the diagnosis is warranted for patients with clinically suspected sleep apnea.
Our study validated the diagnostic relevance of age, sex, and BMI for OSA. The generated LR- and ANN-based model effectively predicted OSA risk using these three simple parameters. Our results, therefore, may improve insights into OSA-related essential risk factors and provide knowledge to facilitate individualized risk stratification and formulate diagnostic decisions for sleep apnea.
This work was funded by the National Science and Technology Council (NSTC 111-2314-B-038-132-MY3) to CC Chung.
The authors declare there is no conflict of interest.
[1] |
Tong SYC, Davis JS, Eichenberger E, et al. (2015) Staphylococcus aureus infections: Epidemiology, pathophysiology, clinical manifestations, and management. Clin Microbiol Rev 28: 603-661. https://doi.org/10.1128/CMR.00134-14 ![]() |
[2] |
Self WH, Wunderink RG, Williams DJ, et al. (2016) Staphylococcus aureus community-acquired pneumonia: prevalence, clinical characteristics, and outcomes. Clin Infect Dis 63: 300-309. https://doi.org/10.1093/CID/CIW300 ![]() |
[3] |
Grapsa J, Blauth C, Chandrashekhar YS, et al. (2022) Staphylococcus aureus infective endocarditis: jacc patient pathways. JACC Case Reports 4: 1-12. https://doi.org/10.1016/J.JACCAS.2021.10.002 ![]() |
[4] |
Kwiecinski JM, Horswill AR (2020) Staphylococcus aureus bloodstream infections: pathogenesis and regulatory mechanisms. Curr Opin Microbiol 53: 51-60. https://doi.org/10.1016/J.MIB.2020.02.005 ![]() |
[5] |
Malachowa N, Deleo FR (2010) Mobile genetic elements of Staphylococcus aureus. Cell Mol Life Sci 67: 3057-3071. https://doi.org/10.1007/s00018-010-0389-4 ![]() |
[6] | Islam T, Kubra K, Hassan Chowdhury MM (2018) Prevalence of methicillin-resistant Staphylococcus aureus in Hospitals in Chittagong, Bangladesh: A threat of nosocomial infection. J Microsc Ultrastruct 6: 188-191. https://doi.org/10.4103/JMAU.JMAU_33_18 |
[7] |
Kayili E, Sanlibaba P (2020) Prevalence, characterization and antibiotic resistance of Staphylococcus aureus isolated from traditional cheeses in Turkey. Int J Food Prop 23: 1441-1451. https://doi.org/10.1080/10942912.2020.1814323 ![]() |
[8] |
Loomba P, Taneja J, Mishra B (2010) Methicillin and vancomycin resistant S. aureus in hospitalized patients. J Glob Infect Dis 2: 275-283. https://doi.org/10.4103/0974-777x.68535 ![]() |
[9] |
Kali A (2015) Antibiotics and bioactive natural products in treatment of methicillin resistant Staphylococcus aureus: a brief review. Pharmacogn Rev 9: 29-34. https://doi.org/10.4103/0973-7847.156329 ![]() |
[10] | Abdallah EM, Mohamed Abdallah E (2016) Medicinal plants as an alternative drug against methicillin resistant Staphylococcus aureus. Int J Microbiol Allied Sci 3: 35-42. |
[11] |
Zhang K, McClure JA, Elsayed S, et al. (2009) Novel staphylococcal cassette chromosome mec type, tentatively designated type VIII, harboring class A mec and type 4 ccr gene complexes in a Canadian epidemic strain of methicillin-resistant Staphylococcus aureus. Antimicrob Agents Chemother 53: 531-540. https://doi.org/10.1128/AAC.01118-08 ![]() |
[12] |
Foster TJ (2017) Antibiotic resistance in Staphylococcus aureus. Current status and future prospects. FEMS Microbiol Rev 41: 430-449. https://doi.org/10.1093/FEMSRE/FUX007 ![]() |
[13] |
Hou Z, Liu L, Wei J, et al. (2023) Progress in the prevalence, classification and drug resistance mechanisms of methicillin-resistant Staphylococcus aureus. Infect Drug Resist 16: 3271-3292. https://doi.org/10.2147/IDR.S412308 ![]() |
[14] | Geoghegan JA, Foster TJ (2017) Cell wall-anchored surface proteins of Staphylococcus aureus: Many proteins, multiple functions. Current Topics in Microbiology and Immunology.Springer Verlag 95-120. https://doi.org/10.1007/82_2015_5002 |
[15] |
Foster TJ, Geoghegan JA, Ganesh VK, et al. (2014) Adhesion, invasion and evasion: The many functions of the surface proteins of Staphylococcus aureus. Nat Rev Microbiol 12: 49-62. https://doi.org/10.1038/nrmicro3161 ![]() |
[16] | Tam K, Torres VJ (2019) Staphylococcus aureus secreted toxins and extracellular enzymes. Microbiol Spectr 7. https://doi.org/10.1128/microbiolspec.gpp3-0039-2018 |
[17] | Yusuf MA, Islam S, Shamsuzzaman A, et al. (2013) Burden of infection caused by methicillin-resistant Staphylococcus aureus in Bangladesh: a systematic review. Glob Adv Res J Microbiol 2: 213-223. |
[18] |
Nickerson EK, West TE, Day NP, et al. (2009) Staphylococcus aureus disease and drug resistance in resource-limited countries in south and east Asia. Lancet Infect Dis 9: 130-135. https://doi.org/10.1016/S1473-3099(09)70022-2 ![]() |
[19] |
Chongtrakool P, Ito T, Ma XX, et al. (2006) Staphylococcal cassette chromosome mec (SCCmec) typing of methicillin-resistant Staphylococcus aureus strains isolated in 11 Asian countries: A proposal for a new nomenclature for SCCmec elements. Antimicrob Agents Chemother 50: 1001-1012. https://doi.org/10.1128/AAC.50.3.1001-1012.2006 ![]() |
[20] |
Hussain K, Rahman M, Nazir KHMNH, et al. (2016) Methicillin pesistant Staphylococcus aureus (MRSA) in Patients of Community Based Medical College Hospital, Mymensingh, Bangladesh. Am J Biomed Life Sci 4: 26-29. https://doi.org/10.11648/j.ajbls.20160403.11 ![]() |
[21] | Roy S, Barman TK, Hossain MA, et al. (2017) Molecular-characterization of methicillin-resistance Staphylococcus aureus (MRSA) from different Tertiary Care Hospitals in Bangladesh. Mymensingh Med J 26: 37-44. |
[22] |
Rahman MM, Amin KB, Rahman SMM, et al. (2018) Investigation of methicillin-resistant Staphylococcus aureus among clinical isolates from humans and animals by culture methods and multiplex PCR. BMC Vet Res 14: 300. https://doi.org/10.1186/s12917-018-1611-0 ![]() |
[23] |
Jorgensen JH, Turnidge JD (2015) Susceptibility test methods: dilution and disk diffusion methods. Manual of Clinical Microbiology.John Wiley & Sons, Ltd 1253-1273. https://doi.org/10.1128/9781555817381.ch71 ![]() |
[24] | CLSIM100-performance standards for antimicrobial susceptibility testing, clinical and laboratory standards institute (2022). |
[25] |
Magiorakos AP, Srinivasan A, Carey RB, et al. (2012) Multidrug-resistant, extensively drug-resistant and pandrug-resistant bacteria: An international expert proposal for interim standard definitions for acquired resistance. Clin Microbiol Infect 18: 268-281. https://doi.org/10.1111/j.1469-0691.2011.03570.x ![]() |
[26] |
Stepanović S, Vuković D, Dakić I, et al. (2000) A modified microtiter-plate test for quantification of staphylococcal biofilm formation. J Microbiol Methods 40: 175-179. https://doi.org/10.1016/S0167-7012(00)00122-6 ![]() |
[27] |
Wick RR, Judd LM, Gorrie CL, et al. (2017) Unicycler: Resolving bacterial genome assemblies from short and long sequencing reads. PLoS Comput Biol 13: e1005595. https://doi.org/10.1371/journal.pcbi.1005595 ![]() |
[28] |
Li H, Handsaker B, Wysoker A, et al. (2009) The sequence alignment/map format and SAMtools. Bioinformatics 25: 2078-2079. https://doi.org/10.1093/BIOINFORMATICS/BTP352 ![]() |
[29] |
Gurevich A, Saveliev V, Vyahhi N, et al. (2013) QUAST: Quality assessment tool for genome assemblies. Bioinformatics 29: 1072-1075. https://doi.org/10.1093/bioinformatics/btt086 ![]() |
[30] |
Brettin T, Davis JJ, Disz T, et al. (2015) RASTtk: A modular and extensible implementation of the RAST algorithm for building custom annotation pipelines and annotating batches of genomes. Sci Rep 5: 8365. https://doi.org/10.1038/srep08365 ![]() |
[31] |
Olson RD, Assaf R, Brettin T, et al. (2023) Introducing the bacterial and viral bioinformatics resource center (BV-BRC): a resource combining PATRIC, IRD and ViPR. Nucleic Acids Res 51: D678-D689. https://doi.org/10.1093/NAR/GKAC1003 ![]() |
[32] |
Wattam AR, Davis JJ, Assaf R, et al. (2017) Improvements to PATRIC, the all-bacterial bioinformatics database and analysis resource center. Nucleic Acids Res 45: D535-D542. https://doi.org/10.1093/nar/gkw1017 ![]() |
[33] |
Grant JR, Enns E, Marinier E, et al. (2023) Proksee: In-depth characterization and visualization of bacterial genomes. Nucleic Acids Res 51: W484-W492. https://doi.org/10.1093/nar/gkad326 ![]() |
[34] |
Alcock BP, Huynh W, Chalil R, et al. (2023) CARD 2023: expanded curation, support for machine learning, and resistome prediction at the Comprehensive Antibiotic Resistance Database. Nucleic Acids Res 51: D690-D699. https://doi.org/10.1093/NAR/GKAC920 ![]() |
[35] |
Ito T, Hiramatsu K, Oliveira DC, et al. (2009) Classification of staphylococcal cassette chromosome mec (SCCmec): Guidelines for reporting novel SCCmec elements. Antimicrob Agents Chemother 53: 4961-4967. https://doi.org/10.1128/AAC.00579-09 ![]() |
[36] |
Kondo Y, Ito T, Ma XX, et al. (2007) Combination of multiplex PCRs for staphylococcal cassette chromosome mec type assignment: Rapid identification system for mec, ccr, and major differences in junkyard regions. Antimicrob Agents Chemother 51: 264-274. https://doi.org/10.1128/AAC.00165-06 ![]() |
[37] |
Camacho C, Coulouris G, Avagyan V, et al. (2009) BLAST+: Architecture and applications. BMC Bioinformatics 10: 421. https://doi.org/10.1186/1471-2105-10-421 ![]() |
[38] |
Larsen MV, Cosentino S, Rasmussen S, et al. (2012) Multilocus sequence typing of total-genome-sequenced bacteria. J Clin Microbiol 50: 1355-1361. https://doi.org/10.1128/JCM.06094-11 ![]() |
[39] |
Griffiths D, Fawley W, Kachrimanidou M, et al. (2010) Multilocus sequence typing of Clostridium difficile. J Clin Microbiol 48: 770-778. https://doi.org/10.1128/JCM.01796-09 ![]() |
[40] |
Jolley KA, Bray JE, Maiden MCJ (2018) Open-access bacterial population genomics: BIGSdb software, the PubMLST.org website and their applications. Wellcome Open Res 3: 124. https://doi.org/10.12688/wellcomeopenres.14826.1 ![]() |
[41] |
Bartels MD, Petersen A, Worning P, et al. (2014) Comparing whole-genome sequencing with sanger sequencing for spa typing of methicillin-resistant Staphylococcus aureus. J Clin Microbiol 52: 4305-4308. https://doi.org/10.1128/JCM.01979-14 ![]() |
[42] |
Cosentino S, Voldby Larsen M, Møller Aarestrup F, et al. (2013) PathogenFinder-distinguishing friend from foe using bacterial whole genome sequence data. PLoS One 8: e77302. https://doi.org/10.1371/journal.pone.0077302 ![]() |
[43] |
Carattoli A, Zankari E, Garciá-Fernández A, et al. (2014) In Silico detection and typing of plasmids using plasmidfinder and plasmid multilocus sequence typing. Antimicrob Agents Chemother 58: 3895-3903. https://doi.org/10.1128/AAC.02412-14 ![]() |
[44] |
Arndt D, Grant JR, Marcu A, et al. (2016) PHASTER: a better, faster version of the PHAST phage search tool. Nucleic Acids Res 44: W16-W21. https://doi.org/10.1093/nar/gkw387 ![]() |
[45] |
Zhou Y, Liang Y, Lynch KH, et al. (2011) PHAST: A fast phage search tool. Nucleic Acids Res 39: W347-W352. https://doi.org/10.1093/nar/gkr485 ![]() |
[46] |
Liu M, Li X, Xie Y, et al. (2019) ICEberg 2.0: An updated database of bacterial integrative and conjugative elements. Nucleic Acids Res 47: D660-D665. https://doi.org/10.1093/nar/gky1123 ![]() |
[47] |
Chen L, Yang J, Yu J, et al. (2005) VFDB: a reference database for bacterial virulence factors. Nucleic Acids Res 33: D325-D328. https://doi.org/10.1093/NAR/GKI008 ![]() |
[48] |
Blin K, Shaw S, Augustijn HE, et al. (2023) antiSMASH 7.0: new and improved predictions for detection, regulation, chemical structures and visualisation. Nucleic Acids Res 51: W46-W50. https://doi.org/10.1093/NAR/GKAD344 ![]() |
[49] |
Akarsu H, Bordes P, Mansour M, et al. (2019) TASmania: A bacterial Toxin-Antitoxin Systems database. PLOS Comput Biol 15: e1006946. https://doi.org/10.1371/JOURNAL.PCBI.1006946 ![]() |
[50] |
Chaudhari NM, Gupta VK, Dutta C (2016) BPGA-an ultra-fast pan-genome analysis pipeline. Sci Rep 6: 24373. https://doi.org/10.1038/srep24373 ![]() |
[51] | Rodriguez-R LM, Konstantinidis KT (2016) The enveomics collection: a toolbox for specialized analyses of microbial genomes and metagenomes. PeerJ Prepr 4: e1900v1. https://doi.org/10.7287/PEERJ.PREPRINTS.1900V1 |
[52] |
Meier-Kolthoff JP, Göker M (2019) TYGS is an automated high-throughput platform for state-of-the-art genome-based taxonomy. Nat Commun 10: 2182. https://doi.org/10.1038/s41467-019-10210-3 ![]() |
[53] |
Kaas RS, Leekitcharoenphon P, Aarestrup FM, et al. (2014) Solving the problem of comparing whole bacterial genomes across different sequencing platforms. PLoS One 9: e104984. https://doi.org/10.1371/JOURNAL.PONE.0104984 ![]() |
[54] |
Letunic I, Bork P (2021) Interactive Tree Of Life (iTOL) v5: an online tool for phylogenetic tree display and annotation. Nucleic Acids Res 49: W293-W296. https://doi.org/10.1093/NAR/GKAB301 ![]() |
[55] |
Alam FM, Tasnim T, Afroz S, et al. (2023) Epidemiology and antibiogram of clinical Staphylococcus aureus isolates from Tertiary Care Hospitals in Dhaka, Bangladesh. Avicenna J Clin Microbiol Infect 9: 137-147. https://doi.org/10.34172/AJCMI.2022.3391 ![]() |
[56] |
Haque N, Aung MS, Paul SK, et al. (2019) Molecular epidemiological characterization of methicillin-susceptible and -resistant Staphylococcus aureus isolated from skin and soft tissue infections in Bangladesh. Microb Drug Resist 25: 241-250. https://doi.org/10.1089/mdr.2018.0123 ![]() |
[57] |
Mairi A, Touati A, Lavigne JP (2020) Methicillin-resistant Staphylococcus aureus ST80 Clone: A systematic review. Toxins 12: 119. https://doi.org/10.3390/toxins12020119 ![]() |
[58] |
Sarkhoo E, Udo EE, Boswihi SS, et al. (2021) The dissemination and molecular characterization of clonal complex 361 (CC361) methicillin-resistant Staphylococcus aureus (MRSA) in Kuwait Hospitals. Front Microbiol 12: 658772. https://doi.org/10.3389/fmicb.2021.658772 ![]() |
[59] |
Islam MA, Parveen S, Rahman M, et al. (2019) Occurrence and characterization of methicillin resistant Staphylococcus aureus in processed raw foods and ready-to-eat foods in an urban setting of a developing country. Front Microbiol 10: 503. https://doi.org/10.3389/FMICB.2019.00503 ![]() |
[60] |
Kinnevey PM, Shore AC, Brennan GI, et al. (2014) Extensive genetic diversity identified among sporadic methicillin-resistant Staphylococcus aureus isolates recovered in Irish hospitals between 2000 and 2012. Antimicrob Agents Chemother 58: 1907-1917. https://doi.org/10.1128/AAC.02653-13 ![]() |
[61] |
Boswihi SS, Udo EE, Al-Sweih N (2016) Shifts in the clonal distribution of methicillin-resistant Staphylococcus aureus in Kuwait Hospitals: 1992-2010. PLoS One 11: e0162744. https://doi.org/10.1371/journal.pone.0162744 ![]() |
[62] |
Afroz S, Kobayashi N, Nagashima S, et al. (2008) Genetic characterization of Staphylococcus aureus isolates carrying Panton-Valentine leukocidin genes in Bangladesh. Jpn J Infect Dis 61: 393-396. https://doi.org/10.7883/yoken.jjid.2008.393 ![]() |
[63] | Weber S, Ehricht R, Slickers P (2010) Genetic fingerprinting of MRSA from Abu Dhabi. ECCMID . |
[64] | Martínez-Meléndez A, Morfín-Otero R, Villarreal-Treviño L, et al. (2015) Staphylococcal Cassette Chromosome mec (SCCmec) in coagulase negative staphylococci. Med Univ 17: 229-233. https://doi.org/10.1016/J.RMU.2015.10.003 |
[65] |
Liakopoulos A, Foka A, Vourli S, et al. (2011) Aminoglycoside-resistant staphylococci in Greece: Prevalence and resistance mechanisms. Eur J Clin Microbiol Infect Dis 30: 701-705. https://doi.org/10.1007/s10096-010-1132-7 ![]() |
[66] | Khoramrooz SS, Alipoor Dolatabad S, Mostafapour Dolatabad F, et al. (2017) Detection of tetracycline resistance genes, aminoglycoside modifying enzymes, and coagulase gene typing of clinical isolates of Staphylococcus aureus in the Southwest of Iran. Iran J Basic Med Sci 20: 912-919. https://doi.org/10.22038/IJBMS.2017.9114 |
[67] |
Becker B, Cooper MA (2013) Aminoglycoside antibiotics in the 21st century. ACS Chem Biol 8: 105-115. https://doi.org/10.1021/CB3005116 ![]() |
[68] |
Floyd JL, Smith KP, Kumar SH, et al. (2010) LmrS is a multidrug efflux pump of the major facilitator superfamily from Staphylococcus aureus. Antimicrob Agents Chemother 54: 5406-5412. https://doi.org/10.1128/AAC.00580-10 ![]() |
[69] |
Costa SS, Sobkowiak B, Parreira R, et al. (2019) Genetic diversity of norA, coding for a main efflux pump of Staphylococcus aureus. Front Genet 9: 710. https://doi.org/10.3389/fgene.2018.00710 ![]() |
[70] |
Silva KPT, Sundar G, Khare A (2023) Efflux pump gene amplifications bypass necessity of multiple target mutations for resistance against dual-targeting antibiotic. Nat Commun 14: 3402. https://doi.org/10.1038/s41467-023-38507-4 ![]() |
[71] |
Sandvik EL, Fazen CH, Henry TC, et al. (2015) Non-monotonic survival of Staphylococcus aureus with respect to ciprofloxacin concentration arises from prophage-dependent killing of persisters. Pharmaceuticals 8: 778-792. https://doi.org/10.3390/ph8040778 ![]() |
[72] |
Lai CC, Chen CC, Lu YC, et al. (2018) The clinical significance of silent mutations with respect to ciprofloxacin resistance in MRSA. Infect Drug Resist 11: 681-687. https://doi.org/10.2147/IDR.S159455 ![]() |
[73] |
Hyun JC, Kavvas ES, Monk JM, et al. (2020) Machine learning with random subspace ensembles identifies antimicrobial resistance determinants from pan-genomes of three pathogens. PLOS Comput Biol 16: e1007608. https://doi.org/10.1371/JOURNAL.PCBI.1007608 ![]() |
[74] |
Huang H, Wan P, Luo X, et al. (2023) Tigecycline resistance-associated mutations in the mepa efflux pump in Staphylococcus aureus. Microbiol Spectr 11: e0063423. https://doi.org/10.1128/spectrum.00634-23 ![]() |
[75] |
Zeng W, Zhang X, Liu Y, et al. (2022) In vitro antimicrobial activity and resistance mechanisms of the new generation tetracycline agents, eravacycline, omadacycline, and tigecycline against clinical Staphylococcus aureus isolates. Front Microbiol 13: 1043736. https://doi.org/10.3389/fmicb.2022.1043736 ![]() |
[76] |
Dashtbani-Roozbehani A, Brown MH (2021) Efflux pump mediated antimicrobial resistance by staphylococci in health-related environments: Challenges and the quest for inhibition. Antibiotics 10: 1502. https://doi.org/10.3390/antibiotics10121502 ![]() |
[77] |
Miklasińska-Majdanik M (2021) Mechanisms of resistance to macrolide antibiotics among Staphylococcus aureus. Antibiotics 10: 1406. https://doi.org/10.3390/antibiotics10111406 ![]() |
[78] |
Rowe SJ, Mecaskey RJ, Nasef M, et al. (2020) Shared requirements for key residues in the antibiotic resistance enzymes ErmC and ErmE suggest a common mode of RNA recognition. J Biol Chem 295: 17476-17485. https://doi.org/10.1074/jbc.RA120.014280 ![]() |
[79] | Duran N, Ozer B, Duran GG, et al. (2012) Antibiotic resistance genes & susceptibility patterns in staphylococci. Indian J Med Res 135: 389-396. |
[80] |
Aziz KE, Abdulrahman ZFA (2021) Detection of tetracycline tet(k) gene in clinical Staphylococcus aureus isolates. IOP Conf Ser Earth Environ Sci 761: 012128. https://doi.org/10.1088/1755-1315/761/1/012128 ![]() |
[81] |
You Y, Hilpert M, Ward MJ (2013) Identification of Tet45, a tetracycline efflux pump, from a poultry-litter-exposed soil isolate and persistence of tet(45) in the soil. J Antimicrob Chemother 68: 1962-1969. https://doi.org/10.1093/JAC/DKT127 ![]() |
[82] |
Truong-Bolduc QC, Villet RA, Estabrooks ZA, et al. (2014) Native efflux pumps contribute resistance to antimicrobials of skin and the ability of Staphylococcus aureus to colonize skin. J Infect Dis 209: 1485-1493. https://doi.org/10.1093/infdis/jit660 ![]() |
[83] |
Chen C, Hooper DC (2018) Effect of Staphylococcus aureus Tet38 native efflux pump on in vivo response to tetracycline in a murine subcutaneous abscess model. J Antimicrob Chemother 73: 720-723. https://doi.org/10.1093/jac/dkx432 ![]() |
[84] |
Vestergaard M, Nøhr-Meldgaard K, Bojer MS, et al. (2017) Inhibition of the ATP synthase eliminates the intrinsic resistance of Staphylococcus aureus towards polymyxins. MBio 8: e01114-17. https://doi.org/10.1128/mBio.01114-17 ![]() |
[85] |
Thompson MK, Keithly ME, Goodman MC, et al. (2014) Structure and function of the genomically encoded fosfomycin resistance enzyme, FosB, from Staphylococcus aureus. Biochemistry 53: 755-765. https://doi.org/10.1021/bi4015852 ![]() |
[86] |
Chen Y, Ji S, Sun L, et al. (2022) The novel fosfomycin resistance gene fosY is present on a genomic island in CC1 methicillin-resistant Staphylococcus aureus. Emerg Microbes Infect 11: 1166-1173. https://doi.org/10.1080/22221751.2022.2058421 ![]() |
[87] |
Nurjadi D, Schäfer J, Friedrich-Jänicke B, et al. (2015) Predominance of dfrG as determinant of trimethoprim resistance in imported Staphylococcus aureus. Clin Microbiol Infect 21: 1095.e5-1095.e9. https://doi.org/10.1016/j.cmi.2015.08.021 ![]() |
[88] |
Pishchany G, Sheldon JR, Dickson CF, et al. (2014) IsdB-dependent hemoglobin binding is required for acquisition of heme by Staphylococcus aureus. J Infect Dis 209: 1764-1772. https://doi.org/10.1093/INFDIS/JIT817 ![]() |
[89] |
Shambat S, Nadig S, Prabhakara S, et al. (2012) Clonal complexes and virulence factors of Staphylococcus aureus from several cities in India. BMC Microbiol 12: 64. https://doi.org/10.1186/1471-2180-12-64 ![]() |
[90] |
Beceiro A, Tomás M, Bou G (2013) Antimicrobial resistance and virulence: A successful or deleterious association in the bacterial world?. Clin Microbiol Rev 26: 185-230. https://doi.org/10.1128/CMR.00059-12 ![]() |
[91] |
Ruiz B, Chávez A, Forero A, et al. (2010) Production of microbial secondary metabolites: Regulation by the carbon source. Crit Rev Microbiol 36: 146-167. https://doi.org/10.3109/10408410903489576 ![]() |
[92] | Grim KP, Radin JN, Solórzano PKP, et al. (2020) Intracellular accumulation of staphylopine can sensitize Staphylococcus aureus to host-imposed zinc starvation by chelation-independent toxicity. J Bacteriol 202: e00014-20. https://doi.org/10.1128/JB.00014-20 |
[93] |
Chen C, Hooper DC (2019) Intracellular accumulation of Staphylopine impairs the fitness of Staphylococcus aureus cntE mutant. FEBS Lett 593: 1213-1222. https://doi.org/10.1002/1873-3468.13396 ![]() |
[94] |
Dale SE, Doherty-Kirby A, Lajoie G, et al. (2004) Role of siderophore biosynthesis in virulence of Staphylococcus aureus: identification and characterization of genes involved in production of a siderophore. Infect Immun 72: 29-37. https://doi.org/10.1128/IAI.72.1.29-37.2004 ![]() |
[95] |
Tripathi A, Schofield MM, Chlipala GE, et al. (2014) Baulamycins A and B, broad-spectrum antibiotics identified as inhibitors of siderophore biosynthesis in Staphylococcus aureus and Bacillus anthracis. J Am Chem Soc 136: 1579-1586. https://doi.org/10.1021/ja4115924 ![]() |
[96] |
Grim KP, San Francisco B, Radin JN, et al. (2017) The metallophore staphylopine enables Staphylococcus aureus to compete with the host for zinc and overcome nutritional immunity. MBio 8: e01281-17. https://doi.org/10.1128/mBio.01281-17 ![]() |
[97] |
Ghssein G, Ezzeddine Z (2022) The key element role of metallophores in the pathogenicity and virulence of Staphylococcus aureus: A review. Biology 11: 1525. https://doi.org/10.3390/biology11101525 ![]() |
[98] |
Parvez A, Giri S, Giri GR, et al. (2018) Novel type iii polyketide synthases biosynthesize methylated polyketides in Mycobacterium marinum. Sci Rep 8: 6529. https://doi.org/10.1038/s41598-018-24980-1 ![]() |
[99] |
Sun F, Cho H, Jeong DW, et al. (2010) Aureusimines in Staphylococcus aureus Are Not Involved in Virulence. PLoS One 5: e15703. https://doi.org/10.1371/journal.pone.0015703 ![]() |
[100] |
Barbosa J, Caetano T, Mendo S (2015) Class I and Class II lanthipeptides produced by Bacillus spp. J Nat Prod 78: 2850-2866. https://doi.org/10.1021/np500424y ![]() |
[101] |
Hudson GA, Mitchell DA (2018) RiPP antibiotics: biosynthesis and engineering potential. Curr Opin Microbiol 45: 61-69. https://doi.org/10.1016/j.mib.2018.02.010 ![]() |
[102] |
Stariha LM, McCafferty DG (2021) Discovery of the Class I antimicrobial lasso peptide arcumycin. ChemBioChem 22: 2632-2640. https://doi.org/10.1002/CBIC.202100132 ![]() |
[103] |
Kamruzzaman M, Iredell J (2019) A ParDE-family toxin antitoxin system in major resistance plasmids of Enterobacteriaceae confers antibiotic and heat tolerance. Sci Rep 9: 9872. https://doi.org/10.1038/s41598-019-46318-1 ![]() |
[104] |
Maisonneuve E, Shakespeare LJ, Jørgensen MG, et al. (2011) Bacterial persistence by RNA endonucleases. Proc Natl Acad Sci USA 108: 13206-13211. https://doi.org/10.1073/pnas.1100186108 ![]() |
[105] |
Dörr T, Vulić M, Lewis K (2010) Ciprofloxacin causes persister formation by inducing the TisB toxin in Escherichia coli. PLOS Biol 8: e1000317. https://doi.org/10.1371/JOURNAL.PBIO.1000317 ![]() |
[106] |
Schuster CF, Bertram R (2013) Toxin–antitoxin systems are ubiquitous and versatile modulators of prokaryotic cell fate. FEMS Microbiol Lett 340: 73-85. https://doi.org/10.1111/1574-6968.12074 ![]() |
[107] |
Mruk I, Kobayashi I (2014) To be or not to be: Regulation of restriction-modification systems and other toxin-antitoxin systems. Nucleic Acids Res 42: 70-86. https://doi.org/10.1093/nar/gkt711 ![]() |
[108] |
Schuster CF, Bertram R (2016) Toxin-antitoxin systems of Staphylococcus aureus. Toxins 8: 140. https://doi.org/10.3390/toxins8050140 ![]() |
[109] |
Motyka-Pomagruk A, Zoledowska S, Misztak AE, et al. (2020) Comparative genomics and pangenome-oriented studies reveal high homogeneity of the agronomically relevant enterobacterial plant pathogen Dickeya solani. BMC Genomics 21: 449. https://doi.org/10.1186/s12864-020-06863-w ![]() |
[110] |
Sivakumar R, Pranav PS, Annamanedi M, et al. (2023) Genome sequencing and comparative genomic analysis of bovine mastitis-associated Staphylococcus aureus strains from India. BMC Genomics 24: 44. https://doi.org/10.1186/s12864-022-09090-7 ![]() |
![]() |
![]() |
Variables | Moderate-to-severe OSA (AHI ≥ 15) |
None-to-mild OSA (AHI < 15) |
p-value |
n | 5, 920 | 3, 502 | |
Age | 50.8 ± 13.5 | 46.6 ± 14.4 | < 0.0001 |
Female, n (%) | 1266 (21.4) | 1652 (47.2) | < 0.0001 |
Height (cm) | 167.2 ± 8.5 | 164.4 ± 8.9 | < 0.0001 |
Weight (kg) | 79.7±16.1 | 66.1 ± 13.1 | < 0.0001 |
BMI (kg/m2) | 28.4 ± 4.8 | 24.3 ± 3.8 | < 0.0001 |
Polysomnography | |||
Total recording time (min) | 366.6±11.9 | 367.8±13.4 | < 0.0001 |
Total sleep time (min) | 275.8±60.2 | 281.4±61.1 | < 0.0001 |
Sleep efficiency (%) | 75.2±16.4 | 76.5±16.5 | 0.0002 |
Non-REM sleep (min) | 240.6±51.7 | 242.2±51.3 | 0.133 |
REM sleep (min) | 35.2±22.5 | 39.1±23.7 | < 0.0001 |
AHI | 44.7±23.8 | 6.4±4.5 | < 0.0001 |
SO2 mean (%) | 94.5±2.6 | 96.6±1.3 | < 0.0001 |
Data are expressed as mean ± standard deviation. The p-value represents the comparison between the AHI ≥ 15 and AHI < 15 groups. |
Unadjusted Model | Adjusted Model | |||
Variables | OR | 95% CI | OR | 95% CI |
Age | 1.02*† | 1.02-1.03 | 1.04*† | 1.04-1.05 |
Female | 0.30* | 0.28-0.33 | 0.28* | 0.25-0.32 |
BMI | 1.27*† | 1.25-1.28 | 1.27*† | 1.26-1.29 |
The odds ratio (OR) and 95% confidence intervals (CI) represent the association between the variables and the presence of moderate-to-severe OSA (AHI ≥ 15). *p < 0.0001; †Odds ratio of per unit changes. |
Variable | Testing set 1 | Testing set 2 | Testing set 3 | Testing set 4 | Testing set 5 | Independent validation set | p-value |
n | 1466 | 1465 | 1466 | 1465 | 1466 | 2094 | |
AHI≥15, n (%) | 920 (62.8) | 919 (62.7) | 920 (62.8) | 919 (62.7) | 920 (62.8) | 1322 (63.1) | 1.000 |
Age | 49.0 ± 0.4 | 49.0 ± 0.4 | 49.9 ± 0.4 | 49.1 ± 0.4 | 49.0 ± 0.4 | 49.3 ± 0.3 | 0.503 |
Female, n (%) | 483 (33.0) | 438 (29.9) | 472 (32.2) | 445 (30.4) | 431 (29.4) | 649 (31.0) | 0.266 |
BMI (kg/m2) | 26.9 ± 4.9 | 26.9 ± 4.9 | 26.9 ± 5.0 | 26.9 ± 5.0 | 26.8 ± 4.8 | 26.9 ± 4.9 | 0.973 |
The p-value represents the multiple comparisons across the five testing and the independent validation sets. |
Variables | Moderate-to-severe OSA (AHI ≥ 15) |
None-to-mild OSA (AHI < 15) |
p-value |
n | 5, 920 | 3, 502 | |
Age | 50.8 ± 13.5 | 46.6 ± 14.4 | < 0.0001 |
Female, n (%) | 1266 (21.4) | 1652 (47.2) | < 0.0001 |
Height (cm) | 167.2 ± 8.5 | 164.4 ± 8.9 | < 0.0001 |
Weight (kg) | 79.7±16.1 | 66.1 ± 13.1 | < 0.0001 |
BMI (kg/m2) | 28.4 ± 4.8 | 24.3 ± 3.8 | < 0.0001 |
Polysomnography | |||
Total recording time (min) | 366.6±11.9 | 367.8±13.4 | < 0.0001 |
Total sleep time (min) | 275.8±60.2 | 281.4±61.1 | < 0.0001 |
Sleep efficiency (%) | 75.2±16.4 | 76.5±16.5 | 0.0002 |
Non-REM sleep (min) | 240.6±51.7 | 242.2±51.3 | 0.133 |
REM sleep (min) | 35.2±22.5 | 39.1±23.7 | < 0.0001 |
AHI | 44.7±23.8 | 6.4±4.5 | < 0.0001 |
SO2 mean (%) | 94.5±2.6 | 96.6±1.3 | < 0.0001 |
Data are expressed as mean ± standard deviation. The p-value represents the comparison between the AHI ≥ 15 and AHI < 15 groups. |
Unadjusted Model | Adjusted Model | |||
Variables | OR | 95% CI | OR | 95% CI |
Age | 1.02*† | 1.02-1.03 | 1.04*† | 1.04-1.05 |
Female | 0.30* | 0.28-0.33 | 0.28* | 0.25-0.32 |
BMI | 1.27*† | 1.25-1.28 | 1.27*† | 1.26-1.29 |
The odds ratio (OR) and 95% confidence intervals (CI) represent the association between the variables and the presence of moderate-to-severe OSA (AHI ≥ 15). *p < 0.0001; †Odds ratio of per unit changes. |
Variable | Testing set 1 | Testing set 2 | Testing set 3 | Testing set 4 | Testing set 5 | Independent validation set | p-value |
n | 1466 | 1465 | 1466 | 1465 | 1466 | 2094 | |
AHI≥15, n (%) | 920 (62.8) | 919 (62.7) | 920 (62.8) | 919 (62.7) | 920 (62.8) | 1322 (63.1) | 1.000 |
Age | 49.0 ± 0.4 | 49.0 ± 0.4 | 49.9 ± 0.4 | 49.1 ± 0.4 | 49.0 ± 0.4 | 49.3 ± 0.3 | 0.503 |
Female, n (%) | 483 (33.0) | 438 (29.9) | 472 (32.2) | 445 (30.4) | 431 (29.4) | 649 (31.0) | 0.266 |
BMI (kg/m2) | 26.9 ± 4.9 | 26.9 ± 4.9 | 26.9 ± 5.0 | 26.9 ± 5.0 | 26.8 ± 4.8 | 26.9 ± 4.9 | 0.973 |
The p-value represents the multiple comparisons across the five testing and the independent validation sets. |