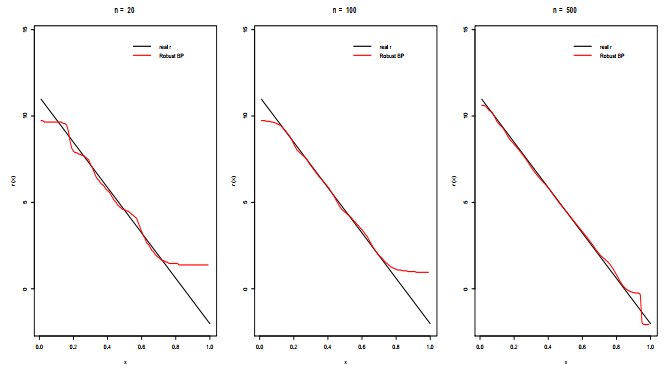
Soluble Fas ligand (sFasL, sCD95L) and its specific soluble binders, soluble Fas receptor (sFas, sCD95) and decoy receptor 3 (DcR3), have been investigated as possible clinical biomarkers in many serious diseases. The present review aimed to provide an overview of the current state of this medically promising research by extensively examining the relevant literature. The summarized results of the survey are presented after classification into six categories according to the type of targeted disease. To date, the studies have been mainly devoted to the diagnosis of disease severity states and prognosis of treatments about various types of cancers and autoimmune diseases represented by autoimmune lymphoproliferative syndrome and systemic lupus erythematosus, because these important life-threatening or intractable diseases were suggested to be most relevant to the impairment of apoptotic cell death-inducing systems, including the Fas receptor-mediated signaling system, and the mechanisms responsible for their onset. However, various more general inflammation-related diseases, including, but not limited to, other autoimmune and allergic diseases (e.g., rheumatoid arthritis and atopic asthma), infectious diseases (e.g., sepsis and chronic hepatitis), cardiovascular system-specific disorders (e.g., acute coronary syndromes and heart failure) as well as other diseases specific to the renal, hepatic, and respiratory systems, etc., have also been targeted as important fields of research. The data obtained so far demonstrated that sFas, sFasL, and DcR3 possess significant potential in the assessment of various disease states, which can contribute to the development of therapeutic interventions. Although further studies in various relevant fields are essential, it is expected that clinical translation of sFas, sFasL, and DcR3 into practical biomarkers will contribute to effective treatments of a wide variety of diseases.
Citation: Michiro Muraki. Soluble Fas ligand, soluble Fas receptor, and decoy receptor 3 as disease biomarkers for clinical applications: A review[J]. AIMS Medical Science, 2022, 9(2): 98-267. doi: 10.3934/medsci.2022009
[1] | Breix Michael Agua, Salim Bouzebda . Single index regression for locally stationary functional time series. AIMS Mathematics, 2024, 9(12): 36202-36258. doi: 10.3934/math.20241719 |
[2] | Fatimah Alshahrani, Wahiba Bouabsa, Ibrahim M. Almanjahie, Mohammed Kadi Attouch . Robust kernel regression function with uncertain scale parameter for high dimensional ergodic data using $ k $-nearest neighbor estimation. AIMS Mathematics, 2023, 8(6): 13000-13023. doi: 10.3934/math.2023655 |
[3] | Oussama Bouanani, Salim Bouzebda . Limit theorems for local polynomial estimation of regression for functional dependent data. AIMS Mathematics, 2024, 9(9): 23651-23691. doi: 10.3934/math.20241150 |
[4] | Salim Bouzebda, Amel Nezzal, Issam Elhattab . Limit theorems for nonparametric conditional U-statistics smoothed by asymmetric kernels. AIMS Mathematics, 2024, 9(9): 26195-26282. doi: 10.3934/math.20241280 |
[5] | Lanyin Sun, Siya Wen . Applications of mixed finite element method based on Bernstein polynomials in numerical solution of Stokes equations. AIMS Mathematics, 2024, 9(12): 35978-36000. doi: 10.3934/math.20241706 |
[6] | Salim Bouzebda . Weak convergence of the conditional single index $ U $-statistics for locally stationary functional time series. AIMS Mathematics, 2024, 9(6): 14807-14898. doi: 10.3934/math.2024720 |
[7] | Jieqiong Lu, Peixin Zhao, Xiaoshuang Zhou . Orthogonality based modal empirical likelihood inferences for partially nonlinear models. AIMS Mathematics, 2024, 9(7): 18117-18133. doi: 10.3934/math.2024884 |
[8] | Said Attaoui, Billal Bentata, Salim Bouzebda, Ali Laksaci . The strong consistency and asymptotic normality of the kernel estimator type in functional single index model in presence of censored data. AIMS Mathematics, 2024, 9(3): 7340-7371. doi: 10.3934/math.2024356 |
[9] | Sima Karamseraji, Shokrollah Ziari, Reza Ezzati . Approximate solution of nonlinear fuzzy Fredholm integral equations using bivariate Bernstein polynomials with error estimation. AIMS Mathematics, 2022, 7(4): 7234-7256. doi: 10.3934/math.2022404 |
[10] | Sangwan Kim, Yongku Kim, Jung-In Seo . Nonparametric Bayesian modeling for non-normal data through a transformation. AIMS Mathematics, 2024, 9(7): 18103-18116. doi: 10.3934/math.2024883 |
Soluble Fas ligand (sFasL, sCD95L) and its specific soluble binders, soluble Fas receptor (sFas, sCD95) and decoy receptor 3 (DcR3), have been investigated as possible clinical biomarkers in many serious diseases. The present review aimed to provide an overview of the current state of this medically promising research by extensively examining the relevant literature. The summarized results of the survey are presented after classification into six categories according to the type of targeted disease. To date, the studies have been mainly devoted to the diagnosis of disease severity states and prognosis of treatments about various types of cancers and autoimmune diseases represented by autoimmune lymphoproliferative syndrome and systemic lupus erythematosus, because these important life-threatening or intractable diseases were suggested to be most relevant to the impairment of apoptotic cell death-inducing systems, including the Fas receptor-mediated signaling system, and the mechanisms responsible for their onset. However, various more general inflammation-related diseases, including, but not limited to, other autoimmune and allergic diseases (e.g., rheumatoid arthritis and atopic asthma), infectious diseases (e.g., sepsis and chronic hepatitis), cardiovascular system-specific disorders (e.g., acute coronary syndromes and heart failure) as well as other diseases specific to the renal, hepatic, and respiratory systems, etc., have also been targeted as important fields of research. The data obtained so far demonstrated that sFas, sFasL, and DcR3 possess significant potential in the assessment of various disease states, which can contribute to the development of therapeutic interventions. Although further studies in various relevant fields are essential, it is expected that clinical translation of sFas, sFasL, and DcR3 into practical biomarkers will contribute to effective treatments of a wide variety of diseases.
Acute-on-chronic liver failure;
Acute coronary syndrome;
Alzheimer's disease;
Adenosine deaminase;
Autosomal dominant polycystic kidney disease;
Acute graft-versus-host disease;
Apnea-hypopnea index;
Allogeneic hematopoietic stem cell transplantation;
Acute kidney injury;
Alanine aminotransferase;
Autoimmune lymphoproliferative syndrome;
Age-related macular degeneration;
Acute myocardial infarction;
Acute physiology and chronic health evaluation;
Acute rejection;
Acute respiratory distress syndrome;
Ascites;
Bladder cancer;
Body-mass-index;
Coronary artery bypass grafting;
Coronary artery disease;
Cord blood;
Coronary collateral circulation;
Coronary heart disease;
Cirrhosis;
Chronic kidney disease;
Compensated liver cirrhosis accompanied with portal hypertension;
Chronic obstructive pulmonary disease;
SARS-CoV-2 virus;
Clinical remission;
C-reactive protein;
Cerebrospinal fluid;
Cardiovascular disease;
Decoy receptor 3;
Diabetic foot lesions;
Death-inducing signaling complex;
Diabetes mellitus;
Extracellular domain;
Estimated glomerular filtration rate;
Erythropoietin;
Erythropoietin-stimulating agent;
End-Stage renal disease;
Ebora virus disease;
Fas-associating protein with death domain;
Fas ligand;
Flare;
Graves' disease;
Graves' hyperthyroidism;
Gastrointestinal stromal carcinoma;
Graves' ophthalmopathy;
Hemoglobin;
Hepatitis-B virus;
Hepatocellular cancer;
Hepatitis-C virus;
Hemodialysis;
Hydrocephalus;
Heart failure;
Hemorrhagic fever with renal syndrome;
Hypoxic-ischemic encephalopathy;
Human immune-deficiency virus;
Head and neck cancer;
Hormone replacement therapy;
Hypertension;
Interferon;
Interleukin;
Intraparenchymal hemorrhage;
Ischemic stroke;
Intrauterine growth restriction;
Kawasaki disease;
Liver fibrosis;
Lupus nephritis;
Mild cognitive impairment;
Model for end-stage liver disease;
Metabolic syndrome;
Multiple sclerosis;
Nonalcoholic fatty liver disease;
Nonalcoholic steatohepatitis;
Non-hemorrhagic;
Nonagenarians;
Non-resolving subphenotype;
Oxygen-gas treatment;
Pleural effusion;
Post-hemorrhagic;
Pediatric kidney transplant recipients;
Plasma;
Postmenopausal syndrome;
Peripartum cardiography;
Prostate cancer;
Preserved renal function;
Pulmonary thromboembolism;
Periventricular leukomalacia;
Rheumatoid arthritis;
Renal cell cancer;
Relapsing-remitting MS;
Saliva;
Sickle cell disease;
Synovial fluid;
Soluble Fas receptor;
Soluble Fas ligand;
Systemic inflammatory response syndrome;
Stevens-Johnson syndrome;
Systemic lupus erythematosus;
Sequential organ failure assessment;
Serum;
Stomach cancer;
Tumor necrosis factor;
Sjögren's syndrome;
Subarachnoid hemorrhage;
Type-1 DM;
Type-2 DM;
Tau protein/amyloid β 1-42 ratio;
Tuberculosis;
Toxic epidermal necrolysis;
Thyroid stimulating hormone receptor antibodies;
Toxic epidermal necrolysis;
Peak oxygen consumption;
Vascular endothelial dysfunction;
Werner syndrome;
Percent body fat;
The area under the curve;
Regression coefficient;
Correlation analysis;
Concordance statistic;
Cutoff value;
Double determinant immunoassay;
Enzyme-linked immunosorbent assay;
Hazard ratio;
Interquartile range;
Liquid chromatography-electrospray ionization mass-spectrometry method;
Median;
Mean;
Multiplex array assay;
Not described;
Not significant;
Odds ratio;
Probability value;
Primer extension assay;
Pearson's correlation coefficient;
Spearman's correlation coefficient;
Determination coefficient;
(whole) Range;
Receiver operating characteristic curve;
Risk ratio;
Standard deviation;
Standard error of the mean;
Western-blotting method;
95% Confidential interval
Regression is the most frequently employed technique in nonparametric statistics to examine the association between two variables X and Y. In this context, Y represents the response variable, while X is a random vector of predictors (covariates) that can assume values in the real number space R. The regression function at a point x∈R is the conditional expectation of Y given X=x, denoted as
r(x):=E(Y|X=x). |
Various techniques can be employed to estimate a regression function, including kernel estimators, regression spline methods, and others. Nevertheless, these methods lack robustness as they are highly susceptible to outliers. Given that outliers are commonly observed in various fields, such as finance, it is essential to handle outliers properly to emphasize a dataset's unique features. Robust regression is a statistical technique used to address the issue of lack of robustness in regression models. It ensures that the model remains stable and resistant to the influence of outliers.
Robust regression holds significant importance within the realm of statistics. It is employed to overcome certain constraints of non-robust regression, specifically when the data exhibit heteroscedasticity or include outliers. The earliest significant outcome in this field can be traced back to Huber's work in [1]. The regression estimation method mentioned has been extensively researched. For empirical data, notable studies include Robinson [2], Collomb and H¨ardle [3], Boente and Fraiman [4,5], and Fan et al. [6] for earlier findings. Recent advancements and references can be found in Laib and Ould-Saïd [7] and Boente and Rodriguez [8]. Traditional kernel estimators often exhibit significant bias near boundaries because the kernel's support can extend beyond them, resulting in inaccurate estimates. Being supported on the entire interval, Bernstein estimators do not suffer from this boundary bias, leading to more accurate estimations near the edges.
The Bernstein polynomial is widely acknowledged as a valuable tool for interpolating functions on a closed interval, rendering it suitable for approximating density functions within that interval.
The use of Bernstein polynomials as density estimators for variables with finite support has been proposed in several articles. Vitale [9] first introduced this concept, followed by Petrone [10,11]. Further studies on this topic were conducted by Babu, et al. [12], Petrone and Wassermann[13], and Kakizawa [14].
Recently, Ouimet [15] studied some asymptotic properties of Bernstein cumulative distribution function and density estimators on the d-dimensional simplex and studied their asymptotic normality and uniform strong consistency. Belalia et al. [16] introduced a two-stage Bernstein estimator for conditional distribution functions. Various other statistical topics related to the Bernstein estimator have been treated; for more references, see Ouimet [15]. Khardani [17] investigated various asymptotic properties (bias, variance, mean squared error, asymptotic normality, uniform strong consistency) for Bernstein estimators of quantiles and cumulative distribution functions when the variable of interest is subject to random right-censoring.
It is essential to mention that several authors have devised Bernstein-based methodologies for addressing non-parametric function estimation problems. Priestley and Chao[18] first proposed the potential application of Bernstein polynomials for regression problems. Tenbusch [19], Brown and Chen [20], Choudhuri, Ghosal, and Roy [21], Chang, Hsiung, Wu, and Yang [22], Kakizawa [23], and Slaoui and Jmaei [24] have all conducted research on various non-parametric function estimation problems.
In this paper, our contribution is to find asymptotic expressions for the bias, variance, and mean squared error (MSE) for the Bernstein robust regression function estimator defined in (2.4) and (2.3) and also prove their asymptotic normality and convergence. We deduce the asymptotically optimal bandwidth parameter m using the expression for the MSE as well. The results provided by our Bernstein approach for the robust regression function are better than those of the traditional kernel estimators. In future work, using some kernels, such as Dirichlet, Wishart, and inverse Gaussian kernels, and the robust function will be investigated in other spaces, such as the simplex, the space of positive definite matrices, and half-spaces, etc.
The subsequent sections of the paper are structured in the following manner. In the next section, we will introduce our model. Section 3 presents notations, assumptions, and investigates various asymptotic properties of the proposed estimator. Section 4 presents a simulation study that evaluates the proposed approach's performance compared to the Bernstein-Nadaraya-Watson estimator and the Nadaraya-Watson estimator. Section 5 discusses a real data application, while the proofs of the results are provided in the Appendix.
Let (X,Y),(X1,Y1),…,(Xn,Yn) be independent, identically distributed pairs of random variables with joint density function g(x,y), and let f denote the probability density of X, which is supported on [0,1]. Let x be a fixed element of R, and let ρ a real-valued Borel function that satisfies specific regularity conditions outlined below. The robust method used to study the links between X and Y belongs to the class of M-estimates introduced by Huber [1]. The robust nonparametric parameter studied in this work, denoted by θx, is implicitly defined as the unique minimizer w.r.t. t of
r(x,t):=E(ρ(Y−t)|X=x), | (2.1) |
that is
θx=argmint∈Rr(x,t). | (2.2) |
This definition covers and includes many important nonparametric models, for example, ρ(t)=t2 yields the non-robust regression, ρ(t)=|t| leads to the conditional median function m(x)=med(Y∣X=x), and the α−th conditional quantile is obtained by setting ρ(t)=|t|+(2α−1)(t). We return to Stone [25] for other examples of the function ρ.
We utilize the techniques outlined in Vitale [9] and Leblanc [26,27] for distribution and density estimation. Additionally, we refer to the work of Slaoui [28] and Tenbusch [19,29] for non-robust regression. Our objective is to establish a Bernstein estimator for robust regression, defined as
ˆθx=argmint∈Rˆrn(x,t), | (2.3) |
with at a given point x∈[0,1] such that f(x)≠0 and
ˆrn(x,t)=∑ni=1ρ(Yi−t)∑mn−1k=0I{kmn<Xi≤k+1mn}Bk(mn−1,x)∑ni=1∑mn−1k=0I{kmn<Xi≤k+1mn}Bk(mn−1,x)=Nn(x,t)fn(x), | (2.4) |
where Bk(m,x)=(mk)xk(1−x)m−k is the Bernstein polynomial of order m. This estimator can be viewed as a generalization of the estimator proposed in Slaoui and Jmaei [28], with
Nn(x,t)=mnnn∑i=1ρ(Yi−t)mn−1∑k=0I{kmn<Xi≤k+1mn}Bk(mn−1,x), |
where fn is Vitale's estimator of the density f defined, for all x∈[0,1], by
fn(x)=mnnn∑i=1mn−1∑k=0I{kmn<X≤k+1mn}Bk(mn−1,x)=mnmn−1∑k=0{Fn(k+1mn)−Fn(kmn)}Bk(mn−1,x), | (2.5) |
with Fn, the empirical distribution function of the variable X.
This paper will use the following notations:
ψ(x)=(4πx(1−x))−1/2,Δ1(x)=12[(1−2x)f′(x)+x(1−x)f′′(x)],Δ2(x)=12{(1−2x)(∂r∂x(x,t)f(x)+f′(x)r(x,t))+x(1−x)(2f′(x)∂r∂x(x,t)+f(x)∂2r∂x2(x,t)+f′′(x)r(x,t))},Δ(x)=12{x(1−x)∂2r∂x2(x,t)+[(1−2x)+2x(1−x)f′(x)f(x)]∂r∂x(x,t)},δ1=∫10Δ2(x)dx,δ2=∫10Var[ρ(Y−t)∣X=x]f(x)ψ(x)dx. |
Moreover, we denote by o the pointwise bound in x (i.e., the error is not uniform in x∈[0,1]).
Remark 2.1. Robust regression is advantageous in real data settings where outliers, non-normal errors, or heteroscedasticity are present, making it a more flexible and resilient choice.
To state our results, we will need to gather some assumptions to make reading our results easier. In what follows, we will assume that the following assumptions hold:
Throughout the paper, C1,C2,C3 represent positive constants, while C denotes a generic constant independent of n. Let I0:={x∈[0,1]:f(x)>0} and S be a compact subset of I0.
H1: mn≥2, mn⟶n→+∞∞ and mn/n⟶n→+∞0.
H2: g(s,t) is twice continuously differentiable with respect to s.
H3: For q∈{0,1,2},s↦∫Rtqg(s,t)dt is a bounded function continuous at s=x.
H4: For q>2,s↦∫R|t|−qg(s,t)dt is a bounded function.
H5: The function ρ(.) is a bounded, monotone, differentiable function. Its derivative is bounded.
H6: The functions r and f are continuous and admit twice continuous and bounded derivatives such that |∂r∂x(x,t)|≥C>0, ∀x∈R.
H7: r(x,.) is of class C1 on [θx−τ,θx+τ] and satisfies inf[θx−τ,θx+τ]|∂r∂t(x,.)|>C3 and uniformly continuous.
The assumptions we make are typical for this type of framework. Assumption (H1) is a technical requirement imposed to make proofs more concise. Assumptions (H2)–(H4) are necessary conditions for the estimation of the regression function in the couple (X,Y), as outlined in the works of Nadaraya [30], Watson [31], and Slaoui and Jmaei [28]. These assumptions pertain to the regularity of the density function. The condition (H5) controls the robustness properties of our model. It maintains the same conditions on the function ρ′ as those provided by Collomb and Härdle [3] and Boente and Rodriguez [8] in the multivariate case. Assumptions (H6) and (H7) deal with some regularity of the function r(.,.). Note that condition (H6) is used to get the asymptotic normality of our estimator, and condition (H7) is somewhat less restrictive compared to that presented in the literature (see Boente and Fraiman [32], L. Aït Hennani, M.Lemdani, and E. Ould Saïd [33], Attouch et al.[34,35]), needed for the consistency result.
Proposition 3.1. Under Assumptions (H1)–(H5), and for x∈[0,1] such that f(x)>0, we have
E[ˆrn(x,t)]−r(x,t)=Δ(x)m−1n+o(m−1n), | (3.1) |
Var[ˆrn(x,t)]={m1/2nnE[(ρ(Y−t))2∣X=x]f(x)ψ(x)+ox(m3/2nn)forx∈(0,1),mnnE[(ρ(Y−t))2∣X=x]f(x)+ox(mnn)forx=0,1, | (3.2) |
MSE[ˆrn(x,t)]={Δ2(x)m−2n+m1/2nnVar(ρ(Y−t)∣X=x)f(x)ψ(x)+o(m−2n)+ox(m1/2nn)ifx∈(0,1),Δ2(x)m−2n+mnVar(ρ(Y−t)∣X=x)f(x)+o(m−2n)+ox(mnn)ifx=0,1. | (3.3) |
To minimize the MSE of ˆrn, for x∈[0,1] such that f(x)>0, the order mn must be equal to
mopt={[4Δ2(x)f(x)Var(ρ(Y−t)∣X=x)ψ(x)]2/5n2/5ifx∈(0,1),[2Δ2(x)f(x)Var(ρ(Y−t)∣X=x)]1/3n1/3ifx=0,1. |
Then,
MSE[ˆrn,mopt(x,t)]={5(Δ(x))2/5(Var(ρ(Y−t)∣X=x)ψ(x))4/5(4f(x))4/5n−4/5+o(n−4/5)ifx∈(0,1),3(Δ(x)Var(ρ(Y−t)∣X=x))2/3(2f(x))2/3n−2/3+o(n−2/3)ifx=0,1. |
Theorem 3.1. Under conditions of Proposition 3.1, we have
ˆθxP⟶n→+∞θx. |
Proposition 3.2. Let Assumptions (H1)–(H7) hold.
1) For x∈(0,1), we have:
i) If nm−5/2n⟶n→+∞c for some constant c≥0, then
n1/2m−1/4n(ˆrn(x,t)−r(x,t))D⟶n→+∞N(√cΔ(x),Var(ρ(Y−t)∣X=x)f(x)ψ(x)). | (3.4) |
ii) If nm−5/2n⟶n→+∞∞, then
mn(ˆrn(x,t)−r(x,t))P⟶n→+∞Δ(x). | (3.5) |
2) For x={0,1}, we have:
i) If nm−3n⟶n→+∞c for some constant c≥0, then
√nm(ˆrn(x,t)−r(x,t))D⟶n→+∞N(√cΔ(x),Var(ρ(Y−t)∣X=x)f(x)). | (3.6) |
ii) If nm−3n⟶n→+∞∞, then
mn(ˆrn(x,t)−r(x,t))P⟶n→+∞Δ(x), | (3.7) |
where D→n→+∞ denotes the convergence in distribution, N the Gaussian distribution, and P→n→+∞ the convergence in probability.
Theorem 3.2. (The Mean Integrated Squared Error (MISE) of ˆrn).
Let Assumptions (H1)–(H7) hold. Then, we have
MISE(ˆrn)=Λ1m2n+Λ2m1/2nn+o(m1/2nn)+o(m−2n). | (3.8) |
Hence, the asymptotically optimal choice of m is
mopt=[4Λ1Λ2]2/5n2/5, |
for which we get
MISE(ˆrn,mopt)=5Λ1/51Λ4/5244/5n−4/5+o(n−4/5). |
Theorem 3.3. Assume that (H1)–(H7) hold. If Γ(x,θx)=E[ρ′(Y−θx)|X=x]≠0, then ˆθx exists and is unique with great probability, and we have:
i) when x∈(0,1) and mn is chosen such that nm−5/2n→0, then
n1/2m−1/4n(ˆθx−θx)D→N(√cΔ(x)Γ(x,θx),σ21(x,θx)), |
ii) when x∈[0,1] and mn is chosen such that nm−3n→0, then
√nmn(ˆθx−θx)D→N(√cΔ(x)Γ(x,θx),σ22(x,θx)), |
where
σ21(x,θx)=Var[ρ(Y−θx)|X=x]f(x)Γ2(x,θx)ψ(x),σ22(x,θx)=Var[ρ(Y−θx)|X=x]f(x)Γ2(x,θx), |
D→n→+∞ denotes the convergence in distribution, and N the Gaussian distribution.
The following corollary directly follows from the previous theorem and provides the weak convergence rate of the estimator ˆθx for x∈[0,1], where f(x)>0. This is specifically for the case when mn is chosen such that nm−5/2n→0 for x∈(0,1) and nm−3n→0 for x∈[0,1].
Corollary 3.1. When x∈(0,1) and mn is chosen such that nm−5/2n→0, then
n1/2m−1/4n(ˆθx−θx)D→N(0,σ21(x,θx)). |
When x∈[0,1] and mn is chosen such that nm−3n→0, then
√nmn(^θx−θx)D→N(0,σ22(x,θx)), |
where
σ21(x,θ)=Var[ρ(Y−θx)|X=x]f(x)Γ2(x,θx)ψ(x),σ22(x,θx)=Var[ρ(Y−θx)|X=x]f(x)Γ2(x,θx). |
This section is divided into two parts: the first shows our estimate's behavior for some particular conditional regression functions, and the second deals with asymptotic normality.
Consider the regression model
Y=r(X)+ε, |
where ε∼N(0,1).
A simulation was conducted to compare the proposed estimators ˆθx (robust Bernstein polynomial estimator) with ˆrBNWn(x) (Bernstein-Nadaraya-Watson estimator) introduced by Slaoui and Jmaei [28] and defined by
ˆrBNWn(x)=∑ni=1Yi∑mn−1k=0I{kmn<Xi≤k+1mn}Bk(mn−1,x)∑ni=1∑mn−1k=0I{kmn<Xi≤k+1mn}Bk(mn−1,x), | (4.1) |
where Bk(m,x)=(mk)xk(1−x)m−k is the Bernstein polynomial of order m, and ˆrNWn(x) (Nadaraya-Watson estimator) is defined, for x∈R such that f(x)≠0, by
ˆrNWn(x)=∑ni=1YiK(x−Xih)∑ni=1K(x−Xih), | (4.2) |
where K:R→R is a nonnegative, continuous, bounded function satisfying ∫RK(z)dz=1,∫RzK(z)dz=1 and ∫Rz2K(z)dz<∞ and h=(hn) is a sequence of positive real numbers that goes to zero.
When using the estimator ˆrNWn(x), we choose the Gaussian kernel K(x)=(2π)−1/2exp(−x2/2) and the bandwidth equal to (hn)=m−1n.
We consider three sample sizes n=20,n=100, and n=500, four regression functions
Yi=−2Xi+5+εilinear case,Yi=2X2i−1+εiparabolic case,Yi=sin(32Xi)+εisine case,Yi=exp(2Xi−3)+εiexponential case, |
and three densities of X: the truncated standard normal density N[0,1](0,1) (X∈[0,1]), the exponential density Exp(2) (X∈[0,∞)), and the standard normal density N(0,1) (X∈(−∞,∞)). It is also possible to use the transformations ˜X=X1+X or ˜X=12+1πtan−1(X) to cover the cases of random variables X with support R+ and R, respectively. These transformations allow for the application of Bernstein polynomials to smooth the empirical distribution function.
The simulation consists of four parts. In the first three parts, the estimators are compared by their average integrated squared error ¯AISE. Every ¯AISE is calculated by a Monte-Carlo simulation with N=1000 repetitions of sample size n,
¯AISE=1NN∑k=1ISE[ˉrk], |
where ˉrk is the estimator (ˆθx or ˆrBNWn(x) or ˆrNWn(x)) computed from the kth sample, and
ISE[ˉrk]=∫10{ˉr(x)−r(x)}2dx. |
According to Figures 1–4, it is evident that the robust Bernstein polynomial estimation converges when n is large. This is observed in all cases.
The ¯AISE of three estimators is graphed in Figure 5 for different parameter values ranging from 1 to 200. The estimators are evaluated for two sample sizes, n=20 and n=500. The outcomes are highly comparable when outlier values are not present. Nevertheless, the analysis of Tables 1–4 demonstrates that both the kernel estimator and the Bernstein-Nadaraya-Watson estimator exhibit significant sensitivity towards outlier values. This heightened sensitivity leads to substantial inaccuracies in predictions. In contrast, our robust Bernstein polynomial estimator consistently sustains its performance irrespective of the quantity of outlier values.
Density of X | Outlier rate | n=20 | n=100 | n=500 | ||||||
ˆrBNWn(x) | ˆθx | ˆrNWn(x) | ˆrBNWn(x) | ˆθx | ˆrNWn(x) | ˆrBNWn(x) | ˆθx | ˆrNWn(x) | ||
(a) N[0,1](0,1) |
0.00% | 0.37777 | 0.38362 | 0.37289 | 0.0386 | 0.04134 | 0.0333 | 0.01564 | 0.01684 | 0.00896 |
0.05% | 598.916 | 3.57632 | 690.548 | 678.998 | 2.20528 | 668.378 | 674.569 | 0.18818 | 692.737 | |
0.10% | 3016.57 | 5.65957 | 3000.05 | 2620.97 | 3.16347 | 2593.3 | 2676.12 | 0.23244 | 2682.89 | |
0.25% | 16083.1 | 14.182 | 15878.1 | 15896.3 | 6.29712 | 15930.6 | 16447.1 | 1.74161 | 16344.1 | |
(b) Exp(2) |
0.00% | 0.35574 | 0.35578 | 0.35517 | 0.05012 | 0.05283 | 0.03747 | 0.01611 | 0.01549 | 0.00794 |
0.05% | 748.855 | 4.2097 | 819.161 | 689.539 | 1.90398 | 692.123 | 683.571 | 0.21493 | 644.829 | |
0.10% | 2408.65 | 5.93149 | 2284.35 | 2501.28 | 3.57741 | 2432.78 | 2681.96 | 0.31808 | 2586 | |
0.25% | 16174.5 | 21.0217 | 16094.6 | 16228.2 | 6.62117 | 16834.3 | 17422.6 | 1.89746 | 17294.2 | |
(c) N(0,1) |
0.00% | 0.3345 | 0.33847 | 0.31945 | 0.05094 | 0.04832 | 0.03983 | 0.01667 | 0.01593 | 0.00886 |
0.05% | 770.807 | 4.51064 | 822.317 | 675.495 | 2.05097 | 665.198 | 698.8 | 0.15089 | 656.339 | |
0.10% | 2746.52 | 7.79586 | 2559.23 | 2436.1 | 3.05173 | 2393.47 | 2497.94 | 0.24955 | 2503.83 | |
0.25% | 19178.1 | 18.1898 | 18006 | 16413.5 | 8.0909 | 16893.9 | 17372.6 | 1.75941 | 17495.9 |
Density of X | Outlier rate | n=20 | n=100 | n=500 | ||||||
ˆrBNWn(x) | ˆθx | ˆrNWn(x) | ˆrBNWn(x) | ˆθx | ˆrNWn(x) | ˆrBNWn(x) | ˆθx | ˆrNWn(x) | ||
(a) N[0,1](0,1) |
0.00% | 1.48199 | 1.48019 | 1.47376 | 0.16874 | 0.25191 | 0.10863 | 0.04375 | 0.05576 | 0.02462 |
0.05% | 29.1125 | 2.49147 | 29.3273 | 14.8531 | 0.74622 | 16.0768 | 15.7507 | 1.66571 | 22.1559 | |
0.10% | 64.0281 | 2.90274 | 73.1002 | 53.5638 | 0.91596 | 49.9706 | 98.0555 | 0.90805 | 67.9633 | |
0.25% | 393.474 | 6.88132 | 326.212 | 1050.14 | 3.46544 | 700.888 | 1100.17 | 0.99655 | 751.459 | |
(b) Exp(2) |
0.00% | 1.39141 | 1.47957 | 1.33418 | 0.17829 | 0.22055 | 0.11882 | 0.05203 | 0.05178 | 0.02936 |
0.05% | 25.489 | 2.38298 | 28.3225 | 13.7623 | 0.54491 | 14.1639 | 31.6953 | 1.13268 | 11.5467 | |
0.10% | 71.5908 | 2.58522 | 75.7357 | 50.8943 | 1.02112 | 58.2273 | 114.747 | 1.50033 | 54.9639 | |
0.25% | 355.306 | 6.60588 | 289.937 | 867.431 | 2.26394 | 454.397 | 1327.96 | 0.47757 | 835.553 | |
(c) N(0,1) |
0.00% | 0.98856 | 1.05261 | 0.97223 | 0.16172 | 0.18081 | 0.09312 | 0.03957 | 0.04478 | 0.02101 |
0.05% | 25.528 | 2.25141 | 32.5343 | 22.6682 | 0.5839 | 15.2797 | 24.7481 | 1.48634 | 15.3258 | |
0.10% | 61.7528 | 2.64854 | 85.8806 | 75.1123 | 1.22781 | 45.4987 | 111.539 | 1.81279 | 64.8843 | |
0.25% | 469.185 | 9.35168 | 398.637 | 692.756 | 3.46427 | 642.386 | 1131.51 | 0.6867 | 526.238 |
Density of X | Outlier rate | n=20 | n=100 | n=500 | ||||||
ˆrBNWn(x) | ˆθx | ˆrNWn(x) | ˆrBNWn(x) | ˆθx | ˆrNWn(x) | ˆrBNWn(x) | ˆθx | ˆrNWn(x) | ||
(a) N[0,1](0,1) |
0.00% | 0.13301 | 0.12525 | 0.1154 | 0.01527 | 0.01522 | 0.01269 | 0.00414 | 0.00436 | 0.00316 |
0.05% | 19.3998 | 0.28717 | 20.5557 | 12.0525 | 0.12604 | 10.3004 | 9.47988 | 0.1339 | 8.91102 | |
0.10% | 58.565 | 0.52241 | 49.1399 | 33.1922 | 0.23475 | 34.4606 | 38.2786 | 0.16123 | 43.7728 | |
0.25% | 294.817 | 2.25567 | 177.939 | 266.142 | 0.54954 | 210.994 | 249.554 | 0.30488 | 273.319 | |
(b) Exp(2) |
0.00% | 0.14836 | 0.15054 | 0.12541 | 0.01324 | 0.0144 | 0.01196 | 0.0055 | 0.00545 | 0.00438 |
0.05% | 18.8191 | 0.2807 | 25.6982 | 10.7864 | 0.14311 | 9.74908 | 10.5176 | 0.18333 | 9.51805 | |
0.10% | 55.1011 | 0.45925 | 48.4941 | 44.8719 | 0.18583 | 33.2084 | 40.9161 | 0.17936 | 39.3012 | |
0.25% | 234.994 | 1.24542 | 189.692 | 251.89 | 0.60327 | 234.829 | 261.372 | 0.34955 | 285.042 | |
(c) N(0,1) |
0.00% | 0.13021 | 0.14029 | 0.12257 | 0.01506 | 0.01511 | 0.01375 | 0.00442 | 0.00442 | 0.00328 |
0.05% | 23.6259 | 0.28918 | 22.6131 | 12.1443 | 0.10116 | 10.529 | 9.82171 | 0.15066 | 9.71418 | |
0.10% | 56.098 | 0.4286 | 55.5514 | 36.7151 | 0.22296 | 36.6241 | 35.7651 | 0.1501 | 40.4612 | |
0.25% | 247.54 | 1.20312 | 237.361 | 224.049 | 0.50768 | 235.812 | 246.816 | 0.30212 | 276.141 |
Density of X | Outlier rate | n=20 | n=100 | n=500 | ||||||
ˆrBNWn(x) | ˆθx | ˆrNWn(x) | ˆrBNWn(x) | ˆθx | ˆrNWn(x) | ˆrBNWn(x) | ˆθx | ˆrNWn(x) | ||
(a) N[0,1](0,1) |
0.00% | 0.74703 | 0.61209 | 0.56581 | 0.17137 | 0.17318 | 0.1214 | 0.10734 | 0.08411 | 0.01222 |
0.05% | 1.98561 | 1.35548 | 1.86444 | 1.56581 | 0.60637 | 1.98072 | 5.36892 | 0.08762 | 3.43111 | |
0.10% | 5.07457 | 1.236 | 4.75581 | 7.88123 | 0.43664 | 7.38618 | 27.4856 | 0.11352 | 12.9594 | |
0.25% | 34.2112 | 2.53764 | 30.5081 | 141.657 | 1.78744 | 166.463 | 366.283 | 0.32839 | 243.809 | |
(b) Exp(2) |
0.00% | 0.52866 | 0.5851 | 0.47474 | 0.10082 | 0.13134 | 0.05472 | 0.03985 | 0.07932 | 0.0121 |
0.05% | 1.79819 | 1.06047 | 1.9109 | 1.31466 | 0.45844 | 1.7421 | 4.25689 | 0.07832 | 4.11877 | |
0.10% | 3.47787 | 1.33077 | 4.26777 | 10.9025 | 0.44565 | 11.0732 | 46.7737 | 0.09391 | 31.0859 | |
0.25% | 66.6146 | 1.7682 | 51.3565 | 196.824 | 1.64135 | 113.157 | 351.074 | 0.30732 | 317.418 | |
(c) N(0,1) |
0.00% | 0.74933 | 0.71865 | 0.5849 | 0.11883 | 0.19364 | 0.11473 | 0.10082 | 0.11145 | 0.01105 |
0.05% | 1.20693 | 0.61275 | 1.51455 | 1.40167 | 0.15919 | 1.27418 | 7.52656 | 0.0597 | 3.79191 | |
0.10% | 3.85005 | 1.01982 | 3.50089 | 14.9906 | 0.41717 | 10.9088 | 39.9271 | 0.11825 | 36.1256 | |
0.25% | 26.8219 | 2.48908 | 25.5696 | 145.728 | 1.13974 | 123.627 | 422.568 | 0.33036 | 202.644 |
The objective is to demonstrate the property of asymptotic normality in the context of the sine regression model. The equation is
Yi=sin(32Xi)+εi. |
Next, let r(x) be defined as the sine function with a coefficient of 32. The data provided is the same as in the previous subsection. The procedure consists of the following steps: We approximate the regression function r(x) using ˆθx0 and compute the normalized deviation between this approximation and the theoretical regression function (refer to Theorem 3.3) for x0=0,0.5and1. Under this scheme, we generate N separate sets of n samples that are not influenced by each other. Next, we analyze the form of the estimated density (with normalized deviation) and compare it to the shape of the standard normal density in the context of the sine regression model. The following figures and table present the density of ˆθx0 as well as the p−value by the Shapiro-Wilk normality test. We examine various values of n, specifically n=20, n=100, and n=500.
Figures 6–8 and Table 5 demonstrate the advantageous characteristics of our asymptotic law compared to the standard normal distribution.
n=20 | n=100 | n=500 | |
x0=0 | 0.0814 | 0.0968 | 0.1728 |
x0=0.5 | 0.5299 | 0.5734 | 0.6603 |
x0=1 | 0.0611 | 0.0702 | 0.0970 |
Air pollution significantly affects the lives of individuals in developed nations. The source of this issue is increased levels of smoke produced by industries or vehicles, prompting authorities to search for more efficient methods to regulate air quality in real-time. London is experiencing a significant problem with air pollution exceeding legal and World Health Organisation limits. An example of this is the incident in 2010 when air pollution caused various health problems in the city, leading to a financial cost of around £3.7 billion.
This segment analyzes the mean daily levels of gases detected at the Marylebone Road monitoring station in London. The dataset includes the average daily measurements recorded throughout 2022 for five important variables: Ozone (O3), Nitric Oxides (NO), Nitrogen Dioxide (NO2), Sulphur Dioxide (SO2), and Particulate Matter (PM10). The main objective of our research is to determine the most practical forecasting models for air pollutant concentration. The data used in this analysis was obtained from the specified website: https://www.airqualityengland.co.uk/site/data?site_id = MY1.
To ensure clarity, let us delineate the mathematical expression representing our prediction objective. Let us consider predicting the daily air pollutant concentration, represented by the variable Y, for 365 days, denoted by X. Formally, we assume that the output variable Y and the input variable X are connected by the following equation:
Yi=r(Xi)+εifor i∈{1,…,n}. |
A dependable data-dependent rule for order selection is crucial when estimating an unknown regression function in any practical scenario. A widely used and effective method is cross-validation:
CV(m)=1nn∑i=1(Yi−ˉr−i(Xi))2, |
where ˉr−i is the regression estimate without the data point (Xi,Yi).
In practice, choosing the right degree m for a Bernstein polynomial requires balancing between the complexity of the model and how well it fits the data. A useful method for this is cross-validation, where the dataset is divided into training and validation sets.
Then, the smoothing parameter is chosen by minimizing
CV(m)=1nn∑i=1(Yi−ˉr−i(Xi))2. |
For convenience, we assume that the minimum of days is 1 and the maximum is 365 (the day data are such that mini(Xi)=1 and maxi(Xi)=365). Finally, we used the cross-validation method to obtain the results in Figures 9–13 and Table 6.
Ozone | Nitric Oxides | Nitrogen Dioxide | Sulphur Dioxide | Particulate Matter | |
ˆrBNWn(x) | 181 | 169 | 197 | 197 | 197 |
ˆθx | 121 | 149 | 101 | 173 | 181 |
Based on the analysis of Figures 9 to 13, it is evident that the two estimators are nearly identical, except for the scenario depicted in Figure 10. In this case, non-robust estimator ˆrBNWn(x) is found to be sensitive to outliers, which provides evidence of the efficiency of our estimator.
Based on the information in Table 6, we can infer that the parameter m can be adjusted. It does not need to be equal to n. Instead, we can choose a lower-degree polynomial to achieve a more favorable outcome.
In this paper, we proposed a new robust regression estimator based on the Bernstein polynomials. Our contribution extends the work of Slaoui and Jmaei [28] to the case of robust regression. The asymptotic properties of this estimator were established. Afterward, we validated the effectiveness of the proposed method through a simulation study and applied it to real data on air pollution,
We found that, in all three models, the average ISE of our robust regression estimator ˆθx, defined in 2.4, was the smallest. We also noted that the robust regression provided better results than the non-robust method when outliers were present, in the sense that, even if the sample size increases, the average ISE decrease. To conclude, the use of the robust regression estimator with Bernstein polynomials successfully addressed the edge problem, yielding results comparable to those of non-robust and Nadaraya-Watson estimators in the absence of outliers.
We believe our research provides a foundational step that can be further developed and expanded. It sets the stage for future work to extend our robust regression estimator using the Bernstein polynomial by considering the interest random variable to be truncated. We also plan to work on the robust regression estimation using Lagrange polynomials.
Sihem Semmar: Conceptualization, data curation, formal analysis, investigation, methodology, software, validation, writing original draft, writing – review & editing; Omar Fetitah, Salah Khardani and Mohammad Kadi Attouch: Conceptualization, supervision, writing–review & editing; Mohammed Kadi Attouch and Ibrahim M. Almanjahie: Resources, validation, writing–review & editing. All authors have read and approved the final version of the manuscript for publication.
The real data used in this application can be found at this link: https://www.airqualityengland.co.uk/site/data?site_id = MY1
The authors thank and extend their appreciation to the funder of this work. This work was supported by the Deanship of Scientific Research and Graduate Studies at King Khalid University through the Large Research Groups Project under grant number R.G.P. 2/338/45.
The authors declare that they have no known competing financial interests or personal relationships that could have appeared to influence the work reported in this paper.
In this section, we present proofs for the results in Section 3. First, we recall a series of results, which are proven in Leblanc [26], linked to different sums of Bernstein polynomial, defined by
Smn(x)=mn−1∑k=0B2k(mn,x). |
These results are given in the following lemma.
Lemma A.1. We have
(i) 0≤Smn(x)≤1,∀x∈[0,1].
(ii) Smn(x)=m−1/2[ψ(x)+ox(1)],∀x∈(0,1).
(iii) Smn(0)=Smn(1)=1.
(iv) Let g be any continuous function on [0,1]. Then, m1/2n∫10g(x)Smn(x)dx=∫10g(x)ψ(x)dx+o(1).
Proof. The proof of this lemma is in Leblanc [26] and Babu et al. [12].
Lemma A.2.
E[Nn(x,t)]−N(x,t)=Δ2(x)m−1n+o(m−1n). | (A.1) |
Proof.
E[Nn(x,t)]=mnE[ρ(Y−t)mn−1∑k=0I{kmn<X≤k+1mn}Bk(mn−1,x)]=mnmn−1∑k=0∫k+1mnkmn(∫Rρ(y−t)g(z,y)dy)dzBk(mn−1,x)=mnmn−1∑k=0(∫k+1mnkmnr(z,t)f(z)dz)Bk(mn−1,x). |
Using a Taylor expansion, we have
r(z,t)f(z)=[r(x,t)+(z−x)∂r∂z(x,t)+(z−x)22∂2r∂z2(x,t)+o((z−x)2)]×[f(x)+(z−x)f′(x)+(z−x)22f′′(x)+o((z−x)2)]=r(x,t)f(x)+(z−x)[∂r∂z(x,t)f(x)+r(x,t)f′(x)]+(z−x)22[∂2r∂z2(x,t)f(x)+f′′(x)r(x,t)+2∂r∂z(x,t)f′(x)]+o((z−x)2), |
and since N(x,t)=r(x,t)f(x), we obtain
E[Nn(x,t)]=r(x,t)f(x)mnmn−1∑k=0(k+1mn−kmn)Bk(mn−1,x)+(∂r∂x(x,t)f(x)+f′(x)r(x,t))mn2mn−1∑k=0{(k+1mn−x)2−(kmn−x)2}Bk(mn−1,x)+(f′(x)∂r∂x(x,t)+f(x)∂2r∂x2(x,t)+f′′(x)r(x,t))mn6mn−1∑k=0{(k+1mn−x)3−(kmn−x)3}Bk(mn−1,x)=N(x,t)+(∂r∂x(x,t)f(x)+f′(x)r(x,t))mn2mn−1∑k=0m−2n(2k+1−2mnx)Bk(mn−1,x)+(2f′(x)∂r∂x(x,t)+f(x)∂2r∂x2(x,t)+f′′(x)r(x,t))mn6mn−1∑k=0m−3n{(k+1−mnx)2+(k−mnx)2+(k+1−mnx)(k−mnx)}Bk(mn−1,x)[1+o(1)]=N(x,t)+(∂r∂x(x,t)f(x)+f′(x)r(x,t))m−1n2{2T1,mn−1(x)+(1−2x)T0,mn−1(x)}+(2f′(x)∂r∂x(x,t)+f(x)∂2r∂x2(x,t)+∂2f∂x2(x)r(x,t))m−2n6mn−1∑k=0{3(k−mnx)2+3(k−mnx)+1}Bk(mn−1,x)[1+o(1)]=N(x,t)+(∂r∂x(x,t)f(x)+f′(x)r(x,t))m−1n2{2T1,mn−1(x)+(1−2x)T0,mn−1(x)}+(2f′(x)∂r∂x(x,t)+f(x)∂2r∂x2(x,t)+f′′(x)r(x,t))m−2n6{3T2,mn−1(x)+3(1−2x)T1,mn−1(x)+(x2−3x+1)T0,mn−1(x)}[1+o(1)], |
where Tj,mn−1(x) are the central moments of the Binomial distribution of order j∈N, defined as
Tj,mn−1(x)=mn−1∑k=0(k−mnx)jBk(mn−1,x),∀j∈N. |
Note that it is easy to obtain
T0,mn−1(x)=1,T1,mn−1(x)=0T2,mn−1(x)=(mn−1)x(1−x). |
Then, we have
E[Nn(x,t)]=N(x,t)+Δ2(x)m−1n+o(m−1n). | (A.2) |
Lemma A.3. We have
Var[Nn(x,t)]={m1/2nnE[(ρ(Y−t))2∣X=x]f(x)ψ(x)+ox(m3/2nn)forx∈(0,1),mnnE[(ρ(Y−t))2∣X=x]f(x)+ox(mnn)forx=0,1. |
Proof. We have
Var[Nn(x,t)]=E[N2n(x,t)]−E2[Nn(x,t)], |
where
N2n(x,t)=m2nn2n∑i=1(ρ(Yi−t))2(mn−1∑k=0I{kmn<Xi≤k+1mn}Bk(mn−1,x))2+m2nn2mn∑i,j=1,i≠jρ(Yi−t)ρ(Yj−t)(mn−1∑k=0I{kmn<Xi≤k+1mn}Bk(mn−1,x))(mn−1∑k=0I{kmn<Xj≤k+1mn}Bk(mn−1,x)). |
So, we have
E[N2n(x,t)]=m2nnE[(ρ(Y−t))2(mn−1∑k=0I{kmn<X≤k+1mn}Bk(mn−1,x))2]+m2nn(n−1)n2E2[mn−1∑k=0I{kmn<X≤k+1mn}Bk(mn−1,x)]=mnnE[(ρ(Y−t))2(mn−1∑k=0I{kmn<X≤k+1mn}Bk(mn−1,x))2]+(1−1n)E2[Nn(x,t)], |
and
Var[Nn(x,t)]=m2nnE[(ρ(Y−t))2(mn−1∑k=0I{kmn<X≤k+1mn}Bk(mn−1,x))2]−1nE2[Nn(x,t)]=m2nnE[(ρ(Y−t))2mn−1∑k=0I{kmn<X≤k+1mn}B2k(mn−1,x)]−1nE2[Nn(x,t)]=m2nnmn−1∑k=0∫k+1mnkmn(∫R(ρ(y−t))2g(z,y)dy)dzB2k(mn−1,x)−1nE2[Nn(x,t)]=m2nnmn−1∑k=0(∫k+1mnkmnE[(ρ(Y−t))2∣X=z]f(z)dz)B2k(mn−1,x)−1nE2[Nn(x,t)]=mnnE[(ρ(Y−t))2∣X=x]f(x)Smn(x)−1nE2[Nn(x,t)]. |
Using Lemma A.1 (ⅱ) and (ⅲ), we obtain
Var [Nn(x,t)]={m1/2nnE[(ρ(Y−t))2∣X=x]f(x)ψ(x)+ox(m3/2nn) for x∈(0,1),mnnE[(ρ(Y−t))2∣X=x]f(x)+ox(mnn) for x=0,1. | (A.3) |
Lemma A.4.
Cov(fn(x),Nn(x,t))={m1/2nnr(x,t)f(x)ψ(x)+ox(m1/2nn)forx∈(0,1),mnnr(x,t)f(x)+ox(mnn)forx=0,1. | (A.4) |
Proof. We have
Cov(fn(x),Nn(x,t))=E[fn(x)Nn(x,t)]−E[fn(x)]E[Nn(x,t)]=m2nnE[ρ(Y−t)(mn−1∑k=0I{kmn<X≤k+1mn}Bk(mn−1,x))2]+n(n−1)m2nn2E2[ρ(Y−t)mn−1∑k=0I{kmn<X≤k+1mn}Bk(mn−1,x)]−E[fn(x)]E[Nn(x,t)]=m2nnE[ρ(Y−t)(mn−1∑k=0I{kmn<X≤k+1mn}Bk(mn−1,x))2]−1nE[fn(x)]E[Nn(x,t)]=m2nnmn−1∑k=0∫k+1mnkmn(∫Rρ(y−t)g(z,y)dy)dzB2k(mn−1,x)−1nE[fn(x)]E[Nn(x,t)]=mnnr(x,t)f(x)Sm(x)−1nE[fn(x)]E[Nn(x,t)]. |
Using Lemma A.1 (ⅱ) and (ⅲ), we get
Cov(fn(x),Nn(x))={m1/2nnr(x)f(x)ψ(x)+ox(m1/2nn) for x∈(0,1),mnnr(x)f(x)+ox(mnn) for x=0,1. | (A.5) |
To obtain the bias of ˆrn(x,t), we let h(x,y)=uv. Using a Taylor expansion, we have
h(u,v)=h(u0,v0)+[u−u0]∂h∂u(u0,v0)+[v−v0]∂h∂v(u0,v0)+12{[u−u0]2∂2h∂u2(u0,v0)+[v−v0]2∂2q∂v2(u0,v0)}+2[u−u0][v−v0]∂2h∂u∂v(u0,v0)+o(‖(u−u0,v−v0)‖2). |
Then, we have
uv=u0v0+1v0(u−u0)−u0v20(v−v0)+u0v30(v−v0)2−1v20(u−u0)(v−v0)+o((u−u0)2+(v−v0)2). |
We set (u,v)=(Nn(x,t),fn(x)) and (u0,v0)=(N(x,t),f(x)). Therefore, we infer that
Nn(x,t)fn(x)=N(x,t)f(x)+1f(x)(Nn(x,t)−N(x,t))−N(x,t)f(x)2(fn(x)−f(x)(x))+N(x,t)f(x)3(fn(x)−f(x))2−1f(x)2(Nn(x,t)−N(x,t))(fn(x)−f(x)))+o((Nn(x,t)−N(x,t))2+(fn(x)−f(x))2).ˆrn(x,t)=r(x,t)+1f(x)(Nn(x,t)−N(x,t))−r(x,t)f(x)(fn(x)−f(x))+r(x,t)f(x)2(fn(x)−f(x))2−1f(x)2(Nn(x,t)−N(x,t))(fn(x)−f(x))+o((Nn(x,t)−N(x,t))2+(fn(x)−f(x))2). |
Hence, we set (u,v)=(fn(x),Nn(x,t)) and (u0,v0)=(f(x),N(x,t)) to obtain
ˆrn(x,t)=r(x,t)−r(x,t)f(x)(fn(x)−f(x))+1f(x)(Nn(x,t)−N(x,t))+r(x,t){f(x)}2(fn(x)−f(x))2−1{f(x)}2(fn(x)−f(x))(Nn(x,t)−N(x,t))+o((fn(x)−f(x))2+(fn(x)−f(x))(Nn(x,t)−N(x,t))). |
Then,
E[ˆrn(x,t)]=r(x,t)−r(x,t)f(x)(E[fn(x)]−f(x))+1f(x)(E[Nn(x,t)]−N(x,t))+r(x,t){f(x)}2(E[fn(x)]−f(x))2−1{f(x)}2E[(fn(x)−f(x))(Nn(x,t)−N(x,t))]+o(E[(fn(x)−f(x))2]+E[(fn(x)−f(x))(Nn(x,t)−N(x,t))]). |
Use Vitale's estimator fn, we get
E[fn(x)]=f(x)+Δ1(x)mn+o(m−1n),∀x∈[0,1] | (A.6) |
and
Var[fn(x)]={m1/2nnf(x)ψ(x)+ox(m1/2nn) for x∈(0,1),mnnf(x)+ox(mnn) for x=0,1. | (A.7) |
To obtain (3.1) of Proposition 3.1, we use (A.6) and (A.2) to obtain
E[ˆrn(x,t)]=r(x,t)+(1f(x)Δ2(x)−r(x,t)f(x)Δ1(x))m−1n+o(m−1n)=r(x,t)+Δ(x)m−1n+o(m−1n),∀x∈[0,1]. |
Now for the variance of ˆrn(x,t), we have
Var(ˆrn(x,t))=Var(r(x,t)−r(x,t)f(x)(fn(x)−f(x))+1f(x)(Nn(x,t)−N(x,t)))[1+o(1)], |
which ensures that
Var(ˆrn(x,t)){r2(x,t)f2(x)Var(fn(x))+1f2(x)Var(Nn(x,t))−2r(x,t)f2(x)Cov(Nn(x,t),fn(x))}[1+o(1)]. |
So, for x=(0,1), we have f,
Var[ˆrn(x,t)]=m1/2nnVar(ρ(Y−t)∣X=x)f(x)+ox(m1/2nn), |
and, for x∈0,1, we have
Var[ˆrn(x,t)]=mnnVar(ρ(Y−t)∣X=x)f(x)+ox(mnn), |
which gives the proof of Proposition 3.1.
Without loss of generality we can suppose that ρ(Y−.) is increasing, with the decreasing case being obtained by considering −ρ(Y−.). As ρ(Y−.) is increasing, then for all ϵ>0,
r(x,θx.+ϵ)≤r(x,θx)≤r(x,θx−ϵ). |
Proposition 3.1 shows that
ˆr(x,t)P→r(x,t), |
for all real t∈[θx−τ,θx+τ]. As r(x,θx)=0, for sufficiently large n and for all ϵ≤τ, this implies
ˆr(x,θx+ϵ)≤0≤ˆr(x,θx−ϵ)in probability. |
Since ˆr(x,ˆθx)=0, and by the continuity of ˆr(x,.) on [θx−τ,θx+τ], we deduce that
θx−ϵ≤^θx≤θx+ϵin probability. |
On the other hand, since θx and ^θx are solutions of r(x,t) and ˆr(x,t), respectively, then we have
ˆr(x,^θx)=r(x,θx)=0. |
Under (H7), and by a Taylor expansion of r(x,.) of order one around ˆθx, we have
ˆr(x,ˆθx)−r(x,ˆθx)=(θx−ˆθx)∂r∂t(x,ξn), |
where ξn is between θx and ^θx. Hence,
|θx−^θx|≤1|infx∈S∂r∂t(x,ξn)||ˆr(x,^θx)−r(x,^θx)|, |
which yields
supx∈S|θx−^θx|≤1C3supx∈S|ˆr(x,^θx)−r(x,^θx)|≤1C3supx∈Ssupt∈[θx−τ,θx+τ]|ˆr(x,t)−r(x,t)|, |
and the rest of the proof is a sequence of Proposition 3.1.
From (2.4), we adopt the decomposition stated as
ˆrn(x,t)−r(x,t)=1fn(x)[(Nn(x,t)−N(x,t))−r(x,t)(fn(x)−f(x))]=1fn(x)[(Nn(x,t)−E(Nn(x,t)))−r(x,t)(fn(x)−E(fn(x))))]+1fn(x)[(E(Nn(x,t))−N(x,t))−r(x,t)(E(fn(x))−f(x))]. |
Lemma A.5. Under Assumptions (H1)–(H3), and for x∈[0,1] such that f(x)>0, we have
fn(x)P→f(x). | (A.8) |
Proof. We have by the results of Lemmas A.2 and A.3, that
E(fn(x))−f(x)→0, |
and
Var(fn(x))→0. |
Hence,
fn(x)P→f(x),∀x∈(0,1). |
Lemma A.6. Under Assumptions (H1)–(H4), and for x∈(0,1) such that f(x)>0, we have:
i) if mn is chosen such that nm−5/2n→c for some constant c≥0, then
n1/2m−1/4nfn(x)[(E(Nn(x,t))−N(x,t))−r(x,t)(E(fn(x))−f(x))]P→√cΔ(x), | (A.9) |
ii) if mn is chosen such that nm−5/2n→∞, then
mnfn(x)[(E(Nn(x,t))−N(x,t))−r(x,t)(E(fn(x))−f(x))]P→Δ(x). | (A.10) |
Proof. By Lemmas A.2 and A.8, we have:
i) if nm−5/2n→c for some constant c≥0, then
n1/2m−1/4nfn(x)[(E(Nn(x,t))−N(x,t))−r(x,t)(E(fn(x))−f(x))]=n1/2m−5/4n(Δ1(x)−r(x,t)Δ2(x)+o(1))fn(x)P→√cΔ(x), |
ii) if nm−5/2n→∞, then
mnfn(x)[(E(Nn(x,t))−N(x,t))−r(x,t)(E(fn(x))−f(x))]=(Δ1(x)−r(x,t)Δ2(x)+o(1))fn(x)P→Δ(x). |
Lemma A.7. Under Assumptions (H1)–(H4), and for x∈(0,1) such that f(x)>0, we have
n1/2m−1/4n[(Nn(x,t)−E(Nn(x,t)))−r(x,t)(fn(x)−E(fn(x)))]D→N(0,Var(ρ(Y−t)∣X=x)f(x)ψ(x)). | (A.11) |
Proof. We write
n1/2m−1/4n[(Nn(x,t)−E(Nn(x,t)))−r(x,t)(fn(x)−E(fn(x)))]=n∑i=1(Li(x)−E(Li(x))), |
where
Li(x)=m3/4nn1/2(ρ(yi−t)−r(x,t))mn−1∑k=0I{kmn<Xi≤k+1mn}Bk(mn−1,x). |
The proof of this lemma is based on the Lyapunov central limit theorem (FELLER, W.[36]) on Li(x), i.e., it suffices to show, for some δ>0, that
∑ni=1E[|Li(x)−E[Li(x)]|2+δ](Var[∑ni=1Li(x)])(2+δ)/2⟶0. | (A.12) |
Clearly,
Var[n∑i=1Li(x)]=nm−1/2nVar[(Nn(x,t)−E(Nn(x,t)))−r(x,t)(fn(x)−E(fn(x)))]=nm−1/2n[Var(Nn(x,t))+r2(x,t)Var(fn(x))−r(x,t)Cov(Nn(x,t),fn(x))]. |
Hence,
Var[n∑i=1Li(x)]=Var(ρ(y−t)2|X=x)f(x)ψ(x)+o(1). |
Therefore, to complete the proof of this lemma, it is enough to show that the numerator of (A.12) converges to 0. For this, we use the Cr-inequality (cf. Loève [37], page 155) to show that
n∑i=1E[|Li(x)−E[Li(x)]|2+δ]≤C1n∑i=1E[|Li(x)|2+δ]+C2n∑i=1|E[Li(x)]|2+δ. |
Recall that, because of Assumption (H4) and Lemma A.1 (ⅱ), we have
n∑i=1E[|Li(x)|2+δ]=n−δ/2(mn)34δ+32[|ρ(Yi−t)−r(x,t)|2+δ(mn−1∑k=0I{kmn<Xi≤k+1mn}Bk(mn−1,x))2+δ]≤n−δ/2(mn)34δ+32mn−1∑k=0∫k+1mnkmn(21+δ∫R|ρ(Y−t)|−(2+δ)g(z,y)dy+21+δ|r(x,t)|2+δ)dzB2+δk(mn−1,x)≤n−δ/2(mn)34δ+32mn−1∑k=0CmnB2+δk(mn−1,x)≤n−δ/2(mn)34δ+32×Cm32n≤C(m32nn)δ2→0. |
Similarly, for the second term (∑ni=1|E[Li(x)]|2+δ), we get
n∑i=1|E[Li(x)]|2+δ≤C(m32nn)δ2→0. |
Finally, (A.9) in Lemma A.6, Lemma A.7, and Slutsky's theorem complete the proof of part 3.4 of Proposition 3.2.
Now, if nm−5/2n→∞, we have
mn[(Nn(x,t)−E(N(x,t)))−r(x,t)(fn(x)−E(fn(x)))]=(n−1/2m5/4n)n1/2m−1/4n[(Nn(x,t)−E(Nn(x,t)))−r(x,t)(fn(x)−E(fn(x)))]. |
Since we have n−1/2m5/4n→0, A.10 in Lemma A.6, Lemma A.7, and Slutsky's theorem complete the proof of part 3.5. Proposition 3.2 follows from (A.11) when x∈{0,1}.
Lemma A.8. Under Assumptions (H1)–(H4), and for x∈{0,1} such that f(x)>0, we have:
i) if mn is chosen such that nm−3n→c for some constant c≥0, then
n1/2m−1/2nfn(x)[(E(Nn(x,t))−N(x,t))−r(x,t)(E(fn(x))−f(x))]P→√cΔ(x), | (A.13) |
ii) if mn is chosen such that nm−3n→∞, then
mnfn(x)[(E(Nn(x,t))−N(x,t)))−r(x,t)(E(fn(x))−f(x))]P→Δ(x). | (A.14) |
Proof. The proof of this lemma is analogous to Lemma A.6.
Lemma A.9. Under Assumptions (H1)–(H4), and for x∈{0,1} such that f(x)>0, we have
n1/2m−1/2n[(Nn(x,t)−E(Nn(x,t)))−r(x,t)(fn(x)−E(fn(x)))]D→N(0,Var(ρ(Yi−t))f(x)). | (A.15) |
Proof. We write
n1/2m−1/2n[(Nn(x,t)−E(Nn(x,t)))−r(x,t)(fn(x)−E(fn(x)))]=n∑i=1(Li(x)−E(Li(x))), |
where
Li(x):=m1/2nn1/2(ρ(Yi−t)−r(x,t))mn−1∑k=0I{kmn<Xi≤k+1mn}Bk(mn−1,x). |
The proof of this lemma is based on the Lyapounov central limit theorem (FELLER, W.[36]) on Li(x). Clearly,
Var[n∑i=1Li(x)]=nm−1nVar[(Nn(x,t)−E(Nn(x,t))))−r(x,t)(fn(x)−E(fn(x))))]=nm−1n[Var(Nn(x,t))+r2(x,t)Var(fn(x))−2r(x,t)Cov(Nn(x,t),fn(x))]. |
Hence,
Var[n∑i=1Li(x)]=Var(ρ(Yi−t))f(x)+o(1). |
Therefore, to complete the proof of this lemma, we follow the same steps as in the proof Lemma A.7, and find that
n∑i=1E[|Li(x)−E[Li(x)]|2+δ]≤Cm12n×(mnn)δ2→0. |
Finally, (A.13) in Lemma A.8, Lemma A.9, and Slutsky's theorem complete the proof of part 3.6 of Proposition 3.2. Now, if nm−3n→∞, we have
mn[(Nn(x,t)−E(Nn(x,t)))−r(x,t)(fn(x)−E(fn(x)))]=(n−1/2m3/2n)n1/2m−1/2n[(Nn(x,t)−E(Nn(x,t)))−r(x,t)(fn(x)−E(fn(x)))]. |
Since we have n−1/2m3/2n→0, A.14 in Lemma A.8, Lemma A.9, and Slutsky's theorem completes the proof of part 3.7 of Proposition 3.2 follows from (A.15).
First, we have
∫10Bias(ˆrn(x,t))2dx=∫10(E[ˆrn(x,t)]−r(x,t))2dx=∫10Δ2(x)m2n+o(1m2n)dx=δ1m2n+o(1m2n). |
Moreover, we have
Var(ˆrn(x,t))={1f2(x)Var(Nn(x,t))+r2(x,t)f2(x)Var(fn(x))−2r(x,t)f2(x)Cov(Nn(x,t),fn(x))}[1+o(1)]. |
Then,
∫10Var(ˆrn(x,t))dx={∫10Var(Nn(x,t))f2(x)dx+∫10r2(x,t)Var(fn(x))f2(x)dx−2∫10r(x,t)Cov(Nn(x,t),fn(x))f2(x)dx}[1+o(1)]. | (A.16) |
First, we have
Var[fn(x)]=1n[Am(x)−f2m(x)], |
where f2m(x)=E2[fn(x)]=f2(x)+O(m−1n), and
Am(x)=m2nmn−1∑k=0[F(k+1m)−F(km)]B2k(mn−1,x)=mn[f(x)Sm−1(x)+O(Hm−1(x))+O(m−1)], |
for x∈[0,1] and mn≥2, where
Hm(x)=m∑k=0|km−x|B2k(mn,x)=Ox(m−3/4n). |
Note that this error term is not uniform. For this, we use the Cauchy-Schwarz inequality to write
Hmn(x)≤[mn∑k=0(kmn−x)2Bk(mn,x)]1/2[mn∑k=0B3k(mn,x)]1/2≤[Smn(x)4mn]1/2, | (A.17) |
for all mn≥1 and x∈[0,1], since 0≤Bk(mn,x)≤1 and
mn∑k=0(kmn−x)2Bk(mn,x)=x(1−x)mn≤14mn. |
Then, starting from Eq (A.17) and applying Jensen's inequality and Lemma A.1 (ⅳ), we have
∫10g(x)Hmn(x)dx≤∫10g(x)[Smn(x)4mn]1/2dx≤[∫10g(x)dx]1/2[14m3/2n∫10g(x)ψ(x)dx+o(m−3/2n)]1/2=O(m−3/4n). |
Then, we infer that
∫10r2(x,t)Var[fn(x)]{f(x)}2dx=1n∫10r2(x,t)Amn(x)−f2mn(x){f(x)}2dx=1n[∫10r2(x,t)Amn(x){f(x)}2dx−∫10r2(x,t)]+O(1mn)=mnn[∫10r2(x,t){f(x)}2(Smn−1(x)+O(Hmn−1(x))+O(m−1n))dx]−1n∫10r2(x,t)+O(1mn)=mnn[∫10r2(x,t)f(x)Smn−1(x)dx+O(m−3/4n)]−1n∫10r2(x,t)+O(1mn), |
and, using Lemma A.1 (ⅳ), we have
∫10r2(x,t)Var[fn(x)]{f(x)}2dx=m1/2nn∫10r2(x,t)f(x)ψ(x)dx−1n∫10r2(x,t)+o(m1/2nn)+O(1mn). | (A.18) |
Second, we have
Cov[fn(x),Nn(x,t)]=1n{m2nmn−1∑k=0(∫k+1mkmnr(z)f(x)dz)B2k(mn−1,x)−E[fn(x)]E[Nn(x,t)]}=m2nnmn−1∑k=0(∫k+1mnkmn[r(x,t)f(x)+O(z−x)]dz)B2k(mn−1,x)−1nf(x)N(x,t)+O(1mn)=mnn[r(x,t)f(x)Smn−1(x)+O(Hmn−1(x))+O(m−1n)]−1nf(x)N(x,t)+O(1mn). |
Then, using the same argument for Hmn−1(x) as previously, we obtain
∫10r(x,t)Cov[fn(x),Nn(x,t)]{f(x)}2dx=mnn[∫10r2(x,t)f(x)Smn−1(x)dx+O(m−3/4n)]−1n∫10r2(x,t)+O(1mn)=m1/2nn∫10r2(x,t)f(x)ψ(x)dx−1n∫10r2(x,t)+o(m1/2nn)+O(1mn). | (A.19) |
Third, we have
Var[Nn(x,t)]=m2nnmn−1∑k=0(∫k+1mnkmnE[ρ(Y−t)2∣X=z]f(z)dz)B2k(mn−1,x)−1nE2[Nn(x,t)]=m2nnmn−1∑k=0(∫k+1mnkmn[E[ρ(Y−t)2∣X=x]f(x)+O(z−x)]dz)B2k(mn−1,x)−1nN2(x,t)+O(1mn)=mnn[E[ρ(Y−t)2∣X=x]f(x)Smn−1(x)+O(Hmn−1(x))+O(m−1n.)]−1nN2(x,t)+O(1mn). |
Then,
∫10Var[Nn(x,t)]{f(x)}2dx=mnn[∫10E[ρ(Y−t)2∣X=x]f(x)Smn−1(x)dx+O(m−3/4n)]−1n∫10r2(x,t)+O(1mn)=m1/2nn∫10E[ρ(Y−t)2∣X=x]f(x)ψ(x)dx−1n∫10r2(x,t)+o(m1/2nn)+O(1mn). | (A.20) |
Finally, substituting (A.18), (A.19), and (A.20) into (A.16), we obtain
∫10Var[ˆrn(x,t)]dx=(∫10E[ρ(Y−t)2∣X=x]f(x)ψ(x)dx−∫10E2[ρ(Y−t)∣X=x]f(x)ψ(x)dx)m1/2nn+o(m1/2nn)=∫10E[ρ(Y−t)2∣X=x]−E2[ρ(Y−t)∣X=x]f(x)ψ(x)dxm1/2nn+o(m1/2nn)=∫10Var[ρ(Y−t)∣X=x]f(x)ψ(x)dxm1/2nn+o(m1/2nn). |
Then, we obtain
MISE(ˆrn)=∫10{Var(ˆrn(x,t))+Bias2(ˆrn(x,t))}=Λ1m2n+Λ2m1/2nn+o(m1/2nn)+o(m−2n). |
Using a Taylor expansion of order one around θ, we get
ˆr(x,^θx)=ˆr(x,θx)+(^θx−θx)∂ˆr∂t(x,ξn), |
with ξn∈(^θx,θx). Because of the definition of ˆθ, we have
^θx−θx=−ˆr(x,θx)∂ˆr∂t(x,ξn). |
We will prove that the numerator is asymptotically normal, whereas the denominator converges in probability to Γ(x,θx); for that, we will use the following decompositions:
i) When x∈(0,1) and mn is chosen such that nm−5/2→c, then
n1/2m−1/4n(ˆθx−θx)=−n1/2m−1/4n[ˆr(x,θx)−r(x,θx)]∂ˆr∂t(x,ξn). |
ii) When x∈{0,1} and mn is chosen such that nm−3→c, then
n1/2m−1/2n(ˆθx−θx)=−n1/2m−1/2n[ˆr(x,θx)−r(x,θx)]∂ˆr∂t(x,ξn). |
So, we state asymptotic normality by Slutsky's Theorem, and by Proposition 3.2 with t=θ. We show that the numerator suitably normalized is asymptotically normally distributed. Then, it suffices to show that the denominator converges in probability to Γ(x,θx) (see Lemma A.10).
Lemma A.10. Under Assumptions (H1)–(H3), and for x∈[0,1] where f(x)>0, we have
∂ˆr∂t(x,ξn)P→Γ(x,θx). |
Proof. We explore the following decomposition:
|∂ˆr∂t(x,ξn)−Γ(x,θx)|≤|∂ˆr∂t(x,ξn)−∂ˆr∂t(x,θx)|+|∂ˆr∂t(x,θx)−Γ(x,θx)|≤J1(x)+J2(x). | (A.21) |
For J1(x), we write
J1(x)≤supy∈[a,b]|∂ρ(y−ξn)∂t−∂ρ(y−θx)∂t|mnfn(x)nn∑i=1m−1∑k=0I{km<Xi≤k+1m}Bk(m−1,x). |
Because ∂ρ(y−t)∂t is continuous at θ uniformly, the use of Theorem 3.1 and the convergence in probability of fn(x) to f(x) show that the first term of (A.21) converges in probability to 0. However, the limit of the second term is obtained by evaluating, separately, the bias and the variance terms of ∂ˆr∂t(x,θx). Clearly, a similar argument to those invoked for proving (3.1) can be used to obtain that
∂ˆr∂t(x,θx)→Γ(x,θx)in probability. |
[1] | McIlwain DR, Berger T, Mak TW (2013) Caspase functions in cell death and disease. Cold Spring Harb Perspect Biol 5: a008656. https://doi.org/10.1101/cshperspect.a008656 |
[2] | Nagata S (1999) Fas ligand-induced apoptosis. Annu Rev Genet 33: 29-55. https://doi.org/10.1146/annurev.genet.33.1.29 |
[3] | Pitti RM, Marsters SA, Lawrence DA, et al. (1998) Genomic amplification of a decoy receptor for Fas ligand in lung and colon cancer. Nature 396: 699-703. https://doi.org/10.1038/25387 |
[4] | Muraki M (2020) Sensitization to cell death induced by soluble Fas ligand and agonistic antibodies with exogenous agents: A review. AIMS Med Sci 7: 122-203. https://doi.org/10.3934/medsci.2020011 |
[5] | Cheng J, Zhou T, Liu C, et al. (1994) Protection from Fas-mediated apoptosis by a soluble form of the Fas molecule. Science 263: 1759-1762. https://doi.org/10.1126/science.7510905 |
[6] | Guégan JP, Legembre P (2018) Nonapoptotic functions of Fas/CD95 in the immune response. FEBS J 285: 809-827. https://doi.org/10.1111/febs.14292 |
[7] | Herrero R, Kajikawa O, Matute-Bello G, et al. (2011) The biological activity of FasL in human and mouse lungs is determined by the structure of its stalk region. J Clin Invest 121: 1174-1190. https://doi.org/10.1172/JCI43004 |
[8] | Malleter M, Tauzin S, Bessede A, et al. (2013) CD95L cell surface cleavage triggers a prometastatic signaling pathway in triple-negative breast cancer. Cancer Res 73: 6711-6721. https://doi.org/10.1158/0008-5472.CAN-13-1794 |
[9] | Holdenrieder S, Stieber P (2004) Apoptotic markers in cancer. Clin Biochem 37: 605-617. https://doi.org/10.1016/j.clinbiochem.2004.05.003 |
[10] | Audo R, Calmon-Hamaty F, Papon L, et al. (2014) Distinct effects of soluble and membrane-bound Fas ligand on fibroblast-like synoviocytes from rheumatoid arthritis patients. Arthritis Rheumatol 66: 3289-3299. https://doi.org/10.1002/art.38806 |
[11] | Hsieh SL, Lin WW (2017) Decoy receptor 3: an endogenous immunomodulator in cancer growth and inflammatory reactions. J Biomed Sci 24: 39. https://doi.org/10.1186/s12929-017-0347-7 |
[12] | Miwa K, Asano M, Horai R, et al. (1998) Caspase1-independent IL-1beta release and inflammation induced by the apoptosis inducer Fas ligand. Nat Med 4: 1287-1297. https://doi.org/10.1038/3276 |
[13] | Kurma K, Boizard-Moracchini A, Galli G, et al. (2021) Soluble CD95L in cancers and chronic inflammatory disorders, a new therapeutic target?. Biochim Biophys Acta Rev Cancer 1876: 188596. https://doi.org/10.1016/j.bbcan.2021.188596 |
[14] | Ueno T, Toi M, Tominaga T (1999) Circulating soluble Fas concentration in breast cancer patients. Clin Cancer Res 5: 3529-3533. |
[15] | Hara T, Tsurumi H, Takemura M, et al. (2000) Serum-soluble Fas level determines clinical symptoms and outcome of patients with aggressive non-Hodgkin's lymphoma. Am J Hematol 64: 257-261. https://doi.org/10.1002/1096-8652(200008)64:4<257::AID-AJH4>3.0.CO;2-2 |
[16] | Konno R, Takao T, Sato S, et al. (2000) Serum soluble Fas level as a prognostic factor in patients with gynecological malignancies. Clin Cancer Res 6: 3576-3580. |
[17] | Tsutsumi S, Kuwano H, Shimura T, et al. (2000) Circulating soluble Fas ligand in patients with gastric carcinoma. Cancer 89: 2560-2564. https://doi.org/10.1002/1097-0142(20001215)89:12<2560::AID-CNCR7>3.0.CO;2-Q |
[18] | Mizutani Y, Hongo F, Sato N, et al. (2001) Significance of serum soluble Fas ligand in patients with bladder carcinoma. Cancer 92: 287-293. https://doi.org/10.1002/1097-0142(20010715)92:2<287::AID-CNCR1321>3.0.CO;2-4 |
[19] | Ugurel S, Rappl G, Tilgen W, et al. (2001) Increased soluble CD95 (sFas/CD95) serum level correlates with poor prognosis in melanoma patients. Clin Cancer Res 7: 1282-1286. |
[20] | Hoffmann TK, Dworacki G, Tsukihiro T, et al. (2002) Spontaneous apoptosis of circulating T lymphocytes in patients with head and neck and its clinical importance. Clin Cancer Res 8: 2553-2562. |
[21] | Akhmedkhanov A, Lundin E, Guller S, et al. (2003) Circulating soluble Fas levels and risk of ovarian cancer. BMC Cancer 3: 33. https://doi.org/10.1186/1471-2407-3-33 |
[22] | Sheen-Chen SM, Chen HS, Eng HL, et al. (2003) Circulating soluble Fas in patients with breast cancer. World J Surg 27: 10-13. https://doi.org/10.1007/s00268-002-6378-5 |
[23] | Wu Y, Han B, Sheng H, et al. (2003) Clinical significance of detecting elevated serum DcR3/TR6/M68 in malignant tumor patients. Int J Cancer 105: 724-732. https://doi.org/10.1002/ijc.11138 |
[24] | Erdoğan B, Uzaslan E, Budak F, et al. (2005) The evaluation of soluble Fas and soluble Fas ligand levels of bronchoalveolar lavage fluid in lung cancer patients. Tuberk Toraks 53: 127-131. |
[25] | Nadal C, Maurel J, Gallego R, et al. (2005) Fas/Fas ligand ratio: a marker of oxaliplatin-based intrinsic and acquired resistance in advanced colorectal cancer. Clin Cancer Res 11: 4770-4774. https://doi.org/10.1158/1078-0432.CCR-04-2119 |
[26] | Shimizu M, Kondo M, Ito Y, et al. (2005) Soluble Fas and Fas ligand new information on metastasis and response to chemotherapy in SCLC patients. Cancer Detect Prev 29: 175-180. https://doi.org/10.1016/j.cdp.2004.09.001 |
[27] | Yatsuya H, Toyoshima H, Tamakoshi K, et al. (2005) Serum levels of insulin-like growth factor I, II, and binding protein 3, transforming growth factor β-1, soluble Fas ligand and superoxide dismutase activity in stomach cancer cases and their controls in the JACC study. J Epidemiol 15: S120-S125. https://doi.org/10.2188/jea.15.S120 |
[28] | Sonoda K, Miyamoto S, Hirakawa T, et al. (2006) Clinical significance of RCAS1 as a biomarker of uterine cancer. Gynecol Oncol 103: 924-931. https://doi.org/10.1016/j.ygyno.2006.05.047 |
[29] | Svatek RS, Herman MP, Lotan Y, et al. (2006) Soluble Fas-a promising novel urinary marker for the detection of recurrent superficial bladder cancer. Cancer 106: 1701-1707. https://doi.org/10.1002/cncr.21795 |
[30] | Baldini E, Ulisse S, Marchioni E, et al. (2007) Expression of Fas and Fas ligand in human testicular germ cell tumours. Int J Andorol 32: 123-130. https://doi.org/10.1111/j.1365-2605.2007.00823.x |
[31] | Simon I, Liu Y, Krall KL, et al. (2007) Evaluation of the novel serum markers B7-H4, spondin 2, and DcR3 for diagnosis and early detection of ovarian cancer. Gynecol Oncol 106: 112-118. https://doi.org/10.1016/j.ygyno.2007.03.007 |
[32] | Aguilar-Lemarroy A, Romero-Ramos JE, Olimon-Andalon V, et al. (2008) Apoptosis induction in Jurkat cells and sCD95 levels in women's sera are related with the risk of developing cervical cancer. BMC Cancer 8: 99. https://doi.org/10.1186/1471-2407-8-99 |
[33] | Chaudhry P, Srinvasan R, Patel FD, et al. (2008) Serum soluble Fas levels and prediction of response to platinum-based chemotherapy in epithelial ovarian cancer. Int J Cancer 122: 1716-1721. https://doi.org/10.1002/ijc.23213 |
[34] | Connor JP, Felder M (2008) Ascites from epithelial ovarian cancer contain high levels of functional decoy receptor 3 (DcR3) and is associated with platinum resistance. Gynecol Oncol 111: 330-335. https://doi.org/10.1016/j.ygyno.2008.07.012 |
[35] | Kilic A, Schubert MJ, Luketich JD, et al. (2008) Use of novel autoantibody and cancer-related protein arrays for the detection of esophageal adenocarcinoma in serum. J Thorac Cardiovasc Surg 136: 199-204. https://doi.org/10.1016/j.jtcvs.2008.01.012 |
[36] | Macher-Goeppinger S, Aulmann S, Wagener N, et al. (2008) Decoy receptor 3 is a prognostic factor in renal cell cancer. Neoplasia 10: 1049-1056. https://doi.org/10.1593/neo.08626 |
[37] | Nolen BM, Marks JR, Ta'san S, et al. (2008) Serum biomarker profiles and response to neoadjuvant chemotherapy for locally advanced breast cancer. Breast Cancer Res 10: R45. https://doi.org/10.1186/bcr2096 |
[38] | Tamakoshi A, Nakachi K, Ito Y, et al. (2008) Soluble Fas level and cancer mortality: Findings from a nested case-control study within a large-scale prospective study. Int J Cancer 123: 1913-1916. https://doi.org/10.1002/ijc.23731 |
[39] | Anderson GL, McIntosh M, Wu L, et al. (2010) Assessing lead time of selected ovarian cancer biomarkers: A nested case-control study. J Natl Cancer Inst 102: 26-38. https://doi.org/10.1093/jnci/djp438 |
[40] | Goon PKY, Lip GYH, Stonelake PS, et al. (2009) Circulating endothelial cells and circulating progenitor cells in breast cancer: Relationship to endothelial damage/dysfunction/apoptosis, clinicopathologic factors, and the Nottingham prognostic index. Neoplasia 11: 771-779. https://doi.org/10.1593/neo.09490 |
[41] | Kavathia N, Jain A, Walston J, et al. (2009) Serum markers of apoptosis decrease with age and cancer stage. Aging 1: 652-663. https://doi.org/10.18632/aging.100069 |
[42] | Vysotskii MM, Digaeva MA, Kushlinskii NE, et al. (2009) Serum sFas, leptin, and VEGF in patients with ovarian cancer and benign tumors. Bull Exp Biol Med 148: 810-814. https://doi.org/10.1007/s10517-010-0823-5 |
[43] | Boroumand-Noughabi S, Sima HR, Ghaffarzadehgan K, et al. (2010) Soluble Fas might serve as a diagnostic tool for gastric adenocarcinoma. BMC Cancer 10: 275. https://doi.org/10.1186/1471-2407-10-275 |
[44] | Habibagahi M, Jaberipour M, Fattahi MJ, et al. (2010) High concentration of serum soluble Fas in patients with head and neck carcinoma: A comparative study before and after surgical removal of tumor. Middle East J Cancer 1: 21-26. |
[45] | Nolen B, Velikokhatnaya L, Marrangoni A, et al. (2010) Serum biomarker panels for the discrimination of benign from malignant cases in patients with an adnexal mass. Gynecol Oncol 117: 440-445. https://doi.org/10.1016/j.ygyno.2010.02.005 |
[46] | Ulukaya E, Acilan C, Yilmaz M, et al. (2010) sFas levels increase in response to cisplatin-based chemotherapy in lung cancer patients. Cell Biochem Funct 28: 565-570. https://doi.org/10.1002/cbf.1689 |
[47] | Yang M, Chen G, Dang Y, et al. (2010) Significance of decoy receptor 3 in sera of hepatocellular carcinoma patients. Upsala J Med Sci 115: 232-237. https://doi.org/10.3109/03009734.2010.516410 |
[48] | Zekri AN, El-Din HMA, Bahnassy AA, et al. (2010) Serum levels of soluble Fas, soluble tumor necrosis factor-receptor II, interleukin-2 receptor and interleukin-8 as early predictors of carcinoma in Egyptian patients with hepatitis C virus genotype-4. Comp Hepatol 9: 1. https://doi.org/10.1186/1476-5926-9-1 |
[49] | Hoogwater FJH, Snoeren N, Nijkamp MW, et al. (2011) Circulating CD95-ligand as a potential prognostic marker for recurrence in patients with synchronous colorectal liver metastases. Anticancer Res 31: 4507-4512. |
[50] | Fersching DMI, Nagel D, Siegele B, et al. (2012) Apoptosis-related biomarkers sFas, MIF, ICAM-1 and PAI-1 in serum of breast cancer patients undergoing neoadjuvant chemotherapy. Anticancer Res 32: 2047-2058. |
[51] | Hammam O, Mahmoud O, Zahran M, et al. (2012) The role of Fas/Fas ligand system in the pathogenesis of liver cirrhosis and hepatocellular carcinoma. Hepat Mon 12: e6132. https://doi.org/10.5812/hepatmon.6132 |
[52] | Izbicka E, Streeper RT, Michalek JE, et al. (2012) Plasma biomarkers distinguish non-small cell lung cancer from asthma and differ in men and women. Cancer Genomics Proteomics 9: 27-35. |
[53] | Eissa S, Swellam M, Abdel-Malak C, et al. (2013) The clinical relevance of urinary soluble fas (sFas) for diagnosis of bilharzial bladder cancer. Asian Biomed 7: 761-767. https://doi.org/10.5372/1905-7415.0706.238 |
[54] | Owonikoko TK, Hossain MS, Bhimani C, et al. (2013) Soluble Fas ligand as a biomarker of disease recurrence in differentiated thyroid cancer. Cancer 119: 1503-1511. https://doi.org/10.1002/cncr.27937 |
[55] | Elbedewy TA, El-Feky S, El Sheikh MA, et al. (2014) Serum levels of soluble Fas and soluble Fas ligand as markers for hepatocellular carcinoma in hepatitis C virus patients. Int J Adv Res 2: 911-919. |
[56] | Xu Y, Zhang L, Sun S, et al. (2014) CC Chemokine ligand 18 and IGF-binding protein 6 as potential serum biomarkers for prostate cancer. Tohoku J Exp Med 233: 25-31. https://doi.org/10.1620/tjem.233.25 |
[57] | Yeh CN, Chung WH, Su SC, et al. (2014) Fas/Fas ligand mediates keratinocyte death in sunitinib-induced hand-foot skin reaction. J Invest Dermatol 134: 2768-2775. https://doi.org/10.1038/jid.2014.218 |
[58] | Kadam CY, Abhang SA (2015) Serum levels of soluble Fas ligand, granzyme B and cytochrome c during adjuvant chemotherapy of breast cancer. Clin Chim Acta 438: 98-102. https://doi.org/10.1016/j.cca.2014.08.012 |
[59] | Kouloubnis A, Sofroniadou S, Panoulas VF, et al. (2015) The role of TNF-α, Fas/Fas ligand system and NT-proBNP in the early detection of asymptomatic left ventricular dysfunction in cancer patients treated with anthracyclines. IJC Heart Vasc 6: 85-90. https://doi.org/10.1016/j.ijcha.2015.01.002 |
[60] | Lancrajan I, Schneider-Stock R, Naschberger E, et al. (2015) Absolute quantification of DcR3 and GDF-15 from human serum by LC-ESI MS. J Cell Mol Med 19: 1656-1671. https://doi.org/10.1111/jcmm.12540 |
[61] | Bamias G, Gizis M, Delladetsima I, et al. (2016) Elevated serum levels of the antiapoptotic protein decoy-receptor 3 are associated with advanced liver disease. Can J Gastroenterol Hepatol 2016: 2637010. https://doi.org/10.1155/2016/2637010 |
[62] | Chang SW, Wang BC, Gong XG, et al. (2016) Serum decoy receptor 3 is a diagnostic and prognostic biomarker in patients with colorectal cancer. Int J Clin Exp Pathol 9: 7488-7492. |
[63] | Dressen K, Hermann N, Manekeller S, et al. (2017) Diagnostic performance of a novel multiplex immunoassay in colorectal cancer. Anticancer Res 37: 2477-2486. https://doi.org/10.21873/anticanres.11588 |
[64] | Hermann N, Dressen K, Schroeder L, et al. (2017) Diagnostic relevance of a novel multiplex immunoassay panel in breast cancer. Tumor Biol 2017: 1-11. https://doi.org/10.1177/1010428317711381 |
[65] | Johdi NA, Mazlan L, Sagap I, et al. (2017) Profiling of cytokines, chemokines and other soluble proteins as a potential biomarker in colorectal cancer and polyps. Cytokine 99: 35-42. https://doi.org/10.1016/j.cyto.2017.06.015 |
[66] | Li J, Xie N, Yuan J, et al. (2017) DcR3 combined with hematological traits serves as a valuable biomarker for the diagnosis of cancer metastasis. Oncotarget 8: 107612-107620. https://doi.org/10.18632/oncotarget.22544 |
[67] | Korczyński P, Mierzejewski M, Krenke R, et al. (2018) Cancer ratio and other new parameters for differentiation between malignant and nonmalignant pleural effusions. Pol Arch Intern Med 128: 354-361. https://doi.org/10.20452/pamw.4278 |
[68] | Ku SC, Ho PS, Tseng YT, et al. (2018) Benzodiazepine-associated carcinogenesis: Focus on lorazepam-associated cancer biomarker changes in overweight individuals. Psychiatry Invest 15: 900-906. https://doi.org/10.30773/pi.2018.05.02.1 |
[69] | Singh RK, Meena RN, Tiwary SK, et al. (2018) Fas ligand as a circulating apoptosis marker in carcinoma breast. World J Surg Res 7: 7-14. |
[70] | Łaniewski P, Cui H, Roe DJ, et al. (2019) Features of the cervicovaginal microenvironment drive cancer biomarker signatures in patients across cervical carcinogenesis. Sci Rep 9: 7333. https://doi.org/10.1038/s41598-019-43849-5 |
[71] | Wei Y, Chen X, Yang J, et al. (2019) DcR3 promotes proliferation and invasion of pancreatic cancer via a DcR3/STAT1/IRF1 feedback loop. Am J Cancer Res 9: 2618-2633. |
[72] | Akhmaltdinova L, Sirota V, Zhumaliyeva V, et al. (2020) Inflammatory serum biomarkers in colorectal cancer in Kazakhstan population. Int J Inflam 2020: 9476326. https://doi.org/10.1155/2020/9476326 |
[73] | Ali AS, Perren A, Lindskog C, et al. (2020) Candidate protein biomarkers in pancreatic neuroendocrine neoplasms grade 3. Sci Rep 10: 10639. https://doi.org/10.1038/s41598-020-67670-7 |
[74] | Chiu CT, Wang PW, Asare-Werehene M, et al. (2020) Circulating plasma gelsolin: A predictor of favorable clinical outcomes in head and neck cancer and sensitive biomarker for early disease diagnosis combined with soluble Fas ligand. Cancers 12: 1569. https://doi.org/10.3390/cancers12061569 |
[75] | Feng K, Liu Y, Zhao Y, et al. (2020) Efficacy and biomarker analysis of nivolumab plus gemcitabine and cisplatin in patients with unresectable or metastatic biliary tract cancers: results from a phase II study. J Immunother Cancer 8: e000367. https://doi.org/10.1136/jitc-2019-000367 |
[76] | Honma N, Inoue T, Tsuchiya N, et al. (2020) Prognostic value of plasminogen activator inhibitor-1 in biomarker exploration using multiplex immunoassay in patients with axitinib. Health Sci Rep 3: e197. https://doi.org/10.1002/hsr2.197 |
[77] | Ghonaim E, El-Haggar S, Gohar S (2021) Possible protective effect of pantoprazole against cisplatin-induced nephrotoxicity in head and neck cancer patients: a randomized controlled trial. Med Oncol 38: 108. https://doi.org/10.1007/s12032-021-01558-y |
[78] | López-Jornet P, Aznar C, Ceron J (2021) Salivary biomarkers in breast cancer: a cross-sectional study. Support Care Cancer 29: 889-896. https://doi.org/10.1007/s00520-020-05561-3 |
[79] | Jodo S, Kobayashi S, Kayagaki N, et al. (1997) Serum levels of soluble Fas/APO-1 (CD95) and its molecular structure in patients with systemic lupus erythematosus (SLE) and other autoimmune diseases. Clin Exp Immunol 107: 89-95. https://doi.org/10.1046/j.1365-2249.1997.d01-901.x |
[80] | Ohtsuka K, Hashimoto M (2000) Serum levels of soluble Fas in patients with Graves' ophthalmopathy. Br J Ophthalmol 84: 103-106. https://doi.org/10.1136/bjo.84.1.103 |
[81] | Wang CY, Zhong WB, Chang TC, et al. (2002) Circulating soluble Fas ligand correlates with disease activity in Graves' Hyperthyroidism. Metabolism 51: 769-773. https://doi.org/10.1053/meta.2002.32034 |
[82] | Boku S, Takahashi T, Watanabe K, et al. (2003) Serum soluble Fas as a seromarker of disease activity in chronic hepatitis C and the possible involvement of peripheral blood mononuclear cells in the production of serum soluble Fas. Acta Med Biol 51: 49-58. |
[83] | Kiraz S, Ertenli I, Öztürk MA, et al. (2003) Increased soluble Fas suggests delayed apoptosis in familial Mediterranean fever complicated with amyloidosis. J Rheumatol 30: 313-315. |
[84] | Silvestris F, Grinello D, Tucci M, et al. (2003) Enhancement of T cell apoptosis correlates with increased serum levels of soluble Fas (CD95/Apo-I) in active lupus. Lupus 12: 8-14. https://doi.org/10.1191/0961203303lu250oa |
[85] | Hayashi H, Maeda M, Murakami S, et al. (2009) Soluble interleukin-2 as an indicator of immunological disturbance found in silicosis patients. Int J Immunol Pharmacol 22: 53-62. https://doi.org/10.1177/039463200902200107 |
[86] | Magerus-Chatinet A, Stolzenberg MC, Loffredo MS, et al. (2009) FAS-L, IL-10, and double-negative CD4−CD8− TCR α/β+ T cells are reliable markers of autoimmune lymphoproliferative syndrome (ALPS) associated with FAS loss of function. Blood 113: 3027-3030. https://doi.org/10.1182/blood-2008-09-179630 |
[87] | Sahebari M, Hatef MR, Rezaieyazdi Z, et al. (2010) Correlation between serum levels of soluble Fas (CD95/Apo-1) with disease activity in systemic lupus erythematosus patients in Khorasan, Iran. Arch Iran Med 13: 135-142. https://doi.org/10.1007/s00296-010-1633-9 |
[88] | Ikonomidis I, Tzortzis S, Lekakis J, et al. (2011) Association of soluble apoptotic markers with impaired left ventricular deformation in patients with rheumatoid arthritis. Effects of inhibition of interleukin-1 activity by anakinra. Thromb Haemost 106: 959-967. https://doi.org/10.1160/TH11-02-0117 |
[89] | Bamias G, Stamatelopoulos K, Zampeli E, et al. (2013) Circulating levels of TNF-like cytokine 1A correlate with the progression of atheromatous lesions in patients with rheumatoid arthritis. Clin Immunol 147: 144-150. https://doi.org/10.1016/j.clim.2013.03.002 |
[90] | Moreno M, Sáenz-Cuesta M, Castilló J, et al. (2013) Circulating levels of soluble apoptosis-related molecules in patients with multiple sclerosis. J Neuroimmunol 263: 152-154. https://doi.org/10.1016/j.jneuroim.2013.07.013 |
[91] | Oka S, Furukawa H, Shimada K, et al. (2013) Serum biomarker analysis of collagen disease patients with acute-onset diffuse interstitial lung disease. BMC Immunol 14: 9. https://doi.org/10.1186/1471-2172-14-9 |
[92] | Rensing-Ehl A, Janda A, Lorenz MR, et al. (2013) Sequential decisions on Fas sequencing guided by biomarkers in patients with lymphoproliferation and autoimmune cytopenia. Heamatologica 98: 1948-1955. https://doi.org/10.3324/haematol.2012.081901 |
[93] | Roberts CA, Ayers L, Bateman EAL, et al. (2013) Investigation of common variable immunodeficiency patients and healthy individuals using autoimmune lymphoproliferative syndrome biomarkers. Hum Immunol 74: 1531-1535. https://doi.org/10.1016/j.humimm.2013.08.266 |
[94] | Bollain-y-Goytia JJ, Arellano-Rodríguez M, Torres-Del-Muro FJ, et al. (2014) Soluble Fas and the -670 polymorphism of Fas in lupus nephritis. Int J Nephrol 2014: 780406. https://doi.org/10.1155/2014/780406 |
[95] | Chen MH, Liu PC, Chang CW, et al. (2014) Decoy receptor 3 suppresses B cell functions and has a negative correlation with disease activity in rheumatoid arthritis. Clin Exp Rheumatol 32: 715-723. |
[96] | Munroe ME, Vista ES, Guthridge JM, et al. (2014) Proinflammatory adaptive cytokine and shed tumor necrosis factor receptor levels are elevated preceding systemic lupus erythematosus disease flare. Arthritis Rheumatol 66: 1888-1899. https://doi.org/10.1002/art.38573 |
[97] | Price S, Shaw PA, Seitz A, et al. (2014) Natural history of autoimmune lymphoproliferative syndrome associated with FAS gene mutations. Blood 123: 1989-1999. https://doi.org/10.1182/blood-2013-10-535393 |
[98] | Romano E, Terenzi R, Manetti M, et al. (2014) Disease activity improvement in rheumatoid arthritis treated with tumor necrosis factor-α inhibitors correlates with increased soluble Fas levels. J Rheumatol 41: 1961-1965. https://doi.org/10.3899/jrheum.131544 |
[99] | Liu J, Zhao Z, Zou Y, et al. (2015) The expression of death decoy receptor 3 was increased in the patients with primary Sjögren's syndrome. Clin Rheumatol 34: 879-885. https://doi.org/10.1007/s10067-014-2853-2 |
[100] | Zijuan X, Hui S, Ye T, et al. (2015) Serum and synovial fluid levels of tumor necrosis factor-like ligand 1A and decoy receptor 3 in rheumatoid arthritis. Cytokine 72: 185-189. https://doi.org/10.1016/j.cyto.2014.12.026 |
[101] | Elsaeed GSM, Elshamaa MF, Salah DM, et al. (2016) Fas-ligand and granzyme-b levels in children with nephrotic syndrome. Int J Pharma Clin Res 8: 1600-1604. |
[102] | Maruyama H, Hirayama K, Nagai M, et al. (2016) Serum decoy receptor 3 levels are associated with the disease activity of MPO-ANCA-associated renal vasculitis. Clin Rheumatol 35: 2469-2476. https://doi.org/10.1007/s10067-016-3321-y |
[103] | Chen MH, Kan HT, Liu CY, et al. (2017) Serum decoy receptor 3 is a biomarker for disease severity in nonatopic asthma patients. J Formos Med Assoc 116: 49-56. https://doi.org/10.1016/j.jfma.2016.01.007 |
[104] | Engmann J, Rüdrich U, Behrens G, et al. (2017) Increased activity and apoptosis of eosinophils in blister fluids, skin and peripheral blood of patients with bullous pemphigoid. Acta Derm Venereol 97: 464-471. https://doi.org/10.2340/00015555-2581 |
[105] | Ding YW, Pan SY, Xie W, et al. (2018) Elevated soluble Fas and FasL in cerebrospinal fluid and serum of patients with anti-N-methyl-D-aspartate receptor encephalitis. Front Neurol 9: 904. https://doi.org/10.3389/fneur.2018.00904 |
[106] | Liphaus BL, Sallum AEM, Aikawa NE, et al. (2018) Increased soluble cytoplasmic Bcl-2 protein serum levels and expression and decreased Fas expression in lymphocytes and monocytes in Juvenile dermatomyositis. J Rheumatol 45: 1577-1580. https://doi.org/10.3899/jrheum.171248 |
[107] | Wigren M, Svenungsson E, Mattisson IY, et al. (2018) Cardiovascular disease in systemic lupus erythematosus is associated with increased levels of biomarkers reflecting receptor-activated apoptosis. Atherosclerosis 270: 1-7. https://doi.org/10.1016/j.atherosclerosis.2018.01.022 |
[108] | Molnár E, Radwan N, Kovács G, et al. (2020) Key diagnostic markers for autoimmune lymphoproliferative syndrome with molecular genetic diagnosis. Blood 136: 1933-1945. https://doi.org/10.1182/blood.2020005486 |
[109] | Sremec J, Tomasović S, Sremec NT, et al. (2020) Elevated concentrations of soluble Fas and FasL in multiple sclerosis patients with antinuclear antibodies. J Clin Med 9: 3845. https://doi.org/10.3390/jcm9123845 |
[110] | Vincent FB, Kandane-Rathnayake R, Koelmeyer R, et al. (2020) Associations of serum soluble Fas and Fas ligand (FasL) with outcomes in systemic lupus erythematosus. Lupus Sci Med 7: e000375. https://doi.org/10.1136/lupus-2019-000375 |
[111] | Kamal A, Abdelmegeid AK, Gabr MAM, et al. (2021) Serum decoy receptor 3 (DcR3): a promising biomarker for atopic asthma in children. Immunol Res 69: 568-575. https://doi.org/10.1007/s12026-021-09218-z |
[112] | Kessel C, Fail N, Grom A, et al. (2021) Definition and validation of serum biomarkers for optimal differentiation of hyperferritinaemic cytokine storm conditions in children: a retrospective cohort study. Lancet Rheumatol 3: 563-573. https://doi.org/10.1016/S2665-9913(21)00115-6 |
[113] | Qi Y, Xu J, Lin Z, et al. (2021) The network of pro-inflammatory factors CD147, DcR3, and IL33 in the development of Kawasaki disease. J Inflamm Res 14: 6043-6053. https://doi.org/10.2147/JIR.S338763 |
[114] | Su KW, Chiu CY, Tsai MH, et al. (2021) Cord blood soluble Fas ligand linked to allergic rhinitis and lung function in seven-year-old children. J Microbiol Immunol Infect . (in press). https://doi.org/10.1016/j.jmii.2021.03.016 |
[115] | De Freitas I, Fernández-Somoza M, Essenfeld-Sekler E, et al. (2004) Serum levels of the apoptosis-associated molecules, tumor necrosis factor-α/tumor necrosis factor type-I receptor and Fas/FasL, in sepsis. Chest 125: 2238-2246. https://doi.org/10.1378/chest.125.6.2238 |
[116] | Paunel-Görgülü A, Flohé S, Scholz M, et al. (2011) Increased serum soluble Fas after major trauma is associated with delayed neutrophil apoptosis and development of sepsis. Crit Care 15: R20. https://doi.org/10.1186/cc9965 |
[117] | Hou YQ, Xu P, Zhang M, et al. (2012) Serum decoy receptor 3, a potential new biomarker for sepsis. Clin Chim Acta 413: 744-748. https://doi.org/10.1016/j.cca.2012.01.007 |
[118] | Huttunen R, Syrjänen J, Vuento R, et al. (2012) Apoptosis markers soluble Fas (sFas), Fas ligand (FasL) and sFas/sFasL ratio in patients with bacteremia: A prospective cohort study. J Infect 64: 276-281. https://doi.org/10.1016/j.jinf.2011.12.006 |
[119] | Kim S, Mi L, Zhang L (2012) Specific elevation of DcR3 in sera of sepsis patients and its potential role as a clinically important biomarker of sepsis. Diagn Microbiol Infect Dis 73: 312-317. https://doi.org/10.1016/j.diagmicrobio.2012.04.008 |
[120] | Liu YJ, Shao LH, Wang Q, et al. (2014) Predictive value of decoy receptor 3 in postoperative nosocomial bacterial meningitis. Int J Mol Sci 15: 19962-19970. https://doi.org/10.3390/ijms151119962 |
[121] | Liu YJ, Shao LH, Zhang J, et al. (2015) The combination of decoy receptor 3 and soluble triggering receptor expressed on myeloid cells-1 for the diagnosis of nosocomial bacterial meningitis. Ann Clin Microbiol Antimicrob 14: 17. https://doi.org/10.1186/s12941-015-0078-0 |
[122] | Chu CM, Kao KC, Huang SH, et al. (2016) Diagnostic value of apoptosis biomarkers in severe sepsis-A pilot study. Cell Mol Biol 62: 32-37. https://doi.org/10.14715/cmb/2016.62.11.6 |
[123] | Carcillo JA, Halstead ES, Hall MW, et al. (2017) Three hypothetical inflammation pathobiology phenotypes and pediatric sepsis-induced multiple organ failure outcome. Pediatr Crit Care Med 18: 513-523. https://doi.org/10.1097/PCC.0000000000001122 |
[124] | Mikacenic C, Price BL, Harju-Baker S, et al. (2017) A two-biomarker model predicts mortality in the critically ill with sepsis. Am J Respir Crit Care Med 196: 1004-1011. https://doi.org/10.1164/rccm.201611-2307OC |
[125] | Gao L, Yang B, Zhang H, et al. (2018) DcR3, a new biomarker for sepsis, correlates with infection severity and procalcitonin. Oncotarget 9: 10934-10944. https://doi.org/10.18632/oncotarget.23736 |
[126] | Garcia-Obregon S, Azkargorta M, Seijas I, et al. (2018) Identification of a panel of serum protein markers in early stage of sepsis and its validation in a cohort of patients. J Microbiol Immunol Infect 51: 465-472. https://doi.org/10.1016/j.jmii.2016.12.002 |
[127] | Hou Y, Liang D, Liu Y, et al. (2018) Up-regulation of DcR3 in microbial toxins-stimulated HUVECs involves NF-κB signaling. BMC Biochem 19: 13. https://doi.org/10.1186/s12858-018-0102-z |
[128] | Zhao JJ, Lou XL, Chen HW, et al. (2018) Diagnostic value of decoy receptor 3 combined with procalcitonin and soluble urokinase-type plasminogen activator receptor for sepsis. Cell Mol Biol Lett 23: 22. https://doi.org/10.1186/s11658-018-0087-z |
[129] | Thompson K, Connor J (2019) When cultures fail: Postmortem decoy receptor 3 (DcR3) as a marker of antemortem sepsis. Acad Forensic Pathol 9: 15-23. https://doi.org/10.1177/1925362119851075 |
[130] | Hassan MH, Yassin MM, Hassan YM, et al. (2020) Decoy receptor 3 as a biomarker for diagnosis of bacterial sepsis. Egypt J Med Microbiol 29: 153-162. https://doi.org/10.51429/EJMM29320 |
[131] | Medina LMP, Rath E, Jahagirdar S, et al. (2021) Discriminatory plasma biomarkers predict specific clinical phenotypes of necrotizing soft-tissue infections. J Clin Invest 131: e149523. https://doi.org/10.1172/JCI149523 |
[132] | Iio S, Hayashi N, Mita E, et al. (1998) Serum levels of soluble Fas antigen in chronic hepatitis C patients. J Hepatol 29: 517-523. https://doi.org/10.1016/S0168-8278(98)80145-1 |
[133] | Raghuraman S, Abraham P, Daniel HD, et al. (2005) Characterization of soluble FAS, FAS ligand and tumour necrosis factor-alpha in patients with chronic HCV infection. J Clin Virol 34: 63-70. https://doi.org/10.1016/j.jcv.2005.01.009 |
[134] | Torre F, Bellis L, Delfino A, et al. (2008) Peripheral blood serum markers for apoptosis and liver fibrosis: Are they trustworthy indicators of liver realness?. Digest Liver Dis 40: 441-445. https://doi.org/10.1016/j.dld.2007.10.027 |
[135] | Hou Y, Xu P, Lou X, et al. (2013) Serum decoy receptor 3 is a useful predictor for the active status of chronic hepatitis B in hepatitis B e antigen-negative patients. Tohoku J Exp Med 230: 227-232. https://doi.org/10.1620/tjem.230.227 |
[136] | Dong Y, Shi D, Li M, et al. (2015) Elevated serum levels of decoy receptor 3 are associated with disease severity in patients with hemorrhagic fever with renal syndrome. Intern Emerg Med 10: 567-573. https://doi.org/10.1007/s11739-015-1195-7 |
[137] | Lin YT, Yen CH, Chen HL, et al. (2015) The serologic decoy receptor 3 (DcR3) levels are associated with slower disease progression in HIV-1/AIDS patients. J Formos Med Assoc 114: 498-503. https://doi.org/10.1016/j.jfma.2013.01.007 |
[138] | Ikomey GM, Julius A, Jacobs GB, et al. (2016) Fas mediated (CD95L) peripheral T-cell apoptosis marker in monitoring HIV-1 disease progression in adults in Yaoundé, Cameroon. Int J Immunol 4: 1-5. https://doi.org/10.11648/j.iji.20160401.11 |
[139] | Ikomey G, Assoumou MCO, Atashili J, et al. (2016) The potentials of Fas receptors and ligands in monitoring HIV-1 disease in children in Yaoundé, Cameroon. J Int Assoc Prov AIDS Care 15: 418-422. https://doi.org/10.1177/2325957413488202 |
[140] | McElroy AK, Harmon JR, Flietstra TD, et al. (2016) Kinetic analysis of biomarkers in a cohort of US patients with Ebola virus disease. Clin Infect Dis 63: 460-467. https://doi.org/10.1093/cid/ciw334 |
[141] | Shin SY, Jeong SH, Sung PS, et al. (2016) Comparative analysis of liver injury-associated cytokines in acute hepatitis A and B. Yonsei Med J 57: 652-657. https://doi.org/10.3349/ymj.2016.57.3.652 |
[142] | Lou X, Hou Y, Cao H, et al. (2018) Clinical significance of decoy receptor 3 upregulation in patients with hepatitis B and liver fibrosis. Oncol Lett 16: 1147-1154. https://doi.org/10.3892/ol.2018.8762 |
[143] | Zhang Z, Dai X, Qi J, et al. (2018) Astragalus mongholicus (Fisch.) Bge improves peripheral Treg cell immunity imbalance in the children with viral myocarditis by reducing the levels of miR-146b and miR-155. Front Pediatr 6: 139. https://doi.org/10.3389/fped.2018.00139 |
[144] | Dambaya B, Nkenfou CN, Ambada G, et al. (2019) Differential expression of Fas receptors (CD95) and Fas ligands (CD95L) in HIV infected and exposed uninfected children in Cameroon versus unexposed children. Pan Afric Med J 34: 39. https://doi.org/10.11604/pamj.2019.34.39.15038 |
[145] | Liang DY, Sha S, Yi Q, et al. (2019) Hepatitis B X protein upregulates decoy receptor 3 expression via the PI3K/NF-κB pathway. Cell Signal 62: 109346. https://doi.org/10.1016/j.cellsig.2019.109346 |
[146] | Shata MTM, Abdel-hameed EA, Rouster SD, et al. (2019) HBV and HIV/HBV infected patients have distinct immune exhaustion and apoptotic serum biomarker profiles. Pathog Immun 4: 39-65. https://doi.org/10.20411/pai.v4i1.267 |
[147] | Mercedes R, Brown J, Minard C, et al. (2020) A liver biopsy validation pilot study of shear wave elastography, APRI, FIB-4, and novel serum biomarkers for liver fibrosis staging in children with chronic viral hepatitis. Glob Pediatr Health 6: 1-8. https://doi.org/10.1177/2333794X20938931 |
[148] | Abers MS, Delmonte OM, Ricotta EE, et al. (2021) An immune-based biomarker signature is associated with mortality in COVID-19 patients. JCI Insight 6: e144455. https://doi.org/10.1172/jci.insight.144455 |
[149] | El-Mesery M, El-Mowafy M, Youssef LF, et al. (2021) Serum soluble fibrinogen-like protein 2 represents a novel biomarker for differentiation between acute and chronic Egyptian hepatitis B virus-infected patients. J Interferon Cytokine Res 41: 52-59. https://doi.org/10.1089/jir.2020.0118 |
[150] | Kessel C, Vollenberg R, Masjosthusmann K, et al. (2021) Discrimination of COVID-19 from inflammation-induced cytokine storm syndromes using disease-related blood biomarkers. Arthritis Rheumatol 73: 1791-1799. https://doi.org/10.1002/art.41763 |
[151] | Armah HB, Wilson NO, Sarfo BY, et al. (2007) Cerebrospinal fluid and serum biomarkers of malaria mortality in Ghanaian children. Malar J 6: 147. https://doi.org/10.1186/1475-2875-6-147 |
[152] | Jain V, Armah HB, Tongren JE, et al. (2008) Plasma IP-10, apoptotic and angiogenic factors associated with fatal cerebral malaria in India. Malar J 7: 83. https://doi.org/10.1186/1475-2875-7-83 |
[153] | Wu SH, Li CT, Lin CH, et al. (2010) Soluble Fas ligand is another good diagnostic marker for tuberculous pleurisy. Diagn Microbiol Infect Dis 68: 395-400. https://doi.org/10.1016/j.diagmicrobio.2010.08.008 |
[154] | Shu CC, Wu MF, Hsu CL, et al. (2013) Apoptosis-associated biomarkers in tuberculosis: promising for diagnosis and prognosis prediction. BMC Infect Dis 13: 45. https://doi.org/10.1186/1471-2334-13-45 |
[155] | Goeijenbier M, Gasem MH, Meijers JCM, et al. (2015) Markers of endothelial cell activation and immune activation are increased in patients with severe leptospirosis and associated with disease severity. J Infect 71: 437-446. https://doi.org/10.1016/j.jinf.2015.05.016 |
[156] | Shu CC, Wang JY, Hsu CL, et al. (2015) Diagnostic role of inflammatory and anti-inflammatory cytokines and effector molecules of cytotoxic T lymphocytes in tuberculous pleural effusion. Respirology 20: 147-154. https://doi.org/10.1111/resp.12414 |
[157] | Korczynski P, Klimiuk J, Safianowska A, et al. (2019) Impact of age on the diagnostic yield of four different biomarkers of tuberculous pleural effusion. Tuberculosis 114: 24-29. https://doi.org/10.1016/j.tube.2018.11.004 |
[158] | Struck NS, Zimmermann M, Krumkamp R, et al. (2020) Cytokine profile distinguishes children with Plasmodium falciparum malaria from those with bacterial blood stream infections. J Infect Dis 221: 1098-1106. https://doi.org/10.1093/infdis/jiz587 |
[159] | Nishigaki K, Minatoguchi S, Seishima M, et al. (1997) Plasma Fas ligand, an inducer of apoptosis, and plasma soluble Fas, an inhibitor of apoptosis, in patients with chronic congestive heart failure. J Am Coll Cardiol 29: 1214-1220. https://doi.org/10.1016/S0735-1097(97)00055-7 |
[160] | Sliwa K, Skudickey D, Bergemann A, et al. (2000) Peripartum cardiomyopathy: Analysis of clinical outcome, left ventricular function, plasma levels of cytokines and Fas/APO-1. J Am Coll Cardiol 35: 701-705. https://doi.org/10.1016/S0735-1097(99)00624-5 |
[161] | Adamopoulos S, Parissis J, Karatzas D, et al. (2002) Physical training modulates proinflammatory cytokines and the soluble Fas/soluble Fas ligand system in patients with chronic heart failure. J Am Coll Cardiol 39: 653-663. https://doi.org/10.1016/S0735-1097(01)01795-8 |
[162] | Shimizu M, Fukuo K, Nagata S, et al. (2002) Increased plasma levels of the soluble form of Fas ligand in patients with acute myocardial infarction and unstable angina pectoris. J Am Coll Cardiol 39: 585-590. https://doi.org/10.1016/S0735-1097(01)01800-9 |
[163] | Blanco-Colio LM, Martín-Ventura JL, Sol JM, et al. (2004) Decreased circulating Fas ligand in patients with familial combined hyperlipidemia or carotid atherosclerosis. Normalization by atorvastatin. J Am Coll Cardiol 43: 1188-1194. https://doi.org/10.1016/j.jacc.2003.10.046 |
[164] | Van der Meer IM, Oei HHS, Hofman A, et al. (2006) Soluble Fas, a mediator of apoptosis, C-reactive protein, and coronary and extracoronary atherosclerosis. The Rotterdam coronary calcification study. Atherosclerosis 189: 464-469. https://doi.org/10.1016/j.atherosclerosis.2006.01.004 |
[165] | Blanco-Colio LM, Martín-Ventura JL, de Teresa E, et al. (2007) Increased soluble Fas plasma levels in subjects at high cardiovascular risk. Atorvastatin on inflammatory markers (AIM) study, a substudy of ACTFAST. Arterioscler Thromb Vasc Biol 27: 168-174. https://doi.org/10.1161/01.ATV.0000250616.26308.d7 |
[166] | Blanco-Colio LM, Martín-Ventura JL, Tuñón J, et al. (2008) Soluble Fas ligand plasma levels are associated with forearm reactive hyperemia in subjects with coronary artery disease. A novel biomarker of endothelial function?. Atherosclerosis 201: 407-412. https://doi.org/10.1016/j.atherosclerosis.2008.02.005 |
[167] | Boos CJ, Balakrishnan B, Blann AD, et al. (2008) The relationship of circulating endothelial cells to plasma indices of endothelial damage/dysfunction and apoptosis in acute coronary syndromes: Implications for prognosis. J Thromb Haemost 6: 1841-1850. https://doi.org/10.1111/j.1538-7836.2008.03148.x |
[168] | Lanfear DE, Hasan R, Gupta RC, et al. (2009) Short term effects of milrinone on biomarkers of necrosis, apoptosis, and inflammation in patients with severe heart failure. J Trans Med 7: 67. https://doi.org/10.1186/1479-5876-7-67 |
[169] | Cardinal H, Brophy JM, Bogaty P, et al. (2010) Usefulness of soluble Fas levels for improving diagnostic accuracy and prognosis for acute coronary syndromes. Am J Cardiol 105: 797-803. https://doi.org/10.1016/j.amjcard.2009.10.061 |
[170] | Oyama JI, Maeda T, Sasaki M, et al. (2010) Green tea catechins improve human forearm vascular function and have potent anti-inflammatory and anti-apoptotic effects in smokers. Intern Med 49: 2553-2559. https://doi.org/10.2169/internalmedicine.49.4048 |
[171] | Cross DS, McCarty CA, Hytopoulos E, et al. (2012) Improved coronary risk assessment among intermediate risk patients using a clinical and biomarker based algorithm developed and validated in two population cohorts. Curr Med Res Opin 28: 1819-1830. https://doi.org/10.1185/03007995.2012.742878 |
[172] | Fertin M, Bauters A, Pinet F, et al. (2012) Circulating levels of soluble Fas ligand and left ventricular remodeling after acute myocardial infarction (from the REVE-2 study). J Cardiol 60: 93-97. https://doi.org/10.1016/j.jjcc.2012.03.001 |
[173] | Kinugawa T, Kato M, Yamamoto K, et al. (2012) Proinflammatory cytokine activation is linked to apoptotic mediator; soluble Fas level in patients with chronic heart failure. Int Heart J 53: 182-186. https://doi.org/10.1536/ihj.53.182 |
[174] | Sahinarslan A, Boyaci B, Kocaman SA, et al. (2012) The relationship of serum soluble Fas ligand (sFasL) level with the extent of coronary artery disease. Int J Angiol 21: 29-34. https://doi.org/10.1055/s-0032-1306418 |
[175] | Nilsson L, Szymanowski A, Swahn E, et al. (2013) Soluble TNF receptors are associated with infarct size and ventricular dysfunction in ST-elevation myocardial infarction. Plos One 8: e55477. https://doi.org/10.1371/journal.pone.0055477 |
[176] | Osmancik P, Teringova E, Tousek P, et al. (2013) Prognostic value of TNF-related apoptosis inducing ligand (TRAIL) in acute coronary syndrome patients. Plos One 8: e53860. https://doi.org/10.1371/journal.pone.0053860 |
[177] | Shah NR, Bieniarz MC, Basra SS, et al. (2013) Serum biomarkers in severe refractory cardiogenic shock. J Am Coll Cardiol HF 1: 200-206. https://doi.org/10.1016/j.jchf.2013.03.002 |
[178] | Szymanowski A, Li W, Lundberg A, et al. (2014) Soluble Fas ligand is associated with natural killer cell dynamics in coronary artery disease. Atherosclerosis 233: 616-622. https://doi.org/10.1016/j.atherosclerosis.2014.01.030 |
[179] | Taneja AK, Gaze D, Coats AJS, et al. (2014) Effects of nebivolol on biomarkers in elderly patients with heart failure. Int J Cardiol 175: 253-260. https://doi.org/10.1016/j.ijcard.2014.05.018 |
[180] | Chang TY, Hsu CY, Huang PH, et al. (2015) Usefulness of circulating decoy receptor 3 in predicting coronary artery disease severity and future major adverse cardiovascular events in patients with multivessel coronary artery disease. Am J Cardiol 116: 1028-1033. https://doi.org/10.1016/j.amjcard.2015.06.041 |
[181] | Adly AA, Ismail EA, Andrawes NG, et al. (2016) Soluble Fas/FasL ratio as a marker of vasculopathy in children and adolescents with sickle cell disease. Cytokine 79: 52-58. https://doi.org/10.1016/j.cyto.2015.12.022 |
[182] | Iso H, Maruyama K, Eshak ES, et al. (2017) Blood soluble Fas levels and mortality from cardiovascular disease in middle-aged Japanese: The JACC study. Atherosclerosis 260: 97-101. https://doi.org/10.1016/j.atherosclerosis.2017.03.020 |
[183] | Mattisson IY, Björkbacka H, Wigren M, et al. (2017) Elevated markers of death receptor-activated apoptosis are associated with increased risk for development of diabetes and cardiovascular disease. EBioMedicine 26: 187-197. https://doi.org/10.1016/j.ebiom.2017.11.023 |
[184] | Yan Y, Song D, Liu L, et al. (2017) The relationship of plasma decoy receptor 3 and coronary collateral circulation in patients with coronary artery disease. Life Sci 189: 84-88. https://doi.org/10.1016/j.lfs.2017.09.025 |
[185] | Li XY, Hou HT, Chen HX, et al. (2018) Increased circulating levels of tumor necrosis factor-like cytokine IA and decoy receptor 3 correlate with SYNTAX score in patients undergoing coronary surgery. J Int Med Res 46: 5167-5175. https://doi.org/10.1177/0300060518793787 |
[186] | Chen X, Wang R, Chen W, et al. (2019) Decoy receptor-3 regulates inflammation and apoptosis via PI3K/AKT signaling pathway in coronary heart disease. Exp Ther Med 17: 2614-2622. https://doi.org/10.3892/etm.2019.7222 |
[187] | Miyoshi T, Hosoda H, Nakai M, et al. (2019) Maternal biomarkers for fetal heart failure in fetuses with congenital heart defects or arrhythmias. Am J Obstet Gynecol 220: 104.e1-15. https://doi.org/10.1016/j.ajog.2018.09.024 |
[188] | Younus M, Fan W, Harrington DS, et al. (2019) Usefulness of a coronary artery disease predictive algorithm to predict global risk for cardiovascular disease and acute coronary syndrome. Am J Cardiol 123: 769-775. https://doi.org/10.1016/j.amjcard.2018.11.044 |
[189] | Doustkami H, Avesta L, Babapour B, et al. (2021) Correlation of serum decoy receptor 3 and interleukin-6 with severity of coronary artery diseases in male acute myocardial infarction patients. Acta Biomed 92: e2021285. https://doi.org/10.23750/abm.v92i5.9711 |
[190] | Masse M, Hérbert MJ, Troyanov S, et al. (2002) Soluble Fas is a marker of peripheral arterial occlusive disease in haemodialysis patients. Nephrol Dial Transplant 17: 485-491. https://doi.org/10.1093/ndt/17.3.485 |
[191] | El-Agroudy AE, El-Baz A (2010) Soluble Fas: a useful marker of inflammation and cardiovascular diseases in uremic patients. Clin Exp Nephrol 14: 152-157. https://doi.org/10.1007/s10157-009-0261-8 |
[192] | Chang EP, Lin YS, Huang SC, et al. (2012) High serum DcR3 levels are associated with the occurrence of peritonitis in patients receiving chronic peritoneal dialysis. J Chin Med Assoc 75: 644-648. https://doi.org/10.1016/j.jcma.2012.09.009 |
[193] | Dounousi E, Koliousi E, Papagianni A, et al. (2012) Mononuclear leukocyte apoptosis and inflammatory markers in patients with chronic kidney disease. Am J Nephrol 36: 531-536. https://doi.org/10.1159/000345352 |
[194] | Hung SC, Hsu TW, Lin YP, et al. (2012) Decoy receptor 3, a novel inflammatory marker, and mortality in hemodialysis patients. Clin J Am Soc Nephrol 7: 1257-1265. https://doi.org/10.2215/CJN.08410811 |
[195] | Bartnicki P, Rysz J, Franczyk B, et al. (2016) Impact of continuous erythropoietin receptor activator on selected biomarkers of cardiovascular disease and left ventricle structure and function in chronic kidney disease. Oxid Med Cell Longev 2016: 9879615. https://doi.org/10.1155/2016/9879615 |
[196] | Bhatraju PK, Robinson-Cohen C, Mikacenic C, et al. (2017) Circulating levels of soluble Fas (sCD95) are associated with risk for development of a nonresolving acute kidney injury subphenotype. Critical Care 21: 217. https://doi.org/10.1186/s13054-017-1807-x |
[197] | Claro LM, Moreno-Amaral AN, Gadotti AC, et al. (2018) The impact of uremic toxicity induced inflammatory response on the cardiovascular burden in chronic kidney disease. Toxins 10: 384. https://doi.org/10.3390/toxins10100384 |
[198] | Raptis V, Bakogiannis C, Loutradis C, et al. (2018) Serum Fas ligand, serum myostatin and urine TGF-β1 are elevated in autosomal dominant polycystic kidney disease patients with impaired and preserved renal function. Kidney Blood Press Res 43: 744-754. https://doi.org/10.1159/000489911 |
[199] | Bhatraju PK, Zelnick LR, Katz R, et al. (2019) A prediction model for severe AKI in critically ill adults that incorporates clinical and biomarker data. Clin J Am Soc Nephrol 14: 506-514. https://doi.org/10.2215/CJN.04100318 |
[200] | Chiloff DM, de Almeida DC, Dalboni MA, et al. (2020) Soluble Fas affects erythropoiesis in vitro and acts as a potential predictor of erythropoiesis-stimulating agent therapy in patients with chronic kidney disease. Am J Physiol Renal Physiol 318: F861-F869. https://doi.org/10.1152/ajprenal.00433.2019 |
[201] | Abu-Rajab Tamimi TI, Elgouhari HM, Alkhouri N, et al. (2011) An apoptosis panel for nonalcoholic steatohepatitis diagnosis. J Hepatol 54: 1224-1229. https://doi.org/10.1016/j.jhep.2010.08.023 |
[202] | Fealy CE, Haus JM, Solomon TPJ, et al. (2012) Short-term exercise reduces markers of hepatocyte apoptosis in nonalcoholic fatty liver disease. J Appl Physiol 113: 1-6. https://doi.org/10.1152/japplphysiol.00127.2012 |
[203] | Page S, Birerdinc A, Estep M, et al. (2013) Knowledge-based identification of soluble biomarkers: Hepatic fibrosis in NAFLD as an example. Plos One 8: e56009. https://doi.org/10.1371/journal.pone.0056009 |
[204] | El Bassat H, Ziada DH, Hasby EA, et al. (2014) Apoptotic and anti-apoptotic seromarkers for assessment of disease severity of non-alcoholic steatohepatitis. Arab J Gastroenterol 15: 6-11. https://doi.org/10.1016/j.ajg.2014.01.009 |
[205] | Buck M, Garcia-Tsao G, Groszmann RJ, et al. (2014) Novel inflammatory biomarkers of portal pressure in compensated cirrhosis patients. Hepatology 59: 1052-1059. https://doi.org/10.1002/hep.26755 |
[206] | Alkhouri N, Alisi A, Okwu V, et al. (2015) Circulating soluble Fas and Fas ligand levels are elevated in children with nonalcoholic steatohepatitis. Dig Dis Sci 60: 2353-2359. https://doi.org/10.1007/s10620-015-3614-z |
[207] | Ajmera V, Perito ER, Bass NM, et al. (2017) Novel plasma biomarkers associated with liver disease severity in adults with nonalcoholic fatty liver disease. Hepatology 65: 65-77. https://doi.org/10.1002/hep.28776 |
[208] | Perito ER, Ajmera V, Bass NM, et al. (2017) Association between cytokines and liver histology in children with nonalcoholic fatty liver disease. Hepatol Comm 1: 609-622. https://doi.org/10.1002/hep4.1068 |
[209] | Lin S, Wu B, Lin Y, et al. (2019) Expression and clinical significance of decoy receptor 3 in acute-on-chronic liver failure. BioMed Res Int 2019: 9145736. https://doi.org/10.1155/2019/9145736 |
[210] | Yasuda N, Gotoh K, Minatoguchi S, et al. (1998) An increase of soluble Fas, an inhibitor of apoptosis, associated with progression of COPD. Respir Med 92: 993-999. https://doi.org/10.1016/S0954-6111(98)90343-2 |
[211] | Takabatake N, Arao T, Sata M, et al. (2005) Circulating levels of soluble Fas ligand in cachexic patients with COPD are higher than those in non-cachexic patients with COPD. Intern Med 44: 1137-1143. https://doi.org/10.2169/internalmedicine.44.1137 |
[212] | Chen CY, Yang KY, Chen MY, et al. (2009) Decoy receptor 3 levels in peripheral blood predict outcomes of acute respiratory distress syndrome. Am J Respir Crit Care Med 180: 751-760. https://doi.org/10.1164/rccm.200902-0222OC |
[213] | Carolan BJ, Hughes G, Morrow J, et al. (2014) The association of plasma biomarkers with computed tomography-assessed emphysema phenotypes. Respir Res 15: 127. https://doi.org/10.1186/s12931-014-0127-9 |
[214] | Stapleton RD, Suratt BT, Neff MJ, et al. (2019) Bronchoalveolar fluid and plasma inflammatory biomarkers in contemporary ARDS patients. Biomarkers 24: 352-359. https://doi.org/10.1080/1354750X.2019.1581840 |
[215] | Aydin H, Tekin YK, Korkmaz I, et al. (2020) Apoptosis biomarkers (APAF-1, sFas, sFas-L, and caspase-9), albumin, and fetuin-A levels in pulmonary thromboembolic patients. Disaster Emerg Med J 5: 1-6. https://doi.org/10.5603/DEMJ.a2020.0005 |
[216] | Ghobadi H, Hosseini N, Aslani MR (2020) Correlations between serum decoy receptors 3 and airflow limitation and quality of life in male patients with stable stage and acute exacerbation of COPD. Lung 198: 515-523. https://doi.org/10.1007/s00408-020-00348-z |
[217] | Felderhoff-Mueser U, Bührer C, Groneck P, et al. (2003) Soluble Fas (CD95/Apo-1), soluble Fas ligand, and activated caspase 3 in the cerebrospinal fluid of infants with posthemorrhagic and nonhemorrhagic hydrocephalus. Pediatr Res 54: 659-664. https://doi.org/10.1203/01.PDR.0000084114.83724.65 |
[218] | Sival DA, Felderhoff-Müser U, Schmitz T, et al. (2008) Neonatal high pressure hydrocephalus is associated with elevation of pro-inflammatory cytokines IL-18 and IFNγ in cerebrospinal fluid. Cerebrospinal Fluid Res 5: 21. https://doi.org/10.1186/1743-8454-5-21 |
[219] | Craig-Schapiro R, Kuhn M, Xiong C, et al. (2011) Multiplexed immunoassay panel identifies novel CSF biomarkers for Alzheimer's disease diagnosis and prognosis. Plos One 6: e18850. https://doi.org/10.1371/journal.pone.0018850 |
[220] | Leifsdottir K, Mehmet H, Eksborg S, et al. (2018) Fas-ligand and interleukin-6 in the cerebrospinal fluid are early predictors of hypoxic-ischemic encephalopathy and long-term outcomes after birth asphyxia in term infants. J Neuroinflammation 15: 223. https://doi.org/10.1186/s12974-018-1253-y |
[221] | Jiang W, Jin P, Wei W, et al. (2020) Apoptosis in cerebrospinal fluid as outcome predictors in severe traumatic brain injury. An observational study. Medicine 99: e20922. https://doi.org/10.1097/MD.0000000000020922 |
[222] | Niewczas MA, Ficociello LH, Johnson AC, et al. (2009) Serum concentrations of markers of TNFα and Fas-mediated pathways and renal function in nonproteinuric patients with type 1 diabetes. Clin J Am Soc Nephrol 4: 62-70. https://doi.org/10.2215/CJN.03010608 |
[223] | Hussian SK, Al-Karawi IN, Sahab KS (2013) Role of Fas/Fas ligand pathway in a sample of Iraqi diabetic foot patients. Diyala J Med 5: 95-107. |
[224] | Stoynev N, Kainov K, Kirilov G, et al. (2014) Serum levels of sFas and sFasL in subjects with type 2 diabetes-the impact of arterial hypertension. Cent Eur J Med 9: 704-708. https://doi.org/10.2478/s11536-013-0318-7 |
[225] | Midena E, Micera A, Frizziero L, et al. (2019) Sub-threshold micropulse laser treatment reduces inflammatory biomarkers in aqueous humour of diabetic patients with macular edema. Sci Rep 9: 10034. https://doi.org/10.1038/s41598-019-46515-y |
[226] | Abe R, Shimizu T, Shibaki A, et al. (2003) Toxic epidermal necrolysis and Stevens-Johnson syndrome are induced by soluble Fas ligand. Am J Pathol 162: 1515-1520. https://doi.org/10.1016/S0002-9440(10)64284-8 |
[227] | Stur K, Karlhofer FM, Stingl G, et al. (2007) Soluble FAS ligand: A discriminating feature between drug-induced skin eruptions and viral exanthemas. J Invest Dermatol 127: 802-807. https://doi.org/10.1038/sj.jid.5700648 |
[228] | Chung WH, Hung SI, Yang JY, et al. (2008) Granulysin is a key mediator for disseminated keratinocyte death in Stevens-Johnson syndrome and toxic epidermal necrolysis. Nat Med 14: 1343-1350. https://doi.org/10.1038/nm.1884 |
[229] | Murata J, Abe R, Shimizu H (2008) Increased soluble Fas ligand levels in patients with Stevens-Johnson syndrome and toxic epidermal necrolysis preceding skin detachment. J Allergy Clin Immunol 122: 992-1000. https://doi.org/10.1016/j.jaci.2008.06.013 |
[230] | Genç ŞÖ, Karakuş S, Çetin A, et al. (2019) Serum Bcl-2, caspase-9 and soluble FasL as perinatal markers in late preterm pregnancies with intrauterine growth restriction. Turk J Pediatr 61: 686-696. https://doi.org/10.24953/turkjped.2019.05.007 |
[231] | Yeh CC, Yang MJ, Lussier EC, et al. (2019) Low plasma levels of decoy receptor 3 (DcR3) in the third trimester of pregnancy with preeclampsia. Taiwan J Obstet Gynecol 58: 349-353. https://doi.org/10.1016/j.tjog.2019.03.011 |
[232] | Oyama JI, Yamamoto H, Maeda T, et al. (2012) Continuous positive airway pressure therapy improves vascular dysfunction and decreases oxidative stress in patients with the metabolic syndrome and obstructive sleep apnea syndrome. Clin Cardiol 35: 231-236. https://doi.org/10.1002/clc.21010 |
[233] | Chedraui P, Escobar GS, Pérez-López FR, et al. (2014) Angiogenesis, inflammation and endothelial function in postmenopausal women screened for the metabolic syndrome. Maturitas 77: 370-374. https://doi.org/10.1016/j.maturitas.2014.01.014 |
[234] | Alkhouri N, Kheirandish-Gozal L, Matloob A, et al. (2015) Evaluation of circulating markers of hepatic apoptosis and inflammation in obese children with and without obstructive sleep apnea. Sleep Med 16: 1031-1035. https://doi.org/10.1016/j.sleep.2015.05.002 |
[235] | Farey JE, Fisher OM, Levert-Mignon AJ, et al. (2017) Decreased levels of circulating cancer-associated protein biomarkers following bariatric surgery. Obes Surg 27: 578-585. https://doi.org/10.1007/s11695-016-2321-y |
[236] | Goto M (2008) Elevation of soluble Fas (APO-1, CD95) ligand in natural aging and Werner syndrome. BioSci Trend 2: 124-127. |
[237] | Jiang S, Moriarty-Craige SE, Li C, et al. (2008) Associations of plasma-soluble Fas ligand with aging and age-related macular degeneration. Invest Ophthalmol Vis Sci 49: 1345-1349. https://doi.org/10.1167/iovs.07-0308 |
[238] | Karaflou M, Kaparos G, Rizos D, et al. (2010) Estrogen plus progestin treatment: effect of different progestin components on serum markers of apoptosis in healthy postmenopausal women. Fertil Steril 94: 2399-2401. https://doi.org/10.1016/j.fertnstert.2010.04.010 |
[239] | Kämppä N, Mäkelä KM, Lyytikäinen LP, et al. (2013) Vascular cell adhesion molecule 1, soluble Fas and hepatocyte growth factor as predictors of mortality in nonagenarians: The vitality 90+ study. Exp Gerontrol 48: 1167-1172. https://doi.org/10.1016/j.exger.2013.07.009 |
[240] | Kangas R, Pöllänen E, Rippo MR, et al. (2014) Circulating miR-21, miR-146a and Fas ligand respond to postmenopausal estrogen-based hormone replacement therapy—a study with monozygotic twin pairs. Mech Ageing Dev 143–144: 1-8. https://doi.org/10.1016/j.mad.2014.11.001 |
[241] | Wu Q, Chen H, Fang J, et al. (2012) Elevated Fas/FasL system and endothelial cell microparticles are involved in endothelial damage in acute graft-versus-host disease: A clinical analysis. Leuk Res 36: 275-280. https://doi.org/10.1016/j.leukres.2011.08.005 |
[242] | Fadel FI, Elshamaa MF, Salah A, et al. (2016) Fas/Fas ligand pathways gene polymorphisms in pediatric renal allograft rejection. Transpl Immunol 37: 28-34. https://doi.org/10.1016/j.trim.2016.04.006 |
[243] | Meki ARAM, Hasan HA, El-Deen ZMM, et al. (2003) Dysregulation of apoptosis in scorpion envenomed children: its reflection on their outcome. Toxicon 42: 229-237. https://doi.org/10.1016/S0041-0101(03)00128-4 |
[244] | Tamakoshi A, Suzuki K, Lin Y, et al. (2009) Cigarette smoking and serum soluble Fas levels: Findings from the JACC study. Mutat Res 679: 79-83. https://doi.org/10.1016/j.mrgentox.2009.08.002 |
[245] | Muraki M (2018) Development of expression systems for the production of recombinant human Fas ligand extracellular domain derivatives using Pichia pastoris and preparation of the conjugates by site-specific chemical modifications: A review. AIMS Bioengineer 5: 39-62. https://doi.org/10.3934/bioeng.2018.1.39 |
[246] | Muraki M, Hirota K (2019) Site-specific biotin-group conjugate of human Fas ligand extracellular domain: preparation and characterization of cell-death-inducing activity. Curr Top Pep Prot Res 20: 17-24. |
[247] | Muraki M, Honda S (2010) Efficient production of human Fas receptor extracellular domain-human IgG1 heavy chain Fc domain fusion protein using baculovirus/silkworm expression system. Prot Expr Purif 73: 209-216. https://doi.org/10.1016/j.pep.2010.05.007 |
[248] | Connolly K, Cho YH, Duan R, et al. (2001) In vivo inhibition of Fas ligand-mediated killing by TR6, a Fas ligand decoy receptor. J Pharmacol Exp Ther 298: 25-33. |
Density of X | Outlier rate | n=20 | n=100 | n=500 | ||||||
ˆrBNWn(x) | ˆθx | ˆrNWn(x) | ˆrBNWn(x) | ˆθx | ˆrNWn(x) | ˆrBNWn(x) | ˆθx | ˆrNWn(x) | ||
(a) N[0,1](0,1) |
0.00% | 0.37777 | 0.38362 | 0.37289 | 0.0386 | 0.04134 | 0.0333 | 0.01564 | 0.01684 | 0.00896 |
0.05% | 598.916 | 3.57632 | 690.548 | 678.998 | 2.20528 | 668.378 | 674.569 | 0.18818 | 692.737 | |
0.10% | 3016.57 | 5.65957 | 3000.05 | 2620.97 | 3.16347 | 2593.3 | 2676.12 | 0.23244 | 2682.89 | |
0.25% | 16083.1 | 14.182 | 15878.1 | 15896.3 | 6.29712 | 15930.6 | 16447.1 | 1.74161 | 16344.1 | |
(b) Exp(2) |
0.00% | 0.35574 | 0.35578 | 0.35517 | 0.05012 | 0.05283 | 0.03747 | 0.01611 | 0.01549 | 0.00794 |
0.05% | 748.855 | 4.2097 | 819.161 | 689.539 | 1.90398 | 692.123 | 683.571 | 0.21493 | 644.829 | |
0.10% | 2408.65 | 5.93149 | 2284.35 | 2501.28 | 3.57741 | 2432.78 | 2681.96 | 0.31808 | 2586 | |
0.25% | 16174.5 | 21.0217 | 16094.6 | 16228.2 | 6.62117 | 16834.3 | 17422.6 | 1.89746 | 17294.2 | |
(c) N(0,1) |
0.00% | 0.3345 | 0.33847 | 0.31945 | 0.05094 | 0.04832 | 0.03983 | 0.01667 | 0.01593 | 0.00886 |
0.05% | 770.807 | 4.51064 | 822.317 | 675.495 | 2.05097 | 665.198 | 698.8 | 0.15089 | 656.339 | |
0.10% | 2746.52 | 7.79586 | 2559.23 | 2436.1 | 3.05173 | 2393.47 | 2497.94 | 0.24955 | 2503.83 | |
0.25% | 19178.1 | 18.1898 | 18006 | 16413.5 | 8.0909 | 16893.9 | 17372.6 | 1.75941 | 17495.9 |
Density of X | Outlier rate | n=20 | n=100 | n=500 | ||||||
ˆrBNWn(x) | ˆθx | ˆrNWn(x) | ˆrBNWn(x) | ˆθx | ˆrNWn(x) | ˆrBNWn(x) | ˆθx | ˆrNWn(x) | ||
(a) N[0,1](0,1) |
0.00% | 1.48199 | 1.48019 | 1.47376 | 0.16874 | 0.25191 | 0.10863 | 0.04375 | 0.05576 | 0.02462 |
0.05% | 29.1125 | 2.49147 | 29.3273 | 14.8531 | 0.74622 | 16.0768 | 15.7507 | 1.66571 | 22.1559 | |
0.10% | 64.0281 | 2.90274 | 73.1002 | 53.5638 | 0.91596 | 49.9706 | 98.0555 | 0.90805 | 67.9633 | |
0.25% | 393.474 | 6.88132 | 326.212 | 1050.14 | 3.46544 | 700.888 | 1100.17 | 0.99655 | 751.459 | |
(b) Exp(2) |
0.00% | 1.39141 | 1.47957 | 1.33418 | 0.17829 | 0.22055 | 0.11882 | 0.05203 | 0.05178 | 0.02936 |
0.05% | 25.489 | 2.38298 | 28.3225 | 13.7623 | 0.54491 | 14.1639 | 31.6953 | 1.13268 | 11.5467 | |
0.10% | 71.5908 | 2.58522 | 75.7357 | 50.8943 | 1.02112 | 58.2273 | 114.747 | 1.50033 | 54.9639 | |
0.25% | 355.306 | 6.60588 | 289.937 | 867.431 | 2.26394 | 454.397 | 1327.96 | 0.47757 | 835.553 | |
(c) N(0,1) |
0.00% | 0.98856 | 1.05261 | 0.97223 | 0.16172 | 0.18081 | 0.09312 | 0.03957 | 0.04478 | 0.02101 |
0.05% | 25.528 | 2.25141 | 32.5343 | 22.6682 | 0.5839 | 15.2797 | 24.7481 | 1.48634 | 15.3258 | |
0.10% | 61.7528 | 2.64854 | 85.8806 | 75.1123 | 1.22781 | 45.4987 | 111.539 | 1.81279 | 64.8843 | |
0.25% | 469.185 | 9.35168 | 398.637 | 692.756 | 3.46427 | 642.386 | 1131.51 | 0.6867 | 526.238 |
Density of X | Outlier rate | n=20 | n=100 | n=500 | ||||||
ˆrBNWn(x) | ˆθx | ˆrNWn(x) | ˆrBNWn(x) | ˆθx | ˆrNWn(x) | ˆrBNWn(x) | ˆθx | ˆrNWn(x) | ||
(a) N[0,1](0,1) |
0.00% | 0.13301 | 0.12525 | 0.1154 | 0.01527 | 0.01522 | 0.01269 | 0.00414 | 0.00436 | 0.00316 |
0.05% | 19.3998 | 0.28717 | 20.5557 | 12.0525 | 0.12604 | 10.3004 | 9.47988 | 0.1339 | 8.91102 | |
0.10% | 58.565 | 0.52241 | 49.1399 | 33.1922 | 0.23475 | 34.4606 | 38.2786 | 0.16123 | 43.7728 | |
0.25% | 294.817 | 2.25567 | 177.939 | 266.142 | 0.54954 | 210.994 | 249.554 | 0.30488 | 273.319 | |
(b) Exp(2) |
0.00% | 0.14836 | 0.15054 | 0.12541 | 0.01324 | 0.0144 | 0.01196 | 0.0055 | 0.00545 | 0.00438 |
0.05% | 18.8191 | 0.2807 | 25.6982 | 10.7864 | 0.14311 | 9.74908 | 10.5176 | 0.18333 | 9.51805 | |
0.10% | 55.1011 | 0.45925 | 48.4941 | 44.8719 | 0.18583 | 33.2084 | 40.9161 | 0.17936 | 39.3012 | |
0.25% | 234.994 | 1.24542 | 189.692 | 251.89 | 0.60327 | 234.829 | 261.372 | 0.34955 | 285.042 | |
(c) N(0,1) |
0.00% | 0.13021 | 0.14029 | 0.12257 | 0.01506 | 0.01511 | 0.01375 | 0.00442 | 0.00442 | 0.00328 |
0.05% | 23.6259 | 0.28918 | 22.6131 | 12.1443 | 0.10116 | 10.529 | 9.82171 | 0.15066 | 9.71418 | |
0.10% | 56.098 | 0.4286 | 55.5514 | 36.7151 | 0.22296 | 36.6241 | 35.7651 | 0.1501 | 40.4612 | |
0.25% | 247.54 | 1.20312 | 237.361 | 224.049 | 0.50768 | 235.812 | 246.816 | 0.30212 | 276.141 |
Density of X | Outlier rate | n=20 | n=100 | n=500 | ||||||
ˆrBNWn(x) | ˆθx | ˆrNWn(x) | ˆrBNWn(x) | ˆθx | ˆrNWn(x) | ˆrBNWn(x) | ˆθx | ˆrNWn(x) | ||
(a) N[0,1](0,1) |
0.00% | 0.74703 | 0.61209 | 0.56581 | 0.17137 | 0.17318 | 0.1214 | 0.10734 | 0.08411 | 0.01222 |
0.05% | 1.98561 | 1.35548 | 1.86444 | 1.56581 | 0.60637 | 1.98072 | 5.36892 | 0.08762 | 3.43111 | |
0.10% | 5.07457 | 1.236 | 4.75581 | 7.88123 | 0.43664 | 7.38618 | 27.4856 | 0.11352 | 12.9594 | |
0.25% | 34.2112 | 2.53764 | 30.5081 | 141.657 | 1.78744 | 166.463 | 366.283 | 0.32839 | 243.809 | |
(b) Exp(2) |
0.00% | 0.52866 | 0.5851 | 0.47474 | 0.10082 | 0.13134 | 0.05472 | 0.03985 | 0.07932 | 0.0121 |
0.05% | 1.79819 | 1.06047 | 1.9109 | 1.31466 | 0.45844 | 1.7421 | 4.25689 | 0.07832 | 4.11877 | |
0.10% | 3.47787 | 1.33077 | 4.26777 | 10.9025 | 0.44565 | 11.0732 | 46.7737 | 0.09391 | 31.0859 | |
0.25% | 66.6146 | 1.7682 | 51.3565 | 196.824 | 1.64135 | 113.157 | 351.074 | 0.30732 | 317.418 | |
(c) N(0,1) |
0.00% | 0.74933 | 0.71865 | 0.5849 | 0.11883 | 0.19364 | 0.11473 | 0.10082 | 0.11145 | 0.01105 |
0.05% | 1.20693 | 0.61275 | 1.51455 | 1.40167 | 0.15919 | 1.27418 | 7.52656 | 0.0597 | 3.79191 | |
0.10% | 3.85005 | 1.01982 | 3.50089 | 14.9906 | 0.41717 | 10.9088 | 39.9271 | 0.11825 | 36.1256 | |
0.25% | 26.8219 | 2.48908 | 25.5696 | 145.728 | 1.13974 | 123.627 | 422.568 | 0.33036 | 202.644 |
n=20 | n=100 | n=500 | |
x0=0 | 0.0814 | 0.0968 | 0.1728 |
x0=0.5 | 0.5299 | 0.5734 | 0.6603 |
x0=1 | 0.0611 | 0.0702 | 0.0970 |
Ozone | Nitric Oxides | Nitrogen Dioxide | Sulphur Dioxide | Particulate Matter | |
ˆrBNWn(x) | 181 | 169 | 197 | 197 | 197 |
ˆθx | 121 | 149 | 101 | 173 | 181 |
Density of X | Outlier rate | n=20 | n=100 | n=500 | ||||||
ˆrBNWn(x) | ˆθx | ˆrNWn(x) | ˆrBNWn(x) | ˆθx | ˆrNWn(x) | ˆrBNWn(x) | ˆθx | ˆrNWn(x) | ||
(a) N[0,1](0,1) |
0.00% | 0.37777 | 0.38362 | 0.37289 | 0.0386 | 0.04134 | 0.0333 | 0.01564 | 0.01684 | 0.00896 |
0.05% | 598.916 | 3.57632 | 690.548 | 678.998 | 2.20528 | 668.378 | 674.569 | 0.18818 | 692.737 | |
0.10% | 3016.57 | 5.65957 | 3000.05 | 2620.97 | 3.16347 | 2593.3 | 2676.12 | 0.23244 | 2682.89 | |
0.25% | 16083.1 | 14.182 | 15878.1 | 15896.3 | 6.29712 | 15930.6 | 16447.1 | 1.74161 | 16344.1 | |
(b) Exp(2) |
0.00% | 0.35574 | 0.35578 | 0.35517 | 0.05012 | 0.05283 | 0.03747 | 0.01611 | 0.01549 | 0.00794 |
0.05% | 748.855 | 4.2097 | 819.161 | 689.539 | 1.90398 | 692.123 | 683.571 | 0.21493 | 644.829 | |
0.10% | 2408.65 | 5.93149 | 2284.35 | 2501.28 | 3.57741 | 2432.78 | 2681.96 | 0.31808 | 2586 | |
0.25% | 16174.5 | 21.0217 | 16094.6 | 16228.2 | 6.62117 | 16834.3 | 17422.6 | 1.89746 | 17294.2 | |
(c) N(0,1) |
0.00% | 0.3345 | 0.33847 | 0.31945 | 0.05094 | 0.04832 | 0.03983 | 0.01667 | 0.01593 | 0.00886 |
0.05% | 770.807 | 4.51064 | 822.317 | 675.495 | 2.05097 | 665.198 | 698.8 | 0.15089 | 656.339 | |
0.10% | 2746.52 | 7.79586 | 2559.23 | 2436.1 | 3.05173 | 2393.47 | 2497.94 | 0.24955 | 2503.83 | |
0.25% | 19178.1 | 18.1898 | 18006 | 16413.5 | 8.0909 | 16893.9 | 17372.6 | 1.75941 | 17495.9 |
Density of X | Outlier rate | n=20 | n=100 | n=500 | ||||||
ˆrBNWn(x) | ˆθx | ˆrNWn(x) | ˆrBNWn(x) | ˆθx | ˆrNWn(x) | ˆrBNWn(x) | ˆθx | ˆrNWn(x) | ||
(a) N[0,1](0,1) |
0.00% | 1.48199 | 1.48019 | 1.47376 | 0.16874 | 0.25191 | 0.10863 | 0.04375 | 0.05576 | 0.02462 |
0.05% | 29.1125 | 2.49147 | 29.3273 | 14.8531 | 0.74622 | 16.0768 | 15.7507 | 1.66571 | 22.1559 | |
0.10% | 64.0281 | 2.90274 | 73.1002 | 53.5638 | 0.91596 | 49.9706 | 98.0555 | 0.90805 | 67.9633 | |
0.25% | 393.474 | 6.88132 | 326.212 | 1050.14 | 3.46544 | 700.888 | 1100.17 | 0.99655 | 751.459 | |
(b) Exp(2) |
0.00% | 1.39141 | 1.47957 | 1.33418 | 0.17829 | 0.22055 | 0.11882 | 0.05203 | 0.05178 | 0.02936 |
0.05% | 25.489 | 2.38298 | 28.3225 | 13.7623 | 0.54491 | 14.1639 | 31.6953 | 1.13268 | 11.5467 | |
0.10% | 71.5908 | 2.58522 | 75.7357 | 50.8943 | 1.02112 | 58.2273 | 114.747 | 1.50033 | 54.9639 | |
0.25% | 355.306 | 6.60588 | 289.937 | 867.431 | 2.26394 | 454.397 | 1327.96 | 0.47757 | 835.553 | |
(c) N(0,1) |
0.00% | 0.98856 | 1.05261 | 0.97223 | 0.16172 | 0.18081 | 0.09312 | 0.03957 | 0.04478 | 0.02101 |
0.05% | 25.528 | 2.25141 | 32.5343 | 22.6682 | 0.5839 | 15.2797 | 24.7481 | 1.48634 | 15.3258 | |
0.10% | 61.7528 | 2.64854 | 85.8806 | 75.1123 | 1.22781 | 45.4987 | 111.539 | 1.81279 | 64.8843 | |
0.25% | 469.185 | 9.35168 | 398.637 | 692.756 | 3.46427 | 642.386 | 1131.51 | 0.6867 | 526.238 |
Density of X | Outlier rate | n=20 | n=100 | n=500 | ||||||
ˆrBNWn(x) | ˆθx | ˆrNWn(x) | ˆrBNWn(x) | ˆθx | ˆrNWn(x) | ˆrBNWn(x) | ˆθx | ˆrNWn(x) | ||
(a) N[0,1](0,1) |
0.00% | 0.13301 | 0.12525 | 0.1154 | 0.01527 | 0.01522 | 0.01269 | 0.00414 | 0.00436 | 0.00316 |
0.05% | 19.3998 | 0.28717 | 20.5557 | 12.0525 | 0.12604 | 10.3004 | 9.47988 | 0.1339 | 8.91102 | |
0.10% | 58.565 | 0.52241 | 49.1399 | 33.1922 | 0.23475 | 34.4606 | 38.2786 | 0.16123 | 43.7728 | |
0.25% | 294.817 | 2.25567 | 177.939 | 266.142 | 0.54954 | 210.994 | 249.554 | 0.30488 | 273.319 | |
(b) Exp(2) |
0.00% | 0.14836 | 0.15054 | 0.12541 | 0.01324 | 0.0144 | 0.01196 | 0.0055 | 0.00545 | 0.00438 |
0.05% | 18.8191 | 0.2807 | 25.6982 | 10.7864 | 0.14311 | 9.74908 | 10.5176 | 0.18333 | 9.51805 | |
0.10% | 55.1011 | 0.45925 | 48.4941 | 44.8719 | 0.18583 | 33.2084 | 40.9161 | 0.17936 | 39.3012 | |
0.25% | 234.994 | 1.24542 | 189.692 | 251.89 | 0.60327 | 234.829 | 261.372 | 0.34955 | 285.042 | |
(c) N(0,1) |
0.00% | 0.13021 | 0.14029 | 0.12257 | 0.01506 | 0.01511 | 0.01375 | 0.00442 | 0.00442 | 0.00328 |
0.05% | 23.6259 | 0.28918 | 22.6131 | 12.1443 | 0.10116 | 10.529 | 9.82171 | 0.15066 | 9.71418 | |
0.10% | 56.098 | 0.4286 | 55.5514 | 36.7151 | 0.22296 | 36.6241 | 35.7651 | 0.1501 | 40.4612 | |
0.25% | 247.54 | 1.20312 | 237.361 | 224.049 | 0.50768 | 235.812 | 246.816 | 0.30212 | 276.141 |
Density of X | Outlier rate | n=20 | n=100 | n=500 | ||||||
ˆrBNWn(x) | ˆθx | ˆrNWn(x) | ˆrBNWn(x) | ˆθx | ˆrNWn(x) | ˆrBNWn(x) | ˆθx | ˆrNWn(x) | ||
(a) N[0,1](0,1) |
0.00% | 0.74703 | 0.61209 | 0.56581 | 0.17137 | 0.17318 | 0.1214 | 0.10734 | 0.08411 | 0.01222 |
0.05% | 1.98561 | 1.35548 | 1.86444 | 1.56581 | 0.60637 | 1.98072 | 5.36892 | 0.08762 | 3.43111 | |
0.10% | 5.07457 | 1.236 | 4.75581 | 7.88123 | 0.43664 | 7.38618 | 27.4856 | 0.11352 | 12.9594 | |
0.25% | 34.2112 | 2.53764 | 30.5081 | 141.657 | 1.78744 | 166.463 | 366.283 | 0.32839 | 243.809 | |
(b) Exp(2) |
0.00% | 0.52866 | 0.5851 | 0.47474 | 0.10082 | 0.13134 | 0.05472 | 0.03985 | 0.07932 | 0.0121 |
0.05% | 1.79819 | 1.06047 | 1.9109 | 1.31466 | 0.45844 | 1.7421 | 4.25689 | 0.07832 | 4.11877 | |
0.10% | 3.47787 | 1.33077 | 4.26777 | 10.9025 | 0.44565 | 11.0732 | 46.7737 | 0.09391 | 31.0859 | |
0.25% | 66.6146 | 1.7682 | 51.3565 | 196.824 | 1.64135 | 113.157 | 351.074 | 0.30732 | 317.418 | |
(c) N(0,1) |
0.00% | 0.74933 | 0.71865 | 0.5849 | 0.11883 | 0.19364 | 0.11473 | 0.10082 | 0.11145 | 0.01105 |
0.05% | 1.20693 | 0.61275 | 1.51455 | 1.40167 | 0.15919 | 1.27418 | 7.52656 | 0.0597 | 3.79191 | |
0.10% | 3.85005 | 1.01982 | 3.50089 | 14.9906 | 0.41717 | 10.9088 | 39.9271 | 0.11825 | 36.1256 | |
0.25% | 26.8219 | 2.48908 | 25.5696 | 145.728 | 1.13974 | 123.627 | 422.568 | 0.33036 | 202.644 |
n=20 | n=100 | n=500 | |
x0=0 | 0.0814 | 0.0968 | 0.1728 |
x0=0.5 | 0.5299 | 0.5734 | 0.6603 |
x0=1 | 0.0611 | 0.0702 | 0.0970 |
Ozone | Nitric Oxides | Nitrogen Dioxide | Sulphur Dioxide | Particulate Matter | |
ˆrBNWn(x) | 181 | 169 | 197 | 197 | 197 |
ˆθx | 121 | 149 | 101 | 173 | 181 |