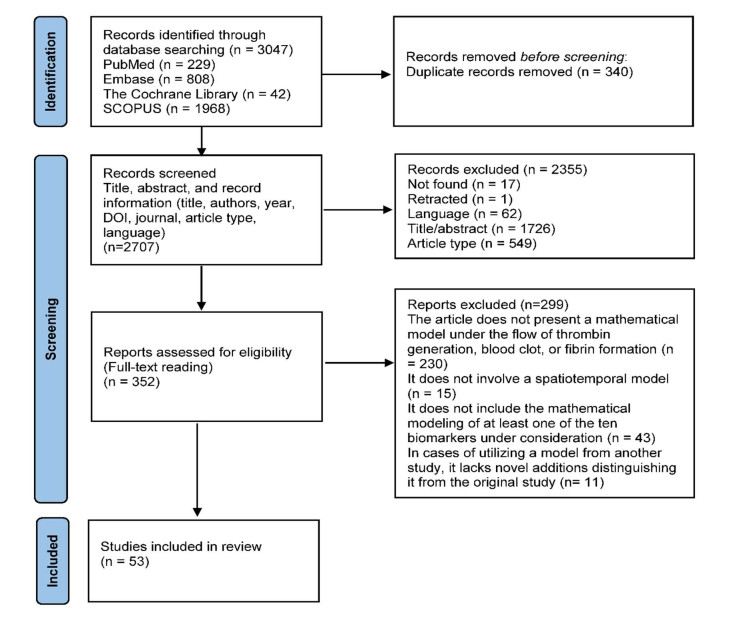
In the pursuit of personalized medicine, there is a growing demand for computational models with parameters that are easily obtainable to accelerate the development of potential solutions. Blood tests, owing to their affordability, accessibility, and routine use in healthcare, offer valuable biomarkers for assessing hemostatic balance in thrombotic and bleeding disorders. Incorporating these biomarkers into computational models of blood coagulation is crucial for creating patient-specific models, which allow for the analysis of the influence of these biomarkers on clot formation. This systematic review aims to examine how clinically relevant biomarkers are integrated into computational models of blood clot formation, thereby advancing discussions on integration methodologies, identifying current gaps, and recommending future research directions. A systematic review was conducted following the PRISMA protocol, focusing on ten clinically significant biomarkers associated with hemostatic disorders: D-dimer, fibrinogen, Von Willebrand factor, factor Ⅷ, P-selectin, prothrombin time (PT), activated partial thromboplastin time (APTT), antithrombin Ⅲ, protein C, and protein S. By utilizing this set of biomarkers, this review underscores their integration into computational models and emphasizes their integration in the context of venous thromboembolism and hemophilia. Eligibility criteria included mathematical models of thrombin generation, blood clotting, or fibrin formation under flow, incorporating at least one of these biomarkers. A total of 53 articles were included in this review. Results indicate that commonly used biomarkers such as D-dimer, PT, and APTT are rarely and superficially integrated into computational blood coagulation models. Additionally, the kinetic parameters governing the dynamics of blood clot formation demonstrated significant variability across studies, with discrepancies of up to 1, 000-fold. This review highlights a critical gap in the availability of computational models based on phenomenological or first-principles approaches that effectively incorporate affordable and routinely used clinical test results for predicting blood coagulation. This hinders the development of practical tools for clinical application, as current mathematical models often fail to consider precise, patient-specific values. This limitation is especially pronounced in patients with conditions such as hemophilia, protein C and S deficiencies, or antithrombin deficiency. Addressing these challenges by developing patient-specific models that account for kinetic variability is crucial for advancing personalized medicine in the field of hemostasis.
Citation: Mohamad Al Bannoud, Tiago Dias Martins, Silmara Aparecida de Lima Montalvão, Joyce Maria Annichino-Bizzacchi, Rubens Maciel Filho, Maria Regina Wolf Maciel. Integrating biomarkers for hemostatic disorders into computational models of blood clot formation: A systematic review[J]. Mathematical Biosciences and Engineering, 2024, 21(12): 7707-7739. doi: 10.3934/mbe.2024339
[1] | Adélia Sequeira, Rafael F. Santos, Tomáš Bodnár . Blood coagulation dynamics: mathematical modeling and stability results. Mathematical Biosciences and Engineering, 2011, 8(2): 425-443. doi: 10.3934/mbe.2011.8.425 |
[2] | Marios G. Krokidis, Themis P. Exarchos, Panagiotis Vlamos . Data-driven biomarker analysis using computational omics approaches to assess neurodegenerative disease progression. Mathematical Biosciences and Engineering, 2021, 18(2): 1813-1832. doi: 10.3934/mbe.2021094 |
[3] | Yan Wang, Yonghui Qiao, Yankai Mao, Chenyang Jiang, Jianren Fan, Kun Luo . Numerical prediction of thrombosis risk in left atrium under atrial fibrillation. Mathematical Biosciences and Engineering, 2020, 17(3): 2348-2360. doi: 10.3934/mbe.2020125 |
[4] | Avner Friedman, Wenrui Hao . Mathematical modeling of liver fibrosis. Mathematical Biosciences and Engineering, 2017, 14(1): 143-164. doi: 10.3934/mbe.2017010 |
[5] | Rebecca Everett, Kevin B. Flores, Nick Henscheid, John Lagergren, Kamila Larripa, Ding Li, John T. Nardini, Phuong T. T. Nguyen, E. Bruce Pitman, Erica M. Rutter . A tutorial review of mathematical techniques for quantifying tumor heterogeneity. Mathematical Biosciences and Engineering, 2020, 17(4): 3660-3709. doi: 10.3934/mbe.2020207 |
[6] | Sangita Swapnasrita, Joost C de Vries, Carl M. Öberg, Aurélie MF Carlier, Karin GF Gerritsen . Computational modeling of peritoneal dialysis: An overview. Mathematical Biosciences and Engineering, 2025, 22(2): 431-476. doi: 10.3934/mbe.2025017 |
[7] | Danilo T. Pérez-Rivera, Verónica L. Torres-Torres, Abraham E. Torres-Colón, Mayteé Cruz-Aponte . Development of a computational model of glucose toxicity in the progression of diabetes mellitus. Mathematical Biosciences and Engineering, 2016, 13(5): 1043-1058. doi: 10.3934/mbe.2016029 |
[8] | James C. L. Chow . Computational physics and imaging in medicine. Mathematical Biosciences and Engineering, 2025, 22(1): 106-108. doi: 10.3934/mbe.2025005 |
[9] | Benjamin Steinberg, Yuqing Wang, Huaxiong Huang, Robert M. Miura . Spatial Buffering Mechanism: Mathematical Model and Computer Simulations. Mathematical Biosciences and Engineering, 2005, 2(4): 675-702. doi: 10.3934/mbe.2005.2.675 |
[10] | Georgiana Eftimie, Raluca Eftimie . Quantitative predictive approaches for Dupuytren disease: a brief review and future perspectives. Mathematical Biosciences and Engineering, 2022, 19(3): 2876-2895. doi: 10.3934/mbe.2022132 |
In the pursuit of personalized medicine, there is a growing demand for computational models with parameters that are easily obtainable to accelerate the development of potential solutions. Blood tests, owing to their affordability, accessibility, and routine use in healthcare, offer valuable biomarkers for assessing hemostatic balance in thrombotic and bleeding disorders. Incorporating these biomarkers into computational models of blood coagulation is crucial for creating patient-specific models, which allow for the analysis of the influence of these biomarkers on clot formation. This systematic review aims to examine how clinically relevant biomarkers are integrated into computational models of blood clot formation, thereby advancing discussions on integration methodologies, identifying current gaps, and recommending future research directions. A systematic review was conducted following the PRISMA protocol, focusing on ten clinically significant biomarkers associated with hemostatic disorders: D-dimer, fibrinogen, Von Willebrand factor, factor Ⅷ, P-selectin, prothrombin time (PT), activated partial thromboplastin time (APTT), antithrombin Ⅲ, protein C, and protein S. By utilizing this set of biomarkers, this review underscores their integration into computational models and emphasizes their integration in the context of venous thromboembolism and hemophilia. Eligibility criteria included mathematical models of thrombin generation, blood clotting, or fibrin formation under flow, incorporating at least one of these biomarkers. A total of 53 articles were included in this review. Results indicate that commonly used biomarkers such as D-dimer, PT, and APTT are rarely and superficially integrated into computational blood coagulation models. Additionally, the kinetic parameters governing the dynamics of blood clot formation demonstrated significant variability across studies, with discrepancies of up to 1, 000-fold. This review highlights a critical gap in the availability of computational models based on phenomenological or first-principles approaches that effectively incorporate affordable and routinely used clinical test results for predicting blood coagulation. This hinders the development of practical tools for clinical application, as current mathematical models often fail to consider precise, patient-specific values. This limitation is especially pronounced in patients with conditions such as hemophilia, protein C and S deficiencies, or antithrombin deficiency. Addressing these challenges by developing patient-specific models that account for kinetic variability is crucial for advancing personalized medicine in the field of hemostasis.
Hemostatic disorders can indicate severe health conditions, including thrombotic and bleeding disorders, with varying degrees of risk, complexity, and patient impact. A procoagulant state can lead to dangerous diseases, such as deep vein thrombosis (DVT) or pulmonary embolism (PE), which are two manifestations of venous thromboembolism (VTE) that pose heightened risks for vascular complications. VTE emerges as the third primary contributor to mortality from cardiovascular diseases [1] and a significant contributor to the worldwide load of illness [2]. Notably, the incidence of thrombosis has increased significantly since the COVID-19 pandemic [3,4,5], emphasizing the need for increased attention to this hemostatic disorder. In contrast, a hypocoagulant state is characterized by a reduced ability of the blood to clot. Hemophilia, a classic example of a hypocoagulant state, involves a deficiency in specific clotting factors [6], impairing the body's capacity to form stable blood clots and resulting in a heightened risk of excessive bleeding, even from minor injuries. Research into the mechanisms underlying hemophilia has led to treatments that enable many patients to achieve a normal life expectancy [6]. Both clinical and computational studies have become valuable tools for deepening our understanding of hemophilia [7] and supporting the development of advanced therapies, including gene therapy [8].
The complexity and individualized nature of hemostatic disorders often render traditional "one-size-fits-all" diagnostic and therapeutic approaches inadequate. Such approaches fail to account for variations in individual patient risk factors, comorbidities, and responses to treatment [9]. Consequently, there has been an increasing shift from standardized treatment models to personalized medicine, which better accommodates patient-specific characteristics [9,10,11]. Personalized approaches address these limitations by tailoring diagnostics and interventions to each patient's unique hemostatic profile, with the potential to improve outcomes, reduce adverse effects, and optimize resource use [12,13,14,15]. Personalized management has shown promise in conditions like hereditary hemorrhagic telangiectasia [16], hemophilia A [17,18], and thrombosis [19]. The complexity of hemostatic disorders continues to pose challenges for researchers and healthcare providers in developing effective, individualized diagnostic and management strategies.
The diagnosis of patients with suspected VTE typically involves three main approaches: clinical pre-test probability assessment, D-dimer testing, and imaging [20]. Clinical pre-test probability, determined through established scoring systems like the Wells score, estimates the likelihood of VTE based on clinical factors and patient history. For instance, the Wells score assesses variables such as recent immobilization or surgery, active cancer, leg swelling, and previous VTE history to classify patients into low, moderate, or high-risk categories for thrombosis. This stratification helps prioritize further testing based on each patient's risk level. The D-dimer test, a blood test that detects fibrin degradation products, is a sensitive (though nonspecific) marker for clot formation. A negative D-dimer result (below a specified threshold) in patients with low clinical pre-test probability can effectively rule out VTE, sparing patients from further imaging. However, a confirmed VTE diagnosis requires imaging, such as ultrasound for DVT or computed tomography pulmonary angiography for PE, which provides direct visualization of the thrombus [21]. Given the high costs and limited accessibility of imaging, there is substantial interest in refining clinical pre-test methods that incorporate cost-effective and accessible tests while maintaining high sensitivity and specificity. Emerging clinical scoring systems and data-driven prediction models are promising, aiming to reduce unnecessary imaging and streamline the diagnostic process [22,23].
The diagnosis of hemophilia involves a series of blood tests to assess the blood's ability to clot and to measure the levels of specific clotting factors. Initially, a complete blood count and coagulation screening tests, such as prothrombin time (PT) and activated partial thromboplastin time (APTT), are conducted to identify any clotting abnormalities [24,25]. If these tests suggest a clotting issue, more specific assays are performed to measure the levels of clotting factor Ⅷ or Ⅸ to determine the type and severity of hemophilia (A or B). Genetic testing may also be used, especially for those with a family history of hemophilia, to identify the specific gene mutations responsible [26]. Early and accurate diagnosis is crucial for managing hemophilia effectively and preventing complications associated with bleeding episodes.
Clinical scores, such as the Wells score, rely on patient characteristics, medical history, symptoms, and exam results to assess the risk of developing VTE and PE [27]. These scoring systems are widely used in clinical practice due to their simplicity and accessibility, allowing quick risk stratification without needing advanced testing. For instance, Kafeza et al. [28] reviewed the Wells score and other clinical scoring systems for DVT, highlighting their widespread use. However, despite their popularity, clinical scores have several limitations that impact their effectiveness across different patient populations, especially in non-hospitalized or outpatient settings where clinical presentation can vary significantly [29,30].
One major limitation is the reliance on subjective clinical judgment in scores like the Wells score. For example, the criterion "an alternative diagnosis is less likely than PE" is open to interpretation, which can introduce variability in scoring and reduce diagnostic consistency across healthcare providers [31]. Additionally, many of these scores, including HERDOO2, Vienna, and DASH, are applied approximately 3–4 months after initiating anticoagulant therapy, limiting their predictive value for patients who discontinue anticoagulants earlier or remain at high risk for recurrence after this period [32]. The Wells score itself is not designed for recurrent VTE and is less effective in patients with atypical risk profiles. Similarly, the HERDOO2 score, used to assess recurrence risk, includes the D-dimer measurement while on anticoagulant therapy, which can impair its predictive accuracy in some instances [32].
Scoring systems specific to high-risk populations, such as those used for cancer patients, also have notable limitations. For instance, the Khorana score, a widely used tool for predicting VTE risk in cancer patients, demonstrates variable predictive performance across different cancer types and has limited accuracy in categorizing patients into high-risk groups. This results in a modest predictive value [33].
For hypocoagulation disorders, the ISTH bleeding score [34] is a valuable tool for assessing bleeding risk and severity in patients with bleeding disorders, including those with hemophilia [35]. However, it has limitations. It is not tailored for all patients, particularly those with rare bleeding disorders, and its reliance on self-reported data introduces variability, as patients may interpret and report bleeding events differently.
Modeling can enhance risk assessment by integrating diverse data sources, such as laboratory results, imaging findings, and detailed patient demographics. This comprehensive approach allows for more precise evaluations of hemostatic disorders by considering factors that may be overlooked in simpler scoring systems. Advanced analytical techniques, such as machine learning algorithms, can analyze complex interactions among multiple variables, revealing patterns that traditional methods might miss. Additionally, these models can provide personalized risk assessments tailored to individual patient profiles, improving predictive accuracy and reducing the likelihood of false positives and negatives [36]. By leveraging real-time data and continuously adapting to new information, predictive models can facilitate timely and informed clinical decisions, thereby improving patient outcomes in VTE management [37].
The growth in data storage capacity, processing capacity, and the expansion of electronic health records has popularized data-driven methodologies for predicting hemostatic disorders using machine learning, mainly because these methods can manage a high number of variables and observations, enabling the extraction of complex patterns and relationships [38] that may elude human perception when faced with large datasets. In this context, leveraging information from blood tests is feasible [39], given their rich array of variables, including biomarkers known to indicate VTE [40,41,42,43].
An alternative now gaining prominence is the use of computational methods based on first principles and mathematical modeling approaches to enhance understanding by incorporating detailed, mechanistic information. Unlike machine learning models, these computational mathematical models offer greater interpretability, the capacity for extrapolation, and deeper insights into the underlying biological and physical mechanisms of disease processes. However, there are contexts where these models can be more challenging to implement due to their higher computational demands and the complexity involved in accurately capturing intricate biological interactions compared to data-driven approaches [44,45].
In general, first-principle models are constructed using coupled ordinary differential equations (ODEs) or partial differential equations (PDEs), as these are well-suited for representing dynamic physical and biological processes [46]. Once the governing principles of a system are well understood, they can be computationally implemented, and the corresponding equations solved. Solving these systems, however, can be computationally intensive, particularly for complex biological or physical interactions [47]. Data-driven approaches, in contrast, typically rely on matrix operations and decision-making algorithms, which are computationally efficient but often demand large datasets and significant computational resources for model training. The process of collecting and processing these extensive datasets can be both time-consuming and costly. Additionally, the training phase itself can be resource-intensive and challenging, requiring various optimization algorithms, including classic methods such as interior-point and sequential quadratic programming, gradient-based algorithms, and even metaheuristic optimization techniques [48].
The computational complexity of first-principle and data-driven models can be considered in two main contexts. First, for pre-built models: Once trained or validated, data-driven models are generally computationally less expensive to deploy in real-time [for instance, replacing a system of ODEs with simpler matrix operations in models such as artificial neural network (ANN) [49]] or patient-specific applications [50]. However, this depends on the complexity of the specific data-driven model, as deep neural networks or other advanced algorithms can also be computationally intensive. Second, first-principle models typically require less empirical data because they are grounded in established physical or biological laws rather than learned patterns. This can make their initial development less data-intensive than data-driven models, which require large datasets and complex, computationally demanding training phases to achieve accuracy. In this context, data-driven models are indeed often more computationally expensive due to the need for high-dimensional data and iterative learning processes, which demand significant computational power and time.
Computational models of blood clot formation serve as valuable tools, offering insights into the complexities of this phenomenon [51]. These models enable the simulation of conditions representative of diseases affecting the hemostatic balance [7], aid in treatment planning [52], and facilitate the assessment of efficacy, safety, and dosage requirements of medications [53]. Despite their potential, these models face challenges in large-scale patient application, primarily due to the difficulties in determining parameters such as blood factor concentrations and kinetic rates because of limitations related to required equipment, time, and costs [52].
In a recent review, Watson et al. [54] examined the clinical challenges associated with VTE, exploring its complex biological aspects and the role of computational modeling in representing these factors. The authors highlighted that while several risk factors for VTE have been identified, the underlying biological mechanisms remain incompletely understood, complicating efforts to develop reliable predictive models. For instance, deficiencies in protein C, protein S, and antithrombin Ⅲ are established risk factors that influence VTE and related hemostatic disorders. Yet, these factors are difficult to integrate effectively in current computational models due to the complexity of their biological interactions. Watson et al. [54] conclude that existing computational models for VTE prediction are not yet sufficiently developed for clinical application, emphasizing the need for ongoing research to enhance their utility. In line with their recommendations, advancing these models may require incorporating additional variables associated with VTE risk, such as levels of protein C, protein S, and antithrombin Ⅲ, as well as biomarkers indicative of hemostatic dysregulation, including D-dimer and P-selectin. Further, incorporating routine coagulation assessments, such as PT and APTT, could improve model accuracy, making them more adaptable to individual patient profiles and, ultimately, more relevant for clinical use.
Our review builds on the insights of Watson et al. [54] by explicitly analyzing the mathematical integration of accessible clinical variables into computational models for blood clot formation, which has not been comprehensively examined in prior literature. The novelty of our review is the unique perspective on computational modeling that aligns with the study presented by Watson et al. [54]. By emphasizing the inclusion of clinically relevant risk factors and biomarkers, our review aims to support the development of more accurate, patient-specific computational tools for predicting conditions such as VTE and other hemostatic disorders.
In the pursuit of personalized medicine and practical clinical implementation, there is a growing need for computational models with more accessible obtainable parameters. Blood tests emerge as promising exams, characterized by their affordability, accessibility, and routine prescription by healthcare professionals. Moreover, blood tests provide crucial measurements of significant biomarkers in hemostasis, including D-dimer, p-selectin, and fibrinogen. In light of the above, we conducted a systematic review to comprehensively analyze and discuss how phenomenological models of blood clot formation under flow can mathematically represent blood biomarkers relevant to hemostatic disorders.
The contributions of this study are as follows:
• Compiling studies on computational models of blood clot formation that incorporate biomarkers.
• Standardizing the mathematical representations of biomarkers and their kinetic constants.
• Analyzing and discussing the integration of biomarkers into computational models with a focus on patient-specific perspectives.
• Identifying limitations and gaps in the mathematical modeling of these biomarkers.
• Recommending directions for future research.
The remaining sections are organized as follows: Section 2 outlines the methodology employed for the inclusion of studies in this systematic review. Section 3 presents the results, summarizing the included studies and providing an in-depth discussion of each study. Finally, the conclusions are presented. The supplementary material (SM) file contains tables detailing the references, mathematical expressions, kinetic parameter values, brief descriptions of the models used (if applicable), and original references for each biomarker. We recommend reading this article in conjunction with the SM file.
Given the abundance of biomarkers that indicate a hemostatic imbalance, this systematic review specifically targets a restricted set of biomarkers with key characteristics: reflection of coagulation, clinical utility, risk assessment, and treatment monitoring. Ten biomarkers and coagulation parameters were chosen: D-dimer, fibrinogen, Von Willebrand factor (vWF), factor Ⅷ, p-selectin, PT, APTT, antithrombin Ⅲ (ATIII), protein C/activated protein C (PC/PCA), and protein S (PS). Several studies have shown the importance of these factors as indicators of disturbances in hemostatic balance [55,56,57,58,59,60].
This systematic review adhered to the Preferred Reporting Items for Systematic Reviews and Meta-Analyses (PRISMA) protocol [61,62]. Literature extraction was conducted through searches in PubMed, Embase, the Cochrane Library, and SCOPUS. The record extraction date was December 31, 2023, with no start date filter applied.
The search strategy involved using keywords related to computational modeling, thrombosis, and the ten selected biomarkers, linked by logical operators "OR" and "AND", as exemplified below: ('mathematical model' "OR" 'mathematical modeling' "OR" 'computational modeling' "OR" 'computational simulation' "OR" 'computer simulation' "OR" 'model simulation') "AND" ('blood clot' "OR" 'thrombus' "OR" 'blood coagulation' "OR" 'blood plug') "AND" ('d-dimer' "OR" 'fibrinogen' "OR" 'von Willebrand factor' "OR" 'factor Ⅷ' "OR" 'p-selectin' "OR" 'prothrombin time' "OR" 'activated partial thromboplastin time' "OR" 'antithrombin' "OR" 'protein C' "OR" 'proteins')
Section S2 of the SM file provides the specific query sentence for each search engine.
The information on each record (title, authors, year, DOI, journal, article type, language) was stored in an electronic spreadsheet, and duplicates were removed. Two authors (MAB and TDM) independently conducted a thorough review and analysis of each record's title, abstract, and overall information. Records were sequentially excluded based on the following criteria: 1) not found; 2) retracted; 3) language (excluding records not in English); 4) article type (limited to full-length articles; reviews, commentaries, letters, and other types were excluded); and 5) abstract (articles not aligned with the topic of computational modeling and blood clot formation were excluded). Any discrepancies between the authors were deliberated and resolved through discussion with the other authors.
The complete texts of the remaining articles were meticulously examined and analyzed by two authors (MAB and TDM). Any discrepancies were resolved through consultation with the other authors. The following eligibility criteria were applied for the inclusion of records in this systematic review: 1) the article must include a mathematical model that accounts for the effects of flow and focuses explicitly on thrombin generation, blood clot formation, or fibrin formation; 2) it involves a spatiotemporal model; 3) it includes the mathematical modeling of at least one of the ten biomarkers under consideration; and 4) in cases where a model from another study is utilized, it introduces novel additions to the mathematical modeling that distinguish it from the original one. Articles failing to meet these four criteria were excluded.
Figure 1 illustrates the selection process for articles included in this review. Initially, a literature search yielded a total of 3047 records, of which 2707 underwent title and abstract screening. Subsequently, 352 studies underwent a full-text review to assess eligibility, resulting in the exclusion of studies that did not meet the eligibility criteria. In total, 53 studies satisfied the eligibility criteria and were included in this systematic review. Table 1 provides a list of these studies along with the biomarkers they included in their mathematical models. Additional abbreviations used are listed in Table S1 of the SM file.
Reference | Biomarkers | |||||||||
D-dimer | P-selectin | PT | APTT | vWF | Fibrinogen | ATIII | FⅧ | PC | PS | |
Sorensen et al. (1999) [63] | X | |||||||||
Boryczko et al. (2004) [64] | X | |||||||||
Pivkin et al. (2006) [65] | X | |||||||||
Xu et al. (2008) [66] | X | X | ||||||||
Xu et al. (2009) [67] | X | |||||||||
Xu et al. (2010) [68] | X | X | X | |||||||
Shibeko et al. (2010) [69] | X | X | X | X | ||||||
Jordan and Chaikof (2011) [70] | X | X | X | |||||||
Leiderman and Fogelson (2011) [71] | X | X | X | |||||||
Fogelson et al. (2012) [72] | X | X | X | |||||||
Leiderman and Fogelson (2013) [73] | X | X | X | |||||||
Tosenberger et al. (2013) [74] | ||||||||||
Sequeira and Bódnar (2014) [75] | X | X | X | X | ||||||
Tosenberger et al. (2015) [76] | X | |||||||||
Rukhlenko et al. (2015) [77] | X | |||||||||
Pavlova et al. (2015) [78] | X | X | X | X | ||||||
Piebalgs and Xu (2015) [79] | X | |||||||||
Li et al. (2015) [80] | X | X | X | X | ||||||
Bouchnita et al. (2016) [81] | X | X | X | |||||||
Seo et al. (2016) [82] | X | X | ||||||||
Ngoepe and Ventikos (2016) [83] | X | X | ||||||||
Bouchnita et al. (2016) [84] | X | X | X | |||||||
Dydek and Chaikof (2016) [85] | X | X | X | |||||||
Pavlova et al. (2016) [86] | X | X | X | |||||||
Tosenberger et al. (2016) [87] | X | |||||||||
Govindarajan et al. (2016) [88] | X | X | X | X | ||||||
Ou et al. (2017) [89] | X | X | X | X | ||||||
Yazdani et al. (2017) [90] | X | X | X | X | ||||||
Hosseinzadegan et al. (2017) [91] | X | |||||||||
Haynes et al. (2017) [92] | X | |||||||||
Kamada et al. (2017) [93] | X | X | ||||||||
Horn et al. (2018) [94] | X | X | X | X | ||||||
Méndez Rojano et al. (2018) [95] | X | |||||||||
Gu et al. (2019) [96] | X | |||||||||
Ayabe et al. (2019) [97] | X | |||||||||
Chen and Diamon (2019) [98] | X | X | ||||||||
Bouchnita and Volpert (2019) [99] | X | X | X | |||||||
Hosseinzadegan and Tafti (2019) [100] | X | |||||||||
Kadri et al. (2019) [101] | X | |||||||||
Du et al. (2020) [102] | X | X | ||||||||
Wu et al. (2020) [103] | X | X | ||||||||
Bouchnita et al. (2020) [104] | X | X | ||||||||
Liu et al. (2021) [105] | X | |||||||||
Kaneva et al. (2021) [106] | X | |||||||||
Yazdani et al. (2021) [107] | X | X | X | X | X | |||||
Ma et al. (2022) [108] | X | |||||||||
Méndez Rojano et al. (2022) [109] | X | X | ||||||||
Rezaeimoghaddam and van de Vosse (2022) [110] | X | |||||||||
Pisaryuk et al. (2022)[111] | X | X | X | X | X | |||||
Zhussupbekov et al. (2022) [112] | X | X | ||||||||
Wang et al. (2023) [113] | X | |||||||||
Petkantchin et al. (2023) [114] | X | |||||||||
Miyazawa et al. (2023)[115] | X | X | X | |||||||
PT: prothrombin time. APTT: activated partial thromboplastin time. vWF: von Willebrand factor. ATIII: antithrombin Ⅲ. FⅧ: factor Ⅷ. PC: protein C. PS: protein S. |
Among the most commonly studied biomarkers, ATIII and fibrin(ogen) are prominently featured, spanning studies from early investigations to the latest research. In contrast, biomarkers such as FⅧ and PC have been examined less frequently. Despite their established roles as thrombosis indicators, D-dimer and p-selectin have been infrequently studied, appearing primarily in studies from 2019 onward. PT and APTT were each investigated in a single recent study in 2022. Notably, the mathematical modeling studies reviewed in this study have not incorporated PS into the computational models.
A consistent feature among these studies is the representation of biomarkers as a reaction source term, depicting their role in the coagulation cascade (consumption/production). For instance, in models utilizing convective-diffusive-reactive (CDR) equations, the temporal and spatial changes for antithrombin are expressed as:
∂(Ci)∂t=∇·(Di∇Ci)−∇·(VCi)+Si | (1) |
Here, Ci represents the concentration of species i, Di represents the diffusion coefficient of species i, V is the velocity field, and Si is the reaction source term (RST).
ATIII stood out as the most commonly modeled biomarker in this systematic review. Table S4 in Section S5 of the SM provides mathematical expressions associated with ATIII. Table 2 outlines the kinetic parameters used in mathematical expressions for antithrombin Ⅲ, along with the initial concentration range of ATIII. Additional values are available in Tables S3 and S4 in the SM file.
Variable | Description | Range |
k++IIa/ATIII | Second-order inhibition rate constant of IIa by ATIII | 4.817 ×103 M-1.s-1 [99,104]–1.19 ×107 M-1.s-1 [75,78,80,90,107,113] |
k++IXa/ATIII | Second-order inhibition rate constant of IXa by ATIII | 1.36 ×102 M-1.s-1 [69] –2.7 ×105 M-1.s-1 [75,78,80,90,107,113] |
k++Xa/ATIII | Second-order inhibition rate constant of Xa by ATIII | 2.5 ×103 M-1.s-1 [111] –5.783 ×106 M-1.s-1 [75,78,80,90,107,113] |
k++XIa/ATIII | Second-order inhibition rate constant of XIa by ATIII | 8.0M-1.s-1 [111] –1.0 ×103 M-1.s-1 [94] |
k++XIIa/ATIII | Second-order inhibition rate constant of XIIa by ATIII | 3.645 ×101 M-1.s-1 [94] |
CATIII(t=0) | Initial concentration of ATIII | 1.566 ×10−6 M [78,86] –3.44 ×10−6 M [78,86] |
The RST term typically assumes the consumption through inhibition within the coagulation cascade, employing first- and second-order kinetics. Equation (2) provides a general representation of second-order kinetics, while Eq (3) represents first-order kinetics:
SATIII=−CATIII∑ni=1k++i,ATIIICi | (2) |
SATIII=−∑ni=1k+i,ATIIICi | (3) |
Here, SATIII is the RST of ATIII, CATIII is the concentration of ATIII, k++i,ATIII and k+i,ATIII are the second-order and first-order kinetic rates of inhibition of species i, …, n by ATIII, respectively, and Ci is the concentration of species i, …, n that suffers inhibition by ATIII.
Rezaeimoghaddam and van de Vosse [110] used coupled CDR equations to illustrate platelet and biomolecule transport, modeling thrombus formation and growth [Eq (S4.1)]. Equation (1) outlines the spatiotemporal dynamics of ATIII, depicting its consumption due to IIa inhibition with a second-order rate [Eq (2)]. This same approach was adopted by Bouchnita et al. [81] and Méndez Rojano et al. [109] [Eq (S4.1)]. In subsequent studies, Bouchnita et al. [99,104] expanded their investigation beyond ATIII consumption via thrombin inhibition alone. They introduced an additional term accounting for consumption through a second-order rate mechanism, specifically targeting the inhibition of the combined factors Ⅸa and Xa by ATIII [Eq (S4.2)]. However, they did not specify the reaction rates for each factor individually, only providing the sum for factors Ⅸa and Xa. Jordan and Chaikof [70] employed a similar approach, offering detailed kinetic rate information for factors Ⅸa and Xa [Eq (S4.3)]. Notably, the rates of Ⅸa and Xa were 1, 000 times greater than those used by Bouchnita et al. [99,104]. This discrepancy can be attributed to the fact that the kinetic constant in the study of Jordan and Chaikof [70] was taken from the study of Rezaie and Olson [116], which investigated the Ca2+ catalyzed reaction of antithrombin Ⅲ with blood factor Xa, resulting in an accelerated kinetic rate. Ou et al. [89] modeled the ATIII source term, considering inhibition of thrombin, factors Ⅸa, and Xa, utilizing a first-order rate constant [Eq (S4.4)].
Most models in this systematic review employed ATIII as an RST, representing a second-order inhibition of factors IIa, Ⅸa, Xa, and XIa [Eq (S4.5)] [75,78,80,90,107,113], based on the model by Anand et al. [117]. For personalized patient modeling, integrating factors IIa, Ⅸa, Xa, and XIa offers two key benefits: 1) it better reflects the dynamics of the coagulation cascade; and 2) it enables modeling of patients with deficiencies in these factors (hemophilia B, factor X deficiency, and hemophilia C). Horn et al. [94] incorporated factor XIIa in the PDE system, inhibited by thrombin with second-order kinetics [see Eq (S4.6)].
Bouchnita et al. [84] overlooked the dynamic evolution of ATIII, using it solely as a thrombin inhibitor [Eq (S4.7)]. Their study explored thrombin generation under two conditions, with and without blood flux, yielding distinct ATIII concentration expressions that impeded thrombin propagation. Remarkably, the threshold was lower with blood flow than without it. Pavlova et al. [78,86] proposed a synthetic blood coagulation model focusing on prothrombinase, thrombin, and fibrin. ATIII inhibits IIa and prothrombinase with second-order kinetics [Eq (S4.8)], providing a simpler alternative to the model by Anand et al. [117]. While flexible for ATIII deficiency studies, it may not suit hemophilia cases, where models involving factors Ⅸa, Xa, and XIa could be more applicable.
Xu et al. [68] simulated thrombin generation under static ODEs and PDEs under flow. In the static model, thrombin production stabilizes after peaking; however, in the ATIII-incorporated model, thrombin concentration decreases over time. The authors attributed this difference to the assumption in the ODE model of an infinite supply of binding sites on platelet surfaces for coagulant factors, contrasting with the finite number in the multiscale model. Both Xu et al. [68] and Ngoepe and Ventikos [83] employed the same expression for the antithrombin RST: second-order rate inhibition of thrombin, intermediate meizothrombin (mIIa), factor Ⅸa, Xa, and TF≡VIIa [Eq (S4.9)].
Dydek and Chaikof [85] incorporated heparin into their mathematical model, enhancing ATIII's thrombin inhibition capacity [Eq (S4.10)]. The model encompasses the inhibition of factors IIa, Ⅸa, and Xa. Heparin interacts with ATIII through second-order kinetics, while the complex dissociation between ATIII surface-bound heparin of lengths 5, 26, and 70 saccharides follows first-order kinetics.
Pisaryuk et al. [111] developed a comprehensive model to define personalized hemostasis profiles under low-molecular-weight heparin therapy [Eq (S4.11)]. Among the reviewed studies, this model stands out for its complexity in representing thrombin dynamics. It includes second-order inhibition kinetics for factors IIa, Ⅸa, Xa, XIa, Xa≡Vae, Xa≡Vav, and HC (see Table S3 of the SM file for variable description), along with thrombin aggregation with fibrinogen and fibrin. Additionally, it models HC≡ATIII dissociation using first-order kinetics.
Several studies [63,67,91,100,103,112] employed Griffith's model [118,119] to simulate the spatiotemporal evolution of ATIII in the presence of heparin. This model elucidates the kinetics of heparin-catalyzed thrombin inactivation by ATIII [Eq (S4.12)]. While Griffith's [118,119] model provides a valuable framework for understanding the kinetics of heparin-catalyzed thrombin inactivation by ATIII, it has several limitations, such as assumptions of homogeneity and simplified kinetics, as well as sensitivity to parameter values, which are challenging to accurately determine experimentally.
Blood clot growth can be modeled by considering platelet binding sites, where coagulation factors bind, react, and produce thrombin, contributing to platelet activation and aggregation. Leiderman and Fogelson [71] proposed a model for platelet aggregation and blood coagulation under flow, focusing on the chemical species bound to activated platelets [see Eq (S4.13)]. However, ATIII's spatiotemporal evolution was not addressed in this model. Instead, ATIII acted as a first-order inhibitor in equations for IIa, Ⅸa, and Xa. In the proposed model, platelet activation and aggregation impact both flow and diffusion processes, as platelet aggregation introduces a resistance term that impedes blood flow. Consequently, the effect of ATIII on other factors cascades through the system, altering thrombin production and further influencing flow and diffusion dynamics. The same model proposed by Leiderman and Fogelson [71] was used in two other studies [73,88] [see Eq (S4.13)]. Fogelson et al. [72] extended the model to include factor XIa, with ATIII also acting as a first-order inhibitor of Xa [see Eq (S4.14)]. Miyazawa et al. [115] introduced an equation describing the temporal evolution of ATIII [Eq (S4.15)] with second-order inhibition of factors IIa, Ⅸa, Xa, XIa, and heparin. Finally, Shibeko et al. [69] considered second-order kinetic pathways for IIa, Ⅸa, Xa, and the complex formed between Xa and Va [Eq (S4.16)].
According to Table 2, kinetic parameter values for ATIII inhibition may vary by up to three orders of magnitude, which significantly impacts the reliability, accuracy, and applicability of the models. ATIII exhibits high sensitivity in the coagulation cascade (Danforth et al. [120] and Naidu and Anand [121]), necessitating exact definitions of the kinetic parameters in the model to achieve the desired outcomes. Initial concentrations typically represent ATIII levels in blood plasma, ranging from 9.08 to 19.52 mg/dL (assuming a molecular weight of 58, 000 g/mol for ATIII [122]), whereas normal blood levels range from 17.0 to 30.0 mg/dL [123]. The models cover scenarios of low ATIII concentration observed in conditions like sepsis or disseminated intravascular coagulation but do not encompass the entire spectrum of typical values (up to 30 mg/dL).
From a patient-specific perspective, a model validated for the entire spectrum of typical ATIII concentrations would be most appropriate. None of the models presented can be considered ideal on their own, as they all rely on additional factors such as the use of heparin and the conditions under which the kinetic constants were obtained (e.g., the presence of Ca2+).
Regarding complexity, the models typically do not exceed summations of nonlinear terms of at most second order. This is advantageous for computational models, as these terms are usually easily resolved by computational software and generic algorithms when included in the source term.
Future studies could enhance the analysis of the range of kinetic values related to ATIII inhibition and the effects of typical patient characteristics, such as genetic differences, heparin resistance, or antithrombin deficiency.
It is crucial to recognize that the choice of the mathematical expression for ATIII significantly impacts the range of diseases the proposed computational model can accurately represent. For instance, a model that includes only the effects of factor IIa (such as [81,109,110]) will be unable to quantitatively capture the impact of deficiencies in factors Ⅸ, Ⅹ, and Ⅺ. Conversely, if the goal is to develop a computational model capable of representing a broader spectrum of coagulation disorders, it is essential to select mathematical formulations that incorporate factors relevant to those conditions (such as [75,78,80,90,107,113]). For example, by including factors Ⅸ, Ⅹ, and Ⅺ in the mathematical expression, the model could quantitatively represent diseases such as hemophilia B, hemophilia C, and factor X deficiency.
Factor Ⅷ and its activated form, factor Ⅷa, are depicted mathematically with a RST, similar to ATIII. The equations extracted from the reviewed studies are listed in Table S5 of the SM file. Table 3 outlines the kinetic parameters used for factor Ⅷ and factor Ⅷa. A complete list of values is available in Tables S3 and S5 in the SM file. Bouchnita et al. [84] presented an essential model where factor Ⅷ is activated by thrombin through first-order kinetics, generating Ⅷa, with subsequent inhibition [Eq (S5.1)]. Seo et al. [82] used the model of coagulation cascade proposed by Jones and Mann [124], depicting factor Ⅷ activation by thrombin and factor Xa via second-order kinetics. This activation is crucial in the intrinsic pathway, leading to the formation of tenase complexes and thrombin generation. The model includes factor Ⅷa consumption by factor Ⅸa, with second-order kinetics [Eq (S5.2)]. Xu et al. [66] employed a similar approach but incorporated mIIa, operating with second-order kinetics to activate factor Ⅷ [Eq (S5.4)]. Ngoepe et al. [83] applied the model of blood clotting proposed by Hockin et al. [125], where factor Ⅷ activation is mediated by thrombin through second-order kinetics. The model also accounts for factor Ⅷa generation through thrombin activation, its consumption in forming the Ⅷa-Ⅸa complex, and its production from dissociating the Ⅷa-Ⅸa complex [Eq (S5.3)]. Additionally, the model distinguishes between the α1 and α2 domains of factor Ⅷa.
Variable | Description | Range |
kcatⅧ/IIa | Catalytic rate for the activation of factor Ⅷ by IIa | 0.9 s-1 [70] –3.24 s-1 [75,78,80,89,90,94,107] |
KmⅧ/IIa | Michaelis–Menten constant for the activation of factor Ⅷ by IIa | 1.47 ×10−7 M [69] –1.12 ×10−4 M [75,78,80,89,90,94,107] |
k++Ⅷ/Xa | Second-order rate constant for the activation of factor Ⅷ by factor Xa | 1.0 ×107 M-1.s-1 [82] –1.0 ×108 M-1.s-1 [95] |
k++Ⅷ/IIa k++Ⅷ,m | Second-order rate constant for the activation of factor Ⅷ by factor IIa | 2.0 ×107 M-1.s-1 [82]–5.0 ×107 M-1.s-1 [88] |
k+Ⅷa1L | First-order rate constant for the formation of Ⅷa1L | 2.2 ×10−5 s-1 [83] –6.0 ×10−3 s-1 [68] |
k++Ⅷa1L/Ⅷa2 | Second-order rate constant for Ⅷa | 2.2 ×104 M-1.s-1 [95]–6.0 ×106 M-1.s-1 [83] |
CⅧ(t=0) | Initial concentration of Ⅷ | 1.0 ×10−10 M [71] –7.0 ×10−10 M [82] |
CⅧa(t=0) | Initial concentration of Ⅷa | 0 M [83]–1.0 ×10−10 M [82] |
Jordan and Chaikof [70] modeled factor Ⅷ activation by thrombin using the Michaelis–Menten mechanism, delineating two kinetic parameters: the catalytic rate and the Michaelis–Menten constant [Eq (S5.5)]. Shibeko et al. [69] adopted a similar approach, including a first-order term for factor Ⅷa inhibition [Eq (S5.6)]. Dydek and Chaikof [85] also utilized the Michaelis–Menten mechanism for factor Ⅷ activation [Eq (S5.7)]. The expression for factor Ⅷa accounts for consumption and generation due to the Ⅷa-Ⅸa complex, as seen in other studies [66,82]. Several studies [75,78,80,89,90,94,107] employed the model for coagulation cascade proposed by Anand et al. [117], akin to the model by Dydek and Chaikof [85], with an additional term for factor Ⅷa consumption by PCA using the Michaelis–Menten mechanism [Eq (S5.8)]. Mendez Rojano et al. [126] adopted the kinetic model proposed by Chatterjee et al. [127], describing factors Ⅷ and Ⅷa dynamics with second-order kinetics, considering thrombin activation, Ⅷ binding to Xa, Ⅷa-Ⅸa complex formation, and domain differentiation [Eq (S5.9)]. Pisaryuk et al. [111] characterized Ⅷ and Ⅷa dynamics based on lipid binding, employing second-order kinetics for binding and first-order kinetics for unbinding [Eq (S5.10)].
Xu et al. [68] introduced a model where factor Ⅷ undergoes activation by thrombin via second-order kinetics and can bind to platelet surfaces through second-order kinetics while unbinding with first-order kinetics. Factor Ⅷa follows a similar pattern, with differentiation between the α1 and α2 domains [Eq (S5.11)]. Leiderman and Fogelson [71] proposed a model where factors Ⅷ and Ⅷa interact with platelet surfaces based on available binding sites [Eq (S5.12)]. This model also includes second-order activation by thrombin and first-order generation of factor Ⅷ from dissociating the Ⅷ-IIa complex. Fogelson et al. [72] and Miyazawa et al. [115] used ODEs instead of PDEs, with convective-diffusive effects represented by a kinetic constant. PCA was incorporated, leading to the consumption of factor Ⅷa through the Ⅷa-PCA complex formation (second-order kinetics) and dissociation (first-order kinetics) [Eq (S5.13)]. Govindarajan et al. [88] proposed a simplified model with second-order activation of factor Ⅷ by thrombin and binding/unbinding of factors Ⅷ and Ⅷa on platelet surfaces [Eq (S5.14)]. Although simplified, considering platelet concentration is appealing from a patient-specific modeling perspective because platelet counts can vary significantly between patients and may indicate certain diseases.
Factor Ⅷ and its activated form, Ⅷa, are crucial components in models of the coagulation cascade, particularly in the intrinsic pathway involving thrombin and factor Xa activation. Various mathematical models have been employed to describe their dynamics, often using second-order kinetics for activation and inhibition processes. These models vary in complexity, ranging from simple Michaelis–Menten mechanisms to more detailed descriptions, including platelet interactions and domain-specific dynamics.
Identifying a single, optimal model for factor Ⅷ dynamics is challenging due to the diversity of approaches used across studies. However, models that integrate both intrinsic pathway activation—via thrombin and factor Xa—and subsequent interactions, such as factor Ⅷa-Ⅸa complex formation, offer comprehensive insights. For instance, models that include the activation of factor Ⅷ by thrombin and factor Xa shed light on the early phases of the coagulation cascade. By incorporating these critical initiators, such models capture the key process of factor Ⅷ activation and the subsequent amplification of clotting signals. This initial phase is especially significant, as thrombin acts as a potent activator of factor Ⅷ, converting it into its active form, factor Ⅷa, which then plays a central role in promoting clot formation. Once activated, factor Ⅷa binds with factor Ⅸa to form the essential factor Ⅷa-Ⅸa complex, which catalyzes the generation of factor Xa. This production of factor Xa sustains the coagulation cascade, establishing a positive feedback loop that ensures robust thrombin generation, ultimately leading to stable clot formation. By modeling these interactions, the framework captures the mechanisms behind factor Ⅷ activation and highlights how the factor Ⅷa-Ⅸa complex supports ongoing thrombin production, reinforcing durable clot formation. Additionally, such models are valuable for simulating factor Ⅸ deficiencies, as seen in hemophilia B. Insufficient factor Ⅸa disrupts the formation and function of the factor Ⅷa-Ⅸa complex, impairing clot formation. This approach provides deeper insights into the effects of factor Ⅸ deficiency on clotting dynamics and can inform targeted treatment strategies for hemophilia B and similar clotting disorders.
One significant limitation is the variation in kinetic parameter values across different models, as shown in Table 3. The wide range of values for catalytic activation rates by thrombin underscores the uncertainty in parameter estimation and the potential impact on model predictions. Simplified assumptions, such as neglecting certain interactions or domain-specific differentiation, also limit the accuracy of some models in capturing real-world complexities.
Table S6 in the SM file presents mathematical equations governing the dynamics of PC and PCA. Like ATIII and factor Ⅷ, these formulations are integrated into the RST. In studies by Bouchnita et al. [81] and Bouchnita et al. [99], the CDR equation models the spatiotemporal distribution of PC and PCA, with both undergoing inactivation via first-order kinetics [Eq (S6.1)]. Dydek and Chaikof [85] employed a Michaelis–Menten mechanism for PC activation, influenced by PC concentrations and thrombomodulin bound with thrombin [Eq (S6.2)]. This representation reflects thrombin's qualitative binding to thrombomodulin, inducing a conformational change and enhancing its ability to cleave PC, producing PCA. PCA acts as an anticoagulant by inhibiting factors Va and Ⅷa, modulating thrombin generation to prevent excessive clotting. In this model, PCA concentration also affects factor Va dynamics. Conversely, Ou et al. [89] did not explicitly consider thrombomodulin bound with thrombin. Instead, a kinetic model with a Michaelis–Menten mechanism accounts for thrombin concentration alone [Eq (S6.3)]. PCA concentration influences factors Va and Ⅷa, serving as inhibitors.
Multiple studies [75,78,80,90,94,107] have utilized the model proposed by Anand et al. [117] to depict PC and PCA dynamics. For PC, thrombin activates it through the Michaelis–Menten mechanism, and it is inhibited by α1-antitrypsin via second-order kinetics [see Eq (S6.4)]. PCA, in turn, inhibits factors Va and Ⅷa. Pavlova et al. [78] expanded on this framework, introducing PCA inhibition by prothrombinase via second-order kinetics [see Eq (S6.5)], although it does not include the dynamics of factors Va and Ⅷa influenced by PCA. Leiderman and Fogelson [71] and Leiderman and Fogelson [73] incorporated PCA≡Va and PCA≡Ⅷa complex formation and dissociation through second and first-order kinetics, respectively [Eq (S6.6)], impacting factors Va and Ⅷa dynamics. Govindarajan et al. [88] modeled APC but omitted PCA≡Ⅷa complex formation [Eq (S6.8)]. In the study conducted by Jordan and Chaikof [70], PC activation solely occurs on the thrombomodulin-thrombin surface via the Michaelis–Menten mechanism, akin to Dydek and Chaikof [85] representation.
Miyazawa et al. [115] employed an ODE to describe PCA, substituting PDE terms with a rate constant for convective and diffusive effects. Since activation occurs in both phases, the convective and diffusive effects influence the spatiotemporal distribution of PCA and its role in the coagulation cascade. The model includes PCA complex formation with factors Ⅷa, Va, and partially activated factor Ⅴ in both the fluid phase and platelet surface. Complex formation operates via second-order kinetics, while dissociation occurs via first-order kinetics [Eq (S6.9)]. Fogelson et al. [72] adopted a similar approach, considering associations/dissociations of factors Va and Ⅷa in both phases Eq (S6.10). Xu et al. [68] presented a similar expression, focusing on platelet surface associations/dissociations [Eq (S6.11)]. Shibeko et al. [69] depicted PC with two terms using the Michaelis–Menten mechanism: one influenced by free thrombin and another by thrombomodulin-thrombin activation. PCA's equation includes these activations and consumption by α2M (α2-macroglobulin), α2AP (α2-antiplasmin), α1AT (α1-antitrypsin), and PCI (protein C inhibitor) (Eq (S6.12)). These components were assumed to have constant concentrations.
Table S7 in the SM file outlines fibrin(ogen) mathematical expressions. In Bouchnita et al. studies [81,84], fibrinogen and fibrin were part of the RST. Fibrinogen depletion occurs through thrombin-induced conversion to fibrin. Fibrin expression includes activation by thrombin and negative conversion to a fibrin polymer. The fibrin polymer is assumed to be immobile in the clot [Eq. (S7.1)]. While this assumption is common and aids in modeling clot formation, it is essential to recognize that in biological reality, fibrin may undergo some degree of dynamic reorganization or degradation, especially under certain physiological or pathological conditions, such as hyperfibrinolysis or hypofibrinolysis. Pisaryuk et al. [111] integrated fibrinogen and fibrin similarly with thrombin activation and ATIII inhibition [Eq (S7.2)]. In Bouchnita et al. [99,104], fibrinogen activation by thrombin followed the Michaelis–Menten mechanism. Fibrin formation included a first-order term for degradation during polymerization [Eq (S7.3)]. Shibeko et al. [69] employed a simpler model with thrombin-driven fibrinogen/fibrin production [Eq (S7.4)], with only a Michaelis–Menten mechanism activation by thrombin. Several studies [75,78,80,86,89,90,94,96,107] adopted the model proposed by Anand et al. [117] for fibrin(ogen) dynamics. Fibrinogen decreases via Michaelis–Menten kinetics regulated by thrombin and fibrinogen concentrations. A similar term positively influences fibrin, while plasmin cleavage negatively impacts fibrin [Eq (S7.5)]. In the model utilized by Govindarajan et al. [88], a departure from previous approaches is observed as fibrinogen activation is driven by the concentration of thrombin present on the platelet surface, operating via second-order kinetics. Furthermore, in the expression for fibrin, the convective term is omitted, with only the diffusive term being accounted for in the PDE [Eq (S7.6)].
Seo et al. [82] adopted a model similar to that proposed by Shibeko et al. [69]. However, they introduced an additional positive term to augment the fibrin concentration. The term is a function of the square of fibrin concentration and a rate constant [Eq (S7.7)]. Tosenberger et al. [74] employed a model where blood and platelets were represented with discrete particle dynamics, while the chemical species involved in the coagulation cascade, including fibrin, were depicted as PDE. Fibrin conversion was characterized in a manner where the RST diminishes as the fibrin concentration nears saturation [Eq (S7.8)]. However, a limitation of this equation is its failure to consider the thrombin concentration. This limitation was addressed in subsequent studies [76,87], where fibrinogen activation was modeled as a function of thrombin concentration through second-order kinetics. A term for fibrinogen saturation was also included, proportional to the disparity between saturated fibrinogen and fibrinogen concentration [Eq (S7.9)]. In the study conducted by Mendez Rojano et al. [109], four classes were delineated: fibrinogen, fibrin, deposited fibrinogen, and deposited fibrin [see Eq (S7.10)]. Similar to other investigations, fibrinogen activation ensues through a thrombin-mediated Michaelis–Menten mechanism. However, in the expressions for fibrinogen and fibrin, positive terms pertaining to platelet embolization are introduced, which are proportional to the concentrations of deposited fibrinogen and fibrin. Conversely, the consumption/generation of deposited fibrinogen and fibrin is augmented based on the concentrations of fibrinogen and fibrin, with a portion converted into fibrinogen and fibrin via embolization. Chen and Diamond [98] introduced a simplified model where fibrin evolution is contingent upon thrombin concentration, featuring a term that can be calibrated to fit experimental data [Eq (S7.11)].
In the study conducted by Rukhlenko et al. [77], the spatiotemporal evolution of fibrinogen is determined by its concentration and the activator of the biochemical network of blood coagulation, employing second-order kinetics. Additionally, the model incorporates a fibrinogen removal term proportional to the difference between the current and initial fibrinogen concentration. Furthermore, the activation of fibrin is characterized in terms of the first and second fibrin moments [Eq (S7.12)]. This modeling approach aimed to represent both polymerized and non-polymerized forms of fibrin, capturing essential phenomenological aspects of fibrin dynamics.
Boryczko et al. [64] introduced a model for fibrin polymerization, where fibrin monomers are incorporated into fluid particles representing plasma. Assumptions included the presence of activated fibrinogen, clotting factors, and fluid particles' ability to form fibrin gel fragments with a specified probability. Bonds between particles are governed by elastic and repulsive forces, with attachment and detachment restrictions. Interaction between fibrin chains and particles forming red blood cells varies based on distance, alongside considering the probability of bond breaking [Eq (S7.13)]. Xu et al. [66] treated fibrin concentration as an ODE, with fibrin growth directly proportional to thrombin concentration [Eq (S7.14)]. Piebalgs and Xu [79] developed a multi-physics continuum model for clot dissolution, influencing clot properties over time [Eq (S7.15)]. Pivkin et al. [65] used a coupling force model to simulate platelet behavior, including fibrin interaction [Eq (S7.16)]. Haynes et al. [92] described thrombin-fibrin binding, considering high- and low-affinity sites [Eq (S7.17)]. Kamada et al. [93] simulated clot formation in narrowed vessels using a mechanical spring model to mimic ligand behavior between glycoprotein IIb/IIIa (αIIbβ3) and fibrinogen [Eq (S7.18)]. Du et al. [102] proposed an alternative model for αIIbβ3–fibrin interaction, emphasizing platelet receptors' role in bond formation [Eq (S7.19)]. Petkantchin et al. [114] introduced a permeability coefficient for fibrin clots based on fiber properties [Eq (S7.20)].
The investigation into fibrin(ogen) dynamics through various mathematical models has revealed more complex and multifaceted interactions compared to the models for ATIII, factor Ⅷ, and PC. The modeling strategies employed encompass a spectrum of factors, including the activation and depletion of fibrinogen, the formation and degradation of fibrin, and the influence of thrombin and other clotting factors. Some models incorporate detailed kinetics, such as second-order and Michaelis–Menten mechanisms, to capture the intricate dynamics of fibrinogen activation and fibrin formation. Others adopt simplified representations to streamline computational complexity while maintaining essential physiological insights. Critical aspects considered in these models include the interplay between thrombin and fibrinogen concentrations, the role of platelet receptors in bond formation, and the influence of mechanical forces on fibrin polymerization. Additionally, factors like thrombin concentration gradients, fibrinogen saturation, and platelet embolization effects are considered to enhance the models' realism.
Despite their differences, these models collectively contribute to our understanding of fibrin (ogen) kinetics and its implications for blood clot formation and dissolution. They offer valuable insights into the underlying mechanisms governing hemostasis and thrombosis, aiding in the development of therapeutic interventions and predictive tools for clinical applications.
Table S8 in the SM file presents the mathematical expressions related to vWF. Kamada et al. [93] employed a spring model to simulate the interaction between GPIB/Ⅸ/Ⅴ receptors and vWF, where virtual springs increase in response to shear rate. Similarly, the same expression was utilized to determine the number of virtual springs for the interaction between GPIIB/Ⅲ and vWF. The adhesion force between an adhered platelet and the injured wall is also modeled based on a spring constant and the distance between the particles [Eq (S8.1)]. Ayabe et al. [97] employed a spring model, specifically the Kelvin–Voigt model. Aligned with the model employed by Kamada et al. [93], the binding between GPIbα and vWF is influenced by shear stress and is determined based on a spring constant.
Additionally, it is assumed that the interaction between GPIIa/IIIa and vWF occurs only when the platelet is in an activated state, resulting in a gradual increase in the spring constant value [Eq (S8.2)]. Du et al. [102] modeled the formation of bridges between two platelets via GPIbα using Eq. S8.3, which incorporates second-order rate kinetics based on platelets in four states: mobile activated and unactivated and bound activated and unactivated.
Wu et al. [103] considered that shear stress accumulation, a crucial factor in modeling platelet activation, is enhanced as a function of vWF. They represented this relationship with a sigmoid characteristic [see Eq (S8.4)]. Kaneva et al. [106] employed both deterministic and stochastic equations [Eqs (S8.5a) and (S8.5b)] to model GPIb-mediated platelet interaction with vWF. Additionally, they introduced an equation to quantify the probability of bond formation based on the level of platelet activation [Eq (S8.5c)]. Liu et al. [105] introduced a model where the on-rate of GP1b-A1 binding to tethered vWF depends on internal tension force, energy barrier, and the maximum on-rate under high vWF tension [Eq (S8.6)]. Ma et al. [108] developed a model to represent the adhesion and aggregation forces mediated by vWF as a function of shear rate [Eq (S8.7)]. Zhussupbekov et al. [112] employed two equations to model vWF in collapsed and stretched conformations. These equations act as an RST and follow first-order kinetics [Eq (S8.8)].
A critical observation among the cited studies is that while the models incorporate qualitative aspects of vWF influence, there is a notable absence of quantitative modeling based on the concentration of vWF in the bloodstream.
None of the reviewed studies directly integrated a D-dimer model into their computational blood coagulation models. However, Chen and Diamon [98] utilized D-dimer measurements to empirically determine the time-varying fibrin concentration, calibrated by end-point D-dimer ELISA. They examined fibrin dynamics by incorporating fluorescent fibrinogen and then calibrated the model using the end-point D-dimer assay following plasmin degradation.
Kadri et al. [101] conducted an in vivo assessment of blood clot mechanics using computational fluid dynamics and intravital microscopy images. They utilized these images to quantify the area of the blood clot nucleus using the p-selectin exposure marking to identify the activated core. Such data are crucial for enhancing our understanding of in vivo blood coagulation, given the inherent challenges in obtaining spatiotemporal data in vivo compared to in vitro experiments.
Pisaryuk et al. [111] proposed a personalized hemostasis profile by adjusting blood factors based on PT and APTT. The adjustment involves modifying the concentrations of fibrinogen, as well as blood factors Ⅷ, Ⅸ, and Ⅺ, according to APTT, based on the following equation:
Fi=F0mean(APTTi)APTTi | (4) |
where Fi is the factor (fibrinogen, factor Ⅷ, Ⅸ, or Ⅺ) of patient i, F0 is the normal average concentration of factor F, and APTTi is the APTT of patient i.
The adjustment of the blood factors Ⅱ, Ⅴ, Ⅶ, and Ⅹ was based on PT, according to the following equation:
Fi=F0mean(PTi)PTi | (5) |
where Fi is the factor (factor Ⅱ, Ⅴ, Ⅶ, or Ⅹ) of patient i, F0 is the normal average concentration of factor F, and APTTi is the APTT of patient i.
F0 values can be readily acquired from existing literature sources. APTT and PT measurements are inexpensive and readily available, often requested by healthcare providers when assessing potential disruptions in the hemostatic system. By gathering patient data and determining the average APTT and PT, personalized concentration values for the mentioned factors can be established for each patient.
The adjustment proposed by Pisaryuk et al. [111] effectively mimics the results of global hemostasis assays, such as thrombin generation and thrombodynamics-4D, for each patient with considerable accuracy. The proposed in silico model offers significant advantages, including reduced treatment costs and the facilitation of therapy adjustments.
One key reason for the improvement in model accuracy when altering initial concentrations is that these initial concentrations and the associated kinetic parameters primarily influence the mathematical modeling of coagulation dynamics. Since the kinetic parameters remain constant, varying the initial concentrations becomes essential for accurate modeling.
Estimating these initial parameters through simple laboratory tests, such as APTT and PT, is particularly advantageous, as it enables the development of a tailored coagulation profile for each patient.
An important aspect of computational models recently gaining attention in the literature is their capacity to represent diseases associated with blood coagulation disorders and their impact on hemostasis. These models frequently identify thrombin as a pivotal factor that amplifies the coagulation cascade and influences platelet activation and aggregation. Such interactions significantly affect the convective and diffusive dynamics within the system. Consequently, any alterations in the coagulation cascade that impact thrombin production can markedly influence the results generated by these computational models. Furthermore, genetic mutations and deficiencies—such as prothrombin mutations, protein C deficiency, and factor Ⅸ deficiency—directly alter various components of the coagulation cascade. For instance, Ranc et al. [128] illustrate how modifications to established numerical methods can effectively simulate the behavior of plasmas deficient in factors Ⅻ, Ⅺ, and Ⅷ, underscoring the critical importance of accurately calibrating initial concentrations and model parameters for effective representation. Despite these advancements, it is noteworthy that the studies reviewed do not explicitly examine the effects of mutations, like those seen in hemophilia, on the spatiotemporal formation of blood clots. This gap presents a compelling opportunity for future research to incorporate disease conditions into computational models, thereby enhancing our understanding of their influence on hemostatic processes. Addressing this void could pave the way for the development of more comprehensive models that accurately reflect the complexities of coagulation disorders and their clinical implications.
Figure 2 summarizes the main factors associated with each biomarker and diseases linked to abnormalities in these factors. These biomarkers are primarily associated with components of the coagulation cascade. Mutations, hemophilia, deficiencies, and treatments affect their concentrations and kinetics, which are crucial for the mathematical model.
An intriguing insight gleaned from this study, particularly from the perspective of personalized medicine, is the underutilization of variables commonly requested to assess hemostatic imbalances, such as D-dimer, APTT, and PT, in computational investigations of blood clot formation under flow conditions. Future research in this domain could greatly benefit from efforts to integrate and enhance the representation of these variables within computational models, whether through direct incorporation or via phenomenological modeling and data-driven approaches. Such endeavors hold the potential to enrich our understanding of clot formation dynamics and contribute to more comprehensive and accurate predictive models in hemostasis research.
In several studies examined in this review, the mathematical modeling of key components such as ATIII, FⅧ, PC, and fibrin(ogen) was accomplished by implementing previously validated models of the coagulation cascade, particularly those proposed by Hockin et al. [129], Anand et al. [117], Jones and Mann [124], and Chatterjee et al. [127]. It is crucial to note that the kinetic parameters utilized in these studies are often derived from experiments conducted under specific experimental conditions. For instance, some parameters were evaluated under non-physiological conditions [129], certain assumptions were made, such as all ATIII being active [117], and some rate constants were deduced, estimated [124], or obtained in solution [127]. Moreover, upon examining the original literature (as cited throughout the SM file), it is noticeable that patient-specific characteristics of the blood analyzed are often not provided. While it has been commonly assumed that data is derived from healthy patients, this lack of patient-specific information presents a challenge when applying these models to patients with hemostatic disorders such as thrombosis predisposition, genetic mutations, or hemophilia. Consequently, the accuracy of patient-specific computational models derived from these generalized parameters may be compromised. Future studies could delve into comparing the kinetic parameters of the coagulation cascade in vivo and under flow conditions between healthy individuals and patients with hemostatic disturbances. Such research endeavors can offer valuable insights into refining and tailoring computational models to accurately represent the dynamics of clot formation in specific patient populations.
An important aspect to consider is the range of kinetic parameters observed across the studies analyzed. These parameters can vary significantly, sometimes by up to 1000 times, among different studies. Such variations can substantially impact critical applications like sensitivity analysis [120,126,130,131] for identifying potential drug targets. Additionally, when modeling individualized or patient-specific conditions (e.g., hemophilia, thrombophilia), variability in kinetic parameters can significantly affect the accuracy of treatment response predictions. For instance, a model using a high inhibition rate for ATIII might predict a lower clotting potential than a model with a lower rate, thereby influencing recommendations for anticoagulant therapy. High variability in kinetic parameters also complicates the validation and reproducibility of coagulation models. Different studies may produce divergent outcomes even when modeling the same clinical condition, making establishing standardized models for general use or regulatory approval challenging. This observation underscores the importance of carefully selecting or calibrating kinetic parameters in coagulation models, particularly when aiming to translate these models into clinical practice or individualized treatment plans.
Furthermore, there is a notable absence of focused analyses on these biomarkers and their individual impacts. Investigating the effects of these biomarkers and kinetic parameters within phenomenological models can be challenging due to the scarcity of in vivo experimental data and the complex, multivariable nature of the phenomenon. Therefore, a valuable direction for future research would be to systematically assess the influence of these markers on clot formation independently. This could involve varying model configurations and kinetic parameters to simulate diverse physiological conditions and diseases.
In conclusion, the studies reviewed underscore the crucial role of computational models in advancing our understanding of blood coagulation dynamics. Mathematical expressions for key factors like ATIII, factor Ⅷ, and PC are typically incorporated into the reactive source term, employing first-order, second-order, and Michaelis–Menten kinetics. Fibrin(ogen) dynamics are also modeled this way, with some studies employing multiscale approaches and alternative models, such as coupled force and spring models. Variables related to hemostatic balance disorders, such as D-dimer, PT, and APTT, are rarely utilized efficiently in computational models of blood clot formation under flow conditions. This study highlights the underutilization of these key variables in computational models, especially concerning personalized medicine. Integrating these variables into computational models could significantly enhance our understanding of clot formation dynamics and lead to more accurate predictive and practical models in hemostasis research.
Our study shows that the kinetic parameters used in models widely used for blood coagulation are often derived from experiments conducted under specific conditions, leading to potential inaccuracies. Additionally, patient-specific characteristics are often lacking, posing challenges when applying these models to individuals with hemostatic disorders. Future research should focus on comparing kinetic parameters between healthy individuals and patients with hemostatic disturbances to improve the accuracy of computational models tailored to specific patient populations.
Future research should also prioritize comparing kinetic parameters between healthy individuals and patients with hemostatic disorders, while efforts to standardize parameter estimation methodologies could improve model accuracy and reliability. Additionally, given the wide range of kinetic parameter variations observed across studies (upon 1000 times), sensitivity analysis becomes crucial for identifying potential drug targets and optimizing therapeutic interventions in the context of hemostasis and thrombosis.
The authors declare they have not used Artificial Intelligence (AI) tools in the creation of this article.
We want to express our gratitude to the Conselho Nacional de Desenvolvimento Científico e Tecnológico (Brazilian National Council for Scientific and Technological Development, CNPq), grant #164134/2022-0, and the São Paulo Research Foundation (FAPESP), grant #2016/14172-6 and #08/67860-3, for their financial support. Additionally, we acknowledge the support of CNPq and CAPES for their assistance in the postgraduate program at the institution where this study was conducted.
The authors declare there is no conflict of interest.
[1] |
S. Z. Goldhaber, H. Bounameaux, Pulmonary embolism and deep vein thrombosis, Lancet (London, England), 379 (2012), 1835–1846. https://doi.org/10.1016/S0140-6736(11)61904-1 doi: 10.1016/S0140-6736(11)61904-1
![]() |
[2] |
G. E. Raskob, P. Angchaisuksiri, A. N. Blanco, H. Buller, A. Gallus, B. J. Hunt, et al., Thrombosis: a major contributor to global disease burden, Arterioscler. Thromb. Vasc. Biol., 34 (2014), 2363–2371. https://doi.org/10.1161/ATVBAHA.114.304488 doi: 10.1161/ATVBAHA.114.304488
![]() |
[3] |
D. Voci, U. Fedeli, I. T. Farmakis, L. Hobohm, K. Keller, L. Valerio, et al., Deaths related to pulmonary embolism and cardiovascular events before and during the 2020 COVID-19 pandemic: An epidemiological analysis of data from an Italian high-risk area, Thromb. Res., 212 (2022), 44–50. https://doi.org/10.1016/j.thromres.2022.02.008 doi: 10.1016/j.thromres.2022.02.008
![]() |
[4] |
I. Katsoularis, O. Fonseca-Rodríguez, P. Farrington, H. Jerndal, E. H. Lundevaller, M. Sund, et al., Risks of deep vein thrombosis, pulmonary embolism, and bleeding after covid-19: nationwide self-controlled cases series and matched cohort study, BMJ, 377 (2022). https://doi.org/10.1136/bmj-2021-069590 doi: 10.1136/bmj-2021-069590
![]() |
[5] |
T. N. Nguyen, M. M. Qureshi, P. Klein, H. Yamagami, M. Abdalkader, R. Mikulik, et al., Global impact of the COVID-19 pandemic on cerebral venous thrombosis and mortality, J. Stroke, 24 (2022), 256–265. https://doi.org/10.5853/jos.2022.00752 doi: 10.5853/jos.2022.00752
![]() |
[6] |
E. Berntorp, K. Fischer, D. P. Hart, M. E. Mancuso, D. Stephensen, A. D. Shapiro, et al., Haemophilia, Nat. Rev. Dis. Prim., 7 (2021), 45. https://doi.org/10.1038/s41572-021-00278-x doi: 10.1038/s41572-021-00278-x
![]() |
[7] |
K. G. Link, M. T. Stobb, M. G. Sorrells, M. Bortot, K. Ruegg, M. J. Manco‐Johnson, et al., A mathematical model of coagulation under flow identifies factor V as a modifier of thrombin generation in hemophilia A, J. Thromb. Haemost., 18 (2020), 306–317. https://doi.org/10.1111/jth.14653 doi: 10.1111/jth.14653
![]() |
[8] |
F. W. G. Leebeek, W. Miesbach, Gene therapy for hemophilia: a review on clinical benefit, limitations, and remaining issues, Blood, 138 (2021), 923–931. https://doi.org/10.1182/blood.2019003777 doi: 10.1182/blood.2019003777
![]() |
[9] |
S. S. G. Halfmann, N. Evangelatos, P. Schröder-Bäck, A. Brand, European healthcare systems readiness to shift from 'One-Size Fits All' to personalized medicine, Per. Med., 14 (2017), 63–74. https://doi.org/10.2217/pme-2016-0061 doi: 10.2217/pme-2016-0061
![]() |
[10] |
T. Behl, I. Kaur, A. Sehgal, S. Singh, A. Albarrati, M. Albratty, et al., The road to precision medicine: Eliminating the "One Size Fits All" approach in Alzheimer's disease, Biomed. Pharmacother., 153 (2022), 113337. https://doi.org/10.1016/j.biopha.2022.113337 doi: 10.1016/j.biopha.2022.113337
![]() |
[11] |
N. M. Hamdy, E. B. Basalious, M. G. El-Sisi, M. Nasr, A. M. Kabel, E. S. Nossier, et al., Advancements in current one-size-fits-all therapies compared to future treatment innovations for better improved chemotherapeutic outcomes: a step-toward personalized medicine, Curr. Med. Res. Opin., 40 (2024), 1–19. https://doi.org/10.1080/03007995.2024.2416985 doi: 10.1080/03007995.2024.2416985
![]() |
[12] |
G. Di Minno, E. Tremoli, Tailoring of medical treatment: hemostasis and thrombosis towards precision medicine, Haematologica, 102 (2017), 411–418. https://doi.org/10.3324/haematol.2016.156000 doi: 10.3324/haematol.2016.156000
![]() |
[13] | D. L. Ornstein, Chapter 41 - Personalized medicine for disorders of hemostasis and thrombosis, in Diagnostic Molecular Pathology (eds. W. B. Coleman and G. J. Tsongalis), Academic Press, (2024), 643–653. https://doi.org/10.1016/B978-0-12-822824-1.00006-7 |
[14] |
S. Nagalla, P. F. Bray, Personalized medicine in thrombosis: back to the future, Blood, 127 (2016), 2665–2671. https://doi.org/10.1182/blood-2015-11-634832 doi: 10.1182/blood-2015-11-634832
![]() |
[15] |
R. J. S. Preston, J. M. O'Sullivan, Personalized approaches to the treatment of hemostatic disorders, Semin. Thromb. Hemostasis, 47 (2021), 117–119. https://doi.org/10.1055/s-0041-1723800 doi: 10.1055/s-0041-1723800
![]() |
[16] |
H. Al‐Samkari, W. Eng, A precision medicine approach to hereditary hemorrhagic telangiectasia and complex vascular anomalies, J. Thromb. Haemost., 20 (2022), 1077–1088. https://doi.org/10.1111/jth.15715 doi: 10.1111/jth.15715
![]() |
[17] |
X. Delavenne, E. Ollier, A. Lienhart, Y. Dargaud, A new paradigm for personalized prophylaxis for patients with severe haemophilia A, Haemophilia, 26 (2020), 228–235. https://doi.org/10.1111/hae.13935 doi: 10.1111/hae.13935
![]() |
[18] |
L. H. Bukkems, L. L. F. G. Valke, W. Barteling, B. A. P. Laros-van Gorkom, N. M. A. Blijlevens, M. H. Cnossen, et al., Combining factor Ⅷ levels and thrombin/plasmin generation: A population pharmacokinetic-pharmacodynamic model for patients with haemophilia A, Br. J. Clin. Pharmacol., 88 (2022), 2757–2768. https://doi.org/10.1111/bcp.15185 doi: 10.1111/bcp.15185
![]() |
[19] |
N. Mackman, W. Bergmeier, G. A. Stouffer, J. I. Weitz, Therapeutic strategies for thrombosis: new targets and approaches, Nat. Rev. Drug. Discov., 19 (2020), 333–352. https://doi.org/10.1038/s41573-020-0061-0 doi: 10.1038/s41573-020-0061-0
![]() |
[20] |
P. S. Wells, R. Ihaddadene, A. Reilly, M. A. Forgie, Diagnosis of venous thromboembolism: 20 years of progress, Ann. Intern. Med., 168 (2018), 131–140. https://doi.org/10.7326/M17-0291 doi: 10.7326/M17-0291
![]() |
[21] |
F. Khan, T. Tritschler, S. R. Kahn, M. A. Rodger, Venous thromboembolism, Lancet (London, England), 398 (2021), 64–77. https://doi.org/10.1016/S0140-6736(20)32658-1 doi: 10.1016/S0140-6736(20)32658-1
![]() |
[22] |
T. D. Martins, S. D. Martins, S. Montalvão, M. Al Bannoud, G. Y. Ottaiano, L. Q. Silva, et al., Combining artificial neural networks and hematological data to diagnose Covid-19 infection in Brazilian population, Neural Comput. Appl., 36 (2024), 4387–4399. https://doi.org/10.1007/s00521-023-09312-3 doi: 10.1007/s00521-023-09312-3
![]() |
[23] |
T. D. Martins, R. Maciel-Filho, S. A. L. Montalvão, G. S. S. Gois, M. Al Bannoud, G. Y. Ottaiano, et al., Predicting mortality of cancer patients using artificial intelligence, patient data and blood tests, Neural Comput. Appl., 36 (2024), 15599–15616. https://doi.org/10.1007/s00521-024-09915-4 doi: 10.1007/s00521-024-09915-4
![]() |
[24] |
F. W. G. Leebeek, New developments in diagnosis and management of acquired hemophilia and acquired von willebrand syndrome, HemaSphere, 5 (2021). https://doi.org/10.1097/HS9.0000000000000586 doi: 10.1097/HS9.0000000000000586
![]() |
[25] |
F. Peyvandi, G. Kenet, I. Pekrul, R. K. Pruthi, P. Ramge, M. Spannagl, Laboratory testing in hemophilia: Impact of factor and non‐factor replacement therapy on coagulation assays, J. Thromb. Haemost., 18 (2020), 1242–1255. https://doi.org/10.1111/jth.14784 doi: 10.1111/jth.14784
![]() |
[26] |
B. Pezeshkpoor, J. Oldenburg, A. Pavlova, Insights into the molecular genetic of hemophilia A and hemophilia B: The relevance of genetic testing in routine clinical practice, Hamostaseologie, 42 (2022), 390–399. https://doi.org/10.1055/a-1945-9429 doi: 10.1055/a-1945-9429
![]() |
[27] |
A. H. Kristoffersen, E. Ajzner, D. Rogic, E. Y. Sozmen, P. Carraro, A. P. Faria, et al., Is D-dimer used according to clinical algorithms in the diagnostic work-up of patients with suspicion of venous thromboembolism? A study in six European countries, Thromb. Res., 142 (2016), 1–7. https://doi.org/10.1016/j.thromres.2016.04.001 doi: 10.1016/j.thromres.2016.04.001
![]() |
[28] |
M. Kafeza, J. Shalhoub, N. Salooja, L. Bingham, K. Spagou, A. H. Davies, A systematic review of clinical prediction scores for deep vein thrombosis, Phlebology, 32 (2017), 516–531. https://doi.org/10.1177/0268355516678729 doi: 10.1177/0268355516678729
![]() |
[29] |
M. T. Greene, A. C. Spyropoulos, V. Chopra, P. J. Grant, S. Kaatz, S. J. Bernstein, et al., Validation of risk assessment models of venous thromboembolism in hospitalized medical patients, Am. J. Med., 129 (2016), 1001.e9–1001.e18. https://doi.org/10.1016/j.amjmed.2016.03.031 doi: 10.1016/j.amjmed.2016.03.031
![]() |
[30] |
P. C. Silveira, I. K. Ip, S. Z. Goldhaber, G. Piazza, C. B. Benson, R. Khorasani, Performance of wells score for deep vein thrombosis in the inpatient setting, JAMA Intern. Med., 175 (2015), 1112–1117. https://doi.org/10.1001/jamainternmed.2015.1687 doi: 10.1001/jamainternmed.2015.1687
![]() |
[31] |
M. Kafeza, J. Shalhoub, N. Salooja, L. Bingham, K. Spagou, A. H. Davies, A systematic review of clinical prediction scores for deep vein thrombosis, Phlebology, 32 (2016), 516–531. https://doi.org/10.1177/0268355516678729 doi: 10.1177/0268355516678729
![]() |
[32] |
I. Nichele, A. Tosetto, Scoring Systems for Estimating the Risk of Recurrent Venous Thromboembolism, Semin. Thromb. Hemost., 43 (2017), 493–499. https://doi.org/10.1055/s-0037-1602662 doi: 10.1055/s-0037-1602662
![]() |
[33] |
A. Muñoz, C. Ay, E. Grilz, S. López, C. Font, V. Pachón, et al., A clinical-genetic risk score for predicting cancer-associated venous thromboembolism: A development and validation study involving two independent prospective cohorts, J. Clin. Oncol., 41 (2023), 2911–2925. https://doi.org/10.1200/JCO.22.00255 doi: 10.1200/JCO.22.00255
![]() |
[34] |
F. Rodeghiero, A. Tosetto, T. Abshire, D. M. Arnold, B. Coller, P. James, et al., ISTH/SSC bleeding assessment tool: a standardized questionnaire and a proposal for a new bleeding score for inherited bleeding disorders, J. Thromb. Haemost., 8 (2010), 2063–2065. https://doi.org/10.1111/j.1538-7836.2010.03975.x doi: 10.1111/j.1538-7836.2010.03975.x
![]() |
[35] |
M. Borhany, N. Fatima, M. Abid, T. Shamsi, M. Othman, Application of the ISTH bleeding score in hemophilia, Transfus. Apher. Sci., 57 (2018), 556–560. https://doi.org/10.1016/j.transci.2018.06.003 doi: 10.1016/j.transci.2018.06.003
![]() |
[36] |
M. Khalifa, M. Albadawy, Artificial intelligence for clinical prediction: Exploring key domains and essential functions, Comput. Methods Programs Biomed. Updat., 5 (2024), 100148. https://doi.org/10.1016/j.cmpbup.2024.100148 doi: 10.1016/j.cmpbup.2024.100148
![]() |
[37] |
T. H. Tan, C. C. Hsu, C. J. Chen, S. L. Hsu, T. L. Liu, H. J. Lin, et al., Predicting outcomes in older ED patients with influenza in real time using a big data-driven and machine learning approach to the hospital information system, BMC Geriatr., 21 (2021), 280. https://doi.org/10.1186/s12877-021-02229-3 doi: 10.1186/s12877-021-02229-3
![]() |
[38] |
C. Guan, F. Ma, S. Chang, J. Zhang, Interpretable machine learning models for predicting venous thromboembolism in the intensive care unit: an analysis based on data from 207 centers, Crit. Care, 27 (2023), 406. https://doi.org/10.1186/s13054-023-04683-4 doi: 10.1186/s13054-023-04683-4
![]() |
[39] |
T. D. Martins, J. M. Annichino-Bizzacchi, A. V. C. Romano, R. Maciel Filho, Artificial neural networks for prediction of recurrent venous thromboembolism, Int. J. Med. Inform., 141 (2020), 104221. https://doi.org/10.1016/j.ijmedinf.2020.104221 doi: 10.1016/j.ijmedinf.2020.104221
![]() |
[40] |
I. Pabinger, C. Ay, Biomarkers and venous thromboembolism, Arter. Thromb. Vasc. Biol., 29 (2009), 332–336. https://doi.org/10.1161/ATVBAHA.108.182188 doi: 10.1161/ATVBAHA.108.182188
![]() |
[41] |
I. Pabinger, J. Thaler, C. Ay, Biomarkers for prediction of venous thromboembolism in cancer, Blood, 122 (2013), 2011–2018. https://doi.org/10.1182/blood-2013-04-460147 doi: 10.1182/blood-2013-04-460147
![]() |
[42] |
F. Galeano-Valle, L. Ordieres-Ortega, C. M. Oblitas, J. del-Toro-Cervera, L. Alvarez-Sala-Walther, P. Demelo-Rodríguez, Inflammatory biomarkers in the short-term prognosis of venous thromboembolism: A narrative review, Int. J. Mol. Sci., 22 (2021), 2627. https://doi.org/10.3390/ijms22052627 doi: 10.3390/ijms22052627
![]() |
[43] |
B. Jacobs, A. Obi, T. Wakefield, Diagnostic biomarkers in venous thromboembolic disease, J. Vasc. Surg. Venous Lymphat. Disord., 4 (2016), 508–517. https://doi.org/10.1016/j.jvsv.2016.02.005 doi: 10.1016/j.jvsv.2016.02.005
![]() |
[44] |
M. A. Bannoud, B. P. Gomes, M. C. de S. P. Abdalla, M. V Freire, K. Andreola, T. D. Martins, et al., Mathematical modeling of drying kinetics of ground Açaí (Euterpe oleracea) kernel using artificial neural networks, Chem. Pap., 78 (2024), 1033–1054. https://doi.org/10.1007/s11696-023-03142-2 doi: 10.1007/s11696-023-03142-2
![]() |
[45] |
M. A. Bannoud, T. D. Martins, B. F. dos Santos, Control of a closed dry grinding circuit with ball mills using predictive control based on neural networks, Digit. Chem. Eng., 5 (2022), 100064. https://doi.org/10.1016/j.dche.2022.100064 doi: 10.1016/j.dche.2022.100064
![]() |
[46] |
J. Berg, K. Nyström, Data-driven discovery of PDEs in complex datasets, J. Comput. Phys., 384 (2019), 239–252. https://doi.org/10.1016/j.jcp.2019.01.036 doi: 10.1016/j.jcp.2019.01.036
![]() |
[47] |
L. Burzawa, L. Li, X. Wang, A. Buganza-Tepole, D. M. Umulis, Acceleration of PDE-based biological simulation through the development of neural network metamodels, Curr. Pathobiol. Rep., 8 (2020), 121–131. https://doi.org/10.1007/s40139-020-00216-8 doi: 10.1007/s40139-020-00216-8
![]() |
[48] |
M. A. Bannoud, C. A. M. da Silva, T. D. Martins, Applications of metaheuristic optimization algorithms in model predictive control for chemical engineering processes: A systematic review, Annu. Rev. Control., 58 (2024), 100973. https://doi.org/10.1016/j.arcontrol.2024.100973 doi: 10.1016/j.arcontrol.2024.100973
![]() |
[49] |
M. A. Bannoud, P. H. N. Ferreira, R. R. de Andrade, C. A. M. da Silva, Control of an integrated first and second-generation continuous alcoholic fermentation process with cell recycling using model predictive control, Chem. Eng. Commun., (2024), 1–24. https://doi.org/10.1080/00986445.2024.2417901 doi: 10.1080/00986445.2024.2417901
![]() |
[50] |
Y. Zhao, C. Li, X. Liu, R. Qian, R. Song, X. Chen, Patient-specific seizure prediction via adder network and supervised contrastive learning, IEEE Trans. Neural Syst. Rehabil. Eng., 30 (2022), 1536–1547. https://doi.org/10.1109/TNSRE.2022.3180155 doi: 10.1109/TNSRE.2022.3180155
![]() |
[51] |
K. Leiderman, S. S. Sindi, D. M. Monroe, A. L. Fogelson, K. B. Neeves, The art and science of building a computational model to understand hemostasis, Semin. Thromb. Hemost., 47 (2021), 129–138. https://doi.org/10.1055/s-0041-1722861 doi: 10.1055/s-0041-1722861
![]() |
[52] |
N. Ratto, A. Bouchnita, P. Chelle, M. Marion, M. Panteleev, D. Nechipurenko, et al., Patient-specific modelling of blood coagulation, Bull. Math. Biol., 83 (2021), 50. https://doi.org/10.1007/s11538-021-00890-8 doi: 10.1007/s11538-021-00890-8
![]() |
[53] |
R. Burghaus, K. Coboeken, T. Gaub, L. Kuepfer, A. Sensse, H.-U. Siegmund, et al., Evaluation of the efficacy and safety of rivaroxaban using a computer model for blood coagulation, PLoS One, 6 (2011), e17626. https://doi.org/10.1371/journal.pone.0017626 doi: 10.1371/journal.pone.0017626
![]() |
[54] |
C. Watson, H. Saaid, V. Vedula, J. C. Cardenas, P. K. Henke, F. Nicoud, et al., Venous thromboembolism: Review of clinical challenges, biology, assessment, treatment, and modeling, Ann. Biomed. Eng., 52 (2024), 467–486. https://doi.org/10.1007/s10439-023-03390-z doi: 10.1007/s10439-023-03390-z
![]() |
[55] |
N. N. Ramli, S. Iberahim, N. H. M. Noor, Z. Zulkafli, T. M. T. M. Shihabuddin, M. H. Din, et al., Haemostasis and inflammatory parameters as potential diagnostic biomarkers for VTE in trauma-immobilized patients, Diagnostics (Basel), 13 (2023), 150. https://doi.org/10.3390/diagnostics13010150 doi: 10.3390/diagnostics13010150
![]() |
[56] |
L. G. R. Ferreira, R. C. Figueiredo, M. das Graças Carvalho, D. R. A. Rios, Thrombin generation assay as a biomarker of cardiovascular outcomes and mortality: A narrative review, Thromb. Res., 220 (2022), 107–115. https://doi.org/10.1016/j.thromres.2022.10.007 doi: 10.1016/j.thromres.2022.10.007
![]() |
[57] |
M. S. Edvardsen, K. Hindberg, E. S. Hansen, V. M. Morelli, T. Ueland, P. Aukrust, et al., Plasma levels of von Willebrand factor and future risk of incident venous thromboembolism, Blood Adv., 5 (2021), 224–232. https://doi.org/10.1182/bloodadvances.2020003135 doi: 10.1182/bloodadvances.2020003135
![]() |
[58] |
L. Anghel, R. Sascău, R. Radu, C. Stătescu, From classical laboratory parameters to novel biomarkers for the diagnosis of venous thrombosis, Int. J. Mol. Sci., 21 (2020), 1920. https://doi.org/10.3390/ijms21061920 doi: 10.3390/ijms21061920
![]() |
[59] |
H. Y. Lim, C. O'Malley, G. Donnan, H. Nandurkar, P. Ho, A review of global coagulation assays — Is there a role in thrombosis risk prediction?, Thromb. Res., 179 (2019), 45–55. https://doi.org/10.1016/j.thromres.2019.04.033 doi: 10.1016/j.thromres.2019.04.033
![]() |
[60] |
H. Hou, Z. Ge, P. Ying, J. Dai, D. Shi, Z. Xu, et al., Biomarkers of deep venous thrombosis, J. Thromb. Thrombolysis., 34 (2012), 335–346. https://doi.org/10.1007/s11239-012-0721-y doi: 10.1007/s11239-012-0721-y
![]() |
[61] |
D. Moher, A. Liberati, J. Tetzlaff, D. G. Altman, Preferred reporting items for systematic reviews and meta-analyses: the PRISMA statement, PLoS Med., 6 (2009), e1000097. https://doi.org/10.1371/journal.pmed.1000097 doi: 10.1371/journal.pmed.1000097
![]() |
[62] |
M. J. Page, J. E. McKenzie, P. M. Bossuyt, I. Boutron, T. C. Hoffmann, C. D. Mulrow, et al., The PRISMA 2020 statement: an updated guideline for reporting systematic reviews, BMJ, 372 (2021), n71. https://doi.org/10.1136/bmj.n71 doi: 10.1136/bmj.n71
![]() |
[63] |
E. N. Sorensen, G. W. Burgreen, W. R. Wagner, J. F. Antaki, Computational simulation of platelet deposition and activation: I. Model development and properties, Ann. Biomed. Eng., 27 (1999), 436–448. https://doi.org/10.1114/1.200 doi: 10.1114/1.200
![]() |
[64] |
K. Boryczko, W. Dzwinel, D. A. Yuen, Modeling fibrin aggregation in blood flow with discrete-particles, Comput. Methods Programs Biomed., 75 (2004), 181–194. https://doi.org/10.1016/j.cmpb.2004.02.001 doi: 10.1016/j.cmpb.2004.02.001
![]() |
[65] |
I. V. Pivkin, P. D. Richardson, G. Karniadakis, Blood flow velocity effects and role of activation delay time on growth and form of platelet thrombi, Proc. Natl. Acad. Sci. USA, 103 (2006), 17164–17169. https://doi.org/10.1073/pnas.0608546103 doi: 10.1073/pnas.0608546103
![]() |
[66] |
Z. Xu, N. Chen, M. M. Kamocka, E. D. Rosen, M. Alber, A multiscale model of thrombus development, J. R. Soc. Interface, 5 (2008), 705–722. https://doi.org/10.1098/rsif.2007.1202 doi: 10.1098/rsif.2007.1202
![]() |
[67] |
Z. Xu, N. Chen, S. C. Shadden, J. E. Marsden, M. M. Kamocka, E. D. Rosen, et al., Study of blood flow impact on growth of thrombi using a multiscale model, Soft. Matter, 5 (2009), 769–779. https://doi.org/10.1039/B812429A doi: 10.1039/B812429A
![]() |
[68] |
Z. Xu, J. Lioi, J. Mu, M. M. Kamocka, X. Liu, D. Z. Chen, et al., A multiscale model of venous thrombus formation with surface-mediated control of blood coagulation cascade, Biophys. J., 98 (2010), 1723–1732. https://doi.org/10.1016/j.bpj.2009.12.4331 doi: 10.1016/j.bpj.2009.12.4331
![]() |
[69] |
A. M. Shibeko, E. S. Lobanova, M. A. Panteleev, F. I. Ataullakhanov, Blood flow controls coagulation onset via the positive feedback of factor Ⅶ activation by factor Xa, BMC Syst. Biol., 4 (2010), 5. https://doi.org/10.1186/1752-0509-4-5 doi: 10.1186/1752-0509-4-5
![]() |
[70] |
S. W. Jordan, E. L. Chaikof, Simulated surface-induced thrombin generation in a flow field, Biophys. J., 101 (2011), 276–286. https://doi.org/10.1016/j.bpj.2011.05.056 doi: 10.1016/j.bpj.2011.05.056
![]() |
[71] |
K. Leiderman, A. L. Fogelson, Grow with the flow: a spatial-temporal model of platelet deposition and blood coagulation under flow, Math. Med. Biol., 28 (2011), 47–84. https://doi.org/10.1093/imammb/dqq005 doi: 10.1093/imammb/dqq005
![]() |
[72] |
A. L. Fogelson, Y. H. Hussain, K. Leiderman, Blood clot formation under flow: The importance of factor XI depends strongly on platelet count, Biophys. J., 102 (2012), 10–18. https://doi.org/10.1016/j.bpj.2011.10.048 doi: 10.1016/j.bpj.2011.10.048
![]() |
[73] |
K. Leiderman, A. L. Fogelson, The influence of hindered transport on the development of platelet thrombi under flow, Bull. Math. Biol., 75 (2013), 1255–1283. https://doi.org/10.1007/s11538-012-9784-3 doi: 10.1007/s11538-012-9784-3
![]() |
[74] |
A. Tosenberger, F. Ataullakhanov, N. Bessonov, M. Panteleev, A. Tokarev, V. Volpert, Modelling of thrombus growth in flow with a DPD-PDE method, J. Theor. Biol., 337 (2013), 30–41. https://doi.org/10.1016/j.jtbi.2013.07.023 doi: 10.1016/j.jtbi.2013.07.023
![]() |
[75] |
A. Sequeira, T. Bodnár, Blood coagulation simulations using a viscoelastic model, Math. Model. Nat. Phenom., 9 (2014), 34–45. https://doi.org/10.1051/mmnp/20149604 doi: 10.1051/mmnp/20149604
![]() |
[76] |
A. Tosenberger, N. Bessonov, V. Volpert, Influence of fibrinogen deficiency on clot formation in flow by hybrid model, Math. Model. Nat. Phenom., 10 (2015), 36–47. https://doi.org/10.1051/mmnp/201510102 doi: 10.1051/mmnp/201510102
![]() |
[77] |
O. S. Rukhlenko, O. A. Dudchenko, K. E. Zlobina, G. T. Guria, Mathematical modeling of intravascular blood coagulation under wall shear stress, PLoS One, 10 (2015), e0134028. https://doi.org/10.1371/journal.pone.0134028 doi: 10.1371/journal.pone.0134028
![]() |
[78] |
J. Pavlova, A. Fasano, J. Janela, A. Sequeira, Numerical validation of a synthetic cell-based model of blood coagulation, J. Theor. Biol., 380 (2015), 367–379. https://doi.org/10.1016/j.jtbi.2015.06.004 doi: 10.1016/j.jtbi.2015.06.004
![]() |
[79] |
A. Piebalgs, X. Y. Xu, Towards a multi-physics modelling framework for thrombolysis under the influence of blood flow, J. R. Soc. Interface, 12 (2015), 20150949. https://doi.org/10.1098/rsif.2015.0949 doi: 10.1098/rsif.2015.0949
![]() |
[80] |
Z. Li, A. Yazdani, A. Tartakovsky, G. E. Karniadakis, Transport dissipative particle dynamics model for mesoscopic advection-diffusion-reaction problems, J. Chem. Phys., 143 (2015), 14101. https://doi.org/10.1063/1.4923254 doi: 10.1063/1.4923254
![]() |
[81] |
A. Bouchnita, K. Bouzaachane, T. Galochkina, P. Kurbatova, P. Nony, V. Volpert, An individualized blood coagulation model to predict INR therapeutic range during warfarin treatment, Math. Model. Nat. Phenom., 11 (2016), 28–44. https://doi.org/10.1051/mmnp/201611603 doi: 10.1051/mmnp/201611603
![]() |
[82] |
J. H. Seo, T. Abd, R. T. George, R. Mittal, A coupled chemo-fluidic computational model for thrombogenesis in infarcted left ventricles, Am. J. Physiol. Heart Circ. Physiol., 310 (2016), H1567–82. https://doi.org/10.1152/ajpheart.00855.2015 doi: 10.1152/ajpheart.00855.2015
![]() |
[83] |
M. N. Ngoepe, Y. Ventikos, Computational modelling of clot development in patient-specific cerebral aneurysm cases, J. Thromb. Haemost., 14 (2016), 262–272. https://doi.org/10.1111/jth.13220 doi: 10.1111/jth.13220
![]() |
[84] |
A. Bouchnita, T. Galochkina, V. Volpert, Influence of antithrombin on the regimes of blood coagulation: Insights from the mathematical model, Acta Biotheor., 64 (2016), 327–342. https://doi.org/10.1007/s10441-016-9291-2 doi: 10.1007/s10441-016-9291-2
![]() |
[85] |
E. V. Dydek, E. L. Chaikof, Simulated thrombin generation in the presence of surface-bound heparin and circulating tissue factor, Ann. Biomed. Eng., 44 (2016), 1072–1084. https://doi.org/10.1007/s10439-015-1377-5 doi: 10.1007/s10439-015-1377-5
![]() |
[86] |
J. Pavlova, A. Fasano, A. Sequeira, Numerical simulations of a reduced model for blood coagulation, Zeitschrift für Angew. Math. und Phys., 67 (2016), 28. https://doi.org/10.1007/s00033-015-0610-2 doi: 10.1007/s00033-015-0610-2
![]() |
[87] |
A. Tosenberger, F. Ataullakhanov, N. Bessonov, M. Panteleev, A. Tokarev, V. Volpert, Modelling of platelet-fibrin clot formation in flow with a DPD-PDE method, J. Math. Biol., 72 (2016), 649–681. https://doi.org/10.1007/s00285-015-0891-2 doi: 10.1007/s00285-015-0891-2
![]() |
[88] |
V. Govindarajan, V. Rakesh, J. Reifman, A. Y. Mitrophanov, Computational study of thrombus formation and clotting factor effects under venous flow conditions, Biophys. J., 110 (2016), 1869–1885. https://doi.org/10.1016/j.bpj.2016.03.010 doi: 10.1016/j.bpj.2016.03.010
![]() |
[89] |
C. Ou, W. Huang, M. M.-F. Yuen, A computational model based on fibrin accumulation for the prediction of stasis thrombosis following flow-diverting treatment in cerebral aneurysms, Med. Biol. Eng. Comput., 55 (2017), 89–99. https://doi.org/10.1007/s11517-016-1501-1 doi: 10.1007/s11517-016-1501-1
![]() |
[90] |
A. Yazdani, H. Li, J. D. Humphrey, G. E. Karniadakis, A general shear-dependent model for thrombus formation, PLoS Comput. Biol., 13 (2017), e1005291. https://doi.org/10.1371/journal.pcbi.1005291 doi: 10.1371/journal.pcbi.1005291
![]() |
[91] |
H. Hosseinzadegan, D. K. Tafti, Prediction of thrombus growth: Effect of stenosis and reynolds number, Cardiovasc. Eng. Technol., 8 (2017), 164–181. https://doi.org/10.1007/s13239-017-0304-3 doi: 10.1007/s13239-017-0304-3
![]() |
[92] |
L. M. Haynes, T. Orfeo, K. G. Mann, S. J. Everse, K. E. Brummel-Ziedins, Probing the dynamics of clot-bound thrombin at venous shear rates, Biophys. J., 112 (2017), 1634–1644. https://doi.org/10.1016/j.bpj.2017.03.002 doi: 10.1016/j.bpj.2017.03.002
![]() |
[93] |
H. Kamada, Y. Imai, M. Nakamura, T. Ishikawa, T. Yamaguchi, Shear-induced platelet aggregation and distribution of thrombogenesis at stenotic vessels, Microcirculation, 24 (2017). https://doi.org/10.1111/micc.12355 doi: 10.1111/micc.12355
![]() |
[94] |
J. D. Horn, D. J. Maitland, J. Hartman, J. M. Ortega, A computational thrombus formation model: application to an idealized two-dimensional aneurysm treated with bare metal coils, Biomech. Model. Mechanobiol., 17 (2018), 1821–1838. https://doi.org/10.1007/s10237-018-1059-y doi: 10.1007/s10237-018-1059-y
![]() |
[95] |
R. Méndez Rojano, S. Mendez, F. Nicoud, Introducing the pro-coagulant contact system in the numerical assessment of device-related thrombosis, Biomech. Model. Mechanobiol., 17 (2018), 815–826. https://doi.org/10.1007/s10237-017-0994-3 doi: 10.1007/s10237-017-0994-3
![]() |
[96] |
B. Gu, A. Piebalgs, Y. Huang, C. Longstaff, A. D. Hughes, R. Chen, et al., Mathematical modelling of intravenous thrombolysis in acute ischaemic stroke: Effects of dose regimens on levels of fibrinolytic proteins and clot lysis time, Pharmaceutics, 11 (2019), 111. https://doi.org/10.3390/pharmaceutics11030111 doi: 10.3390/pharmaceutics11030111
![]() |
[97] |
K. Ayabe, S. Goto, H. Oka, H. Yabushita, M. Nakayama, A. Tomita, et al., Potential different impact of inhibition of thrombin function and thrombin generation rate for the growth of thrombi formed at site of endothelial injury under blood flow condition, Thromb. Res., 179 (2019), 121–127. https://doi.org/10.1016/j.thromres.2019.05.007 doi: 10.1016/j.thromres.2019.05.007
![]() |
[98] |
J. Chen, S. L. Diamond, Reduced model to predict thrombin and fibrin during thrombosis on collagen/tissue factor under venous flow: Roles of γ'-fibrin and factor XIa, PLoS Comput. Biol., 15 (2019), e1007266. https://doi.org/10.1371/journal.pcbi.1007266 doi: 10.1371/journal.pcbi.1007266
![]() |
[99] |
A. Bouchnita, V. Volpert, A multiscale model of platelet-fibrin thrombus growth in the flow, Comput. Fluids, 184 (2019), 10–20. https://doi.org/10.1016/j.compfluid.2019.03.021 doi: 10.1016/j.compfluid.2019.03.021
![]() |
[100] |
H. Hosseinzadegan, D. K. Tafti, A predictive model of thrombus growth in stenosed vessels with dynamic geometries, J. Med. Biol. Eng., 39 (2019), 605–621. https://doi.org/10.1007/s40846-018-0443-5 doi: 10.1007/s40846-018-0443-5
![]() |
[101] |
O. E. Kadri, V. D. Chandran, M. Surblyte, R. S. Voronov, In vivo measurement of blood clot mechanics from computational fluid dynamics based on intravital microscopy images, Comput. Biol. Med., 106 (2019), 1–11. https://doi.org/10.1016/j.compbiomed.2019.01.001 doi: 10.1016/j.compbiomed.2019.01.001
![]() |
[102] |
J. Du, D. Kim, G. Alhawael, D. N. Ku, A. L. Fogelson, Clot permeability, agonist transport, and platelet binding kinetics in arterial thrombosis, Biophys. J., 119 (2020), 2102–2115. https://doi.org/10.1016/j.bpj.2020.08.041 doi: 10.1016/j.bpj.2020.08.041
![]() |
[103] |
W. T. Wu, M. Zhussupbekov, N. Aubry, J. F. Antaki, M. Massoudi, Simulation of thrombosis in a stenotic microchannel: The effects of vWF-enhanced shear activation of platelets, Int. J. Eng. Sci., 147 (2020), 103206. https://doi.org/10.1016/j.ijengsci.2019.103206 doi: 10.1016/j.ijengsci.2019.103206
![]() |
[104] |
A. Bouchnita, K. Terekhov, P. Nony, Y. Vassilevski, V. Volpert, A mathematical model to quantify the effects of platelet count, shear rate, and injury size on the initiation of blood coagulation under venous flow conditions, PLoS One, 15 (2020), e0235392. https://doi.org/10.1371/journal.pone.0235392 doi: 10.1371/journal.pone.0235392
![]() |
[105] |
Z. L. Liu, D. N. Ku, C. K. Aidun, Mechanobiology of shear-induced platelet aggregation leading to occlusive arterial thrombosis: A multiscale in silico analysis, J. Biomech., 120 (2021), 110349. https://doi.org/10.1016/j.jbiomech.2021.110349 doi: 10.1016/j.jbiomech.2021.110349
![]() |
[106] |
V. N. Kaneva, J. L. Dunster, V. Volpert, F. Ataullahanov, M. A. Panteleev, D. Y. Nechipurenko, Modeling thrombus shell: Linking adhesion receptor properties and macroscopic dynamics, Biophys. J., 120 (2021), 334–351. https://doi.org/10.1016/j.bpj.2020.10.049 doi: 10.1016/j.bpj.2020.10.049
![]() |
[107] |
A. Yazdani, Y. Deng, H. Li, E. Javadi, Z. Li, S. Jamali, et al., Integrating blood cell mechanics, platelet adhesive dynamics and coagulation cascade for modelling thrombus formation in normal and diabetic blood, J. R. Soc. Interface., 18 (2021), 20200834. https://doi.org/10.1098/rsif.2020.0834 doi: 10.1098/rsif.2020.0834
![]() |
[108] |
C. Ma, Y. Ren, Q. Zheng, J. Wang, A computational model on cartesian adaptive grid for thrombosis simulation, IEEE Access, 10 (2022), 67694–67702. https://doi.org/10.1109/ACCESS.2022.3184123 doi: 10.1109/ACCESS.2022.3184123
![]() |
[109] |
R. Méndez Rojano, A. Lai, M. Zhussupbekov, G. W. Burgreen, K. Cook, J. F. Antaki, A fibrin enhanced thrombosis model for medical devices operating at low shear regimes or large surface areas, PLoS Comput. Biol., 18 (2022), e1010277. https://doi.org/10.1371/journal.pcbi.1010277 doi: 10.1371/journal.pcbi.1010277
![]() |
[110] |
M. Rezaeimoghaddam, F. N. van de Vosse, Continuum modeling of thrombus formation and growth under different shear rates, J. Biomech., 132 (2022), 110915. https://doi.org/10.1016/j.jbiomech.2021.110915 doi: 10.1016/j.jbiomech.2021.110915
![]() |
[111] | A. S. Pisaryuk, N. M. Povalyaev, A. V Poletaev, A. M. Shibeko, Systems biology approach for personalized hemostasis correction, J. Pers. Med., 12 (2022). https://doi.org/10.3390/jpm12111903 |
[112] |
M. Zhussupbekov, R. Méndez Rojano, W. T. Wu, J. F. Antaki, von Willebrand factor unfolding mediates platelet deposition in a model of high-shear thrombosis, Biophys. J., 121 (2022), 4033–4047. https://doi.org/10.1016/j.bpj.2022.09.040 doi: 10.1016/j.bpj.2022.09.040
![]() |
[113] |
Y. Wang, J. Luan, K. Luo, T. Zhu, J. Fan, Multi-constituent simulation of thrombosis in aortic dissection, Int. J. Eng. Sci., 184 (2023), 103817. http://dx.doi.org/10.1016/j.ijengsci.2023.103817 doi: 10.1016/j.ijengsci.2023.103817
![]() |
[114] |
R. Petkantchin, A. Rousseau, O. Eker, K. Zouaoui Boudjeltia, F. Raynaud, B. Chopard, A simplified mesoscale 3D model for characterizing fibrinolysis under flow conditions, Sci. Rep., 13 (2023), 13681. https://doi.org/10.1038/s41598-023-40973-1 doi: 10.1038/s41598-023-40973-1
![]() |
[115] |
K. Miyazawa, A. L. Fogelson, K. Leiderman, Inhibition of platelet-surface-bound proteins during coagulation under flow Ⅱ: Antithrombin and heparin, Biophys. J., 122 (2023), 230–240. https://doi.org/10.1016/j.bpj.2022.10.038 doi: 10.1016/j.bpj.2022.10.038
![]() |
[116] |
A. R. Rezaie, S. T. Olson, Calcium enhances heparin catalysis of the antithrombin-factor Xa reaction by promoting the assembly of an intermediate heparin-antithrombin-factor Xa bridging complex: Demonstration by rapid kinetics studies, Biochemistry, 39 (2000), 12083–12090. https://doi.org/10.1021/bi0011126 doi: 10.1021/bi0011126
![]() |
[117] |
M. Anand, K. Rajagopal, K. R. Rajagopal, A model for the formation, growth, and lysis of clots in quiescent plasma: A comparison between the effects of antithrombin Ⅲ deficiency and protein C deficiency, J. Theor. Biol., 253 (2008), 725–738. https://doi.org/10.1016/j.jtbi.2008.04.015 doi: 10.1016/j.jtbi.2008.04.015
![]() |
[118] | M. J. Griffith, The heparin-enhanced antithrombin Ⅲ/thrombin reaction is saturable with respect to both thrombin and antithrombin Ⅲ, J. Biol. Chem., 257 (1982), 13302–13899. |
[119] | M. J. Griffith, Kinetics of the heparin-enhanced antithrombin Ⅲ/thrombin reaction. Evidence for a template model for the mechanism of action of heparin, J. Biol. Chem., 257 (1982), 7360–7365. |
[120] |
C. M. Danforth, T. Orfeo, K. G. Mann, K. E. Brummel-Ziedins, S. J. Everse, The impact of uncertainty in a blood coagulation model, Math. Med. Biol., 26 (2009), 323–336. https://doi.org/10.1093/imammb/dqp011 doi: 10.1093/imammb/dqp011
![]() |
[121] |
P. P. Naidu, M. Anand, Importance of Ⅷa inactivation in a mathematical model for the formation, growth, and lysis of clots, Math. Model. Nat. Phenom., 9 (2014), 17–33. https://doi.org/10.1051/mmnp/20149603 doi: 10.1051/mmnp/20149603
![]() |
[122] |
F. Saitta, J. Masuri, M. Signorelli, S. Bertini, A. Bisio, D. Fessas, Thermodynamic insights on the effects of low-molecular-weight heparins on antithrombin Ⅲ, Thermochim. Acta, 713 (2022), 179248. https://doi.org/10.1016/j.tca.2022.179248 doi: 10.1016/j.tca.2022.179248
![]() |
[123] |
H. Minakami, M. Morikawa, T. Yamada, T. Yamada, Candidates for the determination of antithrombin activity in pregnant women, J. Perinat. Med., 39 (2011), 369–374. https://doi.org/10.1515/jpm.2011.026 doi: 10.1515/jpm.2011.026
![]() |
[124] | K. C. Jones, K. G. Mann, A model for the tissue factor pathway to thrombin. Ⅱ: A mathematical simulation, J. Biol. Chem., 269 (1994), 23367–23373. |
[125] |
M. F. Hockin, K. C. Jones, S. J. Everse, K. G. Mann, A model for the stoichiometric regulation of blood coagulation, J. Biol. Chem., 277 (2002), 18322–18333. https://doi.org/10.1074/jbc.m201173200 doi: 10.1074/jbc.m201173200
![]() |
[126] |
R. Méndez Rojano, S. Mendez, D. Lucor, A. Ranc, M. Giansily-Blaizot, J. F. Schved, et al., Kinetics of the coagulation cascade including the contact activation system: sensitivity analysis and model reduction, Biomech. Model. Mechanobiol., 18 (2019), 1139–1153. https://doi.org/10.1007/s10237-019-01134-4 doi: 10.1007/s10237-019-01134-4
![]() |
[127] |
M. S. Chatterjee, W. S. Denney, H. Jing, S. L. Diamond, Systems biology of coagulation initiation: Kinetics of thrombin generation in resting and activated human blood, PLOS Comput. Biol., 6 (2010), e1000950. https://doi.org/10.1371/journal.pcbi.1000950 doi: 10.1371/journal.pcbi.1000950
![]() |
[128] |
A. Ranc, S. Bru, S. Mendez, M. Giansily-Blaizot, F. Nicoud, R. Méndez Rojano, Critical evaluation of kinetic schemes for coagulation, PLoS One, 18 (2023), e0290531. https://doi.org/10.1371/journal.pone.0290531 doi: 10.1371/journal.pone.0290531
![]() |
[129] |
M. F. Hockin, K. C. Jones, S. J. Everse, K. G. Mann, A model for the stoichiometric regulation of blood coagulation, J. Biol. Chem., 277 (2002), 18322–18333. https://doi.org/10.1074/jbc.m201173200 doi: 10.1074/jbc.m201173200
![]() |
[130] |
D. Luan, M. Zai, J. D. Varner, Computationally derived points of fragility of a human cascade are consistent with current therapeutic strategies, PLoS Comput. Biol., 3 (2007), 1347–1359. https://doi.org/10.1371/journal.pcbi.0030142 doi: 10.1371/journal.pcbi.0030142
![]() |
[131] |
K. G. Link, M. T. Stobb, J. Di Paola, K. B. Neeves, A. L. Fogelson, S. S. Sindi, et al., A local and global sensitivity analysis of a mathematical model of coagulation and platelet deposition under flow, PLoS One, 13 (2018), e0200917. https://doi.org/10.1371/journal.pone.0200917 doi: 10.1371/journal.pone.0200917
![]() |
![]() |
![]() |
1. | Emmanuel Papadakis, Eleni Gavriilaki, Nikolaos Kotsiou, Antonella Tufano, Benjamin Brenner, Fright of Long-Haul Flights: Focus on Travel-Associated Thrombosis, 2025, 0094-6176, 10.1055/s-0045-1805038 |
Reference | Biomarkers | |||||||||
D-dimer | P-selectin | PT | APTT | vWF | Fibrinogen | ATIII | FⅧ | PC | PS | |
Sorensen et al. (1999) [63] | X | |||||||||
Boryczko et al. (2004) [64] | X | |||||||||
Pivkin et al. (2006) [65] | X | |||||||||
Xu et al. (2008) [66] | X | X | ||||||||
Xu et al. (2009) [67] | X | |||||||||
Xu et al. (2010) [68] | X | X | X | |||||||
Shibeko et al. (2010) [69] | X | X | X | X | ||||||
Jordan and Chaikof (2011) [70] | X | X | X | |||||||
Leiderman and Fogelson (2011) [71] | X | X | X | |||||||
Fogelson et al. (2012) [72] | X | X | X | |||||||
Leiderman and Fogelson (2013) [73] | X | X | X | |||||||
Tosenberger et al. (2013) [74] | ||||||||||
Sequeira and Bódnar (2014) [75] | X | X | X | X | ||||||
Tosenberger et al. (2015) [76] | X | |||||||||
Rukhlenko et al. (2015) [77] | X | |||||||||
Pavlova et al. (2015) [78] | X | X | X | X | ||||||
Piebalgs and Xu (2015) [79] | X | |||||||||
Li et al. (2015) [80] | X | X | X | X | ||||||
Bouchnita et al. (2016) [81] | X | X | X | |||||||
Seo et al. (2016) [82] | X | X | ||||||||
Ngoepe and Ventikos (2016) [83] | X | X | ||||||||
Bouchnita et al. (2016) [84] | X | X | X | |||||||
Dydek and Chaikof (2016) [85] | X | X | X | |||||||
Pavlova et al. (2016) [86] | X | X | X | |||||||
Tosenberger et al. (2016) [87] | X | |||||||||
Govindarajan et al. (2016) [88] | X | X | X | X | ||||||
Ou et al. (2017) [89] | X | X | X | X | ||||||
Yazdani et al. (2017) [90] | X | X | X | X | ||||||
Hosseinzadegan et al. (2017) [91] | X | |||||||||
Haynes et al. (2017) [92] | X | |||||||||
Kamada et al. (2017) [93] | X | X | ||||||||
Horn et al. (2018) [94] | X | X | X | X | ||||||
Méndez Rojano et al. (2018) [95] | X | |||||||||
Gu et al. (2019) [96] | X | |||||||||
Ayabe et al. (2019) [97] | X | |||||||||
Chen and Diamon (2019) [98] | X | X | ||||||||
Bouchnita and Volpert (2019) [99] | X | X | X | |||||||
Hosseinzadegan and Tafti (2019) [100] | X | |||||||||
Kadri et al. (2019) [101] | X | |||||||||
Du et al. (2020) [102] | X | X | ||||||||
Wu et al. (2020) [103] | X | X | ||||||||
Bouchnita et al. (2020) [104] | X | X | ||||||||
Liu et al. (2021) [105] | X | |||||||||
Kaneva et al. (2021) [106] | X | |||||||||
Yazdani et al. (2021) [107] | X | X | X | X | X | |||||
Ma et al. (2022) [108] | X | |||||||||
Méndez Rojano et al. (2022) [109] | X | X | ||||||||
Rezaeimoghaddam and van de Vosse (2022) [110] | X | |||||||||
Pisaryuk et al. (2022)[111] | X | X | X | X | X | |||||
Zhussupbekov et al. (2022) [112] | X | X | ||||||||
Wang et al. (2023) [113] | X | |||||||||
Petkantchin et al. (2023) [114] | X | |||||||||
Miyazawa et al. (2023)[115] | X | X | X | |||||||
PT: prothrombin time. APTT: activated partial thromboplastin time. vWF: von Willebrand factor. ATIII: antithrombin Ⅲ. FⅧ: factor Ⅷ. PC: protein C. PS: protein S. |
Variable | Description | Range |
k++IIa/ATIII | Second-order inhibition rate constant of IIa by ATIII | 4.817 ×103 M-1.s-1 [99,104]–1.19 ×107 M-1.s-1 [75,78,80,90,107,113] |
k++IXa/ATIII | Second-order inhibition rate constant of IXa by ATIII | 1.36 ×102 M-1.s-1 [69] –2.7 ×105 M-1.s-1 [75,78,80,90,107,113] |
k++Xa/ATIII | Second-order inhibition rate constant of Xa by ATIII | 2.5 ×103 M-1.s-1 [111] –5.783 ×106 M-1.s-1 [75,78,80,90,107,113] |
k++XIa/ATIII | Second-order inhibition rate constant of XIa by ATIII | 8.0M-1.s-1 [111] –1.0 ×103 M-1.s-1 [94] |
k++XIIa/ATIII | Second-order inhibition rate constant of XIIa by ATIII | 3.645 ×101 M-1.s-1 [94] |
CATIII(t=0) | Initial concentration of ATIII | 1.566 ×10−6 M [78,86] –3.44 ×10−6 M [78,86] |
Variable | Description | Range |
kcatⅧ/IIa | Catalytic rate for the activation of factor Ⅷ by IIa | 0.9 s-1 [70] –3.24 s-1 [75,78,80,89,90,94,107] |
KmⅧ/IIa | Michaelis–Menten constant for the activation of factor Ⅷ by IIa | 1.47 ×10−7 M [69] –1.12 ×10−4 M [75,78,80,89,90,94,107] |
k++Ⅷ/Xa | Second-order rate constant for the activation of factor Ⅷ by factor Xa | 1.0 ×107 M-1.s-1 [82] –1.0 ×108 M-1.s-1 [95] |
k++Ⅷ/IIa k++Ⅷ,m | Second-order rate constant for the activation of factor Ⅷ by factor IIa | 2.0 ×107 M-1.s-1 [82]–5.0 ×107 M-1.s-1 [88] |
k+Ⅷa1L | First-order rate constant for the formation of Ⅷa1L | 2.2 ×10−5 s-1 [83] –6.0 ×10−3 s-1 [68] |
k++Ⅷa1L/Ⅷa2 | Second-order rate constant for Ⅷa | 2.2 ×104 M-1.s-1 [95]–6.0 ×106 M-1.s-1 [83] |
CⅧ(t=0) | Initial concentration of Ⅷ | 1.0 ×10−10 M [71] –7.0 ×10−10 M [82] |
CⅧa(t=0) | Initial concentration of Ⅷa | 0 M [83]–1.0 ×10−10 M [82] |
Reference | Biomarkers | |||||||||
D-dimer | P-selectin | PT | APTT | vWF | Fibrinogen | ATIII | FⅧ | PC | PS | |
Sorensen et al. (1999) [63] | X | |||||||||
Boryczko et al. (2004) [64] | X | |||||||||
Pivkin et al. (2006) [65] | X | |||||||||
Xu et al. (2008) [66] | X | X | ||||||||
Xu et al. (2009) [67] | X | |||||||||
Xu et al. (2010) [68] | X | X | X | |||||||
Shibeko et al. (2010) [69] | X | X | X | X | ||||||
Jordan and Chaikof (2011) [70] | X | X | X | |||||||
Leiderman and Fogelson (2011) [71] | X | X | X | |||||||
Fogelson et al. (2012) [72] | X | X | X | |||||||
Leiderman and Fogelson (2013) [73] | X | X | X | |||||||
Tosenberger et al. (2013) [74] | ||||||||||
Sequeira and Bódnar (2014) [75] | X | X | X | X | ||||||
Tosenberger et al. (2015) [76] | X | |||||||||
Rukhlenko et al. (2015) [77] | X | |||||||||
Pavlova et al. (2015) [78] | X | X | X | X | ||||||
Piebalgs and Xu (2015) [79] | X | |||||||||
Li et al. (2015) [80] | X | X | X | X | ||||||
Bouchnita et al. (2016) [81] | X | X | X | |||||||
Seo et al. (2016) [82] | X | X | ||||||||
Ngoepe and Ventikos (2016) [83] | X | X | ||||||||
Bouchnita et al. (2016) [84] | X | X | X | |||||||
Dydek and Chaikof (2016) [85] | X | X | X | |||||||
Pavlova et al. (2016) [86] | X | X | X | |||||||
Tosenberger et al. (2016) [87] | X | |||||||||
Govindarajan et al. (2016) [88] | X | X | X | X | ||||||
Ou et al. (2017) [89] | X | X | X | X | ||||||
Yazdani et al. (2017) [90] | X | X | X | X | ||||||
Hosseinzadegan et al. (2017) [91] | X | |||||||||
Haynes et al. (2017) [92] | X | |||||||||
Kamada et al. (2017) [93] | X | X | ||||||||
Horn et al. (2018) [94] | X | X | X | X | ||||||
Méndez Rojano et al. (2018) [95] | X | |||||||||
Gu et al. (2019) [96] | X | |||||||||
Ayabe et al. (2019) [97] | X | |||||||||
Chen and Diamon (2019) [98] | X | X | ||||||||
Bouchnita and Volpert (2019) [99] | X | X | X | |||||||
Hosseinzadegan and Tafti (2019) [100] | X | |||||||||
Kadri et al. (2019) [101] | X | |||||||||
Du et al. (2020) [102] | X | X | ||||||||
Wu et al. (2020) [103] | X | X | ||||||||
Bouchnita et al. (2020) [104] | X | X | ||||||||
Liu et al. (2021) [105] | X | |||||||||
Kaneva et al. (2021) [106] | X | |||||||||
Yazdani et al. (2021) [107] | X | X | X | X | X | |||||
Ma et al. (2022) [108] | X | |||||||||
Méndez Rojano et al. (2022) [109] | X | X | ||||||||
Rezaeimoghaddam and van de Vosse (2022) [110] | X | |||||||||
Pisaryuk et al. (2022)[111] | X | X | X | X | X | |||||
Zhussupbekov et al. (2022) [112] | X | X | ||||||||
Wang et al. (2023) [113] | X | |||||||||
Petkantchin et al. (2023) [114] | X | |||||||||
Miyazawa et al. (2023)[115] | X | X | X | |||||||
PT: prothrombin time. APTT: activated partial thromboplastin time. vWF: von Willebrand factor. ATIII: antithrombin Ⅲ. FⅧ: factor Ⅷ. PC: protein C. PS: protein S. |
Variable | Description | Range |
k++IIa/ATIII | Second-order inhibition rate constant of IIa by ATIII | 4.817 ×103 M-1.s-1 [99,104]–1.19 ×107 M-1.s-1 [75,78,80,90,107,113] |
k++IXa/ATIII | Second-order inhibition rate constant of IXa by ATIII | 1.36 ×102 M-1.s-1 [69] –2.7 ×105 M-1.s-1 [75,78,80,90,107,113] |
k++Xa/ATIII | Second-order inhibition rate constant of Xa by ATIII | 2.5 ×103 M-1.s-1 [111] –5.783 ×106 M-1.s-1 [75,78,80,90,107,113] |
k++XIa/ATIII | Second-order inhibition rate constant of XIa by ATIII | 8.0M-1.s-1 [111] –1.0 ×103 M-1.s-1 [94] |
k++XIIa/ATIII | Second-order inhibition rate constant of XIIa by ATIII | 3.645 ×101 M-1.s-1 [94] |
CATIII(t=0) | Initial concentration of ATIII | 1.566 ×10−6 M [78,86] –3.44 ×10−6 M [78,86] |
Variable | Description | Range |
kcatⅧ/IIa | Catalytic rate for the activation of factor Ⅷ by IIa | 0.9 s-1 [70] –3.24 s-1 [75,78,80,89,90,94,107] |
KmⅧ/IIa | Michaelis–Menten constant for the activation of factor Ⅷ by IIa | 1.47 ×10−7 M [69] –1.12 ×10−4 M [75,78,80,89,90,94,107] |
k++Ⅷ/Xa | Second-order rate constant for the activation of factor Ⅷ by factor Xa | 1.0 ×107 M-1.s-1 [82] –1.0 ×108 M-1.s-1 [95] |
k++Ⅷ/IIa k++Ⅷ,m | Second-order rate constant for the activation of factor Ⅷ by factor IIa | 2.0 ×107 M-1.s-1 [82]–5.0 ×107 M-1.s-1 [88] |
k+Ⅷa1L | First-order rate constant for the formation of Ⅷa1L | 2.2 ×10−5 s-1 [83] –6.0 ×10−3 s-1 [68] |
k++Ⅷa1L/Ⅷa2 | Second-order rate constant for Ⅷa | 2.2 ×104 M-1.s-1 [95]–6.0 ×106 M-1.s-1 [83] |
CⅧ(t=0) | Initial concentration of Ⅷ | 1.0 ×10−10 M [71] –7.0 ×10−10 M [82] |
CⅧa(t=0) | Initial concentration of Ⅷa | 0 M [83]–1.0 ×10−10 M [82] |