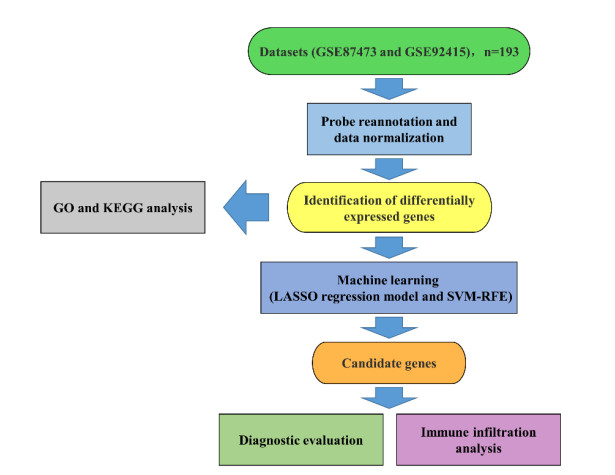
Ulcerative colitis (UC) is an idiopathic inflammatory disease with an increasing incidence. This study aimed to identify potential UC biomarkers and associated immune infiltration characteristics.
Two datasets (GSE87473 and GSE92415) were merged to obtain 193 UC samples and 42 normal samples. Using R, differentially expressed genes (DEGs) between UC and normal samples were filtered out, and their biological functions were investigated using Gene Ontology and Kyoto Encyclopedia of Genes and Genomes analyses. Promising biomarkers were identified using least absolute shrinkage selector operator regression and support vector machine recursive feature elimination, and their diagnostic efficacy was evaluated through receiver operating characteristic (ROC) curves. Finally, CIBERSORT was used to investigate the immune infiltration characteristics in UC, and the relationship between the identified biomarkers and various immune cells was examined.
We found 102 DEGs, of which 64 were significantly upregulated, and 38 were significantly downregulated. The DEGs were enriched in pathways associated with interleukin-17, cytokine–cytokine receptor interaction and viral protein interactions with cytokines and cytokine receptors, among others. Using machine learning methods and ROC tests, we confirmed DUOX2, DMBT1, CYP2B7P, PITX2 and DEFB1 to be essential diagnostic genes for UC. Immune cell infiltration analysis revealed that all five diagnostic genes were correlated with regulatory T cells, CD8 T cells, activated and resting memory CD4 T cells, activated natural killer cells, neutrophils, activated and resting mast cells, activated and resting dendritic cells and M0, M1 and M2 macrophages.
DUOX2, DMBT1, CYP2B7P, PITX2 and DEFB1 were identified as prospective biomarkers for UC. A new perspective on understanding the progression of UC may be provided by these biomarkers and their relationship with immune cell infiltration.
Citation: Yuedan Wang, Jinke Huang, Jiaqi Zhang, Fengyun Wang, Xudong Tang. Identifying biomarkers associated with the diagnosis of ulcerative colitis via bioinformatics and machine learning[J]. Mathematical Biosciences and Engineering, 2023, 20(6): 10741-10756. doi: 10.3934/mbe.2023476
[1] | Xuesi Chen, Qijun Zhang, Qin Zhang . Predicting potential biomarkers and immune infiltration characteristics in heart failure. Mathematical Biosciences and Engineering, 2022, 19(9): 8671-8688. doi: 10.3934/mbe.2022402 |
[2] | Ahmed Hammad, Mohamed Elshaer, Xiuwen Tang . Identification of potential biomarkers with colorectal cancer based on bioinformatics analysis and machine learning. Mathematical Biosciences and Engineering, 2021, 18(6): 8997-9015. doi: 10.3934/mbe.2021443 |
[3] | Chenyang Jiang, Weidong Jiang . AGTR1, PLTP, and SCG2 associated with immune genes and immune cell infiltration in calcific aortic valve stenosis: analysis from integrated bioinformatics and machine learning. Mathematical Biosciences and Engineering, 2022, 19(4): 3787-3802. doi: 10.3934/mbe.2022174 |
[4] | Chen Ma, Zhihao Yao, Qinran Zhang, Xiufen Zou . Quantitative integration of radiomic and genomic data improves survival prediction of low-grade glioma patients. Mathematical Biosciences and Engineering, 2021, 18(1): 727-744. doi: 10.3934/mbe.2021039 |
[5] | Fang Niu, Zongwei Liu, Peidong Liu, Hongrui Pan, Jiaxue Bi, Peng Li, Guangze Luo, Yonghui Chen, Xiaoxing Zhang, Xiangchen Dai . Identification of novel genetic biomarkers and treatment targets for arteriosclerosis-related abdominal aortic aneurysm using bioinformatic tools. Mathematical Biosciences and Engineering, 2021, 18(6): 9761-9774. doi: 10.3934/mbe.2021478 |
[6] | Mingshuai Chen, Xin Zhang, Ying Ju, Qing Liu, Yijie Ding . iPseU-TWSVM: Identification of RNA pseudouridine sites based on TWSVM. Mathematical Biosciences and Engineering, 2022, 19(12): 13829-13850. doi: 10.3934/mbe.2022644 |
[7] | Shuo Sun, Xiaoni Cai, Jinhai Shao, Guimei Zhang, Shan Liu, Hongsheng Wang . Machine learning-based approach for efficient prediction of diagnosis, prognosis and lymph node metastasis of papillary thyroid carcinoma using adhesion signature selection. Mathematical Biosciences and Engineering, 2023, 20(12): 20599-20623. doi: 10.3934/mbe.2023911 |
[8] | Natalya Shakhovska, Vitaliy Yakovyna, Valentyna Chopyak . A new hybrid ensemble machine-learning model for severity risk assessment and post-COVID prediction system. Mathematical Biosciences and Engineering, 2022, 19(6): 6102-6123. doi: 10.3934/mbe.2022285 |
[9] | Yu Jin, Zhe Ren, Wenjie Wang, Yulei Zhang, Liang Zhou, Xufeng Yao, Tao Wu . Classification of Alzheimer's disease using robust TabNet neural networks on genetic data. Mathematical Biosciences and Engineering, 2023, 20(5): 8358-8374. doi: 10.3934/mbe.2023366 |
[10] | Aiying Ying, Yueguang Zhao, Xiang Hu . Identification of biomarkers related to prostatic hyperplasia based on bioinformatics and machine learning. Mathematical Biosciences and Engineering, 2023, 20(7): 12024-12038. doi: 10.3934/mbe.2023534 |
Ulcerative colitis (UC) is an idiopathic inflammatory disease with an increasing incidence. This study aimed to identify potential UC biomarkers and associated immune infiltration characteristics.
Two datasets (GSE87473 and GSE92415) were merged to obtain 193 UC samples and 42 normal samples. Using R, differentially expressed genes (DEGs) between UC and normal samples were filtered out, and their biological functions were investigated using Gene Ontology and Kyoto Encyclopedia of Genes and Genomes analyses. Promising biomarkers were identified using least absolute shrinkage selector operator regression and support vector machine recursive feature elimination, and their diagnostic efficacy was evaluated through receiver operating characteristic (ROC) curves. Finally, CIBERSORT was used to investigate the immune infiltration characteristics in UC, and the relationship between the identified biomarkers and various immune cells was examined.
We found 102 DEGs, of which 64 were significantly upregulated, and 38 were significantly downregulated. The DEGs were enriched in pathways associated with interleukin-17, cytokine–cytokine receptor interaction and viral protein interactions with cytokines and cytokine receptors, among others. Using machine learning methods and ROC tests, we confirmed DUOX2, DMBT1, CYP2B7P, PITX2 and DEFB1 to be essential diagnostic genes for UC. Immune cell infiltration analysis revealed that all five diagnostic genes were correlated with regulatory T cells, CD8 T cells, activated and resting memory CD4 T cells, activated natural killer cells, neutrophils, activated and resting mast cells, activated and resting dendritic cells and M0, M1 and M2 macrophages.
DUOX2, DMBT1, CYP2B7P, PITX2 and DEFB1 were identified as prospective biomarkers for UC. A new perspective on understanding the progression of UC may be provided by these biomarkers and their relationship with immune cell infiltration.
Ulcerative colitis (UC) is a type of idiopathic, immune-mediated inflammatory bowel disease (IBD) with symptoms of diarrhea, abdominal pain and mucopurulent bloody stools [1]. The prevalence of IBD is more than 0.3% in numerous nations in North America, Oceania and Europe, being the highest in Norway: 505 UC cases per 100,000 people [2]. The mean annual healthcare cost per UC patient is approximately?2088, and its burden is increasing globally [3].
The pathogenesis of UC, including infection details, role of the immune system and the environment and sensitive genes, has not been fully delineated yet [4]. UC treatment aims to induce and maintain remission. However, the management of UC is extremely difficult due to its poorly known etiology [1]. The establishment of novel diagnostic biomarkers that reflect the inflammatory damage to the intestinal mucosa is important for the timely diagnosis and treatment of UC.
Recent high-throughput microarray analysis of specimens from patients and healthy subjects has allowed us to investigate various diseases at multiple levels, revealing the numerous genes activated in different tissues as well as their physiological and pathological states [5,6]. Bioinformatics and machine learning can be efficiently combined to analyze high-throughput microarray data to further explore disease mechanisms and identify potential biomarkers.
Several studies have analyzed UC and IBD microarray data [7,8,9]. Zhang identified five genes as potential indicators for UC tissue biopsy and found the DUOX2/DUOXA2 and CXCL1/CXCR2 pathways to be crucial in the development of UC [8]. Shi discovered that core differentially expressed genes (DEGs) found in UC are associated with inflammation and the immune response and that CXCR2 may indicate the degree of inflammation in UC patients [9]. These findings further reveal core features of UC pathogenesis. Further bioinformatic analysis of UC is now possible with the advances in bioinformatics technology and the application of machine learning to pathology. In this study, bioinformatic and machine learning analyses of microarray data were employed to identify prospective biomarkers for the diagnosis of UC.
The flowchart of analysis steps is shown in Figure 1. GSE87473 and GSE92415 were downloaded from Gene Expression Omnibus (www.ncbi.nlm.nih.gov/geo/) [10]. Expression data for GSE87473 were generated using 106 cases of UC and 21 healthy controls, and data for GSE92415 were generated using 87 cases of UC and 21 healthy controls. Gene probe IDs in the matrix file were converted into international standard gene names using Perl. The two datasets were normalized and merged into a metadata cohort for subsequent integrated analysis using the "limma" package in R. Furthermore, the "SVA" package in R was applied to remove batch effects. All gene expression data were subjected to log2 transformation.
Linear models from the "limma" package in R were used to identify DEGs between colon tissues from UC patients and healthy subjects. The Benjamini–Hochberg approach was used to control the false discovery rate. Genes with adjusted p < 0.05 and log2 (fold change) > 2 were considered as DEGs. Finally, the "ggplot2" and "pheatmap" packages were used to plot the volcano plots and heat map of the DEGs, respectively.
Gene Ontology (GO) is a bioinformatics resource for annotating genomes with terms such as molecular functions, cellular components and biological processes. As a comprehensive database resource, the Kyoto Encyclopedia of Genes and Genomes (KEGG) aims to provide biological interpretation of high-throughput data or genomic data, ultimately helping identify functional and metabolic pathways. In this study, GO and KEGG enrichment analyses of DEGs were performed using the "clusterProfiler" package in R. p < 0.05 was considered to indicate significant enrichment of DEGs.
Two machine learning methods were applied to the potential diagnostic criteria for predicting UC status. Using the "glmnet" package in R, least absolute shrinkage selector operator (LASSO) regression was carried out to identify genes significantly associated with the discrimination between UC and healthy samples. Support vector machine (SVM) is a machine learning method that is frequently employed for classification and regression analysis. With the aim of preventing overfit, the optimum genes in the metadata cohort were filtered using recursive feature elimination (RFE). Consequently, SVM-RFE was used to filter acceptable features to discover the gene set with the highest discrimination capacity.
To estimate the prediction value for UC diagnosis, the "pROC" package in R was used. Area under the curve (AUC) of the receiver operating characteristic (ROC) curve was calculated to judge the accuracy of the diagnostic biomarkers: The higher the AUC value is, the higher the accuracy.
To reveal the differences in immune characteristics between UC patients and health individuals, the CIBERSORT algorithm in R was applied to evaluate the score distribution and differential expression of 22 immune cells in the gene expression profile. The advantages of using CIBERSORT for gene expression profiling data are the removal of unknown mixture content and the removal of artifacts through deconvolution, ultimately providing an estimate of the relative proportions of the 22 immune cell subpopulations, which were displayed using the "ggpubr'' package in R. The "ggplot2" package in R was applied to create a boxplot depicting the differences between the UC and normal groups.
Continuous variables were expressed as the mean ± standard deviation, whereas categorical variables were expressed as percentages. The Mann–Whitney U test or Kruskal–Wallis H test was used to compare between non-normal data, t-test or one-way analysis of variance was used for normally distributed data, and Fisher's exact probability method or chi-squared test was used for supCount data. All data analyses in this study were performed in R, and a p-value < 0.05 was regarded as significant.
The two gene expression datasets included 193 UC cases and 42 healthy controls. In total, 102 DEGs were identified from the expression data, including 64 upregulated genes and 38 downregulated genes. Figure 2 depicts the heatmap and volcano plot for the discovered DEGs.
GO analysis revealed that DEGs associated with antimicrobial humoral immune response, humoral immune response, reaction to molecule of bacterial origin and neutrophil chemotaxis were considerably enriched (Figure 3A). KEGG analysis showed that DEGs were significantly enriched in pathways involving interleukin-17 (IL-17), cytokine–cytokine receptor interaction, rheumatoid arthritis, viral protein interaction with cytokine–cytokine receptor, Staphylococcus aureus infection and others (Figure 3B).
To identify potential biomarkers for UC, two different arithmetic methods were used. LASSO regression identified 13 variables as diagnostic markers for UC (Figure 4A). SVM-RFE was used to determine a subset of 10 DEG features (Figure 4B). Five features that overlapped between the two arithmetic methods—DUOX2, DMBT1, CYP2B7P, PITX2 and DEFB1—were finally selected as key genes in UC development (Figure 4C).
The expression levels of DUOX2 and DMBT1 were significantly higher in UC samples than in healthy samples, while those of CYP2B7P, PITX2 and DEFB1 were significantly lower in UC samples (Figure 5).
ROC curve analysis was carried out to determine the utility of these diagnostic markers. The AUC values for DUOX2, CYP2B7P, PITX2, DEFB1 and DMBT1 were 0.985, 0.966, 0.968, 0.966 and 0.896, respectively, indicating that all of them displayed a strong ability to distinguish UC cases from normal samples (Figure 6).
CIBERSORT was used to elucidate the relative proportions of 22 immune cells in UC and normal tissues, as well as their relationships with one another (Figure 7A, B). Differences were observed in the levels of memory B cells, CD8 T cells, activated and resting memory CD4 T cells, activated natural killer (NK) cells, neutrophils, activated and resting mast cells, activated and resting dendritic cells and M0, M1 and M2 macrophages.
The associations between diagnostic marker expression and immune cell infiltration were investigated in greater detail. All five DEGs were correlated with regulatory T cells, CD8 T cells, resting and activated memory CD4 T cells, activated NK cells, neutrophils, resting and activated mast cells, M0, M1 and M2 macrophages and resting and activated dendritic cells. DUOX2, DMBT1, CYP2B7P, PITX2 and DEFB1 may be implicated in the progression of UC by modulating several immune cells.
UC is a common type of IBD with various degrees of mucosal lesions and recurrent episodes [11]. By definition, UC is considered to be limited to the superficial layer of the rectal and colonic mucosa, but given its proximal expansion and effects on colonic function, it should actually be viewed as a progressive condition with a dismal prognosis [12]. Prompt diagnosis and treatment are crucial for patients with UC, but such early diagnostic tools are still absent from clinical practice. Based on our examination of the two Gene Expression Omnibus datasets, we could highlight 102 DEGs that distinguished UC samples from healthy controls. Analysis of these DEGs using GO terms revealed that they were disproportionately involved in processes related to neutrophil chemotaxis, response to molecules of bacterial origin, humoral immune response and antimicrobial peptide-mediated antimicrobial humoral immune response. These DEGs were primarily implicated in pathways associated with IL-17, cytokine–cytokine receptor interaction, rheumatoid arthritis, viral protein interaction with cytokine–cytokine receptor and S. aureus infection, as shown by KEGG analysis. These findings suggest that these DEGs are actively engaged in immunological processes and may be critical for the development of UC.
From the initial screen of 102 DEGs, two machine learning techniques identified five genes as potential diagnostic biomarkers for UC. Dual oxidase 2 (DUOX2) is a member of the nicotinamide adenine dinucleotide phosphate oxidase family. It is expressed apically in the epithelial cells of the ileum and colon, where it catalyzes the generation of reactive oxygen species during normal cellular processes and phagocytosis [13]. DUOX2 can regulate the interaction between the intestinal microbiota and the mucosa while maintaining the normal intestinal mucosal barrier function [8,14]. Deleted in malignant brain tumors 1 (DMBT1) is an innate immune protein expressed on the mucosal surfaces of various human tissues, particularly throughout the luminal gastrointestinal tract [15,16]. It promotes epithelial differentiation and angiogenesis. Cytochrome 2B7P (CYP2B7P) is a pseudogene from the cytochrome P450 family. Its involvement in disease progression has been rarely reported [17]. Paired like homeodomain 2 (PITX2) contributes to intestinal formation and symmetry through multiple signaling pathways [18,19]. Due to its importance in DNA methylation and tumor immune infiltration, it may be connected to an increased risk of carcinogenesis in UC patients [20,21]. Beta-defensin 1 (DEFB1) is an antimicrobial peptide associated with surface resistance of epithelial cells to microbial invasion. Downregulation of DEFB1 leads to an impaired intestinal mucosal barrier function, which is closely related to the development of UC [22,23]. ROC assays confirmed the strong ability of these five diagnostic genes to screen UC specimens from normal specimens, verifying their promise as prospective biomarkers for UC.
Recent evidence highlights the critical role of immune cell infiltration in the onset and progression of UC. Dendritic cells and macrophages present antigens and secrete several proinflammatory cytokines, inducing a Th2 cell immune response [24,25]. Compared with healthy controls, UC patients had a lower percentage of Foxp3+CD4+ T cells within their peripheral blood mononuclear cell population [26], an accumulation of activated neutrophils in the circulation and colonic tissue [27] and NK cells with impaired mitochondrial function and attenuated killing ability [28]. The intestinal T cell infiltrates in UC patients were richer in CD4+, regulatory and central memory T cells, while the proportions of CD8+ and CD103+ T cells were lower [29]. The evolutionary direction of colonic T cells in UC patients is in the GZMK+TNF+ effector state and in the immunomodulatory characteristic with clonally expanded IL-26 state. The imbalance in their evolutionary expressions may promote intestinal tissue destruction [30]. Mast cells are engaged in regulating intestinal mucosal permeability and barrier function, and their degranulation has been linked to the etiology of UC [31,32]. In the absence of IL-10 signaling, macrophages promote the progression of UC by activating NOD-like receptor pyrin domain-containing 3 inflammasome and enhancing proinflammatory cytokine production [33].
Assessing the extent and diversity of immune cell infiltration offers insights into the molecular mechanisms underlying UC and helps identify new immunotherapeutic targets. Regulatory T cells, CD8 T cells, resting and activated memory CD4 T cells, activated NK cells, neutrophils, resting and activated mast cells, M0 M1 and M2 macrophages and resting and activated dendritic cells were all associated with the five core DEGs in this study, suggesting that these genes may play a role in the pathogenesis of UC by forming close connections with immune cells.
To assist the early diagnosis and to further characterize the pathogenesis of UC, LASSO regression and SVM-RFE were used to screen potential biomarkers, while CIBERSORT was used to profile the immune cell infiltrates in UC, which has been rarely reported in the past. This study has some limitations. First, molecular biology experiments or large-scale clinical trials were not performed to validate the diagnostic efficacy of the five core genes. Second, this study only establishes a correlation between UC and immune cells, not a causal relationship. More convincing evidence is required to reveal the intricate interactions between immune cells and genes.
Computational biology research provides new ideas and prospects for biological experiments in the study of UC [9]. Computational biology techniques are evolving, and microRNAs (miRNAs) and long noncoding RNAs (lncRNAs) may be the future direction for UC biomarker research. In recent years, several new biocomputational models have been developed, including graph convolutional neural networks and conditional random fields [34], logistic matrix factorization with neighborhood regularized [35] and a network distance analysis model for predicting lncRNA–miRNA associations [36]. Applying these new models to UC research will help reveal the critical role of miRNA–lncRNA interactions and their functions in the pathogenesis of UC. Deep learning algorithms are also improving constantly [37,38]. The use of updated bioinformatic analysis tools offers an in-depth understanding of the essential characteristics of UC.
This study identified five candidate genes—DUOX2, DMBT1, CYP2B7P, PITX2 and DEFB1—as prospective biomarkers for UC, offering new insight into the development of the disease.
This work was supported by the National Natural Science Foundation of China (No. 81830118), China Academy of Chinese Medical Sciences Innovation Fund (No. CI 2021A01012), Administration of Traditional Chinese Medicine Digestive Refractory Disease Inheritance and Innovation Team Project (No. ZYYCXTD-C-C202010) and China Academy of Chinese Medical Sciences Excellent Young Talent Cultivation Fund (No. ZZ 15-YQ-002).
The authors declare that they have no potential conflicts of interest.
[1] |
R. Ungaro, S. Mehandru, P. B. Allen, L. Peyrin-Biroulet, J. F. Colombel, Ulcerative colitis, Lancet, 389 (2017), 1756–1770. http://doi.org/10.1016/s0140-6736(16)32126-2 doi: 10.1016/s0140-6736(16)32126-2
![]() |
[2] |
S. C. Ng, H. Y. Shi, N. Hamidi, F. E. Underwood, W. Tang, E. I. Benchimol, et al., Worldwide incidence and prevalence of inflammatory bowel disease in the 21st century: a systematic review of population-based studies, Lancet, 390 (2017), 2769–2778. http://doi.org/10.1016/s0140-6736(17)32448-0 doi: 10.1016/s0140-6736(17)32448-0
![]() |
[3] |
J. Burisch, H. Vardi, D. Schwartz, M. Friger, G. Kiudelis, J. Kupčinskas, et al., Health-care costs of inflammatory bowel disease in a pan-European, community-based, inception cohort during 5 years of follow-up: a population-based study, Lancet Gastroenterol. Hepatol., 5 (2020), 454–464. http://doi.org/10.1016/s2468-1253(20)30012-1 doi: 10.1016/s2468-1253(20)30012-1
![]() |
[4] |
M. Gajendran, P. Loganathan, G. Jimenez, A. P. Catinella, N. Ng, C. Umapathy, et al., A comprehensive review and update on ulcerative colitis, Dis. Mon., 65 (2019), 100851. http://doi.org/10.1016/j.disamonth.2019.02.004 doi: 10.1016/j.disamonth.2019.02.004
![]() |
[5] |
C. Parola, D. Neumeier, S. T. Reddy, Integrating high-throughput screening and sequencing for monoclonal antibody discovery and engineering, Immunology, 153 (2018), 31–41. http://doi.org/10.1111/imm.12838 doi: 10.1111/imm.12838
![]() |
[6] |
M. A. Dillies, A. Rau, J. Aubert, C. Hennequet-Antier, M. Jeanmougin, N. Servant, et al., A comprehensive evaluation of normalization methods for Illumina high-throughput RNA sequencing data analysis, Briefings Bioinf., 14 (2013), 671–683. http://doi.org/10.1093/bib/bbs046 doi: 10.1093/bib/bbs046
![]() |
[7] |
A. F. Di Narzo, S. E. Telesco, C. Brodmerkel, C. Argmann, L. A. Peters, K. Li, et al., High-throughput characterization of blood serum proteomics of IBD patients with respect to aging and genetic factors, PLoS Genet., 13 (2017). http://doi.org/10.1371/journal.pgen.1006565 doi: 10.1371/journal.pgen.1006565
![]() |
[8] |
J. Zhang, X. Wang, L. Xu, Z. Zhang, F. Wang, X. Tang, Investigation of potential genetic biomarkers and molecular mechanism of ulcerative colitis utilizing bioinformatics analysis, Biomed. Res. Int., 2020 (2020). http://doi.org/10.1155/2020/4921387 doi: 10.1155/2020/4921387
![]() |
[9] |
L. Shi, X. Han, J. X. Li, Y. T. Liao, F. S. Kou, Z. B. Wang, et al., Identification of differentially expressed genes in ulcerative colitis and verification in a colitis mouse model by bioinformatics analyses, World J. Gastroenterol., 26 (2020), 5983–5996. http://doi.org/10.3748/wjg.v26.i39.5983 doi: 10.3748/wjg.v26.i39.5983
![]() |
[10] |
T. Barrett, D. B. Troup, S. E. Wilhite, P. Ledoux, D. Rudnev, C. Evangelista, et al., NCBI GEO: mining tens of millions of expression profiles--database and tools update, Nucleic Acids Res., 35 (2007), 760–765. http://doi.org/10.1093/nar/gkl887 doi: 10.1093/nar/gkl887
![]() |
[11] |
B. Verstockt, B. Bressler, H. Martinez-Lozano, D. McGovern, M. S. Silverberg, Time to revisit disease classification in inflammatory bowel disease: Is the current classification of inflammatory bowel disease good enough for optimal clinical management, Gastroenterology, 162 (2022), 1370–1382. http://doi.org/10.1053/j.gastro.2021.12.246 doi: 10.1053/j.gastro.2021.12.246
![]() |
[12] |
N. Cleveland, J. Torres, D. T. Rubin, What does disease progression look like in ulcerative colitis, and how might it be prevented, Gastroenterology, 162 (2022), 1396–1408. http://doi.org/10.1053/j.gastro.2022.01.023 doi: 10.1053/j.gastro.2022.01.023
![]() |
[13] |
J. P. Taylor, H. M. Tse, The role of NADPH oxidases in infectious and inflammatory diseases, Redox Biol., 48 (2021), 102159. http://doi.org/10.1016/j.redox.2021.102159 doi: 10.1016/j.redox.2021.102159
![]() |
[14] |
H. Grasberger, J. Gao, H. Nagao-Kitamoto, S. Kitamoto, M. Zhang, N. Kamada, et al., Increased expression of DUOX2 is an epithelial response to mucosal dysbiosis required for immune homeostasis in mouse intestine, Gastroenterology, 149 (2015), 1849–1859. http://doi.org/10.1053/j.gastro.2015.07.062 doi: 10.1053/j.gastro.2015.07.062
![]() |
[15] |
H. Müller, A. Schmiedl, C. Weiss, M. Ai, S. Jung, M. Renner, DMBT1 is upregulated in lung epithelial cells after hypoxia and changes surfactant ultrastructure, Pediatr. Pulmonol., 55 (2020), 2964–2969. http://doi.org/10.1002/ppul.25018 doi: 10.1002/ppul.25018
![]() |
[16] |
A. Nexoe, A. Pedersen, S. von Huth, S. Detlefsen, P. L. Hansen, U. Holmskov, Immunohistochemical localization of deleted in malignant brain tumors 1 in normal human tissues, J. Histochem. Cytochem., 68 (2020), 377–387. http://doi.org/10.1369/0022155420927109 doi: 10.1369/0022155420927109
![]() |
[17] |
H. Sezutsu, G. Le Goff, R. Feyereisen, Origins of P450 diversity, Phil. Trans. R. Soc. B, 368 (2013). http://doi.org/10.1098/rstb.2012.0428 doi: 10.1098/rstb.2012.0428
![]() |
[18] |
T. Q. Tran, C. Kioussi, Pitx genes in development and disease, Cell. Mol. Life Sci., 78 (2021), 4921–4938. http://doi.org/10.1007/s00018-021-03833-7 doi: 10.1007/s00018-021-03833-7
![]() |
[19] |
B. D. Sanketi, N. Zuela-Sopilniak, E. Bundschuh, S. Gopal, S. Hu, J. Long, et al., Pitx2 patterns an accelerator-brake mechanical feedback through latent TGFβ to rotate the gut, Science, 377 (2022). http://doi.org/10.1126/science.abl3921 doi: 10.1126/science.abl3921
![]() |
[20] |
Y. Zhao, J. Zhao, M. Zhong, Q. Zhang, F. Yan, Y. Feng, et al., The expression and methylation of PITX genes is associated with the prognosis of head and neck squamous cell carcinoma, Front. Genet., 13 (2022). http://doi.org/10.3389/fgene.2022.982241 doi: 10.3389/fgene.2022.982241
![]() |
[21] |
A. Semaan, B. Uhl, V. Branchi, P. Lingohr, F. Bootz, G. Kristiansen, et al., Significance of PITX2 promoter methylation in colorectal carcinoma prognosis, Clin. Colorectal Cancer Gastrointest. Malig., 17 (2018), 385–393. http://doi.org/10.1016/j.clcc.2018.02.008 doi: 10.1016/j.clcc.2018.02.008
![]() |
[22] |
J. Wehkamp, J. Harder, M. Weichenthal, O. Mueller, K. R. Herrlinger, K. Fellermann, et al., Inducible and constitutive beta-defensins are differentially expressed in Crohn's disease and ulcerative colitis, Inflammatory Bowel Dis., 9 (2003), 215–223. http://doi.org/10.1097/00054725-200307000-00001 doi: 10.1097/00054725-200307000-00001
![]() |
[23] |
G. Barbara, M. R. Barbaro, D. Fuschi, M. Palombo, F. Falangone, C. Cremon, et al., Inflammatory and microbiota-related regulation of the intestinal epithelial barrier, Front. Nutr., 8 (2021). http://doi.org/10.3389/fnut.2021.718356 doi: 10.3389/fnut.2021.718356
![]() |
[24] |
C. Ohnmacht, J. H. Park, S. Cording, J. B. Wing, K. Atarashi, Y. Obata, et al., The microbiota regulates type 2 immunity through RORγt⁺ T cells, Science, 349 (2015), 989–993. http://doi.org/10.1126/science.aac4263 doi: 10.1126/science.aac4263
![]() |
[25] |
F. Heller, P. Florian, C. Bojarski, J. Richter, M. Christ, B. Hillenbrand, et al., Interleukin-13 is the key effector Th2 cytokine in ulcerative colitis that affects epithelial tight junctions, apoptosis, and cell restitution, Gastroenterology, 129 (2005), 550–564. http://doi.org/10.1016/j.gastro.2005.05.002 doi: 10.1016/j.gastro.2005.05.002
![]() |
[26] |
N. Kulkarni, H. T. Meitei, S. A. Sonar, P. K. Sharma, V. R. Mujeeb, S. Srivastava, et al., CCR6 signaling inhibits suppressor function of induced-Treg during gut inflammation, J. Autoimmun., 88 (2018), 121–130. http://doi.org/10.1016/j.jaut.2017.10.013 doi: 10.1016/j.jaut.2017.10.013
![]() |
[27] |
N. Narula, E. Wong, J. F. Colombel, R. Riddell, J. K. Marshall, W. Reinisch, et al., Early change in epithelial neutrophilic infiltrate predicts long-term response to biologics in ulcerative colitis, Clin. Gastroenterol. Hepatol., 20 (2022), 1095–1104. http://doi.org/10.1016/j.cgh.2021.07.005 doi: 10.1016/j.cgh.2021.07.005
![]() |
[28] |
V. Bittencourt, F. Jones, M. Tosetto, G. A. Doherty, E. J. Ryan, Dysregulation of metabolic pathways in circulating natural killer cells isolated from inflammatory bowel disease patients, J. Crohn's Colitis, 15 (2021), 1316–1325. http://doi.org/10.1093/ecco-jcc/jjab014 doi: 10.1093/ecco-jcc/jjab014
![]() |
[29] |
C. Smids, C. S. Horjus-Talabur Horje, J. Drylewicz, B. Roosenboom, M. Groenen, E. van Koolwijk, et al., Intestinal T cell profiling in inflammatory bowel disease: Linking T cell subsets to disease activity and disease course, J. Crohn's Colitis, 12 (2018), 465–475. http://doi.org/10.1093/ecco-jcc/jjx160 doi: 10.1093/ecco-jcc/jjx160
![]() |
[30] |
D. Corridoni, A. Antanaviciute, T. Gupta, D. Fawkner-Corbett, A. Aulicino, M. Jagielowicz, et al., Single-cell atlas of colonic CD8 (+) T cells in ulcerative colitis, Nat. Med., 26 (2020), 1480–1490. http://doi.org/10.1038/s41591-020-1003-4 doi: 10.1038/s41591-020-1003-4
![]() |
[31] |
M. Raithel, S. Winterkamp, A. Pacurar, P. Ulrich, J. Hochberger, E. G. Hahn, Release of mast cell tryptase from human colorectal mucosa in inflammatory bowel disease, Scand. J. Gastroenterol., 36 (2001), 174–179. https://doi.org/10.1080/00365520119214 doi: 10.1080/00365520119214
![]() |
[32] |
E. Chen, L. S. Chuang, M. Giri, N. Villaverde, N. Y. Hsu, K. Sabic, et al., Inflamed ulcerative colitis regions associated with MRGPRX2-Mediated mast cell degranulation and cell activation modules, defining a new therapeutic target, Gastroenterology, 160 (2021), 1709–1724. http://doi.org/10.1053/j.gastro.2020.12.076 doi: 10.1053/j.gastro.2020.12.076
![]() |
[33] |
W. Ip, N. Hoshi, D. S. Shouval, S. Snapper, R. Medzhitov, Anti-inflammatory effect of IL-10 mediated by metabolic reprogramming of macrophages, Science, 356 (2017), 513–519. http://doi.org/10.1126/science.aal3535 doi: 10.1126/science.aal3535
![]() |
[34] |
W. Wang, L. Zhang, J. Sun, Q. Zhao, J. Shuai, Predicting the potential human lncRNA-miRNA interactions based on graph convolution network with conditional random field, Briefings Bioinf., 23 (2022). http://doi.org/10.1093/bib/bbac463 doi: 10.1093/bib/bbac463
![]() |
[35] |
H. Liu, G. Ren, H. Chen, Q. Liu, Y. Yang, Q. Zhao, Predicting lncRNA-miRNA interactions based on logistic matrix factorization with neighborhood regularized, Knowledge-Based Syst., 191 (2020), 105261. http://doi.org/10.1016/j.knosys.2019.105261 doi: 10.1016/j.knosys.2019.105261
![]() |
[36] |
L. Zhang, P. Yang, H. Feng, Q. Zhao, H. Liu, Using network distance analysis to predict lncRNA-miRNA interactions, Interdiscip. Sci.: Comput. Life Sci., 13 (2021), 535–545. http://doi.org/10.1007/s12539-021-00458-z doi: 10.1007/s12539-021-00458-z
![]() |
[37] |
T. Wang, J. Sun, Q. Zhao, Investigating cardiotoxicity related with hERG channel blockers using molecular fingerprints and graph attention mechanism, Comput. Biol. Med., 153 (2023), 106464. http://doi.org/10.1016/j.compbiomed.2022.106464 doi: 10.1016/j.compbiomed.2022.106464
![]() |
[38] |
F. Sun, J. Sun, Q. Zhao, A deep learning method for predicting metabolite-disease associations via graph neural network, Briefings Bioinf., 23 (2022). http://doi.org/10.1093/bib/bbac266 doi: 10.1093/bib/bbac266
![]() |
1. | Chiraag Kulkarni, Derek Liu, Touran Fardeen, Eliza Rose Dickson, Hyunsu Jang, Sidhartha R. Sinha, John Gubatan, Artificial intelligence and machine learning technologies in ulcerative colitis, 2024, 17, 1756-2848, 10.1177/17562848241272001 | |
2. | Petar Uchikov, Usman Khalid, Nikola Vankov, Maria Kraeva, Krasimir Kraev, Bozhidar Hristov, Milena Sandeva, Snezhanka Dragusheva, Dzhevdet Chakarov, Petko Petrov, Bistra Dobreva-Yatseva, Ivan Novakov, The Role of Artificial Intelligence in the Diagnosis and Treatment of Ulcerative Colitis, 2024, 14, 2075-4418, 1004, 10.3390/diagnostics14101004 | |
3. | Man Ni, Weilong Peng, Xiaoguang Wang, Jingui Li, Role of Aging in Ulcerative Colitis Pathogenesis: A Focus on ETS1 as a Promising Biomarker, 2025, Volume 18, 1178-7031, 1839, 10.2147/JIR.S504040 |