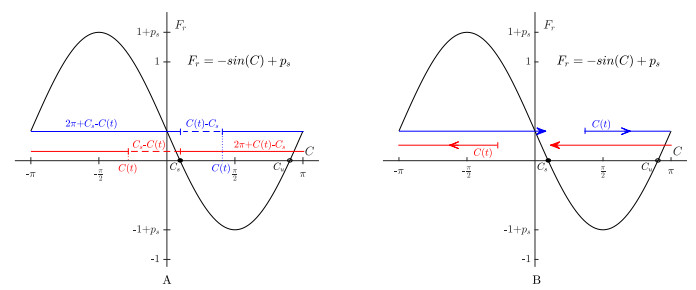
To comprehend the etiology and pathogenesis of many illnesses, it is essential to identify disease-associated microRNAs (miRNAs). However, there are a number of challenges with current computational approaches, such as the lack of "negative samples", that is, confirmed irrelevant miRNA-disease pairs, and the poor performance in terms of predicting miRNAs related with "isolated diseases", i.e. illnesses with no known associated miRNAs, which presents the need for novel computational methods. In this study, for the purpose of predicting the connection between disease and miRNA, an inductive matrix completion model was designed, referred to as IMC-MDA. In the model of IMC-MDA, for each miRNA-disease pair, the predicted marks are calculated by combining the known miRNA-disease connection with the integrated disease similarities and miRNA similarities. Based on LOOCV, IMC-MDA had an AUC of 0.8034, which shows better performance than previous methods. Furthermore, experiments have validated the prediction of disease-related miRNAs for three major human diseases: colon cancer, kidney cancer, and lung cancer.
Citation: Zejun Li, Yuxiang Zhang, Yuting Bai, Xiaohui Xie, Lijun Zeng. IMC-MDA: Prediction of miRNA-disease association based on induction matrix completion[J]. Mathematical Biosciences and Engineering, 2023, 20(6): 10659-10674. doi: 10.3934/mbe.2023471
[1] | Adelheid L. J. Thieme . Wanderings with Lady M.: A happy threesome. Mathematical Biosciences and Engineering, 2010, 7(1): iv-ix. doi: 10.3934/mbe.2010.7.1iv |
[2] | Mubashir Ahmad, Saira, Omar Alfandi, Asad Masood Khattak, Syed Furqan Qadri, Iftikhar Ahmed Saeed, Salabat Khan, Bashir Hayat, Arshad Ahmad . Facial expression recognition using lightweight deep learning modeling. Mathematical Biosciences and Engineering, 2023, 20(5): 8208-8225. doi: 10.3934/mbe.2023357 |
[3] | Leo Turner, Andrew Burbanks, Marianna Cerasuolo . PCa dynamics with neuroendocrine differentiation and distributed delay. Mathematical Biosciences and Engineering, 2021, 18(6): 8577-8602. doi: 10.3934/mbe.2021425 |
[4] | Jiayu Fu, Haiyan Wang, Risu Na, A Jisaihan, Zhixiong Wang, Yuko Ohno . A novel "five-in-one" comprehensive medical care framework for rehabilitation and nursing. Mathematical Biosciences and Engineering, 2023, 20(3): 5004-5023. doi: 10.3934/mbe.2023232 |
[5] | Bruno Buonomo, Deborah Lacitignola . On the stabilizing effect of cannibalism in stage-structured population models. Mathematical Biosciences and Engineering, 2006, 3(4): 717-731. doi: 10.3934/mbe.2006.3.717 |
[6] | Christopher M. Kribs-Zaleta . Sociological phenomena as multiple nonlinearities: MTBI's new metaphor for complex human interactions. Mathematical Biosciences and Engineering, 2013, 10(5&6): 1587-1607. doi: 10.3934/mbe.2013.10.1587 |
[7] | Martha Garlick, James Powell, David Eyre, Thomas Robbins . Mathematically modeling PCR: An asymptotic approximation with potential for optimization. Mathematical Biosciences and Engineering, 2010, 7(2): 363-384. doi: 10.3934/mbe.2010.7.363 |
[8] | Kai Zhang, Xinwei Wang, Hua Liu, Yunpeng Ji, Qiuwei Pan, Yumei Wei, Ming Ma . Mathematical analysis of a human papillomavirus transmission model with vaccination and screening. Mathematical Biosciences and Engineering, 2020, 17(5): 5449-5476. doi: 10.3934/mbe.2020294 |
[9] | Mario Grassi, Giuseppe Pontrelli, Luciano Teresi, Gabriele Grassi, Lorenzo Comel, Alessio Ferluga, Luigi Galasso . Novel design of drug delivery in stented arteries: A numerical comparative study. Mathematical Biosciences and Engineering, 2009, 6(3): 493-508. doi: 10.3934/mbe.2009.6.493 |
[10] | Jacques Demongeot, Pierre Magal . Population dynamics model for aging. Mathematical Biosciences and Engineering, 2023, 20(11): 19636-19660. doi: 10.3934/mbe.2023870 |
To comprehend the etiology and pathogenesis of many illnesses, it is essential to identify disease-associated microRNAs (miRNAs). However, there are a number of challenges with current computational approaches, such as the lack of "negative samples", that is, confirmed irrelevant miRNA-disease pairs, and the poor performance in terms of predicting miRNAs related with "isolated diseases", i.e. illnesses with no known associated miRNAs, which presents the need for novel computational methods. In this study, for the purpose of predicting the connection between disease and miRNA, an inductive matrix completion model was designed, referred to as IMC-MDA. In the model of IMC-MDA, for each miRNA-disease pair, the predicted marks are calculated by combining the known miRNA-disease connection with the integrated disease similarities and miRNA similarities. Based on LOOCV, IMC-MDA had an AUC of 0.8034, which shows better performance than previous methods. Furthermore, experiments have validated the prediction of disease-related miRNAs for three major human diseases: colon cancer, kidney cancer, and lung cancer.
Happiness has captured the attention and aspirations of humankind throughout history. The reason seems quite simple; nobody wants to suffer and everyone wants to be happy. Despite this common wish for happiness, and arguably due to its subjective nature, happiness remains a concept difficult to define, measure and predict. Influenced by ancient Greek wisdom, positive psychology recognizes happiness as a construct comprising hedonic and eudaimonic well-being dimensions [1,2,3]. The hedonic dimension, which has philosophical roots in Aristippus' notion of well-being, is generally characterized by the prevalence of positive emotions and pleasure [4], and the eudaimonic dimension, which has philosophical roots in Aristotle's notion of well-being, is characterized by the development of our full human potential, also referred to as flourishing [4,5].
While the components of these dimensions have been thoroughly studied (positive and negative emotions and life satisfaction, for example), experts in the field of well-being point out the need for theoretical models that offer a richer understanding of how the components of well-being interact, account for the dynamical nature of well-being, and contribute to the multimethod approach of the study of well-being [6].
Motivated by this need, we take a very first step towards expressing the dynamical interrelation of key components of well-being with a mathematical model.
Since the components of happiness are certainly dynamic (our emotions for example), it is clear that individual happiness also is dynamic and fluctuates over time. This is where mathematical modelling becomes relevant. A continuous dynamical mathematical model can help us understand the effects of the different determinants of happiness on its temporal dynamics, which in turn may provide guidelines for making meaningful life changes to better our well-being. This mathematical approach is illustrated in the pioneering dynamical model of happiness developed by Sprott [7], which describes the dynamics of a person's happiness in response to external events. While the model successfully captures some hedonic aspects of happiness, it does not account for the multidimensional nature of happiness and its derivation is, to a certain degree, arguable. First, the model's derivation is not based on any of the current views of well-being but simply on the assumption that the dynamics of an individual's happiness is equivalent to a like-dislike interaction among two individuals. Second, the model supports a view where human beings are seen as passive individuals subjected to external events, which according to positive psychology, is what led psychology in the past to become a "victimology" [5,8]. As a consequence, the model concludes that sustained happiness is unrealistic as it would require an ever-increasing set of positive events for an individual to experience lasting happiness.
In this work, we overcome such limitations and develop a more comprehensive mathematical model of happiness based on current positive psychology views of well-being where individuals are not seen as victims of external forces but as proactive and self-determined beings who can master their well-being and potentially achieve lasting happiness [5,8]. The formulation of the model is based on a set of theory-led assumptions which translate into a coherent definition of happiness and a description of its dynamics with a nonlinear system of ordinary differential equations.
The dynamics of the model offer insights into the role that emotions play in our happiness, the reasons why we struggle to attain sustainable happiness and why we are often left treading the hedonic treadmill and oscillating around a stable level of well-being in a hedonic homeostasis [3,9]. Moreover, the model stability analysis indicates that lasting happiness may be achievable by developing constant eudaimonic emotions or human altruistic qualities that overcome the boundaries of the hardwired homeostatic hedonic system; in mathematical terms, this process of overcoming the hedonic homeostasis is expressed as distinct dynamical bifurcations. This mathematical description is consistent with the idea that eudaimonic well-being is beyond the boundaries of hedonic homeostasis [10,11].
One innovative and plausible aspect of Sprott's dynamical model is that it defines happiness as a rate of change [7]; initially, the rate of change of the emotion love. Although this approach has not been derived from basic psychological principles and reducing happiness to love does not account for its complexities, linking happiness to a rate of change is - as we shall see - a meaningful proposition for the mathematical dynamical description of happiness.
Indeed, the idea of happiness being a rate of change, and in particular, the rate of change of satisfaction, is known to both Psychology and Philosophy. In the context of goal and discrepancy theory, Carver and Scheier [12] suggested that the rate of change of satisfaction, also referred to as the rate of change of progress towards a goal [5], rather than satisfaction itself, determines the experience of happiness. Here, satisfaction is understood to be determined by the discrepancy or gap between one's present perceived reality and what one aspires or thinks one deserves [13,14,15,16]. As the discrepancy decreases, the satisfaction increases.
Interestingly, this idea of happiness being the rate of change of satisfaction also has philosophical roots dating back to the nineteenth century. In particular, Schopenhauer [17] described human happiness not as satisfaction per se but as the quick transition from wish and will striving to satisfaction and from satisfaction to a new wish; in other words, happiness is described as the rate of change of satisfaction.
Perhaps the greatest challenge in developing a dynamical model for happiness is the need of clearly defining happiness. Thus, for this purpose and using the idea of happiness as the rate of change of satisfaction, we would like to describe mathematically the level of an individual's happiness, H, as
H(t)=dC(t)dt, | (2.1) |
for a variable C (still to be determined) that could reflect the levels of an individual's satisfaction or contentment.
The exact definition of the variable C and its range of values will become apparent as we develop the mathematical model to describe an individual's happiness over time. In order to build the model we need to identify the main factors or 'forces' causing changes in the levels of happiness. From a positive psychology perspective, emotions play a crucial role in an individual's well-being. In particular, positive emotions increase levels of well-being while negative ones decrease levels of well-being [18,19,20]. Thus, both positive and negative emotions are driving 'forces' on the levels of an individual's happiness, and if F(t) denotes the intensity of emotions being experienced (affect), it is reasonable to assume that
dH(t)dt=F(t), | (2.2) |
Note that this basic model for the dynamics of happiness implies that if F(t)>0 (the emotion being experienced is positive), then dH(t)dt>0 and the levels of happiness increase. Analogously, if F(t)<0 (the emotion being experienced is negative), then dH(t)dt<0 and the levels of happiness decrease. This is consistent with the positive psychology view that positive/negative emotions increase/decrease the levels of well-being.
In order to unfold the structure of F(t), it is necessary to consider not only our human emotional inputs but also other well known mechanisms acting on these emotional inputs. In particular, using information from adaptation, discrepancy, and goal-oriented theories, we will see in the next sections that
F(t)=Fe+Fd+Fr, | (2.3) |
where Fe, Fd, and Fr denote the emotional force, the hedonic damping, and the homeostatic restoring force, respectively.
Although there seems to be a clear consensus that positive emotions increase levels of well-being and that negative ones decrease them, the fact that there are different classification systems and measurement tools for positive and negative emotions [21,22,23] can pose a challenge not only for defining what a positive or negative emotion is but also for determining the relative intensity of positive and negative emotions. For this reason, and for the sake of developing a simple and understandable model for happiness, we will define the emotional force Fe(t) as the overall intensity of an individual's emotions that arise at time t.
If the overall intensity of emotions at a particular time is positive, i.e., Fe(t)>0, we will refer to it as a positive emotional force or input, and if the overall intensity of emotions at a particular time is negative, i.e., Fe(t)<0, we will refer to it as a negative emotional force or input. Thus, from Eq (2.2), we notice that positive emotional inputs have the potential to increase a person's happiness and negative ones the potential to decrease it. However, we shall see that the impact of these emotional inputs on the actual experience, F(t), of these emotions is affected by intrinsic human mechanisms of adaptation and self-preservation.
One of the mechanisms involved in reducing the impact of emotional inputs is known as hedonic adaptation [24,25]. This psychological process allows human beings to adapt to both favorable and unfavorable circumstances and appears to reduce the affective impact of emotional inputs. For example, when facing favorable circumstances that elicit positive emotions, which in turn can cause an increase in the levels of happiness, the hedonic adaptation mechanism will reduce this effect and try to prevent intense emotions causing euphoric states. Analogously, when unfavorable circumstances elicit negative emotions, which can cause a decrease in the levels of happiness, the hedonic adaptation mechanism will reduce this effect and try to prevent intense negative emotions causing depressive states. Therefore, it is reasonable to describe the hedonic adaptation mechanism with the following hedonic damping term, Fd, that reduces the impact of emotional inputs on a person's levels of happiness:
Fd=−βdCdt=−βH, | (2.4) |
where β>0 denotes the hedonic damping.
Another intrinsic psychological mechanism to be considered in the model is the subjective well-being or hedonic homeostatic system that attempts to keep us with a positive view of ourselves by trying to bring us to an equilibrium set-point of satisfaction [16,26]. In order to describe this homeostatic system mathematically, we will consider some aspects of discrepancy and goal-oriented theories. In particular, we will assume that the function of emotions is to help us progress towards a goal [22,27]; the closer to the goal, the higher the satisfaction, i.e., the lesser the distance between our perceived reality and where we would like to be [12]. In the context of discrepancy and goal-oriented theories, we are continuously assessing our level of satisfaction. In the presence of discrepancy we adjust our emotional response in the hope of getting closer to the goal and, in consequence, feeling satisfied [12].
Under these assumptions, if the set-point level of satisfaction (which helps us keep a positive view of the self) is perturbed, the hedonic homeostatic system will try to restore our stable level of satisfaction. Thus, any emotional effort towards a goal will be affected by this restoring homeostatic system.
Moreover, one basic notion that characterizes the hedonic homeostatic system is that goal achievement is analogous or equivalent to a return to equilibrium [9]. Thus, two scenarios are possible when an emotional input intends to move us towards a goal and the set-point of satisfaction is perturbed, namely, the goal might not be achieved and the homeostatic system will bring us back to the equilibrium set-point of satisfaction, or the goal is achieved and we also return to the equilibrium set-point of satisfaction. Thus, the homeostatic system has a cyclic or periodic aspect to it with respect to the levels of satisfaction. As we would like to capture this cyclic behaviour with a simple periodic function, we describe the full mechanism of the hedonic homeostatic system with the following homeostatic restoring force
Fr=−sin(C)+ps, | (2.5) |
where C∈(−π,π], as we will see shortly, is a state variable that describes indirectly the level of satisfaction, and the parameter ps, where −1<ps<1, will determine the stable or set-point level of satisfaction.
To appreciate the mathematical description of this homeostatic restoring force, illustrated in Figure 1, we summarize its significant features and implications as follows:
1) Given the periodic nature of the of the hedonic homeostatic system with respect to the levels of satisfaction, we restrict the domain of the homeostatic restoring force, Fr, to (−π,π], where C=−π is identified with C=π.
2) There are two C-intercepts of Fr=−sin(C)+ps; one closer to C=0, denoted as Cs, and one further away, denoted as Cu. In the following sections, we will see that Cs represents a stable level of satisfaction, whereas Cu is an unstable steady state.
3) Being away from the equilibrium Cs at a particular time t places people in a position of dissatisfaction or discontentment, and they might aspire to a new state of satisfaction. For example, if Cs<C(t)<Cu for a particular time t, the distance or discrepancy to a new state of satisfaction could be given by 2π+Cs−C(t); however, a person might not aspire to that new state of satisfaction, in which case the discrepancy could be C(t)−Cs (see Figure 1A). In general, if we represent each of these two different choices (aspiring or not aspiring to a new state of satisfaction) with m∈{0,1}, the discrepancy D(t) at a particular time is determined by how far a person is to the equilibrium set-point of satisfaction Cs in the following way:
Case ⅰ. When Cs≤C(t)≤π,
D(t)=2πm−(−1)m(Cs−C(t)). | (2.6) |
This case is illustrated with the blue lines in Figure 1A.
Case ⅱ. When −π<C(t)<Cs,
D(t)=2πm+(−1)m(Cs−C(t)). | (2.7) |
This case is illustrated with the red lines in Figure 1A.
Note that D(t)∈[0,2π]; the closer D(t) is to 0 the more satisfaction there is, and the closer D(t) is to 2π, the more dissatisfaction there is.
4) The magnitude of the restoring homeostatic force describes the adjustment needed in our emotional response to progress towards a new state of satisfaction or goal.
5) The progress towards a state of satisfaction reduces the discrepancy D(t) and can be either adequate or inadequate [12]. In the first case, dCdt=H>0, and in the second case, dCdt=H<0 (Figure 1B). Note that in both cases, the discrepancy is reduced, i.e. dDdt<0. For this reason, the definition of happiness is given by the rate of change of the variable C and not by the rate of change of discrepancy reduction. The following next two points describe an adequate progress on the domain of the homeostatic force (an analogous description can be made for an inadequate progress).
6) The range of values in the interval (Cs,Cu), determined by the zeros of Fr, represent values of positive dissatisfaction. Within this interval, positive levels of emotional inputs are required to progress towards a new state of satisfaction. The range of values (0,1−ps) of Fg determines the intensity of the homeostatic restoring system that needs to be overcome with a positive emotional input. The highest level of the homeostatic restoring force is encountered at C=π/2; after this value, the levels of emotional inputs required to progress towards a new state of satisfaction, decrease.
7) For values of C above Cu, i.e., within the range (Cu,π]∪(−π,Cs), no more emotional effort is required to progress towards the new state of satisfaction, as the restoring force will take C there. For this reason, we can refer to (Cu,π]∪(−π,Cs) as a range of high certainty (that the new state of satisfaction will be achieved), and to (Cs,Cu) as a range of low certainty. The behaviour within these ranges is consistent with the psychological view that contentment arises under conditions that include high certainty and low effort [28]. Note that this ideal dynamics, along the blue trajectory in Figure 1B, describes an adequate progress towards a goal, is obtained through a positive emotional force, and results in the experience of happiness (dCdt=H>0).
8) Analogously, we could describe an inadequate progress towards a new state of satisfaction or goal. This dynamics is represented with the red trajectory in Figure 1B.
9) If Fe(t)≥1−ps for a particular time t, we can think of the positive emotion at that time as being eudaimonic or flourishing in nature as it tries to overcome the homeostatic system in a positive way. This mathematical interpretation of an eudaimonic emotion is consistent with the positive psychology view that eudaimonic feelings overcome the limits of hedonic homeostasis [11]. Similarly, if Fe(t)≤−1−ps for a particular time t, we can think of the negative emotion as being destructive in nature as it tries to overcome the homeostatic system in a negative way.
10) The parameter ps can be interpreted as a genetic parameter determining the stable set-point level of satisfaction Cs. Note that the higher the value of this set-point related parameter ps, the wider the range of high certainty; or equivalently, the narrower the range of low certainty. In other words, an individual with a high value of ps has a homeostatic goal-oriented mechanism that provides favorable conditions for an adequate progress towards a goal.
Having unfolded the structure of the intensity of the affect F(t), we substitute its components given by the emotional force, Fe, the hedonic damping, Fd, and the homeostatic restoring force, Fr, into equation Eq (2.2), to obtain
dHdt=−βdC(t)dt−sin(C)+ps+Fe(t). | (2.8) |
Taking into account Eq (2.1), we can rewrite this last expression as the following second-order differential equation describing the dynamics of happiness,
d2Cdt2+βdCdt+sin(C)=ps+Fe(t), | (2.9) |
where C∈(−π,π] is a state variable that describes indirectly the level of satisfaction, H=dCdt denotes the level of happiness, β>0 is the hedonic damping coefficient, −1<ps<1 is the well-being set-point related parameter, and Fe(t) is the emotional input. Interestingly, this equation, which describes the dynamics of happiness, is the same differential equation used to describe the angular position of a damped pendulum forced with a torque.
Notice that since our interest is to describe the dynamics of happiness, H=dCdt, it is reasonable to express the second-order differential equation given by Eq (2.9) as the following system of first-order differential equations
[CH]′=[010−β][CH]+[0−sin(C)+ps]+[0Fe(t)], | (2.10) |
where ′ denotes the derivative with respect to t, and (C,H)∈(−π,π]×(−∞,+∞). In other words, the dynamics of happiness can be described on the surface of a cylinder (a cylindrical phase space) where the angular position describes indirectly the level of satisfaction and the height describes the level of happiness.
In the following sections, we will explore the effect of simple emotional inputs and different parameter values for β and ps on the dynamics of happiness. In particular, we will show how emotional impulses and increasing-decreasing positive emotional inputs can lead to a treadmill dynamics of happiness, and investigate how the development of constant eudaimonic or flourishing emotions can lead to lasting happiness. For the purpose of illustrating the results, all the solutions of the happiness model in the following sections were obtained numerically using the ode solver ode45 in Matlab [29], which implements a Runge-Kutta method for the computations.
In order to understand the effect of an emotional impulse, we first analyze the basic dynamics of the model in the absence of emotional inputs (i.e., Fe(t)=0) by carrying out a linear stability analysis.
Assuming Fe(t)=0 and, for the sake of simplicity, a well-being set-point related parameter ps=0, the proposed model for the dynamics of happiness given by Eq (2.10) becomes
[CH]′=[010−β][CH]+[0−sin(C)], | (3.1) |
By equating the vector field to zero we find that the fixed points (C∗,H∗) of the system are given by (Cs,0)=(0,0) and (Cu,0)=(π,0). Notice that these fixed points are determined by the zeros of the homeostatic restoring force. Moreover, by calculating the eigenvalues of the Jacobian matrix of the vector field, it is easy to verify that (Cs,0)=(0,0) is a spiral sink for 0<β<2 and stable node for β≥2, and that (Cu,0)=(π,0) is a saddle point. The phase portraits of these two cases are illustrated, respectively, in Figure 2A, B.
The importance of establishing first the dynamics of the happiness model with no emotional input, given by Eq (3.1), stems from the fact that if we describe an emotional impulse as a dirac delta function Fe(t−t0)=kδ(t−t0), where k and t0 are the intensity and the time of the emotional impulse, it can be proved, using Laplace transforms, that solving the following system with an impulsive emotional input for t>t0,
[CH]′=[010−β][CH]+[0−sin(C)+ps]+[0kδ(t−t0)];[C(t)H(t)]=[00],t<t0, | (3.2) |
is equivalent to solving the system with no emotional input
[CH]′=[010−β][CH]+[0−sin(C)+ps];[C(t0)H(t0)]=[0k], | (3.3) |
for t>t0. Therefore, an emotional impulse causes a translation (equivalent to the intensity of the impulse) in the happiness state variable of Eq (3.3) at the moment of the impulse. Figure 3A, B illustrates how positive and negative emotional impulses perturb the stable steady state of the system causing a sudden increase or decrease in the level of happiness, which then returns to its stable state.
Also, Figure 3C shows that, if the intensity of the emotional impulse is high enough, it can temporarily overcome the restoring homeostatic system, resulting in the solution going around the cylindrical phase portrait before returning to the stable steady state.
Note that if our emotions comprise a series of positive and negative impulses, we will be permanently subject to tread on the hedonic treadmill with ups and downs in our levels of happiness. Moreover, emotional impulses describe particular emotional inputs that might not necessarily be the most efficient way to progress towards a new state of satisfaction or to experience happiness.
In this section, we will describe how an increasing-decreasing positive emotion can overcome the boundaries of the well-being homeostatic system temporarily, help us progress positively towards a new state of satisfaction, and increase the time spent in a state of happiness. In particular, we consider an emotional input of the form Fe(t)=kG(t), where
G(t)={0,0≤t<ti,(t−ti)/(tm−ti),ti≤t≤tm,(t−tf)/(tm−tf),tm<t≤tf,0,t>tf, | (3.4) |
ti is the time when the positive emotion starts increasing, tm is the time when the maximum value k of the emotion is reached and starts to decrease, and tf is the time when the positive emotion is no longer present.
This profile for Fe describes fairly reasonably how positive emotions can be regulated for the purpose of reaching a new state of satisfaction. This is illustrated through an example in Figure 4.
In this example, after resting at a steady state a person has an initial desire to reach a new state of satisfaction; we could say that this person is positively dissatisfied. At this moment (time ti=2 in Figure 4A), a positive emotion arises and increases in an effort to overcome the restoring homeostatic force. Notice that the positive emotion reaches its maximum value at time tm=12, and that C(tm)>π/2 (Figure 4B); therefore, the positive emotion has already overcome the highest level of the homeostatic restoring force (reached when C=π/2; see Figure 1). For this reason, the emotional response is adjusted and starts to decrease until tf=17. At this time, the effort of an emotional positive input is not needed any longer as −π<C(tf)<0 (Figure 4B) and the positive homeostatic force will take the person to the new state of satisfaction (Figure 1), represented, in this case, by the stable steady state (C∗,H∗)=(0,0).
During this positive progression towards a new state of satisfaction, the levels of happiness are positive (Figure 4C) and the discrepancy, described by Eq (2.6) and Eq (2.7), decreases (Figure 4D), which in turn results in an increasing satisfaction. Given the transient, piecewise linear nature of the emotional input in this case, the model was solved numerically first for the increasing part of the emotional input, which then allowed us to have a new initial condition for solving the model with the decreasing part of the emotional input, which in turn provided a new initial condition to solve the model with no emotional input.
The full dynamics of the happiness model on the cylindrical phase space is described in Figure 4E. Note that the emotional input, interpreted as a response of the homeostatic restoring force, allowed the solution to go around the cylindrical phase space. This is not the case if the positive emotion starts to decrease before the maximum value of the homeostatic restoring is reached (let's say, for example, tm=10<12); in other words, if the increasing positive emotional effort does not last long enough to fully overcome the homeostatic restoring force. This results in an unsuccessful progress towards a new state of satisfaction and the trajectory of the solution of the happiness model will not go completely around the cylindrical phase space (Figure 4F).
We can see how the well-being homeostatic system plays a double role in the dynamics of happiness. On the one hand, it tries to keep us content and with a positive view of ourselves by bringing us to an equilibrium set-point of satisfaction. On the other hand, it challenges us to overcome its restoring force in order to achieve a goal or new state of satisfaction. Therefore, the homeostatic system has a survival function and also a human development function. By embracing the latter, we experience temporary moments when the homeostatic system is broken; in other words, moments that allow us to flourish and experience longer periods of happiness and eudaimonia.
If we assume that emotions are composed not only of discrete moments of positive and negative impulses, but also of successful and unsuccessful attempts to adequately or inadequately progress towards new goals or states of satisfaction, then our happiness level (driven mainly by these emotions) will be subject to highs and lows (as shown in this and the previous section) and our well-being experience could be described by a walk on the hedonic treadmill with moments of failures and moments of flourishing.
Nonetheless, the dynamics of the model suggests that we can go beyond the hedonic treadmill, and overcome the well-being homeostatic system. The following section is devoted to further explore this dynamical behaviour by studying the effect that a constant positive emotional input can have on our experience of happiness.
In the previous sections, we observed how the proposed model highlights the important role that emotions play in the dynamics of the happiness and the satisfaction an individual experiences. In particular, it demonstrates how emotional impulses and increasing-decreasing emotions that temporarily overcome the well-being homeostatic system can leave us treading the hedonic treadmill around positive and negative levels of happiness. Considering these dynamics, the question that remains to be answered is whether lasting happiness is possible, or in other words, whether it is possible to overcome the homeostatic system permanently. In order to answer this question, our focus in this section will be on the effect that a constant positive emotional input can have in the dynamics of happiness, i.e., we will consider an emotional input in Eq (2.10) of the form
Fe(t)=q, | (3.5) |
where q>0.
Note that a constant positive emotional input directly and constantly affects the restoring homeostatic force described by Eq (2.5). This becomes evident when we substitute the constant emotional input, given by Eq (3.5), into the happiness model described by Eq (2.10), which now becomes
[CH]′=[010−β][CH]+[0−sin(C)+ps+q], | (3.6) |
Notice that the restoring force, Fr, is affected by the constant emotional input q, and the C component of the steady states of the happiness model given by Eq (3.6) is determined by the solution of
Fr+Fe=−sin(C)+p=0, | (3.7) |
where p=ps+q.
Thus, we can interpret the development of a constant positive emotional input as a way of shifting up the parameter ps and bringing up the homeostatic restoring force illustrated in Eq 2.5. In fact, it is apparent that if a constant positive emotion is high enough such that p>1 then the homeostatic system can be permanently overcome. For the purpose of understanding how the overcoming of the hedonic homeostatic system leads to lasting happiness, we start by carrying out a bifurcation analysis at p=1.
Keeping in mind Eq (3.7), we note that there are two steady states, (C1,0) and (C2,0), of the happiness model that come closer together as p increases and approaches 1. When p=1, there is a single equilibrium at (π/2,0), which then disappears when p>1. Moreover, as the Jacobian matrix of the vector field of Eq (3.6), given by
J=[01−cos(C)−β], | (3.8) |
has a characteristic polynomial
p(λ)=λ2+βλ+cos(C), | (3.9) |
with roots
λ1,2=(−β±√β2−4cos(C))/2, | (3.10) |
we conclude that when p approaches 1 from below the steady state (C1,0), where C1>π/2, is a saddle since λ1<0<λ2, and the steady state (C2,0), where C2<π/2, is a stable node since λ1,λ2<0.
This suggests that the homeostatic system can be broken through a saddle-node bifurcation as p becomes greater than 1. Specifically, the steady state (π/2,0) undergoes a saddle-node bifurcation at the parameter value p=1. In order to offer a rigorous proof of this bifurcation, we will show that the (ⅰ) singularity, (ⅱ) nondegeneracy and (ⅲ) transversality conditions for a saddle-node bifurcation to occur are met. These conditions are the basic assumptions of the saddle-note Theorem 8.6 and the corresponding Corollary 8.7 stated by Meiss [30].
(ⅰ) Singularity:
To verify the singularity condition, we first introduce the following translation variables and new parameter, respectively
z1=C−π/2,z2=H,μ=p−1. | (3.11) |
Therefore, Eq (3.6) is transformed into the system
z′1=z2=f1(z1,z2;μ),z′2=−βz2−cos(z1)+1+μ=f2(z1,z2;μ), | (3.12) |
with a Jacobian matrix for f(z1,z2;μ)=(f1(z1,z2;μ),f2(z1,z2;μ)) evaluated at (z1,z2;μ)=(0,0;0) given by
Jf(0,0;0)=[010−β]. | (3.13) |
Thus, f(z1,z2;μ) satisfies the singularity conditions
f(0,0;0)=(0,0),spec(Jf(0,0;0))={0,−β;β≠0}. | (3.14) |
(ⅱ) Nondegeneracy and (ⅲ) Transversality:
Note that the system in Eq (3.12) can be rewritten in its matrix form as
[z1z2]′=[010−β][z1z2]+[0−cos(z1)+1+μ]=Jf(0,0;0)[z1z2]+[0−cos(z1)+1+μ]. | (3.15) |
To verify the nondegeneracy and transversality conditions, we need to transform Eq (3.15) into its normal form. For this, we need to transform the Jacobian matrix of f at the origin, Jf(0,0;0), into Jordan normal form, JN, by finding the nonsingular matrix P of eigenvectors of Jf(0,0;0), such that
JN=P−1Jf(0,0;0)P. | (3.16) |
Note that the eigenvectors corresponding to the eigenvalues 0 and −β of Jf(0,0;0) are given by (1,0)T and (−1,β)T, respectively. Thus,
P=[1−10β],P−1=[11/β01/β], | (3.17) |
and
JN=P−1Jf(0,0;0)P=[000−β]. | (3.18) |
Consider now the transformation of variables
[z1z2]=P[x1x2]=[1−10β][x1x2]=[x1−x2βx2], | (3.19) |
and its derivatives
P[x1x2]′=[z1z2]′. | (3.20) |
Substituting Eq (3.15) into Eq (3.20), multiplying both sides of the resulting equation from the left by P−1, and using Eq (3.19), we obtain the normal form of equation Eq (3.15)
[x1x2]′=P−1Jf(0,0;0)P[x1x2]+P−1[0−cos(x1−x2)+1+μ], | (3.21) |
which can be rewritten, using Eq (3.17) and Eq (3.18), as
[x1x2]′=[000−β][x1x2]+[g1(x1,x2;μ)g2(x1,x2;μ)], | (3.22) |
where
g1(x1,x2;μ)=g2(x1,x2;μ)=−cos(x1−x2)/β+1/β+μ/β, | (3.23) |
g(0,0;0)=(g1(0,0;0),g2(0,0;0))=(0,0), | (3.24) |
and a Jacobian matrix of g(x1,x2;μ)=(g1(x1,x2;μ),g2(x1,x2;μ)) evaluated at (x1,x2;μ)=(0,0;0)
Jg(0,0;0)=[0000]. | (3.25) |
Having the normal form in Eq (3.22) allows to show that the nondegeneracy condition
∂2∂x12g1(0,0;0)=1β≠0, | (3.26) |
and the transversality condition
∂∂μg1(0,0;0)=1β≠0, | (3.27) |
in the saddle-node Theorem 8.6 and corresponding Corollary 8.7 in [30] are satisfied. Thus, we conclude that the steady state (π/2,0) of the happiness model given by Eq (3.6) undergoes a saddle-node bifurcation at the parameter value p=1. Also, if the constant emotional input is sufficiently high such that p>1, there are no equilibrium points and the hedonic homeostatic system is permanently overcome. We will show that this leads to a stable state of lasting happiness described by a limit cycle.
In order to prove the existence of a stable and lasting state of happiness when a sufficiently high constant emotional input has been developed, we will prove the existence, uniqueness and global stability of a periodic orbit with positive values of happiness around the cylindrical phase space when p>1.
Note that H′<0 when H>(p+1)/β and H′>0 when H<(p−1)/β. Then, if we choose constants
H∗1<(p−1)/β,andH∗2>(p+1)/β, | (3.28) |
all trajectories in the region H∗1<H<H∗2 must, by Poincaré-Bendixson on the cylinder, stay in the region and approach a periodic orbit as there are no fixed points when p>1. This means that the periodic orbit, indeed, exists. Moreover, this periodic orbit cannot be a libration (a periodic orbit that does not circuit around the cylindrical phase space) as if it were, it should encircle a fixed point, but again, there are no fixed points when p>1. Therefore, the existing limit cycle is a rotation with values of H>0.
To prove that this limit cycle is unique, we first need to show that the following equality holds for any rotation H(C),
∫π−πH(C)dC=2πpβ. | (3.29) |
On the one hand, using Eq (3.6), we obtain that
∫π−πdHdtdC=∫π−π(−βH−sin(C)+p)dC=−β∫π−πHdC+2πp. | (3.30) |
On the other hand, keeping in mind that the rotation is periodic with period denoted as T, we can also compute the previous integral as
∫π−πdHdtdC=∫T0dHdtdCdtdt=∫T0dHdtHdt=−∫T0HdHdtdt, | (3.31) |
which implies that
∫π−πdHdtdC=0. | (3.32) |
Note that the substitution of Eq (3.32) into Eq (3.30), results in Eq (3.29).
Now, let us assume that there are two different rotations H1(C) and H2(C). As trajectories cannot cross, we can also assume, without loss of generality, that H2(C)>H1(C) for all values of −π<C≤π. Thus,
∫π−πH2(C)dC>∫π−πH1(C)dC, | (3.33) |
which contradicts Eq (3.29), and consequently, we conclude that the limit cycle for p>1 is unique.
To prove that this limit cycle is globally asymptotically stable, note that H∗1 and H∗2 in Eq (3.28) can have values arbitrarily negative and positive, respectively, Therefore, using again Poincaré-Bendixson on the cylinder and the uniqueness of the limit cycle, we conclude that all the trajectories of the happiness model approach the limit cycle asymptotically.
Thus, the globally attracting limit cycle with H>0 that exists when p>1 describes a state of lasting happiness that can be achieved through the development of a constant positive emotion that overcomes the hedonic homeostatic system.
It is reasonable to think that the development of a constant positive emotion capable of overcoming the hedonic homeostatic system, as illustrated in Figure 5A, can occur gradually until p>1. In this section, we shall see that this gradual development, which could be described by different values of a constant positive emotion (and therefore, different values of p>0), can go through two distinctive paths leading to the state of lasting happiness explained in the previous section, namely, a path that goes through a homoclinic bifurcation before encountering the saddle-node bifurcation at p=1 and another path that does not go through any bifurcation before encountering the saddle-node bifurcation at p=1.
Based on the bifurcation analysis carried out above, it is logical to consider a region in the βp-parameter space, determined by p=1, where the saddle-node bifurcation takes place, as shown in Figure 5B. Within this region, the dynamics of the happiness model with a constant emotional input, given by Eq (3.6), can be unfolded analogously to how the dynamics of the Josephson junction and the damped pendulum with a constant torque are unfolded [31,32,33,34]. This dynamics, which will be illustrated shortly, is described by the two dimensional bifurcation diagram in Figure 5C, which shows the curves where homoclinic, saddle-node and saddle-node on the invariant circle bifurcations occur in the βp-parameter space. Given the relevance of the happiness model in offering a resulting dynamics describing an achievable state of lasting happiness and the importance of the saddle-node bifurcation experienced at p=1 in identifying the threshold of the constant emotional input necessary to achieve this state of lasting happiness, we focus the rigorous mathematical analysis on these significant aspects. In particular, we carried out, in the previous sections, an in-depth saddle-node bifurcation analysis at p=1 and also a meticulous global stability analysis for the attracting limit cycle that exists for p>1. The rigorous study of the homoclinic bifurcation would be lengthy and would deviate from the main narrative and scope of this manuscript. For this reason, we refer the reader interested in the thorough details of such bifurcation to Levi and Hoppensteadt [31] and Guckenheimer and Holmes [32].
The homoclinic bifurcation curve in Figure 5C was generated numerically by carrying out simulations of the happiness model in Eq (3.6) for starting values of β and p close to zero and close to the line p=4β/π to spot the homoclinic orbits. The reason for using this line is that Guckenheimer and Holmes [32] derived a analytical approximation for the homoclinic bifurcation curve for values of β close to zero and showed that this approximation is tangent to the line p=4β/π as β approaches zero. Thus, after graphing the homoclinic bifurcation curve in Figure 5C for the initial values of β and p, we continued generating it by tracking the homoclinic orbit of the happiness model within increments of length 0.05 for β until the homoclinic bifurcation curve touched p=1.
In order to illustrate the dynamics of the happiness model with a constant emotional input, shown in the βp-bifurcation diagram, we consider two fixed values for β (β=0.5 and β=1.5) and let p vary across the homoclinic, the saddle-node and the saddle-node on the invariant circle bifurcation curves. The particular values of p, shown in Figure 5B, were carefully chosen after having generated Figure 5C, so that (ⅰ) p1, p2, and p3 lie, respectively, below, over, and above, the homoclinic bifurcation curve, (ⅱ) p4, p5, and p6 lie, respectively, below, over, and above, the saddle-node bifurcation curve given by p=1 with a pairing value of β such that p4 falls in a bistability region, and (ⅲ) p7, p8, and p9 lie, respectively, below, over, and above, the saddle-node on the invariant circle bifurcation curve given by p=1, with a pairing value of β such that p7 does not fall in a bistabilty region.
Homoclinic path towards lasting happiness.
Using β=0.5 and letting p vary across the homoclinic bifurcation curve with values of p1=0.5, p2=0.6, and p3=0.7, we can see in Figure 5D–F, how the dynamics of the happiness model varies accordingly to these parameter values.
Figure 5D, obtained when p=p1, describes the dynamics of the model before the homoclinic bifurcation occurs, and therefore shows the existence of a stable fixed point and an unstable one (a saddle). In this case, all the trajectories, except the ones starting at the saddle point or its stable manifold, will go to the stable fixed point. This same dynamics is shared when the choice of parameter values falls in the 'stable fixed point' region in Figure 5C.
Figure 5E, obtained when p=p2, describes the dynamics of the model when the homoclinic bifurcation occurs, and therefore shows the existence of a homoclinic curve that arises when one of the branches of the unstable manifold of the saddle point joins one of the branches its stable manifold. This dynamical behaviour is shared when the choice of parameter values lies on the homoclinic bifurcation curve in Figure 5C.
As p increases, for example when p=p3, a stable limit cycle arises from the homoclinic bifurcation, as shown in Figure 5F, creating a bistable dynamics, where some of the trajectories will be attracted to the limit cycle and others to the stable fixed point. This dynamics occurs when the choice of parameter values falls on the 'bistability' region in Figure 5C.
Notice, on the one hand, that if the constant positive emotional input Fe(t)=q is not high enough to make p=ps+q greater than the p-values given by the homoclinic bifurcation curve, then the dynamics of happiness will be determined by the stable steady state and will be subject to the well-being homeostatic system just as in the previous sections. In other words, if this constant positive emotional input is accompanied by a series of positive and negative impulses that perturb the stable steady state, and by successful and unsuccessful attempts to achieve new states of satisfaction, a person will be left treading the hedonic treadmill with ups and downs in the levels of happiness. However, it is important to mention that even though the qualitative dynamics in this case does not differ to the one described in the previous sections, the development of a constant positive emotion will always bring the worthy benefit of lifting up the homeostatic restoring force, which puts an individual in a better position to adequately progress towards new goals or states of satisfaction.
On the other hand, if the constant positive emotional input Fe(t)=q is high enough and p=ps+q<1 becomes greater than the p-values given by the homoclinic bifurcation curve, the bistability dynamics will allow for the possibility of experiencing a 'taste' of lasting happiness even though the well-being homeostatic system is not fully broken. This is represented by trajectories starting at a certain region of the cylindrical phase space that are attracted to the stable limit cycle, which in turn oscillates with positive values of happiness H (Figure 5F). We call this experience 'shakable lasting happiness' as a negative emotional input can bring a person to points on the cylindrical phase space leading to trajectories that are attracted to the stable steady state.
We refer to this possible progression in the development of a constant positive emotional input passing through the homoclinic bifurcation curve as the homoclinic path towards lasting happiness.
Saddle-node path towards lasting happiness.
In order to follow the dynamics after the homoclinic bifurcation, we keep β=0.5 and let p increase across p=1 with values p4=0.99, p5=1, and p6=1.1. Figure 6A–C show how the dynamics of the happiness model varies according to these parameter values. Note that the choice p=p4 falls in the 'bistability' region, and the model dynamics, described by Figure 6A, shares the same bistable dynamical feature as the previous case (i.e., p=p3) but with the steady states closer together and the stable fixed point becoming a stable node.
When p reaches the SN (saddle-node) bifurcation value p5=1, the stable node and the saddle collide into a unique unstable fixed point (Figure 6B), which then disappears as p reaches values greater than one, for example, p=p6 (Figure 6B), while the stable limit cycle, which came originally from the homoclinic bifurcation, persists.
A similar, but mathematically slightly different, behaviour occurs when the value of the hedonic damping coefficient β is too high (for example, β=1.5) for the model dynamics to undergo a homoclinic bifurcation. In this case, the only path to lasting happiness is through p crossing the SNIC (saddle-node on the invariant circle) bifurcation value. This is described in Figure 6D–F as p crosses the saddle-node bifurcation curve with values of p7=0.95, p8=1, and p9=1.1. In this case, a limit cycle did not exist before the saddle-node bifurcation occurred, but since this bifurcation takes place on the invariant circle formed by the two branches of the unstable manifold of the saddle with the stable steady state (Figure 6D, E), a stable limit cycle arises as p becomes greater than one (Figure 6F).
Thus, for both these saddle-node bifurcation cases (SN and SNIC), if a person develops a constant emotional input q>0, such that p=ps+q>1, there will be a stable limit cycle oscillating with positive values of happiness H attracting all the trajectories of the model, as proved in Section 3.3.1. In other words, the person fully overcomes the well-being homeostatic system and guarantees the experience of lasting happiness.
Low and high hedonic damping progression towards lasting happiness.
In order to further describe the path towards lasting happiness through the development of a constant positive emotional input, we graph the one-dimensional bifurcation diagrams with respect to p when a person is characterized with low and high hedonic damping coefficients.
Figure 7A, B shows the bifurcation diagrams with respect to the parameter p for the state variables H and C, respectively, when a person is characterized with a low β=0.5, which can be interpreted as a value characterizing a person with high sensitivity to emotional inputs. The presence of a bistability region as p passes the value p=0.6 becomes evident in Figure 7A, which shows how a stable limit cycle (with maximum and minimum values described by the red and blue curves in the figure) appears after the homoclinic bifurcation. This limit cycle persists beyond the occurrence of the SN bifurcation at p=1 (shown in Figure 7B for the phase variable C), which guarantees the experience of lasting happiness.
An interesting phenomenon indicated by the arrows in the bifurcation diagram of Figure 7A is that of hysteresis. If the value of p and H are initially small, then as p increases, H will continue to be attracted to the fixed point (H=0) even after passing the homoclinic bifurcation value p=0.6. When p exceeds the saddle-node bifurcation value p=1, the happiness H will jump up into a positive state given by the limit cycle. If at this state p is brought back down below p=1, the happiness H will not jump back down or be attracted to the fixed point, instead it will be attracted to the stable limit cycle that persisted for values less than 1. The H values of the limit cycle will tend to zero as p decreases towards the homoclinic bifurcation value p=0.6. Thus, if a person develops a positive constant emotional input that makes p>1 and overcomes the well-being homeostatic system, then the hysteretic phenomenon allows the person to relax the value of p below the saddle-node bifurcation value, regain the well-being homeostatic system and still enjoy being in a state of lasting happiness described by the positive limit cycle.
In Figure 7C, we plot the bifurcation diagram for the happiness variable H when the hedonic damping β=1.5, which can be interpreted as a value characterizing a person less sensitive to emotional inputs (i.e., a person who is not as impacted by emotional inputs). Note that the progress towards lasting happiness does not go through a homoclinic bifurcation, and a hysteretic phenomenon is not present. The bifurcation diagram for C, in this case, would look like the one in Figure 7B, and as mentioned before, the path to lasting occurs as p passes the SNIC bifurcation at p=1.
We could, therefore, think that having a low hedonic damping β is advantageous as it would allow for the possibility of experiencing a shakable sustainable happiness due to the bistable dynamics arising from the homoclinic bifurcation. This is true; however, a low hedonic damping also poses the disadvantage of ups and downs between positive and negative values of happiness as a response to emotional disturbances around a spiral fixed point. The reverse situation applies when the hedonic damping β is high; the advantage of reducing the impact of emotional inputs is having a more steady return to the fixed point, and the disadvantage would be the absence of bistable dynamics.
Nonetheless, regardless of how well an individual reduces the impact of emotional inputs, the development of a constant eudaimonic or altruistic emotion will overcome the well-being homeostatic system and will lead to a path of lasting happiness. Once this altruistic state is reached, the concept of a personal satisfaction described by the happiness model is dissolved as the well-being homeostatic system is overcome and the fixed points determined by it are now nonexistent.
The progression of regulating our emotional input in an increasing positive and constant fashion is represented by different temporal dynamics of happiness in Figure 7D–F for increasing values of p=ps+q chosen to be below the homoclinic bifurcation value (Figure 7D), within the region of bistability (Figure 7E), and above the saddle-node bifurcation value (Figure 7E).
Assuming a path towards lasting happiness through both the homoclinic and the saddle-node bifurcations, the initial stage of this progression requires the development of a "not so high" constant positive emotional input that falls in the "stable fixed point" region of the two-dimensional bifurcation diagram represented in Figure 5C. At this stage, the main gain is the beneficial effect of lifting up the restoring homeostatic system, which in turn places a person in a better position to achieve new states of satisfaction or goals. An example of the temporal dynamics of happiness at this stage is given in Figure 7D. The next stage would require the development of a "high enough" constant positive emotional input that falls into the "bistability" region of the bifurcation diagram and allows for the possibility to experience a "taste" of lasting happiness. An example of the two possible temporal dynamical behaviours for happiness is given in Figure 7E. Finally, the last stage in the path towards lasting happiness requires the development of a "high" and "altruistic" constant positive emotional input that falls into the "stable limit cycle" region of the bifurcation diagram, overcomes the well-being homeostatic system, and guarantees the experience of lasting happiness, as illustrated in Figure 7F and proved in Section 3.3.1.
As the purpose of the proposed model is to increase our understanding of the dynamical nature of happiness and gain insight into the theoretical underpinnings of well-being components, its validity will rest not only on its ability to fit empirical data but also on its ability to predict qualitatively expected conceptual outcomes related to well-being. We can think of this as a distinction between the quantitative and the qualitative predictive ability of the model. The focus of this section is to offer an initial discussion of these two aspects.
Given the novel mathematical modelling approach to happiness presented in this work, we have no experimental data available that can be currently used to test the quantitative predictive ability of the model. However, we can still provide a brief discussion on some basic ideas of an experimental design to test the model, its potential applicability, and the qualitative predictions that the model does offer.
From the previous sections, we notice that the dynamics of an individual's happiness is subjected to emotional inputs; therefore, a test of the model could involve inducing basic positive or negative emotions in relation to a goal with a subsequent assessment of self-reported levels of happiness. The experimental design could utilize well-established methods for inducing basic emotions [35], and should aim at estimating the parameters of the model, namely the hedonic damping β and the set-point related parameter ps.
Once these parameters are determined, they can first be used to characterize an individual based on these personality traits. For example, β, which is the hedonic damping parameter, describes how emotionally sensitive a person is; the lower the value of β the more emotionally sensitive a person is, in other words, the more intense the experience of emotional inputs is. The quantification and estimation of this parameter has the potential to offer new insights into the psychological construct of emotional sensitivity, which according to Wall et al. [36], remains to be fully understood. In the case of the set-point related parameter ps, if we remember its effect on the well-being homeostatic system described by Fr, we note that the value of ps determines a range of high certainty that provides favorable conditions for an adequate progress towards a goal, and also a range of low certainty. If the full range (of both high and low certainty) is considered to be 100%, then estimating ps is equivalent to estimating the percentage range of high certainty, which in turn can be interpreted as a subjective well-being set-point measurement. For instance, if an individual has 70% range of high certainty or favorable conditions for being satisfied, then we characterize the individual with a well-being set-point of 70%. If this interpretation is assumed, the process of estimating ps can be significantly simplified by using existing methods for assessing the subjective well-being set-point [37,38] to indirectly estimate the set-point related parameter ps.
After such a characterization, the model parameter estimates can also be used to simulate different dynamical scenarios with the intend of suggesting possible emotion regulation strategies [39] that can provide individuals with guidelines for making meaningful life changes to better their well-being. We hope that the present work can serve as a first step towards robust experimental designs that allow to quantitatively assess the validity of the model.
As mentioned above, the validity of the model also rests on its ability to offer qualitative predictions that are conceptually plausible. This is the case for the proposed model, which qualitatively predicts expected conceptual outcomes related to well-being. First, the model offers a direct causal relation between positive affects and the experience of happiness, which is in accordance with the prevalence of positive affects in the experience of high subjective well-being [18]. Second, the model simulates the return to a steady state of happiness after the experience of an emotional impulse in line with the expected outcome of hedonic adaptation [24,25], where individual differences of this return are explained by distinct values of the hedonic adaptation or damping parameter β. Third, the model predicts that lasting happiness is achievable by permanently overcoming the homeostatic hedonic system (described in the model by the term Fr) with an eudaimonic emotion (described in the model by an emotional input Fe(t)≥1−ps for all t), which is consistent with the idea that eudaimonic well-being is beyond the boundaries of hedonic homeostasis [10,11]. This last qualitative prediction adds significant validity to the model; by distancing itself from the old "victimology" view of psychology (where human beings are seen as passive individuals subjected to external events) and being built on the basis of existing positive psychology views of well-being, we would expect a qualitative prediction of the model dynamics that allows for the possibility of lasting happiness.
In this study, we sought to model the dynamics of happiness by incorporating the hedonic and eudaimonic views of well-being and a set of theory-led assumptions into a differential equation. The model, given by Eq (2.10), describes happiness as a rate of change that distinguishes between adequate and inadequate progress towards a goal or new state of satisfaction, considers emotions as driving forces of its dynamics, and assumes the existence of intrinsic human mechanisms, such as the hedonic adaptation and the well-being homeostatic systems, which modulate the impact of emotions.
The model was built under these theory-led assumptions, which were described with particular mathematical functions thoughtfully chosen. In the case of the homeostatic restoring force, given by Eq (2.5), it is worthwhile to mention that even though its features describing the well-being homeostatic system are consistent with the key aspects of discrepancy and goal-oriented theories and the hedonic homeostasis, one could potentially define the homeostatic restoring force, Fr, more generally on the basis of those features and consider other functions with those characteristics on the interval (−π,π], where C=−π is identified with C=π. For example, one could choose a polynomial function, say of degree three, or a piecewise linear function. The advantage of using the sine function, besides being a simple periodic function that successfully describes all the key features of the hedonic homeostasis, is that the model for the dynamics of happiness is described by a well-known differential equation, that of the damped pendulum forced with a torque. This allowed us to use the existing knowledge of the dynamics of the equation and interpret it from the perspective of the dynamics of happiness. However, as the equation could be found difficult to treat analytically, the use a polynomial function or a piecewise linear function might have the advantage of obtaining a model that could be less difficult to treat analytically. For example, if the hedonic homeostatic force were chosen to be a piecewise-linear function, the resulting model (with no emotional input) would be described by a non-smooth piecewise-linear system, and one could use a different set of techniques to find a solution and analyse the model. Although beyond the scope of this manuscript, we recognize that it would be valuable to explore other possible functions to describe the restoring force.
The basic dynamics of the proposed happiness model were characterized through the study of the effect of emotional inputs. These emotional inputs did not have a time scale associated to them, as it does not affect the analysis carried out in this study. However, we can think of the time scale of the emotional inputs as determining the time scale of the model. By taking simple profiles, such as impulse, linear increasing-decreasing, and constant functions as emotional inputs, the dynamics of the model provided insight on how the hedonic aspect of happiness (subject to the hedonic adaptation and well-being homeostatic systems) leaves people treading the hedonic treadmill with positive and negative levels of happiness. Note that the effect of other types of emotional inputs (that might also leave us with fluctuating levels of happiness) could also be analysed; for instance, it is reasonable to study the effect of periodic emotional inputs (of the sine type, for example) on the model dynamics, as emotions might oscillate periodically in relation to periodic external phenomena. This is, in fact, an interesting problem that is currently under investigation and is expected to add more richness to the model dynamics and implications.
The dynamics of the model also described how the eudaimonic or flourishing aspect of happiness, if interpreted as overcoming the limiting influences of the well-being homeostatic system, can lead to lasting happiness through the development of altruistic constant positive emotions. We could argue that an altruistic emotion that is maintained at a constant level becomes simply an altruistic quality. Therefore, on the basis of the model dynamics and assumptions, we can state, in accord with current theories of flourishing and well-being, that lasting happiness is possible through the development of altruistic qualities that free us from a well-being homeostatic system which wants to keep us on a hedonic treadmill rushing from one personal state of satisfaction to the next.
The model dynamics can result in the two distinctive types of happiness described in western psychology, namely a hedonic happiness, experienced subjected to the hedonic homeostatic system and that leaves us treading the hedonic treadmill with positive and negative levels of happiness, and a eudaimonic happiness, experienced when the hedonic homeostatic system has been permanently overcome by a constant altruistic emotion. Interestingly, these two types of happiness closely resemble the two types of happiness described by the eastern tradition of Buddhism, namely relative or conditioned happiness, and absolute or unconditioned happiness [40]. This relation has been made apparent by the 'self-centeredness/selflessness happiness model' developed with insights of eastern wisdom by Dambrun and Ricard [41]. According to the theoretical psychological model, a self-centered psychological functioning, characterized by several interconnected psychological components such as hedonic emotions, behaviours and motivations, lead to a fluctuating happiness, whereas a selfless psychological functioning, also characterized by some interconnected psychological components such as altruistic and eudaimonic emotions, behaviours and motivations, lead to an authentic-durable happiness. Thus, the proposed mathematical model for happiness also adds to the parallels between the western and eastern psychology views of happiness.
Another interesting implication coming from the happiness model proposed in this article is that the model does not put in opposition the hedonic and eudaimonic aspects of happiness, but it rather highlights the synergistic effect of the hedonic aspect of happiness on its eudaimonic aspect. Specifically, the progression to lasting happiness, described in section 3.3, requires the development of a constant positive emotion that initially keeps us subject to the well-being homeostatic system, but with a higher level of confidence to remain engaged in goal-directed efforts and achieve new states of satisfaction [42]. Even though still driven by the hedonic aspect of happiness, a higher level of confidence allows us to develop higher constant levels of positive emotions and ultimately provides a better position for overcoming the well-being homeostatic system. In other words, developing our human potential through the hedonic experience of happiness is part of the path to flourishing and to freeing ourselves from the hardwired well-being homeostatic system.
The proposed model provides insights into the dynamical experience of happiness, consistent with its hedonic and eudaimonic views, and stresses the important influence of emotions on the levels of happiness and how the development of altruistic qualities (constant altruistic or eudaimonic emotions that overcome the well-being homeostatic system) can lead to lasting happiness. We hope these uplifting theoretical results can bring awareness to the importance of regulating emotions as means to gradually develop altruistic qualities and sustain positive levels of happiness.
This work was supported by the Athabasca University Academic Research Fund (Grant Number: 22910). J.M. acknowledges the support received from the Mitacs Globalink Research Award. The authors thank the reviewers, whose thoughtful comments allowed for significant improvements in the article.
The authors declare that they have no conflict of interest.
[1] | G. Meister, T. Tuschl, Mechanisms of gene silencing by double-stranded RNA, emphNature, 431 (2004), 343–349. https://doi.org/10.1038/nature02873 |
[2] | S. M. Hammond, An overview of microRNAs, Adv. Drug Deliv. Rev., 87 (2015), 3–14. https://doi.org/10.1016/j.addr.2015.05.001 |
[3] |
S. Rajasekaran, D. Pattarayan, P. Rajaguru, P. S. Gandhi, R. K. Thimmulappa, MicroRNA Regulation of Acute Lung Injury and Acute Respiratory Distress Syndrome, J. Cell. Physiol., 231 (2016), 2097–2106. https://doi.org/10.1002/jcp.25316 doi: 10.1002/jcp.25316
![]() |
[4] |
Y. Meng, C. Lu, M. Jin, J. Xu, X. Zeng, J. Yang, A weighted bilinear neural collaborative filtering approach for drug repositioning, Brief. Bioinformatics, 2 (2022), bbab581. https://doi.org/10.1093/bib/bbab581 doi: 10.1093/bib/bbab581
![]() |
[5] |
Y. W. Kong, D. Ferland-McCollough, T. J. Jackson, M. Bushell, microRNAs in cancer management, Lancet Oncol., 13 (2012), e249–e258. https://doi.org/10.1016/S1470-2045(12)70073-6 doi: 10.1016/S1470-2045(12)70073-6
![]() |
[6] |
M. Chen, Y. Zhang, A. Li, Z. Li, W. Liu, Z. Chen, Bipartite heterogeneous network method based on co-neighbor for MiRNA-disease association prediction, Front. Genet., 10 (2019), 385. https://doi.org/10.3389/fgene.2019.00385 doi: 10.3389/fgene.2019.00385
![]() |
[7] |
L. Cai, M. Gao, X. Ren, X. Fu, J. Xu, P. Wang, et al., MILNP: Plant lncRNA-miRNA Interaction Prediction Based on Improved Linear Neighborhood Similarity and Label Propagation, Front. Plant Sci., 7 (2017), page 637. https://doi.org/10.3389/fpls.2022.861886 doi: 10.3389/fpls.2022.861886
![]() |
[8] | L. Zhuo, S. Pan, J. Li, X. Fu Predicting miRNA-lncRNA interactions on plant datasets based on bipartite network embedding method, 207 (2022), 97–102. https://doi.org/10.1016/j.ymeth.2022.09.002 |
[9] | L. Peng, Y. Tu, L. Huang, Y. Li, X. Fu, X. Chen, DAESTB: inferring associations of small molecule–miRNA via a scalable tree boosting model based on deep autoencoder, Briefings in Bioinformatics, 23 (2022), bbac478. https://doi.org/10.1093/bib/bbac478 |
[10] | J. Wei, L. Zhuo, Z. Zhou, X. Lian, X. Fu, X. Yao, GCFMCL: predicting miRNA-drug sensitivity using graph collaborative filtering and multi-view contrastive learning, Briefings in Bioinformatics, 24 (2023), bbad247. https://doi.org/10.1093/bib/bbad247 |
[11] |
Y. Li, C. Liang, K. Wong, J. Luo, Z. Zhang, Mirsynergy: detecting synergistic miRNA regulatory modules by overlapping neighbourhood expansion, Bioinformatics, 30 (2014), 2627–2635. https://doi.org/10.1093/bioinformatics/btu373 doi: 10.1093/bioinformatics/btu373
![]() |
[12] |
Q. Jiang, Y. Wang, Y. Hao, L. Juan, M. Teng, X. Zhang, et al., miR2Disease: a manually curated database for microRNA deregulation in human disease, Nucleic Acids Res., 37 (2009), D98–D104. https://doi.org/10.1093/nar/gkn714 doi: 10.1093/nar/gkn714
![]() |
[13] |
Z. Yang, F. Ren, C. Liu, S. He, G. Sun, Q. Gao, et al., dbDEMC: a database of differentially expressed miRNAs in human cancers, BMC Genom., 11 (2010), 1–8. https://doi.org/10.1186/1471-2164-11-S4-S5 doi: 10.1186/1471-2164-11-S4-S5
![]() |
[14] | Q. Jiang, G. Wang, T. Zhang, Y. Wang, Predicting human microrna-disease associations based on support vector machine, 2010 IEEE Int. Confer. Bioinformatics Biomed., (2010), 467–472. https://doi.org/10.1109/BIBM.2010.5706611 |
[15] |
P. Wang, W. Zhu, B. Liao, L. Cai, L. Peng, J. Yang, Predicting influenza antigenicity by matrix completion with antigen and antiserum similarity, Front. Microbiol., 9 (2018), 2500. https://doi.org/10.3389/fmicb.2018.02500 doi: 10.3389/fmicb.2018.02500
![]() |
[16] |
L. Shen, F. Liu, L. Huang, G. Liu, L. Zhou, L. Peng, VDA-RWLRLS: An anti-SARS-CoV-2 drug prioritizing framework combining an unbalanced bi-random walk and Laplacian regularized least squares, Comput. Biol. Med., 140 (2022), 105–119. https://doi.org/10.1016/j.compbiomed.2021.105119 doi: 10.1016/j.compbiomed.2021.105119
![]() |
[17] |
L. Cai, C. Lu, J. Xu, Y. Meng, P. Wang, X. Fu, et al., Drug repositioning based on the heterogeneous information fusion graph convolutional network, Brief. Bioinformatics, 22 (2021), bbab319. https://doi.org/10.1093/bib/bbab319 doi: 10.1093/bib/bbab319
![]() |
[18] | Y. Chen, X. Fu, Z. Li, L. Peng, L. Zhuo, Prediction of lncRNA–protein interactions via the multiple information integration, Front. Bioeng. Biotechnol., 9 (2021), 647113. https://doi.org/10.3389/fbioe.2021.647113 |
[19] | J. Wei, L. Zhuo, S. Pan, X. Lian, X. Yao, X. Fu, Headtailtransfer: An efficient sampling method to improve the performance of graph neural network method in predicting sparse ncRNA–protein interactions, Comput. Biol. Med., 157 (2023), 106783. https://doi.org/10.1016/j.compbiomed.2023.106783 |
[20] | L. Zhuo, B. Song, Y. Liu, Z. Li, X. Fu, Predicting ncRNA–protein interactions based on dual graph convolutional network and pairwise learning, Brief. Bioinformatics, 23 (2022), bbac339. https://doi.org/10.1093/bib/bbac339 |
[21] | X. Zhang, X. Zeng, Integrative approaches for predicting microRNA function and prioritizing disease-related microRNA using biological interaction networks, Bio-inspired Comput. Model. Algorithms, (2019), 75–105. https://doi.org/10.1142/9789813143180_0003 |
[22] |
Q. Zou, J. Li, L. Song, X. Zeng, G. Wang, Similarity computation strategies in the microRNA-disease network: a survey, Brief Funct. Genomics, 15 (2016), 55–64. https://doi.org/10.1093/bfgp/elv024 doi: 10.1093/bfgp/elv024
![]() |
[23] | L. Cai, X. Ren, X. Fu, L. Peng, M. Gao, X. Zeng, iEnhancer-XG: interpretable sequencebased enhancers and their strength predictor, Bioinformatics, 37 (2021), 1060–1067. https://doi.org/10.1093/bioinformatics/btaa914 |
[24] | X. Fu, L. Cai, X. Zeng, Q. Zou, StackCPPred: a stacking and pairwise energy content-based prediction of cell-penetrating peptides and their uptake efficiency, Bioinformatics, 36 (2020), 3028–3034. https://doi.org/10.1093/bioinformatics/btaa131 |
[25] | X. Fu, L. Ke, L. Cai, X. Chen, X. Ren, M. Gao, Improved prediction of cell-penetrating peptides via effective orchestrating amino acid composition feature representation, IEEE Access, 7 (2019), 163547–163555. https://doi.org/10.1109/ACCESS.2019.2952738 |
[26] | W. Liu, T. Tang, X. Lu, X. Fu, Y. Yang, L. Peng, MPCLCDA: predicting circRNA–disease associations by using automatically selected meta-path and contrastive learning, Brief. Bioinformatics, 24 (2023), bbad227. https://doi.org/10.1093/bib/bbad227 |
[27] | L. Peng, C. Yang, Y. Chen, W. Liu, Predicting CircRNA-disease associations via feature convolution learning with heterogeneous graph attention network, IEEE J. Biomed. Health. Inform., 27 (2023), 3072–3082. https://doi.org/10.1109/JBHI.2023.3260863 |
[28] | T. Wang, W. Wang, X. Jiang, J. Mao, L. Zhuo, M. Liu, et al., ML-NPI: predicting interactions between noncoding RNA and protein based on meta-learning in a large-scale dynamic graph, J. Chem. Inf. Model., 64 (2023), 2912–2920. https://doi.org/10.1021/acs.jcim.3c01238 |
[29] | Z. Zhou, Z. Du, J. Wei, L. Zhuo, S. Pan, X. Fu, et al., MHAM-NPI: Predicting ncRNA-protein interactions based on multi-head attention mechanism, Comput. Biol. Med., 163 (2023), 107143. https://doi.org/10.1016/j.compbiomed.2023.107143 |
[30] | Q. Liao, X. Fu, L. Zhuo, H. Chen, An efficient model for predicting human diseases through miRNA based on multiple-types of contrastive learning, Front. Microbiol., 14 (2023), 1325001. https://doi.org/10.3389/fmicb.2023.1325001 |
[31] | W. Liu, H. Lin, L. Huang, L. Peng, T. Tang, Q. Zhao, et al., Identification of miRNA–disease associations via deep forest ensemble learning based on autoencoder, Brief. Bioinformatics, 23 (2022), bbac104. https://doi.org/10.1093/bib/bbac104 |
[32] |
Q. Jiang, G. Wang, Y. Wang, An approach for prioritizing disease-related microRNAs based on genomic data integration, 2010 3rd Int. Confer. Biomed. Eng. Inform., 6 (2010), 2270–2274. https://doi.org/10.1109/BMEI.2010.5639313 doi: 10.1109/BMEI.2010.5639313
![]() |
[33] |
J. Xu, C. Li, J. Lv, Y. Li, Y. Xiao, T. Shao, et al., Prioritizing Candidate Disease miRNAs by Topological Features in the miRNA Target–Dysregulated Network: Case Study of Prostate Cancer, Mol. Cancer Ther., 10 (2011), 1857–1866. https://doi.org/10.1158/1535-7163.MCT-11-0055 doi: 10.1158/1535-7163.MCT-11-0055
![]() |
[34] |
X. Zeng, Y. Liao, Y. Liu, Q. Zou, Prediction and validation of disease genes using HeteSim Scores, IEEE/ACM Trans. Comput. Biol. Bioinform., 14 (2016), 687–695. 10.1109/TCBB.2016.2520947 doi: 10.1109/TCBB.2016.2520947
![]() |
[35] |
Q. Xiao, J. Luo, C. Liang, J. Cai, P. Ding, A graph regularized non-negative matrix factorization method for identifying microRNA-disease associations, Bioinformatics, 34 (2018), 239–248. https://doi.org/10.1093/bioinformatics/btx545 doi: 10.1093/bioinformatics/btx545
![]() |
[36] |
J. Xu, L. Cai, B. Liao, W. Zhu, P. Wang, Y. Meng, et al., Identifying potential mirnas–disease associations with probability matrix factorization, Front. Genet., 10 (2019), 1234. https://doi.org/10.3389/fgene.2019.01234 doi: 10.3389/fgene.2019.01234
![]() |
[37] |
X. Chen, G. Yan, Semi-supervised learning for potential human microRNA-disease associations inference, Sci. Rep., 4 (2014), 1–10. https://doi.org/10.1038/srep05501 doi: 10.1038/srep05501
![]() |
[38] | W. Liu, X. Sun, L. Yang, K. Li, Y. Yang, X. Fu, NSCGRN: a network structure control method for gene regulatory network inference, Brief. Bioinform., (2022). https://doi.org/10.1093/bib/bbac156 |
[39] | Q. Qu, X. Chen, B. Ning, X. Zhang, H. Nie, L. Zeng, et al., Prediction of miRNA-disease associations by neural network-based deep matrix factorization, Methods, 212 (2023), 1–9. https://doi.org/10.1016/j.ymeth.2023.02.003 |
[40] | W. Liu, Y. Yang, X. Lu, X. Fu, R. Sun, L. Yang, et al., NSRGRN: a network structure refinement method for gene regulatory network inference, Brief. Bioinformatics, 24 (2023), bbad129. https://doi.org/10.1093/bib/bbad129 |
[41] | L. Peng, C. Yang, L. Huang, X. Chen, X. Fu, W. Liu, RNMFLP: predicting circRNA–disease associations based on robust nonnegative matrix factorization and label propagation, Brief. Bioinformatics, 24 (2023), bbac155. https://doi.org/10.1093/bib/bbad155 |
[42] |
C. Gu, B. Liao, X. Li, K. Li, Network consistency projection for human miRNA-disease associations inference, Sci. Rep., 6 (2016), 1–10. https://doi.org/10.1038/srep36054 doi: 10.1038/srep36054
![]() |
[43] |
X. Chen, C. C. Yan, X. Zhang, Z. You, L. Deng, Y. Liu, et al., WBSMDA: within and between score for MiRNA-disease association prediction, Sci. Rep., 6 (2016), 1–9. https://doi.org/10.1038/srep21106 doi: 10.1038/srep21106
![]() |
[44] |
Y. Liu, X. Zeng, Z. He, Q. Zou, Inferring microRNA-disease associations by random walk on a heterogeneous network with multiple data sources, IEEE/ACM Trans. Comput. Biol. Bioinform., 14 (2016), 905–915. https://doi.org/10.1109/TCBB.2016.2550432 doi: 10.1109/TCBB.2016.2550432
![]() |
[45] |
A. Li, Y. Deng, Y. Tan, M. Chen, A novel mirna-disease association prediction model using dual random walk with restart and space projection federated method, PLoS One, 6 (2021), e0252971. https://doi.org/10.1371/journal.pone.0252971 doi: 10.1371/journal.pone.0252971
![]() |
[46] |
X. Chen, M. Liu, G. Yan, RWRMDA: predicting novel human microRNA–disease associations, Mol. BioSyst., 8 (2012), 2792–2798. https://doi.org/10.1039/c2mb25180a doi: 10.1039/c2mb25180a
![]() |
[47] |
P. Xuan, K. Han, M. Guo, Y. Guo, J. Li, J. Ding, et al., Prediction of microRNAs Associated with Human Diseases Based on Weighted k Most Similar Neighbors, PloS One, 8 (2013), e70204. https://doi.org/10.1371/journal.pone.0070204 doi: 10.1371/journal.pone.0070204
![]() |
[48] |
P. Xuan, C. Sun, T. Zhang, Y. Ye, T. Shen, Y. Dong, Gradient boosting decision tree-based method for predicting interactions between target genes and drugs, Front. Genet., 10 (2019), 459. https://doi.org/10.3389/fgene.2019.00459 doi: 10.3389/fgene.2019.00459
![]() |
[49] |
H. Chen, Z. Zhang, Similarity-based methods for potential human microRNA-disease association prediction, BMC Med. Genom., 6 (2013), 1–9. https://doi.org/10.1186/1755-8794-6-12 doi: 10.1186/1755-8794-6-12
![]() |
[50] |
D. Wang, J. Wang, M. Lu, F. Song, Q. Cui, Inferring the human microRNA functional similarity and functional network based on microRNA-associated diseases, Bioinformatics, 26 (2010), 1644–1650. https://doi.org/10.1093/bioinformatics/btq241 doi: 10.1093/bioinformatics/btq241
![]() |
[51] | P. Jain, I. S. Dhillon, Provable inductive matrix completion, arXiv preprint, (2013), arXiv: 1306.0626. |
[52] |
D. Wang, J. Wang, M. Lu, F. Song, Q. Cui, H. Yu, et al., Large-scale prediction of microRNA-disease associations by combinatorial prioritization algorithm, Sci. Rep., 7 (2017), 1–15. https://doi.org/10.1038/srep43792 doi: 10.1038/srep43792
![]() |
1. | Reuben D. Rusk, An Adaptive Motivation Approach to Understanding the ‘How’ and ‘Why’ of Wellbeing, 2022, 19, 1660-4601, 12784, 10.3390/ijerph191912784 | |
2. | Yongsong Wu, Yang Cao, Research on the Influence of Ecological Environment Satisfaction and Income Level on Chinese Residents’ Happiness: Empirical Analysis Based on CGSS Data, 2023, 15, 2071-1050, 8175, 10.3390/su15108175 | |
3. | Jean-Denis Mathias, Nicolas Pellerin, Gustavo Carrero, Eric Raufaste, Michael Dambrun, Running on the Hedonic Treadmill: A Dynamical Model of Happiness Based on an Approach–Avoidance Framework, 2024, 25, 1389-4978, 10.1007/s10902-024-00766-3 | |
4. | Zeynep Hasgul, Anne Spanjaart, Sumreen Javed, Ali Akhavan, Marie José Kersten, Mohammad S. Jalali, Health-related quality of life dynamics: modeling insights from immunotherapy, 2024, 0962-9343, 10.1007/s11136-024-03810-0 | |
5. | Yunpeng Hu, Guanghui Tian, Lan Gao, Yangyang Yu, Guodong Ni, Formation Mechanism and Evolutionary Laws of Well-Being at Work Among the New Generation of Construction Workers in China, 2025, 15, 2075-5309, 799, 10.3390/buildings15050799 |