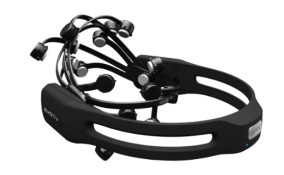
BMI has attracted widespread attention in the past decade, which has greatly improved the living conditions of patients with motor disorders. The application of EEG signals in lower limb rehabilitation robots and human exoskeleton has also been gradually applied by researchers. Therefore, the recognition of EEG signals is of great significance. In this paper, a CNN-LSTM neural network model is designed to study the two-class and four-class motion recognition of EEG signals. In this paper, a brain-computer interface experimental scheme is designed. Combining the characteristics of EEG signals, the time-frequency characteristics of EEG signals and event-related potential phenomena are analyzed, and the ERD/ERS characteristics are obtained. Pre-process EEG signals, and propose a CNN-LSTM neural network model to classify the collected binary and four-class EEG signals. The experimental results show that the CNN-LSTM neural network model has a good effect, and its average accuracy and kappa coefficient are higher than the other two classification algorithms, which also shows that the classification algorithm selected in this paper has a good classification effect.
Citation: Ying Chang, Lan Wang, Yunmin Zhao, Ming Liu, Jing Zhang. Research on two-class and four-class action recognition based on EEG signals[J]. Mathematical Biosciences and Engineering, 2023, 20(6): 10376-10391. doi: 10.3934/mbe.2023455
[1] | Sakorn Mekruksavanich, Wikanda Phaphan, Anuchit Jitpattanakul . Epileptic seizure detection in EEG signals via an enhanced hybrid CNN with an integrated attention mechanism. Mathematical Biosciences and Engineering, 2025, 22(1): 73-105. doi: 10.3934/mbe.2025004 |
[2] | Yanling An, Shaohai Hu, Shuaiqi Liu, Bing Li . BiTCAN: An emotion recognition network based on saliency in brain cognition. Mathematical Biosciences and Engineering, 2023, 20(12): 21537-21562. doi: 10.3934/mbe.2023953 |
[3] | Dingxin Xu, Xiwen Qin, Xiaogang Dong, Xueteng Cui . Emotion recognition of EEG signals based on variational mode decomposition and weighted cascade forest. Mathematical Biosciences and Engineering, 2023, 20(2): 2566-2587. doi: 10.3934/mbe.2023120 |
[4] | Ravichandra Madanu, Farhan Rahman, Maysam F. Abbod, Shou-Zen Fan, Jiann-Shing Shieh . Depth of anesthesia prediction via EEG signals using convolutional neural network and ensemble empirical mode decomposition. Mathematical Biosciences and Engineering, 2021, 18(5): 5047-5068. doi: 10.3934/mbe.2021257 |
[5] | Tianhui Sha, Yikai Zhang, Yong Peng, Wanzeng Kong . Semi-supervised regression with adaptive graph learning for EEG-based emotion recognition. Mathematical Biosciences and Engineering, 2023, 20(6): 11379-11402. doi: 10.3934/mbe.2023505 |
[6] | Xuelin Gu, Banghua Yang, Shouwei Gao, Lin Feng Yan, Ding Xu, Wen Wang . Application of bi-modal signal in the classification and recognition of drug addiction degree based on machine learning. Mathematical Biosciences and Engineering, 2021, 18(5): 6926-6940. doi: 10.3934/mbe.2021344 |
[7] | Su-na Zhao, Yingxue Cui, Yan He, Zhendong He, Zhihua Diao, Fang Peng, Chao Cheng . Teleoperation control of a wheeled mobile robot based on Brain-machine Interface. Mathematical Biosciences and Engineering, 2023, 20(2): 3638-3660. doi: 10.3934/mbe.2023170 |
[8] | Jia-Gang Qiu, Yi Li, Hao-Qi Liu, Shuang Lin, Lei Pang, Gang Sun, Ying-Zhe Song . Research on motion recognition based on multi-dimensional sensing data and deep learning algorithms. Mathematical Biosciences and Engineering, 2023, 20(8): 14578-14595. doi: 10.3934/mbe.2023652 |
[9] | Xian Hua, Jing Li, Ting Wang, Junhong Wang, Shaojun Pi, Hangcheng Li, Xugang Xi . Evaluation of movement functional rehabilitation after stroke: A study via graph theory and corticomuscular coupling as potential biomarker. Mathematical Biosciences and Engineering, 2023, 20(6): 10530-10551. doi: 10.3934/mbe.2023465 |
[10] | Zizhuo Wu, Qingshan She, Zhelong Hou, Zhenyu Li, Kun Tian, Yuliang Ma . Multi-source online transfer algorithm based on source domain selection for EEG classification. Mathematical Biosciences and Engineering, 2023, 20(3): 4560-4573. doi: 10.3934/mbe.2023211 |
BMI has attracted widespread attention in the past decade, which has greatly improved the living conditions of patients with motor disorders. The application of EEG signals in lower limb rehabilitation robots and human exoskeleton has also been gradually applied by researchers. Therefore, the recognition of EEG signals is of great significance. In this paper, a CNN-LSTM neural network model is designed to study the two-class and four-class motion recognition of EEG signals. In this paper, a brain-computer interface experimental scheme is designed. Combining the characteristics of EEG signals, the time-frequency characteristics of EEG signals and event-related potential phenomena are analyzed, and the ERD/ERS characteristics are obtained. Pre-process EEG signals, and propose a CNN-LSTM neural network model to classify the collected binary and four-class EEG signals. The experimental results show that the CNN-LSTM neural network model has a good effect, and its average accuracy and kappa coefficient are higher than the other two classification algorithms, which also shows that the classification algorithm selected in this paper has a good classification effect.
BMI systems have attracted widespread attention in the past decade because of their potential to improve human life, especially for those affected by motor disorders. Since gait defects are usually associated with spinal cord injury (SCI), limb loss and neurodegenerative diseases, it is necessary to study innovative therapies to restore the gait of such patients. Exoskeleton has become an important tool for rehabilitation of SCI and stroke patients [1]. BMI has been deployed to infer the user's intention from the user's brain activity to generate output signals to control the dynamic exoskeleton for upper and lower limb rehabilitation [2,3]. Presacco et al. showed that the gait kinematics of the treadmill during walking was decoded from the EEG of healthy subjects, and its accuracy was equivalent to that of the similar research of implanting electrodes in the brain of non-human primates [4]. In order to control the device through BMI, it is necessary to accurately identify the different brain activity patterns generated by the user through the neural interface system and convert them into appropriate commands. It is possible to consider discrete decoding of intentions from EEG signals [5]. Brain-computer interface is a new way of communication, which allows non-physical communication between humans and machines. As an emerging technology, there are many shortcomings, such as low information transmission rate and poor usability. Gait recognition based on EEG signals is a method to recognize and classify different types of gait using EEG signals, such as horizontal walking, slope descending, slope ascending, stair descending and stair ascending [6]. This method can be used to control systems that require precise gait phase classification. Gait recognition methods based on electroencephalogram (EMG) signals include collecting the movement tracks of key joints of the lower limbs, as well as surface EMG signals and EEG signals. The addition of EEG signals helps to improve the accuracy of gait recognition by providing more information about motion intention. Research shows that EEG signals before exercise can be used to predict the intention of the beginning or end of human gait [7]. This shows that EEG signals can be used to accurately identify different types of gait.
As a pattern recognition problem, advanced machine learning technology is needed to accurately convert brain electrical activity into meaningful control commands. Because the accuracy of traditional methods in EEG classification is generally between 70 and 85%, with the development of artificial intelligence, scholars began to search for new EEG signal processing methods in the field of deep learning. Among them, Ian Walker of the UK achieved the highest accuracy rate of 80% and the lowest accuracy rate of 42% on the four classification issues [8]; Leonardo Garrido of the United States directly adopted the convolution neural network method to achieve a classification accuracy rate of 70% on the binary classification problem [9]; In China, Tang Zhichuan and others used convolutional neural network to extract and classify features after manually selecting channels, and obtained 88.75% classification accuracy [10]. But these cannot meet the classification requirements of EEG signals, so it is also necessary to study the classification and recognition of EEG signals. Many machine learning methods, such as linear discriminant analysis (LDA), support vector machine (SVM), CNN and Bayesian classifier, have been applied to EEG signal classification in different BMI applications [3,11,12,13,14]. However, most of them are "black boxes", because we do not know how brain activity changes when using BMI for a long time, nor how brain regions participate in the classification process when people perform different tasks. The human brain is composed of more than 100 billion cells, which are usually divided into different regions by neuroanatomists. Different regions have their specific functions in coordinating and completing daily tasks. In addition, the specific contribution of brain regions to classification may change due to learning BMI. Therefore, identifying and tracking these changes is very important to improve our understanding of brain function, BMI learning and performance. In this context, the hypothesis of this study is that different brain regions have different contributions to BMI. Kernel learning methods have been effectively applied to many machine learning problems, including feature selection, data regression and EEG signal classification [15,16,17]. SVM is one of the most popular core methods in pattern recognition. However, one problem with using the standard SVM in BMI application is that it does not have a deep understanding of the importance of different features, so little is known about the biophysical properties of the relevant features used in decoding/classification. Multi-core learning (MKL) uses the combination of base cores to represent different types of features or data, which has been proved to be superior to traditional single-core machines in different aspects [18,19,20]. Neeraj et al. proposed a deep learning architecture, which is a combination of FFT-CNN-LSTM and the recently proposed attention mechanism for extracting spatiotemporal features from multi-channel EEG signals. The proposed architecture can classify subjects as alcoholics or controllers with high accuracy by analyzing their EEG signals, and can be used for automatic alcoholism detection. The analysis results using the proposed architecture show 98.83% accuracy, which makes it superior to most of the most advanced algorithms. This also shows that the CNN-LSTM model can identify the direction of EEG signal classification [21]. Gait recognition is the process of identifying individuals according to walking patterns. The combination of convolutional neural network (CNN) and short-term memory (LSTM) algorithm has been used in several studies of gait recognition [22,23]. CNN layer is used to extract features from input data, while LSTM layer processes time series [22]. A study proposed an abnormal gait recognition algorithm, which can detect some abnormal gait patterns, including hemiplegia, tiptoe and cross-threshold gait [24]. Another study developed a new gait pattern recognition method based on LSTM-CNN for lower limb exoskeleton [22]. The inertial measurement unit (IMU) installed on the exoskeleton collects motion information as input to the LSTM-CNN model. This study considered five common gait patterns: walking, going up stairs, going down stairs, sitting down and standing up [22]. The CNN and LSTM fusion algorithm has also been used for inertia-based gait recognition. This research aims to identify target users from their gait patterns through accelerometer and gyroscope signals provided by inertial sensors such as smart phones. This method uses deep learning as a general feature extractor for gait recognition. In short, CNN-LSTM algorithm has been applied to many studies of gait recognition. CNN-LSTM algorithm is a powerful tool to solve the problem of spatial input sequence prediction. It has different applications in different fields.
Although most of the researches on EEG based biometrics are focused on epilepsy diagnosis, some researches have explored its application in identity recognition. In one such study, a new EEG based recognition system with different entropy and continuous CNN classifier was proposed. The performance of the proposed method was evaluated experimentally through emotional EEG data, showing an average accuracy of 99.7% and a fast training time. In short, gait recognition of EEG signals based on CNN-LSTM algorithm is an active research field in the field of BCI and biometrics. Although most studies have focused on the diagnosis of epilepsy, some studies have explored its application in identity recognition and achieved good results. Using CNN-LSTM algorithm to recognize gait from EEG signals is a research topic in the field of brain-computer interface (BCI) and biometric recognition. In this paper, we propose the combination of short and long term memory (LSTM) network and convolutional neural network (CNN) for gait recognition of EEG signals. The establishment of the artificial neural network model is very similar to the function and structure of the human brain. At the same time, the algorithm has the characteristics of self-learning ability and parallel processing of a large amount of data. The neural network algorithm can approximate any continuous function with arbitrary accuracy and is widely used in the field of pattern recognition. Therefore, this paper will use neural network to classify the patterns of the motion imagination EEG signals. CNN is a deep feedforward neural network, which is the most common deep learning model. Combined with the characteristics of the long-short memory network LSTM mentioned above, this paper uses the combination of LSTM and CNN neural network to build a CNN-LSTM network model to realize the action classification of EEG signals.
The human brain is composed of tens of thousands of nerves. German psychiatrists were the first to record human EEG signals. All parts of the brain are composed of neurons. A neuron is generally composed of a cell body and several dendrites and an axonal nerve fiber emitted from it. It is the basic structure or functional unit of the nervous system. When neurons are stimulated by various stimuli, they produce and transmit excitatory or inhibitory nerve impulses, and the axons and dendrites complete the interaction between nerve cells. The end of the axon bifurcates into synaptic terminals and contacts with the cells of other neurons or effectors. The excitatory conduction between synaptic neurons is conducted through synapses. Axons are mainly used as efferent fibers, and dendrites are divided into short dendrites and apical dendrites. The short dendrites connect with nerve cells. The apical dendrites originate from the pyramidal cells in the third and fifth layers of the cerebral cortex and extend to the surface of the cortex.
At present, most scholars believe that the apical dendrites play a leading role in the formation of brain waves. EEG activity is generated by the postsynaptic potential of the pyramidal nerve cells and their apical dendrites in the vertical direction. When nerve impulses are transmitted from presynaptic neurons to postsynaptic neurons through the synapse, neurotransmitters are released from the synaptic body and act on the postsynaptic membrane through the gap, which changes the permeability of the postsynaptic membrane to ions, changes the membrane potential and generates local current, which is transmitted to the next neuron through accumulation. According to the theory of neuron feedback circuit, most neurons and intermediate neurons form a closed circuit. When the neurons are excited, the nerve impulses through the feedback circuit can be stimulated by the intermediate neurons themselves to produce a series of repeated stimuli, which will produce periodic and repetitive neuronal discharges, thus recording a continuous comprehensive postsynaptic potential, There is also a rhythmic electrical activity in the cerebral cortex. EEG signal is a kind of bioelectric signal, and its acquisition is also very important.
Due to the low frequency and amplitude of EEG signal, and easy to be interfered by the tested person and the surrounding environment, the EEG acquisition equipment and acquisition method greatly affect the quality of the collected EEG signal. At present, EEG signals are usually collected in two ways: invasive and non-invasive.
Due to the short time required for an EEG signal acquisition experiment, a reasonable design of the experimental scheme is crucial to the real-time and accuracy of the signal. Based on the characteristics of EEG signal movement imagination, this paper designs a complete EEG signal acquisition experimental platform. The EEG signal acquisition platform mainly includes: experimental equipment, stimulation interface, experimental time and process, and requirements for testers.
This paper is to analyze and study EEG signals based on the way of motion imagination. When people conduct motor imagination thinking activities, relevant motor potential signals will be generated, and ERD/ERS phenomenon will occur at the left and right leads of the human brain. This paper will collect the potential of relevant tasks for analysis, so as to get relevant conclusions.
In this experiment, it is necessary to collect EEG signals of different tasks in real time. In this paper, based on the EEG signal acquisition examples and the time sampling rate selection of other laboratories, the EPOC+ helmet instrument of Emotiv Company of the United States is selected as the EEG signal acquisition equipment; Matlab software is used to carry out correlation analysis and processing of the collected original signals, and the recognition results of EEG signals are obtained.
The EEG signal acquisition device used in this paper is EPOC+ helmet instrument, which has 16 electrode sensors (14 acquisition electrodes and 2 reference electrodes, the specific distribution position is shown in Figure 1) and a USB receiver. EEG data are collected at a sampling frequency of 128 Hz, and connected to the USB port by the USB receiver and stored to the computer. Emotiv EPOC+ is a wireless and multi-channel EEG signal acquisition product. This product is specially designed for scenario research and brain-computer interface system research. The raw EEG signal collected has the characteristics of high quality, high temporal and spatial resolution. Emotiv EPOC+ also supports the recognition of human emotions, psychological activities and facial expressions. In addition, the product supports SDK program development. During the data saving process, the measurement data is saved in combination with the software Emotiv Xavier Pure. EEG and exported as CSV file type.
For the collection of human EEG signals, the electrode used by the helmet instrument is wet electrode, as shown in Figure 2.
The EEG signal acquisition platform built in this experiment is shown in Figure 3. The tester wears an Emotiv EPOC+ helmet instrument and plugs the USB receiver into the USB interface of the computer. In the process of EEG signal acquisition, the tester is required to perform the motion imagination task displayed on the stimulation interface. During the whole experiment, the tester is required to keep the correct sitting posture, keep the head as still as possible and concentrate.
In addition, the stimulation interface design in the acquisition experiment is completed by the software Visual Studio 2015 MFC. The interface design is mainly composed of start and end buttons, instruction arrows, and experimental time, task and number display boxes. The experimental interface uses the left arrow and the right arrow to send the action command by flashing prompt and voice prompt at the frequency of 0.5 seconds. In the course of the experiment, the two ways of sending instructions can ensure the accuracy of the tester's brain wakefulness and the type of imaginary tasks.
The traditional analysis methods of EEG signals mainly start from four aspects: time domain analysis, frequency domain analysis, time-frequency analysis and spatial domain analysis.
1) Time domain analysis: all the information of EEG is included in the collected time domain waveform, so time domain analysis is the most intuitive method, which is usually used for preliminary analysis of the amplitude, period and other information of EEG signal. The advantage of this method is that it can extract useful waveform features directly from the time domain, which is simple and convenient, and does not need to assume the stability of EEG signals. Common time-domain analysis methods include amplitude, variance, period analysis, histogram analysis, zero-crossing analysis, etc. These methods can clearly and intuitively express the waveform characteristics, and have been widely used in long-term sleep EEG signal analysis.
2) Frequency domain analysis: Although the time domain analysis method has been applied to some extent, the characteristics of EEG signals mainly come from the frequency domain and spatial domain. The frequency domain analysis method is based on power spectrum estimation. This method converts the time-domain EEG signal with amplitude varying with time into the frequency spectrum with power varying with frequency, which plays a very important role in EEG analysis. By calculating the power spectral density (PSD), we can intuitively see that δ Wave θ Wave α Wave β Wave γ. The distribution and change of the wave highlight the energy characteristics of the EEG signal. Spectral estimation is divided into parameter estimation and non-parametric estimation. Parameter estimation includes power spectrum estimation based on AR model and power spectrum estimation based on AAR model. This method assumes that the signal is generated by a model with known functional form. The parameters of the model are estimated and the spectral characteristics are obtained from it. Non-parametric estimation mainly includes periodogram method based on Fourier transform, correlation graph method, etc.
3) Time-frequency analysis: In the frequency domain analysis, once the signal is transferred from the time domain to the frequency domain, the trend, mutation, the beginning and end of the event in the original time domain signal are all lost. The time-frequency analysis method combines the time domain analysis and the frequency domain analysis, which is a method that can analyze EEG signals more comprehensively. At present, the widely used time-frequency analysis methods are mainly wavelet transform and wavelet packet transform. Wavelet transform has the characteristics of multi-resolution. It has good resolution both in time domain and frequency domain. It is an effective method to analyze non-stationary processes, while wavelet packet transform is a more precise signal decomposition and reconstruction method than wavelet packet transform. Because EEG signals are also non-stationary and nonlinear, both wavelet transform and wavelet packet transform are very suitable for the analysis of EEG signals.
4) Spatial analysis: In the process of EEG signal acquisition, dozens of lead electrodes are usually placed at different positions of the brain. If we study the correlation between the EEG signals recorded by these conductive electrodes at different positions, we need to use the spatial analysis method. Typical spatial analysis methods include common spatial pattern method and independent component analysis method. The common spatial pattern algorithm completes the spatial feature extraction of EEG signals by constructing a spatial filter to maximize the difference of energy distribution of different types of EEG waves projected on the filter. Independent component analysis (ICA) algorithm decomposes the original signal into different independent sub-components, which can be applied not only to the extraction of EEG signal spatial features, but also to the removal of EEG artifacts.
The event related synchronization (ERS)/desynchronization (ERD) phenomenon is an electrophysiological phenomenon that can be used as a feature of the EEG signal of motor imagination. Among them, the ERD phenomenon is specifically manifested as follows: when the unilateral limb is subject to motion imagination or real motion, the somatosensory area and somatosensory area of the contralateral cerebral cortex will be activated, and the increase of blood flow in this area will occur at the same time as the acceleration of metabolism α Reduction of wave frequency and amplitude (α Wave blocking phenomenon); Correspondingly, the ERS phenomenon is specifically manifested as follows: the somatosensory area and somatosensory area of the ipsilateral cerebral cortex will appear because they are not activated α The phenomenon of wave frequency and amplitude rising. ERD and ERS phenomena are closely related and can interact with each other. Research shows that once ERD phenomenon begins to occur in a certain region, it will be accompanied by ERS phenomenon in the adjacent cortex region, and with the passage of time, ERD phenomenon will gradually spread to the somatosensory motor regions on both sides.
Generally, lead C3 and C4 signals contain more signals related to motion. In this paper, only the data on lead C3 and C4 are selected for ERD/ERS phenomenon analysis. According to the ERD/ERS analysis method proposed by G. Pfurtscheller et al. [25], it is described as follows:
1) The raw EEG signal data of all experiments are processed by band-pass filtering;
2) Calculate the square of EEG signal amplitude to obtain its power value;
3) Average the power values of all experiments;
4) Re-average the power value data to achieve curve smoothing and make the ERD/ERS characteristics more significant;
5) Calculate the percentage of relative power change of ERD/ERS from formula (1):
Assume that the power value of the relevant rhythm in the reference period before the exercise imagination experiment is R, and the power value of the relevant rhythm after the exercise imagination experiment is A, then the percentage of the relative power change of ERD/ERS:
(1) |
According to formula (1), when the percentage value of ERD/ERS is positive, the EEG signal shows ERS characteristics; When its value is negative, the EEG signal shows ERD characteristics; When its value is 0, the signal has no ERD/ERS characteristic. In addition, the larger the absolute value of ERD/ERS percentage, the more significant the ERD/ERS characteristic.
This paper analyzes the ERD/ERS phenomenon using the relevant EEG signal data. Among them, the obtained power value curve of mu rhythm section is shown in Figure 4, and the power curve after smoothing is shown in Figure 5.
By analyzing Figures 4 and 5, it can be seen that the power value of the EEG signal of leads C3 and C4 increases/decreases during the left movement imagination. In the first 3 seconds of the experiment, the subjects did not perform the motor imagination thinking task, and the power values of EEG signals of lead C3 and C4 did not change significantly; However, within 3–5 seconds, the signal power value of lead C3 increases, leading to the occurrence of ERS phenomenon, while the signal power value of lead C4 decreases rapidly, leading to ERD phenomenon. During the right movement imagination, the signal power of lead C4 increased in 3–5 seconds, resulting in the occurrence of ERS phenomenon, while the signal power of lead C3 decreased rapidly, resulting in ERD phenomenon.
Take the power value within 1~2.5 seconds as the reference energy value to obtain the ERD/ERS percentage, as shown in Figure 6.
Analyze the ERD/ERS phenomenon of beta rhythm (26~30Hz) in the motion imagination EEG signal, and the percentage curve of ERD/ERS is shown in Figure 7.
It can be seen from Figure 7 that when a person performs a specific motor imagination task (such as right motor imagination), the beta wave signal generated in the time period t = 3–4 seconds, the opposite lead (C3) signal has obvious ERD phenomenon, while the lead (C4) signal on the same side has ERS phenomenon, and at the end of the imagination action, the opposite lead (C3) signal has ERS phenomenon.
To sum up, the electrophysiological phenomenon of ERD/ERS is the physiological basis for the BCI system to support the brain wave generated by the way of motion imagination as the input signal. This paper also carries out feature extraction based on the difference in the distribution area and intensity of brain wave energy caused by the ERD/ERS phenomenon when the subject is performing imaginative movement, so as to classify the subject's imaginative content.
Research shows that motion imagination is closely related to ERS/ERD phenomenon. Researchers have adopted many different traditional machine learning algorithms to process and analyze MI EEG information. Although these methods have achieved high classification accuracy to a certain extent, they also have many shortcomings. The reason is that traditional machine learning algorithms need a lot of prior knowledge and constantly optimize the parameters of the classifier, which will lead to the whole process becoming more cumbersome, and the final classification accuracy and efficiency will also be greatly reduced. Moreover, EEG signal is a kind of complex nonlinear non-stationary random signal with low signal-to-noise ratio. It will lose some useful information only through traditional machine learning method to process EEG signal. Therefore, it is still a difficult problem for researchers to find more effective methods to recognize EEG signals.
EEG signals are highly uncertain and complex. In recent years, as deep learning [26] has achieved good results in many fields, more and more researchers have begun to use deep learning methods to study EEG signals. BCI establishes an additional pathway between the brain and the external environment. A researcher has created a brain-computer interface dialing system, which has the advantages of non-invasive, real-time, no training, and the adaptability of using back-propagation (BP) neural network to different users. The experimental results show that using BP neural network can improve the accuracy of the dialing system [27]. Deep Belief Network (DBN) [28], CNN [29] and LSTM [30] are all applied in EEG signal classification, and the classification effect is good. Different deep learning methods have different characteristics and advantages. Only by combining them can they all display their own advantages. Therefore, the comprehensive application of multiple methods is the development trend of EEG pattern recognition. Therefore, based on LSTM and CNN neural network, this paper establishes a CNN-LSTM algorithm for EEG signal action classification. The experimental results show that the CNN-LSTM algorithm is superior to other models in the classification of EEG signals, and the stability and accuracy of action classification are also improved. The CNN-LSTM algorithm was proposed by researchers in recent years, and the search for CNN-LSTM showed that the research enthusiasm has been gradually increasing since 2016, and the CNN-LSTM algorithm is rarely used in the field of EEG motion recognition. Therefore, the research on the CNN-LSTM algorithm in EEG motion classification has good research significance.
In this section, a CNN-LSTM neural network model is designed to classify EEG signals into two categories and four categories. Firstly, the feature of EEG signal after signal processing is automatically extracted using one-dimensional convolution neural network; Then, the temporal features are extracted by using the modeling ability of LSTM network on the sequence; Finally, the whole connection layer and softmax layer classify the motor imagery EEG signals into two categories and four categories. This paper adjusts the number of layers in the CNN-LSTM model, the number of neurons in each layer, the value of drop out, and so on by reducing the error between the predicted value and the actual label to optimize the CNN-LSTM neural network model. The specific steps of the CNN-LSTM neural network model are as follows: First, input the EEG data, and then go through three convolution layers for normalization processing, although the pooling layer can reduce the parameters and computation, It also reduces the possibility of over-fitting, but in order to avoid losing data information, no pooling layer is added after the convolution layer. In order to extract timing information, a deep LSTM network with three LSTM layers is behind the three CNN layers, and the last layer is the full connection layer. The activation function is softmax. The CNN-LSTM neural network model is shown in Figure.8.
To extract more effective feature quantity, the signal in the second to eighth second of the experiment is processed to obtain two-dimensional data. In this paper, the feature quantity of 40 experimental data sets will be selected as the training set, and the remaining 20 experimental data feature quantities will be selected as the test set. This paper selects the accuracy rate and Kappa coefficient as the indicators of the evaluation model, and compares the training accuracy rate with the test accuracy rate. In addition, the average correct rate of EEG data obtained from multiple motor imagination tests of three subjects in this experiment is shown in Table 1.
BP | GSA-SVM | CNN-LSTM | |
M01 | 85.83 | 89.17 | 90.83 |
M02 | 87.50 | 89.17 | 92.50 |
F01 | 84.17 | 86.67 | 94.17 |
Then this paper further studies the influence of different subject data sets on the classification effect of the CNN-LSTM model. First, according to the test results in Table 1, the Kappa coefficient is selected as the evaluation standard to evaluate the classification performance of the model, and the Kappa coefficient of the classifier for each test object is calculated and obtained, as shown in Table 2.
BP | GSA-SVM | CNN-LSTM | |
M01 | 0.7167 | 0.7833 | 0.8167 |
M02 | 0.7500 | 0.7833 | 0.8500 |
F01 | 0.6833 | 0.7333 | 0.8833 |
The CNN-LSTM model performs best in the data set of the study object F01 in the classification of EEG signals, with the test accuracy of 94.17% and Kappa coefficient of 0.8833; However, the data set of M01 was the worst, with the test accuracy of only 90.83% and Kappa coefficient of only 0.8167; The average test accuracy of the final three subjects was 92.50%, and the average Kappa coefficient was 0.85. The results show that the accuracy of classification of EEG signals of different subjects by CNN-LSTM model was higher than 90%, and the maximum difference was only about 3%. The CNN-LSTM model classification is superior to the other two-class algorithms, and is much better than the traditional classification methods. The CNN-LSTM model is also more effective than the relevant algorithms mentioned in the introduction. The experiment shows that the CNN-LSTM model has better recognition effect for the second type of EEG signal.
In this paper, relevant algorithms are used to identify the binary data; This section will add two kinds of motor imagination tasks on this basis, namely four categories of questions (left, right, front and rear). This paper sets the left, right, forward and backward action labels as 1, 2, 3 and 4 respectively.
This experiment collects the EEG signals generated by three subjects during the four types of imaginative actions. In the experiment, each experiment period was set as 10 seconds, and the subjects were required to imagine four different types of motion in the time period t = 2~8 seconds. In this experiment, each subject is required to imagine movement for 120 times, that is, 30 times for each movement imagination experiment. Among them, 80 sports imagination experimental data are training samples, and the remaining 40 experimental data are test samples. This paper selects the accuracy rate and Kappa coefficient as the indicators of the evaluation model, and compares the training accuracy rate with the test accuracy rate. In addition, according to the EEG data of multiple motor imagination tests of three test subjects, the average correct rate of four-classification movement recognition of EEG signals obtained in this experiment is shown in Table 3.
BP | GSA-SVM | CNN-LSTM | |
M01 | 85.42 | 88.33 | 89.58 |
M02 | 85.42 | 85.83 | 90.83 |
F01 | 84.79 | 87.50 | 91.25 |
Then this paper further studies the influence of different subject data sets on the classification effect of the CNN-LSTM model. First, according to the test results in Table 3, the Kappa coefficient is selected as the evaluation standard to evaluate the classification performance of the model, and the Kappa coefficient of the classifier for each test object is calculated and obtained, as shown in Table 4.
BP | GSA-SVM | CNN-LSTM | |
M01 | 0.8056 | 0.8444 | 0.8611 |
M02 | 0.8056 | 0.8111 | 0.8742 |
F01 | 0.7972 | 0.8333 | 0.8833 |
CNN-LSTM model performs best in the four-class action recognition of EEG signals, with the test accuracy of 91.25% and Kappa coefficient of 0.8833; However, the data set of M01 was the worst, with the test accuracy of only 89.58% and Kappa coefficient of only 0.8611; The average test accuracy of the final three subjects was 90.55%, and the average Kappa coefficient was 0.8729. The results show that the classification accuracy of the CNN-LSTM model for the four types of motor imagery EEG signals of different subjects is higher than the other two classification algorithms. The CNN-LSTM model has a higher recognition rate in the four-class action recognition of EEG signals than the relevant algorithms mentioned in the introduction. The experimental results show that the model has good recognition effect for four-class of EEG signals.
In this paper, we propose a new method of two-class and four-class motor imagery EEG recognition with higher classification accuracy, which is based on deep learning theory and includes two parts of signal preprocessing and classification recognition. The CNN-LSTM hybrid network model is designed, including feature extraction and feature classification; Finally, the parameters of the model are adjusted through experiments to complete the training of the model. In this paper, the classification performance of the model in two-classification and four-classification is tested on the collected data set, and both have been greatly improved, which also shows that the CNN-LSTM model has good classification effect for two-classification and four-classification EEG signals.
Based on the brain-computer interface motion imagination mode, this paper designs a brain-computer interface experiment scheme. Combining the process of motion imagination and the characteristics of EEG signals, it collects the experimental data of EEG signals, selects the average accuracy rate and kappa coefficient commonly used in classification effect, analyzes the time-frequency characteristics of EEG signals and event-related potential phenomena, and obtains the ERD/ERS characteristics. This paper expounds the relevant theoretical basis of the classification algorithm of EEG signals, and applies three algorithms to the action recognition research of the collected two-class and four-class EEG signals. The experimental results of the two-class and four-class show that the CNN-LSTM neural network model has a good effect, and its average accuracy and kappa coefficient are higher than the other two classification algorithms, which also shows that the classification algorithm selected in this paper has a good classification effect.
The authors declare there is no conflict of interest.
[1] |
A. Venkatakrishnan, G. E. Francisco, J. L. Contreras-Vidal, Applications of brain–machine interface systems in stroke recovery and rehabilitation, Curr. Phys. Med. Rehabil. Rep., 2 (2014), 93–105. https://doi.org/10.1007/s40141-014-0051-4 doi: 10.1007/s40141-014-0051-4
![]() |
[2] |
I. K. Niazi, N. Jiang, M. Jochumsen, J. F. Nielsen, K. Dremstrup, D. Farina, Detection of movement re1ated cortical potentials based on subject independent training, Med. Biol. Eng. Comput., 51 (2013), 507–512. https://doi.org/10.1007/s11517-012-1018-1 doi: 10.1007/s11517-012-1018-1
![]() |
[3] |
A. Presacco, L. W. Forrester, J. L. Contreras-Vidal, Decoding intra-limb and inter-limb kinematics during treadmill walking from scalp electroencephalographic (EEG) signals, IEEE Trans. Neural Syst. Rehabil. Eng., 20 (2012), 212–219. https://doi.org/10.1109/TNSRE.2012.2188304 doi: 10.1109/TNSRE.2012.2188304
![]() |
[4] |
N. A. Fitzsimmons, M. A. Lebedev, I. D. Peikon, M. A. L. Nicolelis, Extracting kinematic parameters for monkey bipedal walking from cortical neuronal ensemble activity, Front. Integr. Neurosci., 3 (2009), 3. https://doi.org/10.3389/neuro.07.003.2009 doi: 10.3389/neuro.07.003.2009
![]() |
[5] |
J. L. Contreras-Vidal, A. Kilicarslan, H. Huang, R. G. Grossman, Human-Centered design of wearable neuroprostheses and exoskeletons, Ai Mag. Artif. Intell., 36 (2015), 12–22. https://doi.org/10.1609/aimag.v36i4.2613 doi: 10.1609/aimag.v36i4.2613
![]() |
[6] | Nidhi, D. Joshi, Terrain-based gait recognition using EEG: Comparing machine learning and deep learning models, in 2021 International Conference on Computational Performance Evaluation (ComPE), (2021), 734–740. https://doi.org/10.1109/ComPE53109.2021.9751957 |
[7] |
S. M. S. Hasan, M. R. Siddiquee, R. Atri, R. Ramon, J. S. Marquez, O. Bai, Prediction of gait intention from pre-movement EEG signals: A feasibility study, J. NeuroEng. Rehabil., 17 (2020), 50. https://doi.org/10.1186/s12984-020-00675-5 doi: 10.1186/s12984-020-00675-5
![]() |
[8] | I. Walker, Deep convolutional neural networks for brain computer interface using motor imaginary, Master thesis, Imperial College London, 2015. |
[9] | L. Clemente, L. Garrido, EEG binary classification using convolutional neural networks, Campus Monterrey, 2016. |
[10] |
Z. C. Tang, K. J. Zhang, C. Li, Classification of motor imagery based on deep convolution neural network and its application in brain-controlled exoskeleton by EEG, J. Comput. Sci., 40 (2017), 12. https://doi.org/10.11897/SP.J.1016.2017.01367 doi: 10.11897/SP.J.1016.2017.01367
![]() |
[11] |
D. J. Leamy, J. Kocijan, K. Domijan, J. Duffin, R. A. Roche, S. Commins, et al., An exploration of EEG features during recovery following stroke-implications for BCI-mediated neurorehabilitation therapy, J. Neuroeng. Rehabil., 11 (2014), 9. https://doi.org/10.1186/1743-0003-11-9 doi: 10.1186/1743-0003-11-9
![]() |
[12] |
E. Hortal, D. Planelles, A. Costa, E. Iáñez, A. Úbeda, J.M. Azorín, et al., SVM-based Brain–Machine Interface for controlling a robot arm through four mental tasks, Neurocomputing, 151 (2015), 116–121. https://doi.org/10.1016/j.neucom.2014.09.078 doi: 10.1016/j.neucom.2014.09.078
![]() |
[13] |
N. Jiang, L. Gizzi, N. Mrachacz-Kersting, K. Dremstrup, D. Farina, A brain-computer interface for single-trial detection of gait initiation from movement related cortical potentials, Clin. Neurophys., 126 (2015), 154–159. https://doi.org/10.1016/j.clinph.2014.05.003 doi: 10.1016/j.clinph.2014.05.003
![]() |
[14] | Z. Jiang, P. Liu, Y. Xia, J. Zhang, Application of CNN in EEG image classification of AD patients, in The 2nd International Conference on Computing and Data Science, 21 (2021), 1–5. https://doi.org/10.1145/3448734.3450473 |
[15] |
D. Garrett, D. A. Peterson, C. W. Anderson, M. H. Thaut, Comparison of linear, nonlinear, and feature selection methods for EEG signal classification, IEEE Trans. Neural Syst. Rehabil. Eng., 11 (2003), 141–144. https://doi.org/10.1109/TNSRE.2003.814441 doi: 10.1109/TNSRE.2003.814441
![]() |
[16] |
T. N. Lal, M. Schröder, T. Hinterberger, J. Weston, M. Bogdan, N. Birbaumer, et al., Support vector channel selection in BCI, IEEE Trans. Biomed. Eng., 51 (2004), 1003–1010. https://doi.org/10.1109/TBME.2004.827827 doi: 10.1109/TBME.2004.827827
![]() |
[17] | F. Lotte, M. Congedo, A. Lécuyer, F. Lamarche, B. Arnaldi, A review of classification algorithms for EEG-based brain–computer interfaces, J. Neural Eng., 4 (2007). https://doi.org/10.1088/1741-2560/4/2/R01 |
[18] |
S. Sonnenburg, G. Rätsch, C. Schäfer, B. Schölkopf, Large scale multiple kernel learning, J. Mach. Learn. Res., 7 (2006), 1531–1565. https://doi.org/10.1007/s10450-006-0008-8 doi: 10.1007/s10450-006-0008-8
![]() |
[19] |
X. Li, X. Chen, Y. Yan, W. Wei, Z. J. Wang, Classification of EEG signals using a multiple kernel learning support vector machine, Sensors, 14 (2014), 12784–12802. https://doi.org/10.3390/s140712784 doi: 10.3390/s140712784
![]() |
[20] |
Y. Zhang, S. Prasad, A. Kilicarslan, J. L. Contreras-Vidal, Multiple kernel based region importance learning for neural classification of gait states from EEG signals, Front. Neurosci., 11 (2017), 170. https://doi.org/10.3389/fnins.2017.00170 doi: 10.3389/fnins.2017.00170
![]() |
[21] |
Neeraj, V. Singhal, J. Mathew, R. K. Behera, Detection of alcoholism using EEG signals and a CNN-LSTM-ATTN network, Comput. Biol. Med., 138 (2021), 104940. https://doi.org/10.1016/j.compbiomed.2021.104940 doi: 10.1016/j.compbiomed.2021.104940
![]() |
[22] |
C. Chen, Z. Du, L. He, Y. Shi, J. Wang, W. Dong, A novel gait pattern recognition method based on LSTM-CNN for lower limb exoskeleton, J. Bionic Eng., 18 (2021), 1059–1072. https://doi.org/10.1007/s42235-021-00083-y doi: 10.1007/s42235-021-00083-y
![]() |
[23] |
L. F. Shi, Z. Y. Liu, K. J. Zhou, Y. Shi, X. Jing, Novel deep learning network for gait recognition using multimodal inertial sensors, Sensors (Basel), 23 (2023), 849. https://doi.org/10.3390/s23020849 doi: 10.3390/s23020849
![]() |
[24] |
J. Gao, P. Gu, Q. Ren, J. Zhang, X. Song, Abnormal gait recognition algorithm based on LSTM-CNN fusion network, IEEE Access, 7 (2019), 163180–163190. https://doi.org/10.1109/ACCESS.2019.2950254. doi: 10.1109/ACCESS.2019.2950254
![]() |
[25] |
G. Pfurtscheller, F. H. L. da Silva, Event-related EEG/MEG synchronization and desynchronization, Clin. Neurophysiol., 110 (1999), 1842–1857. https://doi.org/10.1016/s1388-2457(99)00141-8 doi: 10.1016/s1388-2457(99)00141-8
![]() |
[26] |
Y. LeCun, Y. Bengio, G. Hinton, Deep learning, Nature, 521 (2015), 436–444. https://doi.org/10.1142/S1793351X16500045 doi: 10.1142/S1793351X16500045
![]() |
[27] | D. Lin, F. Duan, W. Li, J. Shen, Q. M. Wang, X. Luo, Optimizing the individual differences of EEG signals through BP neural network algorithm for a BCI dialing system, in International Conference on Brain & Health Informatics, (2013), 479–488. https://doi.org/10.1007/978-3-319-02753-1_48 |
[28] | X. An, D. Kuang, X. Guo, Y. Zhao, L. He, A deep learning method for classification of EEG data based on motor imagery, in International Conference on Intelligent Computing, (2014), 203–210. https://doi.org/10.1007/978-3-319-09330-7_25 |
[29] |
S. U. Amin, M. Alsulaiman, G. Muhammad, M. A. Mekhtiche, M. S. Hossain, Deeplearning for EEG motor imagery classification based on multi-layer CNNs feature fusion, Future Gener. Comput. Syst., 101 (2019), 542–554. https://doi.org/10.1016/j.future.2019.06.027 doi: 10.1016/j.future.2019.06.027
![]() |
[30] | N. Nazari, S. A. Mirsalari, S. Sinaei, M. E. Salehi, M. Daneshtalab, Multi-level binarized LSTM in EEG classification for wearable devices, (2020), 175–181. https://doi.org/10.1109/PDP50117.2020.00033 |
1. | Yilong Huang, Lingling Yang, Zeqiang Lin, 2023, Gait Synergy Modeling and Joint Angle Prediction Based on LSTM, 979-8-3503-2800-4, 166, 10.1109/RAIIC59453.2023.10281040 | |
2. | Huichao Duan, Aihui Wang, Shengda Gao, Hengyi Li, Xuebin Yue, Yan Wang, 2024, Analysis of Motion Intention Prediction Based on Human Gait Data, 979-8-3503-6860-4, 4949, 10.1109/CAC63892.2024.10865184 |
BP | GSA-SVM | CNN-LSTM | |
M01 | 85.83 | 89.17 | 90.83 |
M02 | 87.50 | 89.17 | 92.50 |
F01 | 84.17 | 86.67 | 94.17 |
BP | GSA-SVM | CNN-LSTM | |
M01 | 0.7167 | 0.7833 | 0.8167 |
M02 | 0.7500 | 0.7833 | 0.8500 |
F01 | 0.6833 | 0.7333 | 0.8833 |
BP | GSA-SVM | CNN-LSTM | |
M01 | 85.42 | 88.33 | 89.58 |
M02 | 85.42 | 85.83 | 90.83 |
F01 | 84.79 | 87.50 | 91.25 |
BP | GSA-SVM | CNN-LSTM | |
M01 | 0.8056 | 0.8444 | 0.8611 |
M02 | 0.8056 | 0.8111 | 0.8742 |
F01 | 0.7972 | 0.8333 | 0.8833 |
BP | GSA-SVM | CNN-LSTM | |
M01 | 85.83 | 89.17 | 90.83 |
M02 | 87.50 | 89.17 | 92.50 |
F01 | 84.17 | 86.67 | 94.17 |
BP | GSA-SVM | CNN-LSTM | |
M01 | 0.7167 | 0.7833 | 0.8167 |
M02 | 0.7500 | 0.7833 | 0.8500 |
F01 | 0.6833 | 0.7333 | 0.8833 |
BP | GSA-SVM | CNN-LSTM | |
M01 | 85.42 | 88.33 | 89.58 |
M02 | 85.42 | 85.83 | 90.83 |
F01 | 84.79 | 87.50 | 91.25 |
BP | GSA-SVM | CNN-LSTM | |
M01 | 0.8056 | 0.8444 | 0.8611 |
M02 | 0.8056 | 0.8111 | 0.8742 |
F01 | 0.7972 | 0.8333 | 0.8833 |