Special Issue: Medical e-Diagnosis by Fusing Deep Learning and Big Data Analytics
Guest Editors
Prof. Simon James Fong
Department of Computer and Information Science
University of Macau, Macau SAR
Email: ccfong@umac.mo
Dr. Gloria Tengyue Li
DACC Laboratory, ZIAT, Zhuhai Chinese Academic of Science, Zhuhai, China
Email: gloria.l@ziat.ac.cn
Manuscript Topics
Medical e-Diagnosis boomed recently in research and applications as empowered by advances of artificial intelligence-based tools which enhanced many aspects in medical diagnostics. By far, these new frontiers have emerged with their unique advantages, solving different medical problems. Individually, they have been applied as a crucial machine learning tool in the field of computer vision, deep learning, AI decision making, and big data analytics in a wide range of medical e-diagnosis. In the field of medical image analysis, deep learning has been one of the most popularly applied tools pertaining to classification, detection, and segmentation. With the emergence of these technological frontiers and rapid adoption of AI-based medical imaging technology, medical image analysis has entered the era of big data, being able to extract and infer useful knowledge from a large volume of medical images while supporting clinical diagnosis with improved accuracy and interpretability. The application of deep learning has opened up a new chapter in facilitating automated analysis of images and enabling medical experts in achieving high-precision intelligent e-diagnosis.
On the other hand, Internet of Medical Things (IoMT) has emerged as another important real-time data-driven e-diagnosis that operate on different sensors and AI algorithms. Lots of progress from recent years spurred over innovations on analyzing sequences of patterns, smart decision support, and big time-series analysis contribute to a new level of paradigm shift in promoting accurate e-diagnosis. With the new enhancement in analysis performance, the medical data platform for e-diagnosis based on IoMT can be connected with the latest cloud and fog networks, to build a holistic medical system for remote monitoring of people’s live, for both prevention, diagnosis and prognosis. This compliments very well as a full process at measuring vital signals from users at one end, while the other end embraces deep learning AI based image diagnosis and prognosis, like two sides of a hand in monitoring users’ health. Big data analytics and processing platform can realize the real-time information interaction between doctors and patients, which assist the physicians through the assistance in effective decision-making from both image-oriented and sensor-oriented channels. Furthermore, several well-established optimization techniques including particle swarm algorithm, genetic algorithm, elephant herding optimization have been incorporated into IoMT to enhance their performances in healthcare. Similarly, such optimization techniques have been widely used in image-based deep learning where the hyperparameters of complex convolution neural network can be fine-tuned to provide the best performance. Hence, the efficient incorporation of these optimization techniques with AI could help address the limitations faced by the existing AI-based solutions across the medical e-diagnosis, in both sensor- and image-based e-diagnosis with a strong support of big data analytics at the backend.
Each of the above-mentioned frontiers have their unique strengths in some areas. There would be a convergence now or in the near future, tapping the best of each frontier, complementing each other, for unprecedented multiplicative and collaborative effects.
As such, this special issue is aimed at exploring fusion ideas, designs, novel concepts, solutions, and current practices for fostering technological advancements across the medical e-diagnosis, by considering integration of deep learning, AI, big data analytics as well as optimization techniques over machine learning and IoMT. We welcome original and high-quality works representing conceptual advancements towards solving challenging medical problems, by covering fusion techniques, architectures, machine/deep learning paradigms, hybrid models of image- and sensor-based e-diagnosis.
The topics of interest include, but are not limited to the following:
• Hybrid e-diagnosis, models, solution, and techniques
• Optimized AI-based medical e-diagnosis
• Optimized AI-based IoMT systems for healthcare
• Optimized AI-based deep learning systems for healthcare
• Innovative frameworks of deep learning and big data for e-diagnosis
• Optimized machine learning based algorithms
• Optimized pattern recognition-based systems
• Biomedical signal measurement/sensing and big data processing systems
• AI-driven rehabilitation and assistive robotic systems with big data
• Intelligent medical e-diagnosis driven system: present and future
Keywords: Information Fusion; Model Fusion; Hybrid System; e-Diagnosis, Deep Learning; IoMT; Big Data Analytics; Medical Frontiers
Instructions for authors
https://www.aimspress.com/mbe/news/solo-detail/instructionsforauthors
Please submit your manuscript to online submission system
https://aimspress.jams.pub/
Paper Submission
All manuscripts will be peer-reviewed before their acceptance for publication. The deadline for manuscript submission is 31 March 2023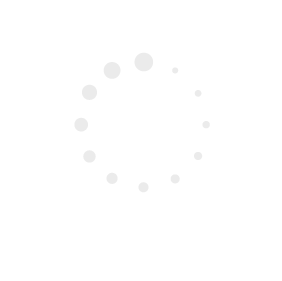