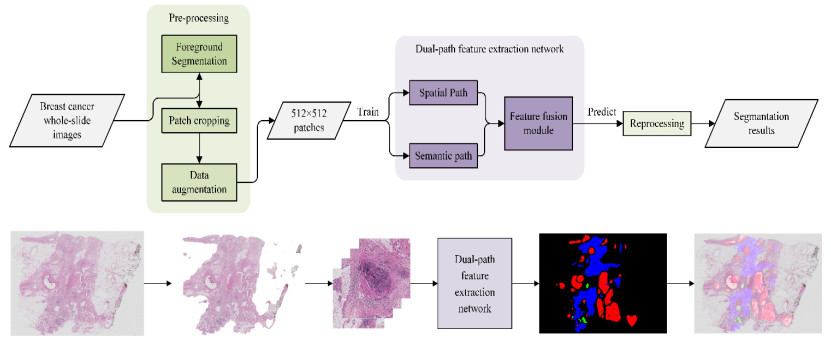
The traditional manual breast cancer diagnosis method of pathological images is time-consuming and labor-intensive, and it is easy to be misdiagnosed. Computer-aided diagnosis of WSIs gradually comes into people*s sight. However, the complexity of high-resolution breast cancer pathological images poses a great challenge to automatic diagnosis, and the existing algorithms are often difficult to balance the accuracy and efficiency. In order to solve these problems, this paper proposes an automatic image segmentation method based on dual-path feature extraction network for breast pathological WSIs, which has a good segmentation accuracy. Specifically, inspired by the concept of receptive fields in the human visual system, dilated convolutional networks are introduced to encode rich contextual information. Based on the channel attention mechanism, a feature attention module and a feature fusion module are proposed to effectively filter and combine the features. In addition, this method uses a light-weight backbone network and performs pre-processing on the data, which greatly reduces the computational complexity of the algorithm. Compared with the classic models, it has improved accuracy and efficiency and is highly competitive.
Citation: Xi Lu, Xuedong Zhu. Automatic segmentation of breast cancer histological images based on dual-path feature extraction network[J]. Mathematical Biosciences and Engineering, 2022, 19(11): 11137-11153. doi: 10.3934/mbe.2022519
[1] | Haiyan Song, Cuihong Liu, Shengnan Li, Peixiao Zhang . TS-GCN: A novel tumor segmentation method integrating transformer and GCN. Mathematical Biosciences and Engineering, 2023, 20(10): 18173-18190. doi: 10.3934/mbe.2023807 |
[2] | Kun Lan, Jianzhen Cheng, Jinyun Jiang, Xiaoliang Jiang, Qile Zhang . Modified UNet++ with atrous spatial pyramid pooling for blood cell image segmentation. Mathematical Biosciences and Engineering, 2023, 20(1): 1420-1433. doi: 10.3934/mbe.2023064 |
[3] | Thaweesak Trongtirakul, Sos Agaian, Adel Oulefki . Automated tumor segmentation in thermographic breast images. Mathematical Biosciences and Engineering, 2023, 20(9): 16786-16806. doi: 10.3934/mbe.2023748 |
[4] | Xi Lu, Zejun You, Miaomiao Sun, Jing Wu, Zhihong Zhang . Breast cancer mitotic cell detection using cascade convolutional neural network with U-Net. Mathematical Biosciences and Engineering, 2021, 18(1): 673-695. doi: 10.3934/mbe.2021036 |
[5] | Jinzhu Yang, Meihan Fu, Ying Hu . Liver vessel segmentation based on inter-scale V-Net. Mathematical Biosciences and Engineering, 2021, 18(4): 4327-4340. doi: 10.3934/mbe.2021217 |
[6] | Venkatesan Rajinikanth, Seifedine Kadry, Ramya Mohan, Arunmozhi Rama, Muhammad Attique Khan, Jungeun Kim . Colon histology slide classification with deep-learning framework using individual and fused features. Mathematical Biosciences and Engineering, 2023, 20(11): 19454-19467. doi: 10.3934/mbe.2023861 |
[7] | Chelsea Harris, Uchenna Okorie, Sokratis Makrogiannis . Spatially localized sparse approximations of deep features for breast mass characterization. Mathematical Biosciences and Engineering, 2023, 20(9): 15859-15882. doi: 10.3934/mbe.2023706 |
[8] | Sushovan Chaudhury, Kartik Sau, Muhammad Attique Khan, Mohammad Shabaz . Deep transfer learning for IDC breast cancer detection using fast AI technique and Sqeezenet architecture. Mathematical Biosciences and Engineering, 2023, 20(6): 10404-10427. doi: 10.3934/mbe.2023457 |
[9] | Jun Gao, Qian Jiang, Bo Zhou, Daozheng Chen . Convolutional neural networks for computer-aided detection or diagnosis in medical image analysis: An overview. Mathematical Biosciences and Engineering, 2019, 16(6): 6536-6561. doi: 10.3934/mbe.2019326 |
[10] | Jian-xue Tian, Jue Zhang . Breast cancer diagnosis using feature extraction and boosted C5.0 decision tree algorithm with penalty factor. Mathematical Biosciences and Engineering, 2022, 19(3): 2193-2205. doi: 10.3934/mbe.2022102 |
The traditional manual breast cancer diagnosis method of pathological images is time-consuming and labor-intensive, and it is easy to be misdiagnosed. Computer-aided diagnosis of WSIs gradually comes into people*s sight. However, the complexity of high-resolution breast cancer pathological images poses a great challenge to automatic diagnosis, and the existing algorithms are often difficult to balance the accuracy and efficiency. In order to solve these problems, this paper proposes an automatic image segmentation method based on dual-path feature extraction network for breast pathological WSIs, which has a good segmentation accuracy. Specifically, inspired by the concept of receptive fields in the human visual system, dilated convolutional networks are introduced to encode rich contextual information. Based on the channel attention mechanism, a feature attention module and a feature fusion module are proposed to effectively filter and combine the features. In addition, this method uses a light-weight backbone network and performs pre-processing on the data, which greatly reduces the computational complexity of the algorithm. Compared with the classic models, it has improved accuracy and efficiency and is highly competitive.
According to the latest global cancer data released by International Agency for Research on Cancer (IARC), female breast cancer has surpassed lung cancer as the most commonly occurring cancer worldwide for the first time [1]. Even though, the mortality rate of breast cancer has been steadily decreasing in recent years, especially in developed countries. The contrast is due in large part to the gradual improvement of clinical treatment methods which depend on early detection and correct diagnosis. Histological examination of biopsy specimen is considered to be the golden standard for breast cancer detection and affirmation [2]. Traditionally pathologists perform operations such as focusing, zooming and panning on the slices of breast tissue under an electronic or light microscope in order to observe and analyze the density and shape of cells, and the structure and morphology of local tissues at different magnifications. Accordingly, whether cancer occurs and the location and extent of the lesions could be determined, and further treatments could be made [3].
With the increasing number of breast cancer patients, the traditional diagnosis method based on manual observation is facing huge challenges. Firstly, observation through microscopes consumes substantial time and effort owing to the complexity and diversity of breast tissue, thus leading to a percentage of misdiagnosis [4]. Furthermore, the average diagnostic concordance among different pathologists' individual interpretations based on a single breast biopsy slide reaches only 75.3% [5] affected by both objective factors such as different staining methods and equipment batches, and subjective factors including diversity of pathologists' personal clinical experience and evaluation criteria as well [6,7]. Such challenges are unfavorable for the determination of therapy courses, which may bring about overtreatment or undertreatment and further lead to harm or even death of patients. Therefore, there is urgent need for an objective, accurate and effective auxiliary system to automatically process histological images and assist the pathologists to make wiser diagnosis and treatment options.
More recently, thanks to the tremendous progress of digital pathology technology and machine learning, computer-aided diagnosis (CAD) has become possible [8]. This paper focuses on the semantic segmentation of whole-slide images (WSIs). In the light of the proliferation degree of breast tumor, each pixel in the WSIs is classified into 4 predominant cancer types: normal, benign, in situ carcinoma and invasive carcinoma, thus WSIs are automatically divided into four regions respectively. The automatic segmentation result could provide pathologists a pixel-wise understanding of WSIs and a scientific basis for correct and fast diagnosis.
In this paper, we propose an end-to-end automatic segmentation system for breast cancer histological images. The main contributions of this paper are as follows:
1) We adopt the dual-path structure to the semantic segmentation of histological images for the first time and modify it to solve the high-resolution and semantically complicated WSIs of breast cancer, consequently improving the segmentation accuracy and efficiency.
2) We introduce dilated convolution structure in the spatial path and draw the attention mechanism into the semantic path as well as the feature fusion module, reconciling the rich context of WSIs with detailed information, enabling the network with powerful feature acquisition and discrimination ability.
3) The pre-processing operation used alleviates the data capacity and the light-weight backbone structure of our network lows the model complexity, which greatly speeds up the segmentation of high-resolution WSIs and further makes automatic diagnosis entering the clinic possible.
The rest of the paper is organized as follows. Section 2 suggests and analyzes the related works of automatic segmentation of medical images. In Section 3, the dataset we used and the steps of automatic segmentation are declared in detail. Section 4 describes experimental details and displays the results. Section 5 makes a discussion on the results. Finally, Section 5 makes a summary on the paper.
The development process of automatic segmentation of pathological images can be roughly divided into two stages, based on the different basic theory used and various methods of feature extraction [9].
In the first stage, researchers employed traditional machine learning algorithms along with handcrafted features of pathological images such as texture, spatial structure and colors. A lot of classical algorithms of feature extraction emerged during this period. Naik et al. [10] used a level-set algorithm to identify the feature of boundaries and a template matching algorithm with shape models to identify glands and nuclei. The accuracy obtained 87% when the algorithm was used to discriminate cancer form non-cancer in breast histology. Jung and Kim [11] proposed a watershed-based method for segmentation of cervical and breast cell images, and introduced ellipsoidal modeling of contours to adjust nuclei contours. Kowal et al. [12] used four different clustering approaches including K-means, fuzzy C-means, competitive learning neural networks and Gaussian mixture models to each extract 42 morphological, topological and texture features. Next these features were put into three different classifiers to decide whether cancer occurred. Belsare et al. [13] proposed a similarity based super pixel generation method and integrate it with texton representation to form spatio-color-texture map of breast histology image. This approach segmented nuclear arrangement in normal or malignant duct. Compared to manual segmentations, the above methods did demonstrate good accuracy on early pathological datasets. However, these operations are time-consuming and computationally expensive [14], and cannot work without professional pathological knowledge. Besides, as the resolution and scale of datasets continue to improve, the low-level visual feature information that is easy to be observed by human eyes no longer meet the requirements, and abstract and high-level features are difficult to be captured. Meanwhile, the limitations of conventional algorithms also lead to poor robustness of the model, which seriously restrict the evolution of medical image segmentation.
In the second stage, deep learning (DL), especially convolutional neural network (CNN) begins to play an important role in the field of computer vision [15]. CNNs can automatically learn useful features from training data by optimizing the loss function, which not only avoids the complexity and limitation of handcrafted features, but also enables image segmentation models better robustness and versatility. For the past few years, CNNs have been widely used in medical image segmentation and have made excellent achievements. Ciresan et al. [16] classified patches with each pixel as the center, and take the classification result as the category of the pixel to get a pixel-wise classification image so that the segmentation completed. This method ranked first in the ICPR2012 mitosis detection competition. But the pixel-by-pixel computation causes high overlapping and wasting, which is not suitable for giga-pixel WSIs. Litjens et al. [17] converted CNN to a fully convolutional network (FCN) and applied it to the WSI to get a likelihood map where each pixel has a continuous potential between 0 and 1 of containing cancer. Compared with pixel-by-pixel method [16], FCN employed transposed convolution instead of simple upsampling and achieved end-to-end segmentation in a more convenient and faster way. In 2015, U-net, one of the most frequently used models in medical field, was proposed by Ronneberger et al. [18]. U-net was an encoder-decoder model, which captured global features on the contraction path and realize accurate location on the extension path, so as to reserve both low-level and high-level information of images. The segmentation accuracy was greatly improved and a lot of state-of-art schemes were the modified version of the encoder-decoder structure. Ho et al. [19], using U-net as the single-magnification network, proposed a multi-encoder multi-decoder multi-concatenation architecture for breast cancer images at different magnifications and segmentated the WSIs into carcinoma, benign epithelial, background, stroma, necrotic and adipose. Anand et al. [20] raised a ResU-Net structure for breast tumor segmentation where residual connections were added to address vanishing gradient. Chen et al. [21] proposed Deeplab which introduced dilated convolution for the first time. Without increasing the amount of calculation, Deeplab can obtain more contextual information, which won the first place in PASCAL VOC competition. The improved Deeplab v3+ was introduced to recognize and segmentate gastric cancer [22], and has proven its worth in automatic medical image processing.
Despite the above studies to some extent achieved satisfactory results, semantic segmentation on breast cancer images still has a long way to go. Most of the studies to date focus on binary classification such as detection of cancer areas, and classification of benign and malignant. Multi-classification concerning the malignant degree of tumor is more clinically valuable because it provides more specific grading information, where interclass difference is much smaller and needs multiscale features integrating more detailed information. Moreover, most of the existing models have complicated structure and a large number of parameters, leading to high memory consumption and low inference speed, which is contrary to the original intention of aiding diagnosis in an efficient way. Considering the above problems, we refer to Bilateral Segmentation Network (BiSeNet) [23] with excellent performance on remote sensing images and proposed a dual-path feature extraction network for breast pathological image segmentation.
In this section, we first display the dataset used in this paper. Then, the pre-processing operations of the whole-slide images (WSIs) are stated. In the last subsection, the architecture of the proposed segmentation model is presented and each module of the model is described in detail.
This paper adopts the standard dataset from ICIAR 2018 Grand Challenge on BreAst Cancer Histology images (BACH) Part B [24]. All the whole-slide images (WSIs) of this dataset were collected from hematoxylin-eosin (H & E) stained slices of breast cancer patients during 2013–2015, in Castelo Beanco, Portugal. The acquisition system was Leica SCN400. WSIs are released in the form of. svs, with a pixel scale of 0.467/pixel. Each image has a different size, with width ∈ [39980, 62952] (pixels) and height ∈ [27972, 44889] (pixels). Ten WSIs out of 30 were annotated by a pathologist and revised by a second one, where there was disagreement were discarded. Benign, in situ carcinoma and invasive carcinoma regions are pixel-wise annotated, and the rest is considered as normal tissue. The corresponding ground truth of each image was provided in the form of.xml files, containing a corresponding set of labelled coordinates of each kind of region.
WSIs comprise breast tissue as well as none-tissue regions. To focus on the tissue area and speed up the following process, this paper designs a method to extract the foreground area from WSI. In the H & E-stained slices, the cell nuclei are purple and the cytoplasm is pink, while the non-tissue area is grayish-white [25]. It is obvious that the more colorful the region, the more likely it is to be a tissue region. In other words, the more inconsistent the RGB channel values of the image, the more it would be preserved.
(1) |
The binary image is obtained by thresholding the range of the RGB channels. As shown in Figure 2, the colorful area is filtered out so that the binary mask is made and the useless none-tissue areas are removed.
WSIs cannot be directly input to the neural network because the existing graphics card cannot support an array of giga-pixel images. What's more, the length and width of the images from ICIAR2018 are not equal. If WSIs are forced into squares, 1/4 of the information will be lost and distortion may occur. Accordingly, we crop WSIs into patches with a size of 512 × 512. The patch of this size is not only large enough to envelop plenty diagnostic information and prevent the imbalance of training categories that may cause overfitting, but also small enough to concentrate on cell-level details and avoid too much memory and time consuming [18]. Finally, considering the great gap in the proportion of samples (normal regions are much more than the other three), we performed data augmentation on the training set to ensure uniform distribution and prevent overfitting [26].
The framework of our network is shown as Figure 3. Two paths are proposed to capture and encode different levels of feature information of breast cancer images, a feature fusion model (FFM) is followed. To realize the end-to-end segmentation, we adopt 8 unsampling operations on the joint spatial-semantic feature. At last, a Softmax function is conducted to supervise the output of the network. The rest of this section specifically illustrates each part of the network.
The structure of spatial path is shown as Figure 4. Firstly, three ordinary convolutions were performed on the pre-processed patch with a kernel size of 3 × 3 and a stride of 2, producing a feature map whose size is 1/8 of the original patch, which directly composes 1/4 of the output of spatial path as identity mapping by residual connection.
We introduce three parallel branches based on the structure of RFB [27], which respectively consist of different size of kernels and dilated convolution layers with various dilation rates. From top to bottom: The first branch consists of a 1 × 1 convolution kernel, a 3 × 3 dilated convolution kernel with dilation rate set to 1, equivalent to a 1 × 1 receptive field; The middle branch compromises a 3 × 3 convolution kernel together with a 3 × 3 dilated convolution kernel with dilation rate set to 3, equivalent to a 1 × 1 receptive field. The third branch introduced two 3 × 3 convolutional layers instead of a 5 × 5 kernel, followed by a 3 × 3 dilated convolution kernel with dilation rate set to 3.
Eventually, the three feature maps extracted by the three parallel branches are concatenated together with the identity mapping, forming the output of spatial path. The output feature map with the size of (64, 64,128) includes multi-scale contextual information and further prepares high-resolution low-level spatial information for the segmentation task.
In contrast to spatial path, semantic path aims at the extraction of high-level semantic information, as it is shown in Figure 5. Xception39 [28] is explored as the backbone network, which is a depthwise separable convolution network designed on the basis of InceptionV3. It is light-weighted compared with other networks [17,18,19,21] commonly used in medical images thus effectively reducing training costs. Xception39 was then is followed by a global average pool to integrate global context information.
Every channel of the feature map corresponds to a specific semantic feature response, while only discriminative features do help with segmentation results [29]. Therefore, this paper designs a feature attention module which aims to pick out discriminative features from the last two stages of Xception36. This module is based on the channel attention mechanism which can capture channel-wise dependencies and put emphasis on salient objects to suppress the redundant discriminative features.
The structure of feature attention module is shown in Figure 6. Compared to SE-Block [29], we conduct both global average and max pool on the input feature map and generating two 1D feature vectors. Then the two vectors are concatenated and then reduced the channel number to 1/16 by a 1 × 1 convolution layer. ReLU function introduces non-linearity after the channel reduction. Then the channel expanded via a 1 × 1 convolution layer to 128, and a sigmoid function is utilized to activate the convolution result so that 1D attentive weight WFAM could be obtained. The value of each element of WFAM is constrained between 0 and 1. The input feature map of feature attention module passes through a 1 × 1 projection layer which calibrates the number of channels to 128, and finally a channel-wise multiplication is performed with attentive weight WFAM to generate the output feature map of feature attentive module.
Back to the bottom of Figure 5, semantic path step-wise combines upsampled output feature maps from the global pool layer and the last two stages of the semantic path.
The features extracted by the two paths have different levels of information. The spatial path captures rich low-level detailed spatial information, while the semantic path encodes high level semantic information. If two different levels of information are integrated forcibly, the huge gap between them may lead to terrible segmentation results. Therefore, a feature fusion module must be proposed to narrow the gap. In our model, the feature fusion module is also based on the channel attention mechanism, as shown in Figure 7.
We first element-wise add two output feature maps from two paths as a preliminary fusion. Then a global average pooling is operated. Next two fully-connected convolution (FC) layers are performed on the 1D feature vector for channel reduction and expansion, which respectively follow the two activation functions of ReLU and Sigmoid. The 1D weight vector WFFM is generated by the preliminary fusion, so it can effectively narrow the gap between high-level and low-level feature information, and guide feature selection. By the means of channel-wise multiplying WFFM with output feature maps from spatial and semantic path, feature representations important for segmentation are adaptively selected and discriminative ones are inhibited. Eventually, the two reweighted feature map are concatenated to generate the final fusion output, which is the end of the whole dual-path feature extraction.
The entire semantic segmentation experiment is completed under the Keras framework built under windows 10. The experimental platform established by Inter ® Xeon E5-2620 v4 CPU 2.4 GHz, and NVIDIA RTX2080 GPU with 8 G GPU memory.
There are 10 pixel-wise annotated WSIs in ICIAR2018 BACH dataset. We use 8 of them for training and 2 for verification. According to the label type of the image center pixel, 2000 normal, 2000 benign and 2000 in situ carcinoma, and 2000 invasive carcinoma patches were randomly extracted from each WSI to form the training dataset. In order to prevent model overfitting [30], all the patches are rotated by 90°, 180° and 270° each, so that the scale of training data is expanded to 4 times the original.
The initial learning rate of the model is set to 0.00 01, and the Adam optimizer is selected for iterative optimization. Small batch stochastic gradient descent algorithm is used. The batch size is set to 4, and the epoch is set to 500. In addition, in order to avoid model overfitting, the regularization method of early termination is used in the training process.
In the segmentation of breast cancer whole-slide images, we adopt three metrics to evaluate the accuracy including Intersection over Union (IoU), mean Intersection over Union (mIoU), and Pixel Accuracy (PA), together with two metrics to estimate effectiveness of the algorithm, involving calculation time and model complexity.
Pixel accuracy (PA) is the ratio of the sum of all correctly classified pixels to the total number of pixels, which is defined as follows:
(2) |
The intersection over union (IoU) of each category is the ratio of the number of real samples to the sum of the numbers of real samples, false negative samples and false positive samples in this category. And mean Intersection over Union (mIoU) averages the IoU of all categories, which is defined as follows:
(3) |
To evaluate the proposed model, we compare the segmentation performance with three other methods based on deep learning, including FCN-32s [17], U-Net [18], and Deeplab [21].
As shown in Table 1, The PA and mIoU scores of the semantic segmentation method proposed in this paper are higher than those of other networks. At the same time, the IoU score of our model in benign, in situ carcinoma and invasive carcinoma is the highest in all networks, and the IoU of normal tissue is slightly lower than that of the best Deeplab network. However, the basic purpose of CAD is to automatically identify the cancer area, which means it is more critical to accurately segment cancerous regions (benign, in situ, and invasive) than normal tissues. Therefore, in terms of overall accuracy, the experimental results on ICIAR2018 BACH dataset strongly verify the effectiveness of the proposed networks' feature capture ability and model performance.
Model | IoU (%) | mIoU (%) | PA (%) | |||
Normal | Benign | In Situ | Invasive | |||
FCN-32s | 56.28 | 59.61 | 57.78 | 61.33 | 58.75 | 72.30 |
U-net | 64.68 | 60.75 | 59.87 | 65.78 | 62.77 | 78.76 |
Deeplab | 68.82 | 62.52 | 64.34 | 64.8 | 65.12 | 82.48 |
Our net | 68.20 | 66.80 | 65.22 | 72.26 | 68.12 | 87.02 |
The fundamental purpose of computer-aided diagnosis is to improve the diagnosis efficiency of pathologists. The blind pursuit of segmentation accuracy while ignoring the calculation cost cannot put the algorithm into practical use. Therefore, efficiency is also an important evaluation index of the semantic segmentation model. As we can see in Table 2, the parameter amount of the FCN is large, and the training time is the longest. Although Deeplab and U-net have nice performance, due to the complex network structure, the running speed is also low. The light-weight network proposed in this paper reaches a better segmentation performance without increasing the number of parameters, and the calculation speed is obviously faster than the other three networks.
Model | Model complexity | Calculation time (min) |
FCN-32s | 54 | 10 |
U-net | 2 | 6–8 |
Deeplab | 1 | 3–5 |
Our net | 0.7 | 2–3 |
Figure 8 shows the comparison between our network and other networks. The superiority of our structure is reflected in the following aspects:
1) The dual-path framework in feature extraction exactly meets the requirements of breast tissue classification. The semantic path can extract high-level semantic features, and the spatial path can supplement the underlying spatial information accordingly, which can fully extract the rich information at the cell level as well as tissue level in the pathological image of breast cancer.
2) Dilated convolution is utilized to enhance the capability of encoding multi-scale contexts and thus resolve high intra-class differences in breast tissue.
3) Feature attention module based on channel attention reinforces representational ability of semantics and thus address low inter-class variations in breast tissue.
4) Channel attention mechanism efficiently fusion the low-level and high-level features. Two levels of feature fused preliminarily and then direct the low-level feature to restore semantic predictions, and also provide high-level feature with contextual particulars. This fusion method closes the distance of feature maps from dual paths and combines them more wisely.
When applied in practice, the load of high-resolution histological images along with large model complexity not only cannot be an effective auxiliary diagnosis tool, but also may become a burden to GPU/CPU memory and put forward higher requirements on the performance of computers in hospitals. So, efficiency is definitely a vital factor when segmentation system is devised. Our method has obvious advantage over other state-of-time models on ICPR2018 dataset as it is suggested in Section 4.3.2. There are mainly three following key concepts attributing to the high efficiency of our segmentation system:
1) The foreground segmentation based on thresholding operated in the pre-processing stage removes nontissue areas in WSIs, which considerably reduces the data that needs to be processed later.
2) In the spatial path of the proposed network, shallow layers are adapted and channel capacity is cautiously arranged to ensure no added model complexity and no loss of accuracy at the same time.
3) The semantic path needs a deep-layer network to capture high-level feature maps. We utilized the light-weight backbone network Xception to alleviate the computational burden caused by deep networks.
To investigate the property of every single part in the proposed network and further maximize the benefits of the model, we conducted separate experiments targeting at different modules.
Firstly, in the spatial path, the three parallel branches involve dilated convolutional layers with three dilated rates. It is elaborated in chapter 3.3.1 that the eccentricity of the receptive field is controlled by dilated rates, which inevitably become the key parameters determining the model performance. Therefore, we decide three sets of dilation rates {1, 3, 5}, {1, 2, 3}, {5, 6, 7} to see their impact on our segmentation model. The results shown in Table.3 confirm that the dilated rate {1, 3, 5} is favorable for the proposed breast cancer segmentation algorithm.
Dilation rates | PA (%) | mIOU (%) |
{1, 3, 5} (Our method) |
87.02 | 68.12 |
{1, 2, 3} | 86.98 | 68.02 |
{5, 6, 7} | 86.79 | 67.93 |
While in the semantic path, the feature attention module plays the dominant role in extracting and selecting discriminative features. Therefore, the ablation study carried out by removing it and replacing it with an alternative module SE-block as shown in Table 4. The accuracy decreased significantly when the channel attention module is not introduced. Our feature attention module is inspired by SE-block [29] but presents a slightly better performance, probably owing to the addition of max pooling operation that aims at selecting the local distinctive feature and thus having a better channel-wise inference of feature mapping highlights.
Channel attention modules in semantic path | PA (%) | mIOU (%) |
No channel attention module | 84.72 | 65.33 |
SE-Block [29] | 86.54 | 68.04 |
Feature attention module (Our method) | 87.02 | 68.12 |
In addition, we also display the ablation analysis using the feature fusion method. We compare the channel–attention-mechanism-based feature fusion module of our network with element-wise addition and concatenation as it is shown in Table 5. Element-wise addition and concatenation could to some extent reduce the model complexity, but show poor segmentation effects, especially in terms of pixel accuracy (PA) score. Our method suggests a significant improvement of model performance, which shows effectiveness in integrating different levels of information from different paths.
Feature fusion method | PA (%) | mIOU (%) |
Element-wise addition | 85.47 | 67.38 |
concatenation [29] | 85.50 | 67.95 |
Channel–attention-based feature fusion module (Our method) | 87.02 | 68.12 |
It can be seen from Figure 8 that although the method proposed in this paper is ahead of other algorithms in terms of segmentation effect, there are still many holes in particular regions, and the boundary is not smooth and clear enough. After analysis, it is found that the main reason is that the 512 × 512 image block cropping operation limits the available context of the convolutional neural network to a certain extent, making the network unable to obtain a larger range of organizational features, and the final segmentation result lacks global shape information. In view of the above problems, this paper implies a conditional random field (CRF) [20] to optimize the images, and the final results of mapping on the original WSIs are shown in Figure 9. The segmented area tends to be complete and smooth, which is closer to the ground truth. Table 6 shows the changes of accuracy and efficiency before and after reprocessing. It can be seen that the morphological pre-processing has a slightly positive impact on the segmentation accuracy, but there is a loss in costing time.
Reprocessing | PA (%) | MIoU (%) | Calculation time (min) |
before | 87.02 | 68.12 | 2–3 |
after | 87.74 | 68.53 | 3–5 |
The automatic segmentation of different regions of breast tissue pathological images is of great significance to the computer-aided diagnosis of breast cancer, and can provide reliable reference for the diagnosis and prognosis of breast cancer. This paper proposes a deep convolutional network based on dual-path feature extraction networks to solve the semantic segmentation task of high-resolution breast cancer WSIs. The light-weight dual-path structure is designed comprising dilated convolution layers in for encoding sufficient multi-scale contexts, feature attention module in semantic path for selecting discriminative semantic features, and feature fusion module for the efficient fusion of heterogeneous outputs of two different levels. This segmentation system focuses on improving the consistency of the same type of regions and alleviate high inter-class variation of breast cancer histological images, reaching a balance between segmentation accuracy and efficiency. On the ICPR2018 dataset, our method outperformed three other networks in segmentation accuracy, especially for the most lethal invasive carcinoma. The PA score of the model in this paper reached 87.02%, and the mIoU score reached 68.12%.
At the same time, the method proposed also has some limitations. Firstly, because the standard medical datasets are scarce and difficult to obtain, this model was only verified on only one dataset. In the future, it should be tested on more datasets and applied to other computer vision tasks based on histological images, such as the detection of mitosis and nuclei. Secondly, according to the Nottingham grading system [31], invasive breast cancer can be further graded with three independent indexes [32], each of which worth future exploration, because the further classification of subtypes and histological grading has better clinic value for breast cancer prognosis.
The authors declare there is no acknowledgments.
The authors declare there is no conflict of interest.
[1] |
H. Sung, J. Ferlay, R. L. Siegel, M. Laversanne, I. Soerjomataram, A. Jemal, et al., Global cancer statistics 2020: GLOBOCAN estimates of incidence and mortality worldwide for 36 cancers in 185 countries, CA Cancer J. Clin., 71 (2021), 209–249. https://doi.org/10.3322/caac.21660 doi: 10.3322/caac.21660
![]() |
[2] |
A. Das, M. S. Nair, S. D. Peter, Computer-aided histopathological image analysis techniques for automated nuclear atypia scoring of breast cancer: a review, J. Dig. Imaging, 33 (2020), 1–31. https://doi.org/10.1007/s10278-019-00295-z doi: 10.1007/s10278-020-00318-0
![]() |
[3] |
L. He, R. Long, S. Antani, G. R. Thoma, Histology image analysis for carcinoma detection and grading, Comput. Methods Prog. Biomed., 107 (2012), 538–556. https://doi.org/10.1016/j.cmpb.2011.12.007 doi: 10.1016/j.cmpb.2011.12.007
![]() |
[4] | L. Dalton, Artificial intelligence grading of breast cancer: A study of ensemble learning, Lab. Investig., 102 (2022), 107–108. |
[5] |
J. G. Elmore, G. M. Longton, P. A. Carney, B. M. Geller, T. Onega, A. N. A. Tosteson, et al., Diagnostic concordance among pathologists interpreting breast biopsy specimens, JAMA, 313 (2015), 1122–1132. https://doi.org/10.1001/jama.2015.1405 doi: 10.1001/jama.2015.1405
![]() |
[6] |
K. E. Lindquist, C. Ciornei, S. Westbom-Fremer, I. Gudinaviciene, A. Ehinger, N. Mylona, et al., Difficulties in diagnostics of lung tumours in biopsies: an interpathologist concordance study evaluating the international diagnostic guidelines, J. Clin. Pathol., 75 (2022), 302–309. http://dx.doi.org/10.1136/jclinpath-2020-207257 doi: 10.1136/jclinpath-2020-207257
![]() |
[7] |
C. Merkouri, D. Grapsa, I. Tourkantonis, I. Gkiozos, A. Charpidou, G. Tournas, et al. Cytology-histology concordance for diagnosis, histological subtyping and molecular profiling of lung cancer, J. Thorac. Oncol., 16 (2021), S463. https://doi.org/10.1016/j.jtho.2021.01.794 doi: 10.1016/j.jtho.2021.01.794
![]() |
[8] |
V. K. Singh, H. A. Rashwan, M. Abdel-Nasser, F. Akram, R. Haffar, N. Pandey, et al., A computer-aided diagnosis system for breast cancer molecular subtype prediction in mammographic images, State Art Neural Networks Their Appl., 1 (2021), 153–178. https://doi.org/10.1016/B978-0-12-819740-0.00008-5 doi: 10.1016/B978-0-12-819740-0.00008-5
![]() |
[9] |
M. Perumal, A research on computer aided detection system for women breast cancer diagnosis from digital mammographic images, Int. J. Recent Technol. Eng., 2021 (2021). https://doi.org/10.35940/ijrte.B1169.0982S1119 doi: 10.35940/ijrte.B1169.0982S1119
![]() |
[10] | S. Naik, S. Doyle, S. Agner, A. Madabhushi, M. Feldman, J. Tomaszewski, Automated gland and nuclei segmentation for grading of prostate and breast cancer histopathology, in 2008 5th IEEE International Symposium on Biomedical Imaging: From Nano to Macro, (2008). https://doi.org/10.1109/ISBI.2008.4540988 |
[11] |
C. Jung, C. Kim, Segmenting clustered nuclei using H-minima transform-based marker extraction and contour parameterization, IEEE Trans. Biomed. Eng., 57 (2010), 2600–2604. https://doi.org/10.1109/TBME.2010.2060336 doi: 10.1109/TBME.2010.2060336
![]() |
[12] |
M. Kowal, P. Filipczuk, A. Obuchowicz, J. Korbicz, R. Monczak, Computer-aided diagnosis of breast cancer based on fine needle biopsy microscopic images, Comput. Biol. Med., 43 (2013), 1563–1572. https://doi.org/10.1016/j.compbiomed.2013.08.003 doi: 10.1016/j.compbiomed.2013.08.003
![]() |
[13] |
A. D. Belsare, M. M. Mushrif, M. A. Pangarkar, N. Meshram, Breast histopathology image segmentation using spatio-colour-texture based graph partition method, J. Microscopy, 262 (2016), 260–273. https://doi.org/10.1111/jmi.12361 doi: 10.1111/jmi.12361
![]() |
[14] |
Z. Senousy, M. M. Abdelsamea, M. M. Mohamed, M. M. Gaber, 3E-Net: Entropy-based elastic ensemble of deep convolutional neural networks for grading of invasive breast carcinoma histopathological microscopic images, Entropy, 23 (2021), 620. https://doi.org/10.3390/e23050620 doi: 10.3390/e23050620
![]() |
[15] |
Y. Yari, T. V. Nguyen, H. T. Nguyen, Deep learning applied for histological diagnosis of breast cancer, IEEE Access, 8 (2020), 162463–162448. https://doi.org/10.1109/ACCESS.2020.3021557 doi: 10.1109/ACCESS.2020.3021557
![]() |
[16] | D. C. Ciresan, A. Giusti, L. M. Gambardella, J. Schmidhuber, Mitosis detection in breast cancer histology images with deep neural networks, in International Conference on Medical Image Computing and Computer-Assisted Intervention, (2013). https://doi.org/10.1007/978-3-642-40763-5_51 |
[17] |
G. Litjens, C. I. Sánchez, N. Timofeeva, M. Hermsen, I. Nagtegaal, I. Kovacs, et al., Deep learning as a tool for increased accuracy and efficiency of histopathological diagnosis, Sci. Rep., 6 (2016), 26286. https://doi.org/10.1038/srep26286 doi: 10.1038/srep26286
![]() |
[18] | O. Ronneberger, P. Fischer, T. Brox, U-Net: Convolutional networks for biomedical image segmentation, in International Conference on Medical Image Computing and Computer-Assisted Intervention, (2015). https://doi.org/10.1007/978-3-319-24574-4_28 |
[19] |
D. J. Ho, D. Yarlagadda, T. M. D'Alfonso, M. G. Hanna, A. Grabenstetter, P. Ntiamoah, et al., Deep multi-magnification networks for multi-class breast cancer image segmentation, Comput. Med. Imaging Graphics, 88 (2021), 101866. https://doi.org/10.1016/j.compmedimag.2021.101866 doi: 10.1016/j.compmedimag.2021.101866
![]() |
[20] |
I. Anand, H. Negi, D. Kumar, M. Mittal, T. Kim, S. Roy, Residual U-network for breast tumor segmentation from magnetic resonance images, Comput. Mater. Continua, 67 (2021), 3107–3127. http://doi.org/10.32604/cmc.2021.014229 doi: 10.32604/cmc.2021.014229
![]() |
[21] |
L. C. Chen, G. Papandreou, I. Kokkinos, K. Murphy, A. L. Yuille, DeepLab: Semantic image segmentation with deep convolutional nets, atrous convolution, and fully connected CRFs, IEEE Trans. Pattern Anal. Mach. Intell., 40 (2018), 834–848. https://doi.org/10.1109/TPAMI.2017.2699184 doi: 10.1109/TPAMI.2017.2699184
![]() |
[22] |
J. Wang, X. Liu, Medical image recognition and segmentation of pathological slices of gastric cancer based on Deeplab v3+ neural network, Comput. Methods Prog. Biomed., 207 (2021), 106210. https://doi.org/10.1016/j.cmpb.2021.106210 doi: 10.1016/j.cmpb.2021.106210
![]() |
[23] | C. Yu, J. Wang, C. Peng, C. Gao, G. Yu, N. Sang, BiSeNet: Bilateral segmentation network for real-time semantic segmentation, in Proceedings of the European Conference on Computer Vision (ECCV), (2018). |
[24] |
G. Aresta, T. Araújo, S. Kwok, S. S. Chennamsetty, M. Safwan, V. Alex, et al., BACH: Grand challenge on breast cancer histology images, Med. Image Anal., 56 (2019), 122–139. https://doi.org/10.1016/j.media.2019.05.010 doi: 10.1016/j.media.2019.05.010
![]() |
[25] |
S. Krishnamurthy, K. Mathews, S. McClure, M. Murray, M. Gilcrease, C. Albarracin, et al., Multi-institutional comparison of Whole slide digital imaging and optical microscopy for interpretation of hematoxylin-eosin-stained breast tissue sections, Arch. Pathol. Lab. Med., 137 (2013), 1733–1739. https://doi.org/10.5858/arpa.2012-0437-OA doi: 10.5858/arpa.2012-0437-OA
![]() |
[26] | A. Canziani, A. Paszke, E. Culurciello, An analysis of deep neural network models for practical applications, preprint, arXiv: 1605.07678. |
[27] | S. Liu, D. Huang, Y. Wang, Receptive field block net for accurate and fast object detection, in Proceedings of the European Conference on Computer Vision (ECCV), (2018). |
[28] | F. Chollet, Xception: Deep learning with depthwise separable convolutions, in 2017 IEEE Conference on Computer Vision and Pattern Recognition (CVPR), (2017). |
[29] | J. Hu, L. Shen, G. Sun, Squeeze-and-excitation networks, in Proceedings of the IEEE Conference on Computer Vision and Pattern Recognition (CVPR), (2018). |
[30] |
A. Buslaev, V. I. Iglovikov, E. Khvedchenya, A. Parinov, M. Druzhinin, A. A. Kalinin, Albumentations: fast and flexible image augmentations, Information, 11 (2020), 125. https://doi.org/10.3390/info11020125 doi: 10.3390/info11020125
![]() |
[31] | S. Mantrala, P. S. Ginter, A. Mitkar, S. Joshi, H. Prabhala, V. Ramachandra, Concordance in breast cancer grading by artificial intelligence on whole slide images compares with a multi-Institutional cohort of breast pathologists, Arch. Pathol. Lab. Med., 2022 (2022), forthcoming. https://doi.org/10.5858/arpa.2021-0299-OA |
Model | IoU (%) | mIoU (%) | PA (%) | |||
Normal | Benign | In Situ | Invasive | |||
FCN-32s | 56.28 | 59.61 | 57.78 | 61.33 | 58.75 | 72.30 |
U-net | 64.68 | 60.75 | 59.87 | 65.78 | 62.77 | 78.76 |
Deeplab | 68.82 | 62.52 | 64.34 | 64.8 | 65.12 | 82.48 |
Our net | 68.20 | 66.80 | 65.22 | 72.26 | 68.12 | 87.02 |
Model | Model complexity | Calculation time (min) |
FCN-32s | 54 | 10 |
U-net | 2 | 6–8 |
Deeplab | 1 | 3–5 |
Our net | 0.7 | 2–3 |
Dilation rates | PA (%) | mIOU (%) |
{1, 3, 5} (Our method) |
87.02 | 68.12 |
{1, 2, 3} | 86.98 | 68.02 |
{5, 6, 7} | 86.79 | 67.93 |
Channel attention modules in semantic path | PA (%) | mIOU (%) |
No channel attention module | 84.72 | 65.33 |
SE-Block [29] | 86.54 | 68.04 |
Feature attention module (Our method) | 87.02 | 68.12 |
Feature fusion method | PA (%) | mIOU (%) |
Element-wise addition | 85.47 | 67.38 |
concatenation [29] | 85.50 | 67.95 |
Channel–attention-based feature fusion module (Our method) | 87.02 | 68.12 |
Reprocessing | PA (%) | MIoU (%) | Calculation time (min) |
before | 87.02 | 68.12 | 2–3 |
after | 87.74 | 68.53 | 3–5 |
Model | IoU (%) | mIoU (%) | PA (%) | |||
Normal | Benign | In Situ | Invasive | |||
FCN-32s | 56.28 | 59.61 | 57.78 | 61.33 | 58.75 | 72.30 |
U-net | 64.68 | 60.75 | 59.87 | 65.78 | 62.77 | 78.76 |
Deeplab | 68.82 | 62.52 | 64.34 | 64.8 | 65.12 | 82.48 |
Our net | 68.20 | 66.80 | 65.22 | 72.26 | 68.12 | 87.02 |
Model | Model complexity | Calculation time (min) |
FCN-32s | 54 | 10 |
U-net | 2 | 6–8 |
Deeplab | 1 | 3–5 |
Our net | 0.7 | 2–3 |
Dilation rates | PA (%) | mIOU (%) |
{1, 3, 5} (Our method) |
87.02 | 68.12 |
{1, 2, 3} | 86.98 | 68.02 |
{5, 6, 7} | 86.79 | 67.93 |
Channel attention modules in semantic path | PA (%) | mIOU (%) |
No channel attention module | 84.72 | 65.33 |
SE-Block [29] | 86.54 | 68.04 |
Feature attention module (Our method) | 87.02 | 68.12 |
Feature fusion method | PA (%) | mIOU (%) |
Element-wise addition | 85.47 | 67.38 |
concatenation [29] | 85.50 | 67.95 |
Channel–attention-based feature fusion module (Our method) | 87.02 | 68.12 |
Reprocessing | PA (%) | MIoU (%) | Calculation time (min) |
before | 87.02 | 68.12 | 2–3 |
after | 87.74 | 68.53 | 3–5 |