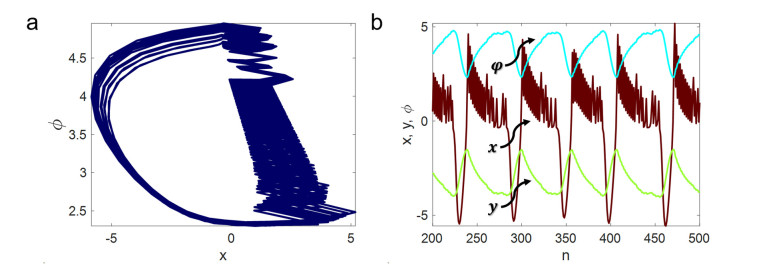
Map-based neuronal models have received much attention due to their high speed, efficiency, flexibility, and simplicity. Therefore, they are suitable for investigating different dynamical behaviors in neuronal networks, which is one of the recent hottest topics. Recently, the memristive version of the Rulkov model, known as the m-Rulkov model, has been introduced. This paper investigates the network of the memristive version of the Rulkov neuron map to study the effect of the memristor on collective behaviors. Firstly, two m-Rulkov neuronal models are coupled in different cases, through electrical synapses, chemical synapses, and both electrical and chemical synapses. The results show that two electrically coupled memristive neurons can become synchronous, while the previous studies have shown that two non-memristive Rulkov neurons do not synchronize when they are coupled electrically. In contrast, chemical coupling does not lead to synchronization; instead, two neurons reach the same resting state. However, the presence of both types of couplings results in synchronization. The same investigations are carried out for a network of 100 m-Rulkov models locating in a ring topology. Different firing patterns, such as synchronization, lagged-phase synchronization, amplitude death, non-stationary chimera state, and traveling chimera state, are observed for various electrical and chemical coupling strengths. Furthermore, the synchronization of neurons in the electrical coupling relies on the network's size and disappears with increasing the nodes number.
Citation: Mahtab Mehrabbeik, Fatemeh Parastesh, Janarthanan Ramadoss, Karthikeyan Rajagopal, Hamidreza Namazi, Sajad Jafari. Synchronization and chimera states in the network of electrochemically coupled memristive Rulkov neuron maps[J]. Mathematical Biosciences and Engineering, 2021, 18(6): 9394-9409. doi: 10.3934/mbe.2021462
[1] | Prasina Alexander, Fatemeh Parastesh, Ibrahim Ismael Hamarash, Anitha Karthikeyan, Sajad Jafari, Shaobo He . Effect of the electromagnetic induction on a modified memristive neural map model. Mathematical Biosciences and Engineering, 2023, 20(10): 17849-17865. doi: 10.3934/mbe.2023793 |
[2] | Gayathri Vivekanandhan, Hamid Reza Abdolmohammadi, Hayder Natiq, Karthikeyan Rajagopal, Sajad Jafari, Hamidreza Namazi . Dynamic analysis of the discrete fractional-order Rulkov neuron map. Mathematical Biosciences and Engineering, 2023, 20(3): 4760-4781. doi: 10.3934/mbe.2023220 |
[3] | Guowei Wang, Yan Fu . Spatiotemporal patterns and collective dynamics of bi-layer coupled Izhikevich neural networks with multi-area channels. Mathematical Biosciences and Engineering, 2023, 20(2): 3944-3969. doi: 10.3934/mbe.2023184 |
[4] | Hai Lin, Jingcheng Wang . Pinning control of complex networks with time-varying inner and outer coupling. Mathematical Biosciences and Engineering, 2021, 18(4): 3435-3447. doi: 10.3934/mbe.2021172 |
[5] | Hwayeon Ryu, Sue Ann Campbell . Stability, bifurcation and phase-locking of time-delayed excitatory-inhibitory neural networks. Mathematical Biosciences and Engineering, 2020, 17(6): 7931-7957. doi: 10.3934/mbe.2020403 |
[6] | Sridevi Sriram, Hayder Natiq, Karthikeyan Rajagopal, Ondrej Krejcar, Hamidreza Namazi . Dynamics of a two-layer neuronal network with asymmetry in coupling. Mathematical Biosciences and Engineering, 2023, 20(2): 2908-2919. doi: 10.3934/mbe.2023137 |
[7] | Shuai Zhang, Yongqing Yang, Xin Sui, Yanna Zhang . Synchronization of fractional-order memristive recurrent neural networks via aperiodically intermittent control. Mathematical Biosciences and Engineering, 2022, 19(11): 11717-11734. doi: 10.3934/mbe.2022545 |
[8] | Andrey Olypher, Jean Vaillant . On the properties of input-to-output transformations in neuronal networks. Mathematical Biosciences and Engineering, 2016, 13(3): 579-596. doi: 10.3934/mbe.2016009 |
[9] | Stefano Cosenza, Paolo Crucitti, Luigi Fortuna, Mattia Frasca, Manuela La Rosa, Cecilia Stagni, Lisa Usai . From Net Topology to Synchronization in HR Neuron Grids. Mathematical Biosciences and Engineering, 2005, 2(1): 53-77. doi: 10.3934/mbe.2005.2.53 |
[10] | Pan Wang, Xuechen Li, Qianqian Zheng . Synchronization of inertial complex-valued memristor-based neural networks with time-varying delays. Mathematical Biosciences and Engineering, 2024, 21(2): 3319-3334. doi: 10.3934/mbe.2024147 |
Map-based neuronal models have received much attention due to their high speed, efficiency, flexibility, and simplicity. Therefore, they are suitable for investigating different dynamical behaviors in neuronal networks, which is one of the recent hottest topics. Recently, the memristive version of the Rulkov model, known as the m-Rulkov model, has been introduced. This paper investigates the network of the memristive version of the Rulkov neuron map to study the effect of the memristor on collective behaviors. Firstly, two m-Rulkov neuronal models are coupled in different cases, through electrical synapses, chemical synapses, and both electrical and chemical synapses. The results show that two electrically coupled memristive neurons can become synchronous, while the previous studies have shown that two non-memristive Rulkov neurons do not synchronize when they are coupled electrically. In contrast, chemical coupling does not lead to synchronization; instead, two neurons reach the same resting state. However, the presence of both types of couplings results in synchronization. The same investigations are carried out for a network of 100 m-Rulkov models locating in a ring topology. Different firing patterns, such as synchronization, lagged-phase synchronization, amplitude death, non-stationary chimera state, and traveling chimera state, are observed for various electrical and chemical coupling strengths. Furthermore, the synchronization of neurons in the electrical coupling relies on the network's size and disappears with increasing the nodes number.
The study of neural behaviors has become one of the hottest issues for many years [1]. Neurons are the fundamental parts of the brain's neural system [2]. Therefore, the investigation of these vital elements has not been limited to medicine, and many mathematical models have been introduced to study neuronal behavior and its structure. Some of these models are flow-based [3,4], and some others are map-based [5,6]. Recently, map-based neuronal models have received much attention due to their computational efficiency, flexibility, simplicity, and high speed [1,7].
A memristor is an electrical component that can describe the impacts of electromagnetic induction in neurons by coupling the membrane potential and the magnetic flux [8,9]. Therefore, some researchers have tried to present memristive neuronal models based on the original non-memristive ones [10,11]. For example, Usha and Subha [12] presented the memristive Hindmarsh-Rose neuronal model. Also, they have investigated the behavior of single and two coupled memristive Hindmarsh-Rose neurons. Hu and Liu [13] presented the memristive version of the well-known Hodgkin-Huxley neuronal model. Another example is the memristive map-based neuronal model, which was introduced by Li et al. [9]. Employing the Rulkov model, a two-dimensional map-based neuronal model, they introduced the m-Rulkov model. Besides, the idea of employing discrete memristors was also used by Bao et al. [14] and Li et al. [15]. In these studies, some memristor-based maps were introduced.
The investigation of the collective behaviors of neurons in a complex network is another exciting field of study [16,17]. A complex network of neurons can be constructed by two independent kinds of synapses, namely chemical and electrical synapses. In fact, these synapses, which can independently exist, are the pathways for transferring information among neurons [18]. Many researchers have explored the different behaviors of diverse neuronal models in an interacting network. For example, Sun and Cao [19] studied the synchronization states of two electrically coupled Rulkov maps. They announced that the Rulkov model cannot get completely synchronized through electrical synaptic couplings. However, Hu and Cao [20] revealed that two chemically coupled Rulkov maps can get synchronized completely. Moreover, Rakshit et al. [21] investigated the different behaviors of two coupled Rulkov neuronal models with both electrical and chemical synapses. They showed that a Rulkov map could be synchronized through electrical and chemical synapses, and the increments of chemical coupling strength can enhance the synchronization. Rulkov model, a two-dimensional map-based neuronal model, has been employed in many other studies. For instance, the effect of Gaussian noise on the collective behaviors of the Rulkov model in a 128×128 grid network was studied by Perc [22]. In this study, the second derivative was considered to couple neighboring neurons. Another study conducted by Sun et al. [23] elaborated on the impact of noise correlation by adding Gaussian noise to the network of electrically coupled Rulkov models. They found a slight impact of noise correlation on the mean firing rate changes and a significant influence of noise intensity on population coherence. Also, Wang et al. [24] studied delay-induced synchronization to show the critical effect of delays in synchronization. This study employed the Rulkov model with additive Gaussian noise in a scale-free network of 200 nodes.
One of the essential collective behavior of neurons is synchronization [25]. Synchronization is a phenomenon or process that occurs in a network consisting of more than one dynamic unit in interaction [26,27]. This phenomenon is present everywhere in nature, and its traces can be observed in many fields, including biology [26]. For example, Fell and Axmacher [28] approved the leading role of synchronization in memory processes in the brain. Their study indicated that phase synchronization plays an essential role in both working memory and long-term memory. Also, as declared by Arnulfo et al. [29], phase synchronization is present in the human brain's cortex. More specifically, they found phase synchronization between the brain's regions in high frequencies by recording and studying local-field potential signals. Moreover, some other studies have shown the presence of synchronization and its prominent role in attention and cognition [30,31]. Also, some other researchers have discussed the relation of synchronization and some diseases like epilepsy [32] and Parkinson's [33].
Another important collective behavior in complex networks is the chimera state [34]. Chimera refers to the state in which some oscillators of the network are synchronous while others are asynchronous [35]. So, chimera can denote partial synchronization in complex networks [36,37]. Chimera was first observed in a network of phase oscillators [38]. However, this state has been found in chemical [39], electrical [40], neuronal [41,42,43] systems, etc. Chimeras are highly dependent on initial conditions [44] and the network's topology [45]. Moreover, different types of chimera, such as amplitude chimera [46], traveling chimera [47], chimera death [46], spiral wave chimera [48], etc., have been reported in the literature. For instance, traveling chimera is a phenomenon in which the displacement of coherent oscillators with a constant pace over time can be observed [34]. This phenomenon which was reported by Simo et al. [49], was observed in a network of Hindmarsh-Rose neuronal models. In another study conducted by Simo et al. [50], the emergence of traveling chimera was detected in a Hindmarsh-Rose neuron network under the electrical field.
As mentioned above, the collective behavior of two electrically, chemically, and electrochemically coupled non-memristive Rulkov models have been investigated. In this paper, the collective behavior of the memristive Rulkov model is investigated by considering two coupled m-Rulkov models and a network of 100 m-Rulkov models in a ring topology. In both conditions, the neurons are connected via electrical synapses, chemical synapses, and both of them. The effects of these synapses and their coupling strengths are under consideration. The paper is organized as follows: The m-Rulkov model and its network are described and detailed in Section 2. Section 3 presents the obtained results for different considered cases. The conclusion of the findings is declared in Section 4.
Rulkov model is basically a two-dimensional map-based neural model which was theoretically designed by Rulkov [5]. In this paper, the memristive version of the Rulkov map, which was presented by Li et al. [9], is employed. The m-Rulkov model is able to present different neuronal behaviors like spiking, bursting, etc., in different parameters' values and initial conditions. The discrete m-Rulkov model is described as follows:
x(n+1)=F(x(n),y(n))+γtanh(φ(n))x(n) | (1) |
y(n+1)=y(n)−μx(n) |
φ(n+1)=φ(n)+εx(n) |
where x(n), y(n), and φ(n) are respectively the excitatory (represents action potential), recovery, and flux (represents electromagnetic induction) variables. Moreover, the term γtanh(φ(n))x(n) denotes the electromagnetic induction impact on the excitatory variable with the strength of γ. In addition, ε determines the time scale when electromotive force is generated, and μ is the control parameter. Also, the discontinuous nonlinear F(x(n),y(n)) is defined as below:
F(x(n),y(n))={α1−x+yx≤0α+y0<x≤α+y−1x≥α+y | (2) |
here, α is the control parameter. In fact, the α parameter determines the system regime in different conditions like resting, spiking or bursting. Considering α=5,μ=ε=0.05, and γ=0.55, the phase diagram and the time series of System (1) are shown in Fig. 1. The parameters are set in a way that the system exhibits chaotic bursting, as mentioned in [9]. The initial conditions are randomly selected for x and y variables in the range [−1 1] and the initial value of the φ variable is set to zero.
To investigate the behavior of the neuronal networks, the map-based memristive Rulkov is employed as the dynamical behavior of each node of the network. The general form of the neuronal network, considering both electrical and chemical synaptic coupling functions, can be formulated as follows:
xi(n+1)=f(xi(n),yi(n))+ϵN∑j=1G1ijf(xj(n),yj(n))+gc(vs−xi(n))N∑j=1G2ijΓ(xj(n)) | (3) |
yi(n+1)=yi(n+1)−μxi(n) |
φi(n+1)=φi(n)+εxi(n) |
where ϵ∑Nj=1G1ijf(xj(n),yj(n)) denotes the electrical synaptic coupling with the strength of ϵ, and gc(vs−xi(n))∑Nj=1G2ijΓ(xj(n)) refers to the chemical synaptic coupling with the strength of gc. Also, Γ(xj)=11+e(−β(xj−Θs)) and f(xi(n),yi(n))=F(xi(n),yi(n))+γtanh(φi(n))xi(n). The parameter vs is the synaptic reversal potential, Θs is the threshold of synaptic firing, and β is the sigmoid slope. Note that the value of d=vs−xi(n) can determine whether the synapse is excitatory or inhibitory. If d>0, the synapse is excitatory, otherwise the synapse is inhibitory. In this study, the parameter values are set at vs=Θs=−1.4, and β=50 as described in [21].
To study the synchronized behaviors of m-Rulkov models, we first consider N=2, i.e., two coupled m-Rulkov maps. Then, considering N=100, we study the different patterns of the network, including synchronization and chimera states. In both cases, the initial conditions are randomly selected between −1 to 1, except the flux variables which are set to zero. In order to determine the synchronous state, the averaged synchronization error, as described in Eq (4), is employed as the synchronization criterion for the neurons' action potentials.
E=1n(N−1)n∑k=1N∑j=2‖x1(k)−xj(k)‖ | (4) |
where N is the number of network nodes, and n is the number of samples for each node. Note that all results are obtained for n=1,…,1000, and the normalized values of error are presented.
In this subsection, the synchronization of two coupled m-Rulkov models is investigated under different couplings with considering α=5,μ=ε=0.05,γ=0.55,vs=Θs=−1.4,and β=50. As declared in [19], two non-memristive Rulkov models cannot get synchronized through the electrical synapses. However, using chemical synaptic couplings, they can be fully synchronized [20]. Moreover, considering electrochemical synapses, it was proved in [21] that two non-memristive Rulkov models could be synchronized. Here, firstly, the synchronization error of the action potential signal is calculated by assuming gc=0 for different electrical synaptic strengths (ϵ). The result is represented in Figure 2(a). Similarly, considering ϵ=0, the synchronization error is calculated for different values of chemical synaptic strength (gc), which is shown in Figure 2(b). As represented in Figure 2(a), two coupled m-Rulkov models can be synchronized through electrical synapses for 0.3≤ϵ≤0.5. Similarly, Figure 2(b) indicates that two m-Rulkov models can be synchronized via chemical synapses for 0.36≤gc≤1. However, in this range, the neurons reach an amplitude death state, not a synchronous oscillation. Therefore, in contrast to the result of coupled non-memristive Rulkov models [19], the network consisting of two memristive Rulkov models can get synchronized even without chemical synapses.
Then, the behavior of two coupled m-Rulkov maps is studied by calculating the normalized synchronization error considering the variation of both electrical (ϵ) and chemical (gc) strengths, which is shown in Figure 3. As demonstrated in Figure 3, two coupled m-Rulkov neurons can be synchronized in the presence of both electrical and chemical synapses. The dark blue regions in Figure 3 determine the states in which the neurons are synchronized, so the synchronization error is almost zero. Furthermore, by varying the electrical and chemical coupling strengths, i.e., ϵ and gc, different firing patterns can be observed, which are shown in Figure 4. As it can be seen in Figure 4, two coupled m-Rulkov models can be synchronized in different spiking, bursting, and resting states.
For deeper investigation, this section considers a ring network of 100 m-Rulkov models coupled via both electrical and chemical synapses to study the different collective behaviors of the memristive Rulkov model in a large network. Similar to the previous section, first, the effect of electrical synapses (assuming gc=0), then the effect of chemical synapses (assuming ϵ=0), and finally, the effect of both electrical and chemical synapses are investigated. To this purpose, by setting α=5,μ=ε=0.05,γ=0.55,vs=Θs=−1.4,and β=50, the synchronization error is calculated for each condition. More specifically, Figure 5(a), (b) respectively show the normalized synchronization error for electrical (gc=0) and chemical (ϵ=0) synapses.
According to Figure 5(a), the neurons cannot get synchronized when the coupling is only via electrical synapses. In the previous subsection, it was shown that the two coupled neurons could reach synchronization. While, for N=100, increasing the electrical coupling leads to instability of the oscillators. Thus, it can be inferred that the synchronization of electrically coupled neurons depends on the number of neurons in the network. To represent this issue, Figure 6 is presented. This figure shows the parameter plane of the network corresponding to the variation of the coupling strength ϵ and the network size (N). The yellow, green, dark magenta, and gray colors in this figure represent the regions of synchronization, amplitude death, asynchronization, and instability. In order to determine different regions, the synchronization error for the N electrically coupled m-Rulkov models is calculated. The asynchronous state is determined by non-zero errors, while zero error represents the synchronization and amplitude death regions. To distinguish synchronization state from amplitude death state, the difference between the mean of some of the last samples is calculated. Besides, in the instability region, the error is an undefined value. It is observed that synchronization is only possible in small networks. Furthermore, increasing the strength of the couplings in small networks leads to the neurons being unstable. As the number of the oscillators increases, by strengthening the electrical coupling, the network's behavior changes from asynchronization to instability.
When the neurons are coupled with chemical synapses, based on Figure 5(b), the error of synchrony becomes zero for 0.187≤gc≤0.712. However, in this case, the pattern is amplitude death state, and the neurons reach a common resting state. In other words, after an asynchronous spike, all neurons stay in their resting state with no oscillation. Also, For gc>0.712, the neurons become unstable.
Figure 7 represents the normalized synchronization error obtained for a specific interval of ϵ and gc, with assuming α=5,μ=ε=0.05,γ=0.55,vs=Θs=−1.4,and β=50. In this figure, the dark blue regions represent the almost zero value of synchronization error. Nevertheless, similar to the chemical coupling in all of these conditions, the coupled neurons evolve into amplitude death, not synchronous firing.
As mentioned above, assuming gc=0 or, in other words, with only considering electrical synapses, the neurons in large networks cannot get synchronized. However, in the asynchronous region, different patterns can be observed, some of which are shown in Figure 8. The figures represent the formation of the non-stationary chimera state since the position of asynchronous neurons changes in time. It can be seen that the increase in coupling strength ϵ from 0.07 (Figure 8(a)) to 0.11 (Figure 8(b)) results in more asynchrony in the pattern. But further increments of ϵ to 0.22 leads to a more synchronous pattern. Although the neurons never get synchronized completely, they represent a sine-like synchronization state which can be seen in Figure 8(c). Note that for ϵ>0.445, the neuron gets unstable, and no complete synchronization can be observed.
To investigate the effect of the chemical synapses, ϵ=0 is considered, and different behavior of the network is studied for different values of gc. By varying gc, two main patterns are observed, which are illustrated in Figure 9. For 0187≤gc≤712, the neurons reach the same resting state as shown in Figure 9(a). Before this state, in some values of coupling strength gc, the chimera patterns can be observed, as represented in Figure 9(b).
In the existence of both electrical and chemical synaptic couplings, more different patterns can be noticed. For instance, Figure 10 illustrates four different patterns of the network. Traveling chimera in ϵ=0.11 and gc=0.8, and lagged-phase synchronization in ϵ=0.1 and gc=0.5 are respectively represented in Figure 10(a), (b). Moreover, other patterns of chimera state are formed, which can have a different level of synchrony. More specifically, larger synchronous clusters can be seen in Figure 10(c), compared with the synchronous clusters in Figure 10(d). In other words, for ϵ=0.13 and gc=0.15, more local neurons are synchronized in comparison with the number of locally synchronized neurons for ϵ=0.08 and gc=0.16.
In the presented paper, different collective behaviors of the memristive version of the Rulkov map, namely, the m-Rulkov map were studied in two main parts. First, the synchronous behavior of two coupled m-Rulkov models was on the focus. Then, considering a network of 100 m-Rulkov models in the ring topology, different collective behaviors were explored. In both cases, the effects of each electrical synapse and chemical synapse, along with the effects of their simultaneous presence, were investigated. The results indicated that two coupled m-Rulkov maps could be synchronized through electrical synaptic coupling; while, as mentioned in [19], two electrically coupled non-memristive Rulkov neurons cannot get synchronized. Besides, similar to [20], two chemically coupled maps could reach the same resting state. In contrast, no other synchronous patterns were recognized through chemical synaptic couplings. Compared with the results expressed in [21], two coupled m-Rulkov neurons could be synchronized, and different synchronization patterns were noticed through electrical and chemical synapses. This synchronization was dependent on the size of the network and was only observed in small networks. Investigation on the ring network of 100 m-Rulkov models, by calculating the synchronization error, indicated that the neurons could not get synchronized via electrical synapses. Nevertheless, non-stationary chimera and sine-like synchronization have been found in electrically coupled neurons. Also, the neurons were attracted by the same fixed point when they were coupled through chemical synapses. However, some chimera patterns could also be found before reaching the resting state. In both cases, the neurons became unstable for high coupling strengths. The presence of both electrical and chemical synaptic couplings could lead to different collective behaviors such as traveling chimera, non-stationary chimera, lagged-phase synchronization, and amplitude death state.
This work is partially funded by Centre for Nonlinear Systems, Chennai Institute of Technology, India vide funding number CIT/CNS/2021/RD/064.
The authors declare that they have no conflict of interest.
[1] |
B. Ibarz, J. M. Casado, M. A. Sanjuán, Map-based models in neuronal dynamics, Phys. Rep., 501 (2011), 1-74. doi: 10.1016/j.physrep.2010.12.003
![]() |
[2] |
J. Ma, J. Tang, A review for dynamics in neuron and neuronal network, Nonlinear Dyn., 89 (2017), 1569-1578. doi: 10.1007/s11071-017-3565-3
![]() |
[3] |
A. L. Hodgkin, A. F. Huxley, A quantitative description of membrane current and its application to conduction and excitation in nerve, J. Physiol., 117 (1952), 500. doi: 10.1113/jphysiol.1952.sp004764
![]() |
[4] | J. L. Hindmarsh, R. Rose, A model of neuronal bursting using three coupled first order differential equations, Proc. R. Soc. London Series B. Biol. Sci., 221 (1984), 87-102. |
[5] |
N. F. Rulkov, Modeling of spiking-bursting neural behavior using two-dimensional map, Phys. Rev. E, 65 (2002), 041922. doi: 10.1103/PhysRevE.65.041922
![]() |
[6] |
E. M. Izhikevich, Simple model of spiking neurons, IEEE Trans. Neural Networks, 14 (2003), 1569-1572. doi: 10.1109/TNN.2003.820440
![]() |
[7] | K. Rajagopal, S. Panahi, M. Chen, S. Jafari, B. Bao, Suppressing spiral wave turbulence in a simple fractional-order discrete neuron map using impulse triggering, Fractals, 29 (2021), 2140030. |
[8] | H. Bao, A. Hu, W. Liu, B. Bao, Hidden bursting firings and bifurcation mechanisms in memristive neuron model with threshold electromagnetic induction, IEEE Trans. Neural Networks Learn. Syst., 31 (2019), 502-511. |
[9] | K. Li, H. Bao, H. Li, J. Ma, Z. Hua, B. C. Bao, Memristive Rulkov neuron model with magnetic induction effects, IEEE Trans. Ind. Inf., 2021. |
[10] |
M. Lv, C. Wang, G. Ren, J. Ma, X. Song, Model of electrical activity in a neuron under magnetic flow effect, Nonlinear Dyn., 85 (2016), 1479-1490. doi: 10.1007/s11071-016-2773-6
![]() |
[11] |
K. Rajagopal, I. Moroz, B. Ramakrishnan, A. Karthikeyn, P. Duraisamy, Modified Morris-Lecar neuron model: effects of very low frequency electric fields and of magnetic fields on the local and network dynamics of an excitable media, Nonlinear Dyn., 104 (2021), 4427-4443. doi: 10.1007/s11071-021-06494-0
![]() |
[12] |
K. Usha, P. Subha, Hindmarsh-Rose neuron model with memristors, Biosystems, 178 (2019), 1-9. doi: 10.1016/j.biosystems.2019.01.005
![]() |
[13] |
X. Hu, C. Liu, Dynamic property analysis and circuit implementation of simplified memristive Hodgkin-Huxley neuron model, Nonlinear Dyn., 97 (2019), 1721-1733. doi: 10.1007/s11071-019-05100-8
![]() |
[14] | H. Bao, Z. Hua, H. Li, M. Chen, B. Bao, Discrete memristor hyperchaotic maps, IEEE Trans. Circuits Syst. I, 2021. |
[15] |
H. Li, Z. Hua, H. Bao, L. Zhu, M. Chen, B. Bao, Two-dimensional memristive hyperchaotic maps and application in secure communication, IEEE Trans. Ind. Electron., 68 (2021), 9931-9940. doi: 10.1109/TIE.2020.3022539
![]() |
[16] |
I. Hussain, S. Jafari, D. Ghosh, M. Perc, Synchronization and chimeras in a network of photosensitive FitzHugh-Nagumo neurons, Nonlinear Dyn., 104 (2021), 2711-2721. doi: 10.1007/s11071-021-06427-x
![]() |
[17] |
A. Bahramian, F. Parastesh, V. T. Pham, T. Kapitaniak, S. Jafari, M. Perc, Collective behavior in a two-layer neuronal network with time-varying chemical connections that are controlled by a Petri net, Chaos: Interdiscip. J. Nonlinear Sci., 31 (2021), 033138. doi: 10.1063/5.0045840
![]() |
[18] |
A. E. Pereda, Electrical synapses and their functional interactions with chemical synapses, Nat. Rev. Neurosci., 15 (2014), 250-263. doi: 10.1038/nrn3708
![]() |
[19] |
H. Sun, H. Cao, Complete synchronization of coupled Rulkov neuron networks, Nonlinear Dyn., 84 (2016), 2423-2434. doi: 10.1007/s11071-016-2654-z
![]() |
[20] |
D. Hu, H. Cao, Stability and synchronization of coupled Rulkov map-based neurons with chemical synapses, Commun. Nonlinear Sci. Numer. Simul., 35 (2016), 105-122. doi: 10.1016/j.cnsns.2015.10.025
![]() |
[21] |
S. Rakshit, A. Ray, B. K. Bera, D. Ghosh, Synchronization and firing patterns of coupled Rulkov neuronal map, Nonlinear Dyn., 94 (2018), 785-805. doi: 10.1007/s11071-018-4394-8
![]() |
[22] |
M. Perc, Thoughts out of noise, Eur. J. Phys., 27 (2006), 451. doi: 10.1088/0143-0807/27/2/026
![]() |
[23] |
X. Sun, M. Perc, Q. Lu, J. Kurths, Effects of correlated Gaussian noise on the mean firing rate and correlations of an electrically coupled neuronal network, Chaos: Interdiscip. J. Nonlinear Sci., 20 (2010), 033116. doi: 10.1063/1.3483876
![]() |
[24] |
Q. Wang, M. Perc, Z. Duan, G. Chen, Synchronization transitions on scale-free neuronal networks due to finite information transmission delays, Phys. Rev. E, 80 (2009), 026206. doi: 10.1103/PhysRevE.80.026206
![]() |
[25] |
S. Boccaletti, J. Kurths, G. Osipov, D. Valladares, C. Zhou, The synchronization of chaotic systems, Phys. Rep., 366 (2002), 1-101. doi: 10.1016/S0370-1573(02)00137-0
![]() |
[26] |
A. Arenas, A. Díaz-Guilera, J. Kurths, Y. Moreno, C. Zhou, Synchronization in complex networks, Phys. Rep., 469 (2008), 93-153. doi: 10.1016/j.physrep.2008.09.002
![]() |
[27] |
K. Rajagopal, S. Jafari, A. Karthikeyan, A. Srinivasan, Effect of magnetic induction on the synchronizability of coupled neuron network, Chaos: Interdiscip. J. Nonlinear Sci., 31 (2021), 083115. doi: 10.1063/5.0061406
![]() |
[28] |
J. Fell, N. Axmacher, The role of phase synchronization in memory processes, Nat. Rev. Neurosci., 12 (2011), 105-118. doi: 10.1038/nrn2979
![]() |
[29] | G. Arnulfo, S. H. Wang, V. Myrov, B. Toselli, J. Hirvonen, M. Fato, et al., Long-range phase synchronization of high-frequency oscillations in human cortex, Nat. Commun., 11 (2020), 1-15. |
[30] |
C. A. Bosman, C. S. Lansink, C. M. Pennartz, Functions of gamma‐band synchronization in cognition: From single circuits to functional diversity across cortical and subcortical systems, Eur. J. Neurosci., 39 (2014), 1982-1999. doi: 10.1111/ejn.12606
![]() |
[31] |
C. A. Bosman, J. M. Schoffelen, N. Brunet, R. Oostenveld, A. M. Bastos, T. Womelsdorf, et al., Attentional stimulus selection through selective synchronization between monkey visual areas, Neuron, 75 (2012), 875-888. doi: 10.1016/j.neuron.2012.06.037
![]() |
[32] |
P. Jiruska, M. De Curtis, J. G. Jefferys, C. A. Schevon, S. J. Schiff, K. Schindler, Synchronization and desynchronization in epilepsy: controversies and hypotheses, J. Physiol., 591 (2013), 787-797. doi: 10.1113/jphysiol.2012.239590
![]() |
[33] | T. Wang, H. Liao, Y. Zi, M. Wang, Z. Mao, Y. Xiang, et al., Distinct changes in global brain synchronization in early-onset vs. late-onset Parkinson disease, Front. Aging Neurosci., 12 (2020). |
[34] | F. Parastesh, S. Jafari, H. Azarnoush, Z. Shahriari, Z. Wang, S. Boccaletti, Chimeras, Phys. Rep., 2020. |
[35] |
A. zur Bonsen, I. Omelchenko, A. Zakharova, E. Schöll, Chimera states in networks of logistic maps with hierarchical connectivities, Eur. Phys. J. B, 91 (2018), 1-12. doi: 10.1140/epjb/e2017-80535-3
![]() |
[36] |
E. Rybalova, T. Vadivasova, G. Strelkova, V. S. Anishchenko, A. Zakharova, Forced synchronization of a multilayer heterogeneous network of chaotic maps in the chimera state mode, Chaos: Interdiscip. J. Nonlinear Sci., 29 (2019), 033134. doi: 10.1063/1.5090184
![]() |
[37] |
M. Winkler, J. Sawicki, I. Omelchenko, A. Zakharova, V. Anishchenko, E. Schöll, Relay synchronization in multiplex networks of discrete maps, EPL, 126 (2019), 50004. doi: 10.1209/0295-5075/126/50004
![]() |
[38] | Y. Kuramoto, D. Battogtokh, Coexistence of coherence and incoherence in nonlocally coupled phase oscillators, Nonlinear Phenom. Complex Syst., 5 (2002), 380-385. |
[39] |
S. Nkomo, M. R. Tinsley, K. Showalter, Chimera and chimera-like states in populations of nonlocally coupled homogeneous and heterogeneous chemical oscillators, Chaos: Interdiscip. J. Nonlinear Sci., 26 (2016), 094826. doi: 10.1063/1.4962631
![]() |
[40] |
L. V. Gambuzza, A. Buscarino, S. Chessari, L. Fortuna, R. Meucci, M. Frasca, Experimental investigation of chimera states with quiescent and synchronous domains in coupled electronic oscillators, Phys. Rev. E, 90 (2014), 032905. doi: 10.1103/PhysRevE.90.032905
![]() |
[41] |
B. K. Bera, D. Ghosh, M. Lakshmanan, Chimera states in bursting neurons, Phys. Rev. E, 93 (2016), 012205. doi: 10.1103/PhysRevE.93.012205
![]() |
[42] |
S. Majhi, M. Perc, D. Ghosh, Chimera states in uncoupled neurons induced by a multilayer structure, Sci. Rep., 6 (2016), 1-11. doi: 10.1038/s41598-016-0001-8
![]() |
[43] |
I. A. Shepelev, T. E. Vadivasova, A. Bukh, G. Strelkova, V. Anishchenko, New type of chimera structures in a ring of bistable FitzHugh-Nagumo oscillators with nonlocal interaction, Phys. Lett. A, 381 (2017), 1398-1404. doi: 10.1016/j.physleta.2017.02.034
![]() |
[44] | V. Dos Santos, F. S. Borges, K. C. Iarosz, I. L. Caldas, J. Szezech, R. L. Viana, et al., Basin of attraction for chimera states in a network of Rössler oscillators, Chaos: Interdiscip. J. Nonlinear Sci., 30 (2020), 083115. |
[45] |
B. K. Bera, S. Majhi, D. Ghosh, M. Perc, Chimera states: effects of different coupling topologies, EPL, 118 (2017), 10001. doi: 10.1209/0295-5075/118/10001
![]() |
[46] |
U. K. Verma, G. Ambika, Amplitude chimera and chimera death induced by external agents in two-layer networks, Chaos: Interdiscip. J. Nonlinear Sci., 30 (2020), 043104. doi: 10.1063/5.0002457
![]() |
[47] |
B. K. Bera, D. Ghosh, T. Banerjee, Imperfect traveling chimera states induced by local synaptic gradient coupling, Phys. Rev. E, 94 (2016), 012215. doi: 10.1103/PhysRevE.94.012215
![]() |
[48] |
I. A. Shepelev, A. V. Bukh, S. S. Muni, V. S. Anishchenko, Quantifying the transition from spiral waves to spiral wave chimeras in a lattice of self-sustained oscillators, Regular Chaotic Dyn., 25 (2020), 597-615. doi: 10.1134/S1560354720060076
![]() |
[49] |
G. R. Simo, P. Louodop, D. Ghosh, T. Njougouo, R. Tchitnga, H. A. Cerdeira, Traveling chimera patterns in a two-dimensional neuronal network, Phys. Lett. A, 409 (2021), 127519. doi: 10.1016/j.physleta.2021.127519
![]() |
[50] |
G. R. Simo, T. Njougouo, R. Aristides, P. Louodop, R. Tchitnga, H. A. Cerdeira, Chimera states in a neuronal network under the action of an electric field, Phys. Rev. E, 103 (2021), 062304. doi: 10.1103/PhysRevE.103.062304
![]() |
1. | Chunni Wang, Guoping Sun, Feifei Yang, Jun Ma, Capacitive coupling memristive systems for energy balance, 2022, 153, 14348411, 154280, 10.1016/j.aeue.2022.154280 | |
2. | Ramesh Ramamoorthy, Zahra Shahriari, Hayder Natiq, Karthikeyan Rajagopal, Chunbiao Li, Chimera states in coupled memristive chaotic systems: Effects of control parameters, 2022, 139, 0295-5075, 41001, 10.1209/0295-5075/ac8179 | |
3. | Shan Wang, Zhouchao Wei, Synchronization of coupled memristive Hindmarsh–Rose maps under different coupling conditions, 2023, 161, 14348411, 154561, 10.1016/j.aeue.2023.154561 | |
4. | Shuai Zhang, Yongqing Yang, Xin Sui, Yanna Zhang, Synchronization of fractional-order memristive recurrent neural networks via aperiodically intermittent control, 2022, 19, 1551-0018, 11717, 10.3934/mbe.2022545 | |
5. | Yong Wu, Qianming Ding, Tianyu Li, Dong Yu, Ya Jia, Effect of temperature on synchronization of scale-free neuronal network, 2023, 111, 0924-090X, 2693, 10.1007/s11071-022-07967-6 | |
6. | Rending Lu, Balamurali Ramakrishnan, Mayadah W. Falah, Alaa Kadhim Farhan, Nadia M. G. Al-Saidi, Viet-Thanh Pham, Synchronization and different patterns in a network of diffusively coupled elegant Wang–Zhang–Bao circuits, 2022, 231, 1951-6355, 3987, 10.1140/epjs/s11734-022-00690-8 | |
7. | Zhen Wang, Huaigu Tian, Ondrej Krejcar, Hamidreza Namazi, Synchronization in a network of map-based neurons with memristive synapse, 2022, 231, 1951-6355, 4057, 10.1140/epjs/s11734-022-00691-7 | |
8. | Gayathri Vivekanandhan, Hayder Natiq, Yaser Merrikhi, Karthikeyan Rajagopal, Sajad Jafari, Dynamical Analysis and Synchronization of a New Memristive Chialvo Neuron Model, 2023, 12, 2079-9292, 545, 10.3390/electronics12030545 | |
9. | Ramesh Ramamoorthy, Salah Boulaaras, Asma Alharbi, K. Sathiyadevi, Impact of repulsive coupling in exhibiting distinct collective dynamical states, 2022, 231, 1951-6355, 4117, 10.1140/epjs/s11734-022-00627-1 | |
10. | Nafise Naseri, Fatemeh Parastesh, Mahdi Karami, Farnaz Ghassemi, An optimization method to keep synchronization features when decreasing network nodes, 2022, 231, 1951-6355, 3971, 10.1140/epjs/s11734-022-00626-2 | |
11. | Weiwei Fan, Huagan Wu, Ze Li, Quan Xu, Synchronization and chimera in a multiplex network of Hindmarsh–Rose neuron map with flux-controlled memristor, 2022, 231, 1951-6355, 4131, 10.1140/epjs/s11734-022-00720-5 | |
12. | Kexin Li, Bocheng Bao, Jun Ma, Mo Chen, Han Bao, Synchronization transitions in a discrete memristor-coupled bi-neuron model, 2022, 165, 09600779, 112861, 10.1016/j.chaos.2022.112861 | |
13. | Yanmei Lu, Chunhua Wang, Quanli Deng, Rulkov neural network coupled with discrete memristors, 2022, 33, 0954-898X, 214, 10.1080/0954898X.2022.2131921 | |
14. | Guowei Wang, Yan Fu, Spatiotemporal patterns and collective dynamics of bi-layer coupled Izhikevich neural networks with multi-area channels, 2022, 20, 1551-0018, 3944, 10.3934/mbe.2023184 | |
15. | Quan Xu, Tong Liu, Shoukui Ding, Han Bao, Ze Li, Bei Chen, Extreme multistability and phase synchronization in a heterogeneous bi-neuron Rulkov network with memristive electromagnetic induction, 2022, 1871-4080, 10.1007/s11571-022-09866-3 | |
16. | Bocheng Bao, Jingting Hu, Jianming Cai, Xi Zhang, Han Bao, Memristor-induced mode transitions and extreme multistability in a map-based neuron model, 2023, 111, 0924-090X, 3765, 10.1007/s11071-022-07981-8 | |
17. | I. Korneev, I. Ramazanov, V. Semenov, A. Slepnev, T. Vadivasova, Synchronization effects in multiplex networks of chaotic maps with memristive interlayer coupling, 2024, 135, 10075704, 108072, 10.1016/j.cnsns.2024.108072 | |
18. | Xun 询 Yan 晏, Zhijun 志军 Li 李, Chunlai 春来 Li 李, Dynamics and synchronization in a memristor-coupled discrete heterogeneous neuron network considering noise, 2024, 33, 1674-1056, 028705, 10.1088/1674-1056/ad062c | |
19. | Sathiyadevi Kanagaraj, Premraj Durairaj, Anitha Karthikeyan, Karthikeyan Rajagopal, Unraveling the dynamics of a flux coupled Chialvo neurons and the existence of extreme events, 2024, 18, 1871-4080, 2211, 10.1007/s11571-024-10079-z | |
20. | ShouFang Huang, ChengYu Yu, ZhengGang Cai, JiQian Zhang, MaoSheng Wang, Fei Xu, Frequency chimera state induced by time delays in FitzHugh-Nagumo neural networks, 2024, 92, 05779073, 115, 10.1016/j.cjph.2024.09.009 | |
21. | Bocheng Bao, Jingting Hu, Han Bao, Quan Xu, Mo Chen, Memristor-coupled dual-neuron mapping model: initials-induced coexisting firing patterns and synchronization activities, 2024, 18, 1871-4080, 539, 10.1007/s11571-023-10006-8 | |
22. | A. Prasina, V. Samuthira Pandi, W. Nancy, K. Thilagam, K. Veena, A. Muniyappan, Synchronization and chimeras in asymmetrically coupled memristive Tabu learning neuron network, 2025, 489, 00963003, 129163, 10.1016/j.amc.2024.129163 | |
23. | Quan Xu, Liping Huang, Ning Wang, Han Bao, Huagan Wu, Mo Chen, Initial-offset-boosted coexisting hyperchaos in a 2D memristive Chialvo neuron map and its application in image encryption, 2023, 111, 0924-090X, 20447, 10.1007/s11071-023-08905-w | |
24. | Han Bao, Kang Rong, Mo Chen, Xi Zhang, Bocheng Bao, Multistability and synchronization of discrete maps via memristive coupling, 2023, 174, 09600779, 113844, 10.1016/j.chaos.2023.113844 | |
25. | Prasina Alexander, Fatemeh Parastesh, Ibrahim Ismael Hamarash, Anitha Karthikeyan, Sajad Jafari, Shaobo He, Effect of the electromagnetic induction on a modified memristive neural map model, 2023, 20, 1551-0018, 17849, 10.3934/mbe.2023793 | |
26. | Gayathri Vivekanandhan, Mahtab Mehrabbeik, Hayder Natiq, Boshra Hatef, Yaser Merrikhi, Sajad Jafari, Synchronization and firing patterns of coupled one-dimensional neuron maps, 2023, 97, 0973-7111, 10.1007/s12043-023-02628-8 | |
27. | P. Nyifeh, Z. T. Njitacke, C. N. Takembo, A. Mvogo, H. P. Ekobena Fouda, J. Awrejcewicz, Unstable wave patterns of information in neural network under light illumination and magnetic field, 2024, 38, 0217-9792, 10.1142/S021797922450200X | |
28. | V. J. Márquez-Rodríguez, K. Tucci, M. G. Cosenza, Chimera states and information transfer in interacting populations of map-based neurons, 2024, 36, 0941-0643, 18151, 10.1007/s00521-024-10050-3 | |
29. | Indranil Ghosh, Sishu Shankar Muni, Hammed Olawale Fatoyinbo, On the analysis of a heterogeneous coupled network of memristive Chialvo neurons, 2023, 111, 0924-090X, 17499, 10.1007/s11071-023-08717-y | |
30. | Zhiruo Guo, Bo Liu, Jianxin Ren, Qing Zhong, Yaya Mao, Xiangyu Wu, Wenchao Xia, Xiumin Song, Shuaidong Chen, Ying Li, Feng Wang, Yongfeng Wu, Multi-Domain Multi-Level Optical Encryption Transmission Scheme Based on Memristor Rulkov Neuron Chaos, 2024, 16, 1943-0655, 1, 10.1109/JPHOT.2024.3429238 | |
31. | Sriram Parthasarathy, Fatemeh Parastesh, Hayder Natiq, Karthikeyan Rajagopal, Sajad Jafari, Fakhteh Ghanbarnejad, Effect of Electrical and Chemical Autapse on the Firing Pattern and Synchronization of the Rulkov Neuron Model, 2023, 2023, 1099-0526, 1, 10.1155/2023/8459009 | |
32. | Mahtab Mehrabbeik, Atefeh Ahmadi, Fatemeh Bakouie, Amir Homayoun Jafari, Sajad Jafari, Dibakar Ghosh, The Impact of Higher-Order Interactions on the Synchronization of Hindmarsh–Rose Neuron Maps under Different Coupling Functions, 2023, 11, 2227-7390, 2811, 10.3390/math11132811 | |
33. | Chenlong Yi, Chunbiao Li, Yongxin Li, Rita Yi Man Li, 2023, When a Neuron and its Self-Cascade System Coupled by a Memristor Synapse, 979-8-3503-1623-0, 1, 10.1109/ICMCA59770.2023.10480973 | |
34. | Negar Massihi, Gokulakrishnan Sriram, Fahimeh Nazarimehr, Farzad Towhidkhah, Karthikeyan Rajagopal, Sajad Jafari, Various dynamics of a ring of non-identical attention deficit disorder maps, 2024, 1951-6355, 10.1140/epjs/s11734-024-01168-5 | |
35. | Deiva Sundari Muthukumar, Dorsa Nezhad Hajian, Hayder Natiq, Mahtab Mehrabbeik, Nikhil Pal, Sajad Jafari, Multilayer structure-induced collective dynamics in uncoupled memristive Rulkov neurons: Impact of field coupling and intralayer connections, 2024, 01672789, 134464, 10.1016/j.physd.2024.134464 | |
36. | Huihai Wang, Hanqi Chen, Kehui Sun, Wanting Zhu, Zhao Yao, Spatiotemporal dynamics and synchronization in a memristive Chialvo neural network, 2025, 0924-090X, 10.1007/s11071-024-10599-7 | |
37. | Vijeesh Vijayan, Hayder Natiq, Shaher Momani, Viet-Thanh Pham, Matjaž Perc, Lag synchronization in an unidirectional ring of memristive neurons, 2025, 1951-6355, 10.1140/epjs/s11734-025-01533-y |