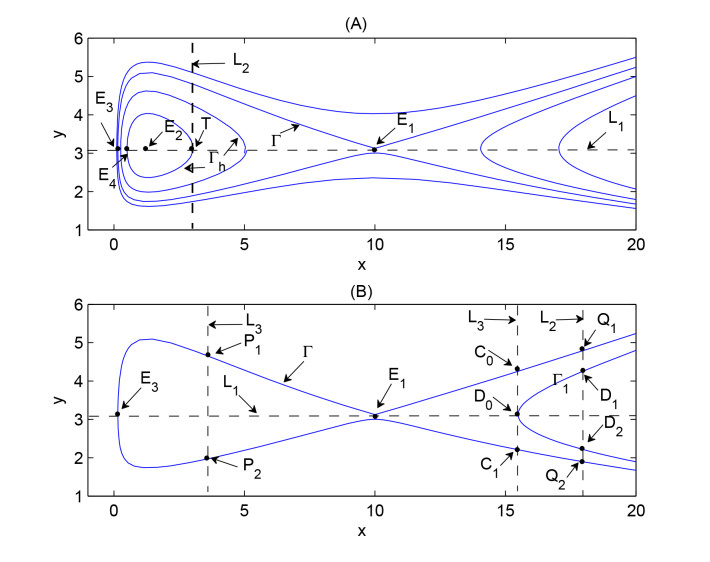
Spraying insecticides and releasing natural enemies are two commonly used methods in the integrated pest management strategy. With the rapid development of biotechnology, more and more realistic factors have been considered in the establishment and implementation of the integrated pest management models, such as the limited resources, the mutual restriction between pests and natural enemies, and the monitoring data of agricultural insects. Given these realities, we have proposed a pest-natural enemy integrated management system, which is a nonlinear state-dependent feedback control model. Besides the anti-predator behavior of the pests to the natural enemies is considered, the density dependent killing rate of pests and releasing amount of natural enemies are also introduced into the system. We address the impulsive sets and phase sets of the system in different cases, and the analytic expression of the Poincaré map which is defined in the phase set was investigated. Further we analyze the existence, uniqueness, global stability of order-1 periodic solution. In addition, the existence of periodic solution of order-k (k≥2) is discussed. The theoretical analyses developed here not only show the relationship between the economic threshold and the other key factors related to pest control, but also reveal the complex dynamical behavior induced by the nonlinear impulsive control strategies.
Citation: Yuan Tian, Sanyi Tang. Dynamics of a density-dependent predator-prey biological system with nonlinear impulsive control[J]. Mathematical Biosciences and Engineering, 2021, 18(6): 7318-7343. doi: 10.3934/mbe.2021362
[1] | Yazhi Wu, Guangyao Tang, Changcheng Xiang . Dynamic analysis of a predator-prey state-dependent impulsive model with fear effect in which action threshold depending on the prey density and its changing rate. Mathematical Biosciences and Engineering, 2022, 19(12): 13152-13171. doi: 10.3934/mbe.2022615 |
[2] | Zhenzhen Shi, Huidong Cheng, Yu Liu, Yanhui Wang . Optimization of an integrated feedback control for a pest management predator-prey model. Mathematical Biosciences and Engineering, 2019, 16(6): 7963-7981. doi: 10.3934/mbe.2019401 |
[3] | Guirong Jiang, Qishao Lu, Linping Peng . Impulsive Ecological Control Of A Stage-Structured Pest Management System. Mathematical Biosciences and Engineering, 2005, 2(2): 329-344. doi: 10.3934/mbe.2005.2.329 |
[4] | Tingting Zhao, Robert J. Smith? . Global dynamical analysis of plant-disease models with nonlinear impulsive cultural control strategy. Mathematical Biosciences and Engineering, 2019, 16(6): 7022-7056. doi: 10.3934/mbe.2019353 |
[5] | Jie Hu, Juan Liu, Peter Yuen, Fuzhong Li, Linqiang Deng . Modelling of a seasonally perturbed competitive three species impulsive system. Mathematical Biosciences and Engineering, 2022, 19(3): 3223-3241. doi: 10.3934/mbe.2022149 |
[6] | Wenjie Qin, Yue Xia, Yi Yang . An eco-epidemic model for assessing the application of integrated pest management strategies. Mathematical Biosciences and Engineering, 2023, 20(9): 16506-16527. doi: 10.3934/mbe.2023736 |
[7] | Yufei Wang, Huidong Cheng, Qingjian Li . Dynamic analysis of wild and sterile mosquito release model with Poincaré map. Mathematical Biosciences and Engineering, 2019, 16(6): 7688-7706. doi: 10.3934/mbe.2019385 |
[8] | Huidong Cheng, Hui Xu, Jingli Fu . Dynamic analysis of a phytoplankton-fish model with the impulsive feedback control depending on the fish density and its changing rate. Mathematical Biosciences and Engineering, 2023, 20(5): 8103-8123. doi: 10.3934/mbe.2023352 |
[9] | Gianni Gilioli, Sara Pasquali, Fabrizio Ruggeri . Nonlinear functional response parameter estimation in a stochastic predator-prey model. Mathematical Biosciences and Engineering, 2012, 9(1): 75-96. doi: 10.3934/mbe.2012.9.75 |
[10] | Yi Yang, Lirong Liu, Changcheng Xiang, Wenjie Qin . Switching dynamics analysis of forest-pest model describing effects of external periodic disturbance. Mathematical Biosciences and Engineering, 2020, 17(4): 4328-4347. doi: 10.3934/mbe.2020239 |
Spraying insecticides and releasing natural enemies are two commonly used methods in the integrated pest management strategy. With the rapid development of biotechnology, more and more realistic factors have been considered in the establishment and implementation of the integrated pest management models, such as the limited resources, the mutual restriction between pests and natural enemies, and the monitoring data of agricultural insects. Given these realities, we have proposed a pest-natural enemy integrated management system, which is a nonlinear state-dependent feedback control model. Besides the anti-predator behavior of the pests to the natural enemies is considered, the density dependent killing rate of pests and releasing amount of natural enemies are also introduced into the system. We address the impulsive sets and phase sets of the system in different cases, and the analytic expression of the Poincaré map which is defined in the phase set was investigated. Further we analyze the existence, uniqueness, global stability of order-1 periodic solution. In addition, the existence of periodic solution of order-k (k≥2) is discussed. The theoretical analyses developed here not only show the relationship between the economic threshold and the other key factors related to pest control, but also reveal the complex dynamical behavior induced by the nonlinear impulsive control strategies.
Lotka-Volterra predator-prey system, which is one of the most successful models used to explain the interactions between two biological species in the early stage of biology. The Volterra principle shows that a disturbance to the predator-prey system (harvesting or killing part of the prey and predator population in proportion) will increase the average amount of the prey [1,2]. The classical Lotka-Volterra model assumes that the relative growth rate of two populations are linear functions, these assumption conditions are limited in describing the interaction of two species. Taking into account the saturation factor of the predator to the prey, based on the experiments and analysis, the ecologist C.S.Holling proposed three types of functional response functions adapted to different organisms in 1965 [3], which made the classical Lotka-Volterra system more realistic.
Lotka-Volterra model and its extended models are widely used in fishery resources, pest control and other fields [4,5,6,7,8,9,10]. The integrated pest management (IPM) [11,12,13,14], which is based on the theoretical knowledge and experimental data of biological population dynamics, pesticide science, economics, combined with physical, chemical and biological technologies, is a dynamic management system. A series of integrated pest control models based on the Lotka-Volterra system can be used effectively for analyzing the changing laws of biological populations, discovering the advantages and disadvantages of treatment measures, and revealing biological principles for the pest management [15,16,17,18,19,20,21]. Tang and Chen developed the Lotka-Volterra model by introducing a proportion poisoning for the pests and releasing a constant number of predators at each impulsive event, and analyzed the impulsive differential system at fixed moments and unfixed moments respectively, which laid the foundation for the development of integrated pest control model [15]. In paper [21], the authors proposed a planar impulsive Holling II prey-predator model and provided a comprehensive qualitative analysis of global dynamics for whole parameter space.
In many previous IPM models, the number of natural enemies (predators) to be released at each impulsive event is a constant, the killing rate is proportional to the density of the pest population (preys) [21,22,23,24,25,26,27,28,29], which means that the control strategy is not closely tied to the related observational data of predators and preys in the field. However, the implementation of control strategy should be closely combined with the data of biological population, economic threshold, and biological resources [30,31,32]. For example, the number of natural enemies to be released should be guided by the density of the current natural enemies. In addition, in the process of biological evolution, the predator will kill the prey to maintain the reproduction and continuation of the population, and the prey will also gradually form a set of anti-predator strategies to deal with foreign predators and self-defense [33,34]. Many experiments shows that some adult preys may attack vulnerable young predators [35,36]. Therefore, the anti-predator behavior should be reflected in the predator-prey models.
Actually, with the development of technology, the automation and intelligence of real-time monitoring and pre-warning system on insect pests provides convenience for observing the populations of the pests and the natural enemies, offers the basis for accurate and effective spraying of pesticides and releasing the natural enemies, and also provides a platform for the development and research of the IPM. Therefore, in order to study how the density dependent control actions affect the dynamic behavior of the biological system under the disturbance of external environment, we propose the following mathematical model:
{dx(t)dt=ax(t)−bx(t)y(t),dy(t)dt=cx(t)y(t)1+ωx(t)−qx(t)y(t)−dy(t),} x(t)<ET,x(t+)=[1−δx(t)x(t)+β]x(t),y(t+)=y(t)+τ1+θy(t),} x(t)=ET, | (1.1) |
where x(t) and y(t) represent the densities of the prey (pest) and predator populations (natural enemies), respectively. a denotes the intrinsic growth rate of the prey, and the prey is hunted by the predator at a rate bxy. cx1+ωx denotes Holling II functional response function, and the digestibility of the predator to the prey gradually slows down with the increase of the density of the prey. The anti-predator behavior is reflected in qxy, i.e., the prey also attack the predator to improve the chances of survival, and the predator declines at a rate dy [16,17,27,37].
Throughout this paper we assume that the initial density of the pest population is less than the economic threshold ET, i.e., x(0+)<ET, and the initial densities of the natural enemy populations y(0+)>0. If the pest's population density reaches the economic threshold ET at time t, then controlling strategies are applied immediately and the numbers of the pest and its natural enemy are updated to (1−δx(t)x(t)+β)x(t) and y(t)+τ1+θy(t) (i.e., x(t+) and y(t+)), respectively. The third and fourth equations reflect the density dependence of the implementation of the control strategy, δ>0 is the maximal killing rate and β>0 denotes the half saturation constant. We employ a nonlinear releasing term τ1+θy(t) to describe the releasing strategy, which is a decreasing function of y(t), thus τ>0 is the maximum number of the predator released, and θ>0 is a shape parameter.
The main purpose of the paper is to investigate the global dynamical behavior of the density dependent nonlinear control model (1.1) and to reveal that how the main parameters of the model (1.1) affect the dynamics of the system. In Section 2, we first analyze the exact impulsive sets and the exact phase sets of system (1.1), the analytical expression of the Poincaré map is defined, and the properties of Poincaré map have been discussed in more detail. Moreover, in Section 3, the existence, stability and uniqueness of order-1 periodic solution, and the existence of order-k (k≥2) periodic solution will be addressed. Finally, the theoretical conclusions and biological significance are presented.
In order to discuss the effect of impulsive control strategies on the dynamic behavior of prey and predator populations, we need some preliminary knowledge of system (1.1) without any impulses, i.e., the corresponding ordinary differential equation (ODE) model of (1.1) should be analyzed. Many scholars have studied this ODE model, improved and extended the ODE model by taking into more realistic factors, which have been applied in many domains, such as control of insect pests, treatment of immunogenic tumours and HIV virus-guided therapy [27,37,38,39,40,41].
The main results of model (1.1) without any impulses that we will use are as follows, and the primary trend of solution trajectories, useful points and lines are as shown in Figure 1.
(1) There is a trivial equilibrium O(0,0); If the inequalities c−q−dω>0, (c−q−dω)2>4qdω hold, then there are two interior equilibria: E1(x∗1,y∗1) is a saddle point, E2(x∗2,y∗2) is a center. Note that x∗i(i=1,2) is the roots of the equation qωx2+(q−c+dω)x+d=0, y∗i=ab;
(2) The family of closed orbits is
Γh={(x,y)|H(x,y)=h,h1<h<h2}, | (2.1) |
where hi=aln(ae−1b)−cωln(1+ωx∗i)+dlnx∗i+qx∗i,i=1,2; Γh converts to the homoclinic cycle (denoted by Γ) as h→h1; Γh converges to the equilibrium E2(x∗2,y∗2) as h→h2.
(3) Denote the point E3(x∗3,y∗3) as the left intersection point of the homoclinic cycle Γ with the line y=ab (denoted by L1). Point E2 is located in inside of the trajectory Γh, which is denoted by E2∈ IntΓh, the right side of Γh is tangent to the line x=ET (denoted by L2) at point T(ET,ab), and the left side of Γh meets the line L1 at point E4(x∗4,y∗4), as shown in Figure 1(A).
(4) The first integral is
H(x,y)=alny−by−cωln(1+ωx)+dlnx+qx=h, | (2.2) |
where h is a constant.
To investigate the dynamics of system (1.1), we need to know whether the trajectories of the system (1.1) reach the impulsive set or not, and if the trajectories reach the impulsive set, whether the system (1.1) exists periodic solutions. Firstly we will discuss the domains of the impulsive set and phase set, which provide a basis for defining the Poincaré map.
From the first equation of system (1.1) we know that there exists a isocline L1: y=ab, which means that [0,ab] is the maximum interval for the vertical components of impulsive set, and any solution of system (1.1) either can't arrive at L2 or reaches L2 and the lower intersection point is located at or below point (ET,ab). Therefore, the basic impulsive set M can be determined as follows:
M={(x,y)∈R2+∣x=ET,0≤y≤ab}. | (2.3) |
According to the impulsive function I:(ET,y)∈M→(x+,y+)=((1−δETET+β)ET,y+τ1+θy), the phase set N which corresponding to the basic impulsive set M is:
N=I(M)={(x+,y+)∈R2+∣x+=(1−δETET+β)ET,y+∈YM}, |
where YM={y+∣ y+=y+τ1+θy,0≤y≤ab}.
Base on the value of economic threshold ET and the position of line x=(1−δETET+β)ET (denoted by L3, as shown in Figure 1(B)) where the phase set is located on, we will obtain the conclusions about the exact domains of the impulsive set for the following different cases:
(A):ET≤x∗2, (1−PET)ET<x∗2, | (2.4) |
(B):x∗2<ET<x∗1{(B11):(1−PET)ET≤x∗4,(B12):(1−PET)ET>x∗4, | (2.5) |
(C):ET≥x∗1{(C11):x∗3≤(1−PET)ET≤x∗1,(C12):(1−PET)ET>x∗1,(C13):(1−PET)ET<x∗3, | (2.6) |
where PET=δETET+β.
Moreover, the Lambert W function [42] and three quantities A0, A1 and A2 are useful throughout the analysis of impulsive set and phase set. For ease of discussion, we denote the Lambert W function by W, and A0, A1 and A2 are defined as
A0=cωln1+ωx∗11+ωET−dlnx∗1ET−q(x∗1−ET), |
A1=cωln(1+ωET1+ω(1−PET)ET)+dln(1−PET)−qPETET, |
A2=cωln(1+ωx∗11+ω(1−PET)ET)−dln(x∗1(1−PET)ET)−q(x∗1−(1−PET)ET). |
Lemma 2.1. The corresponding impulsive sets of the system (1.1) in different cases are as follows:
(A):M2, (B):{(B11):M2,(B12):M, (C):{(C11):M1,(C12):M2,(C13):M2,
where
M1={(x,y)∈R2+∣x=ET,0≤y≤Yis}, |
M2={(x,y)∈R2+∣x=ET,0≤y≤Y1is}, |
and
Yis=−abW(−e−1−A0a), Y1is=−abW(−e−1+A1a). |
Proof. Firstly, for case (C)(C11), i.e., ET≥x∗1 and x∗3≤(1−PET)ET≤x∗1, according to the trend of solution trajectory of (1.1), it is easy to know that the right branch of homoclinic cycle Γ intersects with line L2 at two points Q1 and Q2, as shown in Figure 1(B). The vertical components of Q1 and Q2 can be solved by the first integral (2.2).
Any solution initiating from the initial point (x0,y0) satisfies the following equation:
alny−by−cωln(1+ωx)+dlnx+qx=h0, | (2.7) |
where h0=alny0−by0−cωln(1+ωx0)+dlnx0+qx0.
Thus, the equation of homoclinic cycle which crosses the point (x∗1,ab) can be determined as:
alny−by−cωln(1+ωx)+dlnx+qx=alnab−b⋅ab−cωln(1+ωx∗1)+dlnx∗1+qx∗1. | (2.8) |
Substituting x=ET into Eq 2.8 yields
alny−by=alnab−a−cωln1+ωx∗11+ωET+dlnx∗1ET+q(x∗1−ET), | (2.9) |
i.e., alny−by=alnab−a−A0. Solving Eq 2.9 with respect to y, the solutions of Eq 2.9 are the vertical components of points Qi(i=1,2).
By using some properties of the Lambert W function, if A0≥0, then the roots of (2.9) are as follows:
Yis=−abW(−e−1−A0a), YIS=−abW(−1,−e−1−A0a), | (2.10) |
therefore, the intersection points of Γ and L2 are Q1(ET,YIs) and Q2(ET,Yis). It is easy to know that if ET≥x∗1, x∗3≤(1−PET)ET≤x∗1, then any solution starting from (x+0,y+0)∈N either can't reach L2 or arrives at the basic impulsive set M and the intersection point is located at or below the point Q2, which means that the exact domain of the impulsive set for case (C)(C11) is M1={(x,y)∈R2+∣x=ET,0≤y≤Yis}.
Next, we will discuss the case (C)(C12). As shown in Figure 1(B), if (1−PET)ET>x∗1, then there is a orbit Γ1 which tangents to the line L3, the coordinates of the tangent point (denoted by D0) are ((1−PET)ET,ab), and Γ1 intersects L2 at two points D1,D2. By the similar method used for case (C)(C11), we can obtain the vertical components of points D1 and D2. Substituting the point ((1−PET)ET,ab) into Eq 2.7 yields the equation of orbital curve which crosses point D0:
alny−by−cωln(1+ωx)+dlnx+qx=alnab−a−cωln(1+ω(1−PET)ET)+dln((1−PET)ET)+q(1−PET)ET. | (2.11) |
If A1<0, then letting x=ET and solve Eq 2.11 with respect to y, we have
Y1is=−abW(−e−1+A1a), Y1IS=−abW(−1,−e−1+A1a). | (2.12) |
Therefore, the intersection points of Γ1 and L2 are D1(ET,Y1IS) and D2(ET,Y1is). If ET≥x∗1 and (1−PET)ET>x∗1, then any solution starting from (x+0,y+0)∈N arrives at the basic impulsive set M and the intersection point is located at or below the point D2, and then the exact domain of impulsive set for case (C)(C12) is M2={(x,y)∈R2+∣x=ET,0≤y≤Y1is}.
For case (A),(B)(B11),(C)(C13), according to the vector field of system (1.1), it's easy to know that there exists an orbital curve tangents to the line L3, and this orbital curve intersects L2 at two points D1 and D2. Therefore, by taking advantage of the similar method, all of the impulsive sets for these cases are M2.
Finally, for case (B)(B12). If x∗2<ET<x∗1 and (1−PET)ET>x∗4, then the closed orbit Γh intersects with L3 at two points, tangents to the line L2 at the point T(ET,ab), and any solution starting from the phase set can reach the set {(x,y)|x=ET,0≤y≤ab}. Therefore, the impulsive set of system (1.1) for this case is M. This completes the proof.
Due to the nonlinear term and the diversity of threshold ET of system (1.1), the dynamic behavior of system (1.1) might be very complex, next we will focus on two representative cases (C)(C11) and (C)(C12) for a comprehensive analysis.
In order to discuss the exact domains of the phase set of the system (1.1), it is necessary to ensure the conditions under which the trajectory starting from (x+0,y+0)∈N is free from pulse effects. By analyzing the dynamics of trajectories, the conclusions can be obtained as follows.
Lemma 2.2. For case (C)(C11), any solution starting from the point (x+0,y+0)∈N with y+0∈[Ymin,Ymax] will be free from pulse effects, where
Ymin=−abW(−e−1−A2a), Ymax=−abW(−1,−e−1−A2a). | (2.13) |
Moreover, x∗3<(1−PET)ET<x∗1 ⇔ A2>0; If (1−PET)ET=x∗1 or (1−PET)ET=x∗3, then A2=0.
Proof. If x∗3≤(1−PET)ET≤x∗1, then the homoclinic cycle Γ intersects with line L3. Substituting x=(1−PET)ET into Eq 2.8 yields
alny−by=alnab−a−cωln(1+ωx∗11+ω(1−PET)ET)+dln(x∗1(1−PET)ET)+q(x∗1−(1−PET)ET), | (2.14) |
i.e.,
alny−by=alnab−a−A2. | (2.15) |
Note that if A2≥0, then Eq 2.15 with respect to y can be solved. Next we will discuss the value of A2. Denote A(x)=cωln(1+ωx∗11+ωx)−dln(x∗1x)−q(x∗1−x), then A2=A((1−PET)ET). Due to the left intersection point of Γ and L1 is E3(x∗3,ab), then substituting the coordinates of E3 into Eq 2.8 yields A(x∗3)=A(x∗1)=0. Moreover, solving A′(x)=0 with respect to x, we have x=x∗1 and x=x∗2, which means that the abscissa of two interior equilibrium satisfy the equation, i.e., A′(x∗1)=A′(x∗2)=0. Note that limx→0+A(x)=−∞, base on the monotonicity and continuity of the function A(x),x∈(0,+∞), we can obtain that A(x)>0 for x∈(x∗3,x∗1)∪(x∗1,+∞). Therefore, for case (C)(C11), if x∗3≤(1−PET)ET≤x∗1, then A2≥0. The two roots of Eq (2.15) with respect to y are as follows:
Ymin=−abW(−e−1−A2a), Ymax=−abW(−1,−e−1−A2a). |
As shown in Figure 1(B), if x∗3≤(1−PET)ET≤x∗1, then the intersection points of homoclinic cycle Γ and L3 are P1=((1−PET)ET,Ymax) and P2=((1−PET)ET,Ymin). Any solution initiating from the point (x+0,y+0) (y+0∈[Ymin,Ymax]) will not reach at the impulsive set M1, on the contrary, any solution initiating from the set (x+0,y+0) (y+0∈Y0=[0,Ymin)∪(Ymax,+∞)) can arrive at the impulsive set M1 and then experiences impulsive effects.
If (1−PET)ET∈(x∗3,x∗1), then A2=A((1−PET)ET)>0; Conversely, (1−PET)ET∈(x∗3,x∗1) once A2>0. If (1−PET)ET=x∗1 or (1−PET)ET=x∗3, then A2=0. This completes the proof.
Next, we will discuss the exact phase sets for case (C)(C11) and (C)(C12), respectively. First of all, it is necessary to analyze the properties of impulsive function y(t+)=y(t)+τ1+θy(t). Obviously, the monotonicity of function y(t+) is related to the value of y(t). Letting F(y)=y+τ1+θy, y∈[0,ab], it is easy to know F′(y)=0 at y=√τθ−1θ. In view of θ>0, we know that if √τθ−1θ≤0, i.e., √τθ−1≤0, then F′(y)≥0 for y∈[0,+∞); If √τθ−1>0, then F′(y)≤0 for y∈[0,√τθ−1θ] and F′(y)>0 for y∈[√τθ−1θ,+∞).
For case (C)(C11), we can discuss the exact domains of the phase sets for three cases: (i) √τθ−1≤0; (ii) √τθ−1θ≥Yis; (iii) 0<√τθ−1θ<Yis.
(i) If √τθ−1≤0, then the impulsive function F(y) is increasing on [0,Yis], F(y)∈[τ,Yis+τ1+θYis], denote Y11=[τ,Yis+τ1+θYis]. As any solution initiating from (x+0,y+0) (y+0∈[Ymin,Ymax]) can not arrive at the impulsive set M1, the phase set which corresponds to the impulsive set M1 is
N11={(x+,y+)∈R2+|x+=(1−PET)ET,y+∈Y011}, |
where Y011=Y11∩Y0.
(ii) If √τθ−1θ≥Yis, then the impulsive function F(y) is decreasing on [0,Yis], and F(y)∈[Yis+τ1+θYis,τ], denote Y12=[Yis+τ1+θYis,τ]. It is similar to the case (i), any solution initiating from (x+0,y+0) (y+0∈[Ymin,Ymax]) can not arrive at the impulsive set M1, then the phase set which corresponds to the impulsive set M1 is
N12={(x+,y+)∈R2+|x+=(1−PET)ET,y+∈Y012}, |
where Y012=Y12∩Y0.
(iii) If 0<√τθ−1θ<Yis, then according to the monotonicity of the impulsive function F(y), we can show that F′(y)≤0, F(y)∈[2√τθ−1θ,τ] for all y∈[0,√τθ−1θ], and F′(y)>0, F(y)∈(2√τθ−1θ,Yis+τ1+Yisθ] for all y∈(√τθ−1θ,Yis]. Denote Y13=[2√τθ−1θ,τ], Y013=Y13∩Y0, Y14=(2√τθ−1θ,Yis+τ1+Yisθ] and Y014=Y14∩Y0. The phase set which corresponds to the impulsive set M1 = M11∪M12 is N13∪N14, where
M11={(x,y)∈R2+|x=ET,0≤y≤√τθ−1θ}, |
M12={(x,y)∈R2+|x=ET,√τθ−1θ<y≤Yis}, |
N13={(x+,y+)∈R2+|x+=(1−PET)ET,y+∈Y013}, |
N14={(x+,y+)∈R2+|x+=(1−PET)ET,y+∈Y014}. |
For case (C)(C12), if (1−PET)ET>x∗1, then Γ intersects with L3 at two points, in order to distinguish this case from case (C)(C11), these two intersection points denoted by C0(xC0,yC0)=((1−PET)ET,Ymax) and C1(xC1,yC1)=((1−PET)ET,Ymin). Considering the characteristics of the homoclinic cycle Γ, the trajectory which initiating from C0 will be close to the point E1 infinitely, but not reach the impulsive set M2. By the similar method used in case (C)(C11), we can discuss the exact phase sets for the following three cases: (i) √τθ−1≤0; (ii) √τθ−1θ≥Y1is; (iii) 0<√τθ−1θ<Y1is.
(i) If √τθ−1≤0, then the impulsive function F(y) is increasing on [0,Y1is], and F(y)∈[τ,Y1is+τ1+θY1is], denote Y21=[τ,Y1is+τ1+θY1is]. As the solution initiating from C0 will not reach the impulsive set M2, the phase set which corresponds to the impulsive set M2 is
N21={(x+,y+)∈R2+|x+=(1−PET)ET,y+∈Y021}, |
where Y021={y+|τ≤y+≤Y1is+τ1+θY1is,y+≠Ymax}.
(ii) If √τθ−1θ≥Y1is, then the impulsive function F(y) is decreasing on [0,Y1is), and F(y)∈[Y1is+τ1+θY1is,τ], denote Y22=[Y1is+τ1+θY1is,τ]. The phase set which corresponds to the impulsive set M2 is
N22={(x+,y+)∈R2+|x+=(1−PET)ET,y+∈Y022}, |
where Y022={y+|Y1is+τ1+θY1is≤y+≤τ,y+≠Ymax}.
(iii) If 0<√τθ−1θ<Y1is, then on the basis of the monotonicity of F(y), it is easy to know F(y)∈[2√τθ−1θ,τ] for all y∈[0,√τθ−1θ], and F(y)∈(2√τθ−1θ,Y1is+τ1+θY1is] for all y∈(√τθ−1θ,Y1is]. Denote Y023={y+|2√τθ−1θ≤y+≤τ,y+≠Ymax} and Y024={y+|2√τθ−1θ<y+≤Y1is+τ1+θY1is,y+≠Ymax}. The phase set which corresponds to the impulsive set M2 = M11∪M21 is N23∪N24, where
M11={(x,y)∈R2+|x=ET,0≤y≤√τθ−1θ}, |
M21={(x,y)∈R2+|x=ET,√τθ−1θ<y≤Y1is}, |
N23={(x+,y+)∈R2+|x+=(1−PET)ET,y+∈Y023}, |
N24={(x+,y+)∈R2+|x+=(1−PET)ET,y+∈Y024}. |
In conclusion, we list all the exact domains of the impulsive sets and phase sets in different parameter spaces for case (C)(C11) and (C)(C12) in Table 1, it can be seen that the changes of key parameters such as economic threshold ET affect the dynamic behaviors of the system (1.1).
Cases | (1−PET)ET | Is | Ps | |
(C11) | (i) | x∗3≤(1−PET)ET≤x∗1 | M1 | N11 |
(ii) | N12 | |||
(iii) | N13∪N14 | |||
(C12) | (i) | (1−PET)ET>x∗1 | M2 | N21 |
(ii) | N22 | |||
(iii) | N23∪N24 |
Base on the impulsive sets and phase sets we discussed above, the main theorems of Poincaré map can be obtained.
Theorem 1. The Poincaré map of model (1.1) for cases (C)(C11) and (C)(C12) can be defined as:
Case(C)(C11) : ET≥x∗1, x∗3≤(1−PET)ET≤x∗1
y+i+1={P(y+i), y+i∈Y011, if √τθ−1≤0,P(y+i), y+i∈Y012, if √τθ−1θ≥ Yis,P(y+i), y+i∈Y013∪Y014, if 0<√τθ−1θ< Yis. | (2.16) |
Case(C)(C12): ET≥x∗1, (1−PET)ET>x∗1.
y+i+1={P(y+i), y+i∈Y021, if √τθ−1≤0,P(y+i), y+i∈Y022, if √τθ−1θ≥ Y1is,P(y+i), y+i∈Y023∪Y024, if 0<√τθ−1θ< Y1is. | (2.17) |
Where
P(y+i)=−abW[−bay+iexp(−bay+i+A1a)]+τ1−θabW[−bay+iexp(−bay+i+A1a)]. | (2.18) |
Proof. Assuming that any solution initiating from z+0=(x+0,y+0)∈N will experience finite or infinite pulse effects. Denote pi=(ET,yi)∈M, and p+i=((1−PET)ET,y+i)∈N, (i=1,2,3⋯), p+i is the point which corresponds to pi in the phase set after a pulse effect. If p+i and pi+1 lie in the same trajectory, then the corresponding coordinates of these two points satisfy the following equation:
cωln(1+ωET1+ω(1−PET)ET)−dln(11−PET)−qPETET=aln(yi+1y+i)−b(yi+1−y+i). | (2.19) |
For case (C)(C11), from the analysis of Lemma 2.2 we know that if the initial point p+0=((1−PET)ET,y+0) lies on the homoclinic cycle Γ or in the interior of Γ, the trajectory starting from p+0 will not arrive at the impulsive set M1, and we can see A2≥0 for x∗3≤(1−PET)ET≤x∗1. Moreover, for case (C)(C12), the trajectory initiating from C0 can not arrive at M2, and A1<0 for (1−PET)ET>x∗1 holds.
Next, we rearrange (2.19) yields
−bayi+1exp(−bayi+1)=−bay+iexp(−bay+i+A1a), i=0,1,2,⋯,k. | (2.20) |
By using the properties of the Lambert W function, we can solve the Eq 2.20 with respect to yi+1:
yi+1=−abW[−bay+iexp(−bay+i+A1a)] i=0,1,2,⋯,k. | (2.21) |
Therefore, we have
y+i+1=−abW[−bay+iexp(−bay+i+A1a)]+τ1−θabW[−bay+iexp(−bay+i+A1a)]≜P(y+i) i=0,1,2,⋯,k. | (2.22) |
Note that the analytical formula of P(y+i) is related to the Lambert W function, according to the definition of the Lambert W function, the analytical equation of P(y+i) can be analysed as follows.
For A1≤0, it is easy to know −bay+iexp(−bay+i+A1a)∈[−e−1,0), and the Eq 2.22 is well defined. From Eq 2.12 we know that A1<0 for case (C)(C12). Therefore, Eq 2.17 is true for different value range of √τθ−1θ.
For A1>0, we need to ensure that −bay+iexp(−bay+i+A1a)≥−e−1, i.e., the following inequality holds:
−bay+iexp(−bay+i)≥−exp(−1−A1a). | (2.23) |
By employing the properties of Lambert W function, it is easy to know that the inequality (2.23) holds for y+i∈(0,Y1min]∪[Y1max,∞), where
Y1min=−abW(−e−1−A1a), Y1max=−abW(−1,−e−1−A1a). |
From the proof of Lemma 2.2 we know that A(x∗1)=0, and A(x)>0 for all x∈(x∗3,x∗1)∪(x∗1,+∞). Thus, A(ET)≥0 for ET≥x∗1, i.e.,
A(ET)=A2−A1=cωln(1+ωx∗11+ωET)−dln(x∗1ET)−q(x∗1−ET)≥0. |
Therefore, if ET≥x∗1, then A2≥A1. According to the monotonicity of Lambert W function, if A2≥A1>0, then we have −abW(−e−1−A2a)≤−abW(−e−1−A1a), and −abW(−1,−e−1−A2a)≥−abW(−1,−e−1−A1a), i.e., Ymin≤Y1min, Ymax≥Y1max. Furthermore, [Y1min,Y1max]⊆[Ymin,Ymax], which means that if A1>0, then Eq 2.23 is true for case (C)(C11). By combining with the conclusion that −bay+iexp(−bay+i+A1a)≥−e−1 for A1≤0, we know that the Poincaré map can be given by (2.16). This completes the proof.
As we can see from the above discussion, the varieties of parameters will produce complex effects on the determining of the Poincaré map. The relations among the key parameters, the signs of A1,A2 and the domains of the Poincaré map as shown in Table 2.
Cases | (1−PET)ET | A1 and A2 | P(y+i) | |
(C)(C11) | (i) | x∗3≤(1−PET)ET≤x∗1 | A2≥0, △ | y+i∈Y011 |
(ii) | y+i∈Y012 | |||
(iii) | y+i∈Y013∪Y014 | |||
(C)(C12) | (i) | (1−PET)ET>x∗1 | A1<0, A2>0 | y+i∈Y021 |
(ii) | y+i∈Y022 | |||
(iii) | y+i∈Y023∪Y024 | |||
△ means the sign of A1 is not necessary for case (C)(C11). |
It follows from Lemma 2.1 and Lemma 2.2 that if ET≥x∗1, x∗3≤(1−PET)ET≤x∗1, then Γ intersects with L3 at points P1 and P2, Γ intersects with L2 at points Q1 and Q2. Moreover, Q+2((1−PET)ET,Yis+τ1+θYis) is the pulse point of Q2 after a singer impulsive effect, which lies on the line L3. If ET≥x∗1, (1−PET)ET>x∗1, then Γ intersects with L3 at points C0 and C1, Γ1 intersects with L2 at points D1 and D2. Furthermore, denote Q(xQ,yQ)=(ET,√τθ−1θ) in view of the particularity of √τθ−1θ, and if the trajectory of system (1.1) arrives at the point Q and experiences impulsive effects, then Q+((1−PET)ET,y+Q) is the pulse point of Q. For case (C)(C11) and case (C)(C12), we can obtain the following monotonicity of Poincaré map in the interval related to these above-mentioned intersection points.
Theorem 2. For case (C)(C11): ET≥x∗1, x∗3≤(1−PET)ET≤x∗1,
(i) If √τθ−1≤0, then the Poincaré map P(y+i) is increasing on [0,Ymin), and decreasing on (Ymax,+∞).
(ii) If √τθ−1θ≥Yis, then the Poincaré map P(y+i) is decreasing on [0,Ymin), and increasing on (Ymax,+∞).
(iii) If 0<√τθ−1θ<Yis, then the Poincaré map P(y+i) is decreasing on [0,ym2] and (Ymax,ym1], and increasing on [ym2,Ymin) and [ym1,+∞), where ym2=min{y+:P(y+)=yQ+},ym1=max{y+:P(y+)=yQ+}.
Proof. Without loss of generality, assuming p+i is the impulsive point which is located in the phase set, if the trajectory initiating from p+i intersects with L2 at pi+1, then there exists a corresponding relationship between the two points p+i and pi+1. Denote a mapping about the ordinate of two points by yi+1=g(y+i).
Based on the proof of Lemma 2.2, we can see that any solution of system (1.1) initiating from (x+0,y+0) with y+0∈[Ymin,Ymax] will be free from impulsive effects. when the trajectory passes through the point p+i and arrive at L2, then the function g(y+i) is increasing on [0,Ymin) and decreasing on (Ymax,+∞) according to the uniqueness of solution of system. Moreover, we have discussed the monotonicity of impulsive function F(y)=y+τ1+θy, y∈[0,ab] for case (C)(C11), and the Poincaré map P(y+i) can be regarded as a composite function of g and F. On the basis of the monotonicity of function g and F, we have the following conclusions.
(i) If √τθ−1≤0, then P(y+i) is increasing on [0,Ymin), and decreasing on (Ymax,+∞).
(ii) If √τθ−1θ≥Yis, then P(y+i) is decreasing on [0,Ymin), and increasing on (Ymax,+∞).
(iii) If 0<√τθ−1θ<Yis, then the solution which passes through the points m1((1−PET)ET,ym1) and m2((1−PET)ET,ym2) intersects with L2 at the point Q(ET,√τθ−1θ), where ym2=min{y+:P(y+)=yQ+},ym1=max{y+:P(y+)=yQ+}, P(y+i) is decreasing on [0,ym2] and (Ymax,ym1], and increasing on [ym2,Ymin) and [ym1,+∞). This completes the proof.
Theorem 3. For case (C)(C12): ET≥x∗1, (1−PET)ET>x∗1,
(i) If √τθ−1≤0, then the Poincaré map P(y+i) is increasing on [0,yD0] (yD0 is the ordinate of D0), and decreasing on (yD0,Ymax) and (Ymax,+∞).
(ii) If √τθ−1θ≥Y1is, then the Poincaré map P(y+i) is decreasing on [0,yD0], and increasing on (yD0,Ymax) and (Ymax,+∞).
(iii) If 0<√τθ−1θ<Y1is, there are three cases for the monotonicity of P(y+i) as follows.
If 0<√τθ−1θ<Yis, then the Poincaré map P(y+i) is decreasing on [0,yM2], [ab,Ymax), and (Ymax,yM1], and increasing on [yM2,ab) and [yM1,+∞), where yM2=min{y+:P(y+)=yQ+},yM1=max{y+:P(y+)=yQ+}.
If Yis<√τθ−1θ<Y1is, then the Poincaré map P(y+i) is decreasing on [0,yM2], [ab,yM1), and increasing on [yM2,ab], [yM1,Ymax) and (Ymax,+∞), where yM2=min{y+:P(y+)=yQ+},yM1=max{y+:P(y+)=yQ+}.
If Yis=√τθ−1θ, then the Poincaré map P(y+i) is decreasing on [0,ymin] and (ab,Ymax), and increasing on [ymin,ab] and (Ymax,+∞).
Proof. It is similar to the Theorem 2, we define the Q(ET,√τθ−1θ) is the intersection point of L2 and a trajectory which pass through the points M1((1−PET)ET,yM1) and M2((1−PET)ET,yM2), where yM2=min{y+:P(y+)=yQ+}, yM1=max{y+:P(y+)=yQ+}. According to the vector field of the solution trajectory, it is easy to see that g(y+i) is increasing on [0,yD0], and decreasing on [yD0,yC0) and (yC0,+∞) (here yD0=ab, yC0=Ymax). Furthermore, we have discussed the monotonicity of impulsive function F(y) for case (C)(C12) in the previous subsection. Therefore, we can obtain the following conclusions of P(y+i) by combining with the monotonicity of functions g and F.
(i) If √τθ−1≤0, then P(y+i) is increasing on [0,ab], and decreasing on (ab,Ymax) and (Ymax,+∞).
(ii) If √τθ−1θ≥Y1is, then P(y+i) is decreasing on [0,ab], and increasing on (ab,Ymax) and (Ymax,+∞).
(iii) If 0<√τθ−1θ<Y1is, then according to the value of √τθ−1θ and Yis, the monotonicity of P(y+i) can be discussed for the following three cases:
(a) If 0<√τθ−1θ<Yis, then the point M2 lies below the point C1, and the point M1 lies above the point C0, the coordinates relationship of these points is: yM2<Ymin<ab<Ymax<yM1. Base on the monotonicity of the functions g and F, we infer that P(y+i) is decreasing on [0,yM2], [ab,Ymax) and (Ymax,yM1], and increasing on [yM2,ab) and [yM1,+∞).
(b) If Yis<√τθ−1θ<Y1is, then the point C1 lies below the point M2, and the point C0 lies above the point M1, the coordinates relationship of these points is: Ymin<yM2<ab<yM1<Ymax. It is easy to know P(y+i) is decreasing on [0,yM2] and [ab,yM1), and increasing on [yM2, ab], [yM1,Ymax) and (Ymax,+∞).
(c) If Yis=√τθ−1θ, then the points Q and Q2 coincide, i.e., Q=Q2, the Poincaré map P(y+i) is decreasing on [0,ymin] and (ab,Ymax), and increasing on [ymin,ab] and [Ymax,+∞). This completes the proof.
Note that the fixed point of Poincaré map P(y+i) corresponds to the periodic solution of system (1.1). Based on the impulsive sets, phase sets and Poincaré map P(y+i) were investigated in the previous sections, we will discuss the existence and stability of the periodic solutions of system (1.1) for some cases.
Theorem 4. If τ=0 and A1=0, then any y+i in the phase set is a fixed point of Poincaré map P(y+i). If τ=0 and A1≠0, then y+i=0 is a unique fixed point of Poincaré map P(y+i).
Proof. The analytical formula of Poincaré map P(y+i) has been analyzed, and the special case of P(y+i) for τ=0 can be defined as follows:
P(y+i)=−abW[−bay+iexp(−bay+i+A1a)]. | (3.1) |
By using the properties of Lambert W function, it is easy to know that y+i=−abW[−bay+iexp(−bay+i)] is true. Therefore, if τ=0 and A1=0, then any y+i in the phase satisfies P(y+i)=y+i, i.e., any y+i in the phase set is a fixed point of P(y+i), which means that any solution which initiating from ((1−PET)ET,y+i) is an order-1 periodic solution for system (1.1). Moreover, if τ=0 and A1≠0, it follows from equality (3.1) that P(y+i)=y+i holds if and only if y+i=0, then y+i=0 is a unique fixed point of P(y+i), which corresponds to a unique boundary order-1 periodic solution with initial point ((1−PET)ET,0) for system (1.1). This completes the proof.
Theorem 5. For case (C)(C11)(i), if τ>Ymax or yQ+2<Ymin (where yQ+2 is the vertical component of point Q+2, yQ+2=Yis+τ1+θYis), then there exists an order-1 periodic solution for system (1.1).
Proof. For case (C)(C11)(i), if τ>Ymax or yQ+2<Ymin, then any solution of system (1.1) will map to the phase set with y+∈[τ,yQ+2] after a single impulsive effect. The point Q+2 is the pulse point of Q2, and it is the highest impulsive point, the solution which crosses Q+2 will reach L2 and experiences impulsive effects, and the pulse point will be located below the point Q+2. Thus we have
P(yQ+2)<yQ+2. | (3.2) |
Moreover, the point Pτ((1−PET)ET,τ) is the lowest impulsive point, the solution which crosses Pτ will reach L2 and experiences impulsive effects, and the pulse point will be located above Pτ, we will obtain
P(τ)>τ. | (3.3) |
On the basis of the continuity of P(y+i), it follows from the inequalities (3.2) and (3.3) that there exists at least one fixed point y∗∈(τ,yQ+2), i.e., P(y∗)=y∗, which corresponds to an order-1 periodic solution for system (1.1). This completes the proof.
Theorem 6. For case (C)(C11)(ii), if τ<Ymin or yQ+2>Ymax, then there exists an order-1 periodic solution for system (1.1).
Proof. For case (C)(C11)(ii), we have known that F(y) is decreasing on [0,Yis). If τ<Ymin or yQ+2>Ymax\rm, then any solution of system (1.1) will map to the phase set with y+∈[yQ+2,τ] after a single impulsive effect. Thus, the point Pτ((1−PET)ET,τ) is the highest impulsive point, which indicated that
P(τ)<τ. | (3.4) |
Furthermore, the point Q+2 is the lowest impulsive point. Then, the solution which initiating from Q+2 will satisfy the following relationship:
P(yQ+2)>yQ+2. | (3.5) |
It follows from the inequalities (3.4) and (3.5) that there exists at least one fixed point y∗∈(yQ+2,τ), i.e., there exists an order-1 periodic solution for system (1.1).
Theorem 7. For case (C)(C11)(iii), if yQ+2<Ymin or yQ+>Ymax, and the phase set of system (1.1) is {(x+,y+)∈R2+|x+=(1−PET)ET,yQ+≤y+≤yQ+2} or {(x+,y+)∈R2+|x+=(1−PET)ET,yQ+≤y+≤τ}, then there exists an order-1 periodic solution for the system (1.1).
Proof. For case (C)(C11)(iii), if yQ+2<Ymin, and the trajectories of system (1.1) map to the phase set with y+∈[yQ+,yQ+2] or [yQ+,τ] after impulsive effects, then we can discuss the existence of the fixed point of P(y+i) for the following two cases:
(1) yQ+2>τ. It has been proved that the impulsive function F(y) is decreasing on [0,yQ] and increasing on (yQ,Yis] for case (C)(C11)(iii). Then, if yQ+2>τ, then the point Q+2 is the highest impulsive point and the point Q+ is the lowest one, where Q+((1−PET)ET,yQ+) is the pulse point of Q(ET,yQ). Moreover, any solution of system (1.1) will map to the phase set with y+∈[yQ+,yQ+2] after a single impulsive effect. Due to the uniqueness of any two solutions, the inequality P(yQ+2)<yQ+2 (3.2) and the following inequality is true:
P(yQ+)≥yQ+. | (3.6) |
Therefore, it follows from (3.2) and (3.6) that there exists at least one fixed point y∗∈[yQ+,yQ+2), which corresponds to an order-1 periodic solution of system (1.1).
(2) yQ+2<τ. For this case, the trajectory of system (1.1) will map to the phase set with y+∈[yQ+,τ] after impulsive effects, Pτ is the highest impulsive point and Q+ is the lowest one. It is easy to know that the inequalities (3.4) and (3.6) are true, then there exists at least one fixed point y∗∈[yQ+,τ), which is indicated that there exists an order-1 periodic solution for system (1.1).
For case (C)(C11)(iii), if yQ+>Ymax, and the trajectories of system (1.1) map to the phase set with y+∈[yQ+,yQ+2] or [yQ+,τ] after impulsive effects, the same conclusions can be proved by using the similar methods. This completes the proof.
Theorem 8. If yQ+2<Ymin for case (C)(C11)(i) (or if yQ+2>Ymax for case (C)(C11)(ii)), then the fixed point y∗ is globally stable provided that it is unique.
Proof. From Theorem 5 we know that if yQ+2<Ymin for case (C)(C11)(i), then there exists a fixed point y∗∈(τ,yQ+2). Assuming it is unique, next we will discuss the global stability of the y∗. For one thing, we have proved that P(y+i) is increasing on [0,Ymin), then y+i<P(y+i)<y∗ for any y+i∈[0,y∗], which indicated that Pk(y+i) is monotonically increasing as k increase, and we have limk→+∞Pk(y+i)=y∗ for y+i∈[0,y∗); Moreover, y∗<P(y+i)<y+i for any y+i∈(y∗,Ymin), which means that Pk(y+i) is monotonically decreasing as k increase, and limk→+∞Pk(y+i)=y∗ for y+i∈[y∗,Ymin). For another, P(y+i)∈[τ,yQ+2] for any y+i∈(Ymax,+∞) is true, then we have limk→+∞Pk+1(y+i)=y∗ for y+i∈(Ymax,+∞). In conclusion, if yQ+2<Ymin for case (C)(C11)(i), then the fixed point y∗ is globally stable provided that it is unique.
If yQ+2>Ymax for case (C)(C11)(ii), the conclusions can be proved by using the similar methods. This completes the proof.
Theorem 9. For case (C)(C11)(ii), if τ<Ymin, and P2(y+i)>y+i for y+i∈[0,y∗) (or for case (C)(C11)(i), if τ>Ymax, and P2(y+i)>y+i for y+i∈(Ymax,y∗)), then there exists an unique fixed point y∗, and it is globally stable.
Proof. From Theorem 6 we know that if τ<Ymin for case (C)(C11)(ii), then any solution of system (1.1) will map to the phase set with y+∈[yQ+2,τ] after a single impulsive effect, and there exists a fixed point y∗∈(yQ+2,τ)⊂[0,Ymin). Moreover, as Poincaré map P(y+i) is decreasing on [0,Ymin), therefore, y∗ is the unique fixed point of P(y+i).
The global stability of y∗ can be discussed as follows. Firstly, P(y+i)>y∗ for y+i∈[0,y∗) due to the monotonicity of P(y+i), and if P2(y+i)>y+i for y+i∈[0,y∗), then the inequality y+i<P2(y+i)<y∗ holds true. By induction, we conclude that P2(k−1)(y+i)<P2k(y+i)<y∗, k≥1. It means that P2k(y+i) monotonically increasing as k increases, and limk→+∞P2k(y+i)=y∗,y+i∈[0,y∗). Secondly, according to the analytic expression of P(y+i) and the properties of Lambert W function, it's not hard to deduce limy+i→+∞P(y+i)=τ, which indicated that if τ<Ymin, then P(y+i)∈(0,y∗] or P2(y+i)∈(0,y∗) is true for any y+i∈(y∗,Ymin)∪(Ymax,+∞), combined with the above analysis, we infer that limk→+∞Pj+2k(y+i)=y∗ (j=1 or 2). In summary, the unique fixed point y∗ is globally stable.
For case (C)(C11)(i), if τ>Ymax, and P2(y+i)>y+i for y+i∈(Ymax,y∗), the conclusions can be proved by employing the same methods. This completes the proof.
Theorem 10. For case (C)(C11)(iii), if there exists a unique fix point y∗ for the the Poincaré map P(y+i), then we can draw the following conclusions:
(1) If yQ+>Ymax, and the phase set is {(x+,y+)∈R2+|x+=(1−PET)ET,yQ+≤y+≤yQ+2} or {(x+,y+)∈R2+|x+=(1−PET)ET,yQ+≤y+≤τ}, then the fixed point y∗ is globally stable provided that one of the following three conditions is satisfied, (a) y∗>ym1; (b) y∗=ym1; (c) y∗<ym1, and P2(y+i)<y+i for any y+i∈(y∗,ym1].
(2) If yQ+2<Ymin, and the phase set is {(x+,y+)∈R2+|x+=(1−PET)ET,yQ+≤y+≤yQ+2} or {(x+,y+)∈R2+|x+=(1−PET)ET,yQ+≤y+≤τ}, then the fixed point y∗ is globally stable provided that one of the following three conditions is satisfied, (a) y∗>ym2; (b) y∗=ym2; (c) y∗<ym2, and P2(y+i)<y+i for any y+i∈(y∗,ym2).
Proof. (1) It follows from Theorem 7 that for case (C)(C11)(iii), if yQ+>Ymax, and any solution of system (1.1) will map to the phase set with [yQ+,yQ+2] or [y+Q,τ] after impulsive effects, then there exists at least one fixed point y∗ for P(y+i), and y∗>yQ+>Ymax. Assuming the fixed point y∗ is unique, the global stability of y∗ can be discussed for the following three situations according to the value of y∗ and ym1:
(a) y∗>ym1. In this situation, there must be y+Q>ym1 based on the uniqueness of y∗. It's easy to see P(y+i) is increasing on [ym1,y∗), and y+i<P(y+i)<y∗ for any y+i∈[ym1,y∗), by induction, we know Pk(y+i) is monotonically increasing as k increase, and limk→+∞Pk(y+i)=y∗; For any y+i∈(y∗,+∞), y∗<P(y+i)<y+i, Pk(y+i) is monotonically decreasing as k increase, and limk→+∞Pk(y+i)=y∗. Furthermore, there must be P(y+i)∈(ym1,+∞) for any y+i∈[0,Ymin)∪(Ymax,ym1), limk→+∞P1+k(y+i)=y∗ is true.
(b) y∗=ym1. From the conclusion of case (a) we know that y∗≤P(y+i)<y+i for any y+i∈[ym1,+∞), Pk(y+i) is monotonically decreasing as k increase, and limk→+∞Pk(y+i)=y∗. For y+i∈[0,Ymin)∪(Ymax,ym1), there must be P(y+i)∈(ym1,+∞), and limk→+∞Pk+1(y+i)=y∗ is true.
(c) y∗<ym1. For this case, we know that y∗⊂[yQ+,ym1)⊂(Ymax,ym1), and the Poincaré map P(y+i) is decreasing on (y∗,ym1]. If P2(y+i)<y+i for any y+i∈(y∗,ym1], then y∗<P2(y+i)<y+i, and P2k(y+i) is monotonically decreasing as k increase, it means that limk→+∞P2k(y+i)=y∗. Moreover, for any y+i∈[0,Ymin)∪(Ymax,y∗)∪(ym1,+∞), there exists a positive integer l such that Pl(y+i)∈[y∗,ym1], and we have limk→+∞Pl+2k(y+i)=y∗.
In summary, the results shown in case (1) are true. For case (2), the conclusions can be proved in a similar way. This completes the proof.
Theorem 11. For case (C)(C12)(i), if yD0<yD+2<yC0 or yD+2<yD0 or τ>yC0, (where yD0, yD+2 and yC0 are the vertical component of points D0, D+2 and C0, respectively), then there exists an order-1 periodic solution for system (1.1).
Proof. For case (C)(C12)(i), the solution which passes through the point D0 intersects with M2 at two points, the lower intersection point is D2, and D+2 is the resetting point of D2, i.e., P(yD0)=yD+2. If yD0<yD+2<yC0, then the phase set is {(x+,y+)∈R2+|x+=(1−PET)ET,τ≤y+≤yD+2}, Pτ is the lowest impulsive point and D+2 is the highest one, therefore, we have the inequalities (3.3): P(τ)>τ, and
P(yD+2)<yD+2. | (3.7) |
It follows from (3.3) and (3.7) that there exists at least one fixed point y∗∈(τ,yD+2), i.e., there exists an order-1 periodic solution for system (1.1). If yD+2<yD0 or τ>yC0 for case (C)(C12)(i), the conclusions can be proved by using the similar methods. This completes the proof.
Theorem 12. For case (C)(C12)(ii), if τ<yC0 or yD+2>yC0, then there exists an order-1 periodic solution for system (1.1).
Proof. It has been proved that the impulsive function F(y) is decreasing on [0,Y1is) for case (C)(C12)(ii). If τ<yC0 or yD+2>yC0, then the phase set is {(x+,y+)∈R2+|x+=(1−PET)ET,yD+2≤y+≤τ}, Pτ is the highest impulsive point and D+2 is the lowest one. Therefore, we have the inequalities (3.4): P(τ)<τ, and
P(yD+2)>yD+2. | (3.8) |
In conclusion, there exists at least one fixed point y∗∈(yD+2,τ), which indicated that there exists an order-1 periodic solution for system (1.1). This completes the proof.
Theorem 13. For case (C)(C12)(iii), there exists an order-1 periodic solution for system (1.1) if either of the following conditions is satisfied.
(1) yD+2>τ, and yD+2<yC0 (or yQ+>yC0).
(2) yD+2<τ, and τ<yC0 (or yQ+>yC0 or yD+2<yD0).
Proof. (1) For case (C)(C12)(iii), if τ<yD+2<yC0 (or yD+2>τ and yQ+>yC0), then the phase set is {(x+,y+)∈R2+|x+=(1−PET)ET,yQ+≤y+≤yD+2}, the point D+2 is the highest impulsive point and Q+ is the lowest one. Therefore, the inequalities (3.7): P(yD+2)<yD+2 and
P(yQ+)≥yQ+ | (3.9) |
are true. In summary, there exists at least one fixed point y∗∈[yQ+,yD+2).
(2) If yD+2<τ, and τ<yC0 or yQ+>yC0, it is easy to know that the solution of system (1.1) will map to the phase set with y+∈[y+Q,τ] after impulsive effects, Pτ is the highest impulsive point and Q+ is the lowest one. Therefore, the two inequalities (3.4): P(τ)<τ, and (3.9): P(yQ+)≥yQ+ are true, which indicated that there exists at least one fixed point y∗∈[yQ+,τ).
If yD+2<yD0, i.e., P(yD0)<yD0, then combined with the inequality (3.9) we can infer that there exists at least one fixed point y∗∈[yQ+,yD0).
In conclusion, the results shown in Theorem 13 are true. This completes the proof.
Theorem 14. For case (C)(C12), if yD+2=yD0, then the trajectory ^D0D2 is an order-1 periodic solution of system (1.1).
Proof. For case (C)(C12), if yD+2=yD0, i.e., P(yD0)=yD0, then the fixed point y∗=yD0, the trajectory ^D0D2 is an order-1 periodic solution for system (1.1). This completes the proof.
Theorem 15. For case (C)(C12)(i), if there exists a fixed point y∗ of Poincaré map P(y+i) for the system (1.1), the stability of y∗ is as follows:
(1) If the fixed point y∗ is unique, then it is globally stable provided either of the following conditions is true.
(a) P(yD0)<yD0;
(b) yD0<P(yD0)<yC0, and P2(y+i)>y+i for any y+i∈[yD0,y∗).
(2) If τ>yC0, then the fixed point y∗ is unique, and y∗ is globally stable provided that P2(y+i)>y+i for any y+i∈[τ,y∗).
Proof. (1) (a): It follows from Theorem 11 that if P(yD0)<yD0 for case (C)(C12)(i), there exists a fixed point y∗, and y∗∈(τ,yD+2)⊂(0,yD0). Assuming the fixed point y∗ of P(y+i) is unique, then we have y+i<P(y+i)<y∗ for any y+i∈[τ,y∗) according to the monotonicity of P(y+i), it means that Pk1(y+i) is monotonically increasing as k1 increase, and limk1→+∞Pk1(y+i)=y∗; Moreover, y∗<P(y+i)<y+i for any y+i∈(y∗,yD+2], Pk2(y+i) is monotonically decreasing as k2 increase, and limk2→+∞Pk2(y+i)=y∗ is also true. In summary, if P(yD0)<yD0, and the fixed point y∗ is unique, then it is globally stable.
(1) (b): It has been proved that P(y+i) is decreasing on [yD0,yC0) for case (C)(C12)(i). If yD0<P(yD0)<yC0, then the phase set is {(x+,y+)∈R2+|x+=(1−PET)ET,τ≤y+≤yD+2}, and it is easy to know P2(yD0)<P(yD0), i.e., P(yD+2)<yD+2. Therefore, the fixed point y∗∈(yD0,yD+2)⊂[τ,yD+2]. Assuming the fixed point y∗ is unique, the stability of y∗ will be discussed in three subinterval: [yD0,y∗), (y∗,yD+2), [τ,yD0].
First of all, according to the monotonicity of P(y+i), we have P(yD0)≥P(y+i)>y∗ and P2(yD0)≤P2(y+i)<y∗ for any y+i∈[yD0,y∗), combined with the inequality P2(y+i)>y+i for y+i∈[yD0,y∗), we will obtain that y+i<P2(y+i)<y∗. By induction, it is easy to know P2(k−1)(y+i)<P2k(y+i)<y∗, which means that P2k(y+i) is monotonically increasing and tends to y∗ as k increase, P2k+1(y+i) is monotonically decreasing and tends to y∗ as k increase. Secondly, we know P(y+i)∈(y∗,yD+2] for any y+i∈[yD0,y∗), base on the conclusion of the former part, limk→+∞P2k(y+i)=y∗ or limk→+∞P2k+1(y+i)=y∗ for any y+i∈(y∗,yD+2] is true. Thirdly, it follows from the monotonicity of P(y+i) that there must exist a positive integer n such that Pn(y+i)∈[yD0,y∗] or (y∗,yD+2) for any y+i∈[τ,yD0). In conclusion, for case (C)(C12)(i), the fixed point y∗ is globally stable provided the conditions (1)b are satisfied.
(2) If τ>yC0, from Theorem 11 we know that there exists a fixed point y∗, and the phase set is {(x+,y+)∈R2+|x+=(1−PET)ET,τ≤y+≤yD+2}. It means that y∗∈[τ,yD+2]⊂[yC0+∞). We have proved that P(y+i) is decreasing on (yD0,yC0)∪(yC0,+∞), then the fixed point y∗ is unique, obviously.
As for the stability of y∗, for one thing, based on the monotonicity of P(y+i), y+i<y∗<P(y+i) for any y+i∈[τ,y∗) is true, combined with P2(y+i)>y+i, we have y∗>P2(y+i)>y+i, by induction, y∗>P2k(y+i)>P2(k−1)(y+i) or y∗<P2k+1(y+i)<P2k−1(y+i) is true, Pk(y+i) is monotone increasing and tends to y∗ as k increase. For another, P(y+i)∈(τ,y∗) for y+i∈(y∗,yD+2], therefore, it can be inferred that limk→+∞Pk(y+i)=y∗. In conclusion, if τ>yC0 for case (C)(C12)(i), the fixed point y∗ of system (1.1) is unique, and y∗ is globally stable provided that P2(y+i)>y+i for y+i∈[τ,y∗). This completes the proof.
Theorem 16. For case (C)(C12)(ii), if there exists a unique fix point y∗ for the Poincaré map P(y+i), then y∗ is globally stable provided that one of the following three conditions is satisfied.
(1) τ<yC0 and yD0<yD+2;
(2) τ<yC0, yD+2<yD0, and P2(y+i)<y+i for any y+i∈(y∗,yD0];
(3) yD+2>yC0.
Proof. (1) If τ<yC0 and yD0<yD+2, it follows from Theorem 12 that any solution of system (1.1) will map to the phase set is {(x+,y+)∈R2+|x+=(1−PET)ET,yD+2≤y+≤τ} after impulsive effects, and there exists a fixed point y∗∈(yD+2,τ)⊂(yD0,yC0), assuming it is unique. It has been proved that P(y+i) is increasing on (yD0,yC0). Therefore, we can infer that Pk1(y+i) is monotone increasing and tends to y∗ as k1 increase for any y+i∈[yD+2,y∗), and Pk2(y+i) is monotone decreasing and tends to y∗ as k2 increase for any y+i∈(y∗,τ]. In summary, the fixed point y∗ is globally stable provided it is unique.
(2) According to the monotonicity of the impulsive function F(x), if yD+2<yD0, i.e., P(yD0)<yD0, then we have P(yD+2)>yD+2, which indicated that y∗∈(yD+2,yD0). Assuming the fixed point is unique, from Theorem 3 we know that P(y+i) is decreasing on [0,yD0], therefore, P(y+i)<y∗<y+i for any y+i∈(y∗,yD0] is true; If P2(y+i)<y+i for any y+i∈(y∗,yD0], it is easy to know y∗<P2(y+i)<y+i. By induction, we can infer that y∗<P2k(y+i)<P2(k−1)(y+i), and limk→+∞P2k(y+i)=y∗ for y+i∈(y∗,yD0]. Moreover, on the basis of τ<yC0 andlimy+i→+∞P(y+i)=τ, there must exist a positive integer l such that Pl(y+i)∈[y∗,yD0] and limk→+∞P2k+l(y+i)=y∗ for any y+i∈[0,y∗)∪(yD0,yC0)∪(yC0,+∞). In conclusion, if τ<yC0 and yD+2<yD0, and P2(y+i)<y+i for any y+i∈(y∗,yD0], then the unique fixed point y∗ is globally stable.
(3) For this case, if yD+2>yC0, then any solution of system (1.1) will map to the phase set {(x+,y+)∈R2+|x+=(1−PET)ET,yD+2≤y+≤τ} after impulsive effects, and there exists a fixed point y∗∈(yD+2,τ)⊂(yC0,+∞). Assuming the fixed point is unique, according to the monotonicity of P(y+i), it is easy to see that Pk1(y+i) is increasing and tends to y∗ as k1 increase for any y+i∈[yD+2,y∗), and Pk2(y+i) is decreasing and tends to y∗ as k2 increase for any y+i∈(y∗,τ]. In summary, if yD+2>yC0, then the unique fixed point y∗ is globally stable. This completes the proof.
Theorem 17. For case (C)(C12)(iii), if there exists a unique fix point y∗ for the Poincaré map P(y+i), and Yis<√τθ−1θ<Y1is, then y∗ is globally stable provided that one of the following four conditions is satisfied.
(a) P(yMi)>yC0(i=1,2);
(b) P(yM1)<yM1, P(yM2)>yM2, yD0<P(yD0)<yC0, τ<yC0, and y∗<P2(y+i)<y+i for any y+i∈(y∗,yM1];
(c) P(yM1)<yM1, P(yM2)>yM2, P(yD0)<yD0, τ<yC0;
(d) P(yMi)<yMi(i=1,2), τ<yC0, and P2(y+i)<y+i for any y+i∈(y∗,yM2].
Proof. For case (C)(C12)(iii), Yis<√τθ−1θ<Y1is means that the point Q is located between the points Q2 and D2, and the coordinates relationship of M1 and M2 is: 0<yM2<yD0<yM1<yC0.
(a) If P(yMi)=yQ+>yC0(i=1,2), then from the Theorem 13 we know that there exists a fixed point y∗, and the solution of system (1.1) will map to the phase set with y+∈[yQ+,yD+2] or [yQ+,τ] after impulsive effects. Assuming y∗∈[yQ+,τ] and it is unique, it has been proved that P(y+i) is increasing on [yQ+,τ], therefore, y+i<P(y+i)<y∗ for any y+i∈[yQ+,y∗) is true, and we conclude that Pk1(y+i) is increasing and tends to y∗ as k1 increase; Moreover, y∗<P(y+i)<y+i for any y+i∈(y∗,τ] is true, and Pk2(y+i) is decreasing and tends to y∗ as k2 increase. In summary, if P(yMi)>yC0, then the unique fixed point y∗ is globally stable. The analysis methods can also be used for y∗∈[yQ+,yD+2].
(b) If τ<yC0, and yD0<P(yD0)<yC0 i.e., yD0<yD+2<yC0, then any solution of system (1.1) will map to the phase set with y+∈[yQ+,yD+2] or [yQ+,τ] after impulsive effects. From P(yD0)>yD0 and P(yM1)<yM1 we know that the fixed point y∗∈(yD0,yM1). Assuming the phase set is {(x+,y+)∈R2+|x+=(1−PET)ET,yQ+≤y+≤yD+2} and y∗ is unique. It is easy to know yQ+∈[yD0,y∗) follows from y∗<P2(y+i)<y+i for any y+i∈(y∗,yM1], which means y∗∈(yQ+,yM1)⊂(yD0,yM1). According to the monotonicity of P(y+i), we infer that y∗<P2k(y+i)<P2(k−1)(y+i) for any y+i∈(y∗,yM1], it means that P2k(y+i) is decreasing and tends to y∗ as k increase, or P2k+1(y+i) is increasing and tends to y∗ as k increase; Moreover, we have P(y+i)∈(y∗,yM1) for any y+i∈[yQ+,y∗); If yD+2>yM1, for any y+i∈(yM1,yD+2], there must exist a positive integer l such that Pl(y+i)∈[y∗,yM1]; In conclusion, limk→+∞Pk(y+i)=y∗ for y+i∈[yQ+,yD+2] is true, and the unique fixed point y∗ is globally stable. If the phase set is {(x+,y+)∈R2+|x+=(1−PET)ET,yQ+≤y+≤τ}, the conclusions can be proved by using the similar analysis methods.
(c) For this case, the solution of system (1.1) will map to the phase set with y+∈[yQ+,yD+2] or [yQ+,τ] after impulsive effects. Q+ is the lowest pulse point, then P(yQ+)>yQ+ is true, combined with the inequality P(yD0)<yD0, we know that the fixed point y∗ is located in the interval (yQ+,yD0), assuming it is unique. If the phase set is {(x+,y+)∈R2+|x+=(1−PET)ET,yQ+≤y+≤yD+2}, then y∗∈(yQ+,yD+2) ⊂(yQ+,yD0). According to the monotonicity of P(y+i), it is easy to infer that Pk(y+i) is increasing and tends to y∗ as k increase for any y+i∈[yQ+,y∗), and Pk(y+i) is decreasing and tends to y∗ as k increase for any y+i∈(y∗,yD+2], thus the fixed point y∗ is globally stable. If the phase set is {(x+,y+)∈R2+|x+=(1−PET)ET,yQ+≤y+≤τ} and yD0≥τ, the conclusions can be proved in a similar way; Moreover, if yD0<τ<yC0, there must exist a positive integer l such that Pl(y+i)∈[yQ+,yD+2] for y+i∈(yD+2,τ]. In conclusion, the unique fixed point y∗ is globally stable.
(d) For this case, Q+ is the lowest pulse point, then P(yQ+)>yQ+ is true, then combined with P(yM2)<yM2, we can infer that the fixed point y∗∈(yQ+,yM2), assuming it is unique. If P(yMi)<yMi(i=1,2) and τ<yC0, it is easy to know P(yD0)<yD0 follows from the monotonicity of P(y+i) and uniqueness of y∗, and y+<yC0 is true for the point (x+,y+) of phase set. According to the monotonicity of P(y+i) on (y∗,yM2] and P2(y+i)<y+i for any y+i∈(y∗,yM2], we can speculate that P2k(y+i) is decreasing and tends to y∗ as k increase. Furthermore, P(y+i)∈(y∗,yM2) for y+i∈[yQ+,y∗) is true, and there must exist a positive integer l such that Pl(y+i)∈(y∗,yM2] for any y+i∈[yM2,yC0). Therefore, for the point (x+,y+) of phase set, limk→+∞Pk(y+)=y∗ is true. In conclusion, the unique fixed point y∗ is globally stable if the conditions of the theorem are satisfied. This completes the proof.
Theorem 18. For case (C)(C12)(iii), if there exists a unique fix point y∗ for the Poincaré map P(y+i), and 0<√τθ−1θ≤Yis, then y∗ is globally stable provided that one of the following four conditions is satisfied.
(a) P(yMi)>yMi(i=1,2);
(b) P(yM1)<yM1, P(yM2)>yM2, yQ+>yC0, and y∗<P2(y+i)<y+i for any y+i∈(y∗,yM1];
(c) P(yM1)<yM1, P(yM2)>yM2, P(yD0)<yD0, and τ<yC0;
(d) P(yMi)<yMi(i=1,2), τ<yC0, and P2(y+i)<y+i for any y+i∈(y∗,yM2].
Proof. For case (C)(C12)(iii), 0<√τθ−1θ≤Yis means that the point Q is located below the point Q2, the coordinates relationship of M1 and M2 is: 0<yM2<yD0<yC0<yM1.
(a) If P(yMi)>yMi(i=1,2), then any solution of system (1.1) will map to the phase set with y+∈[yQ+,yD+2] or [yQ+,τ] after impulsive effects. If the phase set is {(x+,y+)∈R2+|x+=(1−PET)ET,yQ+≤y+≤τ}, then Pτ is the highest impulsive point and Q+ is the lowest one, it follows from P(yQ+)>yQ+ and P(τ)<τ that the fixed point y∗∈(yQ+,τ)⊂(yM1,+∞), assuming it is unique. Because the Poincaré map P(y+i) is increasing on [yM1,+∞), it is easy to know that Pk(y+i) is increasing and tends to y∗ as k increase for any y+i∈[yQ+,y∗), and Pk(y+i) is decreasing and tends to y∗ as k increase for any y+i∈(y∗,τ], which indicated that the unique fixed point y∗ is globally stable. If the phase set is {(x+,y+)∈R2+|x+=(1−PET)ET,yQ+≤y+≤yD+2}, the conclusions can be proved by using the similar methods.
(b) If P(yM1)=P(yM2)=yQ+>yC0, then any pulse point will be located above the point C0, and the solution of system (1.1) will map to the phase set with y+∈[yQ+,yD+2] or [yQ+,τ] after impulsive effects, Q+ is the lowest impulsive point. It follows from P(yQ+)>yQ+ and P(yM1)<yM1 that the fixed point y∗∈(yQ+,yM1), assuming it is unique. It is has been proved that P(y+i) is decreasing on [yQ+,yM1] and increasing on (yM1,+∞), if y∗<P2(y+i)<y+i for any y+i∈(y∗,yM1], then we can infer that y∗<P2k(y+i)<P2(k−1)(y+i), which means limk→+∞P2k(y+)=y∗ for y+i∈(y∗,yM1]. Furthermore, P(y+i)∈(y∗,yM1) for any y+i∈(yQ+,y∗) is true, and there must exist a positive integer l such that Pl(y+i)∈[y∗,yM1] for any y+i∈(yM1,yD+2] or (yM1,τ]. In summary, the unique fixed point y∗ is globally stable.
For case (c) and case (d), it can be noticed that the conditions of Theorem 18 are the same as Theorem 17 for these two cases, respectively. Therefore, the global stability of the fixed point y∗ can be proved by taking advantage of the similar method. This completes the proof.
In the previous subsection, we have analyzed the existence and global stability of order-1 periodic solutions. The existence of order-k(k≥2) periodic solutions of system (1.1) will be discussed as follows.
Theorem 19. If one of the following conditions is satisfied, then there only exists order-1 periodic solutions for system (1.1).
(1) For case (C)(C11)(i), yQ+2<Ymin;
(2) For case (C)(C11)(ii), yQ+2>Ymax;
(3) For case (C)(C12)(i), yD+2<yD0;
(4) For case (C)(C12)(ii), yD+2>yC0;
(5) For case (C)(C12)(ii), τ<yC0 and yD+2>yD0.
Proof. From the analysis of previous subsection we know that there exists an order-1 periodic solution for system (1.1) if one of the five conditions of Theorem 19 is satisfied.
For case (C)(C11)(i), it has been proved that Poincaré map P(y+i) is increasing on [0,Ymin). If yQ+2<Ymin, then the phase set is {(x+,y+)∈R2+|x+=(1−PET)ET,τ≤y+≤yQ+2}. Assuming the order-1 periodic solution passes through the points P(ET,η0) and P+((1−PET)ET,η+0), which are located on the line L2 and L3, respectively. The trajectory initiating from point Q+2 intersects with line L2 at point Q3, the point Q3 is located below the point Q2 according to the disjointness of any two trajectories. Moreover, we have proved that the pulse function F is increasing on [0,Yis], then the pulse point Q+3 will lie below the point Q+2, by induction, the relationship of the series of pulse points is: Ymin>yQ+2>yQ+3>yQ+4>⋯>yQ+i>⋯>η+0>⋯>τ. It means that the series of pulse points Q+i is decreasing as i increase, a periodic solution with order-k(k≥2) does not exist for system (1.1).
For case (C)(C12)(ii), it has been proved that Poincaré map P(y+i) is decreasing on [0,yD0], and increasing on (yD0,Ymax). If τ<yC0 and yD+2>yD0, then the solution of system (1.1) will map to the phase set with y+∈[yD+2,τ] after impulsive effects, [yD+2,τ]⊂(yD0,Ymax). The solution initiating from point D+2 intersects with line L2 at D3 and then experiences impulsive effects, D+3 is the pulse point of D3, it is obviously that D3 lies below D2, and D+3 lies above D+2, by induction, the relationship about the coordinate of pulse points is: yD0<yD+2<yD+3<⋯<η+0<⋯<τ, where η+0 is the fixed point of P(y+i). Therefore, an order-k(k≥2) periodic solution does not exist for system (1.1) in the view of this relationship of the pulse points.
If yQ+2>Ymax for case (C)(C11)(ii) or if yD+2<yD0 for case (C)(C12)(i) or if yD+2>yC0 for case (C)(C12)(ii), the conclusions can also be proved by using the same methods. This completes the proof.
Theorem 20. If one of the following conditions is satisfied, then there only exists order-1 or order-2 periodic solutions for system (1.1).
(1) For case (C)(C11)(i), τ>Ymax;
(2) For case (C)(C11)(ii), τ<Ymin;
(3) For case (C)(C12)(i), τ>yC0 (or yD0<yD+2<yC0 and yD+3≥yD0).
Proof. For case (C)(C11)(i), if τ>Ymax, then the phase set is {(x+,y+)∈R2+|x+=(1−PET)ET,τ≤y+≤yQ+2}. The solution which initiating from the point Q+2 intersects with line L2 at Q3, then map to Q+3 after a single impulsive effect. According to the monotonicity of the pulse function F(y) on [0,Yis], it is easy to see the pulse point Q+3 lies below the point Q+2; Moreover, the solution which passes through the point Q+3 intersects with line L2 at Q4, then the pulse point Q+4 lies between Q+2 and Q+3. By induction, we infer that the relationship about the vertical component of these pulse points is: Ymax<τ<yQ+3<yQ+5<⋯<yQ+2i−1<yQ+2i+1<⋯<yQ+2i+2<yQ+2i<⋯<yQ+4<yQ+2. From this relationship we see that there are two series of pulse point: yQ+2i+1 and yQ+2i, the series yQ+2i+1 is increasing as i increase and the series yQ+2i is decreasing as i increase. Therefore, we have limi→+∞yQ+2i+1=y∗1, limi→+∞yQ+2i=y∗2, and the limit value y∗1=y∗2 or y∗1≠y∗2, which means that there exists an order-1 or order-2 periodic solution for the system (1.1), and an order-k(k≥3) periodic solution does not exist.
If τ<Ymin for case (C)(C11)(ii) or if τ>yC0 for case (C)(C12)(i), or if yD0<yD+2<yC0 and yD+3≥yD0 for case (C)(C12)(i), the conclusions can also be proved by using the same methods. This completes the proof.
The IPM strategy based on chemical and biological techniques promotes the development of a series of impulsive predator-prey models [17,18,19,20,21,22,23,24,25,26,27,28,29]. The research of the models not only promoted the theoretical development of impulsive dynamical system, but also provided an effective foundation for control of pests. In order to control pests effectively and reduce the environmental pollution caused by the excessive use of chemical pesticides, the IPM strategy should be more realistic for practical environment. Considering the wide application of pest real-time monitoring technology in agriculture, we propose a predator-prey model concerning density guided releasing natural enemies and spraying pesticide with nonlinear state-dependent feedback control strategy. The main purpose of this paper is to explore the complex dynamic behavior of the model, and to reveal whether the pests can be effectively controlled under the interference of external environment and control measures, and research how the key factors affect the dynamic behaviors.
Firstly, we summarize the basic conclusions of the corresponding ODE system of (1.1) in the case of no external interference. Secondly, in order to study the dynamics of model (1.1) under impulsive disturbance, we make a qualitative analysis for the model (1.1) by using the basic theory of impulsive semi-dynamic system: a basic pulse set M and the corresponding basic phase set N are defined according to the isoline. Due to the fact that there is a saddle point and a center in the system, the judgment of the exact impulsive and phase sets needs to be classified and discussed according to the position relationship between the saddle point, the center and the line where the impulsive set and the phase set are located in. We obtain the coordinates of important points on the solution trajectory by using the first integral and Lambert W function. The exact impulsive sets under different conditions with pulse interference are determined, and the exact phase sets of two representative cases (C)(C11) and (C)(C12) are discussed, as shown in Table 1. We analysed the conditions about the solution of system (1.1) will be free from impulsive effects, and the analytic expression of Poincaré map P(y+i) for the sequence of impulsive points is defined. Table 2 shows the relationship between the domain of Poincaré map and some important parameters. According to the value range of the key parameter √τθ−1θ, we study the properties of Poincaré map, such as the monotonicity of P(y+i). On the basis of these analysis, the existence, uniqueness, stability of the order-1 periodic solutions of system (1.1) in case (C)(C11) and (C)(C12) and the existence of order-k(k≥2) periodic solutions have been provided.
Compared with the IPM model that has been studied before, we have consider more practical elements, such as the anti-predator behavior which can reflect the interaction between pests and natural enemies[16,21,24,29], the killing rate of pests and the amount of natural enemies to be released are depend on the real-time density of them. In particular, the natural enemy density regulatory factor θ in the model not only reflecting the application of real-time monitoring and early warning technology for plant diseases and insect pests in agriculture, but also brings new research subjects and challenges for the nonlinear impulsive prey-predator model. The discussion of the definition and properties of Poincaré map, the existence and stability of periodic solutions fully reflects the complexity of the dynamic behavior of the density-dependent nonlinear model.
The theoretical analysis results of the article show that the effective control of pests depends on the initial density of pests and natural enemies, and the implementation of control methods. The dynamic behavior of the system is affected by the maximum killing rate of pests, the half-saturation constant, the maximum amount of natural enemies to be released and regulatory factor in the nonlinear term of the system, and all these factors will determine whether the pests can be controlled. It can be seen that under some conditions, there exists a unique globally asymptotically stable order-1 periodic solution for the system, which means that the pests and natural enemies can coexist. As long as the pest monitoring technology is fully utilized, spraying insecticides and releasing natural enemies reasonably, the pests can be controlled economically and effectively without destroying the ecological balance. The theoretical analysis of the article verifies that the model is meaningful for the research of IPM strategies, and it promotes the further development of nonlinear state-dependent feedback control system. Due to the complexity of the system, we only selected two representative case (C)(C11) and case (C)(C12) for discussion in the analysis of the exact phase set and Poincaré map. Therefore, the novel analytical techniques should be developed in near future, and more generalized models with complex nonlinear impulsive control effects could be investigated.
This work was partially supported by the National Natural Science Foundation of China (NSFCs: 12031010, 11631012, 61772017, 11961024), and by the Scientific Research Foundation of the Education Department of Hubei Province B2018091, and by Doctorial Foundation of Hubei Minzu University MD2020B020.
All authors declare that they have no competing interests.
[1] |
V. Volterra, Variations and fluctuations of the number of individuals in animal species living together, ICES J. Mar. Sci., 3 (1928), 3–51. doi: 10.1093/icesjms/3.1.3
![]() |
[2] |
A. Lotka, Undamped oscillations derived from the law of mass action, J. Am. Chem. Soc., 42 (1920), 1595–1599. doi: 10.1021/ja01453a010
![]() |
[3] |
C. Holling, The functional response of predators to prey density and its role in mimicry and population regulation, Mem. Entomol. Soc. Can., 97 (1965), 1–60. doi: 10.4039/Ent971-1
![]() |
[4] |
P. J. Wangersky, Lotka-volterra population models, Ann. Rev. Ecol. Syst., 9 (1978), 189–218. doi: 10.1146/annurev.es.09.110178.001201
![]() |
[5] |
B. Liu, Y. J. Zhang, L. S. Chen, Dynamic complexities in a lotka-volterra predator-prey model concerning impulsive control strategy, Int. J. Bifurcat. Chaos, 15 (2005), 517–531. doi: 10.1142/S0218127405012338
![]() |
[6] |
I. Perissi, U. Bardi, T. E. Asmar, A. Lavacchi, Dynamic patterns of overexploitation in fisheries, Ecol. Model., 359 (2017), 285–292. doi: 10.1016/j.ecolmodel.2017.06.009
![]() |
[7] |
A. T. Keong, H. M. Safuan, K. Jacob, Dynamical behaviours of prey-predator fishery model with harvesting affected by toxic substances, Matematika, 34 (2018), 143–151. doi: 10.11113/matematika.v34.n1.1018
![]() |
[8] | J. López-Gómez, R. Ortega, A. Tineo, The periodic predator-prey Lotka-Volterra model, Adv. Differential Equ., 1 (1996), 403–423. |
[9] |
L. L. Feng, Z. J. Liu, An impulsive periodic predator-prey Lotka-Volterra type dispersal system with mixed functional responses, J. Appl. Math Comput., 45 (2014), 235–257. doi: 10.1007/s12190-013-0721-x
![]() |
[10] | S. Choo, Global stability in n-dimensional discrete Lotka-Volterra predator-prey models, Adv. Differ. Equ.-NY., 11 (2014), 1–17. |
[11] | J. C. Van Lenteren, Integrated pest management in protected crops, in Integrated pest management, Chapman & Hall, (1995), 311–320. |
[12] |
M. Kogan, Integrated pest management: historical perspectives and contemporary developments, Annu. Rev. Entomol., 43 (1998), 243–270. doi: 10.1146/annurev.ento.43.1.243
![]() |
[13] |
M. Barzman, P. Bàrberi, A. N. E. Birch, P. Boonekamp, S. Dachbrodt-Saaydeh, B. Graf, et al., Eight principles of integrated pest management, Agron. Sustain. Dev., 35 (2015), 1199–1215. doi: 10.1007/s13593-015-0327-9
![]() |
[14] | M. L. Flint, R. Ven den Bosch, Introduction to integrated pest management, Springer-Verlag, New York, 1981. |
[15] | S. Y. Tang, L. S. Chen, Modelling and analysis of integrated pest management strategy, Discrete Cont. Dyn.-B, 4 (2004), 759–768. |
[16] |
S. Y. Tang, Y. N. Xiao, L. S. Chen, R. A. Cheke, Integrated pest management models and their dynamical behaviour, B. Math. Biol., 67 (2005), 115–135. doi: 10.1016/j.bulm.2004.06.005
![]() |
[17] |
B. Liu, Y. J. Zhang, L. S. Chen, L. H. Sun, The dynamics of a prey-dependent consumption model concerning integrated pest management, Acta. Math. Sin., 21 (2005), 541–554. doi: 10.1007/s10114-004-0476-2
![]() |
[18] |
X. N. Liu, L. S. Chen, Complex dynamics of Holling type II Lotka-Volterra predator-prey system with impulsive perturbations on the predator, Chaos, Solitons Fract., 16 (2003), 311–320. doi: 10.1016/S0960-0779(02)00408-3
![]() |
[19] | X. Wang, Y. Tian, S. Y. Tang: A Holling type II pest and natural enemy model with density dependent IPM strategy, Math. Probl. Eng., 2017 (2017), 8683207. |
[20] | G. Jiang, Q. Lu, The dynamics of a prey-predator model with impulsive state feedback control, Discrete Cont. Dyn.-B, 6 (2006), 1301–1320. |
[21] |
S. Y. Tang, B. Tang, A. L. Wang, Y. N. Xiao, Holling II predator-prey impulsive semi-dynamic model with complex Poincare map, Nonlinear Dynam., 81 (2015), 1575–1596. doi: 10.1007/s11071-015-2092-3
![]() |
[22] |
S. Y. Tang, R. A. Cheke, State-dependent impulsive models of integrated pest management (IPM) strategies and their dynamic consequences, J. Math. Biol., 50 (2005), 257–292. doi: 10.1007/s00285-004-0290-6
![]() |
[23] |
J. Yang, S. Y. Tang, Holling type II predator-prey model with nonlinear pulse as state-dependent feedback control, J. Comput. Appl. Math., 291 (2016), 225–241. doi: 10.1016/j.cam.2015.01.017
![]() |
[24] |
W. Gao, S. Y. Tang, The effects of impulsive releasing methods of natural enemies on pest control and dynamical complexity, Nonlinear Anal.- Hybri., 5 (2011), 540–553. doi: 10.1016/j.nahs.2010.12.001
![]() |
[25] |
T. Y. Wang, L. S. Chen, Nonlinear analysis of a microbial pesticide model with impulsive state feedback control, Nonlinear Dyn., 65 (2011), 1–10. doi: 10.1007/s11071-010-9828-x
![]() |
[26] | B. Liu, Y. Tian, B. L. Kang, Dynamics on a Holling II predator-prey model with state-dependent impulsive control, Int. J. Biomath, 5 (2012), 1–18. |
[27] |
S. Y. Tang, W. H. Pang, R. A. Cheke, J. H. Wu, Global dynamics of a state-dependent feedback control system, Adv. Differ. Equ., 2015 (2015), 322. doi: 10.1186/s13662-015-0661-x
![]() |
[28] |
G. Jiang, Q. Lu, L. Qian, Complex dynamics of a Holling type II prey-predator system with state feedback control, Chaos, Solitons Fract., 31 (2007), 448–461. doi: 10.1016/j.chaos.2005.09.077
![]() |
[29] | W. J. Qin, S. Y. Tang, R. A. Cheke, The effects of resource limitation on a predator-prey model with control measures as nonlinear pulses, Math. Probl. Eng., 2014 (2014), 450935. |
[30] | M. J. B. Vreysen, Monitoring sterile and wild insects in area-wide integrated pest management programmes, in Sterile Insect Technique (eds. V.A. Dyck, J. Hendrichs and A.S. Robinson), Springer-Verlag, (2005), 325–361. |
[31] |
R. L. Nadel, M. J. Wingfield, M. C. Scholes, S. A. Lawson, B. Slippers, The potential for monitoring and control of insect pests in Southern Hemisphere forestry plantations using semiochemicals, Ann. Forest Sci., 69 (2012), 757–767. doi: 10.1007/s13595-012-0200-9
![]() |
[32] |
F. W. Ravlin, Development of monitoring and decision-support systems for integrated pest management of forest defoliators in North America, Forest Ecol. Manag., 39 (1991), 3–13. doi: 10.1016/0378-1127(91)90156-P
![]() |
[33] |
A. R. Ives, A. P. Dobson, Antipredator behavior and the population dynamics of simple predator-prey systems, Am. Nat., 130 (1987), 431–447. doi: 10.1086/284719
![]() |
[34] |
R. Ramos-jiliberto, E. Frodden, A. Aránguiz-Acu\bar na, Pre-encounter versus post-encounter inducible defense in predator-prey systems, Ecol. Model., 200 (2007), 99–108. doi: 10.1016/j.ecolmodel.2006.07.023
![]() |
[35] |
Y. Sait\bar o, Prey kills predator: counter-attack success of a spider mite against its specific phytoseiid predator, Exp. Appl. Acarol., 2 (1986), 47–62. doi: 10.1007/BF01193354
![]() |
[36] | Y. Choh, M. Ignacio, M. W. Sabelis, A. Janssen, Predator-prey role reversals, juvenile experience and adult antipredator behaviour, Sci. Rep., 2 (2012), 1–6. |
[37] | I. U. Khan, S. Y. Tang, The impulsive model with pest density and its change rate dependent feedback control, Discrete Dyn. Nat. Soc., 2020 (2020), 4561241. |
[38] |
V. A. Kuznetsov, I. A. Makalkin, M. A. Talor, A. S. Perelson, Nonlinear dynamics of immunogenic tumors: Parameter estimation and global bifurcation analysis, Bull. Math. Biol., 56 (1994), 295–321. doi: 10.1016/S0092-8240(05)80260-5
![]() |
[39] |
B. Tang, Y. N. Xiao, S. Y. Tang, R. A. Cheke, A feedback control model of comprehensive therapy for treating immunogenic tumours, Int. J. Bifurcat. Chaos, 26 (2016), 1650039. doi: 10.1142/S0218127416500395
![]() |
[40] |
B. Tang, Y. N. Xiao, R. A. Cheke, N. Wang, Piecewise virus-immune dynamic model with HIV-1 RNA-guided therapy, J. Theor. Biol., 377 (2015), 36–46. doi: 10.1016/j.jtbi.2015.03.040
![]() |
[41] | Q. Li, Y. N. Xiao, Global dynamics of a virus-immune system with virus-guided therapy and saturation growth of virus, Math. Probl. Eng., 2018 (2018), 4710586. |
[42] | R. M. Corless, G. H. Gonnet, D. E. G. Hare, D. J. Jeffrey, D. E. Knuth, On the Lambert W Function, Adv. Comput. Math., 5 (1996), 329–359. |
1. | Yuan Tian, Yan Gao, Kaibiao Sun, A fishery predator-prey model with anti-predator behavior and complex dynamics induced by weighted fishing strategies, 2023, 20, 1551-0018, 1558, 10.3934/mbe.2023071 | |
2. | Liping Wu, Zhongyi Xiang, Dynamic analysis of a predator-prey impulse model with action threshold depending on the density of the predator and its rate of change, 2024, 9, 2473-6988, 10659, 10.3934/math.2024520 | |
3. | Doaa M. Fawzy, A. Elsaid, W. K. Zahra, Ayman A. Arafa, Qualitative analysis of a Filippov wild-sterile mosquito population model with immigration, 2023, 33, 1054-1500, 10.1063/5.0167157 | |
4. | Baolin Kang, Xiang Hou, Bing Liu, Threshold control strategy for a Filippov model with group defense of pests and a constant-rate release of natural enemies, 2023, 20, 1551-0018, 12076, 10.3934/mbe.2023537 |
Cases | (1−PET)ET | Is | Ps | |
(C11) | (i) | x∗3≤(1−PET)ET≤x∗1 | M1 | N11 |
(ii) | N12 | |||
(iii) | N13∪N14 | |||
(C12) | (i) | (1−PET)ET>x∗1 | M2 | N21 |
(ii) | N22 | |||
(iii) | N23∪N24 |
Cases | (1−PET)ET | A1 and A2 | P(y+i) | |
(C)(C11) | (i) | x∗3≤(1−PET)ET≤x∗1 | A2≥0, △ | y+i∈Y011 |
(ii) | y+i∈Y012 | |||
(iii) | y+i∈Y013∪Y014 | |||
(C)(C12) | (i) | (1−PET)ET>x∗1 | A1<0, A2>0 | y+i∈Y021 |
(ii) | y+i∈Y022 | |||
(iii) | y+i∈Y023∪Y024 | |||
△ means the sign of A1 is not necessary for case (C)(C11). |
Cases | (1−PET)ET | Is | Ps | |
(C11) | (i) | x∗3≤(1−PET)ET≤x∗1 | M1 | N11 |
(ii) | N12 | |||
(iii) | N13∪N14 | |||
(C12) | (i) | (1−PET)ET>x∗1 | M2 | N21 |
(ii) | N22 | |||
(iii) | N23∪N24 |
Cases | (1−PET)ET | A1 and A2 | P(y+i) | |
(C)(C11) | (i) | x∗3≤(1−PET)ET≤x∗1 | A2≥0, △ | y+i∈Y011 |
(ii) | y+i∈Y012 | |||
(iii) | y+i∈Y013∪Y014 | |||
(C)(C12) | (i) | (1−PET)ET>x∗1 | A1<0, A2>0 | y+i∈Y021 |
(ii) | y+i∈Y022 | |||
(iii) | y+i∈Y023∪Y024 | |||
△ means the sign of A1 is not necessary for case (C)(C11). |