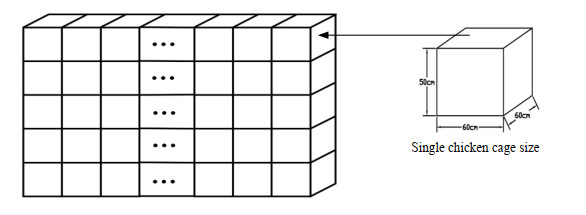
With the continuous enrichment of scientific and technological means, the production of most chicken farms has been able to achieve automation, but for the dead and sick chickens in the farm, there is no automatic monitoring step, only through continuous manual inspection and discovery. In the face of this problem, there are many solutions to identify dead and sick chickens through sound and image, but they can not achieve the ideal effect. In this paper, a sensor detection method based on artificial intelligence is proposed. This method 1) The maximum displacement of chicken activity is measured by fastening a foot ring on each chicken, and the three-dimensional total variance is designed and calculated to represent the chicken activity intensity. 2) The detection terminal collects the sensing data of foot ring through ZigBee network. 3) The state of chicken (dead chicken and sick chicken) can be identified by machine learning algorithm. This method of artificial intelligence combined with sensor network not only has high recognition rate, but also can reduce the operation cost. The practical results show that the accuracy of the system to identify dead and sick chickens is 95.6%, and the cost of the system running for 4 years can be reduced by 25% compared with manual operation.
Citation: Yiqin Bao, Hongbing Lu, Qiang Zhao, Zhongxue Yang, Wenbin Xu. Detection system of dead and sick chickens in large scale farms based on artificial intelligence[J]. Mathematical Biosciences and Engineering, 2021, 18(5): 6117-6135. doi: 10.3934/mbe.2021306
[1] | Giuseppe Ciaburro . Machine fault detection methods based on machine learning algorithms: A review. Mathematical Biosciences and Engineering, 2022, 19(11): 11453-11490. doi: 10.3934/mbe.2022534 |
[2] | Anastasia-Maria Leventi-Peetz, Kai Weber . Probabilistic machine learning for breast cancer classification. Mathematical Biosciences and Engineering, 2023, 20(1): 624-655. doi: 10.3934/mbe.2023029 |
[3] | Ivan Izonin, Nataliya Shakhovska . Special issue: informatics & data-driven medicine-2021. Mathematical Biosciences and Engineering, 2022, 19(10): 9769-9772. doi: 10.3934/mbe.2022454 |
[4] | Wang Cai, Jianzhuang Wang, Longchao Cao, Gaoyang Mi, Leshi Shu, Qi Zhou, Ping Jiang . Predicting the weld width from high-speed successive images of the weld zone using different machine learning algorithms during laser welding. Mathematical Biosciences and Engineering, 2019, 16(5): 5595-5612. doi: 10.3934/mbe.2019278 |
[5] | Naila Naz, Muazzam A Khan, Suliman A. Alsuhibany, Muhammad Diyan, Zhiyuan Tan, Muhammad Almas Khan, Jawad Ahmad . Ensemble learning-based IDS for sensors telemetry data in IoT networks. Mathematical Biosciences and Engineering, 2022, 19(10): 10550-10580. doi: 10.3934/mbe.2022493 |
[6] | Di Zhang, Bing Fan, Liu Lv, Da Li, Huijun Yang, Ping Jiang, Fangmei Jin . Research hotspots and trends of artificial intelligence in rheumatoid arthritis: A bibliometric and visualized study. Mathematical Biosciences and Engineering, 2023, 20(12): 20405-20421. doi: 10.3934/mbe.2023902 |
[7] | Yuanyao Lu, Kexin Li . Research on lip recognition algorithm based on MobileNet + attention-GRU. Mathematical Biosciences and Engineering, 2022, 19(12): 13526-13540. doi: 10.3934/mbe.2022631 |
[8] | Swati Shinde, Madhura Kalbhor, Pankaj Wajire . DeepCyto: a hybrid framework for cervical cancer classification by using deep feature fusion of cytology images. Mathematical Biosciences and Engineering, 2022, 19(7): 6415-6434. doi: 10.3934/mbe.2022301 |
[9] | Rachael C. Adams, Behnam Rashidieh . Can computers conceive the complexity of cancer to cure it? Using artificial intelligence technology in cancer modelling and drug discovery. Mathematical Biosciences and Engineering, 2020, 17(6): 6515-6530. doi: 10.3934/mbe.2020340 |
[10] | Vinh Huy Chau . Powerlifting score prediction using a machine learning method. Mathematical Biosciences and Engineering, 2021, 18(2): 1040-1050. doi: 10.3934/mbe.2021056 |
With the continuous enrichment of scientific and technological means, the production of most chicken farms has been able to achieve automation, but for the dead and sick chickens in the farm, there is no automatic monitoring step, only through continuous manual inspection and discovery. In the face of this problem, there are many solutions to identify dead and sick chickens through sound and image, but they can not achieve the ideal effect. In this paper, a sensor detection method based on artificial intelligence is proposed. This method 1) The maximum displacement of chicken activity is measured by fastening a foot ring on each chicken, and the three-dimensional total variance is designed and calculated to represent the chicken activity intensity. 2) The detection terminal collects the sensing data of foot ring through ZigBee network. 3) The state of chicken (dead chicken and sick chicken) can be identified by machine learning algorithm. This method of artificial intelligence combined with sensor network not only has high recognition rate, but also can reduce the operation cost. The practical results show that the accuracy of the system to identify dead and sick chickens is 95.6%, and the cost of the system running for 4 years can be reduced by 25% compared with manual operation.
Nowadays, in modern chicken farms, the supply of feed and water, the collection of eggs and chicken manure, the control of temperature and wind speed, etc. have been automated. But for the detection of dead chickens and sick chickens, it still uses manual regular inspection and inspection. If dead chickens and sick chickens are not checked and cleared in time, it will cause great harm and loss. On the one hand, according to medical knowledge, two hours after the normal death of the chicken, the muscle and blood began to breed bacteria, five hours later, the number of bacteria multiplied, which will cause great harm. If the abnormal death caused by infectious diseases, it may lead to cross infection between chickens, if not cleared in time, the chicken farm will suffer huge economic losses. On the other hand, the chicken epidemic, especially the avian influenza epidemic, is still an important factor to inhibit the development of China's poultry industry. There is no effective treatment and prevention method for the avian influenza epidemic. Once the outbreak of the epidemic, only through a large number of slaughtering to prevent the spread of the epidemic, causing huge losses to farmers. It is an important way to reduce economic losses to find out the epidemic situation in time and take countermeasures as soon as possible [1,2,3]. The manual inspection of dead and sick chickens is a trivial and meticulous work, and it will have a serious impact on the health of workers if they travel in the poor environment for a long time. Therefore, the development of an automatic detection system for dead and sick chickens is of great significance to reduce the labor intensity of workers, improve work efficiency and improve the working environment of workers [4,5].
With the development of information processing technology, artificial intelligence technology and Internet of things technology, it has a profound impact on the traditional way of livestock breeding in the past. Wang et al. [6] reviewed the monitoring technology of poultry behavior and physiological information, Wang et al. [7] studied the method of identifying sick chicken by broiler feces, and Liu et al. [8] designed the method of detecting sick chicken by abnormal sound of broiler, Aydin [9] carried out computer vision research on lameness of broilers, Cedric et al. [10] studied the early detection and prediction system of sick birds through machine vision system, Aydin [11] used three-dimensional vision camera system to automatically evaluate the inactivity level of broilers.
The above research is aimed at the artificial intelligence method of chicken feces, sounds, images, etc., but because of large-scale chicken farms, chickens live in a narrow space in chicken cages, so it is not easy to obtain the characteristics of chicken feces, sounds, images and other characteristics. There are many questions about information noise, which leads to low recognition rate, so it can only stay in the theoretical research of ideal environment, It is difficult to be practical. Not only that, but also the implementation cost.
In view of the shortcomings of artificial detection of dead and sick chickens and the difficulty of identifying dead and sick chickens, this paper proposes a detection method of dead and sick chickens based on artificial intelligence. This method measures the maximum displacement and three-dimensional total variance of the chicken by fastening a foot ring on each chicken, and identifies the state of the chicken by machine learning method, so as to identify the dead chicken and sick chicken. Through the wearable multi-sensor system to detect the shape of animals, there are also related studies in the intelligent farm [12,13,14]. In this paper, the foot ring of chicken adopts 6-axis MPU6050 sensor to calculate the three-dimensional displacement of chicken with high precision (the precision is several centimeters). The detection terminal displays the activity state of each chicken through wireless ad hoc network with foot ring. The detection terminal is connected to the system through network, so as to realize the automatic detection of dead and sick chicken.
The contributions and innovations of this paper are summarized as follows:
● The system architecture of dead and sick chicken characteristic analysis and detection.
● The calculation method of three-dimensional total variance of chicken was put forward.
● The realization principle of foot ring and detection terminal.
● Research on machine learning recognition method of chicken activity state.
The rest of the paper is organized as follows. In the second section, the analysis and system architecture of the detection system for dead and sick chickens are carried out. In the third section, the calculation method of three-dimensional total variance is proposed, and the implementation principle of foot ring and detection terminal is introduced. In the fourth section, the machine learning recognition method of chicken activity state is proposed. The fifth section is the comparative analysis of experiment and results, and the sixth section is the conclusion.
The characteristics of dead chicken should be analyzed first. The previous methods of artificial experience analysis include:
● Feel your temperature. The thigh roots of sick chickens are hot and cold, and the crowns are hot; The thigh root of healthy chicken is cold and hot, the comb is not hot.
● Check the skin color. The muscle of sick chicken is thin, stiff and inelastic, and the skin color is dark red; The skin color of healthy chicken is reddish, and the muscle is plump and elastic.
● Look at the anus. In addition, the anus is dark red, and its feces are mostly white or yellow green. The villi near the anus of healthy chickens are clean and dry, and the anus is moist and reddish, and its feces are semi-solid.
● Look at your eyes. The eyes of diseased chickens are dull, weeping, or there are cheese like secretions around the eyes, and the eyes of individual chickens will become longer; Healthy chicken has bright eyes and flexible eyeballs.
● Listen to the sound. At 3–5 o'clock in the morning, when you listen to the chicken's voice quietly, the sick chicken will make a slight "hiss" call, or even no sound, and there will also be a similar frog's purr, scream, or strange cry; Healthy chickens make loud, clear calls.
● We should pay attention to behavior. Sick chicken spirit is dispirited, the pace is unsteady, the appetite is weak, also is not willing to move to eat the material, if the chicken died, will not move; Healthy chicken is lively and active, with strong legs and feet, and willing to move to eat.
In the process of raising chickens, we can find the dead chickens as early as possible by analyzing the characteristics of chicken flock from the above six aspects. However, for large-scale breeding of chickens, the number of chickens is very large and dense. There are hundreds of thousands or even millions of chickens. If they pass the artificial detection, it will cost huge human and financial resources. Suppose that in the narrow space of chicken cage, it is not easy to obtain the accurate information of chicken's sound, image, temperature and other characteristics through the detection method from the above 1)–5), and there is also information noise; It is a good choice to judge whether the chicken is sick or dead by the detection method of 6) and the behavior of the chicken obtained by the sensor. There have been relevant researches, which use acceleration sensors to judge people's behavior. Jia et al. [15] have studied people recognition based on walking acceleration information segmentation, and Zhang et al. [16] have studied gait recognition based on acceleration. Therefore, starting from this idea, the architecture of the whole system is designed.
In modern chicken farms, chickens are very concentrated, for example, a breeding room for 40,000 chickens, a chicken cage with a size of about 60 cm * 60 cm * 50 cm, and 8 chickens need to be cultured, as shown in Figure 1. It is still a little difficult to find dead sick chickens automatically in such a small space. Through the analysis of the characteristics in Section 2.1, it is a good choice to adopt the acceleration measurement technique.
As shown in Figure 2, it is a chicken cage detection unit. The chicken cage detection unit is mainly composed of eight chicken foot rings and a detection terminal. Each chicken foot is covered with a foot ring, eight LEDs of the detection terminal indicate the activity state of the chicken.
The status of the LED light indicates the activity status of the chicken, as shown in Table 1. The management personnel can not only see the status of the chicken at the scene and judge the dead chicken through the LED light in front of the chicken cage, but also find the dead chicken through the system software or app remotely.
LED status | Chicken status |
Red light | Dead chicken |
Yellow light | Sick chicken |
Green light | Active weak chicken |
The light is not bright | Active chicken |
According to the above requirements, the dead chicken detection system is designed, which can realize on-site detection and remote detection. The framework of the system is shown in Figure 3. The hardware part of the system is mainly composed of several chicken cage detection units, which are composed of eight foot rings and one detection terminal; The system software is mainly composed of communication front-end processor, data server, web monitoring client and app.
The measurement method adopted by the system is to measure the displacement through the acceleration sensor, and there are many related researches. Bi et al. [17] measured the body trajectory through the acceleration, and Li et al. [18] measured the seismic scene stratification through the built-in acceleration of the mobile phone. In the design of the system, we should first know the principle of acceleration measuring displacement, and then verify the feasibility of the method through simulation experiments.
When the acceleration signal is integrated to calculate the displacement, the acceleration data obtained is a group of discrete values [19]. When n > 1, in the discrete domain, the velocity and displacement formula of n point are calculated by acceleration, as shown in Eqs (1) and (2).
v[n]=n∑k=1a[k]+a[k−1]2Δt=v[0]+12(a[0]+a[n])⋅Δt+(a[1]+a[2]+⋯a[n−1])⋅Δt | (1) |
s[n]=n∑k=1v[k]+v[k−1]2Δt=12(v[0]+v[n])·Δt+(v[1]+v[2]+⋯v[n−1])·Δt | (2) |
In Eqs (1) and (2), a [n] is the acceleration reading value at n point, v [n] and s [n] are the velocity and displacement values at the corresponding time. According to Eqs (1) and (2), the displacement of the target in one direction at n poin can be obtained, and the calculation formula is Eq (3).
s[n]=n⋅v[0]⋅Δt+{(n−1)⋅a[1]+(n−2)⋅a[2]+⋯a[n−1]}⋅Δt2+14(a[0]+a[n])⋅Δt2 | (3) |
● By sampling the acceleration value, the displacement from T1 to T2 is calculated. If the acquisition interval is set as △ t = 100 ms and the acceleration sampling time is 100 ms, the total sampling times from T1 to T2 is n = (t2-t1) / △ T, the real-time motion displacement is s [n], and the calculation formula is Eq (4).
s[n]={(n−1)⋅a[1]+(n−2)⋅a[2]+⋯a[n−1]}⋅Δt2+14(a[0]+a[n])⋅Δt2 | (4) |
● There are M time periods (t2-t1) in t time, and the calculation formula is Eq (5).
M=T/(t2-t1) | (5) |
● Calculate the average displacement in t time, and the calculation formula is Eq (6).
ˉs=1MM∑i=1s[i] | (6) |
As the variance is the square of the data, it is too different from the detection value itself, so it is difficult for people to measure it intuitively. Therefore, we often use the root of variance to convert it back. This is what we call the standard deviation. The variance describes the degree of deviation and fluctuation. and the calculation formula is Eq (7).
σ=1M√M∑i=1(s[i]−ˉs)2 | (7) |
The variance of displacement in three directions, and the calculation formula is Eqs (8)–(10).
σx=1M√M∑i=1(sx[i]−ˉsx)2 | (8) |
σy=1M√M∑i=1(sy[i]−ˉsy)2 | (9) |
σz=1M√M∑i=1(sz[i]−ˉsz)2 | (10) |
The three-dimensional total variance is shown in Eq (11):
σtotal=13√(σx2+σy2+σz2) | (11) |
Almost all mobile phones contain three-axis acceleration. In order to verify the feasibility of the algorithm design, we first write a mobile phone app for simulation test verification. Finally, the design and implementation of the foot ring are completed.
Android operating system is selected for the test mobile phone, which is developed by Android studio tool. The three-axis acceleration is tested by using sensormanager class. In the static state, the three-axis acceleration data curve can be tested in real time. Due to noise interference, the curve will fluctuate [20]. The data curve is shown in Figure 4.
Because the z-axis is vertical, the z-axis acceleration is about 9.8 m/s2, and the x-axis and y-axis acceleration is about 0.0m/s2. Therefore, the data curve of the adjusted three-axis acceleration is shown in Figure 5.
In the horizontal direction, when the mobile phone moves slightly, even a few centimeters, the x-axis acceleration or y-axis acceleration will fluctuate. For example, the x-axis acceleration fluctuation curve in the three-axis acceleration is shown in Figure 6.
According to the principle of Section 3, the main steps are as follows:
● Set the acceleration sampling frequency. Set △T = 100 ms and sample the acceleration once. Because of the different sensitivity, the first step is to correct. After correction, the three-axis accelerations Gx, Gy and Gz are almost 0.
● Set the positioning time t to calculate the displacement s. Set T = 1 minute, calculate the displacement once in 60 seconds, n = 60 / 0.1 = 600 times, calculate the minute displacement through Eq (4), which are Sx, Sy, Sz respectively. The total displacement S = Sx + Sy + Sz is calculated.
● The cumulative m-minute triaxial displacement variance was calculated. The mean displacement in M minutes is calculated by Eq (6), and then the variance Va, Vb and Vc of triaxial displacement are calculated by Eqs (8)–(10).
● The cumulative M minute displacement variance was calculated. The total variance Vtotal is calculated by Eq (11).
● The maximum displacement Smax of cumulative m minutes is calculated.
Total variance Vtotal and maximum displacement Smax are used as the input of machine learning classification algorithm.
The interface of a group of mobile app real-time data is shown in Figure 7. The process data included in the interface are: three axis real-time acceleration, three axis real-time displacement, cumulative minute, three axis minute displacement. The final calculated data are: maximum minute displacement, total three-dimensional displacement and total variance.
Through the simulation test of the acceleration of the mobile phone, the results are as follows: 1) when the mobile phone is not moving, the maximum total displacement and three-dimensional total variance are almost 0; 2) no matter the mobile phone moves up and down, left and right for 1cm, there will be displacement in three directions; 3) calculate the displacement per minute. By calculating the maximum minute displacement, we can know the maximum activity of the mobile phone, 4) By calculating the variance of three-dimensional displacement per minute, the total variance of three-dimensional displacement can be calculated, so that the deviation and rhythm of mobile phone movement can be known.
It is not difficult to see from the simulation test results of mobile phone that it is feasible to apply this calculation method to the foot ring of chicken. Through the mobile phone test, it provides a feasible technical solution for the implementation of the system.
The hardware part of the system is mainly composed of several chicken cage detection units. The chicken cage detection unit is composed of eight foot rings and one detection terminal. The eight foot rings and one detection terminal automatically form a ZigBee wireless network. The detection terminal collects sensor data and displays the activity status of the chicken through the indicator light.
The foot ring is mainly composed of sensor unit, battery unit and microprocessor unit. Its composition is shown in Figure 8.
● The sensor unit adopts the first sensor MPU-6050 which integrates six axis motion processing components in the world. Compared with the previous multi-component scheme, MPU-6050 avoids the difference between gyroscope and accelerometer, and reduces a large number of packaging space, which makes it compact and efficient. When the chicken ring moves, MPU-6050 produces triaxial acceleration and calculates it by microprocessor, and obtains the maximum displacement and three-dimensional variance of chicken. The detection terminal indicates the activity intensity of chicken after receiving these data.
● The main function of the battery unit is to supply power to the whole equipment and ensure that the equipment can run. In the choice of battery, we need to consider two points: 1) the battery volume is relatively small, to ensure the whole volume of the chicken ring in a small volume, let the chicken wear the chicken ring is more comfortable, will not let the chicken to deliberately break the chicken ring phenomenon. 2) Relative to the maximum power under the volume, the volume ensures the maximum power under the limited basis, which can ensure that the chicken ring can run for a long time without frequent replacement of equipment, so as to save the economic cost and time cost.
● The microprocessor unit adopts the ZigBee chip CC2630 of Texas Instruments (TI). Compared with the same ZigBee bit processor CC2530, the advantages of CC2630 are: more powerful MCU processing power and lower power consumption. CC2630 contains a 32-bit arm cotex-m3 core. Compared with the 8051 core of CC2530, the performance of CC2630 is more powerful and the cost is also lower. At the same time, CC2630 has a very unique ultra-low power sensor controller based on cotex-m0. CC2630 has a rich set of peripheral functions. In addition, the function set is designed to connect with CC2630's unique ultra-low power consumption sensor controller. This unique connection mode can ensure the independent collection of analog and digital data without waking up the main control unit of MCU. Therefore, the data collection is realized through battery power supply in zibgbee network, CC2630 is the best choice.
● The actual development pictures of the foot ring is shown in Figure 9, the physical simulation picture is shown in Figure 9(a), and the actual picture of the foot ring is shown in Figure 9(b).
The detection terminal is mainly composed of storage unit, battery unit, indicator unit, wireless transmission unit and microprocessor unit. The principle block diagram of the detection terminal is shown in Figure 10.
● The storage unit is mainly composed of flash chip. The main function of the storage unit is to store the collected data of the foot ring. Wireless transmission of data is very limited consumption, so the collected data can not be uploaded to the front-end computer in the form of real-time data. It needs to be stored and transmitted to the master station at a time.
● The battery cell is used for power supply. Compared with the chicken ring, the battery cell has no volume requirements, and its capacity can be larger. It does not need high size and capacity requirements like the limb ring.
● The indicator light unit is composed of eight LED lights, corresponding to the status of eight chickens in the chicken cage. In this way, the chicken farm managers can more intuitively judge the sick chickens in the chicken cage, so as to improve the efficiency.
● The wireless transmission unit is mainly composed of WiFi module and ZigBee module. The WiFi module is mainly used to upload the collected sensing data to the front-end computer, which is convenient for the master station to analyze. The WiFi module uses the ESP32 of Shanghai Lexin. After the ESP32 is powered on, it is set as the terminal and automatically connects to the wireless router to realize data transmission. ZigBee wireless ad hoc network is a function of microprocessor CC2530.
● Microprocessor unit, CC2530 of Ti. Although CC2630 is also ZigBee network microprocessor, Ti defines CC2630 as the ZigBee network terminal with ultra low power consumption. CC2630 has the function of adding ZigBee network, but not the function of setting up ZigBee network. Therefore, the microprocessor adopts CC2530 chip. CC2530 establishes ZigBee network through panid and channel, and the pin ring adds to the corresponding ZigBee network according to these two parameters to realize the interaction of ZigBee messages, the communication protocol adopts industry standard MODBUS; After the artificial intelligence learning of the collected data of the foot ring, the state of the chicken is analyzed, and the corresponding LED light is illuminated to display the status of the chicken intuitively; Meanwhile, the status information of chicken will be sent to the main station of the front-end computer through WiFi module and via WiFi network, and the alarm information will be sent to the administrator for troubleshooting.
Artificial intelligence learning is carried out by supervised learning classification method. The training and learning steps are shown in Figure 11. Firstly, the acceleration sensor is used to collect the data of chicken foot ring; Then, the displacement is calculated to obtain the maximum displacement and the three-dimensional total variance as (x1, x2); The following is divided into two parts: a) through machine learning classification algorithm training data, get the best training model. b) The activity behavior of chicken was identified by training model.
Through the data collected from the gateway by the foot ring sensor, the machine learning is carried out to judge the activity state of the chicken. Since the activity time of chicken is from 5: 00 am to 8: 00 pm, the data is collected from 5: 00 am for 15 hours, and the maximum displacement Smax and total variance vtotal are finally collected.
● Sample set definition:
Sample data: X= (x1, x2), x1 is the maximum displacement Smax, x2 is the total variance Vtotal.
Classification Y: 0-dead chicken, 1-sick chicken, 2-active weak chicken, 3-active strong chicken.
● Examples of typical sample sets:
A typical sample set of four classifications is shown in Table 2.
x1 Smax (m) | x2 Vtotal (m) | Y Classification |
0.025 | 0.012 | 0 |
4.458 | 12.231 | 1 |
21.286 | 13.378 | 2 |
98.523 | 14.236 | 3 |
● Training data:
In the actual test, 2000 sample data are collected for training test.
Through five kinds of classification algorithm SVM, KNN, DT, NB, BP for abnormal behavior recognition effect.
● SVM is a new learning method proposed by Cortes and Vapnik according to the statistical learning theory. Its biggest feature is that according to the structural risk minimization criterion, it constructs the optimal classification hyperplane with the maximum classification interval to improve the generalization ability of learning, and better solves the problems of nonlinearity, high dimension, local minima and so on [21,22].
● KNN is a case-based classification method. This method is to find out the K training samples closest to the unknown sample x, and gather x into the category that most of the K samples belong to [23].
● DT is a case-based inductive learning algorithm, which aims to infer the classification rules represented by DT from a group of unordered and irregular cases. The purpose of constructing DT is to find out the relationship between eigenvalues and categories, and use it to predict the categories of unknown samples [24,25].
● NB is a simple probability Classification Based on Bayesian theorem of independent hypothesis. Its principle is to use Bayesian formula to calculate the posterior probability through the prior probability of an object, that is, the probability that the object belongs to a certain class, and select the class with the maximum posterior probability as the class that the object belongs to [26,27,28].
● BP neural network is a kind of multilayer feedforward network trained by error back propagation (referred to as error back propagation). Its algorithm is called BP algorithm. Its basic idea is gradient descent method. Gradient search technology is used to minimize the mean square error between the actual output value and the expected output value of the network, Its outstanding advantages are strong nonlinear mapping ability and flexible network structure [29,30,31,32,33].
In the whole system, the design of power consumption and communication performance of the terminal is very important. It needs to pass the necessary tests to determine whether it meets the requirements of the design and site. The test of terminal equipment is divided into two parts:
● Power consumption test: since the feet are powered by batteries, it is necessary to ensure that the batteries will not be changed in a chicken breeding cycle (400 days), so there is a design requirement: the power consumption should be less than 400 mAh. CC2630 chip is used in the design, which has very low power consumption, is usually in sleep state, and uploads to the detection terminal once every 10 minutes. The measured power consumption data of the foot ring 0.9 mAh per day, then 360 mAh per 400 days. Only one detection terminal is placed in a chicken cage unit, so the battery is easy to replace and can be replaced once a month. Therefore, there is a design requirement: the power consumption should be less than 2000 mAh. CC2530 chip is used in the design, which also has very low power consumption and can collect sensor data, the power consumption in one day is 62.5 mAh, then 1875 mAh in 30 days. The specific data are shown in Table 3.
Power consumption | Packet loss rate | Communication distance | ||||
Design requirement | Test result | Design requirement | Test result | Design requirement | Test result | |
Foot ring | < 400 mAh | 360 mAh | 99% | 99.9% | > 1 m | 3.4 m |
Detection terminal | < 2000 mAh | 1875 mAh |
● Communication test: the test data of communication packet loss rate and communication distance are shown in Table 3. Since eight foot rings and one detection terminal communicate through ZigBee protocol ad-hoc network, although the communication channel is the same in design, the PanID is different to distinguish each ad-hoc network. The foot ring sends data regularly, and the detection terminal is responsible for receiving data. Therefore, the communication packet loss rate can be measured from the detection terminal. The design packet loss rate is required to be 99%, and the tset result is 99.9%. Because the size of chicken cage is about 60 cm * 60 cm * 50 cm, the design communication distance is required to be more than 1 m, while the measured distance of a Zigbee network is still 3.4 m in the case of very small antenna.
Using the sample data of 40,000 chickens to test, using five machine learning methods to compare, as shown in Table 4. The results are as follows:
Method | Dead chicken | Sick chicken | Active weak chicken | Active chicken |
SVM | 100% | 92.7% | 92.8% | 99.8% |
KNN | 100% | 91.3% | 90.9% | 99.5% |
DT | 100% | 90.5% | 89.7% | 99.2% |
NB | 100% | 90.2% | 89.4% | 99.1% |
BP | 100% | 95.6% | 95.2% | 99.9% |
● Because the dead chicken is inactive, the maximum displacement Smax and three-dimensional total variance vtotal are almost 0, the recognition accuracy of the five kinds of chicken is 100%, and the recognition accuracy of the active strong chicken is almost 100%. The key point is that it is difficult to distinguish the sick chicken and the active weak chicken. Because some good chickens are weak in activity and behave like sick chickens, it is sometimes difficult to distinguish them. However, due to the weak activity of chicken to eat, the maximum displacement will be large, so it can also be distinguished, but the recognition accuracy will be low.
● Through the operation of five classification algorithms, BP algorithm has the highest recognition rate, so BP is selected as the final classification algorithm.
● If the sick chicken and the weak chicken are classified into one category, then the recognition rate is higher, but in the practical application, more than once, but the detection of the sick chicken will not be missed.
Through field research, suppose a chicken shed with 40,000 chickens needs 4 workers for artificial breeding and 2 workers for systematic breeding. The salary cost of a worker is 100,000 yuan a year, and the material cost of a chicken foot ring is 10 yuan (about 10 yuan in fact). Then, compare and analyze the total cost of labor and system, and the comparison results are shown in Table 5. It can be seen from the results that the cost of running the system for 2 years is equivalent to that of labor, and the cost can be saved after 2 years.
Method | Number of years | Number of people | Cost of persons (104 yuan) | Material cost (104 yuan) | Total cost (104 yuan) |
Manual | 1 | 4 | 40 | 0 | 40 |
2 | 4 | 40 | 0 | 80 | |
3 | 4 | 40 | 0 | 120 | |
4 | 4 | 40 | 0 | 160 | |
System | 1 | 2 | 20 | 40 | 60 |
2 | 2 | 20 | 40 | 80 | |
3 | 2 | 20 | 40 | 100 | |
4 | 2 | 20 | 40 | 120 |
From the results in Table 5, it can be seen that the total cost of running the system will continue to decrease over time. The comparison of cost histograms for four years is shown in Figure 12.
According to the results in Table 5, we can also analyze and calculate the total cost trend of 8-year operation, and compare it with the labor operation cost. The cost trend curve is shown in Figure 13.
Through the comparison curve of cost trend in Figure 12, we can see that: 1) the cost can be recovered after 2 years of operation, 2) the cost can be reduced by 25% after 4 years of operation, 3) the economic benefit of one million will be produced after 8 years of operation, and 4) the greater economic benefit will be produced if a larger scale chicken farm is operated. Therefore, compared with manual operation cost, the system not only improves the recognition accuracy, but also creates higher economic value.
In view of the shortcomings of artificial detection of dead and sick chickens, this paper proposes a detection method of dead and sick chickens based on artificial intelligence. This method measures the three-dimensional displacement of the chicken by fastening a foot ring on each chicken, and designs and calculates the three-dimensional total variance to express the activity intensity of the chicken. Finally, the machine learning classification method is used to identify the status of the chicken, so as to realize the correct judgment of dead chicken and sick chicken. The foot ring and detection terminal are powered by ZigBee ad hoc network and low-power battery. The maximum displacement and three-dimensional total variance are used to represent the activity state of chicken. The experiment shows that the recognition accuracy is 95.6%, which can achieve practical effect, reduce manual labor and improve the work efficiency. However, in the implementation of the whole system, the workload is a bit heavy, there is still room for further research in the aspect of big data mining and analysis after sensor acquisition.
The follow-up work will do further research on big data mining and analysis. In addition, on the cost of installation and implementation, we need to spend more time on research, more efforts should be made to prepare for the large-scale promotion of this technology. If the system can be successfully promoted, it will produce greater economic and practical value for human large-scale breeding.
This study was supported by the Natural Science Foundation Project of China (61976118), the Natural Science Foundation Project of Jiangsu Province (BK20180142), and the key topics of the "13th five-year plan" for Education Science in Jiangsu Province (B-b /2020/01/18).
All authors declare no conflicts of interest in this paper.
[1] |
E. F. Briefer, Vocal expression of emotions in mammals: mechanisms of production and evidence, J. Zool., 288 (2012), 1-20. doi: 10.1111/j.1469-7998.2012.00920.x
![]() |
[2] |
V. S. Roneel, J. M. Tom, An overview of applications and advancements in automatic sound recognition, Neurocomputing, 200 (2016), 22-34. doi: 10.1016/j.neucom.2016.03.020
![]() |
[3] |
J. D. Huang, W. Q. Wang, T. M. Zhang, Method for detecting avian influenza disease of chickens based on sound analysis, Biosyst. Eng., 180 (2019), 16-24. doi: 10.1016/j.biosystemseng.2019.01.015
![]() |
[4] |
X. L. Zhuang, M. N. Bi, J. L. Guo, S. Y. Wu, T. M. Zhang, Development of an early warning algorithm to detect sick broilers, Comput. Electron. Agr., 144(2018), 102-113. doi: 10.1016/j.compag.2017.11.032
![]() |
[5] |
X. L. Zhuang, T. M. Zhang, Detection of sick broilers by digital image processing and deep learning, Biosyst. Eng., 179 (2019), 106-116. doi: 10.1016/j.biosystemseng.2019.01.003
![]() |
[6] | K. Y. Wang, X. Y. Zhao, Y. He, Review on noninvasive monitoring technology of poultry behavior and physiological information, T. Chin. Soc. Agr. Eng., 33 (2017), 197-209. |
[7] | J. T. Wang, M. X. Shen, L. S. Liu, Y. Xu, C. D. Okinda, G. V. Diraco, Recognition and classification of broiler droppings based on deep convolutional neural network, J. Sens., 2019 (2019), 1-11. |
[8] | L. S. Liu, B. Li, R. Q. Zhao, W. Yao, M. X. Shen, J. Yang, et al., A novel method for broiler abnormal sound detection using WMFCC and HMM, J. Sens., 2020(2020), 1-7. |
[9] |
A. Aydin, Development of an early detection system for lameness of broilers using computer vision, Comput. Electron. Agr., 136 (2017), 140-146. doi: 10.1016/j.compag.2017.02.019
![]() |
[10] | C. Okinda, M. Z. Lu, L. S Liu, I. Nyalala, C. Muneri, J. T. Wang, et al., A machine vision system for early detection and prediction of sick birds: A broiler chicken model, Comput. Electron. Agr., 188 (2019), 229-242. |
[11] |
A. Aydin, Using 3D vision camera system to automatically assess the level of inactivity in broiler chickens, Comput. Electron. Agr., 135 (2017), 4-10. doi: 10.1016/j.compag.2017.01.024
![]() |
[12] | M. J. Zhang, H. H. Feng, H. L. Luo, Z. G. Li, X. S. Zhang, Comfort and health evaluation of live mutton sheep during the transportation based on wearable multi-sensor system, Comput. Electron. Agr., 176 (2020), 1-10. |
[13] | M. J. Zhang, X. P. Wang, H. H. Feng, Q. Y. Huang, X. Q. Xiao, X. S. Zhang, Wearable internet of things enabled precision livestock farming in smart farms: A review of technical solutions for precise perception, biocompatibility, and sustainability monitoring, J. Clean. Prod., 312 (2021), 1-13. |
[14] | B. Achour, M. Belkadi, R. Aoudjit, M. Laghrouche, Unsupervised automated monitoring of dairy cows' behavior based on inertial measurement unit attached to their back, Comput. Electron. Agr., 167 (2019), 1-12. |
[15] | Z. Jia, C. Li, C. Y. Wan, X. J. Chen, Pedestrian recognition based on pedestrian acceleration information segmentation, Comput. Eng. Appl., 55 (2019), 203-209. |
[16] | X. G. Zhang, H. Tang, C. J. Fu, Y. L. Shi, A gait recognition algorithm based on Hidden Markov model, Comput. Sci., 43 (2016), 285-289. |
[17] | C. G. Bi, L. M. Xu, A tracking mechanism for mobile agents based on multi-sensor data fusion, Comput. Sci., 42 (2015), 544-549. |
[18] | X. G. Li, F. L. Bu, X. Xu, Hierarchical recognition method of seismic scene based on mobile accelerometer, Comput. Eng. Appl., 53 (2017), 252-257. |
[19] | W. M. Niu, L. Q. Fang, Z. Y. Qi, D. Q. Guo, Displacement measurement system based on MEMS acceleration sensor, Instrum. Tech. Sens., 3 (2020), 62-66. |
[20] | G. H. Wang, J. Z. Liang, J. Chen, X. J. Zhu, Acceleration difference finite state machine step counting algorithm, Comput. Sci. Expl., 10 (2016), 1133-1142. |
[21] | T. Li, X. C. Liu, S. Zhang, K. Wang, Y. L. Yang, Parallel support vector machine training with hybrid programming model, J. Comput. Res. Dev., 52 (2015), 1098-1108. |
[22] |
Q. Zhang, H. G. Li, An improved least squares SVM with adaptive PSO for the prediction of coal spontaneous combustion, Math. Biosci. Eng., 16 (2019), 3169-3182. doi: 10.3934/mbe.2019157
![]() |
[23] | Z. M. Bao, S. R. Gong, S. Zhong, R. Yan, X. H. Dai, Person re-identification algorithm based on bidirectional KNN ranking optimization, Comput. Sci., 46 (2019), 267-271. |
[24] | Y. M. Ji, Y. P. Zhang, X. B. Lang, D. C. Zhang, R. H. Wang, Parallel of decision tree classification algorithm for stream data, J. Comput. Res. Dev., 54 (2017), 1945-1957. |
[25] |
W. D. Gao, Y. B. Xu, S. S. Li, Y. J. Fu, D. Y. Zheng, Y. J. She, Obstructive sleep apnea syndrome detection based on ballistocardiogram via machine learning approach, Math. Biosci. Eng., 16 (2019), 5672-5686. doi: 10.3934/mbe.2019282
![]() |
[26] | Q. S. Wang, R. Y. Wai, Bayesian Chinese spam filtering method based on phrases, Comput. Sci., 43 (2016), 256-259. |
[27] |
Y. C. He, Z. Liu, Z. Li, J. R. Wu, J. Y. Fu, Modal identification of a high-rise building subjected to a landfall typhoon via both deterministic and Bayesian methods, Math. Biosci. Eng., 16(2019), 7155-7176. doi: 10.3934/mbe.2019359
![]() |
[28] |
Y. H. Zhuang, K. Wade, L. M. Saba, K. Kechris, Development of a tissue augmented Bayesian model for expression quantitative trait loci analysis, Math. Biosci. Eng., 17 (2020), 122-143. doi: 10.3934/mbe.2020050
![]() |
[29] | L. Q. Du, L. Liu, Compensation method of grinder force error based on BP neural network, Mach. Tools Hyd., 49 (2021), 1-5. |
[30] | Q. S. Zhang, K. H. Yang, L. X. Li, Study on inversion method of left ventricular myocardial tissue parameters based on BP neural network, Biomed. Eng. Res., 40 (2021), 8-14. |
[31] | H. W. Shen, Vibration fault detection of stamping machine based on BP neural network, Ind. Heat., 49 (2020), 29-33. |
[32] |
S. K. Tian, N. Dai, L. L. Li, W. W. Li, Y. H. Sun, X. S. Cheng, Three-dimensional mandibular motion trajectory-tracking system based on BP neural network, Math. Biosci. Eng., 17 (2020), 5709-5726. doi: 10.3934/mbe.2020307
![]() |
[33] |
H. J. Xu, S. Li, S. D. Fan, M. Chen, A new inconsistent context fusion algorithm based on BP neural network and modified DST, Math. Biosci. Eng., 18 (2021), 968-982. doi: 10.3934/mbe.2021051
![]() |
1. | Hongyun Hao, Peng Fang, Enze Duan, Zhichen Yang, Liangju Wang, Hongying Wang, A Dead Broiler Inspection System for Large-Scale Breeding Farms Based on Deep Learning, 2022, 12, 2077-0472, 1176, 10.3390/agriculture12081176 | |
2. | S. Suthagar, G. Mageshkumar, M. Ayyadurai, C. Snegha, M. Sureka, S. Velmurugan, 2023, Chapter 64, 978-981-19-7401-4, 903, 10.1007/978-981-19-7402-1_64 | |
3. | Xing Qin, Chenxiao Lai, Zejun Pan, Mingzhong Pan, Yun Xiang, Yikun Wang, Recognition of Abnormal-Laying Hens Based on Fast Continuous Wavelet and Deep Learning Using Hyperspectral Images, 2023, 23, 1424-8220, 3645, 10.3390/s23073645 | |
4. | Weihong Ma, Xingmeng Wang, Simon X. Yang, Xianglong Xue, Mingyu Li, Rong Wang, Ligen Yu, Lepeng Song, Qifeng Li, Autonomous inspection robot for dead laying hens in caged layer house, 2024, 227, 01681699, 109595, 10.1016/j.compag.2024.109595 | |
5. | S Harshitha, K Likhitha, Katta R G Varshini, Sonali Jagadish Mahure, Anaparthi Yasaswi, 2024, Poultry Pathogen Detection: Using Deep Learning for Coccidiosis Identification, 979-8-3503-6717-1, 178, 10.1109/ICIPCN63822.2024.00037 | |
6. | Ramesh Bahadur Bist, Sachin Subedi, Xiao Yang, Lilong Chai, Automatic Detection of Cage-Free Dead Hens with Deep Learning Methods, 2023, 5, 2624-7402, 1020, 10.3390/agriengineering5020064 | |
7. | Ninad Deo, Aagam Bakliwal, Amit D. Joshi, Suraj T. Sawant, 2024, Non-Intrusive Detection of Poultry Diseases through Faecal Analysis using Deep Learning, 979-8-3503-8681-3, 1, 10.1109/ICITIIT61487.2024.10580821 | |
8. | Hassan M. Taleb, Khalid Mahrose, Amal A. Abdel-Halim, Hebatallah Kasem, Gomaa S. Ramadan, Ahmed M. Fouad, Asmaa F. Khafaga, Norhan E. Khalifa, Mahmoud Kamal, Heba M. Salem, Abdulmohsen H. Alqhtani, Ayman A. Swelum, Anna Arczewska-Włosek, Sylwester Świątkiewicz, Mohamed E. Abd El-Hack, Using artificial intelligence to improve poultry productivity – a review, 2024, 0, 2300-8733, 10.2478/aoas-2024-0039 | |
9. | Jikang Yang, Tiemin Zhang, Cheng Fang, Haikun Zheng, Chuang Ma, Zhenlong Wu, A detection method for dead caged hens based on improved YOLOv7, 2024, 226, 01681699, 109388, 10.1016/j.compag.2024.109388 | |
10. | Rahul Singh, Neha Sharma, Rahul Chauhan, Ankur Choudhary, Rupesh Gupta, 2023, Chicken Disease Classification from Faeces Samples Using a Fine-Tuned EfficientNet B5 Model, 979-8-3503-0884-6, 1, 10.1109/INCOFT60753.2023.10425511 | |
11. | Mukti Budiarto, Hindriyanto Dwi Purnomo, Budhi Kristianto, Sendy Zul Friandi, Ananda Alifia Putri, 2023, CCTV Camera With Intelligence Technology to Minimize Chicken Mortality Due to Hazardous Gas, 979-8-3503-0596-8, 1, 10.1109/CITSM60085.2023.10455231 | |
12. | Gyu-Sung Ham, Kanghan Oh, Dead Broiler Detection and Segmentation Using Transformer-Based Dual Stream Network, 2024, 14, 2077-0472, 2082, 10.3390/agriculture14112082 | |
13. | Murat Erhan Çimen, Comparison of Deep Learning and Yolov8 Models for Fox Detection Around the Henhouse, 2024, 2757-6787, 10.58769/joinssr.1498561 | |
14. | Ridip Khanal, Wenqin Wu, Joonwhoan Lee, Automated Dead Chicken Detection in Poultry Farms Using Knowledge Distillation and Vision Transformers, 2024, 15, 2076-3417, 136, 10.3390/app15010136 | |
15. | Hongyun Hao, Fanglei Zou, Enze Duan, Xijie Lei, Liangju Wang, Hongying Wang, Research on Broiler Mortality Identification Methods Based on Video and Broiler Historical Movement, 2025, 15, 2077-0472, 225, 10.3390/agriculture15030225 | |
16. | Helen K. Joy, N. Sandhyana, Manjunath R. Kounte, 2024, Advanced Poultry Disease Detection with Deep Convolutional Neural Networks, 979-8-3503-8747-6, 786, 10.1109/ICTACS62700.2024.10841189 | |
17. | Xiao Yang, Jinchang Zhang, Bidur Paneru, Jiakai Lin, Ramesh Bahadur Bist, Guoyu Lu, Lilong Chai, Precision Monitoring of Dead Chickens and Floor Eggs with a Robotic Machine Vision Method, 2025, 7, 2624-7402, 35, 10.3390/agriengineering7020035 |
LED status | Chicken status |
Red light | Dead chicken |
Yellow light | Sick chicken |
Green light | Active weak chicken |
The light is not bright | Active chicken |
x1 Smax (m) | x2 Vtotal (m) | Y Classification |
0.025 | 0.012 | 0 |
4.458 | 12.231 | 1 |
21.286 | 13.378 | 2 |
98.523 | 14.236 | 3 |
Power consumption | Packet loss rate | Communication distance | ||||
Design requirement | Test result | Design requirement | Test result | Design requirement | Test result | |
Foot ring | < 400 mAh | 360 mAh | 99% | 99.9% | > 1 m | 3.4 m |
Detection terminal | < 2000 mAh | 1875 mAh |
Method | Dead chicken | Sick chicken | Active weak chicken | Active chicken |
SVM | 100% | 92.7% | 92.8% | 99.8% |
KNN | 100% | 91.3% | 90.9% | 99.5% |
DT | 100% | 90.5% | 89.7% | 99.2% |
NB | 100% | 90.2% | 89.4% | 99.1% |
BP | 100% | 95.6% | 95.2% | 99.9% |
Method | Number of years | Number of people | Cost of persons (104 yuan) | Material cost (104 yuan) | Total cost (104 yuan) |
Manual | 1 | 4 | 40 | 0 | 40 |
2 | 4 | 40 | 0 | 80 | |
3 | 4 | 40 | 0 | 120 | |
4 | 4 | 40 | 0 | 160 | |
System | 1 | 2 | 20 | 40 | 60 |
2 | 2 | 20 | 40 | 80 | |
3 | 2 | 20 | 40 | 100 | |
4 | 2 | 20 | 40 | 120 |
LED status | Chicken status |
Red light | Dead chicken |
Yellow light | Sick chicken |
Green light | Active weak chicken |
The light is not bright | Active chicken |
x1 Smax (m) | x2 Vtotal (m) | Y Classification |
0.025 | 0.012 | 0 |
4.458 | 12.231 | 1 |
21.286 | 13.378 | 2 |
98.523 | 14.236 | 3 |
Power consumption | Packet loss rate | Communication distance | ||||
Design requirement | Test result | Design requirement | Test result | Design requirement | Test result | |
Foot ring | < 400 mAh | 360 mAh | 99% | 99.9% | > 1 m | 3.4 m |
Detection terminal | < 2000 mAh | 1875 mAh |
Method | Dead chicken | Sick chicken | Active weak chicken | Active chicken |
SVM | 100% | 92.7% | 92.8% | 99.8% |
KNN | 100% | 91.3% | 90.9% | 99.5% |
DT | 100% | 90.5% | 89.7% | 99.2% |
NB | 100% | 90.2% | 89.4% | 99.1% |
BP | 100% | 95.6% | 95.2% | 99.9% |
Method | Number of years | Number of people | Cost of persons (104 yuan) | Material cost (104 yuan) | Total cost (104 yuan) |
Manual | 1 | 4 | 40 | 0 | 40 |
2 | 4 | 40 | 0 | 80 | |
3 | 4 | 40 | 0 | 120 | |
4 | 4 | 40 | 0 | 160 | |
System | 1 | 2 | 20 | 40 | 60 |
2 | 2 | 20 | 40 | 80 | |
3 | 2 | 20 | 40 | 100 | |
4 | 2 | 20 | 40 | 120 |