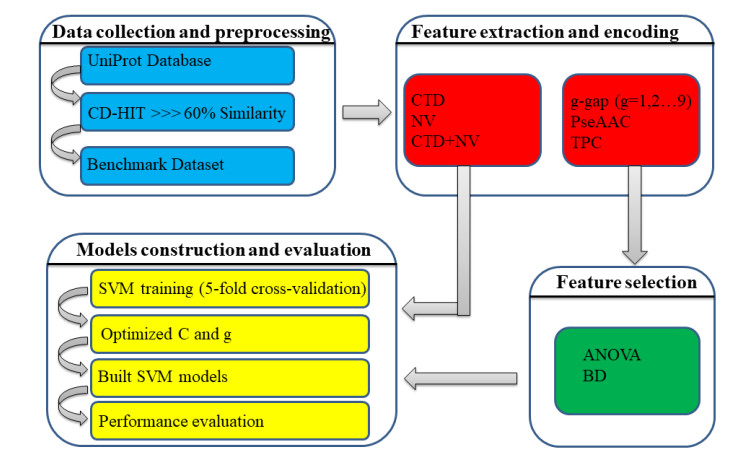
Citation: Vinh Huy Chau. Powerlifting score prediction using a machine learning method[J]. Mathematical Biosciences and Engineering, 2021, 18(2): 1040-1050. doi: 10.3934/mbe.2021056
[1] | Hong Yuan, Jing Huang, Jin Li . Protein-ligand binding affinity prediction model based on graph attention network. Mathematical Biosciences and Engineering, 2021, 18(6): 9148-9162. doi: 10.3934/mbe.2021451 |
[2] | Jun Yan, Tengsheng Jiang, Junkai Liu, Yaoyao Lu, Shixuan Guan, Haiou Li, Hongjie Wu, Yijie Ding . DNA-binding protein prediction based on deep transfer learning. Mathematical Biosciences and Engineering, 2022, 19(8): 7719-7736. doi: 10.3934/mbe.2022362 |
[3] | Yuqing Qian, Tingting Shang, Fei Guo, Chunliang Wang, Zhiming Cui, Yijie Ding, Hongjie Wu . Identification of DNA-binding protein based multiple kernel model. Mathematical Biosciences and Engineering, 2023, 20(7): 13149-13170. doi: 10.3934/mbe.2023586 |
[4] | Mingshuai Chen, Xin Zhang, Ying Ju, Qing Liu, Yijie Ding . iPseU-TWSVM: Identification of RNA pseudouridine sites based on TWSVM. Mathematical Biosciences and Engineering, 2022, 19(12): 13829-13850. doi: 10.3934/mbe.2022644 |
[5] | Jian Zhang, Xingchen Liang, Feng Zhou, Bo Li, Yanling Li . TYLER, a fast method that accurately predicts cyclin-dependent proteins by using computation-based motifs and sequence-derived features. Mathematical Biosciences and Engineering, 2021, 18(5): 6410-6429. doi: 10.3934/mbe.2021318 |
[6] | Xiao Chen, Zhaoyou Zeng . Bird sound recognition based on adaptive frequency cepstral coefficient and improved support vector machine using a hunter-prey optimizer. Mathematical Biosciences and Engineering, 2023, 20(11): 19438-19453. doi: 10.3934/mbe.2023860 |
[7] | Haipeng Zhao, Baozhong Zhu, Tengsheng Jiang, Zhiming Cui, Hongjie Wu . Identification of DNA-protein binding residues through integration of Transformer encoder and Bi-directional Long Short-Term Memory. Mathematical Biosciences and Engineering, 2024, 21(1): 170-185. doi: 10.3934/mbe.2024008 |
[8] | Ying Sun, Wei Du, Lili Yang, Min Dai, Ziying Dou, Yuxiang Wang, Jining Liu, Gang Zheng . Computational methods for recognition of cancer protein markers in saliva. Mathematical Biosciences and Engineering, 2020, 17(3): 2453-2469. doi: 10.3934/mbe.2020134 |
[9] | Xiao Wang, Jianbiao Zhang, Ai Zhang, Jinchang Ren . TKRD: Trusted kernel rootkit detection for cybersecurity of VMs based on machine learning and memory forensic analysis. Mathematical Biosciences and Engineering, 2019, 16(4): 2650-2667. doi: 10.3934/mbe.2019132 |
[10] | Linlu Song, Shangbo Ning, Jinxuan Hou, Yunjie Zhao . Performance of protein-ligand docking with CDK4/6 inhibitors: a case study. Mathematical Biosciences and Engineering, 2021, 18(1): 456-470. doi: 10.3934/mbe.2021025 |
Hormone-binding protein (HPB) is a kind of protein that selectively and non-covalently binds to hormone. HPB is a soluble outer region of the growth hormone receptor (HR), and is an important component of the growth hormone (GH)-insulin-like growth factor axis [1]. The abnormal expression of HBP can cause a variety of diseases [2]. Due to the complex in vivo effects of HBP, its biological function is still not fully understood [1]. Therefore, accurate identification of HBP will be helpful to understand the molecular mechanisms and regulatory pathways of HBP.
Traditional methods to identify HBP were wet biochemical experiments, such as immunoprecipitation, chromatography, crosslinking assays, etc [3,4,5,6]. However, the disadvantages of these methods, such as time-consuming and expensive, make them are unable to keep up with the rapid growth of protein sequences in the post-genomic era. Therefore, it is necessary to develop automatics machine learning methods to identify HBP. As a pioneer work, Tang et al. developed a support vector machine-based method to identify HBP in which proteins were encoded using the optimal features obtained by adopting optimized dipeptide composition [7]. Subsequently, Basith et al. developed a computational predictor named iGHBP, in which an optimal feature set was obtained based on combining dipeptide composition and amino acid index value by adopting two-step feature selection protocol [8]. However, the overall accuracy was still far from satisfactory. In order to improve the performance for the identification of HBP, it is necessary to apply new feature extraction and selection methods to select optimal features to represent HBP.
In this paper, by examining 5 feature encoding methods and 2 feature selection methods, we investigated the advantages and disadvantages of various models for identifying HBP and then established a predictor called HBPred2.0 based on the optimal model. Finally, a user-friendly webserver was established for HBPred2.0. The paper is organized based on the following aspects (Figure 1): (1) The construction of benchmark dataset, (2) feature extraction and selection, (3) machine learning method, and (4) performance evaluation.
This paper adopted the benchmark dataset built by Tang et al. [7]. In the database, there are 123 hormone-binding proteins (HBPs) and 123 none hormone-binding proteins (non-HBPs). To verify the portability and validity of the model, we built a high quality independent dataset by obeying following rules. Firstly, we selected the 357 manually annotated and reviewed HBP proteins from Universal Protein Resource (UniProt) [9] using 'hormone-binding' as keywords in molecular function item of Gene Ontology. Subsequently, we excluded the proteins with sequence identity > 60% by using CD-HIT [10]. Thirdly, sequences that appear in the training dataset were excluded. As a result, 46 HBPs were obtained as independent positive samples. Negative samples were randomly selected from UniProt while using 'hormone' and 'DNA damage binding' as keywords in molecular function item of Gene Ontology, respectively. The sequence identities of negative samples are also ≤ 60%. Finally, 46 non-HBPs (37 hormone proteins and 9 DNA damage binding proteins) were randomly obtained. It should be noted that there is no similar sequences between the training and testing data. All data could be downloaded from http://lin-group.cn/server/HBPred2.0/download.html.
Suppose a sample protein P with L residues, it can be expressed as below.
P=R1R2…Ri…RL | (1) |
where Ri represents the i-th amino acid residue of the sample protein P; i = (1, 2, …L). The Natural Vector Method (NV) method is briefly described as follows [11]:
For each of the 20 amino acid k, define:
wk(⋅):(A,C,D,E,…,W,Y)→(0,1) | (2) |
where wk(Ri) = 1, if Ri = k. otherwise, wk(Ri) = 0.
Let nk be the number of amino acid k in the protein sequence P, which can be calculated as:
nk=∑Li=1wk(Ri) | (3) |
Let s(k)(i) be the distance from the first amino acid (regarded as origin) to the i-th amino acid k in the protein sequence. Let Tk be the total distance of each set of the 20 amino acids. Let μk be the mean position of the amino acid k. And they can be calculated as:
{s(k)(i)=i×wk(Ri)Tk=∑nki=1s(k)(i)μk=Tk/nk | (4) |
Let D2k be the second-order normalized central moments, which can be calculated as:
Dk2=∑nki=1(s(k)(i)−μk)2nk×L | (5) |
Thus, a sample protein P can be formulated as:
P=[nA,μA,DA2,…,nR,μR,DRi2,…nY,μY,DY2]T | (6) |
where the symbol T is the transposition of the vector.
The CTD was first proposed for protein folding class prediction by Dubchak et al. in 1995 [12]. It's a global composition feature extraction method includes hydrophobicity, polarity, normalized van der Waals volume, polarizability, predicted secondary structure, solvent accessibility and so on. In this method, 20 amino acids were divided into 3 different groups: polar, neutral, and hydrophobic. For each of the amino acids attributes, three descriptors (C, T, D) were calculated. 'C' stands for 'Composition', which represents the composition percentage of each group in the peptide sequence, and thus can yield 3 features. 'T' stands for 'Transition', which represents the transition probability between two neighboring amino acids belonging to two different groups, and thus can yield 3 features. 'D' stands for 'Distribution', which represents the position (the first, 25%, 50%, 75%, or 100%) of amino acids in each group in the protein sequence, and thus can yield 5 features for each group (total 15 features).
In this paper, the sequence description of a sample protein P in term of hydrophobicity consists of 3 + 3 + 15 = 21 features.
Adjacent dipeptide composition can only express the correlation between two adjacent amino acid residues. In fact, the amino acids with g-gap residues may be adjacent in three-dimensional space [13]. To find important correlations in protein sequences, we used the g-gap dipeptide composition that extends from adjacent dipeptides. A protein P can be formulated as below by using this method.
P=[vg1,vg2,…,vgi,…vg400]T | (7) |
where the symbol T is the transposition of the vector; the vig is the frequency of the i-th (i = 1, 2, …, 400) g-gap dipeptide and can be formulated as:
vgi=ngiL−g−1 | (8) |
where nig is the number of the i-th g-gap dipeptide; L is the length of the protein P; g is the number of amino acid residues separated by two amino acid residues.
In this paper, we studied the cases of g ranging from 1 to 9 because the case of g = 0 has been studied in reference [7].
The PseAAC method can not only include amino acid composition, but also the correlation of physicochemical properties between two residues [14,15]. In this paper, we adopted the type Ⅱ PseAAC, in which a sample protein P can be formulated as below.
P=[x1,x2…,x400,x401,…x400+9λ]T | (9) |
where '9' is the number of amino acid physicochemical properties considered, namely, hydrophobicity, hydrophilicity, mass, pK1, pK2, pI, rigidity, flexibility and irreplaceability; 'λ' is the rank of correlation; 'x' is the frequencies for each element and is formulated as:
xu={fu∑400i=1fu+ω∑9λj=1τj,(1≤u≤400)ωτj∑400i=1fu+ω∑9λj=1τj,(401≤u≤400+9λ) | (10) |
where ω is the weight factor for the sequence order effect; fu is the frequency of the 400 dipeptides; τj is the correlation factor of the physicochemical properties between residues. More detailed information about the formula derivation process can be found in the reference [16].
In this paper, the parameter λ is from 1 to 95 with the step of 1, the parameter ω is from 0.1 to 1 with the step of 0.1. Therefore, 95×10 = 950 feature subsets based on PseAAC will be obtained.
Tripeptide is composed of three adjacent amino acids in a protein sequence, which is a biosignaling with minimal functionality. By adopting TPC, a sample protein P can be formulated by:
P=[t1,t2,…ti,…,t8000]T | (11) |
where the symbol T is the transposition of the vector; the ti is the frequency of the i-th (i = 1, 2, …, 8000) tripeptide and can be formulated as:
ti=niL−2 | (12) |
where ni is the number of the i-th tripeptide; L is the length of the protein P.
Feature selection is important to improve the classification performance. It can filter the noisy features [17,18,19,20]. We adopted the ANOVA method to select optimal features from g-gap dipeptide compositions and PseAAC. The ANOVA method calculated the ratio of the variance among groups and the variance within groups for each attribute [21,22]. The formula expressions can be described as follows:
F(i)=S2b(i)S2w(i) | (13) |
where F(i) is the score of the i-th feature, a high F(i)-value means a high ability to identify the sample; Sw2(i) is the variance within groups; Sb2(i) is the variance among groups; and they can be calculated as follows:
{S2b(i)=SSb(i)K−1S2w(i)=SSw(i)N−K | (14) |
where SSb(i) is the sum of the squares between the groups; SSw(i) is the sum of squares within the groups; K is the total number of classes; N is the total number of samples.
We adopted the BD method to select optimal features from tripeptide composition [21]. In this algorithm, the confidence level (CL) of each feature can be calculated by:
CLij=1−∑Nik=nijNi!k!(Ni−k)!qkj(1−qj)Ni−k | (15) |
where CLij is the confidence level for the i-th tripeptide in the j-th type; j denotes the type of samples (positive sample or negative sample); Ni is the total number of the i-th tripeptide in the dataset; the probability qj is the relative frequency of type j in the dataset;
According to the formula as defined in Eq. (15), a high CL-value means a high ability to identify the sample. The BD method can extract the over-represented motifs, which is an excellent statistical method widely used in bioinformatics [23,24].
In general, if a model was built on a low-dimensional feature subset, it will not provide enough information. On the contrary, if a model was built on a high-dimensional feature subset, it can lead to information redundancy and overfitting problems. Therefore, the ANOVA and BD method with the IFS process and 5-fold cross-validation was applied to investigate the optimal feature set with the maximum accuracy [7,25,26,27] (Figure 2). We ranked all features according to the F(i)-values or CL-values and obtained new feature vectors, which are shown below.
P′=[g′1,g′2,…g′n]T | (16) |
The first feature subset contains the feature with the highest F(i)-value or CL-value, P' = [g1']T; By adding the second highest F(i)-value or CL-value to the first subset, the second feature subset P' = [g1', g2']T is formed. The procedure was repeated until all features were considered.
The support vector machine (SVM) is a supervised machine learning method and has been widely used in bioinformatics [28,29,30,31,32,33]. Its main idea is to map the input features from low-dimensional space to a high-dimensional space through nonlinear transformation and find the optimal linear classification surface. For convenience, SVM software packages LibSVM can be download from https://www.csie.ntu.edu.tw/~cjlin/libsvm/. In the current study, the LibSVM-3.22 package was adopted to investigate the performance for identifying HBP. Besides, the radical basis function kernel was selected to perform predictions. The grid search spaces are [2-5, 215] with step of 2 for penalty parameter C and [23, 2-15] with step of 2-1 for kernel parameter g.
Three cross-validation methods, namely, the independent dataset test, the sub-sampling test, and the jackknife test, are widely used to investigate the performance of a predictor in practical application [30,34,35,36,37,38,39,40,41]. In order to save computing time, the 5-fold cross-validation test was adopted to calculate the optimal parameter C and g of SVM in this paper.
Five evaluation indexes were adopted to evaluate the models [42,43,44,45,46,47,48,49]. Sensitivity (Sn) is used to evaluate the model's ability to correctly predict positive samples. Specificity (Sp) is used to evaluate the model's ability to correctly predict negative samples. Overall Accuracy (Acc) reflects the proportion of the entire benchmark dataset that can be correctly predicted. The Matthew correlation coefficient (Mcc) is used to evaluate the reliability of the algorithm. Area under the ROC curve (AUC) reflects model's classification ability across decision values. They can be calculated as follows:
{Sn=TPTP+FNSp=TNTN+FPAcc=TP+TNTP+TN+FN+FPMcc=TP×TN−FP×FN√(TP+FP)(TP+FN)(TN+FP)(TN+FN) | (16) |
where TP, TN, FP, and FN represent the number of the correctly recognized positive samples, the number of the correctly recognized negative samples, the number of negative samples recognized as positive samples, and the number of positive samples recognized as negative samples, respectively.
In this study, we examined the performance of 5 feature extraction methods and their combinations. Based on CTD, NV, CTD+NV methods, protein samples can be expressed as 21-D (dimensional), 60-D and 81-D vector, respectively. The Accs of 60.16%, 70.33% and 67.07% were obtained by using SVM in the 5-fold cross-validation, respectively (as shown in Table 1). It was found that the prediction performances were far from satisfactory.
Feature extraction | C | g | Sn(%) | Sp(%) | Acc(%) | Mcc | AUC |
CTD (21-D) | 2 | 23 | 36.59 | 83.74 | 60.16 | 0.230 | 0.654 |
NV (60-D) | 2-5 | 2-13 | 70.73 | 69.92 | 70.33 | 0.407 | 0.762 |
CTD+NV (81-D) | 29 | 2-7 | 70.73 | 63.41 | 67.07 | 0.342 | 0.709 |
Based on the g-gap method, a protein sample can be expressed as a 400-D vector. By changing the value of g from 1 to 9, we obtained 9 feature subsets. Firstly, we investigated the performances of these 400-D features subsets based on SVM. The results were reported in Figure 3A. Subsequently, the ANOVA method with the IFS process was applied to investigate the optimal feature set, and the results were recorded in Figure 3B. One may notice that while g = 1, a maximum Acc of 80.89% was obtained when the top 144 features were used. Obviously, Accs were significantly increased by adopting ANOVA method. However, prediction performances still needed to improve.
Based on the PseAAC method, we obtained 95×10 = 950 (95 kinds of λ and 10 kinds of ω) feature subsets. Firstly, we investigated the performances of these 950 models by using SVM in the 5-fold cross-validation test and reported the results in Figure 4A. It was found that the maximum Acc of 76.83% was achieved when λ = 18 and ω = 0.1. In order to improve Acc, the ANOVA method was adopted to rank the 400 + 18 × 9 = 572 features. By adopting SVM with IFS, a maximum Acc of 84.15% was obtained when the top 194 features were used (Figure 4B). Although the result was encouraging, the Acc still has room to rise.
Based on the TPC method, 8000 features were extracted for each protein sequence. Considering that it would lead to overfitting problem, the BD method was adopted as the feature selection method. By adopting SVM with IFS process in the 5-fold cross-validation test, a maximum Acc of 97.15% was obtained when the top 1169 features were used (Figure 5). In this case, the Sn, Sp and Mcc are 96.75%, 97.56%, and 0.943, respectively The AUC reached 0.994, this result indicates that the performance of the model based on the optimal TPC is smart and reliable for identifying HBP.
In order to show the superiority of SVM to identify HBP, we compared its performance with those of other machine learning algorithms based on the same feature subset (i.e. 1169 optimal features). From Table 2, we can find that the SVM classifier could produce the best performance among these algorithms. Thus, the final model was constructed based on SVM.
Classifier | Sn (%) | Sp (%) | Acc (%) | Mcc | AUC |
J48 | 63.41 | 56.91 | 60.16 | 0.204 | 0.601 |
Bagging | 80.49 | 57.72 | 69.11 | 0.392 | 0.770 |
Random Forest | 88.62 | 84.55 | 86.59 | 0.732 | 0.945 |
Naive Bayes | 95.93 | 92.68 | 94.31 | 0.887 | 0.965 |
SVM | 96.75 | 97.56 | 97.15 | 0.943 | 0.994 |
It is also necessary to compare the methods proposed in this paper with existing methods. Table 3 shows the detailed results of different methods for identifying HBP. Based on the same benchmark dataset, Tang et al. achieved an Acc of 84.9% by using a SVM-based method, in which proteins sequences were encoded using the optimal 0-gap dipeptide composition features obtained by the ANOVA feature selection technique [7]. Basith et al. obtained an Acc of 84.96% in cross-validation test by training an extremely randomized tree with optimal features obtained from dipeptide composition and amino acid index values based on two-step feature selection [8]. Our proposed method could produce an Acc of 97.15% which is superior to the two published results, demonstrating that our method is more powerful for identifying HBP.
Reference | Methods | Sn (%) | Sp (%) | Acc (%) | Mcc | AUC |
[7] | HBPred | 88.6 | 81.3 | 84.9 | - | - |
[8] | iGHBP | 88.62 | 81.30 | 84.96 | - | 0.701 |
This work | HBPred2.0 | 96.75 | 97.56 | 97.15 | 0.943 | 0.994 |
For further comparing the performance of these methods, an independent dataset was used. The results were recorded in Table 4. One may observe that the HBPred2.0 predictor achieved the best performance among the three predictors, suggesting that HBPre2.0 has better generalization ability.
Reference | Methods | Sn (%) | Sp (%) | Acc (%) | Mcc | AUC |
[7] | HBPred | 80.43 | 56.52 | 68.48 | 0.381 | 0.714 |
[8] | iGHBP | 86.96 | 47.83 | 67.39 | 0.380 | - |
This work | HBPred2.0 | 89.13 | 80.43 | 84.78 | 0.698 | 0.814 |
Specificity could reflect the discriminated capability of model on negative samples. From the Table 4, a higher specificity of the HBPred2.0 indicates that the model could produce less false positives.
In this paper, we systematically investigated the performances of various features and classifiers on HBP prediction. By a great number of experiments, we obtained the best model by combining SVM with optimal tripeptide composition. This model could produce the overall accuracy of 84.78% on the independent data. Finally, Due to published database [50,51,52,53] and webserver [54,55,56,57,58,59,60,61,62,63] could provide more convenience for scientific community, we established a free webserver for the proposed method, called HBPred2.0, which can be free accessed form http://lin-group.cn/server/HBPred2.0/. We expect that the tool will help scholars to study the mechanism of HBP's function, and promote the development of related drug research.
This work was supported by the National Nature Scientific Foundation of China (61772119, 31771471, 61702430), Natural Science Foundation for Distinguished Young Scholar of Hebei Province (No. C2017209244), the Central Public Interest Scientific Institution Basal Research Fund (No. 2018GJM06).
The authors declare that there is no conflict of interest.
[1] | D. J. V. D. Windt, V. Sud, H. Zhang, A. Tsung, H. Huang, The effects of physical exercise on fatty liver disease, J. Liver Res., 18 (2018), 89–101. |
[2] |
D. Song, D. S. F. Yu, P. W. C. Li, Y. Lei, The effectiveness of physical exercise on cognitive and psychological outcomes in individuals with mild cognitive impairment: A systematic review and meta-analysis, Int. J. Nurs. Stud., 79 (2018), 155–164. doi: 10.1016/j.ijnurstu.2018.01.002
![]() |
[3] | M. G. Benedetti, G. Furlini, A. Zati, G. L. Mauro, The effectiveness of physical exercise on bone density in osteoporotic patients, Bio. Med. Res. Int., (2018), 4840531. |
[4] |
M. M. Ziaaldini, E. Marzetti, A. Picca, Z. Murlasits, Biochemical pathways of sarcopenia and their modulation by physical exercise: A narrative review, Front. Med., 4 (2017), 167. doi: 10.3389/fmed.2017.00167
![]() |
[5] |
C. M. Hearing, W. C. Chang, K. L. Szuhany, T. Deckersbach, A. A. Nierenberg, L. G. Sylvia, Physical exercise for treatment of mood disorders: A critical review, Curr. Behav. Neurosci. Rep., 3 (2016), 350–359. doi: 10.1007/s40473-016-0089-y
![]() |
[6] | L. W. Judge, L. J. Urbina, D. L. Hoover, B. W. Craig, L. M. Judge, B. M. Leitzelar, et al., The impact of competitive trait anxiety on collegiate powerlifting performance, J. Strength Cond. Res., 30 (2016), 2399–2405. |
[7] | M. Mottaghi, A. Atarodi, Z. Rohani, The relationship between coaches' and athletes' competitive anxiety and their performance, Iran. J. Psychiatry Behav. Sci., 7 (2013), 68–76. |
[8] |
H. J. Pritchard, D. A. Tod, M. J. Barnes, J. W. Keogh, M. R. McGuigan, Tapering practices of New Zealand's elite raw powerlifters, J. Strength Cond. Res., 30 (2016), 1796–1804. doi: 10.1519/JSC.0000000000001292
![]() |
[9] |
M. Zawadka, M. S. Paszkowska, P. Gawda, E. Lukasik, J. Smolka, M. Jablonski, What factors can affect lumbopelvic flexion-extension motion in the sagittal plane?: A literature review, Hum. Mov. Sci., 58 (2018), 205–218. doi: 10.1016/j.humov.2018.02.008
![]() |
[10] | A. C. Fry, W. J. Kraemer, M. H. Stone, L. P. Koziris, J. T. Thrush, S. J. Fleck, Relationships between serum testosterone, cortisol and weightlifting performance, J. Strength Cond. Res., 14 (2000), 338–343. |
[11] | I. Sandau, M. Witt, The influence of stress factors on joint loading of lower extremities in weightlifting, Ger. J. Sports Med./Deutsch. Z. Sportmed., 69 (2018), 1–10. |
[12] | P. A. Solberg, W. G. Hopkins, G. Paulsen, T. A. Haugen, Peak age and performance progression in world-class weightlifting and powerlifting athletes, Int. J. Sports Physiol. Perform., (2019), 1–24. |
[13] |
C. Latella, W. P. Teo, J. Spathis, D. V. D. Hoek, Long-term strength adaptation: A 15-year analysis of powerlifting athletes, J. Strength Cond. Res., 34 (2020), 2412. doi: 10.1519/JSC.0000000000003657
![]() |
[14] |
C. Latella, D. V. D. Hoek, W. P. Teo, Factors affecting powerlifting performance: an analysis of age-and weight-based determinants of relative strength, Int. J. Perform. Anal. Sport, 18 (2018), 532–544. doi: 10.1080/24748668.2018.1496393
![]() |
[15] | M. M. Anton, W. W. Spirduso, H. Tanaka, Age-related declines in anaerobic muscular performance: Weightlifting and powerlifting, Med. Sci. Sports Exercise, 36 (2014), 143–147. |
[16] |
L. P. James, P. Comfort, T. J. Suchomel, V. G. Kelly, E. M. Beckman, G. G. Haff, The impact of power clean ability and training age on adaptations to weightlifting-style training, J. Strength Cond. Res., 33 (2019), 2936–2944. doi: 10.1519/JSC.0000000000002534
![]() |
[17] |
J. Pearson, J. G. Spathis, D. J. V. D. Hoek, P. J. Owen, J. Weakley, C. Latella, Effect of competition frequency on strength performance of powerlifting athletes, J. Strength Cond. Res., 34 (2020), 1213–1219. doi: 10.1519/JSC.0000000000003563
![]() |
[18] |
N. A. Coker, A. N. Varanoske, K. M. Baker, D. L. H. Vaughn, A. J. Wells, Predictors of competitive success of national-level powerlifters: A multilevel analysis, Int. J. Perform. Anal. Sport, 18 (2018), 796–805. doi: 10.1080/24748668.2018.1519751
![]() |
[19] | S. K. Travis, M. C. Zourdos, C. D. Bazyler, Weight selection attempts of elite classic powerlifters, Percept. Mot. Skills, 2020. |
[20] | M. Z. Naser, A. Alavi, Insights into performance fitness and error metrics for machine learning, preprint, arXiv: 2006.00887. |
[21] | A. Botchkarev, Performance metrics (error measures) in machine learning regression, forecasting and prognostics: Properties and typology, preprint, arXiv: 1809.03006. |
[22] |
G. B. Huang, Q. Y. Zhu, C. K. Siew, Extreme learning machine: Theory and applications, Neurocomputing, 70 (2006), 489–501. doi: 10.1016/j.neucom.2005.12.126
![]() |
[23] | M. Rocca, C. Perna, Nonlinear autoregressive sieve bootstrap based on extreme learning machines, Math. Biosci. Eng., 17 (2019), 636–653. |
[24] | G. B. Huang, H. Zhou, X. Ding, R. Zhang, Extreme learning machine for regression and multiclass classification, IEEE Trans. Syst., Man, Cybern., Part B (Cybern.), 42 (2011), 513–529. |
[25] | J. Tang, C. Deng, G. B. Huang, Extreme learning machine for multilayer perceptron, IEEE Trans. Neural Networks Learn. Syst., 27 (2015), 809–821. |
[26] | W. Deng, Q. Zheng, L. Chen, Regularized extreme learning machine, 2009 IEEE Symp. Comput. Intel. Data Min., (2009), 389–395. |
[27] | Y. Zheng, B. Chen, S. Wang, W. Wang, W. Qin, Mixture correntropy-based kernel extreme learning machines, IEEE Trans. Neural Networks Learn. Syst., 2020. |
[28] | J. Cao, H. Dai, B. Lei, C. Yin, H. Zeng, A. Kummert, Maximum correntropy criterion-based hierarchical one-class classification, IEEE Trans. Neural Networks Learn. Syst., 2020. |
[29] |
O. F. Ertugrul, A novel randomized machine learning approach: Reservoir computing extreme learning machine. Appl. Soft Comput., 94 (2020), 106433. doi: 10.1016/j.asoc.2020.106433
![]() |
[30] |
S. Mirjalili, A. Lewis, The whale optimization algorithm. Adv. Eng. Software, 95 (2016), 51–67. doi: 10.1016/j.advengsoft.2016.01.008
![]() |
[31] |
L. L. Li, J. Sun, M. L. Tseng, Z. G. Li, Extreme learning machine optimized by whale optimization algorithm using insulated gate bipolar transistor module aging degree evaluation, Expert Syst. Appl., 127 (2019), 58–67. doi: 10.1016/j.eswa.2019.03.002
![]() |
[32] | https://www.powerlifting.sport/championships/results.html |
[33] | https://www.kaggle.com/open-powerlifting/powerlifting-database |
[34] | T. Yang, Study on the growth rule of the olympic men's weightlifting champion and winning factors. Sichuan Sports Sci., 38 (2019), 54–58. |
[35] |
B. T. Le, D. Xiao, Y. Mao, D. He, Coal analysis based on visible-infrared spectroscopy and a deep neural network, Infrared Phys. Technol., 93 (2018), 34–40. doi: 10.1016/j.infrared.2018.07.013
![]() |
[36] | D. Xiao, B. T. Le, Rapid analysis of coal characteristics based on deep learning and visible-infrared spectroscopy, Microchem. J., (2020), 104880. |
1. | Zhihua Chen, Xinke Wang, Peng Gao, Hongju Liu, Bosheng Song, Predicting Disease Related microRNA Based on Similarity and Topology, 2019, 8, 2073-4409, 1405, 10.3390/cells8111405 | |
2. | Lei Zheng, Dongyang Liu, Wuritu Yang, Lei Yang, Yongchun Zuo, RaacLogo: a new sequence logo generator by using reduced amino acid clusters, 2020, 1467-5463, 10.1093/bib/bbaa096 | |
3. | Leyi Wei, Wenjia He, Adeel Malik, Ran Su, Lizhen Cui, Balachandran Manavalan, Computational prediction and interpretation of cell-specific replication origin sites from multiple eukaryotes by exploiting stacking framework, 2020, 1467-5463, 10.1093/bib/bbaa275 | |
4. | Chaolu Meng, Shunshan Jin, Lei Wang, Fei Guo, Quan Zou, AOPs-SVM: A Sequence-Based Classifier of Antioxidant Proteins Using a Support Vector Machine, 2019, 7, 2296-4185, 10.3389/fbioe.2019.00224 | |
5. | Xing Gao, Guilin Li, A Cytokine Protein Identification Model Based on the Compressed PseKRAAC Features, 2020, 8, 2169-3536, 141422, 10.1109/ACCESS.2020.3013409 | |
6. | Meijie Zhang, Luyang Cheng, Yina Zhang, Characterization of Dysregulated lncRNA-Associated ceRNA Network Reveals Novel lncRNAs With ceRNA Activity as Epigenetic Diagnostic Biomarkers for Osteoporosis Risk, 2020, 8, 2296-634X, 10.3389/fcell.2020.00184 | |
7. | Yu-Miao Chen, Xin-Ping Zu, Dan Li, Identification of Proteins of Tobacco Mosaic Virus by Using a Method of Feature Extraction, 2020, 11, 1664-8021, 10.3389/fgene.2020.569100 | |
8. | Qingwen Li, Wenyang Zhou, Donghua Wang, Sui Wang, Qingyuan Li, Prediction of Anticancer Peptides Using a Low-Dimensional Feature Model, 2020, 8, 2296-4185, 10.3389/fbioe.2020.00892 | |
9. | Xudong Zhao, Hanxu Wang, Hangyu Li, Yiming Wu, Guohua Wang, Identifying Plant Pentatricopeptide Repeat Proteins Using a Variable Selection Method, 2021, 12, 1664-462X, 10.3389/fpls.2021.506681 | |
10. | Hasan Zulfiqar, Muhammad Shareef Masoud, Hui Yang, Shu-Guang Han, Cheng-Yan Wu, Hao Lin, Balachandran Manavalan, Screening of Prospective Plant Compounds as H1R and CL1R Inhibitors and Its Antiallergic Efficacy through Molecular Docking Approach, 2021, 2021, 1748-6718, 1, 10.1155/2021/6683407 | |
11. | Wei Chen, Kewei Liu, Analysis and Comparison of RNA Pseudouridine Site Prediction Tools, 2020, 15, 15748936, 279, 10.2174/1574893614666191018171521 | |
12. | Lei Xu, Guangmin Liang, Baowen Chen, Xu Tan, Huaikun Xiang, Changrui Liao, A Computational Method for the Identification of Endolysins and Autolysins, 2020, 27, 09298665, 329, 10.2174/0929866526666191002104735 | |
13. | Fang Wang, Zheng-Xing Guan, Fu-Ying Dao, Hui Ding, A Brief Review of the Computational Identification of Antifreeze Protein, 2019, 23, 13852728, 1671, 10.2174/1385272823666190718145613 | |
14. | Zhiyu Tao, Benzhi Dong, Zhixia Teng, Yuming Zhao, The Classification of Enzymes by Deep Learning, 2020, 8, 2169-3536, 89802, 10.1109/ACCESS.2020.2992468 | |
15. | Meng-Lu Liu, Wei Su, Jia-Shu Wang, Yu-He Yang, Hui Yang, Hao Lin, Predicting Preference of Transcription Factors for Methylated DNA Using Sequence Information, 2020, 22, 21622531, 1043, 10.1016/j.omtn.2020.07.035 | |
16. | Zi-Mei Zhang, Zheng-Xing Guan, Fang Wang, Dan Zhang, Hui Ding, Application of Machine Learning Methods in Predicting Nuclear Receptors and their Families, 2020, 16, 15734064, 594, 10.2174/1573406415666191004125551 | |
17. | Kuan Li, Yue Zhong, Xuan Lin, Zhe Quan, Predicting the Disease Risk of Protein Mutation Sequences With Pre-training Model, 2020, 11, 1664-8021, 10.3389/fgene.2020.605620 | |
18. | Chen-Chen Li, Bin Liu, MotifCNN-fold: protein fold recognition based on fold-specific features extracted by motif-based convolutional neural networks, 2020, 21, 1467-5463, 2133, 10.1093/bib/bbz133 | |
19. | Lifu Zhang, Benzhi Dong, Zhixia Teng, Ying Zhang, Liran Juan, Identification of Human Enzymes Using Amino Acid Composition and the Composition of k-Spaced Amino Acid Pairs, 2020, 2020, 2314-6133, 1, 10.1155/2020/9235920 | |
20. | Chunyu Wang, Kai Sun, Juexin Wang, Maozu Guo, Data fusion-based algorithm for predicting miRNA–Disease associations, 2020, 88, 14769271, 107357, 10.1016/j.compbiolchem.2020.107357 | |
21. | Ruirui Liang, Jiayang Xie, Chi Zhang, Mengying Zhang, Hai Huang, Haizhong Huo, Xin Cao, Bing Niu, Identifying Cancer Targets Based on Machine Learning Methods via Chou’s 5-steps Rule and General Pseudo Components, 2019, 19, 15680266, 2301, 10.2174/1568026619666191016155543 | |
22. | Ting Liu, Hua Tang, A Brief Survey of Machine Learning Methods in Identification of Mitochondria Proteins in Malaria Parasite, 2020, 26, 13816128, 3049, 10.2174/1381612826666200310122324 | |
23. | Zijie Sun, Shenghui Huang, Lei Zheng, Pengfei Liang, Wuritu Yang, Yongchun Zuo, ICTC-RAAC: An improved web predictor for identifying the types of ion channel-targeted conotoxins by using reduced amino acid cluster descriptors, 2020, 89, 14769271, 107371, 10.1016/j.compbiolchem.2020.107371 | |
24. | Ying Wang, Juanjuan Kang, Ning Li, Yuwei Zhou, Zhongjie Tang, Bifang He, Jian Huang, NeuroCS: A Tool to Predict Cleavage Sites of Neuropeptide Precursors, 2020, 27, 09298665, 337, 10.2174/0929866526666191112150636 | |
25. | Shanwen Sun, Hui Ding, Donghua Wang, Shuguang Han, Identifying Antifreeze Proteins Based on Key Evolutionary Information, 2020, 8, 2296-4185, 10.3389/fbioe.2020.00244 | |
26. | Nguyen Quoc Khanh Le, Fertility-GRU: Identifying Fertility-Related Proteins by Incorporating Deep-Gated Recurrent Units and Original Position-Specific Scoring Matrix Profiles, 2019, 18, 1535-3893, 3503, 10.1021/acs.jproteome.9b00411 | |
27. | Zhourun Wu, Qing Liao, Bin Liu, idenPC-MIIP: identify protein complexes from weighted PPI networks using mutual important interacting partner relation, 2021, 22, 1477-4054, 1972, 10.1093/bib/bbaa016 | |
28. | Zhe Liu, Yingli Gong, Yihang Bao, Yuanzhao Guo, Han Wang, Guan Ning Lin, TMPSS: A Deep Learning-Based Predictor for Secondary Structure and Topology Structure Prediction of Alpha-Helical Transmembrane Proteins, 2021, 8, 2296-4185, 10.3389/fbioe.2020.629937 | |
29. | Hao Lv, Fu-Ying Dao, Zheng-Xing Guan, Dan Zhang, Jiu-Xin Tan, Yong Zhang, Wei Chen, Hao Lin, iDNA6mA-Rice: A Computational Tool for Detecting N6-Methyladenine Sites in Rice, 2019, 10, 1664-8021, 10.3389/fgene.2019.00793 | |
30. | Ruiyan Hou, Jin Wu, Lei Xu, Quan Zou, Yi-Jun Wu, Computational Prediction of Protein Arginine Methylation Based on Composition–Transition–Distribution Features, 2020, 5, 2470-1343, 27470, 10.1021/acsomega.0c03972 | |
31. | Xiao-Yang Jing, Feng-Min Li, Predicting Cell Wall Lytic Enzymes Using Combined Features, 2021, 8, 2296-4185, 10.3389/fbioe.2020.627335 | |
32. | Hong-Fei Li, Xian-Fang Wang, Hua Tang, Predicting Bacteriophage Enzymes and Hydrolases by Using Combined Features, 2020, 8, 2296-4185, 10.3389/fbioe.2020.00183 | |
33. | Wei Chen, Pengmian Feng, Xiaoming Song, Hao Lv, Hao Lin, iRNA-m7G: Identifying N7-methylguanosine Sites by Fusing Multiple Features, 2019, 18, 21622531, 269, 10.1016/j.omtn.2019.08.022 | |
34. | Chao Wang, Pingping Wang, Shuguang Han, Lida Wang, Yuming Zhao, Liran Juan, FunEffector-Pred: Identification of Fungi Effector by Activate Learning and Genetic Algorithm Sampling of Imbalanced Data, 2020, 8, 2169-3536, 57674, 10.1109/ACCESS.2020.2982410 | |
35. | Yanjuan Li, Zitong Zhang, Zhixia Teng, Xiaoyan Liu, Hui Ding, PredAmyl-MLP: Prediction of Amyloid Proteins Using Multilayer Perceptron, 2020, 2020, 1748-6718, 1, 10.1155/2020/8845133 | |
36. | Wanben Zhong, Bineng Zhong, Hongbo Zhang, Ziyi Chen, Yan Chen, Identification of Anti-cancer Peptides Based on Multi-classifier System, 2020, 22, 13862073, 694, 10.2174/1386207322666191203141102 | |
37. | Xiaoqing Ru, Peigang Cao, Lihong Li, Quan Zou, Selecting Essential MicroRNAs Using a Novel Voting Method, 2019, 18, 21622531, 16, 10.1016/j.omtn.2019.07.019 | |
38. | Feifei Cui, Zilong Zhang, Quan Zou, Sequence representation approaches for sequence-based protein prediction tasks that use deep learning, 2021, 20, 2041-2649, 61, 10.1093/bfgp/elaa030 | |
39. | Muhammad Arif, Farman Ali, Saeed Ahmad, Muhammad Kabir, Zakir Ali, Maqsood Hayat, Pred-BVP-Unb: Fast prediction of bacteriophage Virion proteins using un-biased multi-perspective properties with recursive feature elimination, 2020, 112, 08887543, 1565, 10.1016/j.ygeno.2019.09.006 | |
40. | Yongxian Fan, Meijun Chen, Qingqi Zhu, lncLocPred: Predicting LncRNA Subcellular Localization Using Multiple Sequence Feature Information, 2020, 8, 2169-3536, 124702, 10.1109/ACCESS.2020.3007317 | |
41. | Changgeng Tan, Tong Wang, Wenyi Yang, Lei Deng, PredPSD: A Gradient Tree Boosting Approach for Single-Stranded and Double-Stranded DNA Binding Protein Prediction, 2019, 25, 1420-3049, 98, 10.3390/molecules25010098 | |
42. | Chunyu Wang, Jialin Li, Ying Zhang, Maozu Guo, Identification of Type VI Effector Proteins Using a Novel Ensemble Classifier, 2020, 8, 2169-3536, 75085, 10.1109/ACCESS.2020.2985111 | |
43. | Zhibin Lv, Hui Ding, Lei Wang, Quan Zou, A Convolutional Neural Network Using Dinucleotide One-hot Encoder for identifying DNA N6-Methyladenine Sites in the Rice Genome, 2021, 422, 09252312, 214, 10.1016/j.neucom.2020.09.056 | |
44. | Xiao-Yang Jing, Feng-Min Li, Identifying Heat Shock Protein Families from Imbalanced Data by Using Combined Features, 2020, 2020, 1748-670X, 1, 10.1155/2020/8894478 | |
45. | Hui Yang, Wuritu Yang, Fu-Ying Dao, Hao Lv, Hui Ding, Wei Chen, Hao Lin, A comparison and assessment of computational method for identifying recombination hotspots in Saccharomyces cerevisiae , 2020, 21, 1467-5463, 1568, 10.1093/bib/bbz123 | |
46. | Balachandran Manavalan, Md. Mehedi Hasan, Shaherin Basith, Vijayakumar Gosu, Tae-Hwan Shin, Gwang Lee, Empirical Comparison and Analysis of Web-Based DNA N4-Methylcytosine Site Prediction Tools, 2020, 22, 21622531, 406, 10.1016/j.omtn.2020.09.010 | |
47. | Zheng-Xing Guan, Shi-Hao Li, Zi-Mei Zhang, Dan Zhang, Hui Yang, Hui Ding, A Brief Survey for MicroRNA Precursor Identification Using Machine Learning Methods, 2020, 21, 13892029, 11, 10.2174/1389202921666200214125102 | |
48. | Balachandran Manavalan, Shaherin Basith, Tae Hwan Shin, Gwang Lee, Computational prediction of species-specific yeast DNA replication origin via iterative feature representation, 2020, 1467-5463, 10.1093/bib/bbaa304 | |
49. | Xian-Fang Wang, Peng Gao, Yi-Feng Liu, Hong-Fei Li, Fan Lu, Predicting Thermophilic Proteins by Machine Learning, 2020, 15, 15748936, 493, 10.2174/1574893615666200207094357 | |
50. | Chang Lu, Yingli Gong, Zhe Liu, Yuanzhao Guo, Zhiqiang Ma, Han Wang, TM-ZC: A Deep Learning-Based Predictor for the Z-Coordinate of Residues in α-Helical Transmembrane Proteins, 2020, 8, 2169-3536, 40129, 10.1109/ACCESS.2020.2976797 | |
51. | Tianyi Zhao, Donghua Wang, Yang Hu, Ningyi Zhang, Tianyi Zang, Yadong Wang, Identifying Alzheimer’s Disease-related miRNA Based on Semi-clustering, 2019, 19, 15665232, 216, 10.2174/1566523219666190924113737 | |
52. | Dan Zhang, Zhao-Chun Xu, Wei Su, Yu-He Yang, Hao Lv, Hui Yang, Hao Lin, Jinbo Xu, iCarPS: a computational tool for identifying protein carbonylation sites by novel encoded features, 2020, 1367-4803, 10.1093/bioinformatics/btaa702 | |
53. | Dan Zhang, Zheng-Xing Guan, Zi-Mei Zhang, Shi-Hao Li, Fu-Ying Dao, Hua Tang, Hao Lin, Recent Development of Computational Predicting Bioluminescent Proteins, 2020, 25, 13816128, 4264, 10.2174/1381612825666191107100758 | |
54. | Yanwen Li, Feng Pu, Yu Feng, Jinchao Ji, Hongguang Sun, Han Wang, MRMD-palm: A novel method for the identification of palmitoylated protein, 2021, 210, 01697439, 104245, 10.1016/j.chemolab.2021.104245 | |
55. | Feng-Min Li, Xiao-Wei Gao, Predicting Gram-Positive Bacterial Protein Subcellular Location by Using Combined Features, 2020, 2020, 2314-6133, 1, 10.1155/2020/9701734 | |
56. | Zhiyu Tao, Yanjuan Li, Zhixia Teng, Yuming Zhao, Hui Ding, A Method for Identifying Vesicle Transport Proteins Based on LibSVM and MRMD, 2020, 2020, 1748-6718, 1, 10.1155/2020/8926750 | |
57. | Duyen Thi Do, Nguyen Quoc Khanh Le, A sequence-based approach for identifying recombination spots in Saccharomyces cerevisiae by using hyper-parameter optimization in FastText and support vector machine, 2019, 194, 01697439, 103855, 10.1016/j.chemolab.2019.103855 | |
58. | Chunyu Wang, Jialin Li, Xiaoyan Liu, Maozu Guo, Predicting Sub-Golgi Apparatus Resident Protein With Primary Sequence Hybrid Features, 2020, 8, 2169-3536, 4442, 10.1109/ACCESS.2019.2962821 | |
59. | Qingwen Li, Benzhi Dong, Donghua Wang, Sui Wang, Identification of Secreted Proteins From Malaria Protozoa With Few Features, 2020, 8, 2169-3536, 89793, 10.1109/ACCESS.2020.2994206 | |
60. | Pengmian Feng, Weiwei Liu, Cong Huang, Zhaohui Tang, Classifying the superfamily of small heat shock proteins by using g-gap dipeptide compositions, 2021, 167, 01418130, 1575, 10.1016/j.ijbiomac.2020.11.111 | |
61. | Hongfei Li, Haoze Du, Xianfang Wang, Peng Gao, Yifeng Liu, Weizhong Lin, Remarks on Computational Method for Identifying Acid and Alkaline Enzymes, 2020, 26, 13816128, 3105, 10.2174/1381612826666200617170826 | |
62. | Shahid Akbar, Salman Khan, Farman Ali, Maqsood Hayat, Muhammad Qasim, Sarah Gul, iHBP-DeepPSSM: Identifying hormone binding proteins using PsePSSM based evolutionary features and deep learning approach, 2020, 204, 01697439, 104103, 10.1016/j.chemolab.2020.104103 | |
63. | He Zhuang, Ying Zhang, Shuo Yang, Liang Cheng, Shu-Lin Liu, A Mendelian Randomization Study on Infant Length and Type 2 Diabetes Mellitus Risk, 2019, 19, 15665232, 224, 10.2174/1566523219666190925115535 | |
64. | Xin Gao, Donghua Wang, Jun Zhang, Qing Liao, Bin Liu, iRBP-Motif-PSSM: Identification of RNA-Binding Proteins Based on Collaborative Learning, 2019, 7, 2169-3536, 168956, 10.1109/ACCESS.2019.2952621 | |
65. | Zihao Yan, Dong Chen, Zhixia Teng, Donghua Wang, Yanjuan Li, SMOPredT4SE: An Effective Prediction of Bacterial Type IV Secreted Effectors Using SVM Training With SMO, 2020, 8, 2169-3536, 25570, 10.1109/ACCESS.2020.2971091 | |
66. | Dan Zhang, Hua-Dong Chen, Hasan Zulfiqar, Shi-Shi Yuan, Qin-Lai Huang, Zhao-Yue Zhang, Ke-Jun Deng, Watshara Shoombuatong, iBLP: An XGBoost-Based Predictor for Identifying Bioluminescent Proteins, 2021, 2021, 1748-6718, 1, 10.1155/2021/6664362 | |
67. | Zi-Mei Zhang, Jiu-Xin Tan, Fang Wang, Fu-Ying Dao, Zhao-Yue Zhang, Hao Lin, Early Diagnosis of Hepatocellular Carcinoma Using Machine Learning Method, 2020, 8, 2296-4185, 10.3389/fbioe.2020.00254 | |
68. | Zifan Guo, Pingping Wang, Zhendong Liu, Yuming Zhao, Discrimination of Thermophilic Proteins and Non-thermophilic Proteins Using Feature Dimension Reduction, 2020, 8, 2296-4185, 10.3389/fbioe.2020.584807 | |
69. | Rajiv G. Govindaraj, Sathiyamoorthy Subramaniyam, Balachandran Manavalan, Extremely-randomized-tree-based Prediction of N6-methyladenosine Sites inSaccharomyces cerevisiae, 2020, 21, 13892029, 26, 10.2174/1389202921666200219125625 | |
70. | Sola Gbenro, Kyle Hippe, Renzhi Cao, 2020, HMMeta, 9781450379649, 1, 10.1145/3388440.3414702 | |
71. | Yixiao Zhai, Yu Chen, Zhixia Teng, Yuming Zhao, Identifying Antioxidant Proteins by Using Amino Acid Composition and Protein-Protein Interactions, 2020, 8, 2296-634X, 10.3389/fcell.2020.591487 | |
72. | Xianhai Li, Qiang Tang, Hua Tang, Wei Chen, Identifying Antioxidant Proteins by Combining Multiple Methods, 2020, 8, 2296-4185, 10.3389/fbioe.2020.00858 | |
73. | Bing Rao, Lichao Zhang, Guoying Zhang, ACP-GCN: The Identification of Anticancer Peptides Based on Graph Convolution Networks, 2020, 8, 2169-3536, 176005, 10.1109/ACCESS.2020.3023800 | |
74. | Ni Kou, Wenyang Zhou, Yuzhu He, Xiaoxia Ying, Songling Chai, Tao Fei, Wenqi Fu, Jiaqian Huang, Huiying Liu, A Mendelian Randomization Analysis to Expose the Causal Effect of IL-18 on Osteoporosis Based on Genome-Wide Association Study Data, 2020, 8, 2296-4185, 10.3389/fbioe.2020.00201 | |
75. | Peng Xu, Geng Zhao, Zheng Kou, Gang Fang, Wenbin Liu, Classification of Cancers Based on a Comprehensive Pathway Activity Inferred by Genes and Their Interactions, 2020, 8, 2169-3536, 30515, 10.1109/ACCESS.2020.2973220 | |
76. | Shi-Hao Li, Zheng-Xing Guan, Dan Zhang, Zi-Mei Zhang, Jian Huang, Wuritu Yang, Hao Lin, Recent Advancement in Predicting Subcellular Localization of Mycobacterial Protein with Machine Learning Methods, 2020, 16, 15734064, 605, 10.2174/1573406415666191004101913 | |
77. | Han Luo, Donghua Wang, Juan Liu, Ying Ju, Zhe Jin, A Framework Integrating Heterogeneous Databases for the Completion of Gene Networks, 2019, 7, 2169-3536, 168859, 10.1109/ACCESS.2019.2954994 | |
78. | Zhibin Lv, Feifei Cui, Quan Zou, Lichao Zhang, Lei Xu, Anticancer peptides prediction with deep representation learning features, 2021, 1467-5463, 10.1093/bib/bbab008 | |
79. | Chang Lu, Zhe Liu, Bowen Kan, Yingli Gong, Zhiqiang Ma, Han Wang, TMP-SSurface: A Deep Learning-Based Predictor for Surface Accessibility of Transmembrane Protein Residues, 2019, 9, 2073-4352, 640, 10.3390/cryst9120640 | |
80. | Yideng Cai, Jiacheng Wang, Lei Deng, SDN2GO: An Integrated Deep Learning Model for Protein Function Prediction, 2020, 8, 2296-4185, 10.3389/fbioe.2020.00391 | |
81. | Hao Wang, Qilemuge Xi, Pengfei Liang, Lei Zheng, Yan Hong, Yongchun Zuo, IHEC_RAAC: a online platform for identifying human enzyme classes via reduced amino acid cluster strategy, 2021, 53, 0939-4451, 239, 10.1007/s00726-021-02941-9 | |
82. | Shanwen Sun, Chunyu Wang, Hui Ding, Quan Zou, Machine learning and its applications in plant molecular studies, 2020, 19, 2041-2657, 40, 10.1093/bfgp/elz036 | |
83. | Yichen Guo, Ke Yan, Hao Wu, Bin Liu, ReFold-MAP: Protein remote homology detection and fold recognition based on features extracted from profiles, 2020, 611, 00032697, 114013, 10.1016/j.ab.2020.114013 | |
84. | Guilin Li, Xing Gao, The Feature Compression Algorithms for Identifying Cytokines Based on CNT Features, 2020, 8, 2169-3536, 83645, 10.1109/ACCESS.2020.2989749 | |
85. | Qilemuge Xi, Hao Wang, Liuxi Yi, Jian Zhou, Yuchao Liang, Xiaoqing Zhao, Yongchun Zuo, Lei Chen, ANPrAod: Identify Antioxidant Proteins by Fusing Amino Acid Clustering Strategy and N -Peptide Combination, 2021, 2021, 1748-6718, 1, 10.1155/2021/5518209 | |
86. | Lesong Wei, Xiucai Ye, Yuyang Xue, Tetsuya Sakurai, Leyi Wei, ATSE: a peptide toxicity predictor by exploiting structural and evolutionary information based on graph neural network and attention mechanism, 2021, 1467-5463, 10.1093/bib/bbab041 | |
87. | Hasan Zulfiqar, Rida Sarwar Khan, Farwa Hassan, Kyle Hippe, Cassandra Hunt, Hui Ding, Xiao-Ming Song, Renzhi Cao, Computational identification of N4-methylcytosine sites in the mouse genome with machine-learning method, 2021, 18, 1551-0018, 3348, 10.3934/mbe.2021167 | |
88. | Yanwen Li, Feng Pu, Jingru Wang, Zhiguo Zhou, Chunhua Zhang, Fei He, Zhiqiang Ma, Jingbo Zhang, Machine Learning Methods in Prediction of Protein Palmitoylation Sites: A Brief Review, 2021, 27, 13816128, 2189, 10.2174/1381612826666201112142826 | |
89. | Shulin Zhao, Ying Ju, Xiucai Ye, Jun Zhang, Shuguang Han, Bioluminescent Proteins Prediction with Voting Strategy, 2021, 16, 15748936, 240, 10.2174/1574893615999200601122328 | |
90. | Yinuo Lyu, Zhen Zhang, Jiawei Li, Wenying He, Yijie Ding, Fei Guo, iEnhancer-KL: A Novel Two-Layer Predictor for Identifying Enhancers by Position Specific of Nucleotide Composition, 2021, 18, 1545-5963, 2809, 10.1109/TCBB.2021.3053608 | |
91. | Hongdi Pei, Jiayu Li, Shuhan Ma, Jici Jiang, Mingxin Li, Quan Zou, Zhibin Lv, Identification of Thermophilic Proteins Based on Sequence-Based Bidirectional Representations from Transformer-Embedding Features, 2023, 13, 2076-3417, 2858, 10.3390/app13052858 | |
92. | Hasan Zulfiqar, Qin-Lai Huang, Hao Lv, Zi-Jie Sun, Fu-Ying Dao, Hao Lin, Deep-4mCGP: A Deep Learning Approach to Predict 4mC Sites in Geobacter pickeringii by Using Correlation-Based Feature Selection Technique, 2022, 23, 1422-0067, 1251, 10.3390/ijms23031251 | |
93. | Ting Liu, Jiamao Chen, Qian Zhang, Kyle Hippe, Cassandra Hunt, Thu Le, Renzhi Cao, Hua Tang, The Development of Machine Learning Methods in Discriminating Secretory Proteins of Malaria Parasite, 2022, 29, 09298673, 807, 10.2174/0929867328666211005140625 | |
94. | Jing Guo, TCN-HBP: A Deep Learning Method for Identifying Hormone-Binding Proteins from Amino Acid Sequences Based on a Temporal Convolution Neural Network, 2021, 2025, 1742-6588, 012002, 10.1088/1742-6596/2025/1/012002 | |
95. | Chaolu Meng, Jin Wu, Fei Guo, Benzhi Dong, Lei Xu, CWLy-pred: A novel cell wall lytic enzyme identifier based on an improved MRMD feature selection method, 2020, 112, 08887543, 4715, 10.1016/j.ygeno.2020.08.015 | |
96. | Zhixia Teng, Zitong Zhang, Zhen Tian, Yanjuan Li, Guohua Wang, ReRF-Pred: predicting amyloidogenic regions of proteins based on their pseudo amino acid composition and tripeptide composition, 2021, 22, 1471-2105, 10.1186/s12859-021-04446-4 | |
97. | Peiran Jiang, Wanshan Ning, Yunshu Shi, Chuan Liu, Saijun Mo, Haoran Zhou, Kangdong Liu, Yaping Guo, FSL-Kla: A few-shot learning-based multi-feature hybrid system for lactylation site prediction, 2021, 19, 20010370, 4497, 10.1016/j.csbj.2021.08.013 | |
98. | Mujiexin Liu, Hui Chen, Dong Gao, Cai-Yi Ma, Zhao-Yue Zhang, Balachandran Manavalan, Identification of Helicobacter pylori Membrane Proteins Using Sequence-Based Features, 2022, 2022, 1748-6718, 1, 10.1155/2022/7493834 | |
99. | Yi-Wei Zhao, Shihua Zhang, Hui Ding, Recent Development of Machine Learning Methods in Sumoylation Sites Prediction, 2022, 29, 09298673, 894, 10.2174/0929867328666210915112030 | |
100. | Juexin Wang, Yan Wang, Towards Machine Learning in Molecular Biology, 2020, 17, 1551-0018, 2822, 10.3934/mbe.2020156 | |
101. | Yuning Yang, Jiawen Yu, Zhe Liu, Xi Wang, Han Wang, Zhiqiang Ma, Dong Xu, An Improved Topology Prediction of Alpha-Helical Transmembrane Protein Based on Deep Multi-Scale Convolutional Neural Network, 2022, 19, 1545-5963, 295, 10.1109/TCBB.2020.3005813 | |
102. | Shihu Jiao, Zheng Chen, Lichao Zhang, Xun Zhou, Lei Shi, ATGPred-FL: sequence-based prediction of autophagy proteins with feature representation learning, 2022, 54, 0939-4451, 799, 10.1007/s00726-022-03145-5 | |
103. | Changli Feng, Haiyan Wei, Deyun Yang, Bin Feng, Zhaogui Ma, Shuguang Han, Quan Zou, Hua Shi, ORS‐Pred: An optimized reduced scheme‐based identifier for antioxidant proteins, 2021, 21, 1615-9853, 2100017, 10.1002/pmic.202100017 | |
104. | Hongliang Zou, Zhijian Yin, m7G-DPP: Identifying N7-methylguanosine sites based on dinucleotide physicochemical properties of RNA, 2021, 279, 03014622, 106697, 10.1016/j.bpc.2021.106697 | |
105. | Yuxin Gong, Bo Liao, Dejun Peng, Quan Zou, Accurate Prediction and Key Feature Recognition of Immunoglobulin, 2021, 11, 2076-3417, 6894, 10.3390/app11156894 | |
106. | Yuxin Guo, Liping Hou, Wen Zhu, Peng Wang, Prediction of Hormone-Binding Proteins Based on K-mer Feature Representation and Naive Bayes, 2021, 12, 1664-8021, 10.3389/fgene.2021.797641 | |
107. | Lesong Wei, Xiucai Ye, Tetsuya Sakurai, Zengchao Mu, Leyi Wei, Pier Luigi Martelli, ToxIBTL: prediction of peptide toxicity based on information bottleneck and transfer learning, 2022, 38, 1367-4803, 1514, 10.1093/bioinformatics/btac006 | |
108. | Yihang Bao, Weixi Wang, Minglong Dong, Fei He, Han Wang, 2021, Discover the Binding Domain of Transmembrane Proteins Based on Structural Universality, 978-1-6654-0126-5, 5, 10.1109/BIBM52615.2021.9669493 | |
109. | Wen Zhu, Yuxin Guo, Quan Zou, Prediction of presynaptic and postsynaptic neurotoxins based on feature extraction, 2021, 18, 1551-0018, 5943, 10.3934/mbe.2021297 | |
110. | Hasan Zulfiqar, Shi-Shi Yuan, Qin-Lai Huang, Zi-Jie Sun, Fu-Ying Dao, Xiao-Long Yu, Hao Lin, Identification of cyclin protein using gradient boost decision tree algorithm, 2021, 19, 20010370, 4123, 10.1016/j.csbj.2021.07.013 | |
111. | Jun Zhang, Qingcai Chen, Bin Liu, DeepDRBP-2L: A New Genome Annotation Predictor for Identifying DNA-Binding Proteins and RNA-Binding Proteins Using Convolutional Neural Network and Long Short-Term Memory, 2021, 18, 1545-5963, 1451, 10.1109/TCBB.2019.2952338 | |
112. | Pengmian Feng, Lijing Feng, Recent Advances on Antioxidant Identification Based on Machine Learning Methods, 2020, 21, 13892002, 804, 10.2174/1389200221666200719001449 | |
113. | Xiaoping Min, Fengqing Lu, Chunyan Li, Sequence-Based Deep Learning Frameworks on Enhancer-Promoter Interactions Prediction, 2021, 27, 13816128, 1847, 10.2174/1381612826666201124112710 | |
114. | Yu-Hao Wang, Yu-Fei Zhang, Ying Zhang, Zhi-Feng Gu, Zhao-Yue Zhang, Hao Lin, Ke-Jun Deng, Identification of adaptor proteins using the ANOVA feature selection technique, 2022, 208, 10462023, 42, 10.1016/j.ymeth.2022.10.008 | |
115. | Shihu Jiao, Quan Zou, Huannan Guo, Lei Shi, iTTCA-RF: a random forest predictor for tumor T cell antigens, 2021, 19, 1479-5876, 10.1186/s12967-021-03084-x | |
116. | Bosheng Song, Zimeng Li, Xuan Lin, Jianmin Wang, Tian Wang, Xiangzheng Fu, Pretraining model for biological sequence data, 2021, 20, 2041-2649, 181, 10.1093/bfgp/elab025 | |
117. | Peijie Zheng, Yue Qi, Xueyong Li, Yuewu Liu, Yuhua Yao, Guohua Huang, A capsule network-based method for identifying transcription factors, 2022, 13, 1664-302X, 10.3389/fmicb.2022.1048478 | |
118. | Hongliang Zou, iAHTP-LH: Integrating Low-Order and High-Order Correlation Information for Identifying Antihypertensive Peptides, 2022, 28, 1573-3904, 10.1007/s10989-022-10414-0 | |
119. | Hui Zhang, Qin Chen, Bing Niu, Risk Assessment of Veterinary Drug Residues in Meat Products, 2020, 21, 13892002, 779, 10.2174/1389200221999200820164650 | |
120. | Ke Yan, Jie Wen, Jin-Xing Liu, Yong Xu, Bin Liu, Protein Fold Recognition by Combining Support Vector Machines and Pairwise Sequence Similarity Scores, 2021, 18, 1545-5963, 2008, 10.1109/TCBB.2020.2966450 | |
121. | Hongliang Zou, Fan Yang, Zhijian Yin, Identification of tumor homing peptides by utilizing hybrid feature representation, 2022, 0739-1102, 1, 10.1080/07391102.2022.2049368 | |
122. | Hasan Zulfiqar, Zhiling Guo, Bakanina Kissanga Grace-Mercure, Zhao-Yue Zhang, Hui Gao, Hao Lin, Yun Wu, Empirical Comparison and Recent Advances of Computational Prediction of Hormone Binding Proteins Using Machine Learning Methods, 2023, 20010370, 10.1016/j.csbj.2023.03.024 | |
123. | Hongliang Zou, iHBPs-VWDC: variable-length window-based dynamic connectivity approach for identifying hormone-binding proteins, 2023, 0739-1102, 1, 10.1080/07391102.2023.2283150 | |
124. | Ali Ghulam, Zar Nawab Khan Swati, Farman Ali, Saima Tunio, Nida Jabeen, Natasha Iqbal, DeepImmuno-PSSM: Identification of Immunoglobulin based on Deep learning and PSSM-Profiles, 2023, 11, 2308-8168, 54, 10.21015/vtcs.v11i1.1396 | |
125. | A. Sherly Alphonse, N. Ani Brown Mary, Classification of anti-oxidant proteins using novel physiochemical and conjoint-quad (PCQ) feature composition, 2023, 83, 1573-7721, 48831, 10.1007/s11042-023-17498-w | |
126. | Zhi-Feng Gu, Yu-Duo Hao, Tian-Yu Wang, Pei-Ling Cai, Yang Zhang, Ke-Jun Deng, Hao Lin, Hao Lv, Prediction of blood–brain barrier penetrating peptides based on data augmentation with Augur, 2024, 22, 1741-7007, 10.1186/s12915-024-01883-4 | |
127. | Zhibin Lv, Mingxuan Wei, Hongdi Pei, Shiyu Peng, Mingxin Li, Liangzhen Jiang, PTSP-BERT: Predict the thermal stability of proteins using sequence-based bidirectional representations from transformer-embedded features, 2025, 185, 00104825, 109598, 10.1016/j.compbiomed.2024.109598 | |
128. | Ali Ghulam, Muhammad Arif, Ahsanullah Unar, Maha A. Thafar, Somayah Albaradei, Apilak Worachartcheewan, StackAHTPs: An explainable antihypertensive peptides identifier based on heterogeneous features and stacked learning approach, 2025, 19, 1751-8849, 10.1049/syb2.70002 | |
129. | Rui Li, Junwen Yu, Dongxin Ye, Shanghua Liu, Hongqi Zhang, Hao Lin, Juan Feng, Kejun Deng, Conotoxins: Classification, Prediction, and Future Directions in Bioinformatics, 2025, 17, 2072-6651, 78, 10.3390/toxins17020078 | |
130. | Firuz Kamalov, Hana Sulieman, Ayman Alzaatreh, Maher Emarly, Hasna Chamlal, Murodbek Safaraliev, Mathematical Methods in Feature Selection: A Review, 2025, 13, 2227-7390, 996, 10.3390/math13060996 |
Feature extraction | C | g | Sn(%) | Sp(%) | Acc(%) | Mcc | AUC |
CTD (21-D) | 2 | 23 | 36.59 | 83.74 | 60.16 | 0.230 | 0.654 |
NV (60-D) | 2-5 | 2-13 | 70.73 | 69.92 | 70.33 | 0.407 | 0.762 |
CTD+NV (81-D) | 29 | 2-7 | 70.73 | 63.41 | 67.07 | 0.342 | 0.709 |
Classifier | Sn (%) | Sp (%) | Acc (%) | Mcc | AUC |
J48 | 63.41 | 56.91 | 60.16 | 0.204 | 0.601 |
Bagging | 80.49 | 57.72 | 69.11 | 0.392 | 0.770 |
Random Forest | 88.62 | 84.55 | 86.59 | 0.732 | 0.945 |
Naive Bayes | 95.93 | 92.68 | 94.31 | 0.887 | 0.965 |
SVM | 96.75 | 97.56 | 97.15 | 0.943 | 0.994 |
Feature extraction | C | g | Sn(%) | Sp(%) | Acc(%) | Mcc | AUC |
CTD (21-D) | 2 | 23 | 36.59 | 83.74 | 60.16 | 0.230 | 0.654 |
NV (60-D) | 2-5 | 2-13 | 70.73 | 69.92 | 70.33 | 0.407 | 0.762 |
CTD+NV (81-D) | 29 | 2-7 | 70.73 | 63.41 | 67.07 | 0.342 | 0.709 |
Classifier | Sn (%) | Sp (%) | Acc (%) | Mcc | AUC |
J48 | 63.41 | 56.91 | 60.16 | 0.204 | 0.601 |
Bagging | 80.49 | 57.72 | 69.11 | 0.392 | 0.770 |
Random Forest | 88.62 | 84.55 | 86.59 | 0.732 | 0.945 |
Naive Bayes | 95.93 | 92.68 | 94.31 | 0.887 | 0.965 |
SVM | 96.75 | 97.56 | 97.15 | 0.943 | 0.994 |
Reference | Methods | Sn (%) | Sp (%) | Acc (%) | Mcc | AUC |
[7] | HBPred | 88.6 | 81.3 | 84.9 | - | - |
[8] | iGHBP | 88.62 | 81.30 | 84.96 | - | 0.701 |
This work | HBPred2.0 | 96.75 | 97.56 | 97.15 | 0.943 | 0.994 |
Reference | Methods | Sn (%) | Sp (%) | Acc (%) | Mcc | AUC |
[7] | HBPred | 80.43 | 56.52 | 68.48 | 0.381 | 0.714 |
[8] | iGHBP | 86.96 | 47.83 | 67.39 | 0.380 | - |
This work | HBPred2.0 | 89.13 | 80.43 | 84.78 | 0.698 | 0.814 |