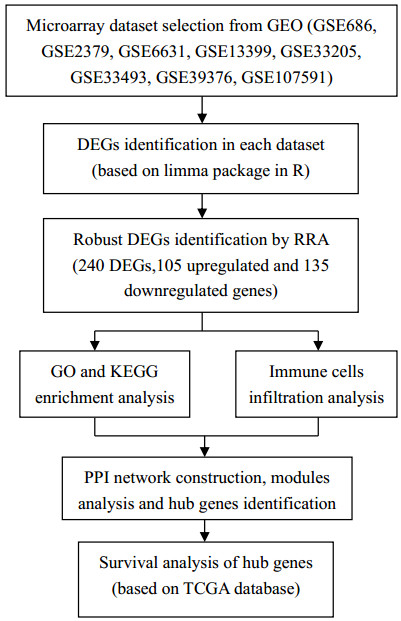
Although multiple hub genes have been identified in head and neck squamous cell cancer (HNSCC) in recent years, because of the limited sample size and inconsistent bioinformatics analysis methods, the results are not reliable. Therefore, it is urgent to use reliable algorithms to find new prognostic markers of HNSCC.
The Robust Rank Aggregation (RRA) method was used to integrate 8 microarray datasets of HNSCC downloaded from the Gene Expression Omnibus (GEO) database to screen differentially expressed genes (DEGs). Later, Gene Ontology (GO) functional annotation together with Kyoto Encyclopedia of Genes and Genomes (KEGG) analysis was carried out to discover functions of those discovered DEGs. According to the KEGG results, those discovered DEGs showed tight association with the occurrence and development of HNSCC. Then cibersort algorithm was used to analyze the infiltration of immune cells of HNSCC and we found that the main infiltrated immune cells were B cells, dendritic cells and macrophages. A protein-protein interaction (PPI) network was established; moreover, key modules were also constructed to select 5 hub genes from the whole network using cytoHubba. 3 hub genes showed significant relationship with prognosis for TCGA-derived HNSCC patients.
The potent DEGs along with hub genes were selected by the combined bioinformatic approach. AURKA, BIRC5 and UBE2C genes may be the potential prognostic biomarker and therapeutic targets of HNSCC.
The Robust Rank Aggregation method and cibersort algorithm method can accurately predict the potential prognostic biomarker and therapeutic targets of HNSCC through multiple GEO datasets.
Citation: Tingting Chen, Wei Hua, Bing Xu, Hui Chen, Minhao Xie, Xinchen Sun, Xiaolin Ge. Robust rank aggregation and cibersort algorithm applied to the identification of key genes in head and neck squamous cell cancer[J]. Mathematical Biosciences and Engineering, 2021, 18(4): 4491-4507. doi: 10.3934/mbe.2021228
[1] | Xuesi Chen, Qijun Zhang, Qin Zhang . Predicting potential biomarkers and immune infiltration characteristics in heart failure. Mathematical Biosciences and Engineering, 2022, 19(9): 8671-8688. doi: 10.3934/mbe.2022402 |
[2] | Fang Niu, Zongwei Liu, Peidong Liu, Hongrui Pan, Jiaxue Bi, Peng Li, Guangze Luo, Yonghui Chen, Xiaoxing Zhang, Xiangchen Dai . Identification of novel genetic biomarkers and treatment targets for arteriosclerosis-related abdominal aortic aneurysm using bioinformatic tools. Mathematical Biosciences and Engineering, 2021, 18(6): 9761-9774. doi: 10.3934/mbe.2021478 |
[3] | Shuo Sun, Xiaoni Cai, Jinhai Shao, Guimei Zhang, Shan Liu, Hongsheng Wang . Machine learning-based approach for efficient prediction of diagnosis, prognosis and lymph node metastasis of papillary thyroid carcinoma using adhesion signature selection. Mathematical Biosciences and Engineering, 2023, 20(12): 20599-20623. doi: 10.3934/mbe.2023911 |
[4] | Yuedan Wang, Jinke Huang, Jiaqi Zhang, Fengyun Wang, Xudong Tang . Identifying biomarkers associated with the diagnosis of ulcerative colitis via bioinformatics and machine learning. Mathematical Biosciences and Engineering, 2023, 20(6): 10741-10756. doi: 10.3934/mbe.2023476 |
[5] | Chenyang Jiang, Weidong Jiang . AGTR1, PLTP, and SCG2 associated with immune genes and immune cell infiltration in calcific aortic valve stenosis: analysis from integrated bioinformatics and machine learning. Mathematical Biosciences and Engineering, 2022, 19(4): 3787-3802. doi: 10.3934/mbe.2022174 |
[6] | Miao Zhu, Tao Yan, Shijie Zhu, Fan Weng, Kai Zhu, Chunsheng Wang, Changfa Guo . Identification and verification of FN1, P4HA1 and CREBBP as potential biomarkers in human atrial fibrillation. Mathematical Biosciences and Engineering, 2023, 20(4): 6947-6965. doi: 10.3934/mbe.2023300 |
[7] | Shengjue Xiao, Yufei Zhou, Ailin Liu, Qi Wu, Yue Hu, Jie Liu, Hong Zhu, Ting Yin, Defeng Pan . Uncovering potential novel biomarkers and immune infiltration characteristics in persistent atrial fibrillation using integrated bioinformatics analysis. Mathematical Biosciences and Engineering, 2021, 18(4): 4696-4712. doi: 10.3934/mbe.2021238 |
[8] | Linlin Tan, Dingzhuo Cheng, Jianbo Wen, Kefeng Huang, Qin Zhang . Identification of prognostic hypoxia-related genes signature on the tumor microenvironment in esophageal cancer. Mathematical Biosciences and Engineering, 2021, 18(6): 7743-7758. doi: 10.3934/mbe.2021384 |
[9] | Ahmed Hammad, Mohamed Elshaer, Xiuwen Tang . Identification of potential biomarkers with colorectal cancer based on bioinformatics analysis and machine learning. Mathematical Biosciences and Engineering, 2021, 18(6): 8997-9015. doi: 10.3934/mbe.2021443 |
[10] | Xin Yu, Jun Liu, Ruiwen Xie, Mengling Chang, Bichun Xu, Yangqing Zhu, Yuancai Xie, Shengli Yang . Construction of a prognostic model for lung squamous cell carcinoma based on seven N6-methylandenosine-related autophagy genes. Mathematical Biosciences and Engineering, 2021, 18(5): 6709-6723. doi: 10.3934/mbe.2021333 |
Although multiple hub genes have been identified in head and neck squamous cell cancer (HNSCC) in recent years, because of the limited sample size and inconsistent bioinformatics analysis methods, the results are not reliable. Therefore, it is urgent to use reliable algorithms to find new prognostic markers of HNSCC.
The Robust Rank Aggregation (RRA) method was used to integrate 8 microarray datasets of HNSCC downloaded from the Gene Expression Omnibus (GEO) database to screen differentially expressed genes (DEGs). Later, Gene Ontology (GO) functional annotation together with Kyoto Encyclopedia of Genes and Genomes (KEGG) analysis was carried out to discover functions of those discovered DEGs. According to the KEGG results, those discovered DEGs showed tight association with the occurrence and development of HNSCC. Then cibersort algorithm was used to analyze the infiltration of immune cells of HNSCC and we found that the main infiltrated immune cells were B cells, dendritic cells and macrophages. A protein-protein interaction (PPI) network was established; moreover, key modules were also constructed to select 5 hub genes from the whole network using cytoHubba. 3 hub genes showed significant relationship with prognosis for TCGA-derived HNSCC patients.
The potent DEGs along with hub genes were selected by the combined bioinformatic approach. AURKA, BIRC5 and UBE2C genes may be the potential prognostic biomarker and therapeutic targets of HNSCC.
The Robust Rank Aggregation method and cibersort algorithm method can accurately predict the potential prognostic biomarker and therapeutic targets of HNSCC through multiple GEO datasets.
Head and neck squamous cell cancer (HNSCC) ranks the 6th place among all cancers globally, which occurs in the oral cavity, nasal cavity, sinus, throat and pharynx. The pathological type of HNSCC is squamous cell carcinoma (except thyroid tumor). In America, the incidence of HNSCC was 7.863% in 2006 [1], in China, the incidence of HNSCC was up to 3.268% [2]. At present, the standard treatment of HNSCC is still surgery, intensity-modulated radiotherapy and platinum-based chemotherapy. However, there is no better treatment plan when first-line therapy is ineffective. Therefore, new treatment is urgently needed for HNSCC to prolong the survival time of patient [3]. In recent years, the emergence of immunotherapy has brought new hope to cancer patients. Nivolumab and Pembrolizumab are approved by the FDA for advanced HNSCC after first-line treatment [4,5]. However, the effectiveness of immunotherapy of HNSCC is still low, in this regard, searching for novel therapeutic targets to treat HNSCC is of great necessity.
With the development of computer science and bioinformatics, network-based methods have become an effective tool for the research of pathogenic mechanism [6]. Robust Rank Aggregation (RRA) method can maximally reduce errors or biases between multiple data sets [7]. Nowadays, RRA method has been widely used in cancer research [7,8,9]. This method is better than RemoveBatchEffect method, which was widely used on analyzing GEO datasets now.
The cibersort algorithm is a general calculation method to quantify cell components from a large number of tissues expression profiles (GEPs). Combined with support vector regression and prior knowledge from the expression profile of purified leukocyte subsets, cibersort algorithm can accurately estimate the immune components of tumor tissues.
The protein-protein interaction (PPI) network exerts a vital part in cancer biology, and it is an effective method for screening cancer related Hub genes. At present, some studies have pointed out that the method based on PPI network can successfully predict the Hub gene of breast cancer [10], liver cancer [11] and gastric cancer [12].
In this study, we downloaded the mircoarray datasets GSE686 [13], GSE2379 [14], GSE6631 [15], GSE13399 [16], GSE33205 [17,18], GSE33493, GSE39376, GSE107591 [19,20] were downloaded from the GEO database (www.ncbi.nlm.nih.gov/geo/). In addition, the RRA approach was used to discover the potent DEGs in normal compared with HNSCC samples. This study discovered altogether 240 potent DEGs, among which, 105 showed up-regulation and 135 showed down-regulation. Further, GO functional annotation and KEGG analyses were conducted to explore the functions of those identified DEGs. Cibersort algorithm was used to analyze the infiltration of immune cells. Then, a PPI network was established, meanwhile, the key modules were also built. At last, we screened 5 hub genes from the whole network using cytoHubba. Hub gene survival was analyzed by the R packages. To sum up, the potent DEGs along with hub genes were discovered in this study by the combined bioinformatic approach, which might be a new and potential prognostic biomarker.
We downloaded 8 head and neck squamous cell carcinoma chips from GEO databases, which contained GSE686 [13], GSE2379 [14], GSE6631 [15], GSE13399 [16], GSE33205 [17,18], GSE33493, GSE39376, GSE107591 [19,20]. These datasets covered both human normal and tumor samples, and each dataset contained at least 20 samples. We downloaded the matrix files of all the microarray along with the platform annotation profiles, so that the names of microarray probes were easily converted into genetic symbols by the use of Perl. We identified the normal tissue from the HNSCC tissues from the 8 datasets using the R package limma function by the thresholds of P-value < 0.05 and log2-fold change (FC) > 0.5.
The RRA method is a standard approach that can minimizes bias and errors between multiple datasets. It can detect genes whose ranking is consistently better than expected under null hypothesis of uncorrelated inputs, and assign a significance score to each gene, the specific algorithmic computations are shown by Eqs (1) and (2). The underlying probability model makes the algorithm parameter independent and robust to outliers, noise and errors. Significance sores also provide a rigorous way to keep only the statistically relevant genes in the final list.
In this study, in order to integrate eight microarray datasets, we used RRA method to determine the robust DEGs. Before RRA analysis, we sequenced the up-regulated and down-regulated were sequenced from each dataset according to FC, then the sequencing results of 8 datasets were combined, and the R package RobustRankAggreg function was used to select robust DEGs according to the above thresholds.
βk,n(x):=∑nℓ=k(nℓ)xℓ(1−x)n−ℓ | (1) |
ρ(r)=mink=1,..,nβk,n(r) | (2) |
For exploring functions of those selected DEGs, the R package "Clusterprofiler" was applied to obtain GO enrichment results, which included biological processes (BPs), cell components (CCs), together with molecular functions (MFs). and we also used the R package to analyzed the KEGG pathway of the robust DEGs. A difference of P < 0.05 indicated statistical significance.
The cibersort algorithm [21] has been developed as the machine learning approach on the basis of linear support vector regression (SVR), as shown by Eq (3), which shows high robustness against noise. This algorithm outperforms others in terms of noise, tightly associated cell types, along with unclear mixture content. This algorithm was used in the present work for predicting the infiltrating degrees of 22 immunocyte types within HNSCC tissues. LM22 is called immune cell gene expression tag matrix. It contained 547 genes and can distinguish 22 kinds of human hematopoietic cell phenotypes. The HNSCC data together with LM22 matrix were used to be the input of the above algorithm to obtain the proportions of those 22 immunocyte types within HNSCC. As a result, the cell composition related to HNSCC response was quantified. Using P < 0.05 as the standard to screen immune cell matrix, the relative expression of immune cells in normal compared with HNSCC samples was detected by R software package. Differences in normal compared with cancer tissues were determined by principal component analysis (PCA).
gSVM=sign(∑Nn=1αnYnZTnZ+b)=sign(∑Nn=1αnYnK(xn,x)+b) | (3) |
We uploaded the differentially expressed genes to string online database, and selected the confidence level > 0.7 as the screening criteria, and removed the free nodes to get the PPI (protein-protein interaction network), and downloaded the gene interaction files. The PPI network was visualized using Cytoscape (Version 3.6.1). In addition, the MCODE plug-in of Cytoscape was used to screen those significant modules from the as-constructed PPI network [22].
The Cytoscape plug-in CytoHubba can be used to sort those network-derived nodes based on network features. Cytohubba offers 11 topological analysis methods, such as edge penetration component, degree, maximum cluster centrality, maximum neighborhood component density, maximum neighborhood component, and six centralities (bottleneck, eccentricity, compactness, radius, intermediate degree and stress) based on the shortest path. Of these 11 approaches, the modified Maximal Clique Centrality (MCC) approach is more effective in predicting the essential proteins in PPI network.
We obtained RNA-seq and clinical data of HNSCC cases from The Cancer Genome Atlas (TCGA) database. Thereafter, hub gene survival was analyzed by survminer and survival functions of R package. A difference of P < 0.05 indicated statistical significance.
The bioinformatic approaches were utilized in the present work for identifying DEGs, and analyze the biological characteristics of these DEGs. We conducted this study according to the workflow shown in Figure 1.
The microarray data of HNSCC GSE686, GSE2379, GSE6631, GSE13399, GSE33205, GSE33493, GSE39376, GSE107591 were downloaded and analyzed by R package limma software. The distribution of DEGs is shown in the volcanic map (Figure 2). A total of 336 samples including 108 normal samples and 258 tumor samples were analyzed in our study. On the basis of the exclusion standard of log2FC > 1 and P < 0.05, there were 4 downregulated genes in GSE686. In GSE2379, there were 443 DEGs selected, among which, 238 showed up-regulation while 205 showed down-regulation. In the GSE6631 dataset, we selected 142 GSEs, among which, 53 showed up-regulation while 89 showed down-regulation. Altogether 322 DEGs were selected from the GSE13399 dataset, among which, 149 showed up-regulation while 173 showed down-regulation. There were 419 DEGs selected from the GSE33205 dataset, of them, 178 showed up-regulation while 241 showed down-regulation. Altogether 3330 DEGs were found from GSE33493, of them, 1773 showed up-regulation while 1557 showed down-regulation. Altogether 940 DEGs were selected from GSE39376, of them, 414 showed up-regulation while 526 showed down-regulation. In addition, altogether 466 DEGs were selected from GSE107591, of them, 200 showed up-regulation while 266 showed down-regulation. The volcano map for each GSE is shown in Figure 2, where the green and red dots indicate genes with down-regulation and up-regulation, separately.
We used the RRA method to integrate eight datasets. We selected altogether 240 DEGs, among which, 105 showed up-regulation while 135 showed down-regulation. According to the P-value threshold for selecting potent DEGs, the top 20 potent DEGs with up-regulation and down-regulation were distributed within the heat map.
Functions of those identified potent DEGs were analyzed by GO as well as KEGG analysis. Three function types were included in the GO analysis results: including BP, CC and MF. For BP, the robust DEGs showed enrichment in extracellular matrix (ECM) organization, skin development and extracellular organization. In the CC term, these genes were enriched in cornified envelope, collagen trimer complex as well as collagen-containing ECM. And in MF sections, the most significantly enriched terms were activity of serine-type endopeptidase, activity of serine-type peptidase, along with activity of serine hydrolase. The Figure 3 also showed the KEGG pathway enrichment analysis. Among them, IL-17 signaling pathway, cell cycle and ECM-receptor interaction are highly related to tumor growth and progress.
Using cibersort algorithm, the barplot of immune cells between normal and tumor samples in HNSCC tissues was shown in Figure 4(A). The heapmap of immune cells between normal and tumor samples in HNSCC tissues was shown in Figure 4(B). From the visualized violin plot (Figure 4C), we could find that B cells memory, B cells naïve, Macrophages M0, M1, T cells CD4 memory activated, Dendritic cells activated and Dendritic cells resting showed significant differences in normal compared with cancer tissues. PCA revealed no difference in normal compared with cancer tissues (Figure 4D).
For better exploring the associations among the potent DEGs, the String database was used to establish the PPI network. The Cytoscape was used to establish the visual PPI network when there were hidden disconnected nodes with the confidence value of > 0.7 (Figure 5A). The final network contained 125 edges along with 115 nodes, and there were 85 upregulated genes and 67 downregulated genes. We selected three key networks from the whole network through MCODE plugin (Figure 5B–D).
CytoHubba was applied for predicting and exploring the key nodes from the as-constructed PPI network. It can score each node in PPI network with topological algorithms. The genes with high scores are identified to be the Hub genes. The present work adopted ten topological algorithms ((Density of Maximum Neighhorhood Component (DMNC), Maximal Clique Centrality (MCC), Degrees, Maximum Neighborhood Component (MNC), BottleNeck, Edge Percolated Component (EPC), Closeness, EcCentricity, Betweenness and Radiality) to determine genes in the entire network. After calculation, there were 5 hub genes selected by the 10 algorithms, there were BIRC5, AURKA, UBE2C, CDC20, COL4A1.
Gene | Full name | Synonyms | Function |
AURKA | Aurora Kinase A | Activation of CDK1 | |
BIRC5 | Baculoviral IAP Repeat Containing 5 | survivin | Regulation of death receptor signaling and TNF signaling |
CDC20 | Cell Division Cycle 20 | Regulation of activated PAK-2p34 by proteasome mediated degradation; APC-CDC 20 mediated degradation of Nek2A | |
COL4A1 | Collagen Type IV Alpha 1 Chain | Focal Adhesion; miRNA targets in ECM and membrane receptors; Overview of nanoparticle effects; Spinal Cord Injury | |
UBE2C | Ubiquitin Conjugating Enzyme E2 C | Ubiquitin-Proteasome Dependent Proteolysis |
We analyzed the relationship between 5 hub genes and the overall survival rate of patients with HNSCC using "survminer" and "survival" packages of R software. Based on the best cutoff value calculated by the "surv_cutpoint" function for all Hub genes, we classified HNSCC samples as 2 groups (namely, high or low expression group), then acquired the respective Kaplan Meier (K-M) survival curves. As a result, BIRC5, AURKA (P = 0.017), UBE2C (P = 0.015) and (P = 0.045) expression was markedly related to the HNSCC survival.
As a result of the development of bioinformatics, there are more and more studies on HNSCC biomarkers in public databases such as GEO and TCGA database. For example, Ding Y et al. [23] and Chamorro Petronacci CM et al. [24] screened potential prognosis biomarkers of HNSCC. Nevertheless, due to the fact that the DEGs in the above research were selected using one dataset only with a small sample size, the results are unstable. In our study, compared with other HNSCC researches, we conformitied eight datasets by RRA methods.
In this study, we selected altogether 240 DEGs, including 105 with up-regulation while 135 with down-regulation. As suggested by GO as well as KEGG enrichment analysis, those potent DEGs were mainly enriched to extracellular matrix organization, skin development, extracellular organization, cornified envelope, complex of collagen trimers, serine-type activity and IL-17 signaling pathway, cell cycle and ECM-receptor interaction, which were related to tumor growth and progress. Then, we performed analysis of the immune cell infiltration between the normal and tumor HNSCC samples by cibersort algorithm. Module analysis was adopted to construct a PPI network based on the STRING database. Finally, 5 hub genes were screened from the whole network by cytoHubba including BIRC5, AURKA, UBE2C, CDC20, COL4A1. And the sample survival was analyzed using the R packages according to hub gene expression.
We also examined the GO terms together with KEGG pathways within the HNSCC samples in enrichment analysis. It has been extensively suggested that, the epithelial-mesenchymal transition (EMT) indicates a metastatic process. In this process, the epithelial cells acquire migratory and invasive mesenchymal phenotypes [25]. Extracellular matrix also exerts a vital part during cancer development, which can regulate cell growth, metabolism, migration, proliferation and differentiation through integrin or other cell surface receptors. Therefore, the degradation of ECM is indispensable for the invasion and metastasis of malignant tumors [26,27]. The high IL-17B level is verified to be tightly associated with dismal prognostic outcomes for cancer patients, such as breast cancer [28,29], gastric cancer [30], colon cancer [31], lung cancer [32] and so on. The IL-17 pathway may also be involved in the development of HNSCC. DNA damage may occur during normal cell division or in the presence of external stimuli. If the repair mechanism of DNA damage is defective, genomic instability can be caused. Cell cycle disorder is an important part of genomic instability, which may promote the further malignant transformation of unstable cells, thus accelerating the occurrence and development of tumors [33,34]. In line with the analysis above, the potent DEGs were tightly related to the HNSCC pathogenic mechanism as well as progression.
An increasing number of recent articles suggest that, tumor microenvironment (TME) exerts a vital part in tumor genesis and progression [35,36]. Typically, TME mainly includes endothelial cells, mesenchymal cells, immune cells, and ECM [37]. From previous studies, we found that the B7 protein family contains seven members: CD80, CD86, ICOS-L, PD-L1, PD-L2, B7-H3 and B7-H4 [38]. All ligands of the B7 family can be detected in dendritic cells, B cells, together with macrophages. To be specific, the B7 family mainly plays a role in regulating immune response. If B7 family gene is knocked out, the mice will suffer from immune deficiency and autoimmune disease [39]. PD-L1 can lead to tumor escape in the immune system by weakening the specific response of T cells to tumor cells [40]. Based on two clinical trials of PD-1 and PD-L1 in HNSCC (KEYNOTE-012 and CheckMate-141), Nivolumab and Pembrolizumab are approved by the FDA to treat advanced HNSCC. In our study, we found that B cells, dendritic cells and macrophages were the principal infiltrating immune cells in HNSCC tissues. The immunotherapy for HNSCC needs to be further explored.
In our study, we discovered 5 hub genes according to the as-constructed PPI network. Among them, 3 key genes were screened for further exploration. AURKA is one of the mitotic serine/threonine kinase family members, which exerts a vital part in a variety of biological events, such as centrosome separation and maturation, chromosome alignment, spindle assembly, as well as G2-to-M transition [41,42]. Besides, AURKA is previously suggested to show over-expression in diverse cancers [43,44,45,46,47], such as neuroblastoma [48], gastric cancer [49,50,51] and so on. AURKA can promote tumor development by inhibiting tumor suppressor genes such as p73 [52,53] and p53 [54], activating β-catenin [55], NF-κB [56] and cap-dependent translation of oncogenes [57]. Nonetheless, it remains unknown about the function of AURKA within HNSCC. BIRC5, which is also referred to as survivin, belongs to the IAP family [58]. Its expression can be detected in different cancers, including breast cancer (BC) [59], colorectal cancer (CRC) [60], liver cancer [61] and so on. It inhibited the Caspase activity and apoptosis by inhibiting the binding of Caspase-3 and Caspase-7, thus leading to the survival of cancer cells in the process of tumorigenesis [62]. UBE2C belongs to the E2 ubiquitin-conjugating enzyme family [63], which exerts an important part in regulating the cell cycle, and this is achieved through the catalysis of polyubiquitination-induced APC/C substrate degradation [64]. Many recent studies report the abnormal expression of UBE2C in different human cancers, like hepatocellular carcinoma (HCC) [65], CRC [66,67], lung cancer [68], BC [69] and so on.
These abundant evidences showed that UBE2C played an important part in tumor genesis and development. According to our results, the high expression of AURKA, BIRC5 and UBE2C genes in HNSCC tumor showed poor survival of patients in TCGA database, suggesting that these genes are related to the prognosis of HNSCC, and they may act as therapeutic targets of HNSCC.
In conclusion, through Robust Rank Aggregation method and cibersort algorithm method, a series of robust DEFs and gene modules were identified in HNSCC. The identified genes were subjected to functional analyses, which revealed their close relationship with the occurrence and development of HNSCC. We not only screened five hub genes, but also analyzed the immune cell infiltration in HNSCC. From above discussion, we found that AURKA, BIRC5 and UBE2C may be considered as new biomarker and therapeutic targets of HNSCC. The Robust Rank Aggregation method and cibersort algorithm method can accurately predict the potential prognostic biomarker and therapeutic targets. Further investigation is warranted to explore their roles in HNSCC in further research.
This work was supported by grants from the National Natural Science Foundation of China (82073344, 81874217, 81703027, 81703028, 81672983).
The authors declare that there is no conflict of interests.
[1] | R. L. Siegel, K. D. Miller, A. Jemal, Cancer statistics, CA Cancer J. Clin., 66 (2016), 7-30. |
[2] | W. Chen, R. Zheng, P. D. Baade, S. Zhang, H. Zeng, F. Bray, et al., Cancer statistics in China, CA Cancer J. Clin., 66 (2016), 115-132. |
[3] |
A. G. Sacco, E. E. Cohen, Current treatment options for recurrent or metastatic head and neck squamous cell carcinoma, J. Clin. Oncol., 33 (2015), 3305-3313. doi: 10.1200/JCO.2015.62.0963
![]() |
[4] | T. Y. Seiwert, B. Burtness, R. Mehra, J. Weiss, R. Berger, J. P. Eder, et al., Safety and clinical activity of pembrolizumab for treatment of recurrent or metastatic squamous cell carcinoma of the head and neck (KEYNOTE-012): an open-label, multicentre, phase 1b trial, Lancet Oncol., 17 (2016), 956-965. |
[5] | R. L. Ferris, G. Blumenschein, J. Fayette, J. Guigay, A. D. Colevas, L. Licitra, et al., Nivolumab for recurrent squamous-cell carcinoma of the head and neck, N. Engl. J. Med., 375 (2016), 1856-1867. |
[6] | Y. Li, X. Q. Tang, Z. Bai, X. Dai, Exploring the intrinsic differences among breast tumor subtypes defined using immunohistochemistry markers based on the decision tree, Sci. Rep., 6 (2016), 35773. |
[7] |
R. Kolde, S. Laur, P. Adler, J. Vilo, Robust rank aggregation for gene list integration and meta-analysis, Bioinformatics, 28 (2012), 573-580. doi: 10.1093/bioinformatics/bts520
![]() |
[8] | Z. Y. Song, F. Chao, Z. Zhuo, Z. Ma, W. Li, G. Chen, Identification of hub genes in prostate cancer using robust rank aggregation and weighted gene co-expression network analysis, Aging (Albany N. Y.), 11 (2019), 4736-4756. |
[9] | Y. Yang, Q. Lu, X. Shao, B. Mo, X. Nie, W. Liu, et al., Development of a three-gene prognostic signature for hepatitis B virus associated hepatocellular carcinoma based on integrated transcriptomic analysis, J. Cancer, 9 (2018), 1989-2002. |
[10] |
D. Y. Zhuang, L. Jiang, Q. Q. He, P. Zhou, T. Yue, Identification of hub subnetwork based on topological features of genes in breast cancer, Int. J. Mol. Med., 35 (2015), 664-674. doi: 10.3892/ijmm.2014.2057
![]() |
[11] | B. Jin, W. Wang, G. Du, G. Z. Huang, L. T. Han, Z. Y. Tang, Identifying hub genes and dysregulated pathways in hepatocellular carcinoma, Eur. Rev. Med. Pharmacol. Sci., 19 (2015), 592-601. |
[12] | W. Chang, L. Ma, L. Lin, L. Gu, X. Liu, H. Cai, et al., Identification of novel hub genes associated with liver metastasis of gastric cancer, Int. J. Cancer, 125 (2009), 2844-2853. |
[13] | C. H. Chung, J. S. Parker, G. Karaca, J. Wu, W. K. Funkhouser, D. Moore, et al., Molecular classification of head and neck squamous cell carcinomas using patterns of gene expression, Cancer Cell, 5 (2004), 489-500. |
[14] | A. Cromer, A. Carles, R. Millon, G. Ganguli, F. Chalmel, F. Lemaire, et al., Identification of genes associated with tumorigenesis and metastatic potential of hypopharyngeal cancer by microarray analysis, Oncogene, 23 (2004), 2484-2498. |
[15] | M. A. Kuriakose, W. T. Chen, Z. M. He, A. G. Sikora, P. Zhang, Z. Y. Zhang, et al., Selection and validation of differentially expressed genes in head and neck cancer, Cell. Mol. Life Sci., 61 (2004), 1372-1383. |
[16] | C. R. Cabanski, Y. Qi, X. Yin, E. Bair, M. C. Hayward, C. Fan, et al., Swiss made: Standardized within class sum of squares to evaluate methodologies and dataset elements, PLoS One, 5 (2010), e9905. |
[17] | W. Sun, D. A. Gaykalova, M. F. Ochs, E. Mambo, D. Arnaoutakis, Y. Liu, et al., Activation of the NOTCH pathway in head and neck cancer, Cancer Res., 74 (2014), 1091-1104. |
[18] | J. C. Stansfield, M. Rusay, R. Shan, C. Kelton, D. A. Gaykalova, E. J. Fertig, et al., Toward signaling-driven biomarkers immune to normal tissue contamination, Cancer Inform., 15 (2016), 15-21. |
[19] | L. Verduci, M. Ferraiuolo, A. Sacconi, F. Ganci, J. Vitale, T. Colombo, et al., The oncogenic role of circPVT1 in head and neck squamous cell carcinoma is mediated through the mutant p53/YAP/TEAD transcription-competent complex, Genome Biol., 18 (2017), 237. |
[20] | A. Sacconi, S. Donzelli, C. Pulito, S. Ferrero, F. Spinella, A. Morrone, et al., TMPRSS2, a SARS-CoV-2 internalization protease is downregulated in head and neck cancer patients, J. Exp. Clin. Cancer Res., 39 (2020), 200. |
[21] | A. M. Newman, C. L. Liu, M. R. Green, A. J. Gentles, W. Feng, Y. Xu, et al., Robust enumeration of cell subsets from tissue expression profiles, Nat. Methods, 12 (2015), 453-457. |
[22] | C. H. Chin, S. H. Chen, H. H. Wu, C. W. Ho, M. T. Ko, C. Y. Lin, cytoHubba: identifying hub objects and sub-networks from complex interactome, BMC Syst. Biol., 8 (2014), S11. |
[23] | Y. Ding, M. Li, T. Tayier, L. Chen, S. Feng, Identification of novel prognostic biomarkers in head and neck squamous cell carcinoma using bioinformatics analysis, Comb. Chem. High Throughput Screen., 2020. |
[24] | C. M. C. Petronacci, A. G. Garcia, E. P. Iruegas, B. R. Mundina, A. I. L. Pouso, M. P. Sayans, Identification of prognosis associated microRNAs in HNSCC subtypes based on TCGA dataset, Medicina (Kaunas), 56 (2020). |
[25] |
J. P. Thiery, H. Acloque, R. Y. Huang, M. A. Nieto, Epithelial-mesenchymal transitions in development and disease, Cell, 139 (2009), 871-890. doi: 10.1016/j.cell.2009.11.007
![]() |
[26] |
K. Kessenbrock, V. Plaks, Z. Werb, Matrix metalloproteinases: regulators of the tumor microenvironment, Cell, 141 (2010), 52-67. doi: 10.1016/j.cell.2010.03.015
![]() |
[27] |
P. Lu, V. M. Weaver, Z. Werb, The extracellular matrix: a dynamic niche in cancer progression, J. Cell Biol., 196 (2012), 395-406. doi: 10.1083/jcb.201102147
![]() |
[28] | S. Furuta, Y. M. Jeng, L. Zhou, L. Huang, I. Kuhn, M. J. Bissell, et al., IL-25 causes apoptosis of IL-25R-expressing breast cancer cells without toxicity to nonmalignant cells, Sci. Transl. Med., 3 (2011), 7831. |
[29] | C. K. Huang, C. Y. Yang, Y. M. Jeng, C. L. Chen, H. H. Wu, Y. C. Chang, et al., Autocrine/paracrine mechanism of interleukin-17B receptor promotes breast tumorigenesis through NF-kappaB-mediated antiapoptotic pathway, Oncogene, 33 (2014), 2968-2977. |
[30] | Q. Bie, P. Zhang, Z. Su, D. Zheng, X. Ying, Y. Wu, et al., Polarization of ILC2s in peripheral blood might contribute to immunosuppressive microenvironment in patients with gastric cancer, J. Immunol. Res., 2014 (2014), 923135. |
[31] | A. Al-Samadi, S. Moossavi, A. Salem, M. Sotoudeh, S. M. Tuovinen, Y. T. Konttinen, et al., Distinctive expression pattern of interleukin-17 cytokine family members in colorectal cancer, Tumour. Biol., 37 (2016), 1609-1615. |
[32] | Y. F. Yang, Y. C. Lee, S. Lo, Y. N. Chung, Y. C. Hsieh, W. C. Chiu, et al., A positive feedback loop of IL-17B-IL-17RB activates ERK/beta-catenin to promote lung cancer metastasis, Cancer Lett., 422 (2018), 44-55. |
[33] |
B. B. Zhou, S. J. Elledge, The DNA damage response: putting checkpoints in perspective, Nature, 408 (2000), 433-439. doi: 10.1038/35044005
![]() |
[34] |
J. H. Hoeijmakers, Genome maintenance mechanisms for preventing cancer, Nature, 411 (2001), 366-374. doi: 10.1038/35077232
![]() |
[35] |
Y. Naito, Y. Yoshioka, Y. Yamamoto, T. Ochiya, How cancer cells dictate their microenvironment: present roles of extracellular vesicles, Cell. Mol. Life Sci., 74 (2017), 697-713. doi: 10.1007/s00018-016-2346-3
![]() |
[36] |
T. L. Whiteside, Exosome and mesenchymal stem cell cross-talk in the tumor microenvironment, Semin. Immunol., 35 (2018), 69-79. doi: 10.1016/j.smim.2017.12.003
![]() |
[37] |
M. De Palma, D. Biziato, T. V. Petrova, Microenvironmental regulation of tumour angiogenesis, Nat. Rev. Cancer, 17 (2017), 457-474. doi: 10.1038/nrc.2017.51
![]() |
[38] |
J. D. Hansen, L. D. Pasquier, M. P. Lefranc, V. Lopez, A. Benmansour, P. Boudinot, The B7 family of immunoregulatory receptors: a comparative and evolutionary perspective, Mol. Immunol., 46 (2009), 457-472. doi: 10.1016/j.molimm.2008.10.007
![]() |
[39] | M. Janakiram, U. A. Shah, W. Liu, A. Zhao, M. P. Schoenberg, X. Zang, The third group of the B7-CD28 immune checkpoint family: HHLA2, TMIGD2, B7x, and B7-H3, Immunol. Rev., 276 (2017), 26-39. |
[40] |
L. Chen, T. Azuma, W. Yu, X. Zheng, L. Luo, L. Chen, B7-H1 maintains the polyclonal T cell response by protecting dendritic cells from cytotoxic T lymphocyte destruction, Proc. Natl. Acad. Sci. U. S. A., 115 (2018), 3126-3131. doi: 10.1073/pnas.1722043115
![]() |
[41] |
S. Dutertre, S. Descamps, C. Prigent, On the role of aurora-A in centrosome function, Oncogene, 21 (2002), 6175-6183. doi: 10.1038/sj.onc.1205775
![]() |
[42] | T. Marumoto, D. Zhang, H. Saya, Aurora-A-a guardian of poles, Nat. Rev. Cancer, 5 (2005), 42-50. |
[43] | P. Cammareri, A. Scopelliti, M. Todaro, V. Eterno, F. Francescangeli, M. P. Moyer, et al., Aurora-a is essential for the tumorigenic capacity and chemoresistance of colorectal cancer stem cells, Cancer Res., 70 (2010), 4655-4665. |
[44] | T. Tanaka, M. Kimura, K. Matsunaga, D. Fukada, H. Mori, Y. Okano, Centrosomal kinase AIK1 is overexpressed in invasive ductal carcinoma of the breast, Cancer Res., 59 (1999), 2041-2044. |
[45] | D. Li, J. Zhu, P. F. Firozi, J. L. Abbruzzese, D. B. Evans, K. Cleary, et al., Overexpression of oncogenic STK15/BTAK/Aurora a kinase in human pancreatic cancer, Clin. Cancer Res., 9 (2003), 991-997. |
[46] | K. Kamada, Y. Yamada, T. Hirao, H. Fujimoto, Y. Takahama, M. Ueno, et al., Amplification/overexpression of Aurora-A in human gastric carcinoma: potential role in differentiated type gastric carcinogenesis, Oncol. Rep., 12 (2004), 593-599. |
[47] | S. B. Yang, X. B. Zhou, H. X. Zhu, L. P. Quan, J. F. Bai, J. He, et al., Amplification and overexpression of Aurora-A in esophageal squamous cell carcinoma, Oncol. Rep., 17 (2007), 1083-1088. |
[48] | Y. Yang, L. Ding, Q. Zhou, L. Fen, Y. Cao, J. Sun, et al., Silencing of AURKA augments the antitumor efficacy of the AURKA inhibitor MLN8237 on neuroblastoma cells, Cancer Cell Int., 20 (2020), 9. |
[49] |
A. Mesic, M. Rogar, P. Hudler, R. Juvan, R. Komel, Association of the AURKA and AURKC gene polymorphisms with an increased risk of gastric cancer, IUBMB Life, 68 (2016), 634-644. doi: 10.1002/iub.1521
![]() |
[50] | X. Liu, Z. Li, Y. Song, R. Wang, L. Han, Q. Wang, et al., AURKA induces EMT by regulating histone modification through Wnt/beta-catenin and PI3K/Akt signaling pathway in gastric cancer, Oncotarget, 7 (2016), 33152-33164. |
[51] | M. Kamran, Z. J. Long, D. Xu, S. S. Lv, B. Liu, C. L. Wang, et al., Aurora kinase a regulates survivin stability through targeting FBXL7 in gastric cancer drug resistance and prognosis, Oncogenesis, 6 (2017), e298. |
[52] |
A. A. Dar, A. Belkhiri, J. Ecsedy, A. Zaika, W. El-Rifai, Aurora kinase a inhibition leads to p73-dependent apoptosis in p53-deficient cancer cells, Cancer Res., 68 (2008), 8998-9004. doi: 10.1158/0008-5472.CAN-08-2658
![]() |
[53] | H. Katayama, J. Wang, W. Treekitkarnmongkol, H. Kawai, K. Sasai, H. Zhang, et al., Aurora kinase-A inactivates DNA damage-induced apoptosis and spindle assembly checkpoint response functions of p73, Cancer Cell, 21 (2012), 196-211. |
[54] | V. Sehdev, A. Katsha, J. Arras, D. Peng, M. Soutto, J. Ecsedy, et al., HDM2 regulation by AURKA promotes cell survival in gastric cancer, Clin. Cancer Res., 20 (2014), 76-86. |
[55] |
A. A. Dar, A. Belkhiri, W. El-Rifai, The aurora kinase a regulates GSK-3beta in gastric cancer cells, Oncogene, 28 (2009), 866-875. doi: 10.1038/onc.2008.434
![]() |
[56] | A. Katsha, M. Soutto, V. Sehdev, D. Peng, M. K. Washington, M. B. Piazuelo, et al., Aurora kinase a promotes inflammation and tumorigenesis in mice and human gastric neoplasia, Gastroenterology, 145 (2013), 1312-1322. |
[57] |
B. S. Kochupurakkal, J. D. Iglehart, Identification of genes responsible for RelA-dependent proliferation arrest in human mammary epithelial cells conditionally expressing RelA, Genom. Data, 7 (2016), 92-93. doi: 10.1016/j.gdata.2015.11.022
![]() |
[58] | P. Gil-Kulik, A. Krzyzanowski, E. Dudzinska, J. Karwat, P. Chomik, M. Swistowska, et al., Potential involvement of BIRC5 in maintaining pluripotency and cell differentiation of human stem cells, Oxid. Med. Cell. Longev., 2019 (2019), 8727925. |
[59] | C. Wang, X. Zheng, C. Shen, Y. Shi, MicroRNA-203 suppresses cell proliferation and migration by targeting BIRC5 and LASP1 in human triple-negative breast cancer cells, J. Exp. Clin. Cancer Res., 31 (2012), 58. |
[60] | Q. Fu, J. Zhang, X. Xu, F. Qian, K. Feng, J. Ma, miR-203 is a predictive biomarker for colorectal cancer and its expression is associated with BIRC5, Tumour. Biol., 2016. |
[61] | L. Cao, C. Li, S. Shen, Y. Yan, W. Ji, J. Wang, et al., OCT4 increases BIRC5 and CCND1 expression and promotes cancer progression in hepatocellular carcinoma, BMC Cancer, 13 (2013), 82. |
[62] | I. Tamm, Y. Wang, E. Sausville, D. A. Scudiero, N. Vigna, T. Oltersdorf, et al., IAP-family protein survivin inhibits caspase activity and apoptosis induced by fas (CD95), bax, caspases, and anticancer drugs, Cancer Res., 58 (1998), 5315-5320. |
[63] |
Y. David, T. Ziv, A. Admon, A. Navon, The E2 ubiquitin-conjugating enzymes direct polyubiquitination to preferred lysines, J. Biol. Chem., 285 (2010), 8595-8604. doi: 10.1074/jbc.M109.089003
![]() |
[64] |
Z. Ping, R. Lim, T. Bashir, M. Pagano, D. Guardavaccaro, APC/C (Cdh1) controls the proteasome-mediated degradation of E2F3 during cell cycle exit, Cell Cycle, 11 (2012), 1999-2005. doi: 10.4161/cc.20402
![]() |
[65] | K. Ieta, E. Ojima, F. Tanaka, Y. Nakamura, N. Haraguchi, K. Mimori, et al., Identification of overexpressed genes in hepatocellular carcinoma, with special reference to ubiquitin-conjugating enzyme E2C gene expression, Int. J. Cancer, 121 (2007), 33-38. |
[66] | Y. Takahashi, Y. Ishii, Y. Nishida, M. Ikarashi, T. Nagata, T. Nakamura, et al., Detection of aberrations of ubiquitin-conjugating enzyme E2C gene (UBE2C) in advanced colon cancer with liver metastases by DNA microarray and two-color FISH, Cancer Genet. Cytogenet., 168 (2006), 30-35. |
[67] | S. Z. Li, Y. Song, H. H. Zhang, B. X. Jin, Y. Liu, W. B. Liu, et al., UbcH10 overexpression increases carcinogenesis and blocks ALLN susceptibility in colorectal cancer, Sci. Rep., 4 (2014), 6910. |
[68] | Z. Zhang, P. Liu, J. Wang, T. Gong, F. Zhang, J. Ma, et al., Ubiquitin-conjugating enzyme E2C regulates apoptosis-dependent tumor progression of non-small cell lung cancer via ERK pathway, Med. Oncol., 32 (2015), 149. |
[69] |
Q. Han, C. Zhou, F. Liu, G. Xu, R. Zheng, X. Zhang, MicroRNA-196a post-transcriptionally upregulates the UBE2C proto-oncogene and promotes cell proliferation in breast cancer, Oncol. Rep., 34 (2015), 877-883. doi: 10.3892/or.2015.4049
![]() |
1. | Yu Xiao, Huan Zhu, Dongmei Chen, Ye Deng, Jun Wu, Measuring robustness in rank aggregation based on the error-effectiveness curve, 2023, 60, 03064573, 103355, 10.1016/j.ipm.2023.103355 | |
2. | Natalia Alonso-Moreda, Alberto Berral-González, Enrique De La Rosa, Oscar González-Velasco, José Manuel Sánchez-Santos, Javier De Las Rivas, Comparative Analysis of Cell Mixtures Deconvolution and Gene Signatures Generated for Blood, Immune and Cancer Cells, 2023, 24, 1422-0067, 10765, 10.3390/ijms241310765 | |
3. | Anuraag S. Parikh, Yize Li, Angela Mazul, Victoria X. Yu, Wade Thorstad, Jason Rich, Randal C. Paniello, Salvatore M. Caruana, Scott H. Troob, Ryan S. Jackson, Patrik Pipkorn, Paul Zolkind, Zongtai Qi, Douglas Adkins, Li Ding, Sidharth V. Puram, Immune Cell Deconvolution Reveals Possible Association of γδ T Cells with Poor Survival in Head and Neck Squamous Cell Carcinoma, 2023, 15, 2072-6694, 4855, 10.3390/cancers15194855 |
Gene | Full name | Synonyms | Function |
AURKA | Aurora Kinase A | Activation of CDK1 | |
BIRC5 | Baculoviral IAP Repeat Containing 5 | survivin | Regulation of death receptor signaling and TNF signaling |
CDC20 | Cell Division Cycle 20 | Regulation of activated PAK-2p34 by proteasome mediated degradation; APC-CDC 20 mediated degradation of Nek2A | |
COL4A1 | Collagen Type IV Alpha 1 Chain | Focal Adhesion; miRNA targets in ECM and membrane receptors; Overview of nanoparticle effects; Spinal Cord Injury | |
UBE2C | Ubiquitin Conjugating Enzyme E2 C | Ubiquitin-Proteasome Dependent Proteolysis |
Gene | Full name | Synonyms | Function |
AURKA | Aurora Kinase A | Activation of CDK1 | |
BIRC5 | Baculoviral IAP Repeat Containing 5 | survivin | Regulation of death receptor signaling and TNF signaling |
CDC20 | Cell Division Cycle 20 | Regulation of activated PAK-2p34 by proteasome mediated degradation; APC-CDC 20 mediated degradation of Nek2A | |
COL4A1 | Collagen Type IV Alpha 1 Chain | Focal Adhesion; miRNA targets in ECM and membrane receptors; Overview of nanoparticle effects; Spinal Cord Injury | |
UBE2C | Ubiquitin Conjugating Enzyme E2 C | Ubiquitin-Proteasome Dependent Proteolysis |