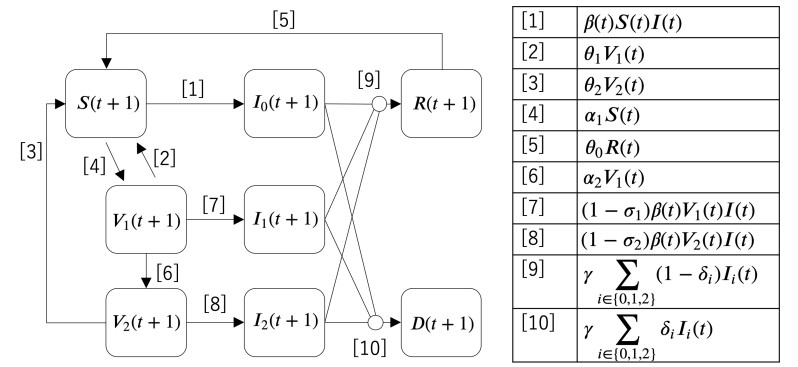
Citation: Fei Tang, Dabin Zhang, Xuehua Zhao. Efficiently deep learning for monitoring Ipomoea cairica (L.) sweets in the wild[J]. Mathematical Biosciences and Engineering, 2021, 18(2): 1121-1135. doi: 10.3934/mbe.2021060
[1] | Sarafa A. Iyaniwura, Rabiu Musa, Jude D. Kong . A generalized distributed delay model of COVID-19: An endemic model with immunity waning. Mathematical Biosciences and Engineering, 2023, 20(3): 5379-5412. doi: 10.3934/mbe.2023249 |
[2] | Tetsuro Kobayashi, Hiroshi Nishiura . Prioritizing COVID-19 vaccination. Part 2: Real-time comparison between single-dose and double-dose in Japan. Mathematical Biosciences and Engineering, 2022, 19(7): 7410-7424. doi: 10.3934/mbe.2022350 |
[3] | A. M. Elaiw, Raghad S. Alsulami, A. D. Hobiny . Global dynamics of IAV/SARS-CoV-2 coinfection model with eclipse phase and antibody immunity. Mathematical Biosciences and Engineering, 2023, 20(2): 3873-3917. doi: 10.3934/mbe.2023182 |
[4] | Tahir Khan, Roman Ullah, Gul Zaman, Jehad Alzabut . A mathematical model for the dynamics of SARS-CoV-2 virus using the Caputo-Fabrizio operator. Mathematical Biosciences and Engineering, 2021, 18(5): 6095-6116. doi: 10.3934/mbe.2021305 |
[5] | Biplab Dhar, Praveen Kumar Gupta, Mohammad Sajid . Solution of a dynamical memory effect COVID-19 infection system with leaky vaccination efficacy by non-singular kernel fractional derivatives. Mathematical Biosciences and Engineering, 2022, 19(5): 4341-4367. doi: 10.3934/mbe.2022201 |
[6] | Somayeh Fouladi, Mohammad Kohandel, Brydon Eastman . A comparison and calibration of integer and fractional-order models of COVID-19 with stratified public response. Mathematical Biosciences and Engineering, 2022, 19(12): 12792-12813. doi: 10.3934/mbe.2022597 |
[7] | A. D. Al Agha, A. M. Elaiw . Global dynamics of SARS-CoV-2/malaria model with antibody immune response. Mathematical Biosciences and Engineering, 2022, 19(8): 8380-8410. doi: 10.3934/mbe.2022390 |
[8] | Ayako Suzuki, Hiroshi Nishiura . Transmission dynamics of varicella before, during and after the COVID-19 pandemic in Japan: a modelling study. Mathematical Biosciences and Engineering, 2022, 19(6): 5998-6012. doi: 10.3934/mbe.2022280 |
[9] | Lixin Lin, Boqiang Chen, Yanji Zhao, Weiming Wang, Daihai He . Two waves of COIVD-19 in Brazilian cities and vaccination impact. Mathematical Biosciences and Engineering, 2022, 19(5): 4657-4671. doi: 10.3934/mbe.2022216 |
[10] | Salman Safdar, Calistus N. Ngonghala, Abba B. Gumel . Mathematical assessment of the role of waning and boosting immunity against the BA.1 Omicron variant in the United States. Mathematical Biosciences and Engineering, 2023, 20(1): 179-212. doi: 10.3934/mbe.2023009 |
As of August 2021, COVID-19 is still spreading in Japan and throughout the world. To overcome this infectious disease, various measures have been implemented (e.g., hand sanitization, mask-wearing, telework [1], quarantine at airports [2], and contact-tracing applications [3]). However, new variants, such as B.1.1.7 (alpha) [4] and B.1.617 (delta) [5] have appeared with even higher infectivity.
In complex situations that combine various conditions, it is important to perform simulations to determine the future infection spread. Before COVID-19 appeared, simulation studies of various viral infectious diseases were reported [6,7]. Moreover, COVID-19 simulations have been reported in various countries [8,9,10,11]. Simulations have been conducted to verify the efficacy of remote working [12], airport quarantines [13], stay-at-home orders [14], and contact-tracing applications [15]. Using the simulation approach, other studies [16,17] have analyzed the fatality curve of COVID-19 infected individuals. As we found in these studies, various complex situations can be surveyed using mathematical models.
To decrease the number of infected individuals, COVID-19 vaccinations (i.e., BNT162b (Pfizer) [18], mRNA-1273 (Moderna) [19], and ChAdOx1 (AstraZeneca) [20]) have been promulgated. In Japan, vaccination began in February 2021 [21], and second vaccinations had been given to 3×107 individuals as of August 2021 [22]. To determine the effect of vaccination, it is necessary to perform simulations using mathematical models. For example, there are some case studies that considered the effect of the vaccine in Malaysia [23], United States [24,25], Spain [26], and Saudi Arabia [27]. However, a case study focused only on Japan is lacking. Therefore, we fill this gap by performing a simulation to account for the effects of vaccination in Japan.
The susceptible–infected–recovered–vaccination (SIRV) model [28,29,30,31] and the susceptible–infected–recovered–vaccination–death (SIRVD) model [32] have been proposed to estimate the state of vaccinations worldwide. However, those models can only account for the first dose. The effects of the first and second vaccinations differ in the case of COVID-19 [33]. Because the previous models [28,29,30,31,32] cannot represent first and second vaccinations, we consider it difficult to appropriately verify the effects of multiple vaccinations. Therefore, we used the susceptible–infected–recovered–vaccination1–vaccination2–death (SIRVVD) model, which represents the first and second vaccinations for the simulation. In this section, we describe the SIRVVD model.
The set T of the simulation periods is
T={0,1,⋯,tend−1,tend}, | (2.1) |
where tend indicates the final day of the simulation. From the basic SIR model, time t is a real number. However, in the case of COVID-19, the Japanese government reports newly infected individuals every other day (one time per day). Therefore, we consider it desirable that time t takes the form of set T.
Herein, we define the number of infected individuals on day t∈T as
I(t)=I0(t)+I1(t)+I2(t), | (2.2) |
where, I0(t) is the number of infected individuals who do not receive the vaccination. I{1,2}(t) is the number of infected individuals who received the first and second vaccinations, respectively (despite being vaccinated and infected).
Then, we define the number of susceptible persons S(t+1), the number of infectors I{0,1,2}(t+1), the number of vaccinated persons V1(t+1) (first dose) and V2(t+1) (second dose), the number of recovered persons R(t+1), and the number of dead persons D(t+1), as
S(t+1)=S(t)−β(t)S(t)I(t)+θ1V1(t)+θ2V2(t)−α1S(t)+θ0R(t), | (2.3) |
V1(t+1)=V1(t)+α1S(t)−θ1V1(t)−α2V1(t)−(1−σ1)β(t)V1(t)I(t), | (2.4) |
V2(t+1)=V2(t)+α2V1(t)−(1−σ2)β(t)V2(t)I(t)−θ2V2(t), | (2.5) |
I0(t+1)=I0(t)+β(t)S(t)I(t)−γI0(t), | (2.6) |
I1(t+1)=I1(t)+(1−σ1)β(t)V1(t)I(t)−γI1(t), | (2.7) |
I2(t+1)=I2(t)+(1−σ2)β(t)V2(t)I(t)−γI2(t), | (2.8) |
R(t+1)=R(t)+γ∑i∈{0,1,2}(1−δi)Ii(t)−θ0R(t), | (2.9) |
D(t+1)=D(t)+γ∑i∈{0,1,2}δiIi(t). | (2.10) |
Variable β(t) is the infectivity on a day t. θ0 is the reduction rate of antibodies from a natural infection. θ{1,2} are the reduction rates of antibodies from the first and second vaccinations, respectively. α1 is the rate of the first dose of vaccination for susceptible persons, S(t). α2 is the rate of the second vaccination of persons who received the first dose of vaccine V1(t). σ{1,2} are the effectiveness of the first and second vaccinations for reducing the infection probability, respectively. γ is the removal rate of the infected individuals. δ0 is the fatality rate of the infected individuals who did not receive vaccination. δ{1,2} are the fatality rates of infected individuals who received the first and second vaccinations, respectively. Because we assume that the validity periods of vaccinations and antibodies from natural infection follow an exponential distribution, θ{0,1,2} are the inverse values of their average periods. Likewise, because we assume that the infection period follows an exponential distribution, γ is the inverse of the average infection period. In the case of the SIR model, some studies have adopted the inverse value of the average infection period as γ [34,35]. Moreover, the total population N is
N=S(t)+I(t)+V1(t)+V2(t)+R(t)+D(t),∀t∈T. | (2.11) |
We show the states transition model in Figure 1. Paths 1, 7, and 8 mean infection. Paths 4 and 6 mean vaccination. Paths 2 and 3 mean the effects of vaccine disappear by elapsed time. Paths 9 and 10 mean recovering and deaths, respectively. The models shown in Figure 1 and Equations (2.3)–(2.10) correspond to each other. Therefore, we can represent the transition of infection states considering the first and second vaccinations using this model.
It is desirable to consider that there is a limit to the number of vaccines available. Therefore, we calculate the amount of vaccinations by
N1(t+1)=N1(t)+α1S(t), | (2.12) |
N2(t+1)=N2(t)+α2V1(t), | (2.13) |
where N{1,2}(t+1) are the numbers of first and second vaccinations on day t+1, respectively.
Moreover, in the case of COVID-19, the number of newly infected individuals is reported every other day. In this model, the number of new infectors I+(t) on day t is
I+(t)=β(t)S(t)I(t)+(1−σ1)β(t)V1(t)I(t)+(1−σ2)β(t)V2(t)I(t). | (2.14) |
I+(t) is the summation of paths 1, 7, and 8.
In this subsection, we explain the proposed SIRVVD model. We carried out a mathematical analysis of the impact of the vaccination on Ij(t) as a supplement. This detail is shown in the Appendix section.
Herein, we present simple calculation examples to understand the features of the mathematical model by using parameters that are easy to understand. First, we explain the adopted parameters (note that the simulations below are fictitious locations and viruses). The total population N is 104 persons. The initial infectors I0(0) are 101 persons. Initial susceptible persons comprise S(0)=N−I0(0)=9,990 individuals. The initial numbers of other statuses are V{1,2}(0)=I{1,2}(0)=R(0)=D(0)=0. The end of the day of simulation tend is 100. The infectivity is β(t)=10−4.5,∀t∈T. The reduction rates of antibodies by vaccination or natural infection θ{0,1,2} are 10−2. The effectiveness of the first and second vaccinations is σ1=0.5 and σ2=0.9, respectively. The removal rate from infection is γ=0.15. The fatality rates are δ0=0.10, δ1=δ0×(1−0.40), and δ2=δ0×(1−0.80). This means that the reduction values of the fatality rate by the first and second vaccinations are 40% and 80%, respectively.
The above parameters are fixed values. In contrast, we set some values to α{1,2} to check the effectiveness of the vaccination. These are
(α1,α2)∈{(0,0),(0.01,0.01),(0.01,0.03),(0.03,0.01),(0.03,0.03),(0.05,0.05)}. | (2.15) |
The simulation results for I(t),R(t), and D(t) are shown in Figure 2. The more α{1,2} increases, the more I(t),R(t), and D(t) decreases, owing to the effectiveness of the vaccination. It was found that the spread of infection slowed with respect to the progress of vaccinations. This change can be understood by reading the discussion given in the Appendix. If we compare (α1,α2)=(0.01,0.03) and (0.03,0.01), the number of infected individuals of (0.03,0.01) is less than that of (0.01,0.03). α2 is the parameter for transition from V1(t) to V2(t). Therefore, if V1(t) is small, even if α2 is high, V2(t) is small. To increase V1(t), it is necessary to set the high value as α1. Therefore, if α1 is small, and α2 is high, such as (α1,α2)=(0.01,0.03), the effect of vaccination on reducing infected individuals is small.
The simulation results for S(t), V1(t), and V2(t) are shown in Figure 3. In all cases, S(t) decreases with the elapsed time. In cases where α1 is small, such as (α1,α2)=(0.01,0.01), and (0.01,0.03), V1(t) is also small because V1(t) and V2(t) are also small. The higher the α1, the higher the V1(t). In the case of (α1,α2)=(0.05,0.05), because the number of transitions from S(t) to V1(t) and from V1(t) to V2(t) is high, V2(t) is also high. Figures 2, 3 indicate that vaccination effectively reduces the number of infected individuals and deaths.
Herein, we compare SIRVD to the proposed SIRVVD model. If we substitute zero for α2, I2(0), and V2(0), which are the parameters related to the vaccine, V2(t) and I2(t) are
V2(t)=0,I2(t)=0,∀t∈T. | (2.16) |
When we substitute these values for the proposed SIRVVD model defined by Equations (2.3) – (2.10), the model can be expressed as
S(t+1)=S(t)−β(t)S(t)I(t)+θ1V1(t)−α1S(t)+θ0R(t), | (2.17) |
V1(t+1)=V1(t)+α1S(t)−θ1V1(t)−(1−σ1)β(t)V1(t)I(t), | (2.18) |
I0(t+1)=I0(t)+β(t)S(t)I(t)−γI0(t), | (2.19) |
I1(t+1)=I1(t)+(1−σ1)β(t)V1(t)I(t)−γI1(t), | (2.20) |
R(t+1)=R(t)+γ∑i∈{0,1}(1−δi)Ii(t)−θ0R(t), | (2.21) |
D(t+1)=D(t)+γ∑i∈{0,1}δiIi(t). | (2.22) |
The forms of Equations (2.17)–(2.22) are similar to the SIRV model to represent the first dose of vaccination, which was proposed by Ishikawa [30] (see Equations (1)–(4). Note that Ishikawa's model cannot represent the state of death caused by infection. To compare the SIRVD and SIRVVD models, we set α1=0.01,0.03 to the SIRVD model expressed by Equations (2.17) – (2.22) and performed simulations. The values of the other parameters are the same as those described in Subsection 2.2.
The maximum number of infected individuals MaxtI(t), and dead persons at the end of simulation D(tend) by the SIRVD model are shown in Table 1. To compare the SIRVD and SIRVVD models, we also show the results of the SIRVVD model (see Subsection 2.2 and Figure 2 for details). In all cases (α1=0.01,0.03), MaxtI(t) and D(tend) by the SIRVD model were higher than those of the SIRVVD model. This is because the SIRVD model cannot represent the effect of the second dose of vaccination. Because the effects of the first and second vaccinations are different [33] in the case of COVID-19, we consider that the proposed SIRVVD model expressed by Equations (2.3)–(2.10) is important.
Model | α1 | α2 | MaxtI(t) | D(tend) |
SIRVD model | 0.01 | - | 1,299 | 653 |
SIRVVD model | 0.01 | 0.01 | 1,204 | 630 |
SIRVVD model | 0.01 | 0.03 | 1,077 | 598 |
SIRVD model | 0.03 | - | 623 | 288 |
SIRVVD model | 0.03 | 0.01 | 438 | 229 |
SIRVVD model | 0.03 | 0.03 | 281 | 164 |
We performed simulations assumed in Japan using the model shown in Figure 1. The simulation period was from February 12, 2020 (t=0), to January 31, 2021 (t=tend=354 days). During this period, there were no vaccinations in Japan. Therefore, the objective of our simulations is to compare the presence and absence of vaccines in Japan. To do this, we used the open data of newly infected individuals reported by the Japanese government [36].
Kobayashi et al. [37] reported that the removal rate γ of COVID-19 in Japan ranges from 0.13 to 0.17. Therefore, we adopt γ=0.15. As mentioned in Section 2.1, we assume that the infection period follows an exponential distribution. In other words, γ−1 is the average infection period, which is γ−1=(0.15)−1=6.67 days. We define f(t;γ) as the exponential distribution of the infection period. In this case, we can estimate the probability of recovering or death F(x;γ) from 0 to x days by integrating f(t;γ) as follows:
F(x;γ)=∫x0f(t;γ)dt=∫x0γe−γtdt=1−e−γx. | (3.1) |
Note that we can only calculate the cases of γ>0. For example, F(14;6.67)=0.878 and F(20;6.67)=0.950, which indicates that approximately 88% and 95% of all infected individuals recovered or died within 14 and 20 days, respectively.
Because the total population in Japan is 1.2×108, we adopted N=1.2×108. The number of initial infected individuals I0(t=0) is the sum of infected individuals from 1 week before the start of the simulation. As a result, I0(t=0)=2 individuals. Because the simulation starting time is February 12th, 2020, this range is from February 5th, 2020, to February 11th, 2020. The infection was not widespread at the start of the simulation, and there were no vaccinations. Therefore, we set R(t=0),D(t=0),V1(t=0),V2(t=0),I1(t=0), and I2(t=0) as 0. Moreover, S(t=0)=N−I0(t=0).
If we assume that the effective period of the antibody by vaccination and natural infection follows an exponential distribution, θ−1{0,1,2} is the average effective period of the antibody. A previous study on the effective period of an antibody by Dan et al. [38] reported that the antibody of COVID-19-infected individuals varies between 6 and 8 months. Therefore, we assume the average effective period to be 7 months (7×30=210 days) and adopt θ{0,1,2}=1/210.
Dagan et al. [33] reported that by undergoing the first and second doses of the vaccine (BNT162b2), the infection probability decreases by 60% and 92%, respectively (Israeli data source). In the case of Japan, these values were almost the same [40] (note that for the B.1.617 delta variant, the effect might decrease [39]). Therefore, we adopted σ1=0.60 and σ2=0.92. Moreover, in Japan, as of February 10th, 2021, the total number of infected and dead persons was 408,000 and 6,507 people, respectively, [41]. Therefore, we adopted δ0=6,507/408,000=0.016 as the fatality rate. Dagan et al. [33] reported that the fatality rate decreased by 84% with the first dose of vaccination (second doses not reported). Therefore, we adopted δ1=δ2=δ0×(1−0.84) as the fatality rate after vaccination.
The research objective is to simulate the actual Japanese conditions. Hence, it is necessary to obtain an infectivity parameter that can reproduce the Japanese spreading rate. Therefore, we searched for infectivity β(t) such that the differences between the SIRVVD model based on the number of newly infected individuals I+(t) defined by Equation (2.14) and the number of newly infected individuals in the case of actual Japan [36] were minimal. The best infectivity βopt(t) is defined by
βopt(t)=Argminβ(t)|I+(t)−I′+(t)|. | (3.2) |
In Figure 4, we show the actual newly infected individuals in Japan I′+(t) [36], and the newly infected individuals I+(t) reproduced by the SIRVVD model (Equation (2.14)) by using βopt(t). Because these are almost the same, we consider βopt(t) to represent infectivity in Japan.
Because the research objective is to verify the effects of vaccination, we set some values as (α1,α2), which are the coefficients for vaccination. These are
(α1,α2)∈{(0,0),(10−4,10−4),(10−3,10−3),(10−2,10−2),(10−4,10−2),(10−2,10−4)}. | (3.3) |
Scenario (α1,α2)=(0,0) represents the absence of a vaccine in Japan. Therefore, the amount reduced from the infected individuals in scenario (α1,α2)=(0,0) indicates the effectiveness of the vaccination.
Figure 5 shows the simulation results based on the conditions described in Section 3.1. This indicates that the higher the (α1,α2), the fewer the newly infected individuals I+(t) and dead persons D(t). It is clear that the speed of the infection spread is suppressed by the vaccination. As the vaccination progresses, the slower the infection spreads, which can be roughly understood by the discussion given in the Appendix. In contrast, in the case of scenario (α1,α2)=(10−4,10−2), the number of newly infected individuals and dead persons is large. This is because V1(t) is small; even if α2 is high, V2(t) becomes small. Therefore, it is important to increase V1(t) by substituting a high value for α1. We show the number of vaccinations N1(t)+N2(t) in the underside of Figure 5. It is desirable to substitute high values for (α1,α2); however, the preparation of many vaccines is required. For example, in the case of (α1,α2)=(10−2,10−2), approximately 3×108 vaccines are required for approximately a year. In Japan, the total vaccination rate was 8×107 for approximately 3 months from May to August 2021 [22]. If Japan can keep this pace, it may achieve approximately 3×108 vaccinations for about a year.
To discuss the effect of the vaccination in detail, the results of substituting various values for (α1,α2) are shown in Figure 6. The other parameters are the same as in the case of Figure 5, meaning that these simulations are assumed to be actual Japan. Figures 6 (A) and (B) show the numbers of first and second doses of the vaccination N1(tend) and N2(tend) at the end of the simulation tend, respectively. Figure 6 (A) shows that as α1 increases, so does N1(tend). Moreover, even if we substituted a high value for α2, in the case of substituting a small value for α1, N2(tend) is small. We can also understand this phenomenon by examining Figure 5. Figures 6 (C) and (D) show the maximum numbers of newly infected individuals MaxtI(t) and the number of dead persons D(tend) at the end of the simulation, respectively. Because N1(tend) increases with an increasing α1, MaxtI(t) and D(tend) decreases, owing to the effect of the vaccination. We can see that α2 also has an effect on reducing MaxtI(t) and D(tend). Moreover, the amount of change in MaxtI(t) and D(tend) in the case of a small α1 is larger than that in the case of a high α1. For example, if we compare (α1,α2)=(0.0002,0) and (0.0006,0), the difference between the maximum number of newly infected individuals is approximately 3,000. By contrast, if we compare (α1,α2)=(0.0012,0) and (0.0016,0), the difference between the maximum number of newly infected individuals is only approximately 50. These results indicate that the relationship between the number of vaccinations and the effect of reducing infected individuals is logarithmic. Therefore, even if the Japanese government prepares only a few vaccines, we infer that there would be a certain effect on reducing infected persons.
In this paper, we presented simulations of COVID-19 infections spreading in Japan considering first and second vaccination events. The mathematical models are found in Equations (2.3)–(2.10), which represent the effect of the first and second doses on reducing the mortality rate and infectivity. For simulation in Japan, we adopted the vaccine, "BNT162b2" [33]. The efficacy of the first and second doses were 60% and 92%, respectively [33]. Note that in the case of the B.1.617 delta variant, the effect of vaccinations may decrease [39]). As shown in Figures 5 and 6, vaccination reduces the number of newly infected individuals and dead persons in Japan.
As of August 8, 2021, the rate of second vaccinations in Japan was approximately 30%. Because this rate is insufficient, COVID-19 infection will continue to spread. However, in the near future, assuming that the rate of vaccination will increase in Japan, the number of infected individuals is expected to decrease. Because there is a possibility of developing new virus variants of COVID-19, we cannot be optimistic. Overcoming COVID-19 infection requires continued performance of various measures to decrease the number of infected individuals, such as vaccination, mask-wearing, teleworking, and contact tracing [15].
This work was supported in part by the Telecommunications Advancement Foundation (Grant No. 20203002 to Y. Omae) and a JSPS Grant-in-Aid for Scientific Research (C) (Grant No. 21K04535 to J. Toyotani).
Here, we discuss the effect of two-step vaccinations on the number of infected individuals. To obtain analytical insights, we simplify the situation: the infectivity β(t), the number of susceptible persons S(t), and vaccinated persons V1(t),V2(t) are constants with respect to time t, respectively. This is valid when the time scale of the infection spread or reduction is much faster than those of β(t),S(t),V1(t), and V2(t). In this situation, Equations (2.6) – (2.8) in the form of a differential equation can be written as
dI0(t)dt=(βS−γ)I0(t)+βS(I1(t)+I2(t)), | (4.1) |
dI1(t)dt={(1−σ1)βV1−γ}I1(t)+(1−σ1)βV1(I0(t)+I2(t)), | (4.2) |
dI2(t)dt={(1−σ2)βV2−γ}I2(t)+(1−σ2)βV2(I0(t)+I1(t)). | (4.3) |
These equations can be formally solved when one treats the second terms on the right-hand side of each equation as non-homogeneous (source terms). The solutions are given as
I0(t)=I0(0)et/τ0+βSet/τ0∫t0e−t′/τ0(I1(t′)+I2(t′))dt′, | (4.4) |
I1(t)=I1(0)et/τ1+(1−σ1)βV1et/τ1∫t0e−t′/τ1(I0(t′)+I2(t′))dt′, | (4.5) |
I2(t)=I2(0)et/τ2+(1−σ2)βV2et/τ2∫t0e−t′/τ2(I0(t′)+I1(t′))dt′, | (4.6) |
where the characteristic time scales, τ0,τ1, and τ2, are given as
τ0=1βS−γ, | (4.7) |
τ1=1{(1−σ1)βV1−γ}, | (4.8) |
τ2=1{(1−σ2)βV2−γ}. | (4.9) |
The first term in each solution has a typical time scale determined by its own contribution, which stems from the first terms in Equations (4.1)–(4.3). The relationships among these time scales are generally
τ0<τ1<τ2. | (4.10) |
Thus, the typical time scale for the infection spread is directly affected by the vaccination: the time scale without the vaccination is much faster than those with the vaccination. These time scales are estimated as τ0=15.81 days, τ1=39.53 days, and τ2=197.64 days, where the following values are used: S=V1=V2=2,000, β=10−4.5, γ=0, σ1=0.60, and σ2=0.92, the vaccine BNT162b2 [33]. These values indicate that the impact of the second vaccination was significant. In addition to the direct contribution (the first terms in Equations (4.4)–(4.6)) for each Ij(t) (j=0,1,2), there are interference terms from the infected individuals with the other vaccination counts, which correspond to the second terms on the right-hand side of Equations (4.4)–(4.6). Owing to the interference terms, multiple time scales exist in each Ij(t); for example, not only does the typical time scale τ1 appear in the time evolution of I1(t), but so does τ0 and τ2. Therefore, when I0(t)≫I1(t),I2(t), which corresponds to the situation immediately after starting vaccinations, I0(t) and I2(t) grow with the time scale of τ0. Additionally, when I2(t)≫I0(t),I1(t), which corresponds to the case when the vaccination is completed, the number of infected individuals varies with the time scale of τ2. Thus, vaccination drastically affects the time scale for the spread of infection.
[1] | Z. Y. Sun, T. J. Zhang, J. Q. Su, W. S. Chow, J. Q. Liu, L. L. Chen, et al., A novel role of ethephon in controlling the noxious weed Ipomoea cairica (Linn.) Sweets, Sci. Rep., 5 (2015), 11372. |
[2] | G. Liu, Y. Gao, F. Huang, M. Yuan, S. Peng, The Invasion of coastal areas in south China by Ipomoea cairica may be accelerated by the ecotype being more locally adapted to salt stress, Plos One, 11 (2016), e0149262. |
[3] |
S. Shen, Z. Shen, M. Zhao, Big Data Monitoring System Design and Implementation of Invasive Alien Plants Based on WSNs and WebGIS, Wireless Pers. Commun., 97 (2017), 4251–4263. doi: 10.1007/s11277-017-4723-0
![]() |
[4] |
M. Mafanya, P. Tsele, J. O. Botai, P. Manyama, G. J. Chirima, T. Monate, Radiometric calibration framework for ultra-high-resolution UAV-derived orthomosaics for large-scale mapping of invasive alien plants in semi-arid woodlands: Harrisia pomanensis as a case study, Int. J. Remote Sens., 39 (2018), 5119–5140. doi: 10.1080/01431161.2018.1490503
![]() |
[5] | M. Liu, H. Li, L. Li, W. Man, M. Jia, Z. Wang, et al., Monitoring the Invasion of Spartina alterniflora Using Multi-source High-resolution Imagery in the Zhangjiang Estuary, China, Remote Sens., 9 (2017), 539. |
[6] | A. M. West, P. H. Evangelista, C. S. Jarnevich, N. E. Young, T. J. Stohlgren, C. Talbert, et al., Integrating remote sensing with species distribution models; mapping Tamarisk invasions using the software for assisted habitat modeling (SAHM), J. Visualized Exp., 116 (2016), e54578. |
[7] | J. Bustamante, D. Aragonés, I. Afán, C. Luque, A. Pérez-Vázquez, E. Castellanos, et al., Hyperspectral sensors as a management tool to prevent the invasion of the exotic cordgrass spartina densiflora in the doñana wetlands, Remote Sens., 8 (2016), 1001. |
[8] | J. M. Barbosa, G. P. Asner, R. E. Martin, C. A. Baldeck, F. Hughes, T. Johnson, Determining subcanopy psidium cattleianum invasion in hawaiian forests using imaging spectroscopy, Remote Sens., 8 (2016), 33. |
[9] |
S. Khare, H. Latifi, S. K. Ghosh, Multi-scale assessment of invasive plant species diversity using Pléiades 1A, RapidEye and Landsat-8 data, Geocarto Int., 33 (2018), 681–698. doi: 10.1080/10106049.2017.1289562
![]() |
[10] | L. Tao, C. Cheng, Plant Identification Based on Image Set Analysis, In: Artificial Intelligence and Mobile Services–AIMS 2018, Lecture Notes in Computer Science, 2018. |
[11] |
S. Ge, R. Carruthers, P. Gong, A. Herrera, Texture analysis for mapping Tamarix parviflora using aerial photographs along the Cache Creek, California, Environ. Monit. Assess., 114 (2006), 65–83. doi: 10.1007/s10661-006-1071-z
![]() |
[12] |
D. Jones, S. Pike, M. Thomas, D. Murphy, Object-based image analysis for detection of Japanese knotweed sl taxa (Polygonaceae) in Wales (UK), Remote Sens., 3 (2011), 319–342. doi: 10.3390/rs3020319
![]() |
[13] | W. Dorigo, A. Lucieer, T. Podobnikar, A. Čarni, Mapping invasive Fallopia japonica by combined spectral, spatial, and temporal analysis of digital orthophotos, Int. J. Appl. Earth Obs. Geoinf., 19 (2012), 185–195. |
[14] | J. Sandino, F. Gonzalez, K. Mengersen, K. J. Gaston, UAVs and machine learning revolutionising invasive grass and vegetation surveys in remote arid lands, Sensors, 18 (2018), 605. |
[15] |
C. Hung, Z. Xu, S. Sukkarieh, Feature learning based approach for weed classification using high resolution aerial images from a digital camera mounted on a UAV, Remote Sens., 6 (2014), 12037–12054. doi: 10.3390/rs61212037
![]() |
[16] | M. P. Pound, J. A. Atkinson, A. J. Townsend, M. H. Wilson, M. Griffiths, A. S. Jackson, et al., Deep machine learning provides state-of-the-art performance in image-based plant phenotyping, GigaScience, 6 (2017), gix083. |
[17] |
A. Fuentes, S. Yoon, S. C. Kim, D. S. Park, A robust deep-learning-based detector for real-time tomato plant diseases and pests recognition, Sensors, 17 (2017), 2022. doi: 10.3390/s17092022
![]() |
[18] |
A. Ramcharan, K. Baranowski, P. McCloskey, B. Ahmed, J. Legg, D. P. Hughes, Deep learning for image-based cassava disease detection, Front. Plant Sci., 8 (2017), 1852. doi: 10.3389/fpls.2017.01852
![]() |
[19] |
H. Lu, Z. Cao, Y. Xiao, B. Zhuang, C. Shen, TasselNet: counting maize tassels in the wild via local counts regression network, Plant Methods, 13 (2017), 79. doi: 10.1186/s13007-017-0224-0
![]() |
[20] | M. Z. Alom, T. M. Taha, C. Yakopcic, S. Westberg, P. Sidike, M. S. Nasrin, et al., The history began from alexnet: A comprehensive survey on deep learning approaches, arXiv preprint arXiv: 1803.01164, 2018. |
[21] |
Y. Lecun, L. Bottou, Y. Bengio, P. Haffner, Gradient-based learning applied to document recognition, Proc. IEEE, 86 (1998), 2278–2324. doi: 10.1109/5.726791
![]() |
[22] |
A. Krizhevsky, I. Sutskever, G. Hinton, Imagenet classification with deep convolutional neural networks, Commun. ACM, 60 (2017), 84–90. doi: 10.1145/3065386
![]() |
[23] | C. Szegedy, W. Liu, Y. Jia, P. Sermanet, S. Reed, D. Anguelov, et al., Going Deeper with Convolutions, 2015 IEEE Conference on Computer Vision and Pattern Recognition (CVPR), Boston, MA, 2015. |
[24] | K. Simonyan, A. Zisserman, Very deep convolutional networks for large-scale image recognition, Comput. Sci., 2014 (2014), 21–30. |
[25] | K. He, X. Zhang, S. Ren, J. Sun, Deep residual learning for image recognition, 2016 IEEE Conference on Computer Vision and Pattern Recognition (CVPR), Las Vegas, NV, 2016. |
1. | Somayeh Momenyan, Mahmoud Torabi, Modeling the spatio‑temporal spread of COVID‑19 cases, recoveries and deaths and effects of partial and full vaccination coverage in Canada, 2022, 12, 2045-2322, 10.1038/s41598-022-21369-z | |
2. | Fehaid Salem Alshammari, Analysis of SIRVI model with time dependent coefficients and the effect of vaccination on the transmission rate and COVID-19 epidemic waves, 2023, 8, 24680427, 172, 10.1016/j.idm.2023.01.002 | |
3. | Yuto Omae, Makoto Sasaki, Jun Toyotani, Kazuyuki Hara, Hirotaka Takahashi, Theoretical Analysis of the SIRVVD Model for Insights Into the Target Rate of COVID-19/SARS-CoV-2 Vaccination in Japan, 2022, 10, 2169-3536, 43044, 10.1109/ACCESS.2022.3168985 | |
4. | A Othman Almatroud, Noureddine Djenina, Adel Ouannas, Giuseppe Grassi, M Mossa Al-sawalha, A novel discrete-time COVID-19 epidemic model including the compartment of vaccinated individuals, 2022, 19, 1551-0018, 12387, 10.3934/mbe.2022578 | |
5. | Viktoriya Petrakova, Olga Krivorotko, 2023, Mean Field Optimal Control Problem for Predicting the Spread of Viral Infections, 979-8-3503-3113-4, 79, 10.1109/OPCS59592.2023.10275759 | |
6. | Fehaid Salem Alshammari, Fahir Talay Akyildiz, Epidemic Waves in a Stochastic SIRVI Epidemic Model Incorporating the Ornstein–Uhlenbeck Process, 2023, 11, 2227-7390, 3876, 10.3390/math11183876 | |
7. | Oscar Espinosa, Laura Mora, Cristian Sanabria, Antonio Ramos, Duván Rincón, Valeria Bejarano, Jhonathan Rodríguez, Nicolás Barrera, Carlos Álvarez-Moreno, Jorge Cortés, Carlos Saavedra, Adriana Robayo, Oscar H. Franco, Predictive models for health outcomes due to SARS-CoV-2, including the effect of vaccination: a systematic review, 2024, 13, 2046-4053, 10.1186/s13643-023-02411-1 | |
8. | Karan Thakkar, Julia Regazzini Spinardi, Jingyan Yang, Moe H. Kyaw, Egemen Ozbilgili, Carlos Fernando Mendoza, Helen May Lin Oh, Impact of vaccination and non-pharmacological interventions on COVID-19: a review of simulation modeling studies in Asia, 2023, 11, 2296-2565, 10.3389/fpubh.2023.1252719 | |
9. | Abdennour Sebbagh, Chemesse Ennehar Bencheriet, Sihem Kechida, A Stochastic Epidemiological SIRD-V Model With LSM-EKF Algorithm for Forecasting and Monitoring the Spread of COVID-19 Pandemic: Real Data, 2024, 12, 2169-3536, 62047, 10.1109/ACCESS.2024.3395544 |
Model | α1 | α2 | MaxtI(t) | D(tend) |
SIRVD model | 0.01 | - | 1,299 | 653 |
SIRVVD model | 0.01 | 0.01 | 1,204 | 630 |
SIRVVD model | 0.01 | 0.03 | 1,077 | 598 |
SIRVD model | 0.03 | - | 623 | 288 |
SIRVVD model | 0.03 | 0.01 | 438 | 229 |
SIRVVD model | 0.03 | 0.03 | 281 | 164 |