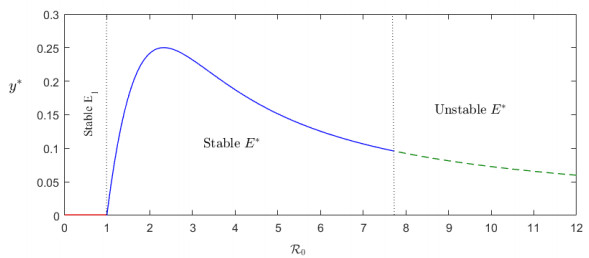
Citation: Fahad Al Basir, Yasuhiro Takeuchi, Santanu Ray. Dynamics of a delayed plant disease model with Beddington-DeAngelis disease transmission[J]. Mathematical Biosciences and Engineering, 2021, 18(1): 583-599. doi: 10.3934/mbe.2021032
[1] | Rundong Zhao, Qiming Liu, Huazong Zhang . Dynamical behaviors of a vector-borne diseases model with two time delays on bipartite networks. Mathematical Biosciences and Engineering, 2021, 18(4): 3073-3091. doi: 10.3934/mbe.2021154 |
[2] | Yan-Xia Dang, Zhi-Peng Qiu, Xue-Zhi Li, Maia Martcheva . Global dynamics of a vector-host epidemic model with age of infection. Mathematical Biosciences and Engineering, 2017, 14(5&6): 1159-1186. doi: 10.3934/mbe.2017060 |
[3] | Jie He, Zhenguo Bai . Global Hopf bifurcation of a cholera model with media coverage. Mathematical Biosciences and Engineering, 2023, 20(10): 18468-18490. doi: 10.3934/mbe.2023820 |
[4] | Sarita Bugalia, Jai Prakash Tripathi, Hao Wang . Mathematical modeling of intervention and low medical resource availability with delays: Applications to COVID-19 outbreaks in Spain and Italy. Mathematical Biosciences and Engineering, 2021, 18(5): 5865-5920. doi: 10.3934/mbe.2021295 |
[5] | Zhenzhen Liao, Shujing Gao, Shuixian Yan, Genjiao Zhou . Transmission dynamics and optimal control of a Huanglongbing model with time delay. Mathematical Biosciences and Engineering, 2021, 18(4): 4162-4192. doi: 10.3934/mbe.2021209 |
[6] | Yan Wang, Minmin Lu, Daqing Jiang . Viral dynamics of a latent HIV infection model with Beddington-DeAngelis incidence function, B-cell immune response and multiple delays. Mathematical Biosciences and Engineering, 2021, 18(1): 274-299. doi: 10.3934/mbe.2021014 |
[7] | Tailei Zhang, Hui Li, Na Xie, Wenhui Fu, Kai Wang, Xiongjie Ding . Mathematical analysis and simulation of a Hepatitis B model with time delay: A case study for Xinjiang, China. Mathematical Biosciences and Engineering, 2020, 17(2): 1757-1775. doi: 10.3934/mbe.2020092 |
[8] | Vanessa Steindorf, Sergio Oliva, Jianhong Wu . Cross immunity protection and antibody-dependent enhancement in a distributed delay dynamic model. Mathematical Biosciences and Engineering, 2022, 19(3): 2950-2984. doi: 10.3934/mbe.2022136 |
[9] | Yuhua Long, Yining Chen . Global stability of a pseudorabies virus model with vertical transmission. Mathematical Biosciences and Engineering, 2020, 17(5): 5234-5249. doi: 10.3934/mbe.2020283 |
[10] | Martin Luther Mann Manyombe, Joseph Mbang, Jean Lubuma, Berge Tsanou . Global dynamics of a vaccination model for infectious diseases with asymptomatic carriers. Mathematical Biosciences and Engineering, 2016, 13(4): 813-840. doi: 10.3934/mbe.2016019 |
Plant has to rely solely on cellular innate immunity to deal with infections as it does not possess any form of mobile defence and therefore it exhibits many plant-specific characteristics [1]. Plants showing recovery phenotype to a specific viral infection initially become affected but later experience new growth that is progressively more resistant to the virus until they finally produce new virus-free leaves with complete immunity [2]. Generally, plant gains hypersensitive resistance by triggering the self-destruction of infected cells, with necrotic tissue forming at and around the infection site. The cells surrounding these necrotic lesions are usually found in the antiviral state. Although some of them may contain traces of the virus, the virus is unable to replicate. This can be explained by the post-transcriptional gene silencing (PTGS), or RNA interference [3]. Besides disease resistance, time delay in disease transmission, known as incubation period, is an important factor that arises from disease resistance. The length of this delay depends on the plant's resistance [4].
Vector-borne plant viral diseases have attracted the interest of researchers who are working with modeling approach [5,6]. Mathematical modeling of vector-borne plant disease is an effective approach to understand the transmission of disease in host plants. With this approach, some effective measures can be done to make appropriate disease control strategy [7].
For the modeling purposes, usually, the rate of infection in most of the models for viral disease is assumed to be bilinear in the carrier vector v and susceptible host x. However, the actual incidence rate is probably not linear over the entire range of v and x. Therefore, it is reasonable to assume the infection rate of plant-vector model as Beddington-DeAngelis functional response, λxv1+ax+cv, where a,c,λ≥0 are constants. The functional response was introduced by Beddington [8] and DeAngelis et al. [9]. It includes nonlinear incidence rate and bilinear functional response, Holling type II functional response and saturation response [10,11]. Mathematical models using Beddington-DeAngelis type infection rate have been proposed and analysed for viral disease dynamics [12,13].
In plant epidemiology, Holt et al. have studied the dynamics of mosaic disease of Cassava which is also carried through whitefly [14]. Venturino et al. have proposed a mathematical model for studying the dynamics of Jatropha curcas mosaic disease vectored by whitefly [15] and observed oscillations in the system due to high infection rate. In [16], authors have proposed a model for the dynamics of soil-borne plant disease with host demography and shown the limit cycle behaviour. In the above mentioned articles authors have used bilinear disease transmission in modeling process. On the other hand, in [17,18], authors have taken nonlinear incidence rate for vector-borne plant disease propagation.
Another important factor in a biological system is the time delay. Vector-borne plant disease models with delay can show stability switches, periodic oscillations, transcritical bifurcation etc. [14,15,16]. However, such an analysis is crucial to assess the persistence of infection and disease control.
Van der Plank [19] first incorporated delay differential equation with the single delay in plant epidemics assuming the time evolution of the density of infected host tissue. Despite many studies that followed Van der Plank's model and that adopted discrete time approximations of it, delay differential equations are rarely used in theoretical studies of plant disease. In [20], the authors introduced plant incubation period as a delay in a plant disease model proposed by Meng et al. [21] to notice the changes in the dynamics of the model. Also in [16], authors have proposed a model for the dynamics of soil-borne plant disease taking a time delay due to latent period of inoculum/vectors. In [22], the authors modified the model by Shi et al. [23] for vector-borne disease in plants by including multiple delays to account for the incubation periods of plants and latent period in vectors. They have also noticed the changes in the dynamics of the system, specifically changes in the solutions trajectories. They have derived the threshold value for delay induced destabilization. But they have missed important dynamics that delay can induce misleading the conclusions that is established in [24,25]. Large delay can stabilise a system or make the system disease-free [26]. Therefore, construction of vector-borne plant disease models with time delays requires a clear understanding of the relevant biological mechanism.
In this article, motivated by the above discussions, we have derived the mathematical model for plants viral disease including plants resistance and incubation delay (section 2). Then some basic properties of the model have been analysed (section 3). The effect of delay on the stability of equilibria has been focused (section 4, 5). Also, Hopf bifurcation due to the stability changes has been analysed and the outcomes are discussed and shown in figures (section 6). Finally, a discussion of the main results concludes the paper (section 7).
Plant and vector populations are considered without explicitly including the mosaic virus. Here, x(t), y(t) denote the abundances of susceptible and infected plants (m−2) respectively. Number of infected vector (virus carrier) is denoted by v(t) (plant−1) at any time t.
Logistic growth for susceptible plant due to the finite area of the plantation is specified by net growth rate r and carrying capacity k. A successful contact between infected vectors with a healthy plant makes it infected [27], m is the natural death rate, d is disease related death rate of infected plants.
Let λ be the maximum contact rate from an infected vector to a susceptible plant. Usually the rate of infection in most of the models for viral disease is assumed to be bilinear in the carrier vector v and susceptible host x. However, the actual incidence rate is probably not linear over the entire range of v and x. Thus, from the above discussion, it is reasonable to assume a Beddington-DeAngelis type infection rate i.e., λxv1+ax+cv, where a is the plant resistance rate, c is rate for the crowding effect of vectors [12,13].
Insect vector is recruited in the system at a rate b and is proportional to infected plant population. The infective insects do not get sick from the virus, they are just carriers [28]. Let μ be the mortality rate of infected vector.
The effect of time delay in terms of incubation period of plant is modeled via the term λe−mτx(t−τ)v(t−τ)1+ax(t−τ)+cv(t−τ). The term e−mτ represents the survival probability of plant through the incubation time τ.
Based on the above assumptions, we have the following mathematical model:
dxdt=rx[1−x+yk]−λxv1+ax+cv,dydt=λe−mτx(t−τ)v(t−τ)1+ax(t−τ)+cv(t−τ)−(m+d)y,dvdt=by−μv. |
Let C denote the Banach space of continuous functions ϕ:[−τ,0]→R3 equipped with the sup-norm,
‖ϕ‖=sup−τ≤γ≤0{|ϕ1(γ)|,|ϕ2(γ)|,|ϕ3(γ)|}, |
where, ϕ=(ϕ1,ϕ2,ϕ3)∈C([−τ,0],R3). For biological reasons, populations always have nonnegative values, therefore, the initial function for model (2.1) is taken as below:
x(γ)=ϕ1(γ),y(γ)=ϕ2(γ),v(γ)=ϕ3(γ)withϕi(γ)≥0,γ∈[−τ,0),ϕi(0)>0,i=1,2,3. | (2.1) |
In this section, some basic properties such as non-negativity, boundedness of solutions and possible equilibria are analysed.
Lemma 1. All solutions of system (2.1) are non-negative on [0,+∞) under the initial conditions (2.1) and are ultimately bounded.
Proof. We rewrite the first equation of (2.1) as
dxdt−x[r(1−x+yk)−λv1+ax+cv]=0. | (3.1) |
Let f(x,y,v)=r(1−x+yk)−λv1+ax+cv, then from above equation, we have
dxdt−xf(x,y,v)=0⇒[dxdt−xf(x,y,v)]exp(−∫t0f(x(ξ),y(ξ),v(ξ))dξ)=0⇒ddt[xexp(−∫t0f(x(ξ),y(ξ),v(ξ))dξ)]=0. |
⇒x(t)=x(0)exp(∫t0f(x(ξ),y(ξ),v(ξ))dξ). |
Since, x(0)=ϕ1(0)>0, then x(t)>0 for t≥0.
Using the method of steps, we show the non-negativity of y and v.
The second equation of system (2.1) gives,
y′(t)≥−(m+d)y(t) and v′(t)≥−μv(t), |
for some constant T>0 and for all t∈(0,T]. Using the standard comparison principle, we have y(t)≥0 and v(t)≥0 for all t∈(0,T].
The above argument can be repeated to deduce the non-negativity of y and v on the interval t∈(T,2T] and so on the successive intervals t∈(nT,(n+1)T],n=2,3,… to include all positive times.
To ensure the model biologically plausible, both plant and vector populations have to remain bounded during their time evolution.
To prove the boundedness of x(t) and y(t), let
F(t)=e−mτx(t)+y(t+τ) |
Then,
dF(t)dt=e−mτdxdt+dy(t+τ)dt=e−mτrx[1−x+yk]−e−mτλxv1+ax+cv+λe−mτxv1+ax+cv−(m+d)y(t+τ)=e−mτrx[1−x+yk]−(m+d)y(t+τ)<e−mτ[(r+q)x−rx2k]−qF(t),where, q=m+d |
Now, (r+q)x−rx2k is a quadratic in x and its maximum value is k(r+q)24r and e−mτ≤1. Using this, we can write
dF(t)dt<k(r+q)24r−qF(t). |
This implies
lim supt→∞F(t)≤k(r+q)24rq. | (3.2) |
and hence, the x(t) and y(t) are bounded. As y is bounded, using the well-known comparison principle, from the third equation of (2.1), v(t) is also bounded.
We follow the method established in the paper by Hefferman et al. [29] for calculating R0.
We consider the next generation matrix G comprised of two parts namely F and V, Fi are the new infections, while the Vi transfers of infections from one compartment to another.
F=[∂Fi(E1)∂xj]=[0λe−mτk1+ak00] |
and
V=[∂Vi(E1)∂xj]=[(d+m)0−bμ] |
where E1(k,0,0) is the disease-free equilibrium and indices i, j correspond, respectively, to y and v. The basic reproduction number R0 is the dominant eigenvalue of the matrix G=FV−1, i.e., Eq (3.3).
R0=kλe−mτbμ(d+m)(1+ak). | (3.3) |
Remark 1. It is important to note that R0 does not depend on c (crowding effect) and hence it is independent that how efficiently vectors interact with healthy plants. At the same time, taking the limit of k→∞ gives
limk→∞R0(ω0)=R∞0≡λe−mτbμ(d+m)a |
In light of the fact that R0 is monotonically decreasing with increasing a, this suggests that eradication of disease, as represented by a stable disease-free steady state E1 is possible if R∞0<1. The available means to achieve this is by increasing the resistance rate or incubation period. This can be done using nutrients, biocontrolling agents, genetic engineering, chemicals etc. [31,32,33,34].
The model (2.1) has three equilibria namely (ⅰ) the trivial equilibrium E0(0,0,0), (ⅱ) the disease-free equilibrium, E1(k,0,0), and (ⅲ) the unique coexistence equilibrium, here E∗(x∗,y∗,v∗) is determined as follows.
From the third equation of (2.1), we can write v∗ as
v∗=by∗μ | (3.4) |
Considering the summation of the first and second equation times emτ of (2.1), we get y∗ in term of x∗ as
y∗=re−mτx∗(k−x∗)k(m+d)+re−mτx∗. | (3.5) |
Putting the value of v∗ and y∗ in the first equation of (2.1), we get x∗ as the positive root of the following equation:
L(x)=L0x2+L1x+L2=0 | (3.6) |
where,
L0=rλe−mτ(−bλe−mτ−bcq+aμq)L1=λ(−bkλe−mτq+akμq2+bcke−mτqr+e−mτμqr)L2=kλμq2>0 | (3.7) |
and q=(m+d).
We can check that L(0)>0 always and L(k)<0 when R0>1. Hence there exists x∗ satisfying 0<x∗<k if R0>1. Note that R0>1 implies that bλe−mτ>aμq, which shows L0<0 when R0>1. Therefore the solution x∗ of L(x∗)=0 is unique and satisfies 0<x∗<k which ensures y∗>0, from (3.5).
Thus we have the following result for the feasibility of endemic steady state E∗.
Proposition 1. Let us assume R0>1. Then there exists a unique endemic equilibrium E∗ of system (2.1).
In this section, the local stability of the delayed system (2.1) is studied around the equilibria. For this, we linearize the system (2.1) about any equilibrium E(x,y,v) and we get
dXdt=PX(t)+QX(t−τ). | (3.8) |
Here P,Q are 3×3 matrices, given below:
P=[pij]=[r(1−2x+yk)−λv(1+cv)(1+ax+cv)2−rxk−λx(1+ax)(1+ax+cv)20−(d+m)00b−μ], |
Q=[qij]=[000λe−mτv(1+cv)(1+ax+cv)20λe−mτx(1+ax)(1+ax+cv)2000]. |
The characteristic equation of the delay system (2.1), at any equilibrium point, is given by
H(ξ,τ)=∣ξI−P−e−ξτQ∣=0. |
This gives
H(ξ,τ)=ξ3+m1ξ2+m2ξ+m5+e−ξτ[m3+m4ξ]=0. | (3.9) |
Here,
m1=−(p11+p22+p33),m2=p22p33+p11p33+p11p22,m4=−(p32q23+p12q21),m3=−p13q21p32+p11q23p32+q21p12p33,m5=−p11p22p33. |
The trivial equilibrium E0(0,0,0) is always unstable in view of the fact that one eigenvalue (ξ=r) is positive. Now we study the stability of disease-free and endemic equilibria.
For the disease-free equilibrium E1,
P=[pij]=[−r−r−λk1+ak0p2200b−μ],Q=[qij]=[00000λe−mτk1+ak000] |
where p22=−(d+m). Now, ∣ξI−P−e−ξτQ∣=0 gives the following characteristic equation at E1,
H(ξ,τ)=(ξ+r)⋅(ξ2+(m+d+μ)ξ+(m+d)μ−bλke−(m+ξ)τ1+ak)=0. | (4.1) |
The following two cases are raised: (ⅰ) τ=0 and (ⅱ) τ>0.
(ⅰ) For τ=0, the characteristic equation is
H(ξ,0)=(ξ+r)⋅(ξ2+(m+d+μ)ξ+(m+d)μ−bλk1+ak)=0. | (4.2) |
Thus, at the disease-free equilibrium E1(k,0,0), one eigenvalue is −r<0, and the rest of the eigenvalues will be negative or with negative real part if (m+d)μ−bλk1+ak>0, which is equivalent to R0<1. Thus for the stability of E1, we have the following theorem.
Theorem 1. For τ=0, the disease-free equilibrium E1 of the model (2.1) is stable if R0<1 and unstable if R0>1.
(ⅱ) For τ>0, one eigenvalue is −r<0, and the remaining roots satisfy the quadratic equation
H(ξ,τ)=ξ2+(m+d+μ)ξ+(m+d)μ−bλke−(m+ξ)τ1+ak=0, | (4.3) |
and we have the following theorem.
Theorem 2. Disease-free equilibrium E1 of the model (2.1) for τ>0 is asymptotically stable for R0<1, and unstable for R0>1.
Proof. Assume that R0>1, then H(0,τ)=(m+d)μ−bkλe−mτ1+ak=(m+d)μ(1−R0)<0. Since limξ→∞H(ξ,τ)=∞, there exists at least one real positive root of the characteristic equation (4.3), implying that E1 is unstable.
Next, let us assume R0<1. We note that in this case for τ=0 all characteristic roots of Eq (4.3) have negative real part (Theorem 1) and therefore, our goal is to show that as τ>0, none of the characteristic roots can reach the imaginary axis.
Let us assume by contradiction that for some τ>0, ξ=iκ is a root of (4.3). Putting ξ=iκ in (4.3) and separating real and imaginary parts, we have
−κ2+M2=M3cosκτ, | (4.4) |
κM1=−M3sinκτ. | (4.5) |
Here M1=(m+d+μ), M2=(m+d)μ, and M3=bkλe−mτ1+ak. Squaring and then adding the above equations, we finally obtain
κ4+κ2(q2+μ2)+(M22−M23)=0. | (4.6) |
Note that R0<1 implies M3<M2. Since this equation has no real roots for κ, it means that the characteristic equation (4.3) cannot have purely imaginary roots. Thus, for R0<1, the steady state E1 is asymptotically stable for all τ≥0.
For the stability of endemic equilibrium E∗, again we consider two cases, τ=0 and τ>0.
When τ=0, the characteristic at E∗ equation becomes
H(ξ,0)=ξ3+m1ξ2+(m2+m4)ξ+(m3+m5)=0. | (5.1) |
Denoting by σ1=m1,σ2=(m2+m4),σ3=(m3+m5) and using the Routh-Hurwitz criterion [30], for τ=0, we can get the following theorem.
Theorem 3. For τ=0, the coexistence equilibrium point E∗(x∗,y∗,v∗) is stable if the conditions
σ1>0,σ3>0andσ1σ2−σ3>0 | (5.2) |
are satisfied.
Now, we study the local Hopf bifurcation of E∗. Any of the parameters of the model may be a bifurcation parameter. We assume, α as the generic bifurcating parameter of the system. The following theorem characterises the possible occurrence of Hopf bifurcation for τ=0.
Theorem 4. The system (2.1) with τ=0, undergoes Hopf bifurcation around the endemic equilibrium point E∗ at α=α∗, where the critical parameter value is contained in the following domain
ΓHB={α∗∈R+:σ1(α∗)σ2(α∗)−σ3(α∗)=0, withσ1(α∗)>0,σ2(α∗)>0,σ′3(α∗)−(σ′1(α∗)σ2(α∗)+σ1(α∗)σ′2(α∗))≠0} | (5.3) |
Primes denote the derivative with respect to α.
Proof. Using the condition, σ1σ2−σ3=0, the characteristic equation (5.1) becomes
(ξ2+σ2)(ξ+σ1)=0, | (5.4) |
which has three roots namely ξ1=+i√σ2,ξ2=−i√σ2 and ξ3=−σ1. Therefore, a pair of purely imaginary eigenvalues exists for σ1σ2−σ3=0. Now we verify the transversality condition.
Differentiating the characteristic equation (5.1) with respect to α, we have
dξdα|ξ=i√σ2=−ξ2σ′1+ξσ′2+σ′33ξ2+2ξσ1+σ2|ξ=i√σ2=σ′3−(σ′1σ2+σ1σ′2)2(σ21+σ2)|ξ=i√σ2+i[√σ2(σ1σ′3+σ2σ′2−σ1σ′1σ2)2σ2(σ21+σ2)]|ξ=i√σ2. |
Therefore,
dReξdα|ξ=i√σ2=σ′3−(σ′1σ2+σ1σ′2)2(σ21+σ2)|ξ=i√σ2≠0⇔σ′3−(σ′1σ2+σ1σ′2)|ξ=i√σ2≠0. | (5.5) |
and thus Hopf bifurcation occurs at α=α∗.
For τ>0, the characteristic equation at E∗ becomes
ψ(ξ,τ)=ξ3+m1ξ2+m2ξ+m5+[m3+m4ξ]e−ξτ=0, | (5.6) |
which is a transcendental equation in ξ with infinitely many roots. It is known that E∗ is locally stable (or unstable) if all the roots of the corresponding characteristic equation have negative real parts (or at least one root has a positive real part). Also, Hopf bifurcating periodic solution exists if purely imaginary roots exist. We shall check the possible occurrence of Hopf bifurcation.
For this, suppose ξ=iw(τ) is a root of the Eq (5.6), then we have
−iw3−m1w2+im2w+(m4iw+m3)(coswτ−isinwτ)+m5=0. | (5.7) |
Separating real and imaginary parts, we obtain the following equations,
w3−m2w=m4wcoswτ−m3sinwτ. | (5.8) |
m1w2−m5=m3coswτ+m4wsinwτ. | (5.9) |
Squaring and adding the real and imaginary parts, we get
w6+(m21−2m2)w4+(m22−2m1m5−m24)w2+(m25−m23)=0. | (5.10) |
Denote z=w2 and the Eq (5.10) becomes
F(z)=z3+S1z2+S2z+S3=0, | (5.11) |
where,
S1=m21−2m2,S2=m22−2m1m5−m24,S3=m25−m23. |
By Descartes rule of signs, we can say that if the conditions S1≥0,S2≥0,S3≥0 are satisfied, then it is easy to show that the Eq (5.11) has no positive real roots.
Lemma 2. If the conditions (i) σ1>0,σ3>0 and σ1σ2−σ3>0, and (ii) S1≥0,S3≥0 and S2≥0 are satisfied then the infected steady state E∗ is asymptotically stable for all τ≥0.
Remark 2. We can say that if the model parameters satisfy the conditions given in Lemma 2, the steady state E∗ of the delay model (2.1) is asymptotically stable for all τ>0 i.e., the stability of the system is independent of delay.
If S3<0, then Eq (5.11) satisfies F(0)<0 and limz→∞F(z)=∞. Thus Eq (5.11) has at least one positive root, r∗ for S3<0, and consequently the characteristic equation (5.6) has a pair of purely imaginary roots ±i√r∗=iw, say.
We have the following lemmas.
Lemma 3. If S3<0, then there exists at least one positive root of Eq (5.11).
Let us assume (5.11) has three positive roots denoted as rk,k=1,2,3. Then (5.10) has three positive roots wr=√rk. Let us define
τ0=minn≥0,1≤k≤3{τnk},w0=wk(τ0). | (5.12) |
For w(τ0)=w0, the Eqs (5.8) and (5.9) give,
τ0=1w0arccos[m4w04−(m2m4−m1m3)w02−m3m5m24w20+m23]+2nπw0,n=0,1,2,…. | (5.13) |
if (m1m4−m3)w30−(m2m3−m4m5)w20>0, and
τ0=1w0arccos[m4w04−(m2m4−m1m3)w02−m3m5m24w20+m23]+2(n+1)πw0,n=0,1,2,…. | (5.14) |
if (m1m4−m3)w30−(m2m3−m4m5)w20≤0. Thus we have the following theorem.
Theorem 5. Suppose that the interior equilibrium point E∗ exists and is locally asymptotically stable for τ=0 i.e., σ1>0,σ3>0 and σ1σ2−σ3>0 hold. If S3<0, then E∗ is asymptotically stable for τ<τ0 and unstable for τ>τ0, where
τ0=1w0arccos[(m4w04)−(m2m4−m1m3)w02−m3m5m24w20+m23]. | (5.15) |
When τ=τ0, Hopf bifurcation occurs, i.e., a family of periodic solution bifurcates at E∗ as τ passes through the critical value τ0 provided the transversality condition,
P(w0)R(w0)−Q(w0)S(w0)≠0, | (5.16) |
is fulfilled.
Proof. Differentiating (5.8) and (5.9) with respect to τ, we have
ddτ[Re{λ(τ)}]|τ=τ0,w=w0=P(w)R(w)−Q(w)S(w)P2(w)+Q2(w)|τ=τ0,w=w0 | (5.17) |
where,
P(w)=−3w2+m2+m4coswτ−τ(m3coswτ+m4wsinwτ)Q(w)=−2m1w+m4sinwτ−τ(m3sinwτ−m4wcoswτ)R(w)=m3wsinwτ−m4w2coswτS(w)=m3wcoswτ+m4w2sinwτ. |
Therefore, ddτ[Re{λ(τ)}]τ=τ0,w=w0≠0 if P(w0)R(w0)−Q(w0)S(w0)≠0. Thus, the transversality condition is satisfied and hence Hopf bifurcation occurs at τ=τ0.
Remark 3. Theorem 5 only holds if (5.11) has at least one positive real root. Lemma 3 guarantees the occurrence of a positive root. However, when S3≥0, it is impractical to consider the analytical distribution of roots.
In this section, the analytical results of the previous sections are corroborated by numerical simulations. We have varied the important parameters, within their feasible range, to observe their impact on both the solution trajectories and equilibria of the model (2.1). We have plotted Figures 2–5 using ode45 solver in MATLAB. Figure 6 is plotted using the sign of eigenvalues at an equilibrium point. The values of parameters used in the numerical simulations are given in Table 1.
Parameters | Description | Values (unit) |
r | growth rate of plant density | 0.1 day−1 |
k | maximum plant density | 1 m−2 |
λ | infection rate of plant | 0.4 vector−1 day−1 |
m | infected plant removal rate | 0.1 day−1 |
d | additional death due to infection | 0.025 day−1 |
b | growth rate of infected vector | 0.4 day−1 |
μ | mortality rate of vector | 0.1 day−1 |
a | resistance rate of plant | 0.5 m 2 |
c | crowding effect of vector | 0.5 plant |
Figure 1 shows how the steady state values of the infected plant change with the infection transmission rate λ. This figure shows that the endemic steady state E∗ becomes feasible for some minimum value of λ that corresponds to R0=1 as discussed in Proposition 1. For values of λ just above its critical value λ∗, the steady state E∗ is unstable.
In Figure 2, numerical solution of the system (2.1) is plotted using different values of λ (the disease transmission rate from vectors to plant) for τ=0. The endemic equilibrium, E∗, is stable for some lower value of λ and system has a periodic orbit for λ=0.4. Oscillation increases the value of λ. In particular, there exists a critical value of λ below which E∗ is stable but if λ crosses this value then the system undergoes bifurcation and a stable periodic solution appears which is clearly presented in the figure. From Figure 3 and using Theorem 4, we observe that Hopf bifurcation occurs at λ=0.3667. The higher transmission rate causes larger amplitude of oscillations around this steady state E∗.
In Figure 4, numerical solution of the system is plotted for three different value of delay τ for a fixed value of λ=0.35 i.e., the E∗ is stable for τ=0 at that value of λ. The system (2.1) has periodic orbits when τ lies in the range τ∈(0,6). That is endemic equilibrium E∗ is stable for higher values of delay. It is clear from Figure 5 that the stability switches of the endemic equilibrium E∗ in terms of time delay τ at two points, nearly at τ≈1.012 days and τ≈5.56 days from stable to unstable and from unstable to stable, respectively.
Figure 6 illustrates the stability of equilibrium points in different parameter planes. It is observed that the stability of equilibria depends on time delay τ, the plant infection rate λ and vector growth rate b and immune response rate a. This figure shows that in the parameter region with R0<1, the system is free of disease, as signified by the stable disease-free equilibrium E1, and in the region with R0>1, the disease is present i.e., the endemic steady state E∗ is feasible there.
Figure 6(a) shows that disease-free state is stable when the product of infection rate λ and infective vector growth rate b is below some threshold value. It also illustrates that when the product of transmission rate λ and b crosses some threshold value, the endemic steady state becomes feasible and stable but it loses its stability for higher values of both λ and b. Figure 6(b), (c) suggest that disease transmission significantly depends on the resistance of plant and the incubation delay. Critical value of λ for Hopf bifurcation depends on these two parameter. Region of stability of equilibria widens as both of them are increased. Figure 6(d) shows that E∗ is unstable for small a and then becomes stable for larger time delay τ. It is also clear that disease resistance rate a and delay parameter τ have the ability to transform the unstable endemic into a stable disease-free state.
In this article, we have formulated a mathematical model using Beddington-DeAngelis functional response type infection rate and studied the dynamics of vector-borne plant disease. We have derived the basic reproduction number, R0, that helps us in understanding the dynamics of the model depending on the time delay and disease resistance. In the case where R0<1, the disease-free state, E1, is stable. This is also corroborated by the numerical calculations. When R0>1, however, the disease-free state becomes unstable and the endemic steady state becomes feasible. Oscillations in the solution of the susceptible plant, infected plant and infective vectors have been observed around the endemic equilibrium point. Resistance rate of the vector has been chosen as the continuation parameter, since it is one value that can be enhanced using nutrients which prevent the disease from occurring [28,34].
Numerical simulations have shown that the model can support resistant and recovery-type behaviours. We also found that the delay preserves the stability property of disease-free equilibrium by failing to induce stability switches when the equilibrium is stable. It is interesting to see that when endemic equilibrium is unstable and a periodic solution emerges at the endemic equilibrium (without delay), large delay or the resistance rate leads to the disappearance of the periodic solutions and later to disease-free situation [35]. This can be done using different control strategies (Remark 1).
In conclusion, Beddington-DeAngelis functional response type disease transmission rate has been successfully fitted in the model. The predictions from the model concerning particular dynamical regimes depending on parameters can provide the managers with effective methods for disease monitoring, as well as for developing proper techniques for successful disease management.
YT's research is supported by Japan Society for the Promotion of Science "Grant-in-Aid 20K03755".
All authors declare no conflicts of interest in this paper.
[1] | J. D. G. Jones, J. L. Dangl, The plant immune system, Nature, 444 (2006), 323–329. |
[2] | R. C. Gergerich, V. V. Dolja, Introduction to plant viruses, the invisible foe, Plant Health Instr., 4 (2006). |
[3] |
G. Neofytou, Y. N. Kyrychko, K.B. Blyuss, Time-delayed model of immune response in plants, J. Theor. Biol., 389 (2016), 28–39. doi: 10.1016/j.jtbi.2015.10.020
![]() |
[4] | M. Leclerc, T. Doré, C. A. Gilligan, P. Lucas, J. A. N. Filipe, Estimating the delay between host infection and disease (incubation period) and assessing its significance to the epidemiology of plant diseases, PLOS ONE, 9 (2014), 1–15. |
[5] | M. J. Jeger, L. V. Madden, F. van den Bosch, Plant Virus Epidemiology: applications and prospects for mathematical modeling and analysis to improve understanding and disease control, Plant Dis., 102 (2018), 837–854. |
[6] | R. P. Almeida, Ecology of emerging vector-borne plant diseases, in Institute of medicine forum on vector-borne diseases: understanding the environmental, human health, and ecological connections., National Academies Press, (2008), 70–77. |
[7] |
M. J. Jeger, J. Holt, F. van Den Bosch, L.V. Madden, Epidemiology of insect-transmitted plant viruses: modelling disease dynamics and control interventions, Physiol. Entomol., 29 (2004), 291– 304. doi: 10.1111/j.0307-6962.2004.00394.x
![]() |
[8] |
J. R. Beddington, Mutual interference between parasites or predators and its effect on searching efficiency, J. Anim. Ecol., 44 (1975), 331–340. doi: 10.2307/3866
![]() |
[9] | D. L. DeAngelis, R. A. Goldstein, R.V. O'Neill, A model for trophic interaction, Ecology, 56 (1975), 881–892. |
[10] |
X. Song, A. Neumann, Global stability and periodic solution of the viral dynamics, J. Math. Anal. Appl., 329 (2007), 281–297. doi: 10.1016/j.jmaa.2006.06.064
![]() |
[11] | R. Xu. Global stability of an HIV-1 infection model with saturation infection and intracellular delay, J. Math. Anal. Appl., 375 (2011), 75–81. |
[12] | G. Huang, W. Ma, Y. Takeuchi, Global analysis for delay virus dynamics model with Beddington– DeAngelis functional response, Appl. Math. Lett., 24 (2011) 1199–1203. |
[13] |
G. Huang, W. Ma, Y. Takeuchi, Global properties for virus dynamics model with Beddington– DeAngelis functional response, Appl. Math. Lett., 22 (2009), 1690–1693. doi: 10.1016/j.aml.2009.06.004
![]() |
[14] |
J. Holt, M. J. Jeger, J. M. Thresh, G. W. Otim-Nape, An epidemiological model incorporating vector population dynamics applied to African cassava mosaic virus disease, J. Appl. Ecol., 34 (1997), 793–806. doi: 10.2307/2404924
![]() |
[15] |
E. Venturino, P. K. Roy, F. A. Basir, A. Datta, A model for the control of the mosaic virus disease in jatropha curcas plantations, Energy Ecol. Environ., 1 (2016), 360–369. doi: 10.1007/s40974-016-0033-8
![]() |
[16] | B. Buonomo, M. Cerasuolo, Stability and bifurcation in plant-pathogens interactions, Appl. Math. Comput., 232 (2014), 858–871. |
[17] |
M. Jackson, B. M. Chen-Charpentier, Modeling plant virus propagation with delays, J. Comput. Appl. Math., 309 (2017), 611–621. doi: 10.1016/j.cam.2016.06.009
![]() |
[18] |
M. Jackson, B. M. Chen-Charpentier, A model of biological control of plant virus propagation with delays, J. Comput. Appl. Math., 330 (2018), 855–865. doi: 10.1016/j.cam.2017.01.005
![]() |
[19] | J. E. van der Plank, Plant Diseases: Epidemics and Control, Academic Press, 1963. |
[20] | T. Zhang, X. Meng, Y. Song, Z. Li, Dynamical analysis of delayed plant disease models with continuous or impulsive cultural control strategies, Abstr. Appl. Anal., 2012 (2012). |
[21] | X. Meng, Z. Li, The dynamics of plant disease models with continuous and impulsive cultural control strategies, J. Theor. Biol., 266 (2010), 2940. |
[22] | M. Jackson, B. M. Chen-Charpentier, Modeling plant virus propagation with delays, J. Comput. Appl. Math, , 309 (2016), 611–621. |
[23] | R. Shi, H. Zhao, S. Tang, Global dynamic analysis of a vector-borne plant disease model, Adv. Differ. Equations., 59 (2014). |
[24] |
M. Banerjee, Y. Takeuchi, Maturation delay for the predators can enhance stable coexistence for a class of prey-predator models, J. Theor. Biol., 412 (2017), 154–171. doi: 10.1016/j.jtbi.2016.10.016
![]() |
[25] |
B. Buonomo, M. Cerasuolo, The effect of time delay in plant-pathogen interactions with host demography, Math. Biosci. Eng., 12 (2015), 473–490. doi: 10.3934/mbe.2015.12.473
![]() |
[26] |
F. A. Basir, S. Ray, Impact of incubation delay in plant-vector interaction, Math. Comput. Simul., 170 (2020), 16–31. doi: 10.1016/j.matcom.2019.09.001
![]() |
[27] |
N. Rakshit, F. A. Basir, A. Banerjee, S. Ray, Dynamics of plant mosaic disease propagation and the usefulness of roguing as an alternative biological control, Ecol. Complex., 38 (2019), 15–23. doi: 10.1016/j.ecocom.2019.01.001
![]() |
[28] |
F. A. Basir, K. B. Blyuss, S. Ray, Modelling the effects of awareness-based interventions to control the mosaic disease of Jatropha curcas, Ecol. Complex., 36 (2018), 92–100. doi: 10.1016/j.ecocom.2018.07.004
![]() |
[29] |
J. M. Heffernan, R. J. Smith, L. M. Wahl, Perspectives on the basic reproductive ratio, J. R. Soc. Interface, 2 (2005), 281–93. doi: 10.1098/rsif.2005.0042
![]() |
[30] |
E. X. DeJesus, C. Kaufman, Routh-Hurwitz criterion in the examination of eigenvalues of a system of nonlinear ordinary differential equations, Phys. Rev. A., 35 (1987), 5288–5290. doi: 10.1103/PhysRevA.35.5288
![]() |
[31] |
D. W. M. Cook, P. J. Long, S. Ganesh, The combined effect of delayed application of yeast biocontrol agents and fruit curing for the inhibition of the postharvest pathogen Botrytis cinerea in kiwifruit, Postharvest Biol. Technol., 16 (1999), 233–243. doi: 10.1016/S0925-5214(99)00003-4
![]() |
[32] | D. Liu, K. G. Raghothama, P. M. Hasegawa, R. A. Bressan, Osmotin overexpression in potato delays development of disease symptoms, Proceedings of the National Academy of Sciences of the United States of America, 2012. |
[33] | K. Niehaus, D. Kapp, A. Pühler, Plant defence and delayed infection of alfalfa pseudonodules induced by an exopolysaccharide (EPS I)-deficient Rhizobium meliloti mutant, Planta, 190 (1993) 415–425. |
[34] | K. B. Blyuss, F. Al Basir, V. A. Tsygankova, L. O. Biliavska, G. O. Iutynska, S. N. Kyrychko, et al., Control of mosaic disease using microbial biostimulants: insights from mathematical modelling, Ric. Mat., 69 (2020), 437–455. |
[35] | F. A. Basir, S. Adhurya, M. Banerjee, E. Venturino, S. Ray, modelling the effect of incubation and latent periods on the dynamics of vector-borne plant viral diseases, Bull. Math. Biol., 82 (2020), 94. |
1. | Benito Chen-Charpentier, Stochastic Modeling of Plant Virus Propagation with Biological Control, 2021, 9, 2227-7390, 456, 10.3390/math9050456 | |
2. | Zhenzhen Liao, Shujing Gao, Shuixian Yan, Genjiao Zhou, Transmission dynamics and optimal control of a Huanglongbing model with time delay, 2021, 18, 1551-0018, 4162, 10.3934/mbe.2021209 | |
3. | Nabeela Anwar, Shafaq Naz, Muhammad Shoaib, Reliable numerical treatment with Adams and BDF methods for plant virus propagation model by vector with impact of time lag and density, 2022, 8, 2297-4687, 10.3389/fams.2022.1001392 | |
4. | Pushpendra Kumar, Dumitru Baleanu, Vedat Suat Erturk, Mustafa Inc, V. Govindaraj, A delayed plant disease model with Caputo fractional derivatives, 2022, 2022, 2731-4235, 10.1186/s13662-022-03684-x | |
5. | Benito Chen-Charpentier, Delays in Plant Virus Models and Their Stability, 2022, 10, 2227-7390, 603, 10.3390/math10040603 | |
6. | Muhammad Farman, Rabia Sarwar, Ali Akgul, Modeling and analysis of sustainable approach for dynamics of infections in plant virus with fractal fractional operator, 2023, 170, 09600779, 113373, 10.1016/j.chaos.2023.113373 | |
7. | Benito Chen-Charpentier, A Model of Hepatitis B Viral Dynamics with Delays, 2024, 4, 2673-9909, 182, 10.3390/appliedmath4010009 | |
8. | Benito Chen-Charpentier, SIRS Epidemic Models with Delays, Partial and Temporary Immunity and Vaccination, 2024, 4, 2673-9909, 666, 10.3390/appliedmath4020036 | |
9. | Sahabuddin Sarwardi, Hasanur Mollah, Aeshah A. Raezah, Fahad Al Basir, Direction and stability of Hopf bifurcation in an eco-epidemic model with disease in prey and predator gestation delay using Crowley-Martin functional response, 2024, 9, 2473-6988, 27930, 10.3934/math.20241356 | |
10. | Benito Chen-Charpentier, Delays and Exposed Populations in Infection Models, 2023, 11, 2227-7390, 1919, 10.3390/math11081919 | |
11. | Kimben M. Gonzales, Juancho A. Collera, Effects of vector preferential settling in the dynamics of a plant virus model with latent periods, 2025, 24058440, e42707, 10.1016/j.heliyon.2025.e42707 |
Parameters | Description | Values (unit) |
r | growth rate of plant density | 0.1 day−1 |
k | maximum plant density | 1 m−2 |
λ | infection rate of plant | 0.4 vector−1 day−1 |
m | infected plant removal rate | 0.1 day−1 |
d | additional death due to infection | 0.025 day−1 |
b | growth rate of infected vector | 0.4 day−1 |
μ | mortality rate of vector | 0.1 day−1 |
a | resistance rate of plant | 0.5 m 2 |
c | crowding effect of vector | 0.5 plant |
Parameters | Description | Values (unit) |
r | growth rate of plant density | 0.1 day−1 |
k | maximum plant density | 1 m−2 |
λ | infection rate of plant | 0.4 vector−1 day−1 |
m | infected plant removal rate | 0.1 day−1 |
d | additional death due to infection | 0.025 day−1 |
b | growth rate of infected vector | 0.4 day−1 |
μ | mortality rate of vector | 0.1 day−1 |
a | resistance rate of plant | 0.5 m 2 |
c | crowding effect of vector | 0.5 plant |