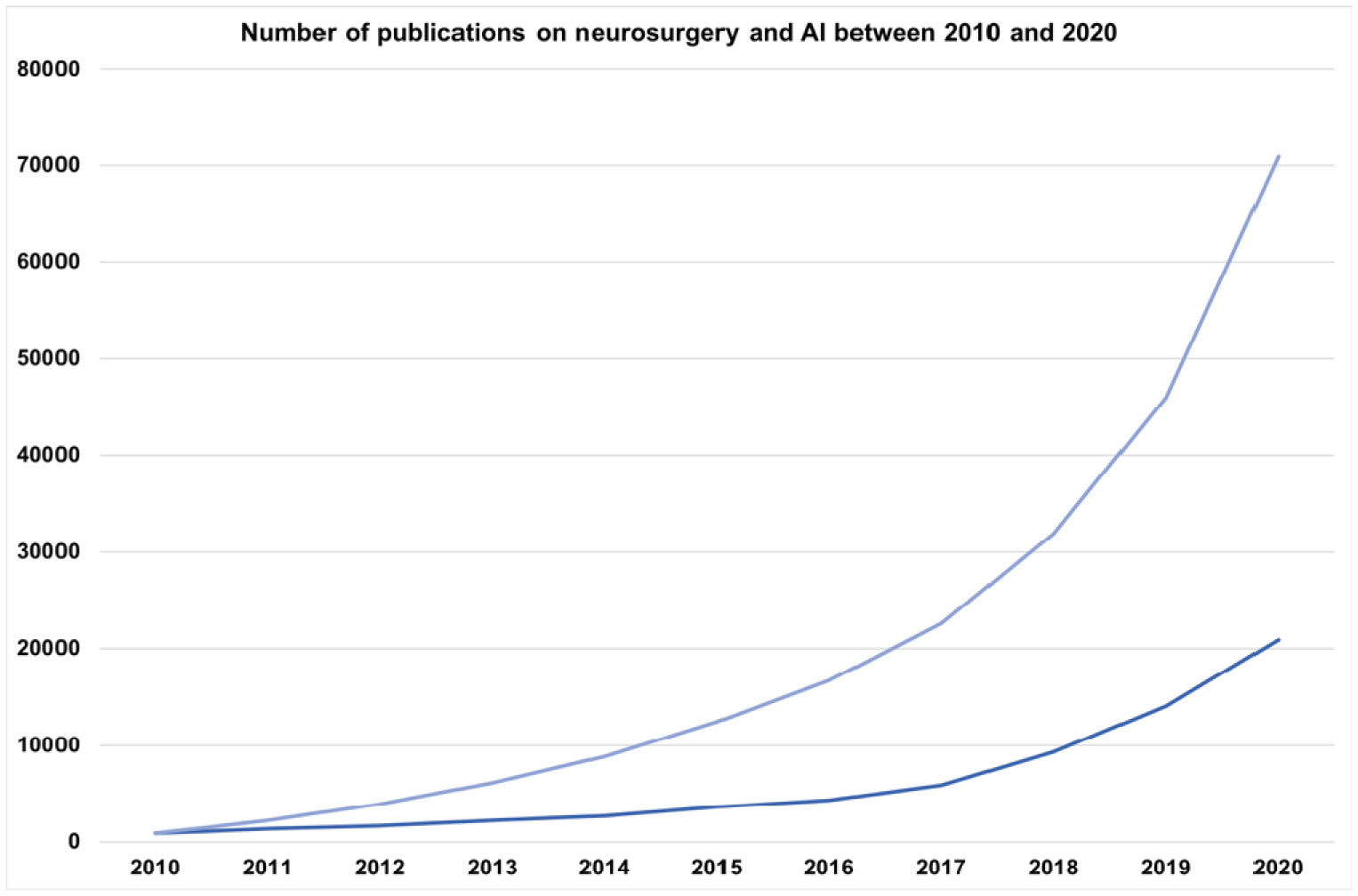
Citation: Francesca Verrilli, Hamed Kebriaei, Luigi Glielmo, Martin Corless, Carmen Del Vecchio. Effects of selection and mutation on epidemiology of X-linked genetic diseases[J]. Mathematical Biosciences and Engineering, 2017, 14(3): 755-775. doi: 10.3934/mbe.2017042
[1] | Elena Vlamou, Basil Papadopoulos . Fuzzy logic systems and medical applications. AIMS Neuroscience, 2019, 6(4): 266-272. doi: 10.3934/Neuroscience.2019.4.266 |
[2] | Barbara Tomasino, Dario Marin, Cinzia Canderan, Marta Maieron, Miran Skrap, Raffaella Ida Rumiati . Neuropsychological Patterns Following Lesions of the Anterior Insula in a Series of Forty Neurosurgical Patients. AIMS Neuroscience, 2014, 1(3): 225-244. doi: 10.3934/Neuroscience.2014.3.225 |
[3] | Zinia Pervin, Julia M Stephen . Effect of alcohol on the central nervous system to develop neurological disorder: pathophysiological and lifestyle modulation can be potential therapeutic options for alcohol-induced neurotoxication. AIMS Neuroscience, 2021, 8(3): 390-413. doi: 10.3934/Neuroscience.2021021 |
[4] | Akshay Reddy, Mohammad Reza Hosseini, Aashay Patel, Ramy Sharaf, Vishruth Reddy, Arman Tabarestani, Brandon Lucke-Wold . Deep brain stimulation, lesioning, focused ultrasound: update on utility. AIMS Neuroscience, 2023, 10(2): 87-108. doi: 10.3934/Neuroscience.2023007 |
[5] | Naeimeh Akbari-Gharalari, Maryam Ghahremani-Nasab, Roya Naderi, Leila Chodari, Farshad Nezhadshahmohammad . The potential of exosomal biomarkers: Revolutionizing Parkinson's disease: How do they influence pathogenesis, diagnosis, and therapeutic strategies?. AIMS Neuroscience, 2024, 11(3): 374-397. doi: 10.3934/Neuroscience.2024023 |
[6] | I. Prieto, A.B. Segarra, M. de Gasparo, M. Ramírez-Sánchez . Neuropeptidases, Stress, and Memory—A Promising Perspective. AIMS Neuroscience, 2016, 3(4): 487-501. doi: 10.3934/Neuroscience.2016.4.487 |
[7] | Arianna Polverino, Pierpaolo Sorrentino, Matteo Pesoli, Laura Mandolesi . Nutrition and cognition across the lifetime: an overview on epigenetic mechanisms. AIMS Neuroscience, 2021, 8(4): 448-476. doi: 10.3934/Neuroscience.2021024 |
[8] | Adi Wijaya, Noor Akhmad Setiawan, Asma Hayati Ahmad, Rahimah Zakaria, Zahiruddin Othman . Electroencephalography and mild cognitive impairment research: A scoping review and bibliometric analysis (ScoRBA). AIMS Neuroscience, 2023, 10(2): 154-171. doi: 10.3934/Neuroscience.2023012 |
[9] | Sarah Ly, Nirinjini Naidoo . Neuroenhancement and the Developing Brain: Commentary on the AIMS Neuroscience Special Issue on “Neuroenhancers”. AIMS Neuroscience, 2015, 2(4): 229-235. doi: 10.3934/Neuroscience.2015.4.229 |
[10] | Shun Sakuma, Yuko Mizuno-Matsumoto, Yoshi Nishitani, Shinichi Tamura . Learning Times Required to Identify the Stimulated Position and Shortening of Propagation Path by Hebb’s Rule in Neural Network. AIMS Neuroscience, 2017, 4(4): 238-253. doi: 10.3934/Neuroscience.2017.4.238 |
Neurosurgery is a demanding profession. Successful neurosurgeons require extensive training, stamina, a high degree of manual dexterity, excellent hand-eye coordination, intelligent decision making, leadership and organisational skills, compassion, communication skills and teamwork [1]. The boundaries and outcomes of surgeries are in part limited by the skills of the operating surgeons [2], rendering variabilities in the outcomes and experience of patients undergoing the same operation within different settings. While successful operations can benefit patients, errors can have undesired outcomes and, sometimes, harmful consequences. For instance, about a quarter of medical errors occurring in neurosurgery are technical errors related to the surgical procedures [3] that can be prevented, emphasising the importance of pragmatic steps to improve the successful outcome of neurosurgical interventions and reducing associated errors to deliver the best possible care for patients. Recent advances in technology have bridged the gap between humans and machines and have enabled computers to mimic, and even outperform, natural human intelligence to create what is called “artificial intelligence” (AI).
Since Kwoh and colleagues used the first robotic procedure in the modern era for computerised tomography (CT)-guided stereotactic brain surgery in 1988 [4], neurosurgeons have always been at the forefront of using cutting-edge technologies to deliver the best possible care for their patients. Noninvasive visualisation techniques such as CT scanning, magnetic resonance imaging (MRI) combined with image guiding, stereotactic surgery, and electrical stimulation have been essential components of neurosurgery within and out of operating rooms [5],[6]. Multiple technologically advanced methods such as self-positioning microscopes and endoscopes or automatic imaging guidance procedures have been used to improve patient outcomes and to reduce the procedural errors associated with neurosurgery [7]–[9]. As the overall life expectancy and the global population levels are rising [10]–[12], the demand for healthcare increases; however, the capacity of healthcare systems cannot address the surge in demand by solely relying on the human capital. The “crisis in human resources” is one of the most significant challenges of the healthcare sector in the recent era [13]. Furthermore, the ease of access to various healthcare services have caused a huge surge in the production and storage of clinical data such as imaging, genomics, and health monitoring [14]. For example, 80% of about 15 million global cancer diagnoses require surgery [15], which, in turn, each requires pre-, intra-, and postoperative clinical data collection, processing, interpretation and storage by human.
More than 13.8 million patients undergo a neurosurgical procedure globally every year [16]. Although it is estimated that there has been a global growth in the number of neurosurgeons to about 50,000 neurosurgeons currently [17], more than 5 million individuals in low- and middle-income countries with treatable neurosurgical conditions remain untreated annually [16], creating a huge gap between essential neurosurgery required and current neurosurgeries performed. Approximately 23,000 neurosurgeons are required to address global deficiencies in neurosurgery, particularly in low- and middle-income countries [16]. However, training neurosurgeons is competitive, lengthy, expensive and requires highly committed mentors and advanced surgical equipment [18]–[21]. These statistics are concerning and require sustainable and thorough solutions to address global health concerns.
Machines, algorithms and AI do not face mental and physical fatigue and can function 24/7, therefore, have a higher safety standard compared to humans. Additionally, machines have a greater capacity to learn and identify patterns that are not obvious to humans [22], thereby demonstrating hidden connections which are hard to discern [23]. Interpretation of clinical data, especially radiological images, can be subjective and qualitative [24],[25] causing heterogeneity of diagnoses among physicians and, in some cases, poor prognosis. AI can benefit neurosurgeons by reducing errors in the surgical arena, reducing costs associated with diagnosis, treatment and prognosis, expanding access to high-quality medical care as well as providing patients with increased autonomy in their own decision-making processes.
The use of technology, especially AI and robotics, in medicine and surgical interventions has been increasing over the past decade [26]–[28]. A search in the database PubMed showed a sustained increasing trend in the number of publications involving neurosurgery and artificial intelligence over the past decade (Figure 1).
While AI can benefit multiple parties such as surgeons, healthcare workers, the overall healthcare system and patients [29], in this review, I mainly focus on the benefits of AI on neurosurgeons while briefly mentioning the wider aspect of AI on the healthcare system.
AI, machine learning (ML) and deep learning (DL) have the potential to transform neurosurgery. AI aims to simulate the behaviour of intelligent beings in computers, whereas ML as a subdomain of AI combines computer science and statistics to enable computers to learn patterns by direct studying of data through experience, autonomous of external programming [30],[31] (Figure 2). Our brain changes as we grow, and so does ML as it trains. In its essence, ML is acting similar to medical students and resident doctors to learn rules from data and to apply general rules to various patients in each case with one chief difference- doing these on a huge scale with an enormous amount of data [22] (Figure 2). ML in medical sciences mainly uses supervised learning through training algorithms such as logistic regression, support vector machine and random forests [25].
AI can improve the accuracy of diagnosis and treatment in neurosurgery and provide neurosurgeons with effective and efficient tools in a timely manner during pre-, intra- and postoperative care. AI can spot subtle abnormalities and malformations from neuroradiological images and clinical data which are not discernible to trained eyes. Deep learning as a subset of ML is based on neural networks, which include multiple layers of learning algorithms [32] (Figure 3).
Patients can experience highly variable outcomes for the same neurosurgical treatments depending on various underlying reasons such as the experience of the operating surgeon, variations in the operating approach and a lack of clear national or international guidelines for common treatments, financial reasons, annual case volume, geographical location and the nature of the practice (academic, private, etc.) [33]–[37]. It is worth mentioning that other factors influencing the patient outcome in neurosurgical interventions can be patient-specific variations such as age, sex, comorbidities, alcohol and tobacco consumption, body mass index, psychological conditions, and etc. [37]. Considering all these variables to reach a decision for the best surgical intervention can be beyond the capacity of a human being. AI can reduce variations in patient outcome by reducing the heterogeneity of the care via providing guidelines to create consensus among neurosurgeons on surgical interventions, thereby improving prognosis and reducing costs.
AI can improve the patient outcomes in neurosurgery in pre-, intra- and postoperative domains as well as neurosurgical research, training and expanding access to high-quality treatments (Figure 4).
In the pre-operative phase of neurosurgery, AI can assist surgeons in diagnosing the condition, selecting patients for the right treatment, and helping patients to make the right decisions [31]. In the intra-operative phase of neurosurgery, AI can enhance the performance of surgeons and reduce errors associated with neurosurgery. In postoperative care, AI can predict prognosis, identify potential postoperative complications and track data for better aftercare and recovery. As such, by making better predictions in the postoperative phase, pre-operative planning can be enhanced to improve patient care and reduce the associated costs. For example, ML can be used for classification, regression and clustering to analyse large data sets, identify risk factors and predict surgical complications such as cardiac complications, wound complications and mortality among patients following a cervical discectomy and posterior lumbar spine fusion [38]–[40]. Research in neurosurgery can benefit from AI by helping researchers to collect, analyse, process, and disseminate data. Since available financial means can be an obstacle for delivering the best possible treatment, AI can facilitate access to high-quality care in less developed areas through teleoperation. I am going to discuss each of these domains below.
High-resolution and ubiquitous radiological imaging combined with electrophysiological data are methods of choice that have provided neurosurgeons with unprecedented and noninvasive intracranial access. Manual decision making in neurosurgical medicine requires neurosurgeons to study, retain, analyse and interpret a large quantity of complicated and dynamic data. Neurosurgeons usually rely on their experience and clinical evidence to make a decision and provide a prognosis [41],[42]. Regardless of how well-trained or experienced a neurosurgeon is, manual handling of such information can be, at its very least, challenging for the human capacity. Furthermore, the sheer volume of data combined with the urge to make a rapid and accurate diagnosis, the presence of atypical cases, and lack of access to trained radiologists can pose bottlenecks for the accurate and timely diagnosis of different conditions manually by trained physicians and be resource-, time-, and labour intensive. As such, errors and discrepancies can be as common as 3–5% in radiology [43]. In addition, time is of crucial importance in neurosurgical interventions, and reducing the door-to-needle times for emergency neurosurgical interventions has been a goal for many clinical settings [44].
Some challenges in neurosurgical care can be circumvented by computer-assisted diagnosis (CAD) and AI. By using a vast amount of anatomical, morphological and connectivity information, AI and CAD can significantly help neuroradiologists and neurosurgeons to make effective and efficient diagnoses, accelerating the triage and hence the workflow to initiate the treatment, reducing the human labour as well as the costs [45]. Multiple studies have demonstrated that door-to-needle times play a crucial role in reducing mortality and improving the prognosis, and obtaining and interpreting radiological images, and the lack of access to neurologists are the major reasons causing delays in delivering emergency treatments for conditions such as stroke [46]–[48]. AI can be used to accelerate brain imaging acquisition and interpretation that can be crucial in assisting clinicians in improving the accuracy of their diagnosis [49],[50]. Additionally, AI can be used independently for automatic classification of epilepsy type with 60% AI accuracy compared with 62% clinician accuracy [51], predicting tumour type with 86% AI accuracy [52], predicting glioma with 86% accuracy [53], diagnosing acute ischaemic events with 56% accuracy [54], and cerebral aneurysms with more than 90% accuracy [55].
In pre-operative planning, AI algorithms have been used for automatic tumour segmentation [41],[56], epileptogenic zone localisation [51], selecting appropriate candidates for epileptic surgery [57], predicting symptomatic cerebral vasospasm after aneurysmal subarachnoid haemorrhage [58], and predicting tissue damage following acute ischaemic stroke [59]. For example, classification of epilepsy and tumour can be subjective [50],[60], therefore causing differences in the decision-making of neurosurgeons. By providing a robust framework and outline, algorithms using AI can reduce the subjective interpretation of the data and thereby diagnose conditions requiring neurosurgical procedures.
Effective and efficient identification of glioma tissue is a crucial step in pre-operative planning and can be dependent on the anatomical knowledge of neuroradiologists and neurosurgeons, and therefore, time-consuming and dependent on the operator variation [61]–[64]. While glioma resection can extend patients' survival, it can have high postoperative risk because gliomas can be found in functional areas which control various functions such as sensory, motor, vision and language [65]. AI was successfully used for MRI-based segmentation of glioma and brainstem tumours and did similarly or outperformed physicians [41],[56],[66].
Classification is another task that can be performed by ML. For example, lumbar disk degeneration can be classified using MRI scans from healthy to severely abnormal disks [67]. ML can cluster patients suffering from osteoporotic vertebral fracture based on their pain progression [68], thereby helping their management. ML helped diagnosing paediatric posterior fossa tumours by categorising them into the primitive neuroectodermal tumour, astrocytoma, or ependymoma with 72% accuracy compared to 73% accuracy of neuroradiologists [69] and in other cases with better accuracy than the neuroradiologists [64], as well as classifying intra-axial cerebral tumours including high- and low-grade gliomas, metastatic tumours and malignant lymphomas and sellar-suprasellar masses by significantly increasing the diagnostic classification of tumours by radiologists [50],[70]. Other studies showed that ML and artificial neural network predicted the glioma according to the World Health Organization (WHO) grade better than radiologists [41],[71]–[73]. Beyond tumour diagnosis, ML outperformed physicians with 82.2% to 62.2% accuracy in predicting the presence of abnormal features in CT scans of paediatric TBI patients [74].
AI outperformed physicians (95.8% compared to 66.7%) for lateralising the affected brain hemisphere in the most common pharmacoresistant and surgically remediable type of epilepsy in adults, temporal lobe epilepsy (TLE), using functional MRI data [75]. This can significantly increase the patient outcome as unclear localisation of the epileptogenic zone can be a significant barrier to allocating eligible patients to appropriate surgeries [76].
There are other examples where AI was used in the diagnosis and classification of neurosurgical diseases without radiological input [74],[77]. For example, AI showed significantly higher accuracy in discriminating between single cells vs multiunit spike clusters from electroencephalography recordings of 12 epilepsy patients requiring implantation of chronic intracranial depth electrodes [77]. Since AI is capable of using multiple variables simultaneously, an attribute that is beyond the capacity of a human operator, it can consider multiple factors when planning the treatment. To this end, a study generated an artificial neural network with 11 clinical inputs to train the algorithm for predicting the survival of TBI patients [78]. ML had a better performance in accuracy and sensitivity and was more specific compared to neurosurgeons and neurosurgery residents [78].
AI can predict the progression of a disease. For example, MRI data from a large, multiinstitutional dataset was used to train deep learning algorithms to replace invasive tissue sampling and predict the progression of glioma noninvasively [79],[80], demonstrating the potential of ML in enhancing capitalisation of the existing data.
The current conventional workflow of intraoperative tissue biopsy where the tissue is being transported to a laboratory, it is processed, and specimen are prepared by highly skilled laboratory personnel before being interpreted by pathologists [81] is time-, labour-and resource-intensive and dates back more than a century old [82]. To provide an example of the use of AI in the intra-operative phase of neurosurgery, Hollon et al. [81] have developed a label-free optical imaging workflow to automatically predict diagnosis in near real-time. Their tumour diagnosis methods can predict the diagnosis of tumours in under 150 seconds compared to conventional methods described earlier, which can take 30 minutes [83], with an overall accuracy of 95%, which slightly outperformed conventional histology workflow with an accuracy of 94% [81].
Since patients might require multiple visits to various geographical locations such as outpatient clinics, inpatient wards, pharmacies, emergency departments, intensive care units, and laboratories, telemedicine can reduce the unnecessary travel by both healthcare professionals and patients [84],[85]. Telemedicine is positively valued by patients and healthcare providers and can improve patient outcomes during postoperative care, especially in geographically restricted areas [86]–[88]. Postoperative videoconferencing was welcomed by the majority of patients and was comparably as safe and as effective compared with in-person clinic visits among patients who had elective neurosurgery [87]. As such, telemedicine can be used for follow-up visits which would have required in-person clinic visits, remote diagnosis of postsurgery complications, as well as continued routine monitoring [89]. In comparison, the use of AI in postoperative care and follow-up of neurosurgery is less agreeable among surgeons and surgical teams compared to other usages such as pre-operative planning and image interpretation [90].
These examples clearly demonstrate the power of AI, ML and DL in pre-, intra- and postoperative phases of neurosurgical diseases. Future work requires integration of AI and ML models to combine pre-, intra- and postoperative algorithms into a single model where the best pre-operative planning, intra-operative surgical intervention, and postoperative follow-up work with associated risk, financial cost, and considerations can be suggested to surgeons. Such algorithms not only can benefit neurosurgeons in their decision-making but also facilitate the delivery of high-quality healthcare to low resources settings and facilitate personalised surgical and postsurgical care.
A number of efforts, such as increasing the capacity of neurosurgical training, can address the disparity associated between the demand and the availability of neurosurgical care discussed earlier in this review; however, such disparities in healthcare still exist.
The COVID-19 pandemic demonstrated the vulnerability of the healthcare systems around the globe. Elective neurosurgeries were cancelled to free up staff and beds for the critical care of pulmonary COVID-19 patients, in addition to a reduced capacity in teaching and training neurosurgery [91]. One of the important lessons that can be learnt to strengthen the healthcare system is the development of telemedicine and teleoperating by incorporating AI in neurosurgery. Robotic surgery with the help of AI can be used to facilitate patient management and surgical operation during the time of increased demand on the healthcare systems such as pandemics [92]. However, the use of teleoperating and telesurgery should not remain restricted to exceptional circumstances, such as infectious diseases pandemic, and it can be extended to deliver high-quality surgical procedures to rural and less accessible areas, battlefields and difficult terrains. Delivery of surgical interventions for patients in situ can minimise the risk and cost associated with patient transfer to large medical centres and decentralising resources available.
There is a clear global disparity in the availability of neurosurgical care, which can result in preventable disease, disability, and death. For instance, while around 7500 neurosurgeons in Japan take care of the neurosurgical needs of the population, it has been estimated that thirty-nine countries around the globe do not have a single practising neurosurgeon [17]. In some low- and middle-income countries, patients must travel in excess of 2 hours to receive emergency neurosurgical care, which itself has a lower quality of care compared to high-income countries [93]. Teleneurosurgery, with the help of AI, can address some of the existing disparities in accessing high-quality healthcare.
Smartphone apps can provide patients with easy access to healthcare providers as well as helping people with behavioural changes to educate patients to improve their general health and well-being and help them monitor their health such as weight management, smoke cessation [84]. Such mobile phone apps can be used for postoperative neurosurgical follow-up. Indeed, AI can be further used to develop mobile phones app for the early detection of other diseases and conditions of the nervous system, such as stress, anxiety and depression, among specific and targeted populations [94] to provide a better prognosis for patients.
Although doctors try to deliver the best possible treatment for their patients, access to high-quality medical care can be restrained by economic means [95], resulting in disparities in access to appropriate healthcare. AI, telemedicine, teleoperating and telesurgery can help ameliorate some of these disparities.
We live in an era that has been named the age of information [96]. One of the major challenges associated with the age of information is the processing and storage of such a vast amount of clinical data that was briefly discussed before. AI and artificial neuronal network (Figure 5) can be useful tools to helps us comprehend the complexity of the nervous system. With the arrival of more data processing power and accumulation of data, AI has become more successful in surgical research in the past decade. Technology is beginning to demystify the notion of “mind control”. Brain-computer interface (BCI) and AI can be combined to restore some of the sensory and motor functions of patients with paralysis, expand the motor ability of healthy people and facilitate the development of next-generation robots [97],[98]. Currently, about 169,000 people live in the United States of America with traumatic spinal cord injury causing tetraplegia [99]. In these patients, the cortex still generates neuronal activity for limb movement, but the firing is not passed to the limbs due to spinal injuries. Signals can be acquired noninvasively from the scalp using electroencephalography (EEG) and processed to control robotic arms [97].
AI and ML can understand the firing of the cortex, and restore motor control by transmitting signals from the human motor cortex to muscles of a quadriplegic person with a C5/C6 cervical spinal cord injury, thereby providing a “neural bypass” [101]. A recent bidirectional BCI, which provides patients with tetraplegia with substantially improved control over the robotic arm by recording neural activity from the cortex and generating tactile sensation through intracortical stimulation of the somatosensory cortex [102], can have a substantial impact on the advancement of BCI and AI in neurosurgical treatment. Such combinations of AI and BCI had the advantage of providing a real-time adjustment for the desired task as the algorithms can learn from the past experience and help the users to an optimal outcome based on the previous task [103].
As AI becomes more prominent in neurosurgery, it can develop a mutual relationship where AI helps neurosurgery and vice versa. AI and ML have enabled data to speak for themselves and guide physicians to make better decisions [14],[26]. Furthermore, AI can help building and testing hypotheses as well as analysing clinical and experimental data. This frees up clinical researchers' valuable time from carrying out mundane analysis and help them focus on the bigger picture [26]. Furthermore, the accuracy of data analysis can be enhanced by minimising human error and bias [3].
Using AI in neurosurgery is not completely harmless. There can be negative primary and secondary consequences associated with over-reliance on AI in neurosurgery. At the primary level, hardware and software malfunctions can cause errors in the surgical procedures [6], as well as misinterpretations of clinical findings, laboratory reports, and image scans leading to mistakes in diagnosis. At the secondary level, over-reliance on AI for surgical interventions can discourage surgeons from learning skills required to master surgical techniques. Over-reliance on algorithms for the diagnosis and treatment of nervous system diseases can be problematic. For example, algorithms used in ML can overfit the data [22] to produce false positives or false negatives. However, such malfunctions and malperformance can be ameliorated by confirming the functionality of the algorithm using validation samples and independent data sets [22].
One of the concerns associated with the use of AI in the medical field has been that it will replace clinicians. It is of paramount importance to bear in mind that the patient is at the centre of medicine and benefit to patient should be the most important criterion in deciding whether AI can benefit medicine or not, AI should not replace the human, but it should work cooperatively with surgeons to complement their skills and improve their performance to provide the best possible care. Recent qualitative studies have shown that while patients and their relatives welcome the use of AI in neurosurgery and find it acceptable, they were reluctant to be treated by fully autonomous neurosurgeries and would like to have neurosurgeons to ultimately remain in control [104]. Similarly, most neurosurgeons welcome the use of AI in neurosurgery [90], so there is a consensus among neurosurgeons and patients on the use of AI in neurosurgery. Multiple studies have shown that the combination of AI with clinicians improved clinical decision making compared to clinicians or AI alone [50],[70],[105],[106]. As such, neurosurgeons will not be deskilled, but they can expand on their expertise to equip themselves with better techniques.
Another problem with the use of AI, and especially BCI, is that it is still very limited, and many practical challenges need to be overcome before the widespread usage of AI in restorative neurosurgery. Multiple challenges exist with respect to implant technology, implant recipients and implantation methodologies [107]. Furthermore, generating and training algorithms requires a vast amount of data [31]. This can be further complicated, given that access to most of the patient data is restricted due to privacy concerns [26]. ML is based on data acquisition and availability and any algorithm is as good as the data used to train it. Therefore, successful acquisition and implementation of data are essential for developing and training ML algorithms. Therefore, noisy and complicated data can itself pose a challenge to develop optimal AI algorithm.
Another challenge with the usage of AI in neurosurgery is the cost. However, while the initial training and operating of AI might have some costs, the long-term benefits of reducing surgeons' workload, increasing the handling of data, and reducing error can outweigh the initial cost. Furthermore, ML can reduce the cost associated with unnecessary testing and defensive medicine. However, this requires a better definition for different diseases and conditions.
Furthermore, the storage of a vast amount of data about our neuronal activity can provide abusers with a means to access information about conscious and subconscious behaviours, intentions, desires, and interests which can be used to manipulate human's behaviour [108], indicating development of ethical regulations in the usage of AI in neurosurgery [109].
AI is an interdisciplinary field in the interface of medicine, neuroscience, and engineering. Neurosurgery can harvest the power of AI to provide patients with the best outcome. AI has the capacity to improve surgeons' skill set in the pre-, intra- and postoperative arena in neurosurgery. Humans and machines can work cooperatively to harness the recent technological advances in AI to enhance the quality of healthcare delivery through image acquisition, processing and interpretation, allocating patients to appropriate surgeries, improving intra-operative work, providing postoperative follow-up and facilitating access to high-quality healthcare. The use of AI can be further expanded for neuromuscular and neurodegenerative diseases to treat conditions such as Parkinson's disease, which are currently treated by medication and deep brain stimulation [110], as well as understanding different aspects of molecular cell biology such as subcellular trafficking of cargoes in single neurons [111],[112].
The emergence of AI in neurosurgery requires careful regulation and monitoring to abide by ethical principles [109]. The use of AI in medical and surgical training should be incorporated early in undergraduate medical school to train the future generation of surgeons with the state of art technologies. Undergraduate medical students have an awareness of the potential of AI in medicine and are not concerned that AI will substitute human radiologists [113].
AI can be a gateway for personalised medicine in the future. Furthermore, as the acquisition of large clinical data is growing, precision medicine aims to establish quantitative models to predict the health outcome, prognosticate disease procedure, prevent diseases and minimise surgical complications [38]. Both personalised medicine and precision medicine can benefit from AI. Future widespread use of AI in neurosurgery requires further research, investment, and multidisciplinary collaborations.
[1] | [ M. AG and D. SS., The Metabolic and Molecular Bases of Inherited Disease, chapter Color vision and its genetic defects, 5955–76, McGraw-Hill, 2001. |
[2] | [ L. Allen,D. Thrasher, The effects of vaccination in an age-dependent model for varicella and herpes zoster, IEEE Transactions on Automatic Control, 43 (1998): 779-789. |
[3] | [ A. Aswani and C. Tomlin, Computer-aided drug discovery for pathway and genetic diseases, in Proc. 49th IEEE Conference on Decision and Control 2010, 2010,4709–4714 |
[4] | [ E. August, G. Craciun and H. Koeppl, Finding invariant sets for biological systems using monomial domination, in CDC, 2012,3001–3006 |
[5] | [ C. F. Baer, Does mutation rate depend on itself PLoS Biol, 6 (2008), e52. |
[6] | [ J. Becker,R. Schwaab,A. Möller-Taube,U. Schwaab,W. Schmidt,H. H. Brackmann,T. Grimm,K. Olek,J. Oldenburg, Characterization of the factor viii defect in 147 patients with sporadic hemophilia a: Family studies indicate a mutation type-dependent sex ratio of mutation frequencies, American Journal of Human Genetics, 58 (1996): 657-670. |
[7] | [ D. Bick, Engineering in genomics-genetic disease diagnosis: Challenges and opportunities, IEEE Engineering in Medicine and Biology Magazine, 14 (1995): 226-228. |
[8] | [ F. Blanchini,E. Franco,G. Giordano, Determining the structural properties of a class of biological models, CDC, 2 (2012): 5505-5510. |
[9] | [ D. J. Bowen, Haemophilia a and haemophilia b: Molecular insights, Molecular Pathology, 55 (2002): 1-18. |
[10] | [ C. Cannings, Equilibrium, convergence and stability at a sex-linked locus under natural selection, Genetics, 56 (1967): 613-618. |
[11] | [ J. Chunyan,J. Daqing,Y. Q. Yang,S. Ningzhong, Dynamics of a multigroup SIR epidemic model with stochastic perturbation, Automatica, 48 (2012): 121-131. |
[12] | [ J. F. Crow, How much do we know about spontaneous human mutation rates?, Environmental and Molecular Mutagenesis, 21 (1993), 122–129, URL http://dx.doi.org/10.1002/em.2850210205. |
[13] | [ J. F. Crow, The origins, patterns and implications of human spontaneous mutation, Nature Reviews Genetics, 1 (2000): 40-47. |
[14] | [ C. Del Vecchio, L. Glielmo and M. Corless, Equilibrium and stability analysis of x-chromosome linked recessive diseases model, in Proc. IEEE 51st Annual Conference on Decision and Control (CDC), 2012,4936–4941 |
[15] | [ C. Del Vecchio, L. Glielmo and M. Corless, Non linear discrete time epidemiological model for x-linked recessive diseases in 22nd Mediterranean Conference on Control and Automation, June 2014, Palermo, Italy 2014. |
[16] | [ A. W. F. Edwards, Foundations of Mathematical Genetics Cambridge University Press, 2000. |
[17] | [ W. J. Ewens, Mathematical Population Genetics Ⅰ: Theoretical Introduction, Springer, 2004. |
[18] | [ D. P. Germain, General aspects of x-linked diseases, in Fabry Disease: Perspectives from 5 Years of FOS, Oxford PharmaGenesis, 2006. |
[19] | [ H. Hethcote, The mathematics of infectious diseases, SIAM Review, 42 (2000): 599-653. |
[20] | [ http://www.istat.it/it/charts/popolazioneresidente, Authors' last visit on February 2015. |
[21] | [ http://www.who.int/genomics/public/geneticdiseases/en/index2.html, Authors' last visit on February 2015. |
[22] | [ C. Hyeygjeon,A. Astolfi, Control of HIV infection dynamics, IEEE Control Systems Magazine, 28 (2008): 28-39. |
[23] | [ B. J., Worldwide prevalence of red-green color deficiency., Journal of the Optical Society of America, 29 (2012): 313-320. |
[24] | [ M. J. Keeling and P. Rohani, Modeling Infectious Diseases in Humans and Animals Princeton University Press, 2008. |
[25] | [ M. J. Khoury, T. H. Beaty and B. H. Cohen, Fundamentals of Genetic Epidemiology, Oxford University Press, 1993. |
[26] | [ M. Lachowicz and J. Miekisz, From Genetics to Mathematics vol. 79 of Series on Advances in Mathematics for Applied Sciences, World Scientific Publications, 2009. |
[27] | [ K. Lange, Mathematical and Statistical Methods for Genetic Analysis, Second Edition, Springer, New York, 2002. |
[28] | [ K. Lange, Calculation of the equilibrium distribution for a deleterious gene by the finite fourier transform, Biometrics, 38 (1982): 79-86. |
[29] | [ K. Lange,B. Redelings, Disease gene dynamics in a population isolate, An Introduction to Mathematical Modeling in Physiology, Cell Biology, and Immunology: American Mathematical Society, Short Course, January 8-9,2001, New Orleans, Louisiana, 59 (2002): 119-138. |
[30] | [ D. Luenberger, Introduction to Dynamic Systems, John Wiley and Sons, 1979. |
[31] | [ T. Nagylaki, Selection and mutation at an x-linked locus, Annals of Human Genetics, 41 (1977): 241-248. |
[32] | [ T. Nagylaki, Introduction to Theoretical Population Genetics, Biomathematics, 21. Springer-Verlag, Berlin, 1992. |
[33] | [ H. A. Orr, Fitness and its role in evolutionary genetics, Nature reviews. Genetics, 10 (2009): 531-539. |
[34] | [ R. H. Post, Population differences in red and green color vision deficiency: A review, and a query on selection relaxation, Social Biology, 29 (1982): 299-315. |
[35] | [ D. B. Saakian,Z. Kirakosyan,C.-K. Hu, Diploid biological evolution models with general smooth fitness landscapes and recombination, Physical Review E, 77 (2008): 061907. |
[36] | [ G. D. Smith,S. Ebrahim,S. Lewis,A. L. Hansell,L. J. Palmer,P. R. Burton, Genetic epidemiology and public health: Hope, hype, and future prospects, The Lancet, 366 (2005): 1484-1498. |
[37] | [ A. E. Stark, Determining the frequency of sporadic cases of rare X-linked disorders, Annals of Translational Medicine, 4 (2016). |
[38] | [ B. Sturmfels, Polynomial equations and convex polytopes, The American Mathematical Monthly, 105 (1998): 907-922. |
[39] | [ J. M. Szucs, Selection and mutation at a diallelic X-linked locus, Journal of Mathematical Biology, 29 (1991): 587-627. |
[40] | [ R. M. Winter, Estimation of male to female ratio of mutation rates from carrier-detection tests in x-linked disorders, American Journal of Human Genetics, 32 (1980): 582-588. |
[41] | [ X. Xia, Estimation of HIV/AIDS parameters, Automatica, 39 (2003): 1983-1988. |
[42] | [ N. Yasuda,K. Kondô, No sex difference in mutations rates of duchenne muscular dystrophy, Journal of Medical Genetics, 17 (1980): 106-111. |
[43] | [ L. Yeghiazarian,M. Kaiser, Markov model of sex-linked recessive trait transmission, Mathematical and Computer Modelling, 29 (1999): 71-81. |
[44] | [ M. Yousefi,A. Datta,E. Dougherty, Optimal intervention in markovian gene regulatory networks with random-length therapeutic response to antitumor drug, Biomedical Engineering, IEEE Transactions on, 60 (2013): 3542-3552. |
1. | Mohammad Mofatteh, Mohammad Sadegh Mashayekhi, Saman Arfaie, Yimin Chen, Asfand Baig Mirza, Jawad Fares, Soham Bandyopadhyay, Edy Henich, Xuxing Liao, Mark Bernstein, Augmented and virtual reality usage in awake craniotomy: a systematic review, 2022, 46, 1437-2320, 10.1007/s10143-022-01929-7 | |
2. | Victor Gabriel El-Hajj, Maria Gharios, Erik Edström, Adrian Elmi-Terander, Artificial Intelligence in Neurosurgery: A Bibliometric Analysis, 2023, 171, 18788750, 152, 10.1016/j.wneu.2022.12.087 | |
3. | Mohamad Bydon, Sulaman Durrani, William Mualem, Commentary: Validation of Machine Learning-Based Automated Surgical Instrument Annotation Using Publicly Available Intraoperative Video, 2022, 23, 2332-4252, e158, 10.1227/ons.0000000000000285 | |
4. | Wireko Andrew Awuah, Jacob Kalmanovich, Aashna Mehta, Helen Huang, Rohan Yarlagadda, Mrinmoy Kundu, Matthew Nasato, Abdul-Rahman Toufik, Precious Peculiar Olatunbosun, Arda Isik, Vladyslav Sikora, Harnessing artificial intelligence to bridge the neurosurgery gap in low-income and middle-income countries, 2022, 0032-5473, postgradmedj-2022-141992, 10.1136/pmj-2022-141992 | |
5. | Javed Iqbal, Kainat Jahangir, Yusra Mashkoor, Nazia Sultana, Dalia Mehmood, Mohammad Ashraf, Ather Iqbal, Muhammad Hassan Hafeez, The future of artificial intelligence in neurosurgery: A narrative review, 2022, 13, 2152-7806, 536, 10.25259/SNI_877_2022 | |
6. | Pooria Sobhanian, Misagh Shafizad, Shaghayegh Karami, Fateme Mozaffari, Amirhossein Arab, Ghazal Razani, Paria Shafiekhani, Saeid Safari, Artificial Intelligence Applications in Clinical Neurosurgery, 2022, 2, 2772-8854, 10.5812/pmco-133563 | |
7. | Nilesh Harshit Barla, Shaeril Michael Almeida, Michael Sebastian Almeida, 2023, Chapter 9, 978-981-19-8295-8, 199, 10.1007/978-981-19-8296-5_9 | |
8. | Eun Kyung Jun, Sunghwan Lim, Joonho Seo, Kae Hong Lee, Jae Hee Lee, Deukhee Lee, Jae Chul Koh, Augmented Reality-Assisted Navigation System for Transforaminal Epidural Injection, 2023, Volume 16, 1178-7090, 921, 10.2147/JPR.S400955 | |
9. | Caroline E. Richburg, Lesly A. Dossett, Tasha M. Hughes, Cognitive Bias and Dissonance in Surgical Practice, 2023, 103, 00396109, 271, 10.1016/j.suc.2022.11.003 | |
10. | Mohammad Mofatteh, Mohammad Sadegh Mashayekhi, Saman Arfaie, Amos Olufemi Adeleye, Edward Olaoluwa Jolayemi, Nathalie C. Ghomsi, Nathan A. Shlobin, Ahmed A. Morsy, Ignatius N. Esene, Tsegazeab Laeke, Ahmed K. Awad, Jason J. Labuschagne, Richard Ruan, Yared Nigusie Abebe, John Nute Jabang, Abiodun Idowu Okunlola, Umaru Barrie, Hervé Monka Lekuya, Ehanga Idi Marcel, Kantenga Dieu Merci Kabulo, Nourou Dine Adeniran Bankole, Idara J. Edem, Chibuikem A. Ikwuegbuenyi, Stephane Nguembu, Yvan Zolo, Mark Bernstein, Awake Craniotomy in Africa: A Scoping Review of Literature and Proposed Solutions to Tackle Challenges, 2023, Publish Ahead of Print, 0148-396X, 10.1227/neu.0000000000002453 | |
11. | Jakub Horák, Martina Turková, J. Horák, A. Rolo, E. Dolejšová, Using artificial intelligence as business opportunities on the market: An overview, 2023, 160, 2261-2424, 01012, 10.1051/shsconf/202316001012 | |
12. | Mohammad Mofatteh, Mohammad Sadegh Mashayekhi, Saman Arfaie, Yimin Chen, Armaan K Malhotra, Mohammed Ali Alvi, Nicholas Sader, Violet Antonick, Mostafa Fatehi Hassanabad, Alireza Mansouri, Sunit Das, Xuxing Liao, Roger S McIntyre, Rolando Del Maestro, Gustavo Turecki, Aaron A Cohen-Gadol, Gelareh Zadeh, Keyoumars Ashkan, Suicidal ideation and attempts in brain tumor patients and survivors: A systematic review, 2023, 5, 2632-2498, 10.1093/noajnl/vdad058 | |
13. | Seth B. Wilson, Jacob Ward, Vikas Munjal, Chi Shing Adrian Lam, Mayur Patel, Ping Zhang, David S. Xu, Vikram B. Chakravarthy, Machine Learning in Spine Oncology: A Narrative Review, 2024, 2192-5682, 10.1177/21925682241261342 | |
14. | Diana T. Mosa, Amena Mahmoud, John Zaki, Shaymaa E. Sorour, Shaker El-Sappagh, Tamer Abuhmed, Sathishkumar V E, Henry gas solubility optimization double machine learning classifier for neurosurgical patients, 2023, 18, 1932-6203, e0285455, 10.1371/journal.pone.0285455 | |
15. | Nathan A. Shlobin, Gail Rosseau, Opportunities and Considerations for the Incorporation of Artificial Intelligence into Global Neurosurgery: A Generative Pretrained Transformer Chatbot-Based Approach, 2024, 186, 18788750, e398, 10.1016/j.wneu.2024.03.149 | |
16. | Mohamed L. Seghier, 7 T and beyond: toward a synergy between fMRI-based presurgical mapping at ultrahigh magnetic fields, AI, and robotic neurosurgery, 2024, 8, 2509-9280, 10.1186/s41747-024-00472-y | |
17. | Xin Li, Zhao-ying Dong, Meng Dong, Lei Chen, Early dopaminergic replacement treatment initiation benefits motor symptoms in patients with Parkinson's disease, 2024, 18, 1662-5161, 10.3389/fnhum.2024.1325324 | |
18. | Benjamin S. Hopkins, Michael B. Cloney, Ekamjeet S. Dhillon, Pavlos Texakalidis, Jonathan Dallas, Vincent N. Nguyen, Matthew Ordon, Najib El Tecle, Thomas C. Chen, Patrick C. Hsieh, John C. Liu, Tyler R. Koski, Nader S. Dahdaleh, Using machine learning and big data for the prediction of venous thromboembolic events after spine surgery: A single-center retrospective analysis of multiple models on a cohort of 6869 patients, 2023, 14, 0974-8237, 221, 10.4103/jcvjs.jcvjs_69_23 | |
19. | Kassandra Roger, Phetsamone Vannasing, Julie Tremblay, Maria L. Bringas Vega, Cyralene P. Bryce, Arielle Rabinowitz, Pedro Antonio Valdes-Sosa, Janina R. Galler, Anne Gallagher, Early childhood malnutrition impairs adult resting brain function using near-infrared spectroscopy, 2024, 17, 1662-5161, 10.3389/fnhum.2023.1287488 | |
20. | Inibehe Ime Okon, Aymar Akilimali, Nathan Simbarashe Chisvo, Bareq Sabeeh Al-Lami, Lorraine Arabang Sebopelo, Tolulope Judah Gbayisomore, Daniel Stephen Masunga, Agbeniga Elijah Temitope, Kwadwo Antwi Boasiako Nkansah-Poku, Emmanuel Emmanuel Edet, Okesanya Olalekan John, Samuel Chukwuebuka Ewelike, Ibrahim Muhammad Bello, Fadele Kehinde Precious, Ugwu Onyedika Mitchell, Michael Omo Erhayanmen, Don Eliseo Lucero-Prisno III, Strengthening neurosurgical training programs in Sub-saharan Africa: a medical student’s perspective, 2024, 47, 1437-2320, 10.1007/s10143-024-02469-y | |
21. | Tej D. Azad, Anmol Warman, Jovanna A. Tracz, Liam P. Hughes, Brendan F. Judy, Timothy F. Witham, Augmented reality in spine surgery – past, present, and future, 2024, 24, 15299430, 1, 10.1016/j.spinee.2023.08.015 | |
22. | Shinya Watanabe, Yasushi Shibata, Eiichi Ishikawa, Idiopathic Normal Pressure Hydrocephalus With Multiple Sulcus Enlargements but No Ventricular Enlargement Resembling Brain Atrophy Over a Long Period Before Diagnosis: A Case Report, 2023, 2168-8184, 10.7759/cureus.49757 | |
23. | Shuhei Morita, Shunji Asamoto, Haruki Sawada, Kota Kojima, Takashi Arai, Nobuhiko Momozaki, Jun Muto, Takakazu Kawamata, The Future of Sustainable Neurosurgery: Is a Moonshot Plan for Artificial Intelligence and Robot-Assisted Surgery Possible in Japan?, 2024, 192, 18788750, 15, 10.1016/j.wneu.2024.08.126 | |
24. | Wireko Andrew Awuah, Favour Tope Adebusoye, Jack Wellington, Lian David, Abdus Salam, Amanda Leong Weng Yee, Edouard Lansiaux, Rohan Yarlagadda, Tulika Garg, Toufik Abdul-Rahman, Jacob Kalmanovich, Goshen David Miteu, Mrinmoy Kundu, Nikitina Iryna Mykolaivna, Recent Outcomes and Challenges of Artificial Intelligence, Machine Learning, and Deep Learning in Neurosurgery, 2024, 23, 25901397, 100301, 10.1016/j.wnsx.2024.100301 | |
25. | Sean D. Jeffries, Eric D. Pelletier, Kevin Song, Zheyan Tu, Avinash Sinha, Thomas M. Hemmerling, Recognition of Vocal Cords During Videolaryngoscopy Based on State-of-the-Art YOLO-V8 Architecture, 2024, 0003-2999, 10.1213/ANE.0000000000007283 | |
26. | Raivat Shah, Vanessa Reese, Martin Oselkin, Stanislaw P. Stawicki, 2023, Chapter 10, 978-1-83768-272-0, 10.5772/intechopen.113034 | |
27. | Daniel N. de Souza, Mitchell Jarmol, Carter A. Bell, Christina Marini, Laura J. Balcer, Steven L. Galetta, Scott N. Grossman, Precision Concussion Management: Approaches to Quantifying Head Injury Severity and Recovery, 2023, 13, 2076-3425, 1352, 10.3390/brainsci13091352 | |
28. | Arun Rabindra Katwaroo, Vivek Shanker Adesh, Amrita Lowtan, Srikanth Umakanthan, The diagnostic, therapeutic, and ethical impact of artificial intelligence in modern medicine, 2024, 100, 0032-5473, 289, 10.1093/postmj/qgad135 | |
29. | George Bcharah, Nithin Gupta, Nicholas Panico, Spencer Winspear, Austin Bagley, Morgan Turnow, Randy D'Amico, Alvan-Emeka K. Ukachukwu, Innovations in Spine Surgery: A Narrative Review of Current Integrative Technologies, 2024, 184, 18788750, 127, 10.1016/j.wneu.2023.12.124 | |
30. | Rohit Prem Kumar, Vijay Sivan, Hanin Bachir, Syed A. Sarwar, Francis Ruzicka, Geoffrey R. O'Malley, Paulo Lobo, Ilona Cazorla Morales, Nicholas D. Cassimatis, Jasdeep S. Hundal, Nitesh V. Patel, Can Artificial Intelligence Mitigate Missed Diagnoses by Generating Differential Diagnoses for Neurosurgeons?, 2024, 187, 18788750, e1083, 10.1016/j.wneu.2024.05.052 | |
31. | Gervith Reyes Soto, Daniel Alejandro Vega-Moreno, Carlos Catillo-Rangel, Alberto González-Aguilar, Oswaldo Alan Chávez-Martínez, Vladimir Nikolenko, Renat Nurmukhametov, Andreina Rosario Rosario , Ulises García-González, Alfonso Arellano-Mata, Mario Antonio Furcal Aybar, Manuel de Jesus Encarnacion Ramirez, Correlation of Edema/Tumor Index With Histopathological Outcomes According to the WHO Classification of Cranial Tumors, 2024, 2168-8184, 10.7759/cureus.72942 | |
32. | Artur Fabijan, Bartosz Polis, Robert Fabijan, Krzysztof Zakrzewski, Emilia Nowosławska, Agnieszka Zawadzka-Fabijan, Artificial Intelligence in Scoliosis Classification: An Investigation of Language-Based Models, 2023, 13, 2075-4426, 1695, 10.3390/jpm13121695 | |
33. | Lacey Marshall, Graham Lynn, Suhrud Pathak, Keyi Liu, Manoj Govindarajulu, Arun H. S. Kumar, Hanan Fahad Alharbi, Hemalatha Selvaraj, Muralikrishnan Dhanasekaran, 2024, Chapter 5, 978-981-97-2576-2, 87, 10.1007/978-981-97-2577-9_5 | |
34. | K.A. Magamaev, K.A. Magamaev, K.D. Deminskaya, K.K. Kadieva, I.R. Guseinov, A.S. Zolotar, A.P. Malevanets, E.E. Meteleva, M.M. Magomedov, E.A. Alekseeva, Application of robotic systems in neurosurgery: problems and prospects, 2024, 132, 10.18137/cardiometry.2024.31.132137 | |
35. | Harry Robertshaw, Lennart Karstensen, Benjamin Jackson, Alejandro Granados, Thomas C. Booth, Autonomous navigation of catheters and guidewires in mechanical thrombectomy using inverse reinforcement learning, 2024, 19, 1861-6429, 1569, 10.1007/s11548-024-03208-w | |
36. | Yosuke Sakai, Tetsuya Tsukada, Toru Watanabe, Yoshio Araki, Yukio Seki, Embolization of Ruptured Aneurysms in the Intracranial Peripheral Arteries Using N-Butyl Cyanoacrylate Glue: A Case Series, 2024, 2168-8184, 10.7759/cureus.71028 | |
37. | Tianshuo Yuan, Yingchuan Chen, Guanyu Zhu, Jianguo Zhang, The Related Factors and Effect of Electrode Displacement on Motor Outcome of Subthalamic Nuclei Deep Brain Stimulation in Parkinson’s Disease, 2023, 12, 2077-0383, 7561, 10.3390/jcm12247561 | |
38. | Aman Bhatia, Jessica Hanna, Tucker Stuart, Kevin Albert Kasper, David Marshall Clausen, Philipp Gutruf, Wireless Battery-free and Fully Implantable Organ Interfaces, 2024, 124, 0009-2665, 2205, 10.1021/acs.chemrev.3c00425 | |
39. | Yuki Kimoto, Naoki Tani, Takuto Emura, Takahiro Matsuhashi, Takuto Yamamoto, Yuya Fujita, Satoru Oshino, Koichi Hosomi, Hui Ming Khoo, Shimpei Miura, Takahiro Fujinaga, Takufumi Yanagisawa, Haruhiko Kishima, Beta-gamma phase-amplitude coupling of scalp electroencephalography during walking preparation in Parkinson’s disease differs depending on the freezing of gait, 2024, 18, 1662-5161, 10.3389/fnhum.2024.1495272 | |
40. | Clayton R. Baker, Matthew Pease, Daniel P. Sexton, Andrew Abumoussa, Lola B. Chambless, Artificial intelligence innovations in neurosurgical oncology: a narrative review, 2024, 169, 0167-594X, 489, 10.1007/s11060-024-04757-5 | |
41. | Jason M. Harley, Tiah Tawakol, Sayed Azher, Andrea Quaiattini, Rolando Del Maestro, The role of artificial intelligence, performance metrics, and virtual reality in neurosurgical education: an umbrella review, 2024, 3, 2731-4588, 10.1007/s44186-024-00284-z | |
42. | Shobha Mandal, Subhadeep Chakraborty, Muhammad Ayaz Tariq, Kamran Ali, Zenia Elavia, Misbah Kamal Khan, Diana Baltodano Garcia, Sofia Ali, Jubran Al Hooti, Divyanshi Vijay Kumar, Artificial Intelligence and Deep Learning in Revolutionizing Brain Tumor Diagnosis and Treatment: A Narrative Review, 2024, 2168-8184, 10.7759/cureus.66157 | |
43. | Khushi Saigal, Anmol Bharat Patel, Brandon Lucke-Wold, Artificial Intelligence and Neurosurgery: Tracking Antiplatelet Response Patterns for Endovascular Intervention, 2023, 59, 1648-9144, 1714, 10.3390/medicina59101714 | |
44. | Gaurav Mittal, Mayur Wanjari, Roshan Prasad, Tanisha Rathi, Tangmi Djabo Eric Adrien, Addressing gaps in neurosurgical training: a perspective on enhancing educational approaches, 2024, 47, 1437-2320, 10.1007/s10143-024-02854-7 | |
45. | Mert Marcel Dagli, Felix Conrad Oettl, Jaskeerat Gujral, Kashish Malhotra, Yohannes Ghenbot, Jang W Yoon, Ali K Ozturk, William C Welch, Clinical Accuracy, Relevance, Clarity, and Emotional Sensitivity of Large Language Models to Surgical Patient Questions: Cross-Sectional Study, 2024, 8, 2561-326X, e56165, 10.2196/56165 | |
46. | Marek Binder, Julia Papiernik, Inga Griskova-Bulanova, Sandra Frycz, Bartłomiej Chojnacki, Urszula Górska-Klimowska, Diagnosing awareness in disorders of consciousness with gamma-band auditory responses, 2024, 17, 1662-5161, 10.3389/fnhum.2023.1243051 | |
47. | Patricia Mae P. Limliman, Aryan Saphira B. Macua, Mary Clare Therese B. Solomon, John Raphael Y. Yu, Elmer P. Dadios, Ryan Rhay P. Vicerra, Argel B. Bandala, Ira C. Valenzuela, 2023, Global Neurobotics: Integration of Artificial Intelligence and Robotics in Neurosurgical Procedures in Improved Diagnosis and Treatment, 979-8-3503-8117-7, 1, 10.1109/HNICEM60674.2023.10589239 | |
48. | Mohammad Mofatteh, Mohammad Sadegh Mashayekhi, Saman Arfaie, Hongquan Wei, Arshia Kazerouni, Georgios P. Skandalakis, Ahmad Pour-Rashidi, Abed Baiad, Lior Elkaim, Jack Lam, Paolo Palmisciano, Xiumei Su, Xuxing Liao, Sunit Das, Keyoumars Ashkan, Aaron A. Cohen-Gadol, Awake craniotomy during pregnancy: A systematic review of the published literature, 2023, 46, 1437-2320, 10.1007/s10143-023-02187-x | |
49. | Liping Qi, Guo-Liang Wang, Zhi-Hao Tian, Shuo Guan, Shu-Ye Yang, Yu-Long Yang, Li-Qing Liu, Yong-Zhong Lin, Prefrontal cortical hemodynamics and functional network organization during Tai Chi standing meditation: an fNIRS study, 2023, 17, 1662-5161, 10.3389/fnhum.2023.1294312 | |
50. | Seth M. Meade, Sebastian Salas-Vega, Matthew R. Nagy, Swetha J. Sundar, Michael P. Steinmetz, Edward C. Benzel, Ghaith Habboub, A Pilot Remote Curriculum to Enhance Resident and Medical Student Understanding of Machine Learning in Healthcare, 2023, 180, 18788750, e142, 10.1016/j.wneu.2023.09.012 | |
51. | Eric Suero Molina, Antonio Di Ieva, 2024, Chapter 16, 978-3-031-64891-5, 265, 10.1007/978-3-031-64892-2_16 | |
52. | Corina Tamas, Flaviu Tamas, Attila Kovecsi, Georgiana Serban, Cristian Boeriu, Adrian Balasa, The Role of Ketone Bodies in Treatment Individualization of Glioblastoma Patients, 2023, 13, 2076-3425, 1307, 10.3390/brainsci13091307 | |
53. | Maria José Uparela-Reyes, Lina María Villegas-Trujillo, Jorge Cespedes, Miguel Velásquez-Vera, Andrés M. Rubiano, Usefulness of Artificial Intelligence in Traumatic Brain Injury: A Bibliometric Analysis and Mini-review, 2024, 188, 18788750, 83, 10.1016/j.wneu.2024.05.065 | |
54. | Kimia Kazemzadeh, Meisam Akhlaghdoust, Alireza Zali, Advances in artificial intelligence, robotics, augmented and virtual reality in neurosurgery, 2023, 10, 2296-875X, 10.3389/fsurg.2023.1241923 | |
55. | Yuan Wu, Qian Zhang, Yutong Deng, Xiang Ding, Han Xie, Shuang Wang, Chang Liu, Ming Li, Lixin Cai, Yuwu Jiang, Impact of epilepsy surgery on developmental trajectories of children under 3 years of age, 2024, 66, 0012-1622, 1215, 10.1111/dmcn.15873 | |
56. | Aaron Lawson McLean, Non-fungible token integration in neurosurgery: a technical review, 2023, 46, 1437-2320, 10.1007/s10143-023-02119-9 | |
57. | Rani G. Ahmad, Artificial intelligence and machine learning in neurosurgery: A review of diagnostic significance and treatment planning efficiency, 2023, 30, 1115-3474, 29, 10.4103/wajr.wajr_32_22 | |
58. | Clara F. Weber, Evelyn M. R. Lake, Stefan P. Haider, Ali Mozayan, Pratheek S. Bobba, Pratik Mukherjee, Dustin Scheinost, Robert T. Constable, Laura Ment, Seyedmehdi Payabvash, Autism spectrum disorder-specific changes in white matter connectome edge density based on functionally defined nodes, 2023, 17, 1662-453X, 10.3389/fnins.2023.1285396 | |
59. | Miranda X. Morris, Davide Fiocco, Tommaso Caneva, Paris Yiapanis, Dennis P. Orgill, Current and future applications of artificial intelligence in surgery: implications for clinical practice and research, 2024, 11, 2296-875X, 10.3389/fsurg.2024.1393898 | |
60. | Avi A. Gajjar, Rohit Prem Kumar, Ethan D. Paliwoda, Cathleen C. Kuo, Samuel Adida, Andrew D. Legarreta, Hansen Deng, Sharath Kumar Anand, D. Kojo Hamilton, Thomas J. Buell, Nitin Agarwal, Peter C. Gerszten, Joseph S. Hudson, Usefulness and Accuracy of Artificial Intelligence Chatbot Responses to Patient Questions for Neurosurgical Procedures, 2024, 0148-396X, 10.1227/neu.0000000000002856 | |
61. | Masood Rashid, Artificial Intelligence in Surgery: Transforming the Future of Operative Care, 2024, 1, 3007-2794, 1, 10.69750/dmls.01.03.034 | |
62. | Akshaya Narayan Shetti, Bhaskaran Ashokan, Mangesh Hivre, Rachita G Mustilwar, Krishan Kant, From scalpels to algorithms: The next step in surgical advancement, 2023, 10, 2394-2789, 61, 10.18231/j.ijpca.2023.012 | |
63. | Harry Robertshaw, Lennart Karstensen, Benjamin Jackson, Hadi Sadati, Kawal Rhode, Sebastien Ourselin, Alejandro Granados, Thomas C. Booth, Artificial intelligence in the autonomous navigation of endovascular interventions: a systematic review, 2023, 17, 1662-5161, 10.3389/fnhum.2023.1239374 | |
64. | Nadya Zaragita, Stefano Zhou, Setyo Widi Nugroho, Chandrasekaran Kaliaperumal, Breaking boundaries in neurosurgery through art and technology: A historical perspective, 2024, 4, 27725294, 102836, 10.1016/j.bas.2024.102836 | |
65. | Sagar Jolly, Shashank Paliwal, Aditya Gadepalli, Sheena Chaudhary, Hemant Bhagat, Rafi Avitsian, Designing Enhanced Recovery After Surgery Protocols in Neurosurgery: A Contemporary Narrative Review, 2024, 36, 0898-4921, 201, 10.1097/ANA.0000000000000946 | |
66. | Leiyi Gao, Jiao Li, Ruixin Zhang, Hailu Hanna Bekele, Junzhu Wang, Yining Cheng, Hongxia Deng, MMGan: a multimodal MR brain tumor image segmentation method, 2023, 17, 1662-5161, 10.3389/fnhum.2023.1275795 | |
67. | Mohammad Mofatteh, Mohammad Sadegh Mashayekhi, Saman Arfaie, Yimin Chen, Armaan K. Malhotra, Georgios P. Skandalakis, Mohammed Ali Alvi, Fardad T. Afshari, Shakila Meshkat, Famu Lin, Ebtesam Abdulla, Ayush Anand, Xuxing Liao, Roger S. McIntyre, Carlo Santaguida, Michael H. Weber, Michael G. Fehlings, Anxiety and Depression in Pediatric-Onset Traumatic Spinal Cord Injury: A Systematic Review, 2024, 184, 18788750, 267, 10.1016/j.wneu.2023.12.092 | |
68. | Jun Li, Jiansheng Zhong, Ziqi Li, Yong Xiao, Shousen Wang, Ectopic Pituitary Neuroendocrine Tumor: A Case Report Written With the Help of ChatGPT, 2023, 2168-8184, 10.7759/cureus.46999 | |
69. | Mohammad Mofatteh, Examining the association between traumatic brain injury and headache, 2021, 20, 0219-6352, 10.31083/j.jin2004109 | |
70. | Sahin Hanalioglu, Siyar Bahadir, Ahmet C. Ozak, Kivanc Yangi, Giancarlo Mignucci-Jiménez, Muhammet Enes Gurses, Alberto Fuentes, Ethan Mathew, Dakota T. Graham, Muhammed Yakup Altug, Egemen Gok, Gregory H. Turner, Michael T. Lawton, Mark C. Preul, Ultrahigh-resolution 7-Tesla anatomic magnetic resonance imaging and diffusion tensor imaging of ex vivo formalin-fixed human brainstem-cerebellum complex, 2024, 18, 1662-5161, 10.3389/fnhum.2024.1484431 | |
71. | Jhon E. Bocanegra-Becerra, Julia Sader Neves Ferreira, Gabriel Simoni, Anthony Hong, Wagner Rios-Garcia, Mohammad Mirahmadi Eraghi, Adriam M. Castilla-Encinas, Jhair Alejandro Colan, Rolando Rojas-Apaza, Emanuel Eduardo Franco Pariasca Trevejo, Raphael Bertani, Miguel Angel Lopez-Gonzalez, Machine Learning Algorithms for Neurosurgical Preoperative Planning: A Comprehensive Scoping Review, 2024, 18788750, 10.1016/j.wneu.2024.11.048 | |
72. | Nehaar Nimmagadda, Edouard Aboian, Sharon Kiang, Uwe Fischer, The Role of Artificial Intelligence in Vascular Care, 2024, 29499127, 100179, 10.1016/j.jvsvi.2024.100179 | |
73. | Abdulsalam M. Aleid, Khalid M. Alshuqayfi, Shahad Alshehri, Maqbel Almajnooni, Almorgl Z. Mohammed, Raghad A. Aljohani, Abdulmajeed A. Aljabr, Sami F. Almalki, Saud N. Aldanyowi, Assessing Patient Preferences and Decision-making Factors in Elective Neurosurgical Procedures: A Cross-sectional Study, 2024, 1, 3050-807X, 667, 10.4103/ATMR.ATMR_132_24 | |
74. | Ryohei Saito, Eijiro Onishi, Sadaki Mitsuzawa, Satoshi Ota, Hisataka Takeuchi, Yoshihiro Tsukamoto, Shinnosuke Yamashita, Daiki Sako, Tadashi Yasuda, Association Between Trauma-Induced Vertebral Fractures and Motor Weakness in Patients With Diffuse Idiopathic Skeletal Hyperostosis, 2024, 2168-8184, 10.7759/cureus.76403 | |
75. | Seelora Sahu, Nidhi Panda, Amlan Swain, Hemant Bhagat, Shalvi Mahajan, Assessment of the Accuracy of Ultrasonographically Measured Optic Nerve Sheath Diameter as a Surrogate for the Detection of Intracranial Hypertension Compared to Optic Nerve Sheath Diameter Measured by MRI: A Prospective Observational Study, 2024, 2168-8184, 10.7759/cureus.76655 | |
76. | Morteza Fattahi, Milad Esmaeil-Zadeh, Hamid Soltanian-Zadeh, Reza Rostami, Jamil Mansouri, Gholam-Ali Hossein-Zadeh, Classification of female MDD patients with and without suicidal ideation using resting-state functional magnetic resonance imaging and machine learning, 2025, 18, 1662-5161, 10.3389/fnhum.2024.1427532 | |
77. | Zhisen Li, Xiaoxia Hou, Yanli Lu, Huimin Zhao, Meixia Wang, Qian Gui, Guanhui Wu, Qinrong Xu, Xiaofeng Dong, Qingzhang Cheng, Xiaowen Xu, Hongxuan Feng, Functional MRI study of neurovascular coupling in patients with non-lesional epilepsy, 2025, 18, 1662-5161, 10.3389/fnhum.2024.1517565 | |
78. | Takeshi Umebayashi, Yasukazu Hijikata, Takaoki Kimura, Nahoko Kikuchi, Takeshi Hara, Keiichi Tsuda, Shinji Kumamoto, Daichi Kawamura, Efficacy of Navigation Systems With Smart Delivery Tools in Enhancing the Accuracy of Percutaneous Pedicle Screw Insertion , 2025, 2168-8184, 10.7759/cureus.78656 | |
79. | Muhammad Mohsin khan, Gianluca Scalia, Noman Shah, Giuseppe Emmanuele Umana, Vishal Chavda, Bipin Chaurasia, Ethical Concerns of AI in Neurosurgery: A Systematic Review, 2025, 15, 2162-3279, 10.1002/brb3.70333 | |
80. | Aishwarya Gadwal, Maria Gabriela Cerdas, Areej Khan, Zainab Khan, Di Carluccio Martina , Sai Priya Nimmagadda, Syed Mustafa Ali Rizvi, Sara Mahmood Aljallawi, Shelly Jaryal, Nuren Tasgaonkar, Adnan Ahmed, Sowmika Reddy Busireddy, The Role of Artificial Intelligence in the Diagnosis and Management of Non-convulsive Seizures: A Narrative Review, 2025, 2168-8184, 10.7759/cureus.79409 | |
81. | Abdallah Abbas, Mohamed Abouzid, Haneen Sabet, Ahmed Mansour, Abdelfattah Arafa, Ahmed Elshahat, Ahmed Mostafa Amin, Mahmoud Tarek Hefnawy, Mohamed El-Moslemani, Amna Hussein, Mostafa Hossam El Din Moawad, Shereen M. Olama, Ahmed Badry Shehata, Mahmoud G. A. Saleh, Ahmad Farag Ibrahim El-Adawy, Ahmed M. Raslan, Mostafa Meshref, Cerebellar transcranial magnetic stimulation in essential tremor: a systematic review and meta-analysis of safety and efficacy, 2025, 0300-9009, 10.1007/s13760-025-02740-z | |
82. | Sijie Zhou, Mohammad Mofatteh, Zhikai Chen, Jinyan Tang, Jicai Ma, Ziqi Ouyang, Jian Wang, Senrong Luo, Yuankang He, Zhenzhang Li, Yuzheng Lai, Xuxing Liao, Predictive outcome of late window ischemic stroke patients following endovascular therapy: a multi-center study, 2025, 16, 1664-2295, 10.3389/fneur.2025.1489714 |