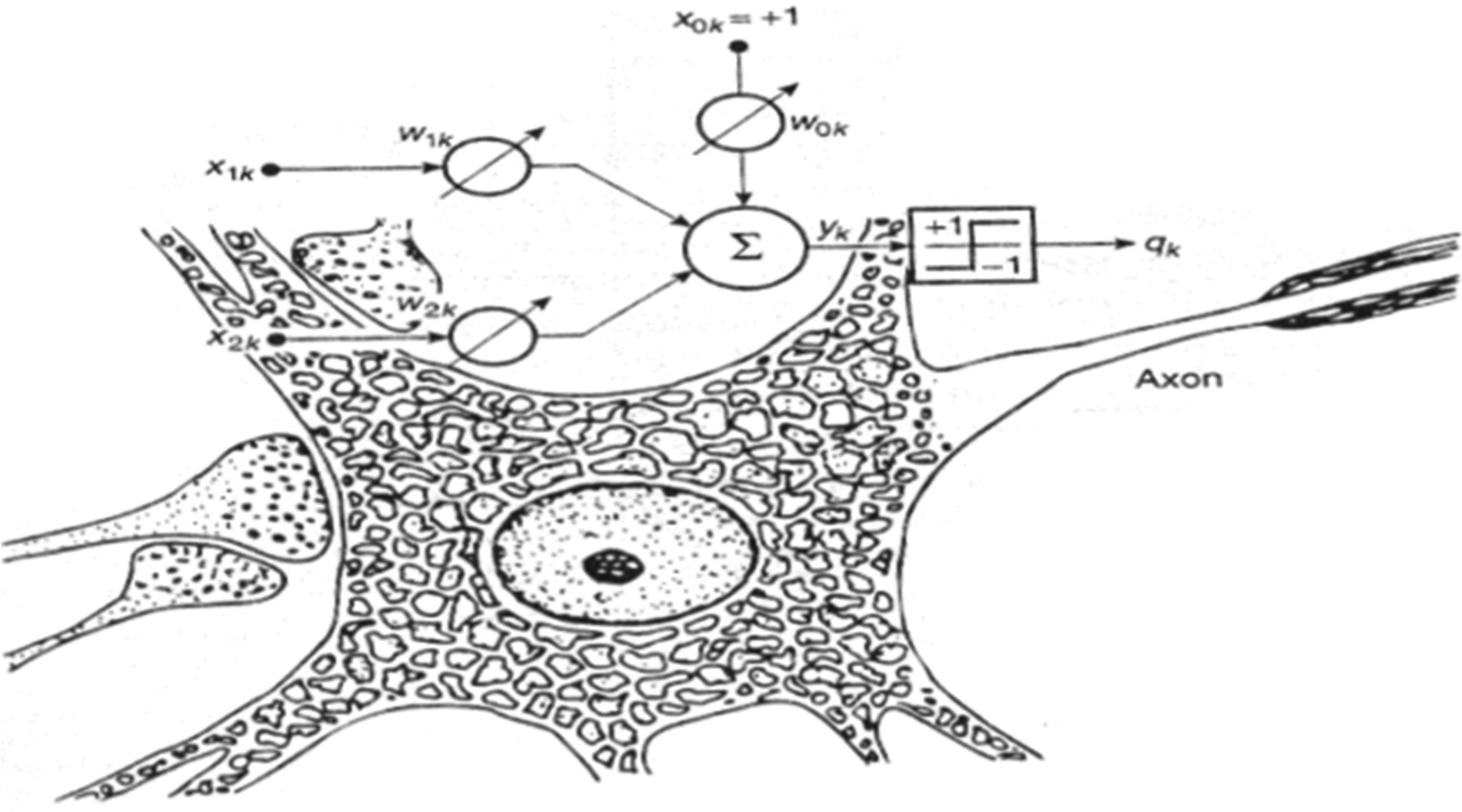
Citation: Elena Vlamou, Basil Papadopoulos. Fuzzy logic systems and medical applications[J]. AIMS Neuroscience, 2019, 6(4): 266-272. doi: 10.3934/Neuroscience.2019.4.266
[1] | Tjokorda Istri Pramitasuri, Ni Made Susilawathi, AA Raka Sudewi . Bacterial meningitis-induced demyelination: A logical fallacy or groundbreaking avenue in neuroscience?. AIMS Neuroscience, 2023, 10(2): 172-174. doi: 10.3934/Neuroscience.2023013 |
[2] | Zohaer Muttalib, Dana Aboukhalil, Chisom Nwosu, Dave D. Manguerra, Jimmy Wen, Ubaid Ansari, Meraj Alam, Ihab Abed, Ethan Tabaie, Ahmed Salem, Forshing Lui . Gluten and its relationship with inflammation and Parkinson's Disease: A literature review. AIMS Neuroscience, 2025, 12(1): 28-41. doi: 10.3934/Neuroscience.2025004 |
[3] | Oussama Gaied Chortane, Elmoetez Magtouf, Wael Maktouf, Sabri Gaied Chortane . Effects of long-term adapted physical training on functional capacity and quality of life in older adults with Parkinson's disease. AIMS Neuroscience, 2024, 11(4): 468-483. doi: 10.3934/Neuroscience.2024028 |
[4] | Sairam Ramesh, Arosh S. Perera Molligoda Arachchige . Depletion of dopamine in Parkinson's disease and relevant therapeutic options: A review of the literature. AIMS Neuroscience, 2023, 10(3): 200-231. doi: 10.3934/Neuroscience.2023017 |
[5] | Ashok Chakraborty, Anil Diwan . Depression and Parkinson's disease: a Chicken-Egg story. AIMS Neuroscience, 2022, 9(4): 479-490. doi: 10.3934/Neuroscience.2022027 |
[6] | Georgia Theocharopoulou . The ubiquitous role of mitochondria in Parkinson and other neurodegenerative diseases. AIMS Neuroscience, 2020, 7(1): 43-65. doi: 10.3934/Neuroscience.2020004 |
[7] | I. Banegas, I. Prieto, A.B. Segarra, M. de Gasparo, M. Ramírez-Sánchez . Study of the Neuropeptide Function in Parkinson’s Disease Using the 6-Hydroxydopamine Model of Experimental Hemiparkinsonism. AIMS Neuroscience, 2017, 4(4): 223-237. doi: 10.3934/Neuroscience.2017.4.223 |
[8] | mgr Natalia Białoń, dr hab. n. o zdr. Dariusz Górka, mgr Mikołaj Górka . The brain-gut axis: communication mechanisms and the role of the microbiome as a neuroprotective factor in the development of neurodegenerative diseases: A literature overview. AIMS Neuroscience, 2024, 11(3): 289-311. doi: 10.3934/Neuroscience.2024019 |
[9] | Panagiotis Vlamos . Novel modeling methodologies for the neuropathological dimensions of Parkinson's disease. AIMS Neuroscience, 2020, 7(2): 89-93. doi: 10.3934/Neuroscience.2020006 |
[10] | Naeimeh Akbari-Gharalari, Maryam Ghahremani-Nasab, Roya Naderi, Leila Chodari, Farshad Nezhadshahmohammad . The potential of exosomal biomarkers: Revolutionizing Parkinson's disease: How do they influence pathogenesis, diagnosis, and therapeutic strategies?. AIMS Neuroscience, 2024, 11(3): 374-397. doi: 10.3934/Neuroscience.2024023 |
This paper highlights the potential uses of fuzzy network structures in the field of medicine and in particular, it focuses on the several methods in which those system in combination with fuzzy logic techniques could be utilized in order to enhance pattern recognition efficiency. Although medicine and control engineering are not directly related, the use of accessible control techniques for on-line devices, particularly in cases of surgical operations and in intensive care units is now feasible. Currently, the application areas of control engineering in medicine range from simple dosage prescription schemes to highly sophisticated adaptive controllers. Real world knowledge can be regarded as incomplete, inaccurate, and inconsistent. Exact medical entities such as fuzzy-sets can be explained by fuzzy logic theory [1]. As it will be reviewed in the following sections, studies have shown that fuzzy logic methodologies can be utilized in early diagnosis of diseases such as Parkinson's disease. Early diagnosis has been proven to be very valuable in creating a more effective treatment plan. Therefore, identifying a method that would allow for early disease diagnosis would be extremely beneficial for the patients. The main contribution of this paper is to analyze various types of fuzzy systems and examine their potential applications in early diagnosis or disease classification.
The attempts to substitute certain brain cognitive functions by a computer system are not hindered by the existing differences between the structure of the human brain and that of a computer. Artificial Intelligence is employed for the creation and application of systems that imitate not only logical thinking and behavior but also human intelligence [2].
A number of issues linked with the evolutionary theory arose, when the idea that the human mind could be perceived as a computer whose processes are observed via reverse engineering was formed. The evolutionary theory states that living species evolve over time [3]. However, a series of adaptive variations result in certain evolutionary changes regarding brain functions. Computer simulation that uses computational models consisting of mathematical equations are utilized for the research of cognitive function processes [4]. Such models include but are not limited to artificial neural networks, which as the name suggests were inspired by biological neural networks. In biology, a neuron is the smallest part of the brain and it constitutes the basic difference between animals and plants (plants do not have neurons). A neuron's main function is to process information. In the cortex of the brain there are approximately 10 billion neurons and 60 trillion connections. As it is shown in Figure 1, the main sections of a neuron include the body, the axis and the dendrites, which receive signals from neighboring neurons [5].
Fuzzy logic–fuzzy systems comprise one of the three pillars of Computational intelligence which in turn is categorised under the broad field of artificial intelligence. The other two pillars are artificial neural networks and evolutionary computing (evolutionary computation). Fuzzy systems, which utilize fuzzy sets and fuzzy logic, are an attempt to effectively describe the uncertainty of the real world. Fuzzy logic is a generalisation of classical logic and provides mechanisms of approximation (approximate reasoning) and inference (decision making). The approximate reasoning is an attempt to model the human way of thinking and inference, as it is known that the human brain performs more approximate considerations based on qualitative criteria of perception than accurate considerations based on a plethora of data [1].
A statement can be true “with some degree of truth” [1], and not just true or false as Boolean logic suggests, the logic on which the modern computer is based on.
Dr. Lutfi Zadeh of the University of California at Berkeley in the 1960s was the first to introduce the concept of fuzzy logic. Fuzzy logic includes 0 and 1 as extreme cases of truth but also incorporates intermediate states of truth [1]. Fuzzy logic resembles the way human brains work.
The development of a fuzzy system with high-performance is not easily accomplished. Several problems arise, including the search of membership functions and appropriate rules, a process which regularly leads in errors. As a result, the learning algorithms were also applied to fuzzy systems. Neural networks, were considered as an alternate way to automate the development of fuzzy systems [6]. The functions of neural networks include but are not limited to process control applications, data analysis and classification, detection of imperfections, and support to decision-making.
Neural networks and fuzzy systems can be fused in order to increase their advantages and to decrease their shortcomings. Neural network learning techniques can be utilized in order to substantially reduce the development time of fuzzy systems as well as the cost while improving the performance rates [7]. Figures 2 and 3 present two potential models of fuzzy neural systems. In Figure 2, the fuzzy interface block provides an input vector to a multi-layer neural network as a response to linguistic statements. Subsequently, the neural network is trained to generate required outputs or decisions [8].
In the second case, the fuzzy inference mechanism is determined by a multi-layered neural network.
The computational characteristics of learning offered by neural networks are obtained by fuzzy systems and in return, neural networks receive the interpretation and clarity of systems representation [9]. A fuzzy neural network or neuro-fuzzy system (NFS) utilizes approximation techniques acquired from neural networks, in order to identify parameters of a fuzzy system (i.e., fuzzy sets, fuzzy rules).
For the model of cooperative neural fuzzy systems as shown in Figures 4 and 5, the artificial neural network (ANN) and fuzzy system work independently. The ANN tries to learn the parameters from the fuzzy system, a process that can be performed either offline or online [8].
In the upper left example of Figure 4, the fuzzy rules provided by the training data combined with the fuzzy sets are utilized to form the fuzzy system (offline determination).
In the upper right model of Figure 4, the fuzzy neural network learns the fuzzy sets from the given training data (offline determination). As it is shown in Figure 4, in the lower left neuro-fuzzy case, the fuzzy rules and membership functions must be defined beforehand, in order for the system to learn all membership function parameters online. For the improvement of the learning step, the error has to be measured. In the lower right model, a rule weight which is interpreted as the influence of a rule, is determined for all fuzzy rules by a neural network (both online and offline determination) [8].
A cooperative system only utilizes neural networks in an initial phase. The neural networks using training data, establish sub-blocks of the fuzzy system. Subsequent removal occurs, resulting in the implementation of only the fuzzy system [6].
In the concurrent neuro-fuzzy system (Figure 6), the neural network and the fuzzy system constantly function in a collective manner, with the neural network pre-processing the inputs of the fuzzy system.
Hybrid neuro-fuzzy systems (Figure 7) utilize neural networks in order to identify certain parameters of a fuzzy system. In this case, the architecture of hybrid NFS offers a great advantage seeing as the fuzzy system and neural network do not have to communicate with each other. In addition, these systems can learn online and offline [6].
Due to their effectiveness, AI (artificial intelligence) techniques, such as fuzzy logic, play a prominent role in the field of medicine. Such methods allow for not only an efficient but also a prompt diagnosis. Guzmán et al., 2019 created a fuzzy classifier in order to perform blood pressure level classification. The main results of this study showed that a type-1 fuzzy inference system or an interval type-2 fuzzy inference system constitute the best architectures to perform said classification [10]. Fuzzy logic has been also applied in order to provide risk assessment for hypertension. Melin, Miramontes and Prado-Arechiga, 2018, designed a model that combined neural networks and fuzzy logic for this purpose. Fuzzy systems were a key part of this study since they regulated the classification uncertainty. This hybrid model provided good results with excellent performance regarding its task [11]. Studies have also shown that fuzzy systems can be applied in Parkinson's diagnosis. Abiyev and Abizade, 2016, proposed a system for Parkinson's disease diagnosis based on the fusion of the fuzzy system and neural networks. The proposed fuzzy neural system (FNS) allows for efficient classification of healthy individuals, a fact that was established through simulation of the system using data obtained from UCI machine learning repository [12].
Another study tested the technique of classifying medical data sets by constructing fuzzy inference systems or fuzzy expert systems. The analysis of data related to Parkinson's yielded a large amount of information. In order to further study and explore the information provided, clinical observations, and disease diagnosis were mathematically translated. Knowledge-based Systems in combination with Data Mining tools and a fuzzy decision maker as well as Artificial Neural Networks Classifiers proved to be useful techniques for mapping clinical data to a numerical data set by exploiting a set of rules [13].
Kaur et al., 2017, described Parkinson's disease using an adaptive neuro-fuzzy technique. According to their results, the adaptive neuro fuzzy expert system showed higher accuracy rates than the fuzzy expert system. In addition, the adaptive neuro fuzzy expert system exhibited higher rate of sensitivity, specificity, and precision when compared to a fuzzy expert system [14].
In this paper, “fuzzy logic” systems which could be used to formalize approximate reasoning in medical diagnostic systems are described. The potential implementation of fuzzy artificial networks in medicine is also analyzed. Authors further work would focus on applying the aforementioned techniques for the establishment of intelligent systems that could be utilized in disease treatment and diagnosis. In more detail, future steps would include the development of a fuzzy expert system that would be utilized in PD diagnosis. This study would include experiments that would evaluate parameters such as accuracy, sensitivity, and specificity.
[1] |
Mahfouf M, Abbod MF, Linkens DA (2001) A survey of fuzzy logic monitoring and control utilisation in medicine. Artif Intell Med 21: 27–42. doi: 10.1016/S0933-3657(00)00072-5
![]() |
[2] |
O'Regan JK, Noë A (2001) A sensorimotor account of vision and visual consciousness. Behav Brain Sci 24: 939–973. doi: 10.1017/S0140525X01000115
![]() |
[3] | Matthen M, Stephens C (2007) Philosophy of biology. Amsterdam: Elsevier. |
[4] | Kowaliw T, Bredeche N, Chevallier S, et al. (2014) Artificial Neurogenesis: An Introduction and Selective Review. In: Growing Adaptive Machines, Berlin: Springer, 1–60. |
[5] | Fuller R (2013) Introduction to Neuro-Fuzzy Systems. London: Springer Science & Business Media. |
[6] | R Fullér (1995) Neural Fuzzy Systems. |
[7] | Karthekeyan AR (2014) Fuzzy neural network based extreme learning machine technique in credit risk management. Int J Res Eng IT Soc Sci 4. |
[8] |
Kruse R (2008) Fuzzy neural network. Scholarpedia 3: 6043. doi: 10.4249/scholarpedia.6043
![]() |
[9] |
Pota M, Esposito M, De Pietro G (2018) Likelihood-Fuzzy analysis: from data, through statistics, to interpretable fuzzy classifiers. Int J Approx Reason 93: 88–102. doi: 10.1016/j.ijar.2017.10.022
![]() |
[10] |
Guzmán JC, Miramontes I, Melin P, et al. (2019) Optimal genetic design of Type-1 and interval Type-2 fuzzy systems for blood pressure level classification. Axioms 8: 8. doi: 10.3390/axioms8010008
![]() |
[11] |
Melin P, Miramontes I, Prado-Arechiga G (2018) A hybrid model based on modular neural networks and fuzzy systems for classification of blood pressure and hypertension risk diagnosis. Expert Syst Appl 107: 146–164. doi: 10.1016/j.eswa.2018.04.023
![]() |
[12] | Abiyev RH, Abizade S (2016) Diagnosing Parkinson's diseases using fuzzy neural system. Comput Math Methods Med 2016: 1267919. |
[13] | Geman O (2013) Nonlinear dynamics, artificial neural networks and neuro-fuzzy classifier for automatic assessing of tremor severity. 2013 E-Health and Bioengineering Conference (EHB). |
[14] | Kaur P, Trehan H, Kaur V, et al. (2017) Analysis of adaptive neuro-fuzzy based expert system for Parkinson's disease diagnosis. Int J Adv Res Ideas Innov Technol 3: 1120–1127. |
1. | Andreas Rosenkranz, Max Marian, Francisco J. Profito, Nathan Aragon, Raj Shah, The Use of Artificial Intelligence in Tribology—A Perspective, 2020, 9, 2075-4442, 2, 10.3390/lubricants9010002 | |
2. | Ogbolu Melvin Omone, Eric Aggrey, Marta Takacs, Miklos Kozlovszky, 2020, Implementation of A Fuzzy Logic Progression For Alcohol Addicts Using Fuzzy Control System(FCS), 978-1-7281-7352-8, 161, 10.1109/SISY50555.2020.9217079 | |
3. | Mengya Zhu, Dengkai Chen, Jingluan Wang, Yiwei Sun, Analysis of oceanaut operating performance using an integrated Bayesian network aided by the fuzzy logic theory, 2021, 83, 01698141, 103129, 10.1016/j.ergon.2021.103129 | |
4. | Celestine Iwendi, Kainaat Mahboob, Zarnab Khalid, Abdul Rehman Javed, Muhammad Rizwan, Uttam Ghosh, Classification of COVID-19 individuals using adaptive neuro-fuzzy inference system, 2021, 0942-4962, 10.1007/s00530-021-00774-w | |
5. | Johnpaul C.I., Munaga V.N.K. Prasad, S. Nickolas, G.R. Gangadharan, Fuzzy representational structures for trend based analysis of time series clustering and classification, 2021, 222, 09507051, 106991, 10.1016/j.knosys.2021.106991 | |
6. | Fei Su, Hong Wang, Linlu Zu, Yan Chen, Closed-loop modulation of model parkinsonian beta oscillations based on CAR-fuzzy control algorithm, 2022, 1871-4080, 10.1007/s11571-022-09820-3 | |
7. | Noureen Talpur, Said Jadid Abdulkadir, Hitham Alhussian, Mohd Hilmi Hasan, Norshakirah Aziz, Alwi Bamhdi, Deep Neuro-Fuzzy System application trends, challenges, and future perspectives: a systematic survey, 2023, 56, 0269-2821, 865, 10.1007/s10462-022-10188-3 | |
8. | Sandeep Kumar Sunori, Amit Mittal, Pradeep Juneja, P.G. Om Prakash, Richa Alagh, Sudhanshu Maurya, 2021, Prediction of Rainfall using GRNN and Neurofuzzy Techniques, 978-1-6654-1836-2, 1, 10.1109/GCAT52182.2021.9587819 | |
9. | Arkadiusz Lewicki, Krzysztof Pancerz, Leszek Puzio, The temporal inference with the use of ant-based clustering algorithm and flow graphs in the problem of prognosing complications of medical surgical procedures, 2021, 17, 1795-6889, 10.14254/1795-6889.2021.17-3.3 | |
10. | Sahar Qazi, Naiyar Iqbal, Khalid Raza, 2022, Chapter 12, 978-981-16-9220-8, 201, 10.1007/978-981-16-9221-5_12 | |
11. | Jing Wang, Long Yu, Shengwei Tian, MsRAN: a multi-scale residual attention network for multi-model image fusion, 2022, 60, 0140-0118, 3615, 10.1007/s11517-022-02690-1 | |
12. | R. Hadjouti, H. Mohand Kaci, F. Benzina, J. N. Furze, Xinhua He, Enhancing agriculture recovery of, 2022, 60, 1838-675X, 850, 10.1071/SR21320 | |
13. | Kanika Garg, Naveen Chauhan, Rajeev Agrawal, Optimized Resource Allocation for Fog Network using Neuro-fuzzy Offloading Approach, 2022, 47, 2193-567X, 10333, 10.1007/s13369-022-06563-5 | |
14. | Supriya Singh, D. P. Vidyarthi, 2023, Chapter 35, 978-3-031-27608-8, 449, 10.1007/978-3-031-27609-5_35 | |
15. | Muhammad Zia Rahman, Muhammad Azeem Akbar, Víctor Leiva, Abdullah Tahir, Muhammad Tanveer Riaz, Carlos Martin-Barreiro, An intelligent health monitoring and diagnosis system based on the internet of things and fuzzy logic for cardiac arrhythmia COVID-19 patients, 2023, 154, 00104825, 106583, 10.1016/j.compbiomed.2023.106583 | |
16. | Xi-Le Wei, Yu-Lin Bai, Jiang Wang, Si-Yuan Chang, Chen Liu, Parkinsonian oscillations and their suppression by closed-loop deep brain stimulation based on fuzzy concept, 2022, 31, 1674-1056, 128701, 10.1088/1674-1056/ac8cd8 | |
17. | Adam Szulc, Piotr Prokopowicz, Krzysztof Buśko, Dariusz Mikołajewski, Model of the Performance Based on Artificial Intelligence–Fuzzy Logic Description of Physical Activity, 2023, 23, 1424-8220, 1117, 10.3390/s23031117 | |
18. | Issah M. Alhamad, Riad Saraiji, Indoor illuminance selection procedure using fuzzy techniques, 2022, 62, 23527102, 105362, 10.1016/j.jobe.2022.105362 | |
19. | Wınıta SULANDARİ, Yudho YUDHANTO, Sri SUBANTİ, Etik ZUKHRONAH, Subanar SUBANAR, Muhammad Hisyam LEE, Forecasting Tourist Arrivals to Sangiran Using Fuzzy with Calendar Variations, 2022, 2147-9100, 10.30519/ahtr.990903 | |
20. | Narges Mohebbi, Meysam Alavi, Mehrdad Kargari, 2022, Determining COVID-19 Severity with Fuzzy Inference System, 978-1-6654-8027-7, 1, 10.1109/CSICC55295.2022.9780483 | |
21. | Cristiano Hora Fontes, Refinement of the feedforward network in multi-class classification problems using a hybrid approach combining supervised clustering and a fuzzy classifier, 2022, 115, 09521976, 105242, 10.1016/j.engappai.2022.105242 | |
22. | Amelia J. Garner, Reza Saatchi, Oliver Ward, Daniel P. Hawley, Juvenile Idiopathic Arthritis: A Review of Novel Diagnostic and Monitoring Technologies, 2021, 9, 2227-9032, 1683, 10.3390/healthcare9121683 | |
23. | Sindhu Rajendran, B. Sahana, Shaik Farheen, B. N. Meghana, Sona Theresa Babu, 2023, Chapter 11, 978-3-031-26331-6, 165, 10.1007/978-3-031-26332-3_11 | |
24. | Sandeep Kumar Sunori, Amit Mittal, Pradeep Juneja, 2023, Design of Neurofuzzy Models for Biological Oxygen Demand Prediction, 979-8-3503-4664-0, 86, 10.1109/ICEARS56392.2023.10085535 | |
25. | Chérubin Mugisha, Incheon Paik, Bridging the Gap between Medical Tabular Data and NLP Predictive Models: A Fuzzy-Logic-Based Textualization Approach, 2023, 12, 2079-9292, 1848, 10.3390/electronics12081848 | |
26. | Patricia Melin, Daniela Sánchez, Oscar Castillo, Interval Type-3 Fuzzy Inference System Design for Medical Classification Using Genetic Algorithms, 2023, 13, 2075-1680, 5, 10.3390/axioms13010005 | |
27. | Tal Sigawi, Yaron Ilan, Using Constrained-Disorder Principle-Based Systems to Improve the Performance of Digital Twins in Biological Systems, 2023, 8, 2313-7673, 359, 10.3390/biomimetics8040359 | |
28. | Siyuan Li, Chao Wang, Ying Wang, Fuzzy evaluation model for physical education teaching methods in colleges and universities using artificial intelligence, 2024, 14, 2045-2322, 10.1038/s41598-024-53177-y | |
29. | Siren Zhao, Jilun Zhang, Jianbin Zhang, Predicting UPDRS in Parkinson’s disease using ensembles of self-organizing map and neuro-fuzzy, 2024, 13, 2192-113X, 10.1186/s13677-024-00641-9 | |
30. | John Byabazaire, Gregory M. P. O’Hare, Rem Collier, Declan Delaney, IoT Data Quality Assessment Framework Using Adaptive Weighted Estimation Fusion, 2023, 23, 1424-8220, 5993, 10.3390/s23135993 | |
31. | L. Garcia-del Rio, P. Diaz-Rodriguez, M. Landin, 2023, 978-1-83916-727-0, 313, 10.1039/BK9781837670055-00313 | |
32. | Mohith Bekal Kar, Mangalpady Aruna, Bijay Mihir Kunar, Fuzzy Logic-Based Rapid Upper Limb Assessment: A Novel Approach to Evaluate the Postural Risk of Dumper Operators, 2023, 104, 2250-0545, 1047, 10.1007/s40032-023-00986-1 | |
33. | Celina Silvia Stafie, Irina-Georgeta Sufaru, Cristina Mihaela Ghiciuc, Ingrid-Ioana Stafie, Eduard-Constantin Sufaru, Sorina Mihaela Solomon, Monica Hancianu, Exploring the Intersection of Artificial Intelligence and Clinical Healthcare: A Multidisciplinary Review, 2023, 13, 2075-4418, 1995, 10.3390/diagnostics13121995 | |
34. | Ibrahim Alhamrouni, Nor Hidayah Abdul Kahar, Mohaned Salem, Mahmood Swadi, Younes Zahroui, Dheyaa Jasim Kadhim, Faisal A. Mohamed, Mohammad Alhuyi Nazari, A Comprehensive Review on the Role of Artificial Intelligence in Power System Stability, Control, and Protection: Insights and Future Directions, 2024, 14, 2076-3417, 6214, 10.3390/app14146214 | |
35. | Shokouh Arjmand, Reza Saboori Amleshi, Francisco S. Guimarães, Gregers Wegener, Advancing psychiatric nosology through philosophical inquiry, 2024, 0924-2708, 1, 10.1017/neu.2024.21 | |
36. | Yaron Ilan, The Co-Piloting Model for Using Artificial Intelligence Systems in Medicine: Implementing the Constrained-Disorder-Principle-Based Second-Generation System, 2024, 11, 2306-5354, 1111, 10.3390/bioengineering11111111 | |
37. | Jagmeet S. Kanwal, From Information to Knowledge: A Role for Knowledge Networks in Decision Making and Action Selection, 2024, 15, 2078-2489, 487, 10.3390/info15080487 | |
38. | Claudia I. Gonzalez, Dementia Classification Approach Based on Non-Singleton General Type-2 Fuzzy Reasoning, 2024, 13, 2075-1680, 672, 10.3390/axioms13100672 | |
39. | Suresh Kumar Srichandan, Santosh Kumar Majhi, Sudarson Jena, Kaushik Mishra, Radhakrishna Bhat, A Secure and Distributed Placement for Quality of Service-Aware IoT Requests in Fog-Cloud of Things: A Novel Joint Algorithmic Approach, 2024, 12, 2169-3536, 56730, 10.1109/ACCESS.2024.3390723 | |
40. | Prometeo Cortés-Antonio, Fevrier Valdez, Patricia Melin, Oscar Castillo, Crips sets, type-1, and type-2 fuzzy sets in the context of linguistic variables and rule-based systems for cognitive computing, 2024, 10641246, 1, 10.3233/JIFS-219368 | |
41. | Javaid Ahmad Shah, Deepak Sukheja, Govinda Patil, Tranjeet Sood, Malaya Nayak, 2024, Chapter 5, 978-981-99-9178-5, 49, 10.1007/978-981-99-9179-2_5 | |
42. | Supriya Singh, D.P. Vidyarthi, An integrated approach of ML-metaheuristics for secure service placement in fog-cloud ecosystem, 2023, 22, 25426605, 100817, 10.1016/j.iot.2023.100817 | |
43. | Alma Y. Alanis, Oscar D. Sanchez, Alonso Vaca-Gonzalez, Marco A. Perez-Cisneros, 2024, 9780443223419, 1, 10.1016/B978-0-44-322341-9.00010-0 | |
44. | Luis Pastor Sánchez-Fernández, Biomechanics of Parkinson’s Disease with Systems Based on Expert Knowledge and Machine Learning: A Scoping Review, 2024, 12, 2079-3197, 230, 10.3390/computation12110230 | |
45. | Amar Salehi, Soleiman Hosseinpour, Nasrollah Tabatabaei, Mahmoud Soltani Firouz, Niloufar Zadebana, Richard Nauber, Mariana Medina‐Sánchez, Advancements in Machine Learning for Microrobotics in Biomedicine, 2024, 2640-4567, 10.1002/aisy.202400458 | |
46. | Rashid Baimukashev, Shirali Kadyrov, Cemil Turan, Systematic Survey of Deep Fuzzy Computer Vision in Biomedical Research, 2024, 16, 1616-8658, 220, 10.26599/FIE.2024.9270043 | |
47. | Changle Sun, Haitao Li, Jun-e Feng, Construction of interpretable hierarchical fuzzy systems subject to incomplete data, 2025, 505, 01650114, 109273, 10.1016/j.fss.2025.109273 | |
48. | Ioana Scrobota, Gilda Mihaela Iova, Olivia Andreea Marcu, Liliana Sachelarie, Siviu Vlad, Ioana Monica Duncea, Florin Blaga, An Artificial Intelligence-Based Fuzzy Logic System for Periodontitis Risk Assessment in Patients with Type 2 Diabetes Mellitus, 2025, 12, 2306-5354, 211, 10.3390/bioengineering12030211 | |
49. | Integrating Fuzzy Logic Systems with Deep Learning Applications Using SPSS, 2025, 4, 1, 10.46632/jdaai/4/1/1 |