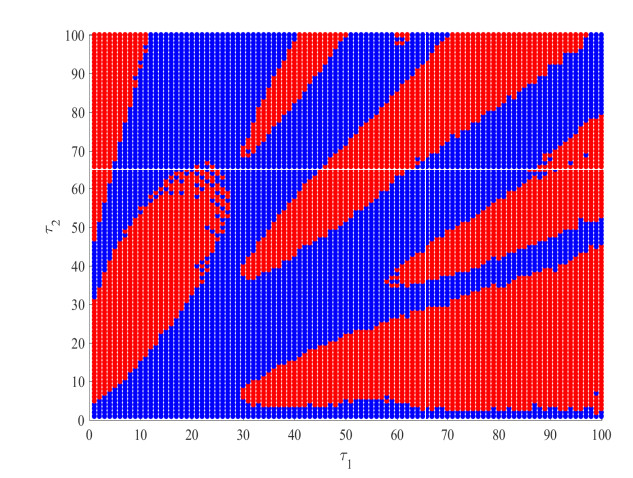
Citation: Frederik Riis Mikkelsen. A model based rule for selecting spiking thresholds in neuron models[J]. Mathematical Biosciences and Engineering, 2016, 13(3): 569-578. doi: 10.3934/mbe.2016008
[1] | Jun Liu, Zhenhua Yan, Chaochao Zhou, Liren Shao, Yuanyuan Han, Yusheng Song . mfeeU-Net: A multi-scale feature extraction and enhancement U-Net for automatic liver segmentation from CT Images. Mathematical Biosciences and Engineering, 2023, 20(5): 7784-7801. doi: 10.3934/mbe.2023336 |
[2] | Yuqing Zhang, Yutong Han, Jianxin Zhang . MAU-Net: Mixed attention U-Net for MRI brain tumor segmentation. Mathematical Biosciences and Engineering, 2023, 20(12): 20510-20527. doi: 10.3934/mbe.2023907 |
[3] | Yiwen Jiang . Surface defect detection of steel based on improved YOLOv5 algorithm. Mathematical Biosciences and Engineering, 2023, 20(11): 19858-19870. doi: 10.3934/mbe.2023879 |
[4] | Qian Wu, Yuyao Pei, Zihao Cheng, Xiaopeng Hu, Changqing Wang . SDS-Net: A lightweight 3D convolutional neural network with multi-branch attention for multimodal brain tumor accurate segmentation. Mathematical Biosciences and Engineering, 2023, 20(9): 17384-17406. doi: 10.3934/mbe.2023773 |
[5] | Mei-Ling Huang, Zong-Bin Huang . An ensemble-acute lymphoblastic leukemia model for acute lymphoblastic leukemia image classification. Mathematical Biosciences and Engineering, 2024, 21(2): 1959-1978. doi: 10.3934/mbe.2024087 |
[6] | Xiaoguang Liu, Yubo Wu, Meng Chen, Tie Liang, Fei Han, Xiuling Liu . A double-channel multiscale depthwise separable convolutional neural network for abnormal gait recognition. Mathematical Biosciences and Engineering, 2023, 20(5): 8049-8067. doi: 10.3934/mbe.2023349 |
[7] | Hongqiang Zhu . A graph neural network-enhanced knowledge graph framework for intelligent analysis of policing cases. Mathematical Biosciences and Engineering, 2023, 20(7): 11585-11604. doi: 10.3934/mbe.2023514 |
[8] | Beijing Chen, Ye Gao, Lingzheng Xu, Xiaopeng Hong, Yuhui Zheng, Yun-Qing Shi . Color image splicing localization algorithm by quaternion fully convolutional networks and superpixel-enhanced pairwise conditional random field. Mathematical Biosciences and Engineering, 2019, 16(6): 6907-6922. doi: 10.3934/mbe.2019346 |
[9] | Jianming Zhang , Chaoquan Lu , Xudong Li , Hye-Jin Kim, Jin Wang . A full convolutional network based on DenseNet for remote sensing scene classification. Mathematical Biosciences and Engineering, 2019, 16(5): 3345-3367. doi: 10.3934/mbe.2019167 |
[10] | Xin Zhou, Jingnan Guo, Liling Jiang, Bo Ning, Yanhao Wang . A lightweight CNN-based knowledge graph embedding model with channel attention for link prediction. Mathematical Biosciences and Engineering, 2023, 20(6): 9607-9624. doi: 10.3934/mbe.2023421 |
With the development of industry and agriculture, a variety of chemical toxins, such as heavy metals and pesticides etc., have been released into aquatic ecosystems. These toxins have the potential to cause an adverse impact on a diverse range of organisms [1], which has become a primary concern all over the world. Many countries have made a list of priority chemicals of concern and designated policies and measures to mitigate the adverse effects of chemical toxins on aquatic environments [2,3]. Accurately assessing the risks of toxins requires understandings of the effects of toxins on organisms, as well as on complex ecological interactions.
During the last several decades, mathematical models including individual-based model, matrix population models, differential equation models, have been developed to understand the ecological effects of toxin exposure. Bartell [4] and Pastorok et al. [5] make a complete review on the realism, relevance, and applicability of distinct types of models from the point of view of evaluating risks caused by environmental toxins. A literature search shows that since a series of work by Hallam et al. [6,7,8,9], a number of differential equation models have been proposed and studied to examine the effects of toxins on aquatic populations. A common feature of these differential equation models is that they involve three state variables: the population concentration, the concentration of toxins in the population, and the concentration of toxins in the environment, hence these models describe the interactions between populations and environmental toxins. In practice, because toxins contained in populations are extremely small portion of the whole environment, population birth, mortality, and metabolism do not significantly affect the concentration of toxins in the environment where the population habit. Based on this fact, Huang et al. [10] developed a body burden-dependent population model in which the concentration of toxins in the environment is treated as an important parameter and the effect of the population on environmental toxins is ignored. The model was then used to understand the toxic effects of methylmercury on the long-term behavior of the rainbow trout (Oncorhynchus mykiss). In terms of the results of model parameterization, the authors provided a threshold concentration of methylmercury in the external environment to maintain population persistence. Moreover, a complete global and bifurcation analysis of the model can be found in [11].
Time delays are very common in the biological processes, which can cause complex dynamic phenomena including limit cycle oscillations, quasi-periodic oscillations, and even chaos. Time delayed models are widely used to model biological systems, such as population dynamics [12,13,14], predator prey system [15,19], tumor immune system [18], gut microbiota system [20], Escherichia coli Tetrahymena system [21], food chain system [22] and so on. Recently, many authors have considered time delays in modeling aquatic population dynamics with the effects of environmental toxins [16,17,23]. For example, Chattopadhyay et al. [16] proposed a toxin-producing phytoplankton and zooplankton interaction model and revealed that the toxin substances produced by the phytoplankton play a significant role in the termination of planktonic blooms. Furthermore, Chattopadhyay et al. [17] extended the previous model [16] by considering the time delay of the liberation of toxic substances by the phytoplankton population, and investigated the delayed effect of toxic phytoplankton causing oscillation behaviors of phytoplankton and zooplankton populations. Besides, Jiang et al. [23] developed a delayed phytoplankton-zooplankton model with the coefficients depending on the delay. They showed that the maturity delay of toxic substances might serve as a key factor in the periodic phytoplankton and algae blooms.
The external changes in the environment have some kind of delayed effects on population dynamics, in particular, when a species live in a polluted aquatic environment, toxins have delayed responses on its growth and death [26]. Casarini et al. used Frog Embryo Teratogenesis Assay–Xenopus (FETAX) assay to measure mortality, delayed growth and embryo deformation. The results showed that the growth rate of the embryos was significantly delayed at the concentrations of 0.1 to 10nM of exposure to Okadaic Acid (OA) [27]. And the delayed mortality is observed in several species 4 to 12 d following the exposure to very low toxicant concentrations [28]. A delayed model for studying the interaction among phytoplankton, zooplankton, and fish in the presence of toxins has been developed in [24]. The delayed effect of toxins in a polluted environment on the growth of the population biomass has been considered in [25].
In this work, we extend the above-mentioned body burden-dependent model in [10,11] to a delay model by incorporating delayed toxic responses. The extension is based on the fact that the effects of toxins on the growth and mortality maybe not immediate, but time-delayed, especially when the population is subject to sublethal toxin levels [29]. We analyze the model in terms of steady states, stability, and bifurcation. By analyzing the transcendental characteristic equation, we investigate the effects of the time delays on the local stability of equilibria. We find that the Hopf bifurcation can occur in the model as the threshold value increases through certain values of the delays.
The paper consists of five sections. In the next section, we propose the toxin-mediated population model with time-delayed toxic responses. Section 3 contains nondimensionalization, existence and local stability of equilibria, and bifurcation analysis. In section 4, we make numerical simulations to verify the theoretical results. The conclusion and discussion are in the final section.
Our model with time delays is based on the following toxin-dependent aquatic population model studied in [10,11]:
dx(t)dt=(α1max{0,1−α2y(t)}1+α3x(t)−ky(t)−m)x(t), | (2.1a) |
dy(t)dt=aTe−(σ+α1max{0,1−α2y(t)}1+α3x(t))y(t), | (2.1b) |
where x(t) represents the concentration of population biomass — the mass of the population per unit volume of the aquatic environment — at time t, y(t) represents the body burden of the population — the mass of toxin per unit population biomass — at time t. The model parameters α1,α2,α3, k,m,a,Te, and σ are all positive constants. See [11] for the detailed model construction.
Equation (2.1a) presents the rate of change of the population biomass under the influence of the toxin. The term, α1max{0,1−α2y(t)}/(1+α3x(t)), describes the population gain rate that depends on the population biomass and the body burden, α1/(1+α3x(t)), a decreasing function with respect to population biomass, is a density-dependent biomass gain rate due to birth and growth, where α1 is the maximum gain rate and α3 denotes the crowding effect. The expression, max{0,1−α2y(t)}, is a fraction between 0 and 1, which represents linear toxic responses for the gain rate. If there is no toxic effect (i.e., body burden y(t)=0), then max{0,1−α2y(t)}=1, the gain rate of population biomass is α1/(1+α3x(t)). If the body burden yi reaches a threshold level 1/α2, then max{0,1−α2y(t)}=0, the gain rate of population biomass is 0. In this case, the population stops reproduction and growth. The term, ky(t)+m, means that the population mortality rate linearly depends on the body burden y(t), where k is the effect coefficient of toxin on mortality, and m is the natural mortality rate. If there is no toxic effect, i.e., y(t)=0, then the mortality rate is given by the natural mortality rate m.
Equation (2.1b) is a balance equation for the body burden, where a represents the toxin uptake rate by the population from the environment and Te is the concentration of toxin in the environment — the mass of toxin per unit volume of the aquatic environment. σ is the toxin elimination rate due to the metabolic processes of the population. The last term of (2.1b), α1max{0,1−α2y(t)}y(t)/(1+α3x(t)), means toxin dilution due to birth and growth.
Model (2.1) assumes that the toxic responses (i.e., the effects of toxin on population growth and mortality) are immediate. In this paper, we extend model (2.1) by considering delayed toxic responses. To this end, we introduce two time delays τ1 and τ2, which are associated with the effects of toxins on growth and mortality, respectively. Then a modified model from (2.1) reads
dx(t)dt=(α1max{0,1−α2y(t−τ1)}1+α3x(t)−ky(t−τ2)−m)x(t), | (2.2a) |
dy(t)dt=aTe−(σ+α1max{0,1−α2y(t−τ1)}1+α3x(t))y(t), | (2.2b) |
We non-dimensionalize system (2.2) by introducing the following dimensionless quantities:
˜x(˜t)=α3x(t),˜y(˜t)=α2y(t),˜t=α1t,˜τ1=α1τ1,˜τ2=α1τ2,˜k=kα1α2,˜m=mα1,˜T=α2aTeα1,˜σ=σα1. |
Dropping the tildes for notational simplicity, we obtain the following scaled model:
dx(t)dt=(max{0,1−y(t−τ1)}1+x(t)−ky(t−τ2)−m)x(t), | (2.3a) |
dy(t)dt=T−(σ+max{0,1−y(t−τ1)}1+x(t))y(t). | (2.3b) |
We denote by C the Banach space of continuous functions ϕ:[−τ,0]→R2 equipped with the suitable norm, where τ=max{τ1,τ2}. Furthermore, let
C+=ϕ=(ϕ1,ϕ2)∈C:ϕi(θ)≥0for allθ∈[−τ,0],i=1,2. |
The initial conditions of system (2.3) are given as
x(θ)=ϕ1(θ)>0,y(θ)=ϕ2(θ)>0,θ∈[−τ,0]. | (2.4) |
First of all, we show that solutions of system (2.3) behave in a biologically reasonable manner.
Proposition 3.1. The solutions of system (2.3) are always nonnegative and bounded under the initial conditions (2.4).
Proof. Since the right-hand side of the system (2.3) is locally Lipschitz-continuous in R2+={(x(t),y(t))|x(t),y(t)≥0}, we obtain
x(t)=x(t0)exp{∫tt0(max{0,1−y(s−τ1)}1+x(s)−ky(s−τ2)−m)ds}>0,y(t)=y(t0)exp{∫tt0(Ty(s)−σ−max{0,1−y(s−τ1)}1+x(s))ds}>0. |
Using the positive property of solutions, we get
dx(t)dt≤x(11+x−m)<1−mx,dy(t)dt≤T−σy. |
According to the comparison principle, we have
lim supt→∞x(t)≤1m,lim supt→∞y(t)≤Tσ. |
So, any solutions of system (2.3) with the initial conditions (2.4) will stay in the rectangular region:
{(x,y)∈R2|0≤x<1m,0≤y<Tσ}. |
The existence and stability conditions of equilibria of system (2.3) without time delays were discussed in [11]. Here we summarize the results as follows.
Lemma 3.1. Consider system (2.3) when τ1=0 and τ2=0.
(i) When 0<σ<1, system (2.3) has the boundary equilibrium
E01=(0,y01),wherey01∈(0,(σ+1)/2),0<T<σ,E0i=(0,y0i),i=1,2,where0<y01<σ+12<y02<1,σ<T<(σ+1)24,E03=(0,y03),wherey03>1,T>(σ+1)24. |
(ii) When σ≥1, system (2.3) has the boundary equilibrium
E01=(0,y01),wherey01∈(0,1),0<T<σ,E03=(0,y03),wherey03>1,T>σ, |
where
y01=σ+1−√(σ+1)2−4T2,y02=σ+1+√(σ+1)2−4T2,y03=Tσ. |
(iii) When
0<m<1,T<(1−m)[σ(k+1)+k+m](k+1)2:=Tc, |
system (2.3) has a unique interior equilibrium
E∗=(1−y∗ky∗+m−1,y∗), |
where
y∗=−(m+σ)+√(m+σ)2+4kT2k. |
Lemma 3.2. Consider system (2.3) when τ1=0 and τ2=0.
(i) When E01 exists, it is stable if σ>1−(2m+k)1+k.
(ii) When E02 exists, it is always unstable.
(iii) When E03 exists, it is always stable.
(iv) When E∗ exists, it is stable if T<kθ2+(m+σ)θ:=Tθ, where
θ=−(2km+3m−2k+σ)+√(2km+3m−2k+σ)2−4(k2+3k)(m2−2m−σ)2(k2+3k). |
Next, we discuss the effects of τ1 and τ2 on the stability of the interior equilibrium E∗. Note that 0<y∗<1, we have max{0,1−y∗}=1−y∗.
Case (I) τ1>0,τ2=0.
Theorem 3.1. For system (2.3), suppose that τ1>0,τ2=0, then when E∗ exists, it is locally asymptotically stable if T<Tθ.
Proof. When τ1>0,τ2=0, linearizing system (2.3) at E∗, we have
dx(t)dt=(1−y∗(1+x∗)2−ky∗−m)x(t)−kx∗y(t)−(x∗1+x∗)y(t−τ1), | (3.1a) |
dy(t)dt=(y∗(1−y∗)(1+x∗)2)x(t)−(σ+1−y∗1+x∗)y(t)+(y∗1+x∗)y(t−τ1). | (3.1b) |
Let →Z(t)=(x(t),y(t))T, system (3.1) can be transformed as
d→Zdt=A→Z(t)+B→Z(t−τ1), | (3.2) |
where
A=[1−y∗(1+x∗)2−ky∗−m−kx∗y∗(1−y∗)(1+x∗)2−(σ+1−y∗1+x∗)],B=[0−x∗1+x∗0y∗1+x∗]. |
Let →Z=eλt→ξ,→ξ≠→0 in (3.2), then we obtain
λeλt→ξ=Aeλt→ξ+Beλ(t−τ1)→ξ, |
that is,
(λI−A−Be−λτ1)→ξ=→0, |
where I is an identity matrix. Therefore, the characteristic equation is
det(λI−A−Be−λτ1)=|λ−1−y∗(1+x∗)2+ky∗+mkx∗+x∗1+x∗e−λτ1−y∗(1−y∗)(1+x∗)2λ+σ+1−y∗1+x∗−y∗1+x∗e−λτ1|=0, |
which can be written as the form
P(λ)+Q(λ)e−λτ1=0, | (3.3) |
where
P(λ)=λ2+D1λ+E1,Q(λ)=D2λ, |
and
D1=(k2+2k)(y∗)2+(2km+2m−2k+σ)y∗+m2−2m−σy∗−1,E1=(ky∗+m)(ky∗+y∗−1+m)(2ky∗+σ+m)y∗−1,D2=k(y∗)2+my∗y∗−1. |
Since E∗=(1−y∗ky∗+m−1,y∗) implies that 0<y∗<1 and ky∗+y∗+m<1, we have that E1>0,D2<0.
Let ω>0 and suppose λ=iω is a pure imaginary root of equation (3.3). Separating the real and imaginary parts, we have
ωD2sin(ωτ1)=ω2−E1, | (3.4a) |
ωD2cos(ωτ1)=−ωD1. | (3.4b) |
Thus
h1(ω)=ω4+a1ω2+a2=0, | (3.5) |
where
a1=D21−2E1−D22,a2=E21>0. |
Then we have
Δ:=(a1)2−4a2=(D21−2E1−D22)2−4E21=(D1+D2)(D1−D2)(D21−4E1−D22). | (3.6) |
Equation (3.5) has positive roots if and only if
a1<0,Δ≥0. |
Since T<Tθ, D1+D2>0, we have D1>0, D1−D2>0. Besides, due to D21−4E1−D22=a1−2E1<0, we have Δ<0. It is a contradiction. So equation (3.5) has no positive root.
Therefore, when τ1>0,τ2=0 and E∗ exists, it is locally asymptotically stable if T<Tθ.
Case (II) τ1=0,τ2>0.
Theorem 3.2. For system (2.3), suppose that τ1=0,τ2>0, if (A1) holds, then there is a positive integer m such that
0<τ(0)2,+<τ(0)2,−<τ(1)2,+<⋯<τ(m−1)2,−<τ(m)2,+. |
E∗ is locally asymptotically stable if τ2∈(0,τ(0)2,+)∪(τ(0)2,−,τ(1)2,+)∪⋯∪(τ(m−1)2,−,τ(m)2,+), and E∗ is unstable if τ2∈(τ(0)2,+,τ(0)2,−)∪(τ(1)2,+,τ(1)2,−)∪⋯∪(τ(m)2,+,∞). Furthermore, if (A2) holds, then system (2.3) undergoes Hopf bifurcation at τ∗2, where τ∗2=τ(0)2=min{τ(0)2,+,τ(0)2,−}.
Proof. When τ1=0,τ2>0, linearizing system (2.3) at E∗, we get
dx(t)dt=(1−y∗(1+x∗)2−ky∗−m)x(t)−kx∗y(t−τ2)−(x∗1+x∗)y(t),dy(t)dt=(y∗(1−y∗)(1+x∗)2)x(t)−(σ+1−2y∗1+x∗)y(t). |
Similarly, the characteristic equation is given by
P(λ)+Q(λ)e−λτ2=0, | (3.7) |
where
P(λ)=λ2+F1λ+G1,Q(λ)=G2, |
and
F1=(k2+3k)(y∗)2+(2km+3m−2k+σ)y∗+m2−2m−σy∗−1,G1=(ky∗+m)(ky∗+y∗−1+m)(ky∗+σ+m)y∗−1,G2=(ky∗+m)(ky∗+y∗−1+m)ky∗y∗−1. |
Since 0<y∗<1 and ky∗+y∗+m<1, we have G1>0,G2>0. And since T<kθ2+(m+σ)θ, we have F1>0.
Let ω>0 and suppose λ=iω is a pure imaginary root of equation (3.3). Separating the real and imaginary parts, we have
G2cos(ωτ2)=ω2−G1, | (3.8a) |
G2sin(ωτ2)=ωF1. | (3.8b) |
Thus
h2(ω)=ω4+b1ω2+b2=0, | (3.9) |
where
b1=F21−2G1,b2=G21−G22>0. |
(A1): Suppose b1<0,(b1)2−4b2>0.
Equation (3.9) has two positive roots ω+ and ω−, where
ω+=(−b1+√(b1)2−4b22)1/2, | (3.10a) |
ω−=(−b1−√(b1)2−4b22)1/2. | (3.10b) |
According to (3.8), we obtain
cos(ω±τ2)=ω2±−G1G2, | (3.11a) |
sin(ω±τ2)=F1ω±G2>0. | (3.11b) |
Then solving equation (3.11) for τ2 yields
τ(j)2=1ω±arccos(ω2±−G1G2)+2jπω±,j=0,1,2,…. | (3.12) |
Define
τ∗2=τ(0)2=min{τ(0)2,+,τ(0)2,−},ω∗=ω(0)2. | (3.13) |
Next we obtain the transversality conditions for the Hopf bifurcation at τ2=τ∗2.
Let λ(τ2)=υ(τ2)+iω(τ2) be the root of equation (3.7). When τ2=τ∗2, we have υ(τ∗2)=0 and ω(τ∗2)=ω∗.
Differentiating equation (3.7) with respect to τ2, we have
(dλdτ2)−1=2λ+F1−G2τ2e−λτ2G2λe−λτ2=(2λ+F1)eλτ2G2λ−τ2λ=2ω2+F21−2G1(ω2−G1)2+F21ω2−τ2λ. |
Thus
sign[d(Re(λ))dτ2]τ2=τ∗2=sign[Re(dλdτ2)−1]τ2=τ∗2=sign[2ω∗2+F21−2G1(ω∗2−G1)2+F21ω∗2]=sign[h′2(ω∗2)]. |
Hence if the following conditon
(A2):h′2(ω∗2)≠0
holds, then the transversality condition for the Hopf bifurcation is satisfied.
Case (III) τ1∈(0,∞),τ2>0.
Theorem 3.3. For system (2.3), suppose that for each fixed τ1∈(0,∞),τ2>0, if (A3) holds, then there is a positive integer n such that
0<τ(1,0)2<τ(2,0)2<τ(3,0)2<τ(4,0)2<τ(1,1)2<⋯<τ(4,n−1)2<τ(1,n)2<τ(2,n)2<τ(3,n)2<τ(4,n)2. |
E∗ is locally asymptotically stable if τ2∈(0,τ(1,0)2)∪(τ(2,0)2,τ(3,0)2)∪⋯∪(τ(3,n)2,τ(4,n)2), and E∗ is unstable if τ2∈(τ(1,0)2,τ(2,0)2)∪(τ(3,0)2,τ(4,0)2)∪⋯∪(τ(4,n)2,∞). Furthermore, if (A4) holds, then system (2.3) undergoes Hopf bifurcation at τ⋆2, where τ⋆2=τ(k0,0)2=mink∈{1,...,km}{τ(k,0)2}.
Proof. We assume that τ1 is arbitrarily fixed within the stable interval τ1∈(0,∞), while τ2 is varied as a control parameter. We linearize the system (2.3) at E∗ to obtain
dx(t)dt=(1−y∗(1+x∗)2−ky∗−m)x(t)−(x∗1+x∗)y(t−τ1)−kx∗y(t−τ2),dy(t)dt=(y∗(1−y∗)(1+x∗)2)x(t)−(σ+1−y∗1+x∗)y(t)+(y∗1+x∗)y(t−τ1). |
Similarly, the characteristic equation is given by
P(λ)+Q(λ)e−λτ1+R(λ)e−λτ2=0, | (3.14) |
where P(λ)=λ2+H1λ+I1, Q(λ)=H2λ, R(λ)=I2,
and
H1=(k2+2k)(y∗)2+(2km+2m−2k+σ)y∗+m2−2m−σy∗−1>0,I1=(ky∗+m)(ky∗+y∗−1+m)(ky∗+σ+m)y∗−1>0,H2=k(y∗)2+my∗y∗−1<0,I2=(ky∗+m)(ky∗+y∗−1+m)ky∗y∗−1>0. |
The corresponding real and imaginary parts become
I2cos(ωτ2)=ω2−I1−H2ωsin(ωτ1), | (3.15a) |
−I2sin(ωτ2)=−H1ω−H2ωcos(ωτ1). | (3.15b) |
We obtain
h3(ω)=ω4+c1ω3+c2ω2+c3ω+c4=0, | (3.16) |
where ci(i=1,2,3,4) are given by
c1=−2H2sin(ωτ1),c2=H21+2H1H2cos(ωτ1)+H22−2I1,c3=2H2I1sin(ωτ1),c4=I21−I22. |
(A3): Suppose that (3.16) has at least one positive root.
Let km denote the maximum number of positive real roots of equation (3.16). For k∈1,⋯,km, positive roots of equation (3.16) are denoted by ωk.
According to (3.15), we obtain
cos(ωkτ2)=ω2k−I1−H2ωksin(ωkτ1)I2:=J(ωk), | (3.17a) |
sin(ωkτ2)=H1ωk+H2ωkcos(ωkτ1)I2:=K(ωk). | (3.17b) |
Then solving the equation (3.17) for τ2 yields
τ(k,j)2={1ωk[arccos(ω2k−I1−H2ωksin(ωkτ1)I2)+2jπ],K(ωk)≥0,j=0,1,2…1ωk[2π−arccos(ω2k−I1−H2ωksin(ωkτ1)I2)+2jπ],K(ωk)<0,j=0,1,2… |
We define
τ⋆2=τ(k0,0)2=mink∈{1,...,km}{τ(k,0)2},ω⋆=ωk0. | (3.18) |
By differentiating equation (3.14) with respect to τ2, we get that
P1(τ2)[d(Reλ)dτ2]τ2=τ⋆2−Q1(τ2)[dωdτ2]τ2=τ⋆2=R1(τ2), | (3.19) |
Q1(τ2)[d(Reλ)dτ2]τ2=τ⋆2+P1(τ2)[dωdτ2]τ2=τ⋆2=S1(τ2). | (3.20) |
where
P1(τ2)=H1+H2cos(ω⋆τ1)+H2τ1ω⋆sin(ω⋆τ1)−I2τ2cos(ω⋆τ2),Q1(τ2)=2ω⋆−H2sin(ω⋆τ1)−H2ω⋆τ1cos(ω⋆τ1)+I2τ2sin(ω⋆τ2),R1(τ2)=−H2(ω⋆)2cos(ω⋆τ1)+I2ω⋆sin(ω⋆τ2),S1(τ2)=H2(ω⋆)2sin(ω⋆τ1)+I2ω⋆cos(ω⋆τ2). |
Consequently, we obtain
[d(Reλ)dτ2]τ2=τ⋆2=P1(τ2)R1(τ2)+Q1(τ2)S1(τ2)P1(τ2)2+Q1(τ2)2|τ2=τ⋆2. |
Hence, if the conditon
(A4): [P1(τ2)R1(τ2)+Q1(τ2)S1(τ2)]|τ2=τ⋆2≠0
holds, then the transversality condition for Hopf bifurcation is satisfied.
In this section, we numerically study the influence of time delays τ1 and τ2 on the stability of the interior equilibrium E∗ of system (2.3). We choose a set of parameter values as k=0.2,m=0.01,σ=0.1,T=0.16. By some calculations, we have E∗=(1.38658,0.660748), Tθ=0.170329,Tc=0.226875. According to Lemma 3.2, E∗ is stable when τ1=τ2=0. Stability regions of the interior equilibrium E∗ of system (2.3) in (τ1,τ2) plane are drawn in Figure 1. We then consider the following three cases and plot some graphs of the numerical solutions of system (2.3).
Case(I) τ1>0,τ2=0.
From (3.5) and (3.6), we have a1=−0.0330221<0 and Δ=−0.00273187<0. So equation (3.5) has no positive root. According to Theorem 3.1, E∗ is locally asymptotically stable when τ1>0,τ2=0, which means that τ1 can not change the stability of E∗ (see Figure 2).
Case(II) τ1=0,τ2>0.
From (3.10a) and (3.10b), we have ω+=0.165797,ω−=0.101075. According to Theorem 3.2, there is a positive integer m=1 such that 0<τ(0)2,+<τ(0)2,−<τ(1)2,+, where τ(0)2,+=4.9121,τ(0)2,−=26.5368,τ(1)2,+=42.8089. E∗ is locally asymptotically stable if τ2∈(0,τ(0)2,+)∪(τ(0)2,−,τ(1)2,+), and E∗ is unstable if τ2∈(τ(0)2,+,τ(0)2,−)∪(τ(1)2,+,∞). When we choose τ2=4, E∗ is stable (see Figure 3a). However, when τ2 increases to 5>4.9121, E∗ becomes unstable and tends to the boundary equilibrium. In this case, the subcritical Hopf bifurcation appears resulting in an unstable limit cycle (see Figure 3b). As τ2 increase to 30, E∗ becomes stable again (see Figure 3c). When τ2 increases to 45>42.8089, E∗ becomes unstable again and tends to the boundary equilibrium (see Figure 3d). Furthermore, system (2.3) undergoes Hopf bifurcation at τ∗2=τ(0)2,+=4.9121 (see Figure 1).
Case(III) τ1∈(0,∞),τ2>0.
Here we fix τ1=5. By calculations, equation (3.16) has two positive roots: ω1=0.105931,ω2=0.0646036. According to Theorem 3.3, there is a positive integer n=1 such that 0<τ(1,0)2<τ(2,0)2<τ(1,1)2, where τ(1,0)2=9.29205,τ(2,0)2=42.7927,τ(1,1)2=68.6063. E∗ is locally asymptotically stable if τ2∈(0,τ(1,0)2)∪(τ(2,0)2,τ(1,1)2), and E∗ is unstable if τ2∈(τ(1,0)2,τ(2,0)2)∪(τ(1,1)2,∞). When we choose τ2=8, E∗ is stable (see Figure 4a). However, when τ2 increases to 10>9.29205, E∗ becomes unstable and tends to the boundary equilibrium. In this case, the subcritical Hopf bifurcation appears resulting in an unstable limit cycle (see Figure 4b). As τ2 increase to 45, E∗ becomes stable again (see Figure 4c). When τ2 increases to 70>68.6063, E∗ becomes unstable again and tends to the boundary equilibrium (see Figure 4d). Furthermore, system (2.3) undergoes Hopf bifurcation at τ⋆2=τ(1,0)2=9.29205 (see Figure 1). Although the introduction of τ1 has no effects on the stability of E∗, it can increase the critical values of τ2 which can destabilize E∗.
In order to understand the effects of toxins on the dynamics of populations in polluted environments, a large number of mathematical models have been developed [4,5]. The existing models usually assume that the effects of toxins on population reproduction and mortality are instant. However, there are evidences showing that toxic effects on populations may be delayed. This motivates us to propose a new mathematical model (2.3) with delayed toxic responses in this paper. In the model, two discrete delays τ1 and τ2 represent the delayed effects of toxins on the reproduction and mortality of the population, respectively. We mainly focused on the effects of τ1 and τ2 on the stability of interior equilibrium E∗, at which the population persists. The results of theoretical analysis showed that the delayed effect on the reproduction of the aquatic population does not change the stability of E∗, but the delayed effect on the mortality of the population significantly affects long-term population dynamics. It turns out that the delayed mortality effects not only produces population oscillations, but also change the fluctuation amplitudes. So, we suggest that experimental biologists should pay more attention to the delayed mortality caused by environmental toxins.
An interesting and challenging future work is the global stability analysis of the equilibria of model (2.3). The delay model (2.3) we studied in this work can be extended in several biologically meaningful ways: (1) The delayed toxic responses in our model are constant. Indeed, the delays can depend on toxin levels or some other environmental factors. We can modify our model such that the delays are functions of the body burden. (2) In a population, different development stages may have different sensitivities to toxins and different delayed toxic responses, so it is worth extending the current model to a stage-structured population model by taking these factors into account. (3) Different species living in the same environment may have different vulnerabilities to toxins, the impact of toxins on the interactions between different species in polluted aquatic environments was studied in [30] and [31]. We plan to investigate how delayed toxic responses affect the long-term dynamics of two interactive species in the future.
The first author is supported by the National Natural Science Foundation of China (11901225), the Natural Science Foundation of Hubei Province (2019CFB189), and the Fundamental Research Funds for the Central Universities (CCNU18XJ041). The third author is supported by the National Natural Science Foundation of China (11871060), the Venture and Innovation Support Program for Chongqing Overseas Returnees (7820100158), the Fundamental Research Funds for the Central Universities (XDJK2018B031), and the faculty startup fund from Southwest University (20710948).
The author declares no conflicts of interest in this paper.
[1] | Lect. Notes Phys., 368 (1990), p5. |
[2] | J. Physiol., 213 (1971), 31-53. |
[3] | Computational Neuroscience, MIT Press, Cambridge, MA, 2001. |
[4] | J. Math. Biol., 67 (2012), 989-1017. |
[5] | J. Math. Biol., 67 (2013), 239-259. |
[6] | Biophys. J., 1 (1961), 445-466. |
[7] | Int. J. Bifurcat. Chaos, 16 (2006), 887-910. |
[8] | J. Physiol., 117 (1952), 500-544. |
[9] | The MIT Press, 2007. |
[10] | Biol. Cybern., 66 (1992), p381. |
[11] | Biofizika, 18 (1973), p506. |
[12] | Biol. Cybern., 67 (1992), 461-468. |
[13] | Biophys. J., 35 (1981), 193-213. |
[14] | Proc. IRE, 50 (1962), 2061-2070. |
[15] | J. Phys. Soc. Jpn., 41 (1976), 1815-1816. |
[16] | Phil. Trans. R Soc. Lond. A, 337 (1991), 275-289. |
[17] | 3rd edition, Texts in Applied Mathematics, 7, Springer, 2000. |
[18] | PLoS Comput. Biol., 6 (2010), e1000850, 16 pp. |
[19] | IEEE T. Bio. Med. Eng., 51 (2004), 1665-1672. |
[20] | Phys. Rev. E, 90 (2014), 022701. |
[21] | in Nonautonomous Dynamical Systems in the Life Sciences, Lecture Nones in Math., 2102, Springer, 2013, 89-132. |
1. | Hewei Gao, Xin Huo, Chao Zhu, 2023, An Input Module of Deep Learning for the Analysis of Time Series with Unequal Length, 979-8-3503-1125-9, 1, 10.1109/ICPS58381.2023.10128044 |