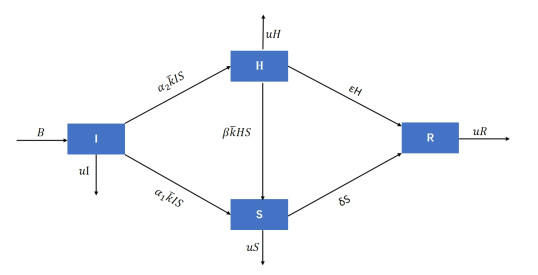
With the rapid rise and widespread adoption of social media, theoretical research on the dynamics of online information dissemination has become increasingly important. Therefore, we developed a new model of information diffusion that took into account the influence of information recipients on the diffusion of information. First, initially, the basic reproduction number of the model was calculated. Then, we analyzed the existence and stability of the equilibrium point. Next, based on the principle of Pontryagin's maximum principle, a control strategy was derived to effectively enhance the propagation of information. Numerical simulations verified the results of theoretical analysis. The results showed that increasing the proportion of propagators and the probability of beneficiaries transitioning into propagators significantly accelerated the speed and extent of information diffusion.
Citation: Xuechao Zhang, Yuhan Hu, Shichang Lu, Haomiao Guo, Xiaoyan Wei, Jun li. Dynamic analysis and control of online information dissemination model considering information beneficiaries[J]. AIMS Mathematics, 2025, 10(3): 4992-5020. doi: 10.3934/math.2025229
[1] | Xintong Wang, Sida Kang, Yuhan Hu . Dynamic analysis of a rumor propagation model considering individual identification ability. AIMS Mathematics, 2025, 10(2): 2295-2320. doi: 10.3934/math.2025107 |
[2] | Youming Guo, Tingting Li . Dynamics and optimal control of an online game addiction model with considering family education. AIMS Mathematics, 2022, 7(3): 3745-3770. doi: 10.3934/math.2022208 |
[3] | E. Bonyah, C. W. Chukwu, M. L. Juga, Fatmawati . Modeling fractional-order dynamics of Syphilis via Mittag-Leffler law. AIMS Mathematics, 2021, 6(8): 8367-8389. doi: 10.3934/math.2021485 |
[4] | Hongshuang Wang, Sida Kang, Yuhan Hu . Dynamic analysis and optimal control of rumor propagation models considering different education levels and hesitation mechanisms. AIMS Mathematics, 2024, 9(8): 20089-20117. doi: 10.3934/math.2024979 |
[5] | Rahim ud Din, Kamal Shah, Manar A. Alqudah, Thabet Abdeljawad, Fahd Jarad . Mathematical study of SIR epidemic model under convex incidence rate. AIMS Mathematics, 2020, 5(6): 7548-7561. doi: 10.3934/math.2020483 |
[6] | Sayed Saber, Azza M. Alghamdi, Ghada A. Ahmed, Khulud M. Alshehri . Mathematical Modelling and optimal control of pneumonia disease in sheep and goats in Al-Baha region with cost-effective strategies. AIMS Mathematics, 2022, 7(7): 12011-12049. doi: 10.3934/math.2022669 |
[7] | Kamel Guedri, Rahat Zarin, and Mowffaq Oreijah . Evaluating the impact of vaccination and progression delays on tuberculosis dynamics with disability outcomes: A case study in Saudi Arabia. AIMS Mathematics, 2025, 10(4): 7970-8001. doi: 10.3934/math.2025366 |
[8] | Yasir Ramzan, Aziz Ullah Awan, Muhammad Ozair, Takasar Hussain, Rahimah Mahat . Innovative strategies for Lassa fever epidemic control: a groundbreaking study. AIMS Mathematics, 2023, 8(12): 30790-30812. doi: 10.3934/math.20231574 |
[9] | Lidiya Melnikova, Valeriy Rozenberg . One dynamical input reconstruction problem: tuning of solving algorithm via numerical experiments. AIMS Mathematics, 2019, 4(3): 699-713. doi: 10.3934/math.2019.3.699 |
[10] | Salma M. Al-Tuwairqi, Asma A. Badrah . Modeling the dynamics of innate and adaptive immune response to Parkinson's disease with immunotherapy. AIMS Mathematics, 2023, 8(1): 1800-1832. doi: 10.3934/math.2023093 |
With the rapid rise and widespread adoption of social media, theoretical research on the dynamics of online information dissemination has become increasingly important. Therefore, we developed a new model of information diffusion that took into account the influence of information recipients on the diffusion of information. First, initially, the basic reproduction number of the model was calculated. Then, we analyzed the existence and stability of the equilibrium point. Next, based on the principle of Pontryagin's maximum principle, a control strategy was derived to effectively enhance the propagation of information. Numerical simulations verified the results of theoretical analysis. The results showed that increasing the proportion of propagators and the probability of beneficiaries transitioning into propagators significantly accelerated the speed and extent of information diffusion.
The rapid development of information technology and the widespread adoption of social networks have led to exponential growth in the speed and influence of information dissemination [1]. While this phenomenon has promoted social progress, it has also introduced issues such as information overload and the spread of false information [2,3]. The importance of information dissemination in modern society has become increasingly prominent, and its dissemination paths, driving mechanisms, and control strategies have become key areas of interest for both academia and practical applications [4,5].
Information dissemination shares significant similarities with the spread of infectious diseases [6,7]. Both information propagation models and rumor spreading frameworks originate from a unified theoretical foundation in epidemiological dynamics. Just as an infectious disease spreads through a population via contact, air, or other means, information can spread rapidly through text, images, or words. The two are also highly correlated [8]. The spread of information affects the prevention and control of epidemics, and the spread of rumors can lead to social panic and hinder epidemic control. Epidemics, in turn, generate a large amount of related information, leading to confusion in the court of public opinion. Understanding and utilizing these similarities are essential for guiding public opinion and effective outbreak control[9].
Early studies on infectious disease transmission laid the foundation for information dissemination models. Ross et al.[10] proposed frequency-dependent models in the early 20th century, considering contact frequency. These models became the cornerstone of mathematical modeling for infectious diseases and inspired later research on rumors and information dissemination. In the 1960s, Daley and Kendall proposed the DK model [11,12]. Later, Maki and Thompson [13] extended it into the MT model in 1973. Based on these models, scholars have proposed various enhancements, such as hesitation mechanisms, time-delay models [14,15,16], and network-based dissemination models [17]. These models reflect how information spreads within network environments, incorporating factors like "small-world" effects [18], tipping points, and universal patterns similar to viral transmission [19].
In recent years, research on information dissemination has shifted from a single-dimensional perspective to a comprehensive view that integrates multiple factors. Some studies focus on the impact of individual behavioral characteristics on dissemination paths, such as individual acceptance, hesitation mechanisms [20,21], and social responsibility on dissemination efficiency [22,23]. Others examine the role of external environmental factors, such as the influence of digital technologies, media guidance, and network structures on the scale and speed of dissemination [24,25]. For instance, trust mechanisms have been shown to effectively suppress the spread of false information while extending the dissemination time window, thereby providing more opportunities for intervention [26]. Additionally, media coverage and government guidance have been verified to enhance dissemination effectiveness in related models [27,28,29]. By comprehensively analyzing the roles of individual behavior and environmental variables, it is possible to gain a more holistic understanding of the dynamic mechanisms underlying information dissemination [30,31].
Existing models, such as the SI (susceptible-infected model) and SHIR (susceptible-hidden-infected-recovered model) models, mainly focus on simple interactions between disseminators and receivers, neglecting the influence of network structure and individual behavior. While some models address group dynamics or individual characteristics, few integrate both perspectives[32,33,34]. This paper introduces a novel model that incorporates network degree and information beneficiaries, considering both individual behavior and network structure, to better predict dissemination outcomes and inform intervention strategies.
Based on previous research, this study proposes a novel information dissemination model that introduces the concept of information beneficiaries and explores their roles in the dissemination process and their impact on dissemination efficiency [35]. Unlike traditional models, this model considers not only the interactions between disseminators and receivers but also integrates network structures and individual behavioral characteristics, thereby constructing a more explanatory and predictive dynamic framework. Through theoretical analysis and numerical simulations, this study reveals the effects of key parameters (e.g., network average degree and dissemination probability) on dissemination scale and time. Additionally, it identifies the phenomenon of dissemination outbreaks, providing new perspectives for predicting and controlling dissemination risks.
The remainder of this paper is organized as follows: First, the construction and assumptions of the model are introduced. Next, the stability of equilibrium points and the influence of key parameters are analyzed. Then, numerical simulations are conducted to verify the model's validity, and intervention strategies for optimizing dissemination are proposed. Finally, research findings are summarized, and future research directions are suggested.
In this work, an open virtual community is considered. The population size is variable at any time t, and the total population is expressed as N(t). The population can be divided into four categories:
(1) The easy adopters who are not exposed to information but easily adopt the information, denoted as I(t);
(2) people who are exposed to information and gain its value, becoming information beneficiaries, are defined as H(t);
(3) people who are exposed to information and become information disseminators are defined as S(t);
(4) the fleeing crowd that is not interested in any kind of information as well as the corresponding variation information, denoted as R(t).
The IHSR information beneficiary dissemination process is shown in Figure 1. In addition, the information beneficiary dissemination rules can be summarized as follows:
● In a social system, the number of individuals generally varies over time. Therefore, this work defines B as the number of immigrants in the social system. At the same time, it considers some individuals that may withdraw from the social system due to some force majeure factors. μ is defined as the emigration rate in this work;
● when the easy adopters come into contact with information disseminators, a portion of the easy adopters, due to subjective reasons, will become information disseminators at a contact rate defined as α1. Another portion of the easy adopters, driven by their own needs or higher self-learning capabilities, will become information beneficiaries at a contact rate defined as α2;
● after a period of learning, some information beneficiaries realize that the information can bring benefits to others, and they will become information disseminators at the progression rate of β, sharing the information with surrounding audience groups. Another group of individuals may forget the information after a period of learning and will become information forgetters at the progression rate of ε;
● the progression rate at which information beneficiaries S transition to information forgetters R, denoted by δ, is influenced by time, external distractions, or environmental factors. This parameter reflects the natural process by which individuals gradually lose previously acquired information due to cognitive decline, diverted attention, or forgetting mechanisms.
In our IHSR information dissemination model, the degree of a node, represented by ⟨k⟩=∑nk=1kp(k), represents the number of connections an individual has and is a key indicator of their influence within the network. Higher-degree nodes typically play a more significant role in the spread of information due to their larger number of connections. The degree distribution P(k) captures the heterogeneity in the network, reflecting the uneven distribution of connections, where few individuals have many links while most have few. This consideration allows our model to better simulate the complexity of information propagation across the population.
In the above analysis, all parameters are positive, thus obtaining a schematic diagram of the impact of network information dissemination on information beneficiaries.
The model for each parameter in the chart is shown in Table 1.
Parameter | Description |
I(t) | The number of individuals actively spreading the information at time t. |
H(t) | The number of individuals benefiting from the information at time t. |
S(t) | The number of individuals unaware of the information at time t. |
R(t) | The number of individuals who forget the information or are unaffected at time t. |
B | The number of immigrants in the social system. |
α1 | Progression rate from state I to S. |
α2 | Progression rate from state I to H. |
β | Progression rate from state S to H. |
ε | Progression rate from state H to R. |
δ | Progression rate from state S to R. |
μ | Removal rate per unit time. |
Based on these elements, we can model and analyze the process of information dissemination and diffusion among populations. We construct a model that considers the impact of network information dissemination on information beneficiaries. The system dynamics equation is described as follows:
{dIdt=B−(α1+α2)⟨k⟩IS−μI,dHdt=α2⟨k⟩IS−β⟨k⟩HS−(ε+μ)H,dSdt=α1⟨k⟩IS+β⟨k⟩HS−(δ+μ)S,dRdt=εH+δS−μR. | (2.1) |
Its initial conditions meet:
I(0)=I0≥0,H(0)=H0≥0,I(0)=I0≥0,R(0)=R0≥0. | (2.2) |
Theorem 1. The closed set Ω={(I,H,S,R)∈R4,N≤Bμ} is the positive invariant set of model (2.1).
Proof. Let the total number of people be N=I+H+S+R. We can get
dNdt=dIdt+dHdt+dSdt+dRdt=B−μN. | (3.1) |
It is clear that N(t)=(N0−Bμ)e−μt+Bμ, where N(t)=N0 and limt→+∞N(t)=Bμ. It can be seen that all solutions of the model are in the closed set Ω when the initial conditions are satisfied, and in summary it follows that the closed set Ω is the positive invariant set of the model (2.1).
Studying its basic regeneration number and equilibrium point is a prerequisite for predicting the population development trend within a population dynamics system. First, the basic reproduction number R0 of system (2.1) is calculated through the next generation matrix.
R0=α1⟨k⟩Bμ(δ+μ). | (3.2) |
We let LetX=(S,H,I,R), The equation of system (2.1) can be written as
dXdt=F(x)−V(x), | (3.3) |
where
F(X)=(α1⟨k⟩IS+β⟨k⟩HS000),V(X)=((δ+μ)S−α2⟨k⟩IS+β⟨k⟩HS+(ε+μ)H−B+(α1+α2)⟨k⟩SI+μI−εH−δS+μR), | (3.4) |
then, we get
F=(α1⟨k⟩I+β⟨k⟩Hβ⟨k⟩S00),V=(δ+μ0−α2⟨k⟩Bμε+μ), | (3.5) |
By calculation,
FV−1=(α1⟨k⟩Bμ(δ+μ)000). | (3.6) |
We obtain the eigenvalues of FV−1
|hE−fv−1(x)|=|h−α1⟨k⟩Bμ(δ+μ)00h|=h(h−α1⟨k⟩Bμ(δ+μ))=0, | (3.7) |
therefore,
R0=α1⟨k⟩Bμ(δ+μ). | (3.8) |
Through the calculation of system (2.1), we can obtain the basic reproduction number and non-negative equilibrium points.
● The information-free equilibrium point E0=(Bμ,0,0,0).
● When μ(ε+μ)(α+β)<(μ2−Bβ)γ is established, the information-free equilibrium point is E∗=(I∗,H∗,S∗,R∗). Here, we construct a basic reproduction number R0=α1⟨k⟩Bμ(δ+μ); when R0>1, there is an information-existence equilibrium point.
Next, we prove the existence of E∗.
{B−(α1+α2)⟨k⟩IS−μI=0,α2⟨k⟩IS−β⟨k⟩HS−(ε+μ)H=0,α1⟨k⟩IS+β⟨k⟩HS−(δ+μ)S=0,εH+δS−μR=0. | (3.9) |
By calculating system (3.9), we obtain the following equation:
R∗=εH+δSμ, | (3.10) |
I∗=B(α1+α2)⟨k⟩S∗+μ, | (3.11) |
H∗=(δ+μ)[(α1+α2)⟨k⟩S∗+μ]−α1⟨k⟩Bβ⟨k⟩[(α1+α2)⟨k⟩S∗+μ], | (3.12) |
S∗=(δ+μ)μ−α1⟨k⟩B(β⟨k⟩+(ε+μ))(δ+μ)(α1+α2)⟨k⟩−βα2⟨k⟩2B−(δ+μ)(α1+α2)⟨k⟩. | (3.13) |
Theorem 2. If R0<1, the information-free equilibrium point E0 is locally asymptotically stable.
Proof.
J(E0)=[−μ0−(α1+α2)⟨k⟩Bμ00−(ε+μ)α2⟨k⟩Bμ000α1⟨k⟩Bμ−(δ+μ)00εδ−μ]. | (3.14) |
Next, we calculate the eigenvalues of matrix (3.14), and we obtain
h1=h2=−μ<0,h3=−(ε+μ)<0,h4=α1⟨k⟩Bμ−(δ+μ)<0. |
Therefore, when R0<1, there is information-free equilibrium point E0 in the system, which is locally asymptotically stable.
Theorem 3. When R0<1, condition I⩽Bμ and B(α1+α2)⟨k⟩S<μ2(H+S+R) are satisfied, and E0 is globally asymptotically stable.
Proof. It is straightforward to observe that I(t)+H(t)+S(t)+R(t)=N(t) and satisfy dN(t)dt<B−μI(t). It illustrates that limt→0supN(t)⩽Bμ.
The positive invariant set of system (2.1) can be written as:
T={I(t),H(t),S(t),R(t)∈R+4:I(t)+H(t)+S(t)+R(t)⩽Bμ}. | (3.15) |
We construct the Lyapunov function[31] L(t)=H(t)+S(t)+R(t), and then we can get L′(t),
L′(t)=H′(t)+S′(t)+R′(t)=α2⟨k⟩IS−β⟨k⟩HS−(ε+μ)H+α1⟨k⟩IS+β⟨k⟩HS−(δ+μ)S+εH+δS−μR=(α1+α2)⟨k⟩IS−μ(H+S+R)⩽(α1+α2)⟨k⟩BμS−μ(H+S+R). | (3.16) |
Obviously, if I⩽Bμ and B(α1+α2)⟨k⟩S<μ2(H+S+R), then L′(t)⩽0 is always true.
Furthermore, L′(t)=0 is true if, and only if, I(t)=I0 and H(t)=S(t)=R(t)=0. From system (2.1), that at that time, E0 is the only solution in T. Therefore, based on the Lyapunov LaSalle invariance principle [33], it can be shown that every solution of the system (2.1) tends to E0 infinitely for t⟶∞. Therefore, the information free equilibrium E0 of system (2.1) is globally asymptotically stable.
Theorem 4. If R0>1, the information-existence equilibrium point E∗ is locally asymptotically stable.
Proof.
J(E∗)=[−(α1+α2)⟨k⟩S∗−μ0−(α1+α2)⟨k⟩I∗0α2⟨k⟩S∗−β⟨k⟩S∗−(ε+μ)α2⟨k⟩I∗−β⟨k⟩H∗0α1⟨k⟩S∗β⟨k⟩S∗α1⟨k⟩I∗+β⟨k⟩H∗−(δ+μ)00εδ−μ]. | (3.17) |
One of the roots of matrix (3.14) is −μ<0, and the other eigenvalues are calculated as follows:
|hE−J(E∗)|=0, | (3.18) |
at once,
|h+(α1+α2)⟨k⟩S∗+μ0(α1+α2)⟨k⟩I∗−α2⟨k⟩S∗h+β⟨k⟩S∗+(ε+μ)−α2⟨k⟩I∗+β⟨k⟩H∗−α1⟨k⟩S∗−β⟨k⟩S∗h−α1⟨k⟩I∗−β⟨k⟩H∗+(δ+μ)| =0. | (3.19) |
By calculating determinant (3.19), we obtain the Eq (3.20)
b3h3+b2h2+b1h+b0=0, | (3.20) |
where
b3=1, | (3.21) |
b2=β⟨k⟩S∗+ε+3μ+(α1+α2)⟨k⟩S∗+δ−α1⟨k⟩I∗−β⟨k⟩H∗, | (3.22) |
b1=(β⟨k⟩S∗+ε+2μ+(α1+α2)⟨k⟩S∗)(δ+μ−α1⟨k⟩I∗−β⟨k⟩H∗)+((α1+α2)⟨k⟩S∗+μ)(β⟨k⟩S∗+ε+μ)+β⟨k⟩S∗(β⟨k⟩H∗−α1⟨k⟩I∗)+α1(α1+α2)⟨k⟩2I∗S∗, | (3.23) |
b0=α2β(α1+α2)⟨k⟩3I∗S∗+α1(α1+α2)⟨k⟩2I∗S∗(β⟨k⟩S∗+ε+μ)+β⟨k⟩S∗(β⟨k⟩H∗−α1⟨k⟩I∗)((α1+α2)⟨k⟩S∗+μ)+((α1+α2)⟨k⟩S∗+μ)(β⟨k⟩S∗+ε+μ)(δ+μ−α1⟨k⟩I∗−β⟨k⟩H∗), | (3.24) |
and
b2b1−b3b0=(β⟨k⟩S∗+ε+μ)[(β⟨k⟩S∗+ε+2μ+(α1+α2)⟨k⟩S∗)(δ+μ−α1⟨k⟩I∗−β⟨k⟩H∗)+((α1+α2)⟨k⟩S∗+μ)(β⟨k⟩S∗+ε+μ)+β⟨k⟩S∗(β⟨k⟩H∗−α1⟨k⟩I∗)]+[δ+μ−α1⟨k⟩I∗−β⟨k⟩H∗][(β⟨k⟩S∗+ε+2μ+(α1+α2)⟨k⟩S∗)(δ+μ−α1⟨k⟩I∗−β⟨k⟩H∗+β⟨k⟩S∗(β⟨k⟩H∗−α1⟨k⟩I∗)+α1(α1+α2)⟨k⟩2I∗S∗]+(μ+(α1+α2)⟨k⟩S∗)[(β⟨k⟩S∗+ε+2μ+(α1+α2)⟨k⟩S∗)(δ+μ−α1⟨k⟩I∗−β⟨k⟩H∗)+((α1+α2)⟨k⟩S∗+μ)(β⟨k⟩S∗+ε+μ)]. | (3.25) |
Therefore, according to the Routh-Hurwitz criterion, when R0>1 and the conditions μ(δ+μ)>2α1⟨k⟩B and μ(δ+μ)>(α1+α2)α2B are satisfied, the information existence equilibrium E∗ is locally asymptotically stable.
Remark 1. All parameters used in the model are positive, as explained in Section 2, 'Model analysis'. In this section, we demonstrate that the positivity of all parameters leads to a schematic diagram illustrating the impact of network information dissemination on information beneficiary.
Theorem 5. When R0>1, the equilibrium point of system E∗ is globally asymptotically stable.
Proof.
L(t)=[(I(t)−I∗)+(H(t)−H∗)+(S(t)−S∗)+(R(t)−R∗)]2, | (3.26) |
and
L′(t)=2[(I(t)−I∗)+(H(t)−H∗)+(S(t)−S∗)+(R(t)−R∗)][I′(t)+H′(t)+S′(t)+R′(t)]=2[(I(t)−I∗)+(H(t)−H∗)+(S(t)−S∗)+(R(t)−R∗)][B−μI−μH−μS−μR]. | (3.27) |
Because E∗ exists, then
B−μI∗−μH∗−μS∗−μR∗=0, | (3.28) |
and
B=μI∗+μH∗+μS∗+μR∗. | (3.29) |
At this point,
L′(t)=2[(I(t)−I∗)+(H(t)−H∗)+(S(t)−S∗)+(R(t)−R∗)]×[μI∗+μH∗+μS∗+μR∗−μI−μH−μS−μR]=−2μ[(I−I∗)+(H−H∗)+(S−S∗)+(R−R∗)]2≤0. | (3.30) |
Furthermore, L′(t)=0 is true if, and only if, I(t)=I∗,H(t)=H∗,S(t)=S∗,R(t)=R∗. Therefore, based on the Lyapunov function and LaSalle invariance principle, it can be obtained that the rumored existence equilibrium point E∗=(I∗,H∗,S∗,R∗) of the system (2.1) is globally asymptotically stable.
In practice, there are numerous factors influencing the information dissemination process. Attempting to control all of these factors would be prohibitively expensive. Therefore, optimal control plays a crucial role by identifying and targeting the most critical factors that have the greatest impact on the spread of information. By focusing on these key factors, we can effectively reduce costs while still achieving significant control over the information dissemination process.
The model is defined as a population within a closed environment. This study proposes three control objectives: Within a specified control period, to increase the proportions of information disseminators and beneficiaries, thereby enhancing system stability. To achieve these objectives, three key parameters in the model are designed as time-dependent control variables: The probability of unaware individuals becoming information disseminators after contacting a disseminator, denoted as α1(t); the probability of unaware individuals becoming beneficiaries after contacting an information beneficiary, denoted as α2(t); and the probability of beneficiaries transforming into disseminators after realizing the benefits of the information, denoted as β(t). Through interventions such as public education, these variables can be adjusted to enhance the efficiency of information dissemination. Specifically, α1(t) controls the likelihood of unaware individuals becoming disseminators, α2(t) governs the conversion of unaware individuals into beneficiaries, and β(t) determines the transition of beneficiaries into disseminators. The control variables are constrained within the range 0≤α1(t),α2(t),β(t)≤1. When these parameter values are increased, the system achieves optimal dissemination efficiency, enabling rapid and effective information propagation. This study defines an objective function to quantitatively evaluate the impact of these optimal control strategies on the system's stability and performance.
Hence, the three proportion constants ω1, ω2, and ω3 in the model are transformed into control variables ω1(t), ω2(t), and ω3(t). The control variables ω1(t), ω2(t), and ω3(t) are used to regulate the proportion of conversions between groups within digitally accelerated systems.
In this model, the control variables are constrained as 0≤ω1(t)≤1, 0≤ω2(t)≤1, and 0≤ω3(t)≤1. When ω1(t)=1, ω2(t)=1, and ω3(t)=1, it indicates that the control effect is optimal, allowing information to be transmitted to the greatest extent. Conversely, when ω1(t)=0, ω2(t)=0, and ω3(t)=0, it signifies that the control measures are completely ineffective.
Hence, we propose an objective function as:
J(α1,α2,β)=tf∫0e−αt[H(t)+S(t)−c12α12(t)−c22α22(t)−c32β2(t)]dt. | (4.1) |
Remark 2. This paper was inspired by the study "Stability analysis of a delayed social epidemics model with general contact rate and its optimal control". From this work, a significant insight was gained regarding the optimization of control systems, particularly in closed environments. The origin of expression (4.1) stems from the need to assess how different control strategies influence system stability and performance. This formula was derived by transforming proportional constants into control variables, which regulate the conversion proportions between groups. The objective function, as defined in the paper, quantifies the effectiveness of these control measures. This formulation is crucial for understanding how optimal control strategies can maximize both information transmission and system stability, ensuring a balance between efficiency and control.
This satisfies the state system as:
{I′=B−(α1+α2)⟨k⟩IS−μI,H′=α2⟨k⟩IS−β⟨k⟩HS−(ε+μ)H,S′=α1⟨k⟩IS+β⟨k⟩HS−(δ+μ)S,R′=εH+δS−μR. | (4.2) |
Therefore
I(0)=I0,H(0)=H0,S(0)=S0,R(0)=R0. | (4.3) |
Here, α1(t), α2(t), β(t) is a measurable function, where α1(t), α2(t), β(t)∈U, and
0≤α1(t),α2(t)≤1,0≤β(t)≤1,∀t∈[0,tf]. | (4.4) |
Consider the control problem with system (4.2). There exists an optimal control:
u∗=(α∗1,α∗2,β∗)∈U, | (4.5) |
such that:
J(α∗1,α∗2,β∗)=minJ(α1(t),α2(t),β(t)). | (4.6) |
If the following conditions are met:
1. Both control variables and state variables are nonnegative.
2. The control admissible set U is convex and closed.
3. The integrand in the objective functional is a convex function defined in the admissible set U.
4. The righthand side of the state system is a linear bounded function of both the control variables and the state variables.
5. The existence of normal numbers d1,d2>0 and g>1 makes the integrand in the objective functional:
L(t;α1,α2,β)≜e−αt[H(t)+S(t)−c12α21(t)−c22α22(t)−c32β2(t)]. | (4.7) |
then, Eq (4.8) is satisfied.
Thus,
L(t;α1,α2,β)≥d1(|α1|2+|α2|2+|β|2)g2−d2. | (4.8) |
The conditions 1–3 are obviously valid, and only conditions 4 and 5 need to be proved.
Given that:
N(t)=I(t)+H(t)+S(t)+R(t), | (4.9) |
state variables have upper bounds N(t), so we also have upper bounds N(t) for each state variable.
S′<B,H′<α2(t)IS,S′<α1(t)IS+β(t)HS,R′<εH+δS. | (4.10) |
Hence, condition 5 is established.
For the last condition:
L(t;α1,α2,β)≥d1(|α1|2+|α2|2+|β|2)g2−d2, | (4.11) |
choosing:
d1=e−αt02min{c1,c2,c3},d2∈R+,g=2, | (4.12) |
can also prove to be satisfied.
According to the optimal control expression given in Eq (4.1), the optimal control system is obtained. To do this, define the following enlarged Hamiltonian operator Z with a penalty term[36]:
Z=e−αt[H(t)+S(t)+c12α21(t)+c22α22(t)+c32β21(t))]+(λ1[B−(α1+α2)⟨k⟩IS−μI]+(λ2[α2⟨k⟩IS−β⟨k⟩HS−(ε+μ)H]+(λ3[α1⟨k⟩IS+β⟨k⟩HS−(δ+μ)S]+(λ4[εH+δS−μR]−ω11(t)α1(t)−ω12(t)(1−α1(t))−ω21(t)α2(t)−ω22(t)(1−α2(t))−ω31(t)β1(t)−ω32(t)(1−β1(t)). | (4.13) |
Theorem 6. For the optimal control pair (α∗1,α∗2,β∗) of state system (4.2), there exist adjoint variables λi(i=1,2,3,4) that satisfy:
{λ′1=λ1[−(α1(t)+α2(t))⟨k⟩S−μ]+λ2α2(t)⟨k⟩S+λ3α1(t)⟨k⟩S,λ′2=−e−αt+λ1(−β(t)⟨k⟩S)+λ2β(t)⟨k⟩S+λ3β(t)⟨k⟩S+λ4ε,λ′3=−e−αt+λ1(−(α1(t)+α2(t))⟨k⟩I)−β(t)⟨k⟩H+λ3(α1(t)⟨k⟩I+β(t)⟨k⟩H−(δ(t)+μ))+λ4δ(t),λ′4=−λ4μ. | (4.14) |
In addition, the optimal control pair (α∗1,α∗2,β∗) of state system (4.2) can be given by:
{α∗1(t)=min{1,max{0,1c1eαt[(λ1−λ2+λ3)⟨k⟩IS+ω12−ω11]}},α∗2(t)=min{1,max{0,1c2eαt[λ2⟨k⟩IS+λ1⟨k⟩IS−ω21+ω22]}},β∗(t)=min{1,max{0,1c3eαt[λ3⟨k⟩HS−λ2⟨k⟩HS+ω32−ω31]}}. | (4.15) |
For the optimal control pair (α1∗,α2∗,β∗) of state system (4.2), there exist adjoint variables λ1,λ2,λ3,λ4 that satisfy:
λ′1=−∂Z∂I,λ′2=−∂Z∂H,λ′3=−∂Z∂S,λ′4=−∂Z∂R, | (4.16) |
with boundary conditions:
λ1=λ2=λ3=λ4=0. | (4.17) |
Take the derivative of Hamilton operator Z with respect to state variable U=(α1,α2,β) and set it to 0, and it can be seen that
{∂Z∂α1=e−αtc1α1(t)+(λ2−λ3)⟨k⟩IS−λ1⟨k⟩IS+ω11−ω12=0,∂Z∂α2=e−αtc2α2(t)−λ2⟨k⟩IS−λ1⟨k⟩IS+ω21−ω22=0,∂Z∂β=e−αtc3β(t)−λ3⟨k⟩HS+λ2⟨k⟩HS+ω31−ω32=0. | (4.18) |
Solve the optimal control expression from it:
{α∗1=1c1eαt[(λ1−λ2+λ3)⟨k⟩IS+ω12−ω11],α∗2=1c2eαt[λ2⟨k⟩IS+λ1⟨k⟩IS−ω21+ω22],β∗=1c3eαt[λ3⟨k⟩HS−λ2⟨k⟩HS+ω32−ω31]. | (4.19) |
In order to obtain the concrete expression of optimal control λi(t)(i=1,2,3,4) without penalty term ωij(i=1,2,3;j=1,2), the standard practice of reference [37] is adopted. Taking β∗1(t) as an example, the specific process is divided into three situations for implementation:
(1) In case 1, when {t|0<β∗(t)<1} is satisfied, let ω31(t)=ω32(t)=0. Therefore, the optimal control is β∗=1c3eαt[λ3⟨k⟩HS−λ2⟨k⟩HS].
(2) In case 2, when {t|β∗(t)=1} is satisfied, let ω31(t)=0. Therefore, 1=β∗=1c3eαt[λ3⟨k⟩HS−λ2⟨k⟩HS+ω32].
(3) In case 3, when {t|β∗(t)=0} is satisfied, let ω32(t)=0. Therefore, 0=beta∗=1c3eαt[λ3⟨k⟩HS−λ2⟨k⟩HS−ω31].
Combining the above three cases, the expression of optimal control β∗(t) can be obtained:
β∗(t)=min{1,max{0,1c3eαt[λ3⟨k⟩HS−λ2⟨k⟩HS+ω32−ω31]}}. |
Similar to the discussion, the corresponding expressions for optimal control α∗1(t),α∗2(t), and β∗(t) can be obtained:
{α∗1(t)=min{1,max{0,1c1eαt[(λ1−λ2+λ3)⟨k⟩IS+ω12−ω11]}},α∗2(t)=min{1,max{0,1c2eαt[λ2⟨k⟩IS+λ1⟨k⟩IS−ω21+ω22]}},β∗(t)=min{1,max{0,1c3eαt[λ3⟨k⟩HS−λ2⟨k⟩HS+ω32−ω31]}}. | (4.20) |
It is worth noting that the optimal control system comprises the state system (4.1) and its initial condition (4.3), as well as the costate system (4.14) and the optimal control form expression (4.15). Every optimal control must fulfill this complex optimal control system structure. The following is the optimal control system for future simulations:
{I′=B−(min{1,max{0,1c1eαt[(λ1−λ2+λ3)⟨k⟩IS+ω12−ω11]}}+min{1,max{0,1c2eαt[λ2⟨k⟩IS+λ1⟨k⟩IS−ω21+ω22]}})IS−μI,H′=min{1,max{0,1c2eαt[λ2⟨k⟩IS+λ1⟨k⟩IS−ω21+ω22]}}IS−min{1,max{0,1c3eαt[λ3⟨k⟩HS−λ2⟨k⟩HS+ω32−ω31]}}HS−(ε+μ)H,S′=min{1,max{0,1c1eαt[(λ1−λ2+λ3)⟨k⟩IS+ω12−ω11]}}IS+min{1,max{0,1c3eαt[λ3⟨k⟩HS−λ2⟨k⟩HS+ω32−ω31]}}HS−(δ+μ)S,R′=εH+δS−μR,λ′1=λ1[−(min(1,max(0,1c1eαt[(λ1−λ2+λ3)⟨k⟩IS+ω12−ω11]))+min(1,max(0,1c2eαt[λ2⟨k⟩IS+λ1⟨k⟩IS−ω21+ω22]))]⟨k⟩S−μ)+λ2min(1,max(0,1c2eαt[λ2⟨k⟩IS+λ1⟨k⟩IS−ω21+ω22]))⟨k⟩S+λ3min(1,max(0,1c1eαt[(λ1−λ2+λ3)⟨k⟩IS+ω12−ω11]))⟨k⟩S,λ′2=−e−αt+λ1(−min(1,max(0,1c3eαt[λ3⟨k⟩HS−λ2⟨k⟩HS+ω32−ω31]))⟨k⟩S)+λ2min(1,max(0,1c3eαt[λ3⟨k⟩HS−λ2⟨k⟩HS+ω32−ω31]))⟨k⟩S+λ3min(1,max(0,1c3eαt[λ3⟨k⟩HS−λ2⟨k⟩HS+ω32−ω31]))⟨k⟩S+λ4ε,λ′3=−e−αt+λ1(−(min(1,max(0,1c1eαt[(λ1−λ2+λ3)⟨k⟩IS+ω12−ω11]))+min(1,max(0,1c2eαt[λ2⟨k⟩IS+λ1⟨k⟩IS−ω21+ω22])))⟨k⟩I−min(1,max(0,1c3eαt[λ3⟨k⟩HS−λ2⟨k⟩HS+ω32−ω31]))⟨k⟩H+λ3(min(1,max(0,1c1eαt[(λ1−λ2+λ3)⟨k⟩IS+ω12−ω11]))⟨k⟩I+min(1,max(0,1c3eαt[λ3⟨k⟩HS−λ2⟨k⟩HS+ω32−ω31]))⟨k⟩H−(δ(t)+μ))+λ4δ(t),λ′4=−λ4μ. | (4.21) |
and
I(0)=I0,H(0)=H0,S(0)=S0,R(0)=R0,λ1(tf)=λ2(tf)=λ3(tf)=λ4(tf). | (4.22) |
In this section, the Rung-Kutta algorithm is adopted for performing numerical simulations to verify the rationality of the theoretical results by MATLAB R2017b. It is noteworthy that the value range of parameters is not clearly defined in previous studies. Therefore, in this work, we combine the value of the basic reproduction number R0 and the stability condition for obtaining and presenting the parameter values of the model.
In Figure 2(a), let B=1,β=0.5,α1=0.35,α2=0.5,μ=0.6, ε=0.4,δ=0.38,<k>=1.367. After calculating R0=0.8137<1, the equilibrium point E0 without information propagation is stable under different initial conditions. From Figure 2(a), it can be seen that the stability of E∗ remains unchanged under different initial value conditions.
In Figure 2(b), assuming B=1, β=0.1, α1=0.3, α2=0.5, μ=0.4, ε=0.22, δ=0.2, <k>=1.367, after calculating R0=1.7088>1, the equilibrium point E∗ for information propagation is stable under different initial conditions. From Figure 2(b), it can be seen that the stability of E∗ remains unchanged under different initial value conditions. In Figure 3, with parameter settings B=1, β=0.3, α2=0.5, μ=0.6, ε=0.4, and δ=0.38, the effects of parameter α1 on the density of different populations (I(t), H(t), S(t), R(t)) are analyzed.
In Figure 3(a), as the parameter α1 increases and the scale of information disseminators expands significantly, reaching a critical point around α1=0.4. At this stage, the density of information disseminators stabilizes between 0.2 and 0.3, while the density of information beneficiaries reaches its peak between 0.15 and 0.2 before showing a gradual decline. This phenomenon highlights that the expansion of communicators drives the reach of information to a peak audience at a specific point. However, the subsequent decline implies that there is a saturation or over-diffusion effect, where the system becomes less effective in disseminating information beyond this threshold. This finding underscores the importance of optimizing α1 to achieve efficient dissemination while avoiding information overload.
In Figure 3(b), with the continued increase of α1, the scale of information disseminators remains within the range 0.2<β<0.4. When α1 reaches its maximum, the density of information disseminators stabilizes between 0.3 and 0.4, while the density of information beneficiaries peaks around α1=0.4 at values between 0.1 and 0.15 before gradually declining. These results suggest that the initial expansion of information dissemination mechanisms increases audience engagement. However, beyond the optimal point, a diminishing return effect emerges, where engagement progressively declines. This indicates the necessity of carefully managing dissemination parameters to maintain high levels of audience interaction and system effectiveness.
In Figure 3(c), the density of information disseminators increases rapidly starting from α1=0.1. Even when α1 reaches its maximum, the density stabilizes between 0.4 and 0.5. However, the density of information beneficiaries peaks within the range 0.1<β<0.2 at values between 0.15 and 0.2, after which it begins to decrease. This asymmetry between the growth of disseminators and beneficiaries suggests that an increase in the number of disseminators does not always result in a proportional increase in beneficiaries. Possible reasons include repetitive information or a lack of content quality, which limits its appeal and impact on the audience. These insights highlight the importance of improving content relevance and diversity to enhance the system's effectiveness.
In Figure 3(d), the density of information disseminators shows a significant increase initially and reaches 0.5 when α1 is at its maximum. However, the density of information beneficiaries, while exceeding 0.2 at the beginning, decreases over time. These observations emphasize the role of network structure in information diffusion. When the average degree of the network is larger, both the number of information disseminators and the scale of information beneficiaries are larger. To maximize the effectiveness of the information dissemination system, it is necessary to optimize α1 by increasing the number of disseminators while ensuring the quality and relevance of the disseminated content. This will help convert a larger proportion of the audience into beneficiaries, enhancing the overall impact of the information system.
In Figure 4(a), let B=1,β=0.3,α1=0.35,μ=0.6,ε=0.4,δ=0.38. In Figure 4(a), with the increase of α2, the number of information disseminators decreases slowly and the number of information beneficiaries increases slowly. When α2 increases to the maximum, the density of information disseminators and information beneficiaries is less than 0.1. This suggests that, in some cases, when information diffusion reaches a certain level, the level of benefit to the audience decreases. This can be explained by the fact that when information is spread too widely, it may be due to an overload effect or information saturation, resulting in recipients no longer absorbing new information effectively. Therefore, in information dissemination strategies, consideration should be given to avoiding information overload and ensuring timely dissemination to maintain audience attention and engagement.
In Figure 4(b), with the increase of α2, the density of information disseminators gradually decreases from about 0.15 to 0.1, the scale of information beneficiaries gradually increases, and the final density is between (0.1,0.2). This image reflects the fact that in information dissemination on a network or social platform, the interaction between the communicator and the audience may be limited by a key parameter. When the scale of information dissemination reaches a certain level, the density of beneficiaries will start to decrease. This may be due to the over-concentration of information in the hands of specific communicators, leading to a reduction in the diversity of information, which in turn affects the interest or engagement of the audience.
In Figure 4(c), with the increase of α2, the initial density of information disseminators is between (0.3,0.4) and then gradually decreases, and the final density is between (0.2,0.3). The size of information beneficiaries gradually increases with the increase of α2, and the final density reaches more than 0.2. This image may suggest the role of "opinion leaders" in a network. Even if the size of the information disseminators is large, the extent to which the beneficiaries can benefit may be limited to a certain threshold. This implies that, in practice, the depth and breadth of communication are not always linear and that too many communicators may lead to a flood of information, which in turn reduces audience acceptance.
In Figure 4(d), with the increase of α2, the initial density of information disseminators decreased from (0.4,0.5), and the size of information beneficiaries increased with the increase of α2. This suggests that in some complex networks, as the number of disseminators increases, the density of the audience also increases until a steady state is reached. This may reflect the phenomenon of "self-regulation" in networks, whereby the flow of information and audience acceptance in a network tend to equalize after a certain number of disseminators has been reached.
In summary, with the gradual increase of the average network degree, the scale of information beneficiaries gradually increases. When ⟨k⟩=4.06, the scale of information beneficiaries reaches a satisfactory effect. If the ⟨k⟩ value gradually increases again, the scale of information beneficiaries is not ideal, or even will gradually decrease. Therefore, while we choose to increase the contact rate of ignorant people and information disseminators, also note the value of ⟨k⟩.
In Figure 5, with parameter settings B=1, α1=0.35, α2=0.5, μ=0.6, ε=0.4, and δ=0.38, the effect of parameter β on the density of information disseminators and beneficiaries is analyzed. In Figure 5(a), as β increases, the number of information disseminators gradually grows, but the change is not significant. Additionally, the number of information beneficiaries remains relatively stable. This indicates that, in certain online communication environments, simply increasing the number of communicators does not lead to a noticeable increase in the audience size. Such scenarios may occur in saturated communication environments or when the audience exhibits low interest in the message being disseminated. As a result, the increase in communicators fails to significantly affect the number of beneficiaries. These findings suggest that increasing the number of communicators alone does not enhance the effectiveness of communication. Instead, other strategies, such as improving the quality of the message or employing targeted communication strategies, are required to achieve better outcomes.
In Figure 5(b), as β continues to increase, the number of information disseminators grows significantly, with the final density stabilizing between 0.2 and 0.25. Concurrently, the number of information beneficiaries first increases and then decreases. When β=0.2, the scale of information beneficiaries reaches its peak, after which it gradually declines. This observation reflects a common pattern in information dissemination: An initial expansion of disseminators leads to a short-term increase in audience size, but over time, the audience begins to decline. This decline may result from over-dissemination or repetitive dissemination of information, which can lead to audience disengagement or information fatigue. These findings underscore the need to maintain diversity and relevance in the information being shared, ensuring that audience attention is not lost due to overexposure or redundancy. In Figure 5(c), with a further increase in β, the scale of information disseminators grows rapidly, with the final density exceeding 0.4. However, the density of information beneficiaries declines slightly at first and ultimately stabilizes between 0.05 and 0.1. This change suggests that a significant increase in the number of disseminators does not necessarily translate into greater audience engagement or interest. Possible explanations include the lack of improvement in information quality or a mismatch between the delivery method and audience needs. This highlights the critical role of content quality and delivery methods in the success of information dissemination, beyond the sheer number of disseminators. In Figure 5(d), the scale of information disseminators increases at a faster rate compared to the previous three cases, while the density of information beneficiaries decreases from an initial range of 0.15 to 0.2 to around 0.15. This trend indicates a gradual decline in audience participation despite the substantial growth in disseminators. The findings suggest that excessive numbers of disseminators may create information redundancy, which can negatively impact audience engagement. Therefore, optimizing the dissemination pathways, improving content relevance, and reducing redundant information are essential for enhancing the overall effectiveness of information dissemination. To sum up, the results demonstrate that as ⟨k⟩ increases, a growing number of information beneficiaries recognize the value of the information and develop a strong sense of social responsibility. Consequently, they are more willing to become information disseminators and share the information with surrounding groups, thereby enabling more individuals to become beneficiaries of the information.
In Figure 6, the effects of optimal control, middle control, and constant control on α1, α2, and β are illustrated, showing their impact on the densities of susceptible individuals S(t) and information beneficiaries H(t). The optimal control strategy consistently yields the best outcomes. Specifically, under optimal control, the number of susceptible individuals S(t) increases and stabilizes at a higher level, while the number of information beneficiaries H(t) is maximized. This indicates that effective control strategies can significantly enhance the number of individuals benefiting from the information, thereby reducing misinformation and maintaining system stability.
In Figure 7, the optimal control strategies applied to α1 and β, as well as α2 and β, demonstrate superior performance. Specifically, under optimal control, the density of S(t) rapidly stabilizes at higher levels, significantly enhancing the recovery of susceptible individuals and improving the overall system stability. Additionally, H(t) exhibits faster and more effective stabilization under optimal control compared to constant and intermediate control measures. These results highlight the optimal control strategy's effectiveness in achieving quicker and more robust system stabilization, thereby outperforming alternative control approaches.
In Figure 8, it illustrates the effects of optimal control, intermediate control, and constant control on α1, α2, and β, with these three parameters jointly regulating the densities of susceptible individuals S(t) and information beneficiaries H(t). The optimal control strategy consistently yields the best results. Specifically, under optimal control, the number of susceptible individuals S(t) significantly increases and stabilizes at a higher level, while the number of information beneficiaries H(t) reaches its maximum value. This indicates that effective control strategies not only significantly increase the number of individuals benefiting from information, but also help maintain the stability of the system.
Considering the parameters α1 and δ, respectively, from the basic reproduction number R0, the following can be obtained:
∂R0∂α1=⟨k⟩Bμ(δ+μ)>0, | (6.1) |
∂R0∂δ=−α1⟨k⟩Bμ(δ+μ)2>0. | (6.2) |
It can be seen from the above formula that the basic reproduction number R0 decreases with the increase of δ, that is, the scale of information dissemination and information beneficiaries decreases. Therefore, if we want to expand the scope of information to maximize the value of information, we should take measures to reduce the value range of δ.
In this part, we focus on the influence of two parameters on R0. Figure 9 shows the influence of a and B on the basic reproduction number R0 when the average degree ⟨k⟩ is different in the information transmission network. In Figure 9, let B=1,μ=0.2. As can be seen from Figure 9, the more the number of communicators in the network nodes, the harder it is for information to spread among the masses. This means that when the connection between nodes in the network is relatively small, the spread of information is more influenced by the information disseminator. On the contrary, the greater the average degree of the network, the easier it is for information to spread through the masses.
On the basis of the classical SIR model, this article proposes an improved IHSR information propagation model that takes into account the state of information beneficiaries, and studies the influence of the average degree of the network, an important network topology parameter, on the dynamic evolution of information propagation. Through a research method combining theoretical analysis and numerical simulation, the propagation law of information in social networks was deeply explored, and the following conclusions were mainly drawn:
● It has been proven that the model has two equilibrium solutions: Information extinction and information constant. The stability theory is used to analyze their local and global stability, revealing the dynamic evolution mechanism from nonequilibrium state to equilibrium state.
● It has been determined that the average network degree and information dissemination coefficient are the two key parameters controlling the dynamic behavior of information dissemination. They play an important role in the endpoint state, scale, and duration of information dissemination by influencing the form of the infection rate function.
● The numerical simulation results show that when the network is highly clustered and information has strong dissemination, the system can generate explosive information dissemination, that is, a sudden state of rapid increase in information scale and dissemination speed. At this time, information will bring benefits to more groups, making them beneficiaries of information.
● The sensitivity analysis method was used to evaluate the impact of different key parameters on system dynamic stability, providing quantitative reference for parameter control and optimization selection.
● After analyzing the equilibrium point and stability of the model and verifying the existence of optimal control, an optimal control strategy was proposed, and its effectiveness was validated through numerical simulation. Ultimately, the sensitivity of the optimal control parameters was analyzed.
This study provides a new theoretical perspective and analytical method for constructing a more accurate and reasonable information dissemination model and conducting information dissemination prediction and control. Subsequent work will continue to expand on this basis by incorporating more general and complex network frameworks considering individual heterogeneity, as well as various information interaction mechanisms in order to establish a more reasonable and comprehensive information dissemination dynamics model.
In future research, we will integrate Hattaf's newly proposed mixed fractional derivative model to provide a novel perspective on modeling information dissemination, particularly by considering the influence of information beneficiaries[38]. By incorporating the concept of social memory, we aim to explore the impact of memory effects on the dynamics of the model. Social memory, as a reflection of the collective understanding and shared narratives of a group regarding past experiences, will add a significant dimension to the dynamic processes of information dissemination. We plan to employ generalized fractional derivatives and fractal-fractional derivatives as alternatives to traditional classical time derivatives, allowing for a more flexible and inclusive approach to capturing memory effects within complex dynamic systems. This methodology will facilitate the establishment of a more comprehensive and realistic model of information dissemination dynamics, broadening our research perspective and providing robust theoretical support for optimizing the information dissemination process.
Xuechao Zhang: Writing-original draft, writing-review and editing; Yuhan Hu: Supervision; Shichang Lu: Supervision, writing-review and editing; Haomiao Guo: Investigation; Xiaoyan Wei: Investigation; Jun li: Software. All authors have read and approved the final version of the manuscript for publication.
The authors declare they have not used Artificial Intelligence (AI) tools in the creation of this article.
We thank the anonymous editors and reviewers warm work earnestly. This study was funded by the Education Department of Liaoning Province (JYTMS20230940).
The authors declare no conflict of interest.
[1] |
M. Castells, Toward a sociology of the network society, Contemp. Sociol., 29 (2000), 693–699. https://doi.org/10.2307/2655234 doi: 10.2307/2655234
![]() |
[2] |
J. H. Kietzmann, K. Hermkens, I. P. Mccarthy, B. S. Silvestre, Social media? Get serious! Understanding the functional building blocks of social media, Bus. Horizons, 54 (2011), 241–251. http://dx.doi.org/10.1016/j.bushor.2011.01.005 doi: 10.1016/j.bushor.2011.01.005
![]() |
[3] |
S. Funk, E. Gilad, C. Watkins, V. A. Jansen, The spread of awareness and its impact on epidemic outbreaks, P. Natl. Acad. Sci., 106 (2009), 6872–6877. http://dx.doi.org/10.1073/pnas.0810762106 doi: 10.1073/pnas.0810762106
![]() |
[4] |
S. Lewandowsky, U. K. H. Ecker, J. Cook, Beyond misinformation: Understanding and coping with the "post-truth" era, J. Appl. Res. Mem. Cogn., 6 (2017), 353–369. http://dx.doi.org/10.1016/j.jarmac.2017.07.008 doi: 10.1016/j.jarmac.2017.07.008
![]() |
[5] | A. E. Marwick, R. Lewis, Media manipulation and disinformation online, J. Amer. Math. Soc., 2017. |
[6] |
T. Preis, H. S. Moat, H. E. Stanley, Quantifying trading behavior in financial markets using google trends, Sci. Rep., 3 (2013), 1684–1684. http://dx.doi.org/10.1090/S0894-0347-1992-1124979-1 doi: 10.1090/S0894-0347-1992-1124979-1
![]() |
[7] |
H. S. Moat, C. Curme, A. Avakian, D. Y. Kenett, H. E. Stanley, T. Preis, Quantifying wikipedia usage patterns before stock market moves, Sci. Rep., 3 (2013), 2045–2322. http://dx.doi.org/10.1038/srep01801 doi: 10.1038/srep01801
![]() |
[8] |
Q. L. Yan, S. Y. Tang, S. Gabriele, J. H. Wu, Media coverage and hospital notifications: Correlation analysis and optimal media impact duration to manage a pandemic, J. Theor. Biol., 390 (2016), 1–13. http://dx.doi.org/10.1090/S0894-0347-1992-1124979-1 doi: 10.1090/S0894-0347-1992-1124979-1
![]() |
[9] |
R. Ross, H. P. Hudson, An application of the theory of probabilities to the study of a priori pathometry, Part Ⅲ, Proc. R. Soc. Lond. A, 89 (1917), 225–240. http://dx.doi.org/10.1098/rspa.1917.0015 doi: 10.1098/rspa.1917.0015
![]() |
[10] |
D. J. Daley, D. G. Kendall, Epidemics and rumours, Nature, 204 (1964), 1118–1118. https://doi.org/10.1038/2041118a0 doi: 10.1038/2041118a0
![]() |
[11] |
D. J. Daley, D. G. Kendall, Stochastic rumours, Ima J. Appl. Math., 1 (1965), 42–55. https://doi.org/10.1093/imamat/1.1.42 doi: 10.1093/imamat/1.1.42
![]() |
[12] | R. Schweizer, Mathematical models and applications: With emphasis on the social, life, and management sciences, 2016. |
[13] |
S. J. Gao, Z. D. Teng, J. J. Nieto, T. Angela, Analysis of an SIR epidemic model with pulse vaccination and distributed time delay, J. Biomed. Biotechnol., 2007 (2007), 1–10. http://dx.doi.org/10.1155/2007/64870 doi: 10.1155/2007/64870
![]() |
[14] |
Y. Liu, S. M. Diao, Y. X. Zhu, Q. Liu, SHIR competitive information diffusion model for online social media, Physica A, 461 (2016), 543–553. http://dx.doi.org/10.1016/j.physa.2016.06.080 doi: 10.1016/j.physa.2016.06.080
![]() |
[15] |
Q. Liu, D. Q. Jiang, T. Hayat, B. Ahmad, Anosov flows with stable and unstable differentiable distributions, Nonlinear Anal.- Hybri., 27 (2018), 29–43. http://dx.doi.org/10.1016/j.nahs.2017.08.002 doi: 10.1016/j.nahs.2017.08.002
![]() |
[16] |
Q. Liu, Q. M. Chen, D. Q. Jiang, The threshold of a stochastic delayed SIR epidemic model with temporary immunity, Physica A, 450 (2016), 115–125. http://dx.doi.org/10.1016/j.physa.2015.12.056 doi: 10.1016/j.physa.2015.12.056
![]() |
[17] |
C. Wan, T. Li, Z. H. Guan, Y. M. Wang, X. D. Liu, Spreading dynamics of an e-commerce preferential information model on scale-free networks, Physica A, 467 (2017), 192–200. http://dx.doi.org/10.1016/j.physa.2016.09.035 doi: 10.1016/j.physa.2016.09.035
![]() |
[18] |
Y. M. Zhang, Y. Y. Su, W. G. Li, H. O. Liu, Rumor and authoritative information propagation model considering super spreading in complex social networks, Physica A, 506 (2018), 359–411. http://dx.doi.org/10.1016/j.physa.2018.04.082 doi: 10.1016/j.physa.2018.04.082
![]() |
[19] |
S. Hosseini, M. A. Abdollahi, The dynamics of an SEIRS-QV malware propagation model in heterogeneous networks, Physica A, 512 (2018), 803–817. http://dx.doi.org/10.1016/j.physa.2018.08.081 doi: 10.1016/j.physa.2018.08.081
![]() |
[20] | F. Wu, B. A. Huberman, Social structure and opinion formation, Comput. Econ., 2004. https://doi.org/10.48550/arXiv.cond-mat/0407252 |
[21] |
M. Small, C. K. Tse, D. M. Walker, Super-spreaders and the rate of transmission of the SARS virus, Physica D, 215 (2006), 146–158. http://dx.doi.org/10.1016/j.physd.2006.01.021 doi: 10.1016/j.physd.2006.01.021
![]() |
[22] |
L. L. Weng, A. Flammini, A. Vespignani, F. Menczer, Competition among memes in a world with limited attention, Sci. Rep., 2 (2012), 335–335. http://dx.doi.org/10.1038/srep00335 doi: 10.1038/srep00335
![]() |
[23] |
A. Guille, H. Hacid, C. Favre, D. A. Zighed, Information diffusion in online social networks: A Survey, SIGMOD Rec., 42 (2013), 17–28. http://dx.doi.org/10.1145/2503792.2503797 doi: 10.1145/2503792.2503797
![]() |
[24] |
J. B. Holthoefer, S. Meloni, B. Gonçalves, Y. Moreno, Emergence of influential spreaders in modified rumor models, J. Stat. Phys., 151 (2013), 383–393. http://dx.doi.org/10.1007/s10955-012-0595-6 doi: 10.1007/s10955-012-0595-6
![]() |
[25] | K. Lerman, R. Ghosh, Information contagion: An empirical study of the spread of news on digg and twitter social networks, In: Proceedings of the International AAAI Conference on Web and Social Media, 52 (2010), 166–176. https://doi.org/10.1609/icwsm.v4i1.14021 |
[26] |
N. Carbonara, Information and communication technology and geographical clusters: Opportunities and spread, Technovation, 25 (2005), 213–222. http://dx.doi.org/10.1016/s0166-4972(03)00095-6 doi: 10.1016/s0166-4972(03)00095-6
![]() |
[27] |
M. Salehi, R. Sharma, M. Marzolla, M. Magnani, P. Siyari, D. Montesi, Spreading processes in multilayer networks, IEEE T. Netw. Sci. Eng., 2 (2015), 65–83. http://dx.doi.org/10.1109/TNSE.2015.2425961 doi: 10.1109/TNSE.2015.2425961
![]() |
[28] |
L. P. Fu, B. Hellinga, Real-time, adaptive prediction of incident delay for advanced traffic management and information systems, J. Am. Math. Soc., 2002, 12–15. http://dx.doi.org/10.1090/S0894-0347-1992-1124979-1 doi: 10.1090/S0894-0347-1992-1124979-1
![]() |
[29] | S. Y. Sun, H. Y. Liu, J. He, X. Y. Du, Web technologies and applications: Detecting event rumors on sina weibo automatically, Berlin, Heidelberg: Springer, 7808 (2013), 120–131. https://doi.org/10.1007/978-3-642-37401-2-14 |
[30] |
S. Kang, X. L. Hou, Y. H. Hu, H. Y. Liu, Dynamical analysis and optimal control of the developed information transmission model, PLOS One, 17 (2022). https://doi.org/10.1371/journal.pone.0268326 doi: 10.1371/journal.pone.0268326
![]() |
[31] |
S. F. Marinósson, Lyapunov function construction for ordinary differential equations with linear programming, Dynam. Syst., 17 (2002), 137–150. https://doi.org/10.1080/0268111011011847 doi: 10.1080/0268111011011847
![]() |
[32] | J. P. Lasalle, The stability of dynamical systems, SIAM, 1976. |
[33] |
Y. Zhang, Z. Chen, SETQR propagation model for social networks, IEEE Access, 7 (2019), 127533–127543. https://doi.org/10.1109/ACCESS.2019.2939150 doi: 10.1109/ACCESS.2019.2939150
![]() |
[34] |
A. Foligno, P. Kos, B. Bertini, Quantum information spreading in generalized dual-unitary circuits, Phys. Rev. Lett., 132 (2024), 250402. https://10.1103/physrevlett.132.250402 doi: 10.1103/physrevlett.132.250402
![]() |
[35] |
W. Q. Pan, W. J. Yan, Y. H. Hu, R. M. He, L. B. Wu, G. Rasool, Dynamic analysis and optimal control of rumor propagation model with reporting effect, Adv. Math. Phys., 2022 (2022), 1–14. https://doi.org/10.1155/2022/5503137 doi: 10.1155/2022/5503137
![]() |
[36] | M. Hale, Y. Wardi, H. Jaleel, M. Egerstedt, Hamiltonian-based algo rithm for optimal control, arXiv Preprint, 2016. https://doi.org/10.48550/arXiv.1603.02747 |
[37] | L. Bittner, L. S. Pontryagin, V. G. Boltyanskii, R. V. Gamkrelidze, E. F. Mishchenko, The mathematical theory of optimal processes, New York: John Wiley & Sons, 43 (1962), 514–515. https://doi.org/10.1002/zamm.19630431023 |
[38] |
K. Hattaf, A new mixed fractional derivative with applications in computational biology, Computation, 1 (2024), 7. https://doi.org/10.3390/computation12010007 doi: 10.3390/computation12010007
![]() |
Parameter | Description |
I(t) | The number of individuals actively spreading the information at time t. |
H(t) | The number of individuals benefiting from the information at time t. |
S(t) | The number of individuals unaware of the information at time t. |
R(t) | The number of individuals who forget the information or are unaffected at time t. |
B | The number of immigrants in the social system. |
α1 | Progression rate from state I to S. |
α2 | Progression rate from state I to H. |
β | Progression rate from state S to H. |
ε | Progression rate from state H to R. |
δ | Progression rate from state S to R. |
μ | Removal rate per unit time. |
Parameter | Description |
I(t) | The number of individuals actively spreading the information at time t. |
H(t) | The number of individuals benefiting from the information at time t. |
S(t) | The number of individuals unaware of the information at time t. |
R(t) | The number of individuals who forget the information or are unaffected at time t. |
B | The number of immigrants in the social system. |
α1 | Progression rate from state I to S. |
α2 | Progression rate from state I to H. |
β | Progression rate from state S to H. |
ε | Progression rate from state H to R. |
δ | Progression rate from state S to R. |
μ | Removal rate per unit time. |