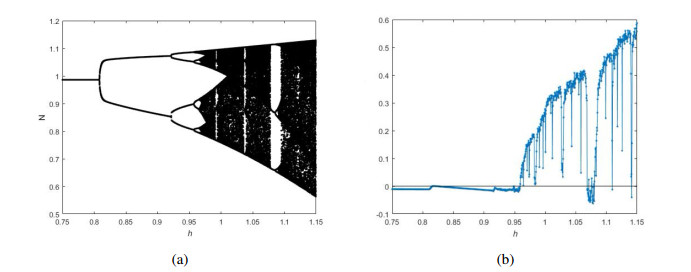
This paper investigates the complex dynamical behavior of a discrete prey-predator system with a fear factor, a strong Allee effect, and prey refuge. The existence and stability of fixed points in the system are discussed. By applying the central manifold theorem and bifurcation theory, we have established the occurrence of various types of bifurcations, including flip bifurcation and Neimark-Sacker bifurcation. Furthermore, to address the observed chaotic behavior in the system, three controllers were designed by employing state feedback control, OGY feedback control, and hybrid control methods. These controllers serve to control chaos in the proposed system and identify specific conditions under which chaos or bifurcations can be stabilized. Finally, the theoretical analyses have been validated through numerical simulations.
Citation: Xiaoming Su, Jiahui Wang, Adiya Bao. Stability analysis and chaos control in a discrete predator-prey system with Allee effect, fear effect, and refuge[J]. AIMS Mathematics, 2024, 9(5): 13462-13491. doi: 10.3934/math.2024656
[1] | Xiongxiong Du, Xiaoling Han, Ceyu Lei . Dynamics of a nonlinear discrete predator-prey system with fear effect. AIMS Mathematics, 2023, 8(10): 23953-23973. doi: 10.3934/math.20231221 |
[2] | Jie Liu, Qinglong Wang, Xuyang Cao, Ting Yu . Bifurcation and optimal harvesting analysis of a discrete-time predator–prey model with fear and prey refuge effects. AIMS Mathematics, 2024, 9(10): 26283-26306. doi: 10.3934/math.20241281 |
[3] | Ali Al Khabyah, Rizwan Ahmed, Muhammad Saeed Akram, Shehraz Akhtar . Stability, bifurcation, and chaos control in a discrete predator-prey model with strong Allee effect. AIMS Mathematics, 2023, 8(4): 8060-8081. doi: 10.3934/math.2023408 |
[4] | Figen Kangalgil, Seval Isșık . Effect of immigration in a predator-prey system: Stability, bifurcation and chaos. AIMS Mathematics, 2022, 7(8): 14354-14375. doi: 10.3934/math.2022791 |
[5] | A. Q. Khan, Ibraheem M. Alsulami . Complicate dynamical analysis of a discrete predator-prey model with a prey refuge. AIMS Mathematics, 2023, 8(7): 15035-15057. doi: 10.3934/math.2023768 |
[6] | Binfeng Xie, Na Zhang . Influence of fear effect on a Holling type III prey-predator system with the prey refuge. AIMS Mathematics, 2022, 7(2): 1811-1830. doi: 10.3934/math.2022104 |
[7] | A. Q. Khan, Ibraheem M. Alsulami, S. K. A. Hamdani . Controlling the chaos and bifurcations of a discrete prey-predator model. AIMS Mathematics, 2024, 9(1): 1783-1818. doi: 10.3934/math.2024087 |
[8] | Fatao Wang, Ruizhi Yang, Yining Xie, Jing Zhao . Hopf bifurcation in a delayed reaction diffusion predator-prey model with weak Allee effect on prey and fear effect on predator. AIMS Mathematics, 2023, 8(8): 17719-17743. doi: 10.3934/math.2023905 |
[9] | Wei Li, Qingkai Xu, Xingjian Wang, Chunrui Zhang . Dynamics analysis of spatiotemporal discrete predator-prey model based on coupled map lattices. AIMS Mathematics, 2025, 10(1): 1248-1299. doi: 10.3934/math.2025059 |
[10] | Weili Kong, Yuanfu Shao . The effects of fear and delay on a predator-prey model with Crowley-Martin functional response and stage structure for predator. AIMS Mathematics, 2023, 8(12): 29260-29289. doi: 10.3934/math.20231498 |
This paper investigates the complex dynamical behavior of a discrete prey-predator system with a fear factor, a strong Allee effect, and prey refuge. The existence and stability of fixed points in the system are discussed. By applying the central manifold theorem and bifurcation theory, we have established the occurrence of various types of bifurcations, including flip bifurcation and Neimark-Sacker bifurcation. Furthermore, to address the observed chaotic behavior in the system, three controllers were designed by employing state feedback control, OGY feedback control, and hybrid control methods. These controllers serve to control chaos in the proposed system and identify specific conditions under which chaos or bifurcations can be stabilized. Finally, the theoretical analyses have been validated through numerical simulations.
In the natural world, the mutual relationship between predators and prey represents one of the most fundamental and critically important types of ecological interactions within population dynamics. Predators exert direct influence on prey population sizes and simultaneously affect their own population dynamics, resulting in complex interplays between them. A fundamental continuous population model was devised in 1926 by American biologist Lotka and Italian mathematician Volterra to explain the relationship between predators and prey [1]. Subsequent to this foundational work, numerous experts conducted in-depth investigations into the predator-prey model [2,3,4]. Nevertheless, due to the model's limited ability to address diverse real-world scenarios and complexities, scholars have developed multifaceted modifications to provide a more realistic interpretation and enhance the understanding of ecosystems. To present a more precise depiction of ecological interactions, several ecological concepts have been incorporated into the predator-prey system. These include functional responses, shelters, harvesting, fear, and the Allee effect [5,6,7].
In the natural world, numerous species of animals, plants, and insects frequently exhibit reduced individual fitness at lower population densities. The ecologist W.C. Allee succinctly summarized this phenomenon as the Allee effect [8]. The Allee effect can be attributed to various environmental factors, including difficulties in mating at low densities, genetic inbreeding, reduced anti-predator defense capabilities, depression, and social hindrances at low densities, among others [9,10,11]. The Allee effect is dichotomized into two distinct categories based on the magnitude of density dependence at low population densities: The strong Allee effect and the weak Allee effect. In the case of the strong Allee effect, the population growth rate becomes negative when the population falls below the Allee effect threshold. Conversely, a weak Allee effect is characterized by a per capita population growth rate at low density that is lower than that at high density, though it remains positive. For endangered species, Allee effects are more likely to occur, underscoring the significance of Allee effects in the management of endangered species conservation, population development, utilization, and species introduction. Recent years have witnessed attention being increasingly dedicated to comprehending the impact of the Allee effect on biological populations [12,13,14], revealing its significant influence on the dynamics of ecological systems.
In the investigation of predator-prey systems, the predominant focus in most studies has revolved around the direct predation exerted by predators on prey populations, given the ease of directly observing such behavior within ecosystems. In recent years, scholars have gradually become aware that prey, upon sensing the presence of predators, exhibit fear responses. This fear can have diverse impacts on various aspects of prey populations, including changes in habitat use, foraging behavior, and vigilance, as well as different psychological changes [15,16,17]. On certain occasions, the effects of this fear may be even more significant than those of direct predation [18,19,20]. To date, numerous scholars have conducted research on predator-prey models that incorporate the effects of fear [21,22,23].
The efficiency of predators in prey capture is intricately tied to the strategies employed by the prey to evade predation. In situations of danger, prey organisms typically seek refuge in areas with lower predation risks, ecologically referred to as prey refuges [24,25,26]. The successful evasion of predation is achieved by certain prey populations through the exploration of refuges, thereby effectively mitigating the pressure of being captured by predators. In 1946, Crombic introduced the concept of refuge into the prey-predator model through experimental investigations [27]. Both theoretical analysis and experimental studies suggest that this type of system is more realistic and holds greater research value [28,29,30].
When examining populations characterized by non-overlapping generations or low numbers, mathematical expressions often present as discrete models. Despite certain variables exhibiting continuous changes in practical applications, the recording of quantity changes often occurs within specific time intervals during data processing. Therefore, utilizing discrete systems to analyze the dynamic behavior of biological populations has immense practical significance. For example, Hadeler and Gerstmann [31] showed that for the Rosenzweig-MacArthur model, the discrete-time version of the model has similar equilibrium points as the continuous-time version, but the discrete-time version of the model exhibits more complex phenomena such as period doubling and chaos, which are phenomena that are not present in the continuous-time model. In turn, we can conclude that discrete systems are better suited to describe the richer patterns and chaotic behavior of nonlinear dynamics. Numerous mathematical methods exist for the discretization of continuous systems, including the forward Euler method, the use of piecewise constant arguments, and the exponential Euler difference scheme [32,33,34,35,36]. Among these, the forward Euler method is widely employed due to its ease of comprehension and computational simplicity. Inspired by the aforementioned literature, we chose to adopt the forward Euler method to discretize continuous systems.
Our focus on understanding system stability and controlling chaos has been driven by the desire to clarify the intricate dynamics between predators and prey in ecosystems, as well as to probe the complexity and stability of community dynamics. Stability analysis offers insights into system resilience under varying environmental conditions, shedding light on how environmental factors influence community structure and evolution. Conversely, the emergence of chaotic behavior can disrupt system predictability and stability, posing risks to ecosystem equilibrium and biodiversity. By investigating stability and chaos control in predator-prey models, we contribute to the broader understanding of ecosystem dynamics, offering insights and methodologies that are crucial for sustainable ecosystem management and conservation.
In 2014, Rana et al. conducted a study on a discrete model of predator-prey systems that incorporate an Allee effect and prey refuge. The findings revealed that moderate levels of prey refuge can stabilize the dynamics of the system through the Allee effect. Additionally, it was observed that the population remained stable at moderate refuge parameter levels, while chaotic oscillations of prey occurred at relatively low and high refuge levels [37]. In 2020, Ma et al. investigated a discrete model of predator-prey dynamics involving fear and refuge. The study identified flip branching at immobile points where only the predator is present, as well as Neimark-Sacker branching at positive immobile points where both prey and predator coexist [38]. In 2023, Hong and Zhang explored a discrete predator-prey system that incorporates an Allee effect and a fear factor. The investigation demonstrated that the system undergoes flip bifurcation and Neimark-Sacker bifurcation at the sole positive immobile point [39]. To the best of our knowledge, there is a dearth of studies focusing on the stability, bifurcation, and chaos control analysis of discrete-time predator-prey models that incorporate a strong Allee effect, the fear effect, and prey refuge. This article serves to contribute to this area of study and address existing gaps in the literature. This study brings to light several significant contributions, notably, those summarize below:
● Utilizing the forward Euler method, we have derived a discrete-time model that aptly represents interactions among non-overlapping populations across generations.
● Stability analysis of the discrete model was conducted from the perspective of the fixed points inherent in the system.
● Investigating internal fixed points, various types of bifurcations were explored by introducing h as the bifurcation parameter, leveraging the center manifold theorem and bifurcation theory. Notably, the proposed discrete system exhibited flip and Neimark-Sacker bifurcations.
● In addressing system-induced chaos, chaos control was implemented in the discrete model through state feedback control, OGY feedback control, and hybrid control methods, effectively managing the chaotic behavior of the system.
The rest of the paper is organized as follows. In Section 2, we formulate a discrete biodynamic system by using the forward Euler method. Subsequently, in Section 3, we analyze the existence and stability of fixed points within the discrete system. Section 4 focuses on demonstrating the possibility of flip bifurcation and Neimark-Sacker bifurcation in the discrete system, considering appropriate parameter values. In Section 5, chaos is effectively controlled through the application of state feedback control, OGY feedback control, and hybrid control methods. The practicality of the main theoretical results is validated through numerical simulations in Section 6. Finally, the paper concludes with a brief summary.
First, we describe the continuous-time prey-predator model with the Allee effect, a fear effect, and a prey refuge [40], as follows:
{u′(t)=ru(1−uk)(u−θ0)11+f0v−a(1−η)uv,v′(t)=aα(1−η)uv−m0v, | (2.1) |
where all coefficients are positive constants; u and v are the prey and predator population densities, respectively; r represents the intrinsic growth rate of the prey; a indicates the predator's rate of predation on prey; k is the maximum prey carrying capacity of the environment; α is the transformation efficiency of a predator as a result of preying on its prey; m0 is the natural mortality rate of predators; 0<θ0<k indicates a strong Allee effect; F(f0,v)=11+f0v is the fear function; 0<η<1 is the prey refuge constant.
For simplicity, we initially rescale system (2.1) by introducing the following parameters: N=uk,P=avrk,τ=aαkt,ε=aαr,θ=θ0k,f=rkf0a,m=m0aαk. Rewriting τ as t, the simplified system (2.1) becomes as follows:
{N′(t)=Nε((1−N)(N−θ)fP+1−(1−η)P),P′(t)=P(N(1−η)−m), | (2.2) |
where 0<θ<1 and 0<m<1.
Implementing the forward Euler scheme to system (2.2) yields the discrete model under scrutiny in this investigation:
{Nn+1=Nn+hε(Nn(1−Nn)(Nn−θ)fPn+1−Nn(1−η)Pn),Pn+1=Pn+h(Nn(1−η)Pn−mPn), | (2.3) |
where h is the step size. The system of difference equation (2.3) can be written in mapping form as follows:
{N→N+hε(N(1−N)(N−θ)fP+1−N(1−η)P),P→P+h(N(1−η)P−mP). | (2.4) |
In this section, the presence of fixed points for the system (2.4) is investigated. Subsequently, an investigation into the stability of these fixed points is conducted, employing either the characteristic polynomial or the eigenvalues of the Jacobian matrix assessed at these fixed points.
The subsequent lemmas aid in examination of the stability of fixed points.
Lemma 3.1. [41] Let F(λ)=λ2+pλ+q be the characteristic equation of the Jacobian matrix at (x,y) and λ1, λ2 be solutions of F(λ)=0; then, (x,y) is a
(1) sink iff |λ1|<1 and |λ2|<1,
(2) saddle point iff |λ1|<1 and |λ2|>1 (or |λ1|>1 and |λ2|<1),
(3) source iff |λ1|>1 and |λ2|>1,
(4) non-hyperbolic point iff |λ1|=1 or |λ2|=1.
Lemma 3.2. [41] Let F(λ)=λ2+pλ+q, where p, q are constants. Suppose that F(1)>0 and λ1, λ2 are roots of F(λ)=0; then,
(1) |λ1|<1 and |λ2|<1 iff F(−1)>0 and F(0)<1,
(2) |λ1|<1 and |λ2|>1 (or |λ2|<1 and |λ1|>1) iff F(−1)<0,
(3) |λ1|>1 and |λ2|>1 iff F(−1)>0 and F(0)>1,
(4) λ1=−1 and |λ2|≠1 iff F(−1)=0 and p≠0,2,
(5) λ1 and λ2 are complex and |λ1|=|λ2|=1 iff p2−4q<0 and F(0)=1.
In this subsection, we will compute the fixed points of system (2.4) and establish the conditions for the asymptotic stability of these fixed points. To determine the fixed points of system (2.4), we need to create the following system of equations:
{N=N+hε(N(1−N)(N−θ)fP+1−N(1−η)P),P=P+h(N(1−η)P−mP). | (3.1) |
Solving the algebraic equation, we get that system (2.4) has the following fixed points:
Theorem 3.1. For system (2.4), the following statements hold true:
(1) A trivial fixed point E0=(0,0),
(2) there are two boundary fixed points E1=(1,0) and E2=(θ,0),
(3) there is a unique positive fixed point E∗=(N∗,P∗), where N∗=m1−η, P∗=−1+√1+4fH2f and H∗=(1−η−m)(m−θ(1−η))(1−η)3,1−mθ<η<1−m.
Theorem 3.2. The trivial fixed point E0=(0,0) is a
(1) sink if 0<h<min{2εθ,2m},
(2) source if max{2εθ,2m}<h,
(3) saddle if 2εθ<h<2m or 2m<h<2εθ,
(4) non-hyperbolic point if h=2m or h=2εθ.
Proof. The Jacobian matrix computed at E0 is given by
J(E0)=[1−hθε00−hm+1]. |
The eigenvalues of J(E0) are λ1=1−hθε and λ2=1−hm. By calculating this, we can get the following:
|1−hθε|{<1 if 0<h<2εθ,=1 if h=2εθ,>1 if h>2εθ,and|1−hm|{<1 if 0<h<2m,=1 if h=2m,>1 if h>2m. |
Remark 1. In the context of system (2.1) [40], the fixed point (0,0) consistently maintains stability. Within the framework of system (2.4), it manifests complex dynamics. From this it can be seen that discrete systems have more complex dynamics than continuous systems.
Theorem 3.3. The boundary fixed point E1=(1,0) is a
(1) sink if {m>1−η,0<h<min{2ε1−θ,2m+η−1},
(2) source if {m>1−η,h>max{2ε1−θ,2m+η−1}, or {m<1−η,h>2ε1−θ,
(3) saddle if any one of the conditions listed below is met:
(a) {m>1−η,2ε1−θ<h<2m+η−1,
(b) {m>1−η,2m+η−1<h<2ε1−θ,
(c) {m<1−η,0<h<2ε1−θ,
(4) non-hyperbolic point if any one of the conditions listed below is met:
(a) h=2ε1−θ,
(b) {m>1−η,h=2m+η−1,
(c) m=1−η.
Proof. The Jacobian matrix computed at E1=(1,0) is given by
J(E1)=[1+h(θ−1)εh(η−1)ε01+(1−η−m)h]. |
The eigenvalues are λ1=1+h(θ−1)ε and λ2=1+h(1−η−m). By calculating this, we can get the following:
|1+h(θ−1)ε|{<1 if 0<h<2ε1−θ,=1 if h=2ε1−θ,>1 if h>2ε1−θ, |
and
|1+h(1−η−m)|{<1 if 0<h<2m+η−1&m>1−η,=1 if m=1−η or h=2m+η−1&m>1−η,>1 if h>2m+η−1&m>1−η or m<1−η&h>0. |
Theorem 3.4. The boundary fixed point E2=(θ,0) is a
(1) source if {m>θ(1−η),h>2m−θ(1−η), or m<θ(1−η),
(2) saddle if {m>θ(1−η),0<h<2m−θ(1−η),
(3) non-hyperbolic point if m=θ(1−η) or {m>θ(1−η),h=2m−θ(1−η).
Proof. The Jacobian matrix computed at E2=(θ,0) is given by
J(E2)=[1+h(−3θ2+(2θ+2)θ−θ)εh((θ3−(θ+1)θ2+θ2)f−θ(1−η))ε01+(θ(1−η)−m)h]. |
The eigenvalues are λ1=1+hθε(1−θ) and λ2=1+h(θ(1−η)−m). By calculating this, we can get the following: |1+hθε(1−θ)|>1 is constant, and
|1+h(θ(1−η)−m)|{<1 if 0<h<2m−θ(1−η)&m>θ(1−η),=1 if m=θ(1−η) or h=2m+θ(η−1)&m>θ(1−η),>1 if h>2m+θ(η−1)&m>θ(1−η) or m<θ(1−η)&h>0. |
Theorem 3.5. The following conditions hold for the unique positive fixed point E∗:
(1) E∗=(N∗,P∗) is a sink if any one of the conditions listed below is met:
(a) −2√S≤A<0 and 0<h<−AS,
(b) A<−2√S and 0<h<−A−√A2−4SS,
(2) E∗=(N∗,P∗) is a source if any one of the conditions listed below is met:
(a) −2√S≤A<0 and h>−AS,
(b) A<−2√S and h>−A+√A2−4SS,
(c) A≥0,
(3) E∗=(N∗,P∗) is a saddle if A<−2√S and −A−√A2−4SS<h<−A+√A2−4SS,
(4) E∗=(N∗,P∗) is non-hyperbolic if any one of the conditions listed below is met:
(a) −2√S<A<0 and h=−AS,
(b) A<−2√S, h=−A±√A2−4SS and h≠−2A,h≠−4A,
where A=m((θ+1)(1−η)−2m)ε(1−η)2(fP∗+1), S=mε((1−η−m)(m−θ(1−η))f(1−η)2(fP∗+1)2+1−η)P∗.
Proof. The Jacobian matrix computed at the point E∗=(N∗,P∗) is as follows:
J(E∗)=[1+mh((θ+1)(1−η)−2m)ε(1−η)2(fP∗+1)−mhε(1−η)((1−η−m)(m−θ(1−η))f(1−η)2(fP∗+1)2+1−η)hP∗(1−η)1]. |
The characteristic polynomial of J(E∗) is given by
F(λ)=λ2−(Ah+2)λ+1+Ah+Sh2=0, |
where
A=m((θ+1)(1−η)−2m)ε(1−η)2(fP∗+1),S=mε((1−η−m)(m−θ(1−η))f(1−η)2(fP∗+1)2+1−η)P∗. |
Then, we get
F(1)=Sh2,F(−1)=4+2Ah+Sh2,F(0)=1+Ah+Sh2. |
It is clear that F(1)=Sh2>0.
Thus, we can conclude with the result.
Here, we choose h as the bifurcation parameter. According to the analysis in Section 3, it is determined that the model can undergo a flip bifurcation when h varies within a small vicinity of L1 or L2, where
L1:h=−A−√A2−4SS,A<−2√S,h≠−2A,−4A,L2:h=−A+√A2−4SS,A<−2√S,h≠−2A,−4A. | (4.1) |
We exclusively examine the flip bifurcation phenomenon when the parameter h undergoes variations within a small neighborhood of L1:h=−A−√A2−4SS. Analogous reasoning can be extended to the case of L2:h=−A+√A2−4SS. Now, we consider a perturbed system derived from system (2.4):
{N→N+(h+h∗)ε(N(1−N)(N−θ)fP+1−N(1−η)P),P→P+(h+h∗)(N(1−η)P−mP), | (4.2) |
where h represents the bifurcation parameter and |h∗|≪1 serves as a small perturbation parameter.
Let x=N−N∗,y=P−P∗. Then, we get the following:
{x→a11x+a12y+a13x2+a14xy+a15y2+a16x3+a17x2y+a18xy2+a19y3+b1xh∗+b2yh∗+b3x2h∗+b4xyh∗+b5y2h∗+O((|x|+|y|+|h∗|)4),y→a21x+a22y+a23x2+a24xy+a25y2+a26x3+a27x2y+a28xy2+a29y3+c1xh∗+c2yh∗+c3x2h∗+c4xyh∗+c5y2h∗+O((|x|+|y|+|h∗|)4), | (4.3) |
where
a11=1+mh((θ+1)(1−η)−2m)ε(1−η)2(fP∗+1),a12=−mhε(1−η)((1−η(m−θ(1−η))−m(m−θ(1−η)))f(1−η)2(fP∗+1)2+1−η),a13=h(−3N∗+θ+1)ε(fP∗+1),a14=h2ε((3(N∗)2−2(θ+1)N∗+θ)f(fP∗+1)2−1+η),a15=h(−(N∗)3+(1+θ)(N∗)2−N∗θ)f2(fP∗+1)3ε,a16=−hε(fP∗+1),a17=h(3N∗−θ−1)f3ε(fP∗+1)2,a18=h(−3(N∗)2+2(1+θ)N∗−θ)f23(fP∗+1)3ε,a19=h((N∗)3−(θ+1)(N∗)2+θN∗)f3(fP∗+1)4ε,b1=12ε(−3(N∗)2+2(θ+1)N∗−θfP∗+1−(1−η)P∗),b2=12ε(((N∗)3−(θ+1)(N∗)2+Nθ)f(fP∗+1)2−N∗(1−η)),b3=−3N∗+θ+13ε(fP∗+1),b4=16ε((3(N∗)2−2(θ+1)N∗+θ)f(fP∗+1)2−1+η),b5=(−(N∗)3+(θ+1)(N∗)2−N∗θ)f23(fP∗+1)3ε,a21=hP∗(1−η),a22=1,a24=12h(1−η),c1=12(1−η)P∗,c2=12(N∗(1−η)−m),c4=16(1−η),a23=a25=a26=a27=a28=a29=c3=c5=0. | (4.4) |
We construct a nonsingular matrix:
T=(a12a12−1−a11λ2−a11), | (4.5) |
and we use the following transformation: (xy)=T(XY). Then, system (4.3) will become as follows:
(XY)→(−100λ2)(XY)+(f(X,Y,h∗)g(X,Y,h∗)), | (4.6) |
where
f(X,Y,h∗)=a13(λ2−a11)a12(λ2+1)x2+a14(λ2−a11)−a12a24a12(λ2+1)xy+a15(λ2−a11)a12(λ2+1)y2+a16(λ2−a11)a12(λ2+1)x3+a17(λ2−a11)a12(λ2+1)x2y+a18(λ2−a11)a12(λ2+1)xy2+a19(λ2−a11)a12(λ2+1)y3+b1(λ2−a11)−a12c1a12(λ2+1)xh∗+b2(λ2−a11)−a12c2a12(λ2+1)yh∗+b3(λ2−a11)a12(λ2+1)x2h∗+b4(λ2−a11)−a12c4a12(λ2+1)xyh∗+b5(λ2−a11)a12(λ2+1)y2h∗+O((|x|+|y|+|h∗|)4),g(X,Y,h∗)=a13(1+a11)a12(λ2+1)x2+a14(1+a11)+a12a24a12(λ2+1)xy+a15(1+a11)a12(λ2+1)y2+a16(1+a11)a12(λ2+1)x3+a17(1+a11)a12(λ2+1)x2y+a18(1+a11)a12(λ2+1)xy2+a19(1+a11)a12(λ2+1)y3+b1(1+a11)+a12c1a12(λ2+1)xh∗+b2(1+a11)+a12c2a12(λ2+1)yh∗+b3(1+a11)a12(λ2+1)x2h∗+b4(1+a11)+a12c4a12(λ2+1)xyh∗+b5(1+a11)a12(λ2+1)y2h∗+O((|x|+|y|+|h∗|)4),x=a12X+a12Y,y=(−1−a11)X+(λ2−a11)Y. |
In accordance with the center manifold theorem, there is the existence of a center manifold denoted as Wc(0,0):
Wc(0,0)={(X,Y,h∗)∈R3:Y=w(X,h∗),w(0,0)=0,Dw(0,0)=0},w(X,h∗)=η1X2+η2Xh∗+η3(h∗)2+O((|X|+|h∗|)3), | (4.7) |
and
Y=η1(−X+f(X,Y,h∗))2+η2(−X+f(X,Y,h∗))h∗+η3(h∗)2=λ2(η1X2+η2Xh∗+η3(h∗)2)+g(X,Y,h∗). |
Through calculation, we obtain
η1=1+a111−λ22(a12a13−a14(1+a11)−a12a24+a15a12(1+a11)2),η2=1(λ2+1)2(b2(1+a11)2a12+(1+a11)(−b1+c2)−a12c1),η3=0. |
The system (2.4) restricted to the center manifold, is given by
F:X→−X+h1X2+h2Xh∗+h3X2h∗+h4X(h∗)2+h5(X)3+O((|X|+|h∗|)4), | (4.8) |
where
h1=(λ2−a11)(a12a13−a14(1+a11)+1a12(1+a11)2)+a12a24(1+a11)1+λ2,h2=b1(λ2−a11)−a12c1−(b2a12(λ2−a11)−c2)(1+a11)1+λ2,h3=(λ2−a11)(a12b3−b4(1+a11)+b5(1+a11)2a12)+(1+a11)a12c41+λ2+η2(2a12a13(λ2−a11)+(−2a11+λ2−1)(a14(λ2−1)−a12a24))1+λ2−2η2a15a12(1+λ2)(λ2−a11)2(1+a11)+η1((λ2−a11)(b1+c2)+b2a12(λ2−a11)2−a12c1)1+λ2,h4=η2((λ2−a11)(b1+c2)+b2a12(λ2−a11)2−a12c1)1+λ2,h5=(λ2−a11)1+λ2(a212a16−a12a17(1+a11)+a18(1+a11)2−a19(1+a11)3a12)+η11+λ2(2a12a13(λ2−a11)+(−2a11+λ2−1)(a14(λ2−1)−a12a24))−2a15η1(λ2−a11)2(1+a11)a12(1+λ2). |
Now, regarding the occurrence of flip bifurcation, it is crucial that the two quantities, α1 and α2, are both non-zero, where
α1=(∂2F∂X∂h+12∂F∂h∂2F∂X2)|(0,0)=h2, | (4.9) |
α2=(16∂3F∂X3+(12∂2F∂X2)2)|(0,0)=h5+(h1)2. | (4.10) |
As a result of the aforementioned analysis, we summarize the following lemma on the existence and direction of the flip bifurcation.
Lemma 4.1. If α1≠0, α2≠0, then system (2.4) undergoes a flip bifurcation at the positive equilibrium point E∗=(N∗,P∗) when h in a small neighborhood of L1. And when α2>0 (respectively, α2<0), system (2.4) bifurcates from the fixed point E∗=(N∗,P∗) to a 2-periodic stable orbit (respectively, unstable).
Neimark-Sacker bifurcation manifests when the eigenvalues of the characteristic equation form complex conjugate pairs with a unit modulus. Based on the preceding discussion, we know that the model can undergo a Neimark-Sacker bifurcation when h varies within a small neighborhood of L3, where
L3:h=−AS,−2√S<A<0. | (4.11) |
Now, we examine a perturbation system derived from system (2.4):
{N→N+(h+ˉh)ε(N(1−N)(N−θ)fP+1−N(1−η)P),P→P+(h+ˉh)(N(1−η)P−mP), | (4.12) |
where h represents the bifurcation parameter, and |ˉh|≪1 serves as a small perturbation parameter. Let x=N−N∗ and y=P−P∗:
{x→a11x+a12y+a13x2+a14xy+a15y2+a16x3+a17x2y+a18xy2+a19y3+O((|x|+|y|)4),y→a21x+a22y+a23x2+a24xy+a25y2+a26x3+a27x2y+a28xy2+a29y3+O((|x|+|y|)4), | (4.13) |
where aij(i=1,2,j=1,2,⋯9) are given in (4.4) by changing h to h+ˉh. The characteristic equation corresponding to the linear part of the system (4.13) at the fixed point (0, 0) is given by
λ2+p(ˉh)λ+q(ˉh)=0, | (4.14) |
where p(ˉh)=−2−A(h+ˉh), q(ˉh)=1+A(h+ˉh)+S(h+ˉh)2.
The roots of (4.14) are complex and given by
λ1(ˉh),λ2(ˉh)=−p(ˉh)2±√4q(ˉh)−p2(ˉh)2i=1+(h+ˉh)(A2±√4S−A22i). | (4.15) |
When ˉh=0 and h∈l, by computation, we obtain q(0)=1,λ1(0), λ2(0)=1+Ah2±h√4S−A22i, and |λ1(0)|=|λ2(0)|=1.
It is clear from the above discussion that
|λ12(ˉh)|=√q(ˉh),d=d|λ(ˉh)|dˉh|ˉh=0=−A2>0. |
In addition, it is required that when ˉh=0, λ1n,λ2n≠1(n=1,2,3.4), which is equivalent to p(0)≠0,1,2,−2. Because h∈L3, we get that p(0)≠2,−2. Additionally, we necessitate that p(0)≠0 and 1, which gives
A2≠2S,A2≠3S. | (4.16) |
Let λ1(0),λ2(0)=ρ±ωi. To bring the linear component of (4.13) into its canonical form at ˉh=0, we apply the following transformation:
(xy)=(ωa11−ρ0a21)(uv). | (4.17) |
So x=ωu+(a11−ρ)v and y=a12v. Under the transformation (4.17), the system (4.13) will become as follows:
(uv)→(ρ−ωωρ)(uv)+(φ(u,v)ϕ(u,v)), | (4.18) |
where
φ(u,v)=a13ωx2+a14a21+a24(ρ−a11)a21ωxy+a15ωy2+a16ωx3+a17ωx2y+a18ωxy2+a19ωy3+O((|x|+|y|)4),ϕ(u,v)=1a21(a24xy+O((|x|+|y|)4)),x=ωu+(a11−ρ)v,y=a12v. |
Then, we get
φ(u,v)=ωa13u2+(2a13(a11−ρ)+(a14a21+a24(−a11+ρ))a12a21)uv+(a13(a11−ρ)2ω+(a14a21+a24(−a11+ρ))(a11−ρ)a12a21ω+a15a212ω)v2+a16ω2u3+(3a16ω(a11−ρ)+a17ωa12)u2v+(3a16(a11−ρ)2+2a17(a11−ρ)a12+a18a212)uv2+1ω(a16(a11−ρ)3+a17(a11−ρ)2a12+a18(a11−ρ)a212+a19a312)v3+O((|u|+|v|)4),ϕ(u,v)=a24a12ωa21uv+a24(a11−ρ)a12a21v2+O((|u|+|v|)4). |
Next, a non zero real number is defined as follows:
˜l=−[Re((1−2λ)λ21−λc11c20)−12|c11|2−|c02|2+Re(ˉλc21)]|ˉh=0, | (4.19) |
where
c20=18[(φuu−φvv+2ϕuv)+i(ϕuu−ϕvv−2φuv)],c11=14[(φuu+φvv)+i(ϕuu+ϕvv)],c02=18[(φuu−φvv−2ϕuv)+i(ϕuu−ϕvv+2φuv)],c21=116[(φuuu+φuvv+ϕuuv+ϕvvv)+i(ϕuuu+ϕuvv−φuuv−φvvv)]. |
Based on the aforementioned calculations, we establish the lemma regarding the presence and direction of Neimark-Sacker bifurcation.
Lemma 4.2. If the condition (4.16) holds and ˜l≠0, then system (2.4) undergoes a Neimark-Sacker bifurcation at the fixed point E∗=(N∗,P∗) when the parameter h varies in a small neighborhood of L3. Moreover, if ˜l<0 (resp., ˜l>0), then an attracting (resp., repelling) invariant closed curve bifurcates from the fixed point for ˉh>0 (resp., ˉh<0).
Chaos is often a detrimental phenomenon for biological systems; they often disrupt the ecological balance of biological population systems and directly affect one's long-term projections of population growth. By implementing appropriate control policies, not only can the size of ecological populations be protected, human beings can also lay a good foundation for the sustainable exploitation of ecological resources. Therefore, three control methods are used in this section to provide an effective control of the chaos generated by system (2.4).
In this section, the state feedback control method [42] will be employed to regulate the chaos exhibited by system (2.4). To achieve this, a feedback control term is introduced into system (2.4):
{Nn+1=Nn+hε(Nn(1−Nn)(Nn−θ)fPn+1−Nn(1−η)Pn)+un,Pn+1=Pn+h(Nn(1−η)Pn−mPn), | (5.1) |
where
un=−r1(Nn−N∗)−r2(Pn−P∗), | (5.2) |
denotes the feedback controlling force, while r1 and r2 represent the feedback gains. The point (N∗,P∗) is the unique positive equilibrium point of system (2.4).
The Jacobian matrix at the equilibrium point (N∗,P∗) for system (5.1) is given by
J=(d11−r1d12−r2d21d22), | (5.3) |
where
d11=1+mh((θ+1)(1−η)−2m)ε(1−η)2(fP∗+1),d12=−mhε(1−η)((1−η(m−θ(1−η))−m(m−θ(1−η)))f(1−η)2(fP∗+1)2+1−η),d21=hP∗(1−η),d22=1. | (5.4) |
Therefore, the characteristic equation associated with J(N∗,P∗) is given by
λ2−(d11+d22−r1)λ+d22(d11−r1)−d21(d12−r2)=0. | (5.5) |
Let λ1 and λ2 represent the eigenvalues of the characteristic Eq (5.5). Then,
λ1+λ2=d11+d22−r1,λ1λ2=d22(d11−r1)−d21(d12−r2). |
Then, we can determine the lines of marginal stability:
l1:r1d22−r2d21=d11d22−d12d21−1, | (5.6) |
l2:r1(1−d22)+r2d21=d11+d22+d21d12−d11d22−1, | (5.7) |
l3:r1(1+d22)−r2d21=d11+d22−d21d12+d11d22+1. | (5.8) |
Theorem 5.1. If r1, r1 lie in a triangular region bounded by lines l1, l2, and l3, it can be concluded that the system (5.1) is stable.
In this subsection, we investigate a technique for chaos control that relies on the OGY method, as outlined in [43]. By taking m as the control parameter, we rewrite the system (2.4) as follows:
{Nn+1=Nn+hε(Nn(1−Nn)(Nn−θ)fPn+1−Nn(1−η)Pn)=f(Nn,Pn,m),Pn+1=Pn+h(Nn(1−η)Pn−mPn)=g(Nn,Pn,m). | (5.9) |
Furthermore, let us assume that m∈(m0−δ0,m0+δ0) with δ0>0 and m0 denotes the nominal value of m. Then, model (5.9) can be approximated near (N∗,P∗) by applying the following linear map:
(Nn+1−N∗Pn+1−P∗)=J(N∗,P∗,m0)(Nn−N∗Pn−P∗)+M(m−m0), | (5.10) |
where
J(N∗,P∗,m0)=(∂f(N∗,P∗,m0)∂N∂f(N∗,P∗,m0)∂P∂g(N∗,P∗,m0)∂N∂g(N∗,P∗,m0)∂P),M=(∂f(N∗,P∗,m0)∂m∂g(N∗,P∗,m0)∂m)=(0−hP∗). |
The controllability of model (5.9) is evident when considering the matrix
˜T=(MJM)=(∂f(N∗,P∗,m0)∂m∂f(N∗,P∗,m0)∂P⋅∂g(N∗,P∗,m0)∂m∂g(N∗,P∗,m0)∂m∂g(N∗,P∗,m0)∂P⋅∂g(N∗,P∗,m0)∂m), | (5.11) |
and ensuring that it has rank 2.
Next, we assume that (m−m0)=−K(Nn−N∗Pn−P∗), where K=[k1k2]. Then, model (5.9) can be written as
(Nn+1−N∗Pn+1−P∗)=(J−MK)(Nn−N∗Pn−P∗). | (5.12) |
Then, the controller model is given by
{Nn+1=Nn+hε(Nn(1−Nn)(Nn−θ)fPn+1−Nn(1−η)Pn),Pn+1=Pn+h(Nn(1−η)Pn−(m−k1(Nn−N∗)−k2(Pn−P∗))Pn). | (5.13) |
Furthermore, the equilibrium point (N∗,P∗) achieves local asymptotic stability if and only if both eigenvalues of the matrix J−MK, denoted as λ1 and λ2, reside within an open unit disk. The matrix J−MK can be expressed as follows:
J−MK=(j11j12j21−βk1j22−βk2), | (5.14) |
where
j11=1+hε(−3(N∗)2+(2θ+2)N∗−θfP∗+1−(1−η)P∗),j12=hε(((N∗)3−(θ+1)(N∗)2+N∗θ)f(fP∗+1)2−N∗(1−η)),j21=hP∗(1−η),j22=1,β=−hP∗. |
Then, the characteristic polynomial is given by
λ2−(j11+j22−βk2)λ+j11(j22−βk2)−j12(j21−βk1)=0. | (5.15) |
Subsequently, we can derive the lines of marginal stability, denoted as follows:
m1:j11(j22−βk2)−j12(j21−βk1)−1=0, | (5.16) |
m2:j22+j12j21+β((j11−1)k2−j12k1)+j11(1−j22)−1=0, | (5.17) |
m3:j22−j12j21+β(j12k1−(j11+1)k2)+j11(1+j22)+1=0. | (5.18) |
Theorem 5.2. If k1, k1 lie in a triangular region bounded by lines m1, m2, and m3, it can be concluded that the system (5.13) is stable.
In this subsection, we employ the hybrid control technique [44] to control chaos. The controlled system discussed herein corresponds to system (2.4), as depicted below:
{Nn+1=ζ(Nn+hε(Nn(1−Nn)(Nn−θ)fPn+1−Nn(1−η)Pn))+(1−ζ)Nn,Pn+1=ζ(Pn+h(Nn(1−η)Pn−mPn))+(1−ζ)Pn, | (5.19) |
where 0<ζ<1. This is a control strategy that combines feedback control and parameter adjustment. The Jacobian matrix at the equilibrium point (N∗,P∗) for the system given by Eq (5.19) is as follows:
J=(σ11σ12σ21σ22), | (5.20) |
where
σ11=ξ(1+hε(−3(N∗)2+(2θ+2)N∗−θfP∗+1−(1−η)P∗))+1−ς,σ12=hξε(((N∗)3−(θ+1)(N∗)2+N∗θ)f(fP∗+1)2−N∗(1−η)),σ21=ξP∗(1−η)h,σ22=1. | (5.21) |
According to the Jury condition, the equilibrium point of the system is stable if and only if the following conditions are satisfied:
|σ11+σ22|<1+σ22σ11−σ21σ12<2. | (5.22) |
Theorem 5.3. If |σ11+σ22|<1+σ22σ11−σ21σ12<2 can hold, it can be concluded that the system (5.19) is stable.
The objective of this section is to illustrate the feasibility of the main theoretical results and to depict the intricate dynamical behaviors of system (2.4). To achieve this, we present bifurcation diagrams and phase portraits through numerical simulations. Exploring the impact of various biological effects on discrete predator-prey systems is crucial. In this section, we will respectively investigate the effects of the fear effect, refuges, and the Allee effect on the system.
We chose the parameter values as follows:
ε=0.1858,θ=0.5,f=0.9,m=0.7,η=0.29,x(1)=0.961,y(1)=0.04,h∈(0.75,1.15). | (6.1) |
The flip bifurcation value is computed as h=−A−√A2−4SS=0.8085, and the interior fixed point is computed as E∗=(0.9859,0.00956). The eigenvalues of the Jacobian matrix are λ1=−1 and λ2=0.991554931907570, as well as α1=−1.235833646 and α2=11.9477919, affirming that system (2.4) undergoes flip bifurcation at E∗ as the bifurcation parameter h crosses h=0.8085. Referring to Figure 1, we see that the bifurcation values obtained in Figure 1(a) align with the conclusions from Lemma 4.1, and it is evident that the prey-eating population experiences a flip bifurcation, eventually leading to chaos. Furthermore, Figure 1(b) shows the maximum Lyapunov exponents to confirm the chaotic behavior of system (2.4). When we compare Figure 1(b) to Figure 1(a), we can observe that the prey population enters a chaotic state when the maximum Lyapunov exponent is greater than 0.
We chose the parameter values as follows:
ε=1,θ=0.1,f=1,m=0.6,η=0.03,x(1)=0.6,y(1)=0.02,h∈(0.5,1.5). | (6.2) |
The Neimark-Sacker bifurcation value is computed as h=−AS=0.6226263491 and the interior fixed point of system (2.4) is identified as E∗=(0.6185,0.1737). The eigenvalues of the Jacobian matrix are given by λ1,2=0.9775042841±0.2109155626i with |λ1|=|λ2|=1, as well as d=0.03613036280>0, confirming the occurrence of Neimark-Sacker bifurcation at E∗. Additionally, the value of the first Lyapunov exponent is computed as ˜l=−0.00080098583420, verifying the validity of Lemma 4.2. The system's two-dimensional bifurcation diagram is shown in Figure 2(a). As h increases, we can see that the system's fixed point shifts from a stable to a chaotic state. The maximum Lyapunov exponent map corresponding to Figure 2(a) is given in Figure 2(b); we observe that, when the maximum Lyapunov exponent exceeds 0, the prey population enters a chaotic state. Some classical phase diagrams are given in Figure 3, which clearly show how smooth invariant circles bifurcate from stable fixed points E∗=(0.6185,0.1737) and eventually develop into chaotic sets.
Remark 2. In the context of the system described in [39], it was found that the system remained non-chaotic as parameters changed during the Neimark-Sacker bifurcation. However, our system in this study demonstrated chaos in the system as parameter h changed Neimark-Sacker bifurcation. Hence, we conclude that the addition of prey refuge increases the complexity of the biological system.
We chose the parameter values as follows:
ε=1,θ=0.1,f=1,m=0.6,η=0.03,x(1)=0.6,y(1)=0.02,h=2.45566. | (6.3) |
By evaluating Figure 3, we can get that the variables N and P in the system (2.4) are in a chaotic state when the parameter is (6.3); the corresponding time series plot is as follows; see Figure 4.
We consider the same parameter values as in (6.3). In Figure 5, the triangular region, as determined by Theorem 5.1, confines the parameters r1 and r2. Within this region, the chaotic behavior generated by the system (2.4) is effectively controlled, leading to asymptotic convergence near the fixed point E∗=(0.6185,0.1737).
For the case that the feedback gains are r1=1.27 and r2=1.1 and the controller is initiated at the 500th iteration of the system, Figure 6 illustrates the control effect, wherein a chaotic trajectory is successfully stabilized at the fixed point E∗=(0.6185,0.1737). Consequently, it can be inferred that the utilization of the feedback control approach proves effective in the mitigation of bifurcation and chaos.
We consider the same parameter values as in (6.3). In Figure 7, the triangular region, as determined by Theorem 5.2, confines the parameters k1 and k2. Within this region, the chaotic behavior generated by the system (2.4) is effectively controlled, leading to asymptotic convergence near the fixed point E∗=(0.6185,0.1737).
The values k1=0.2 and k2=−3 were taken in the stabilization region and the controller was initiated at the 500th iteration of the system; Figure 8 illustrates the control effect, wherein a chaotic trajectory is successfully stabilized at the fixed point E∗=(0.6185,0.1737). Consequently, it can be inferred that the utilization of the OGY control method approach proves effective in the mitigation of bifurcation and chaos.
For the controlled system (5.19), when we consider the same parameter values as in (6.3), according to Theorem 5.3, the stability range of the hybrid control system is 0<ξ<0.831662531. Taking ξ=0.4, Figure 9 documents the bifurcation of the controlled system; by comparison with Figure 2(a), it can be seen that the Neimark-Sacker bifurcation of the system is delayed. By employing small values of the control parameter ξ, the Neimark-Sacker bifurcation can be postponed over a broader range of h. Consequently, it can be inferred that the utilization of the hybrid control approach proves effective in the mitigation of bifurcation and chaos.
We chose the parameter values as follows:
ε=1,θ=0.1,f=0.3,m=0.6,η=0.03,x(1)=0.6,y(1)=0.02. | (6.4) |
Neimark-Sacker bifurcation value is calculated as h=−AS=0.6775878938, and the internal immobility point is calculated as E∗=(0.6186,0.1928). See Figure 10. Comparison with Figure 2(a) shows that the Neimark-Sacker bifurcation of the system was delayed from h=0.6226263491 to h=0.6775878938 when the fear effect constant was varied from 1 to 0.3. This means that the change of biological populations in the system will not be too drastic. This helps to maintain biodiversity in the ecosystem so that various biological populations can coexist in a relatively stable manner.
We chose the parameter values as follows:
ε=1,θ=0.1,f=1,m=0.6,η=0.01,x(1)=0.6,y(1)=0.02. | (6.5) |
The Neimark-Sacker bifurcation value is calculated as h=−AS=0.4954418794, and the internal immobility point is calculated as E∗=(0.6061,0.1718). See Figure 11. Comparison with Figure 2(a) shows that the Neimark-Sacker bifurcation of the system was advanced from h=0.6226263491 to h=0.4954418794 when the refuge constant was varied from 0.03 to 0.01. This means that ecosystems are more susceptible to external disturbances, thereby increasing the risk of abrupt changes in the system. This is an important warning sign for environmental conservation and resource managers that ecosystem change and stability need to be more closely monitored.
We chose the parameter values as follows:
ε=1,θ=0.05,f=1,m=0.6,η=0.03,x(1)=0.6,y(1)=0.02. | (6.6) |
The Neimark-Sacker bifurcation value is calculated as h=−AS=0.7678598057, and the internal immobility point is calculated as E∗=(0.6186,0.1882). See Figure 12. In terms of comparison with Figure 2(a), Figure 12 shows that the Neimark-Sacker bifurcation of the system was delayed from h=0.6226263491 to h=0.7678598057 when the Allee effect constant was varied from 0.1 to 0.05. This means that the change of biological populations in the system will not be too drastic. This helps to maintain biodiversity in the ecosystem so that various biological populations can coexist in a relatively stable manner.
This study investigated a discrete prey-predator system that incorporates a fear factor, a strong Allee effect, and prey refuge. Employing the forward Euler scheme on the system (2.2) yielded the discrete system (2.4). Notably, the fixed points of the discrete system (2.4) align with those of its continuous counterpart (2.2). However, despite this similarity, the dynamic behaviors of systems (2.2) and (2.4) diverge significantly. We performed a comprehensive examination of the local stability from the perspective of the fixed points, delving meticulously into the specifics of local bifurcations that occur at the interior fixed point. Our findings revealed that system (2.4) undergoes both flip and Neimark-Sacker bifurcations. Under the conditions of system (2.2), the fixed point (0,0) consistently maintains stability. However, within the framework of system (2.4), it manifests complex dynamics. Furthermore, the topological classification of fixed points in the system (2.4) is contingent upon the chosen step size, denoted as h. Our numerical simulations demonstrated that flip and Neimark-Sacker bifurcations manifest when a large step size is employed in Euler's method. In contrast, the dynamics of the system (2.2) remain independent of h. Consequently, we have compelling grounds to assert that the dynamic behavior of the system (2.4) is more intricate than that of the system (2.2).
We also discuss the impacts of the fear effect, refuge, and the Allee effect on the system. Decreasing the fear or Allee effect parameters delays the Neimark-Sacker bifurcation and results in an increase in the population densities of prey and predators at the stable fixed point. Conversely, reducing prey refuge size parameters advances the Neimark-Sacker bifurcation and leads to a decrease in the population densities of prey and predators at the stable fixed point. Hence, all three effects significantly influence the system.
In addition to investigating the dynamic behavior of discrete predator-prey systems under various biological effects, it is imperative to understand the biological significance of chaos control. Effectively regulating chaos in ecological systems holds profound implications for ecosystem stability and biodiversity conservation. Chaotic dynamics in predator-prey interactions can lead to unpredictable population fluctuations and destabilize ecosystems, potentially threatening the survival of species and disrupting ecological balance. By applying these three chaos control methods, i.e., state feedback control, OGY feedback control, and hybrid control, we effectively managed the chaotic behavior and bifurcations generated by the system (2.4). This intervention allowed us to mitigate the adverse effects of chaos and bifurcations, consequently enhancing ecosystem resilience. Furthermore, this approach not only advances our comprehension of ecological processes, it also offers invaluable insights for sustainable ecosystem management and biodiversity preservation.
To validate and illustrate our theoretical analysis, we conducted numerical simulations. The outcomes of these simulations are presented in the forms of bifurcation diagrams, phase portraits, and time-series plots, providing a comprehensive visualization of the system's dynamic behavior as discussed in the theoretical framework. Furthermore, in the numerical simulations, we observed that feedback control methods and the OGY control method are simple and intuitive, allowing for the control of chaotic systems by adjusting parameters or applying external forcing. However, the abundance of parameters and their interdependence necessitate meticulous calibration. In contrast, hybrid control, which does not require precise system models, combines the advantages of parameter perturbation control and state feedback control, thus enhancing adaptability to chaotic systems.
In future work, the model can be discretized by using different discretization methods. In addition, new discretization parameters could be chosen for the study in order to obtain specific biological properties to explore the influence of different ecological effects on the predator-prey model.
Here are some useful lemmas for novice authors to include in their articles.
Lemma 7.1. (Jacobian matrix [45]) Let f(x)=[f1(x),f2(x),...,fm(x)]T be a vector-valued function of n variables x1,x2,...,xn, where x=[x1,x2,...,xn]T is an n-dimensional vector and f1,f2,...,fm are real-valued functions. If each component function f1,f2,...,fm has continuous first-order partial derivatives at some point, then the Jacobian matrix J is an m×n matrix, where the element in the i-th row and j-th column is the partial derivative of the component function fi with respect to the variable xj, given by
J=[∂f1∂x1∂f1∂x2⋯∂f1∂xn∂f2∂x1∂f2∂x2⋯∂f2∂xn⋮⋮⋱⋮∂fm∂x1∂fm∂x2⋯∂fm∂xn]. |
Assume the following discrete system:
xk→f(xk), | (7.1) |
where f:G→Rn is a continuous smooth map from the Rn open region G to Rn. The mapping (7.1) can be transformed by a linear transformation into the following form
(γκ)→(Bγ+g1(γ,κ)Cκ+g2(γ,κ)), | (7.2) |
where B has eigenvalues on the unit disk and all C eigenvalues are either inside or outside of the circle.
Lemma 7.2. [46] The localized center manifold theorem for map (7.2) can be expressed as follows:
Wc={(γ,κ)|κ=Z(γ),|γ|<δ,Z(0)=0,DZ(0)=0,δ≤1}. | (7.3) |
The center manifold provides an effective way to reduce the dimensionality of the system, thereby simplifying the description and analysis of the system. By studying the dynamic behavior of the system on the center manifold, we can better understand the stability and dynamical characteristics of the system near fixed points.
The authors declare they have not used Artificial Intelligence (AI) tools in the creation of this article.
This work was supported by the National Natural Science Foundation of China (No. 61074005) and the Talent Project of the High Education of Liaoning Province (LR2012005), as well as by the Youth Research Project of the Educational Department of Liaoning Province (JYTQN2023284) and the Science and Technology Project of the Science of Technology Department of Liaoning Province (2023BSBA241).
The authors declare no conflict of interest.
[1] |
Y. Nutku, Hamiltonian structure of the Lotka-Volterra equations, Phys. Lett. A, 145 (1990), 27–28. https://doi.org/10.1016/0375-9601(90)90270-X doi: 10.1016/0375-9601(90)90270-X
![]() |
[2] |
M. Haque, A predator-prey model with disease in the predator species only, Nonlinear Anal.-Real, 11 (2010), 2224–2236. https://doi.org/10.1016/j.nonrwa.2009.06.012 doi: 10.1016/j.nonrwa.2009.06.012
![]() |
[3] |
M. Haque, A detailed study of the Beddington-DeAngelis predator-prey model, Math. Biosci., 234 (2011), 1–16. https://doi.org/10.1016/j.mbs.2011.07.003 doi: 10.1016/j.mbs.2011.07.003
![]() |
[4] |
P. Majumdar, B. Mondal, S. Debnathm, U. Ghosh, Controlling of periodicity and chaos in a three dimensional prey predator model introducing the memory effect, Chaos Soliton. Fract., 164 (2022), 112585. https://doi.org/10.1016/j.chaos.2022.112585 doi: 10.1016/j.chaos.2022.112585
![]() |
[5] |
Q. L. Chen, Z. D. Teng, Z. Y. Hu, Bifurcation and control for a discrete-time prey-predator model with Holling-Ⅳ functional response, Int. J. Ap. Mat. Com.-Pol., 23 (2013), 247–261. https://doi.org/10.2478/amcs-2013-0019 doi: 10.2478/amcs-2013-0019
![]() |
[6] |
J. L. Chen, Z. L. Zhu, X. Q. He, F. D. Chen, Bifurcation and chaos in a discrete predator-prey system of Leslie type with Michaelis-Menten prey harvesting, Open Math., 20 (2022), 608–628. https://doi.org/10.1515/math-2022-0054 doi: 10.1515/math-2022-0054
![]() |
[7] |
J. Roy, S. Dey, M. Banerjee, Maturation delay induced stability enhancement and shift of bifurcation thresholds in a predator-prey model with generalist predator, Math. Comput. Simulat., 211 (2023), 368–393. https://doi.org/10.1016/j.matcom.2023.04.019 doi: 10.1016/j.matcom.2023.04.019
![]() |
[8] | W. C. Allee, Animal aggregations: A study in general sociology, Chicago: University of Chicago Press, 1931. https://doi.org/10.5962/bhl.title.7313 |
[9] |
P. A. Stephens, W. J. Sutherland, Consequences of the Allee effect for behaviour, ecology and conservation, Trends Ecol. Evol., 14 (1999), 401–405. https://doi.org/10.1016/S0169-5347(99)01684-5 doi: 10.1016/S0169-5347(99)01684-5
![]() |
[10] |
E. Angulo, G. M. Luque, S. D. Gregory, J. W. Wenzel, C. B. Gomes, L. Berec, et al., Allee effects in social species, J. Anim. Ecol., 87 (2018), 47–58. https://doi.org/10.1111/1365-2656.12759 doi: 10.1111/1365-2656.12759
![]() |
[11] |
W. Z. Lidicker Jr, The Allee effect: Its history and future importance, Open Ecol. J., 3 (2010), 71–82. https://doi.org/10.2174/1874213001003010071 doi: 10.2174/1874213001003010071
![]() |
[12] |
A. Arsie, C. Kottegoda, C. Shan, A predator-prey system with generalized Holling type Ⅳ functional response and Allee effects in prey, J. Differ. Equations, 309 (2022), 704–740. https://doi.org/10.1016/j.jde.2021.11.041 doi: 10.1016/j.jde.2021.11.041
![]() |
[13] |
L. M. Zhang, T. Wang, Qualitative properties, bifurcations and chaos of a discrete predator-prey system with weak Allee effect on the predator, Chaos Soliton. Fract., 175 (2023), 113995. https://doi.org/10.1016/j.chaos.2023.113995 doi: 10.1016/j.chaos.2023.113995
![]() |
[14] |
Y. Chang, W. Feng, M. Freeze, X. Lu, C. Smith, Elimination, permanence, and exclusion in a competition model under Allee effects, AIMS Math., 8 (2023), 7787–7805. https://doi.org/10.3934/math.2023391 doi: 10.3934/math.2023391
![]() |
[15] |
S. Creel, D. Christianson, S. Liley, J. A. Winnie Jr, Predation risk affects reproductive physiology and demography of elk, Science, 315 (2007), 960–960. https://doi.org/10.1126/science.1135918 doi: 10.1126/science.1135918
![]() |
[16] |
E. L. Preisser, D. I. Bolnick, The many faces of fear: Comparing the pathways and impacts of nonconsumptive predator effects on prey populations, PloS One, 3 (2008). https://doi.org/10.1371/journal.pone.0002465 doi: 10.1371/journal.pone.0002465
![]() |
[17] |
K. B. Altendorf, J. W. Laundré, C. A. L. González, J. S. Brown, Assessing effects of predation risk on foraging behavior of mule deer, J. Mammal., 82 (2001), 430–439. https://doi.org/10.1644/1545-1542(2001)082<0430:AEOPRO>2.0.CO;2 doi: 10.1644/1545-1542(2001)082<0430:AEOPRO>2.0.CO;2
![]() |
[18] |
S. Creel, D. Christianson, Relationships between direct predation and risk effects, Trends Ecol. Evol., 23 (2008), 194–201. https://doi.org/10.1016/j.tree.2007.12.004 doi: 10.1016/j.tree.2007.12.004
![]() |
[19] |
S. L. Lima, Nonlethal effects in the ecology of predator-prey interactions, Bioscience, 48 (1998), 25–34. https://doi.org/10.2307/1313225 doi: 10.2307/1313225
![]() |
[20] |
S. L. Lima, Predators and the breeding bird: Behavioral and reproductive flexibility under the risk of predation, Biol. Rev., 84 (2009), 485–513. https://doi.org/10.1111/j.1469-185X.2009.00085.x doi: 10.1111/j.1469-185X.2009.00085.x
![]() |
[21] |
Z. W. Liang, X. Y. Meng, Stability and Hopf bifurcation of a multiple delayed predator-prey system with fear effect, prey refuge and Crowley-Martin function, Chaos Soliton. Fract., 175 (2023), 113955. https://doi.org/10.1016/j.chaos.2023.113955 doi: 10.1016/j.chaos.2023.113955
![]() |
[22] |
S. Debnath, P. Majumdar, S. Sarkar, U. Ghosh, Memory effect on prey-predator dynamics: Exploring the role of fear effect, additional food and anti-predator behaviour of prey, J. Comput. Sci., 55 (2023), 101929. https://doi.org/10.1016/j.jocs.2022.101929 doi: 10.1016/j.jocs.2022.101929
![]() |
[23] |
B. F. Xie, N. Zhang, Influence of fear effect on a Holling type Ⅲ prey-predator system with the prey refuge, AIMS Math., 7 (2022), 1811–1830. https://doi.org/10.3934/math.2022104 doi: 10.3934/math.2022104
![]() |
[24] |
J. N. McNair, The effects of refuges on predator-prey interactions: A reconsideration, Theor. Popul. Biol., 29 (1986), 38–63. https://doi.org/10.1016/0040-5809(86)90004-3 doi: 10.1016/0040-5809(86)90004-3
![]() |
[25] |
A. Sih, Prey refuges and predator-prey stability, Theor. Popul. Biol., 31 (1987), 1–12. https://doi.org/10.1016/0040-5809(87)90019-0 doi: 10.1016/0040-5809(87)90019-0
![]() |
[26] |
V. Krivan, Effects of optimal antipredator behavior of prey on predator-prey dynamics: The role of refuges, Theor. Popul. Biol., 53 (1998), 131–142. https://doi.org/10.1006/tpbi.1998.1351 doi: 10.1006/tpbi.1998.1351
![]() |
[27] |
A. C. Crombie, Further experiments on insect competition, P. Roy. Soc. London S., 133 (1946), 76–109. https://doi.org/10.1098/rspb.1946.0004 doi: 10.1098/rspb.1946.0004
![]() |
[28] |
J. Ghosh, B. Sahoo, S. Poria, Prey-predator dynamics with prey refuge providing additional food to predator, Chaos Soliton. Fract., 96 (2017), 110–119. https://doi.org/10.1016/j.chaos.2017.01.010 doi: 10.1016/j.chaos.2017.01.010
![]() |
[29] |
A. Das, G. P. Samanta, A prey-predator model with refuge for prey and additional food for predator in a fluctuating environment, Phys. A, 538 (2020), 1228449. https://doi.org/10.1016/j.physa.2019.122844 doi: 10.1016/j.physa.2019.122844
![]() |
[30] |
A. A. Thirthar, S. J. Majeed, M. A. Alqudah, P. Panja, T. Abdeljawad, Fear effect in a predator-prey model with additional food, prey refuge and harvesting on super predator, Chaos Soliton. Fract., 159 (2022), 112091. https://doi.org/10.1016/j.chaos.2022.112091 doi: 10.1016/j.chaos.2022.112091
![]() |
[31] |
K. P. Hadeler, H. Gerstmann, The discrete Rosenzweig model, Math. Biosci., 98 (1990), 49–72. https://doi.org/10.1016/0025-5564(90)90011-M doi: 10.1016/0025-5564(90)90011-M
![]() |
[32] |
M. B. Ajaz, U. Saeed, Q. Din, I. Ali, I. M. I. Siddiqui, Bifurcation analysis and chaos control in discrete-time modified Leslie-Gower prey harvesting model, Adv. Differential Equ., 2020 (2020), 1–24. https://doi.org/10.1186/s13662-020-2498-1 doi: 10.1186/s13662-020-2498-1
![]() |
[33] |
P. A. Naik, Z. Eskandari, M. Yavuz, J. Zu, Complex dynamics of a discrete-time Bazykin-Berezovskaya prey-predator model with a strong Allee effect, J. Comput. Appl. Math., 413 (2022), 114401. https://doi.org/10.1016/j.cam.2022.114401 doi: 10.1016/j.cam.2022.114401
![]() |
[34] |
T. W. Zhang, Y. T. Liu, H. Z. Qu, Global mean-square exponential stability and random periodicity of discrete-time stochastic inertial neural networks with discrete spatial diffusions and Dirichlet boundary condition, Comput. Math. Appl., 141 (2023), 116–128. https://doi.org/10.1016/j.camwa.2023.04.011 doi: 10.1016/j.camwa.2023.04.011
![]() |
[35] |
T. W. Zhang, Z. H. Li, Switching clusters' synchronization for discrete space-time complex dynamical networks via boundary feedback controls, Pattern Recogn., 143 (2023), 109763. https://doi.org/10.1016/j.patcog.2023.109763 doi: 10.1016/j.patcog.2023.109763
![]() |
[36] |
T. W. Zhang, Y. K. Li, Global exponential stability of discrete-time almost automorphic Caputo-Fabrizio BAM fuzzy neural networks via exponential Euler technique, Knowl.-Based Syst., 246 (2022), 108675. https://doi.org/10.1016/j.knosys.2022.108675 doi: 10.1016/j.knosys.2022.108675
![]() |
[37] |
S. Rana, A. R. Bhowmick, S. Bhattacharya, Impact of prey refuge on a discrete time predator-prey system with Allee effect, Int. J. Bifurcat. Chaos, 24 (2014), 1450106. https://doi.org/10.1142/S0218127414501065 doi: 10.1142/S0218127414501065
![]() |
[38] |
R. Ma, Y. Z. Bai, F. Wang, Dynamical behavior analysis of a two-dimensional discrete predator-prey model with prey refuge and fear factor, J. Appl. Anal. Comput., 10 (2020), 1683–1697. https://doi.org/10.11948/20190426 doi: 10.11948/20190426
![]() |
[39] |
B. H. Hong, C. R. Zhang, Bifurcations and chaotic behavior of a predator-prey model with discrete time, AIMS Math., 8 (2023), 13390–13410. https://doi.org/10.3934/math.2023678 doi: 10.3934/math.2023678
![]() |
[40] |
Y. Huang, Z. Zhu, Z. Li, Modeling the Allee effect and fear effect in predator-prey system incorporating a prey refuge, Adv. Differ. Equ., 321 (2020), 1–13. https://doi.org/10.1186/s13662-020-02727-5 doi: 10.1186/s13662-020-02727-5
![]() |
[41] | A. C. Luo, Regularity and complexity in dynamical systems, New York: Springer, 2012. https://doi.org/10.1007/978-1-4614-1524-4 |
[42] | G. Chen, X. Dong, From chaos to order: Perspectives, methodologies, and applications, Singapore: World Scientificr, 1998. https://doi.org/10.1142/3033 |
[43] |
Q. Din, A. A. Elsadany, S. Ibrahim, Bifurcation analysis and chaos control in a second-order rational difference equation, Int. J. Nonlin. Sci. Num., 19 (2018), 53–68. https://doi.org/10.1515/ijnsns-2017-0077 doi: 10.1515/ijnsns-2017-0077
![]() |
[44] |
X. S. Luo, G. R. Chen, B. H. Wang, J. Q. Fang, Hybrid control of period-doubling bifurcation and chaos in discrete nonlinear dynamical systems, Chaos Soliton. Fract., 181 (2003), 775–783. https://doi.org/10.1016/s0960-0779(03)00028-6 doi: 10.1016/s0960-0779(03)00028-6
![]() |
[45] | T. S. Shores, Applied linear algebra and matrix analysis, Switzerland: Springer Cham, 2018. https://doi.org/10.1007/978-3-319-74748-4 |
[46] | J. Carr, Applications of centre manifold theory, New York: Springer New York, 1982. https://doi.org/10.1007/978-1-4612-5929-9 |
1. | Limei Liu, Xitong Zhong, Analysis and anti-control of period-doubling bifurcation for the one-dimensional discrete system with three parameters and a square term, 2025, 10, 2473-6988, 3227, 10.3934/math.2025150 | |
2. | Pinar Baydemir, Huseyin Merdan, Bifurcation analysis, chaos control, and FAST approach for the complex dynamics of a discrete-time predator–prey system with a weak Allee effect, 2025, 196, 09600779, 116317, 10.1016/j.chaos.2025.116317 |