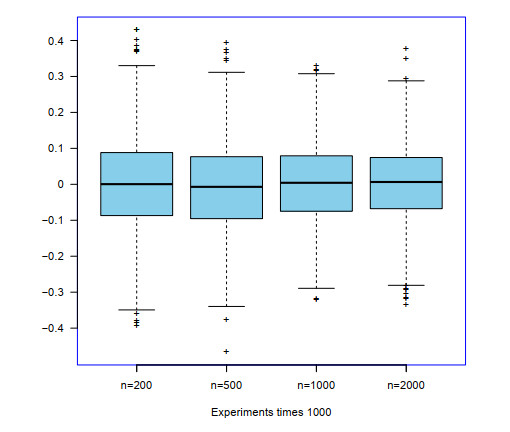
In this work, the law of the single logarithm for randomly weighted sums of widely orthant dependent random variables was studied, which improved and generalized some corresponding results in the literature. As an application, the rate of complete convergence for random weighting estimation with widely orthant dependent samples was obtained under general assumptions. Some numerical analyses were also conducted to confirm the theoretical results.
Citation: Yi Wu, Duoduo Zhao. Law of the single logarithm for randomly weighted sums of dependent sequences and an application[J]. AIMS Mathematics, 2024, 9(4): 10141-10156. doi: 10.3934/math.2024496
[1] | Mingzhou Xu . Complete convergence and complete moment convergence for maximal weighted sums of extended negatively dependent random variables under sub-linear expectations. AIMS Mathematics, 2023, 8(8): 19442-19460. doi: 10.3934/math.2023992 |
[2] | Huiming Zhang, Hengzhen Huang . Concentration for multiplier empirical processes with dependent weights. AIMS Mathematics, 2023, 8(12): 28738-28752. doi: 10.3934/math.20231471 |
[3] | Yongfeng Wu . Limit theorems for negatively superadditive-dependent random variables with infinite or finite means. AIMS Mathematics, 2023, 8(11): 25311-25324. doi: 10.3934/math.20231291 |
[4] | He Dong, Xili Tan, Yong Zhang . Complete convergence and complete integration convergence for weighted sums of arrays of rowwise m-END under sub-linear expectations space. AIMS Mathematics, 2023, 8(3): 6705-6724. doi: 10.3934/math.2023340 |
[5] | Haiwu Huang, Yuan Yuan, Hongguo Zeng . An extension on the rate of complete moment convergence for weighted sums of weakly dependent random variables. AIMS Mathematics, 2023, 8(1): 622-632. doi: 10.3934/math.2023029 |
[6] | Lunyi Liu, Qunying Wu . Complete integral convergence for weighted sums of negatively dependent random variables under sub-linear expectations. AIMS Mathematics, 2023, 8(9): 22319-22337. doi: 10.3934/math.20231138 |
[7] | Chengcheng Jia, Qunying Wu . Complete convergence and complete integral convergence for weighted sums of widely acceptable random variables under the sub-linear expectations. AIMS Mathematics, 2022, 7(5): 8430-8448. doi: 10.3934/math.2022470 |
[8] | Qunying Wu . The convergence rate for the laws of logarithms under sub-linear expectations. AIMS Mathematics, 2023, 8(10): 24786-24801. doi: 10.3934/math.20231264 |
[9] | Shuyan Li, Qunying Wu . Complete integration convergence for arrays of rowwise extended negatively dependent random variables under the sub-linear expectations. AIMS Mathematics, 2021, 6(11): 12166-12181. doi: 10.3934/math.2021706 |
[10] | Mingzhou Xu, Kun Cheng, Wangke Yu . Complete convergence for weighted sums of negatively dependent random variables under sub-linear expectations. AIMS Mathematics, 2022, 7(11): 19998-20019. doi: 10.3934/math.20221094 |
In this work, the law of the single logarithm for randomly weighted sums of widely orthant dependent random variables was studied, which improved and generalized some corresponding results in the literature. As an application, the rate of complete convergence for random weighting estimation with widely orthant dependent samples was obtained under general assumptions. Some numerical analyses were also conducted to confirm the theoretical results.
Let {X,Xn,n≥1} be a sequence of independent and identically distributed (i.i.d., for short) random variables and set Sn=∑ni=1Xi. Hsu and Robbins [1] proved that if EX2<∞ and EX=μ, then for all ϵ>0,
∞∑n=1P(|n−1Sn−μ|>ϵ)<∞. |
The aforementioned result is the well-known complete convergence, which can derive that Sn/n→0 almost surely (a.s., for short) by the Borel-Cantelli lemma. For a sequence {X,Xn,n≥1} of i.i.d. random variables with zero mean, Baum and Katz [2] proved that for all ϵ>0,
∞∑n=1nr−2P(|n∑i=1Xi|>ϵn1/p)<∞ |
if, and only if, E|X|rp<∞, where r>1 and 1≤p<2. For p=2, Lai [3] proved that for any ε>√r−1,
∞∑n=1nr−2P(|n∑i=1Xi|>ε√2nlogn)<∞ |
if EX=0, EX2=1 and E(X2/log|X|)r<∞. This result is called the law of the single logarithm. Chen and Wang [4] showed that the series is divergent if ε<√r−1 and, recently, Chen and Sung [5] proved the sufficiency part for negatively orthant dependent (NOD) random variables by applying a Bernstein type inequality for unbounded NOD random variables.
It is known that many useful linear statistics, such as least squares estimator, nonparametric regression function estimator, and jackknife estimator, are the form of weighted sums, i.e., ∑ni=1aniXi, where ani,1≤i≤n,n≥1 are called the weights and {Xn,n≥1} is a sequence of random variables. Therefore, it is of great interest to extend the law of the single logarithm for partial sums to weighted sums. Bai et al. [6] proved that
lim supn→∞|∑ni=1aniXi|√2nlogn≤√EX2lim supn→∞√∑ni=1a2nina.s., |
where {X,Xn,n≥1} is a sequence of i.i.d. random variables with EX=0, E|X|β<∞, and {ani,1≤i≤n,n≥1} is an array of constants satisfying ∑ni=1|ani|α=O(n) for α>0, β>0, and 1/α+1/β=1/2. Chen and Gan [7] pointed out that the moment condition in Bai et al. [6] is nonoptimal and they proved that the necessary condition is E(|X|/log1/2|X|)β<∞. Sung [8] extended the result of Chen and Gan [7] from classical probability space to Banach space. Chen et al. [9] extended the result of Chen and Gan [7] to a more general case. More recently, Liu et al. [10] also extended the result of Lai [3] for partial sums to weighted sums.
The randomly weighted sums also play an essential role in various theoretical problems and practical applications. For example, in the field of queueing theory, the randomly weighted sum ∑ni=1AniXi represents the total output for a customer being served by n machines, where the Ani is the service time in the i-th machine and Xi is the output from the i-th machine. In a linear-time-invariant system, the convergence analysis of the state observers will reduce to the persistent excitation condition and the randomly weighted sums if the sampling time sequence is a random process; In statistics, the random weighting estimate of sample mean, bootstrap sample mean, and the least squares estimators in a simple linear regression model with random design are all the form of randomly weighted sums. The nonrandomly weighted sums can be regarded as a special case of randomly weighted sums, but not vice versa. The study on randomly weighted sums seems much more meaningful, but what followed is the increase in the difficulty of proofs. In comparison to the study on nonrandomly weighted sums, there are not so many investigations on the law of the single logarithm for randomly weighted sums. Cuzick [11] proved the law of single logarithm
lim supn→∞|∑ni=1AniXi|√2nlogn=1a.s. |
for randomly weighted sums providing that Ani,1≤i≤n,n≥1 is an array of independent Rademacher random variables, and EX2=1. Chen et al. [12] investigated the law of single logarithm in the sense of almost sure convergence for randomly weighted sums of NOD random variables.
In this paper, we will further investigate the law of single logarithm in the sense of complete convergence for randomly weighted sums of widely orthant dependent (WOD) random variables, while the weights are also assumed to be WOD.
Let us recall the concept of WOD random variables, which was raised by Wang et al. [13] as follows.
Definition 1.1. A sequence {Xn,n≥1} of random variables is said to be widely upper orthant dependent (WUOD) if there exists a finite sequence {gU(n),n≥1} of positive numbers such that for each n≥1 and for all xi∈(−∞,∞), 1≤i≤n,
P(X1>x1,X2>x2,⋯,Xn>xn)≤gU(n)n∏i=1P(Xi>xi). |
A sequence {Xn,n≥1} of random variables is said to be widely lower orthant dependent (WLOD) if there exists a finite sequence {gL(n),n≥1} of positive numbers such that for each n≥1 and for all xi∈(−∞,∞), 1≤i≤n,
P(X1≤x1,X2≤x2,⋯,Xn≤xn)≤gL(n)n∏i=1P(Xi≤xi). |
If {Xn,n≥1} is both WUOD and WLOD, then it is said to be WOD with dominating coefficients g(n)=max{gU(n),gL(n)}.
By letting xi→−∞ in the former inequality or xi→∞ in the latter one above, for all 1≤i≤n, it is easy to see that g(n)≥1. Wang et al. [13] gave some examples to show that WOD random variables contain negatively associated (NA) random variables, negatively supper-additive dependent (NSD) random variables, NOD random variables, and extended negatively dependent (END) random variables, the concepts of which were introduced by Alam and Saxena [14], Hu [15], Lehmann [16], and Liu [17], respectively. Wang et al. [13] further presented examples to show that WOD random variables also include some positively dependent random variables. Therefore, it is of interest to investigate the limiting behaviors of random variables processing this dependence structure.
In this paper, the law of the single logarithm for randomly weighted sums of WOD random variables is studied. The result improves and generalizes some corresponding ones in the literature. As an application, the rate of complete convergence for random weighting estimation with WOD samples is obtained under general assumptions. We also carry out some numerical simulations to support the theoretical results.
Throughout the paper, let C be a positive constant, which may vary in different places. Let logx=lnmax(x,e). Define x+=xI(x≥0) and x−=−xI(x<0), where I(A) is the indicator function of event A. an=O(bn) stands for an≤Cbn, and an=o(bn) means an/bn→0 as n→∞, where {an,n≥1} and {bn,n≥1} are two sequences of positive numbers. Let {ξn,n≥1} be a sequence of random variables. If ∑∞n=1P(|ξn|/an>M)<∞ for some M>0, we abbreviate it to |ξn|=Oa.c.(an).
For an array {Ani,1≤i≤n,n≥1} of random variables, denote
ρ=inf{η:∞∑n=1nr+τ1+τ2−1exp{−ηnlogn∑ni=1EA2ni}<∞}. | (2.1) |
It follows that if ∑ni=1E|Ani|2=O(n), then
ρ∈[(r+τ1+τ2)lim infn→∞1nn∑i=1EA2ni,(r+τ1+τ2)lim supn→∞1nn∑i=1EA2ni]⊂[0,∞), |
and
ρ=(r+τ1+τ2)limn→∞1nn∑i=1EA2ni |
if the limit above exists. Particularly, we have ρ=r+τ1+τ2 if Ani≡1 for each 1≤i≤n.
We now state our main result as follows.
Theorem 2.1. Let r>0. Let {X;Xn,n≥1} be a sequence of identically distributed WOD random variables with g1(n)=O(nτ1) for some τ1≥0, and let {Ani,1≤i≤n,n≥1} be an array of WOD random variables with g2(n)=O(nτ2) for some τ2≥0. Suppose that {Ani,1≤i≤n,n≥1} is independent of {X;Xn,n≥1} and
n∑i=1E|Ani|α=O(n) | (2.2) |
for some α≥2(r+1+τ1+τ2). If EX=0 and
{E(|X|log1/2|X|)2(r+1+τ1+τ2)log|X|<∞,for α=2(r+1+τ1+τ2),E(|X|log1/2|X|)2(r+1+τ1+τ2)<∞,for α>2(r+1+τ1+τ2), | (2.3) |
then for any ε>√2ρEX2,
∞∑n=1nr−1P(|n∑i=1AniXi|>4ε√nlogn)<∞. | (2.4) |
Remark 2.1. We point out that if {X;Xn,n≥1} is a sequence of i.i.d. random variables and {Ani,1≤i≤n,n≥1} is an array of independent random variables, then (2.4) can be replaced with
∞∑n=1nr−1P(|n∑i=1AniXi|>ε√nlogn)<∞. |
If we further take EX2=1 and replace {Ani,1≤i≤n,n≥1} with an array {ani,1≤i≤n,n≥1} of constants, Theorem 2.1 degenerates to the convergence part of Theorem 1.1 in Liu et al. [10] when α≥2(r+1). Furthermore, for α=2(r+1), the moment condition E|X|2(r+1)<∞ in Liu et al. [10] is weakened to E(|X|/log1/2|X|)2(r+1)log|X|<∞.
By taking r=1 in Theorem 2.1, we can obtain the following result.
Corollary 2.1. Let {X;Xn,n≥1} be a sequence of identically distributed WOD random variables with g1(n)=O(nτ1) for some τ1≥0, and let {Ani,1≤i≤n,n≥1} be an array of WOD random variables with g2(n)=O(nτ2) for some τ2≥0. Suppose that {Ani,1≤i≤n,n≥1} is independent of {X;Xn,n≥1} and satisfies (2.2) for some α≥2(2+τ1+τ2). If EX=0 and
{E(|X|log1/2|X|)2(2+τ1+τ2)log|X|<∞,for α=2(2+τ1+τ2),E(|X|log1/2|X|)2(2+τ1+τ2)<∞,for α>2(2+τ1+τ2), |
then for any ε>√2ρEX2,
∞∑n=1P(|n∑i=1AniXi|>4ε√nlogn)<∞, |
and thus
lim supn→∞|n∑i=1AniXi|√nlogn≤4√2ρEX2a.s.. |
Remark 2.2. If {X;Xn,n≥1} is a sequence of identically distributed NOD random variables and {Ani,1≤i≤n,n≥1} is an array of NOD random variables, Corollary 2.1 exhibits the same rate as Theorem 2 in Chen et al. [12] for α>4. Under this circumstance, g1(n)=g2(n)=1. Moreover, the case α=4 was not considered in Chen et al. [12]. Note that the complete convergence in Corollary 2.1 is stronger than almost sure convergence in Chen et al. [12]. Therefore, our result improves and extends the corresponding one in Chen et al. [12] from the NOD setting to the WOD setting.
Random weighting is an emerging computational method which pocesses many advantages such as the simplicity in computation, the suitability for large samples, and there is no need to know the distribution function. Assume that X1,X2,⋯,Xn are identically distributed random variables, and the corresponding sample mean is defined as ˉXn=n−1∑ni=1Xi. The random weighting estimation (RWE) of ˉXn can be defined as
Hn=n∑i=1WniXi, |
where Wni's are independent of Xi's and the random vector W=(Wn1,⋯,Wnn) obeys the Dirichlet distribution. In general, (Wn1,⋯,Wnn)∼D(1,⋯,1), namely, ∑ni=1Wni=1 and the joint density of W is fW(wn1,⋯,wnn)=Γ(n), where (Wn1,⋯,Wnn)∈Dn−1 and Dn−1={(Wn1,⋯,Wnn):Wni≥0,i=1,⋯,n−1,∑n−1i=1Wni≤1}. The asymptotic behavior of RWE has been investigated by some authors. Since the RWE was introduced by Zheng [18], some meaningful results have been investigated. For more details, we refer to Gao et al. [19], Xue and Zhu [20], Gao and Zhong [21], Roozegar and Soltani [22], and the references therein.
As an application of our main result, we obtain the rate of complete convergence for the RWE of the WOD sample mean as follows.
Theorem 2.2. Let {X;Xn,n≥1} be a sequence of identically distributed WOD random variables with g1(n)=O(nτ1) for some τ1≥0 and E(|X|log1/2|X|)2(2+τ1)<∞, then
Hn−ˉXn=Oa.c.(√lognn). |
Remark 2.3. For i.i.d. samples, Gao et al. [19] obtained that
P(supn≥N|Hn−ˉXn|>ϵ)→0asN→∞, for any ϵ>0, |
i.e., Hn−ˉXn→0a.s.,asn→∞ under the moment condition E|X|1+θ<∞, where 0<θ<1. The result of Gao et al. [19] does not reveal the convergence rate. Hence, Theorem 2.2 complements and improves the corresponding result of Gao et al. [19] from an independent setting to a WOD setting.
In this subsection, we will carry out some simulations to study the numerical performance of the RWE of the WOD sample mean. For fixed n, we generate Xi,1≤i≤n under the following two cases.
Case 1: WOD sample. The marginal distributions of X1,⋯,Xn are the uniform distribution on [0,1], while (X1,X2), (X3,X4), ⋯, (X2m−1,X2m), ⋯ respectively follow a joint distribution of the Farlie-Gumbel-Morgenstern (FGM) copula
Cθn(u,v)=uv+θnuv(1−u)(1−v),(u,v)∈[0,1]×[0,1] |
with the parameter θn=n−1. It follows from Wang et al. [13] that {Xn,n≥1} is a sequence of identically distributed WOD random variables with the dominating coefficients g(n)=O(n).
Case 2: NA sample. For any fixed n≥3, let (X1,X2,⋯,Xn)∼N(0,Σ), where 0 represents zero vector and
Σ=[1+ν2−ν0⋯000−ν1+ν2−ν⋯0000−ν1+ν2⋯000⋮⋮⋮⋮⋮⋮000⋯1+ν2−ν0000⋯−ν1+ν2−ν000⋯0−ν1+ν2]n×n, |
where 0<ν<1. In this paper, without specified, we will take ν=0.1,0.5,0.8 to show how this parameter will affect the performance. By Joag-Dev and Proschan [23], it can be seen that (X1,X2,⋯,Xn) is an NA vector and is thus WOD.
We carry out a simulation to confirm the consistency for RWE of the WOD sample mean that we established in Theorem 2.2. The random weights satisfy that (Wn1,⋯,Wnn)∼D(1,…,1). For the two cases above, by choosing the sample size n=200, 500, 1000, 2000, respectively, we adopt the R software to compute √n/logn(Hn−ˉXn) for 1000 times and present the boxplots in Figure 1 to Figure 4.
From Figure 1 to Figure 4, we can see that the values of √n/logn(Hn−ˉXn) are bounded for each sample size, no matter if the samples are NA or WOD. Moreover, there is no obvious difference for the RWE of the WOD sample mean with different values of ν. This implies that Hn−ˉXn is convergent with the rate O(√logn/n) completely, which is consistent with what we have proved in Section 2.2.
To prove the main results of the paper, we need the following important lemmas. The first one is a basic property for WOD random variables, which was presented by Wang et al. [24].
Lemma 3.1. Let {Xn,n≥1} be a sequence of WOD random variables. If {fn(⋅),n≥1} are all nondecreasing (or nonincreasing), then {fn(Xn),n≥1} are still WOD.
The following important conclusion for WOD random variables can be found in Wu et al. [25].
Lemma 3.2. Let {Xn,n≥1} be a nonnegative sequence of WOD random variables with the dominating coefficients g1(n), and let {Yn,n≥1} also be a nonnegative sequence of WOD random variables with the dominating coefficients g2(n). Assume that {Xn,n≥1} is independent of {Yn,n≥1}, then {XnYn,n≥1} is still a sequence of WOD random variables with the dominating coefficients g1(n)g2(n).
The next lemma concerning the Bernstein inequality for unbounded WOD random variables was proved in Deng and Wang [26].
Lemma 3.3. Let X1,X2,⋯,Xn be WOD random variables with the dominating coefficients g(n) and EXi=0, E|Xi|s<∞ for each 1≤i≤n, where s≥2. Set B2n=∑ni=1EX2i, then for any ε>0 and δ>0,
P(|n∑i=1Xi|>ε)≤2g(n)exp{−ε2(2+δ)B2n}+Cδ,sg(n)n∑i=1E|Xi|s/εs, |
where Cδ,s>0, depending only on δ and s.
Lemma 3.4. Let r>0, τ≥0, and α≥2(r+1+τ). Let {X;Xn,n≥1} be a sequence of identically distributed random variables and {Ani,1≤i≤n,n≥1} be an array of random variables independent of {X;Xn,n≥1}. If ∑ni=1E|Ani|α=O(n), then
∞∑n=1nr+τ−1n∑i=1P(|AniXi|>√nlogn)≤{CE(|X|log1/2|X|)2(r+1+τ)log|X|,for α=2(r+1+τ),CE(|X|log1/2|X|)2(r+1+τ),for α>2(r+1+τ). |
Proof. It follows from Jensen's inequality and Hölder's inequality that for any 0<α′<α,
n∑i=1E|Ani|α′≤n∑i=1(E|Ani|α)α′/α≤(n∑i=1E|Ani|α)α′/α(n∑i=11)1−α′/α=O(n). | (3.1) |
It is easy to check that
P(|AniXi|>√nlogn)=P(|AniXi|>√nlogn,|Xi|>√nlogn)+P(|AniXi|>√nlogn,|Xi|≤√nlogn)≤P(|Xi|>√nlogn)+(nlogn)−α/2E|Ani|αE|Xi|αI(|Xi|≤√nlogn). | (3.2) |
On one hand, we can obtain by (3.1) and some standard calculation that
∞∑n=1nr+τ−1n∑i=1P(|Xi|>√nlogn)=∞∑n=1nr+τ∞∑j=nP(√jlogj<|X|≤√(j+1)log(j+1))≤C∞∑j=1jr+1+τP(√jlogj<|X|≤√(j+1)log(j+1))≤CE(|X|log1/2|X|)2(r+1+τ). | (3.3) |
On the other hand, we also obtain that
∞∑n=1nr+τ−1(nlogn)−α/2n∑i=1E|Ani|αE|Xi|αI(|Xi|≤√nlogn)≤C∞∑n=1nr+τ−α/2(logn)−α/2n∑j=1E|X|αI(√(j−1)log(j−1)<|X|≤√jlogj)=C∞∑j=1E|X|αI(√(j−1)log(j−1)<|X|≤√jlogj)∞∑n=jnr+τ−α/2(logn)−α/2. |
Hence, if α=2(r+1+τ), we have
∞∑n=1nr+τ−1(nlogn)−α/2n∑i=1E|Ani|αE|Xi|αI(|Xi|≤√nlogn)≤C∞∑j=1(logj)−r−τE|X|αI(√(j−1)log(j−1)<|X|≤√jlogj)≤CE(|X|log1/2|X|)2(r+1+τ)log|X|; | (3.4) |
and if α>2(r+1+τ),
∞∑n=1nr+τ−1(nlogn)−α/2n∑i=1E|Ani|αE|Xi|αI(|Xi|≤√nlogn)≤C∞∑j=1jr+1+τ−α/2(logj)−α/2E|X|αI(√(j−1)log(j−1)<|X|≤√jlogj)≤CE(|X|log1/2|X|)2(r+1+τ). | (3.5) |
A combination of (3.2)–(3.5) derives the desired result immediately. The proof of the lemma is completed.
Lemma 3.5. Let r>0, τ≥0, and α≥2(r+1+τ). Let {X;Xn,n≥1} be a sequence of identically distributed random variables and let {Ani,1≤i≤n,n≥1} be an array of random variables independent of {X;Xn,n≥1}. If ∑ni=1E|Ani|α=O(n), then for any s≥α,
∞∑n=1nr+τ−1(nlogn)−s/2n∑i=1E|AniXi|sI(|AniXi|≤√nlogn)≤{CE(|X|log1/2|X|)2(r+1+τ)log|X|,for α=2(r+1+τ),CE(|X|log1/2|X|)2(r+1+τ),for α>2(r+1+τ). |
Proof. Similar to the procedure of Lemma 3.4, we also have
(nlogn)−s/2E|AniXi|sI(|AniXi|≤√nlogn)=(nlogn)−s/2E|AniXi|sI(|AniXi|≤√nlogn,|Xi|>√nlogn)+(nlogn)−s/2E|AniXi|sI(|AniXi|≤√nlogn,|Xi|≤√nlogn)≤P(|Xi|>√nlogn)+(nlogn)−α/2E|Ani|αE|Xi|αI(|Xi|≤√nlogn), |
which, together with (3.3)–(3.5), completes the proof of the lemma.
Proof of Theorem 2.1. By virtue of EX=0 and the independence of {Ani,1≤i≤n,n≥1} and {X;Xn,n≥1}, we have that
n∑i=1AniXi=n∑i=1(AniXi−EAniXi)=n∑i=1(A+niX+i−EA+niX+i)−n∑i=1(A+niX−i−EA+niX−i)−n∑i=1(A−niX+i−EA−niX+i)+n∑i=1(A−niX−i−EA−niX−i), |
and, hence,
∞∑n=1nr−1P(|n∑i=1AniXi|>4ε√nlogn)≤∞∑n=1nr−1P(|n∑i=1(A+niX+i−EA+niX+i)|>ε√nlogn)+∞∑n=1nr−1P(|n∑i=1(A+niX−i−EA+niX−i)|>ε√nlogn)+∞∑n=1nr−1P(|n∑i=1(A−niX+i−EA−niX+i)|>ε√nlogn)+∞∑n=1nr−1P(|n∑i=1(A−niX−i−EA−niX−i)|>ε√nlogn). |
Therefore, in order to prove (2.4), we only need to show
∞∑n=1nr−1P(|n∑i=1(AniXi−EAniXi)|>ε√nlogn)<∞, | (4.1) |
with Ani≥0 and Xi≥0 for each 1≤i≤n, n≥1. For the given 1≤i≤n, n≥1 define
Yni=AniXiI(AniXi≤√nlogn)+√nlognI(AniXi>√nlogn). |
It follows from Lemmas 3.1 and 3.2 successively that {Yni,1≤i≤n,n≥1} is still an array of row-wise WOD random variables with dominating coefficients g1(n)g2(n)=O(nτ1+τ2). Note by (3.1) that
(nlogn)−1/2|n∑i=1(EYni−EAniXi)|≤(nlogn)−1/2n∑i=1EAniXiI(AniXi>√nlogn)≤(nlogn)−1n∑i=1EA2niEX2≤C(logn)−1→0 as n→∞. | (4.2) |
Moreover, for any ε>√2ρEX2, there exist ε1>√2ρEX2 and ε2>0 such that ε=ε1+ε2. Therefore, by virtue of (4.2), we have that for all n large enough,
|n∑i=1(EYni−EAniXi)|≤ε2√nlogn, |
which implies
∞∑n=1nr−1P(|n∑i=1(AniXi−EAniXi)|>ε√nlogn)≤∞∑n=1nr−1P(max1≤i≤nAniXi>√nlogn)+∞∑n=1nr−1P(|n∑i=1(Yni−EYni)|>ε1√nlogn)=:I1+I2. |
It follows from τ1≥0, τ2≥0, and Lemma 3.4 that
I1≤∞∑n=1nr−1P(n⋃i=1{AniXi>√nlogn})≤∞∑n=1nr+τ1+τ2−1n∑i=1P(|AniXi|>√nlogn)<∞. |
It remains to deal with I2<∞. Since ε1>√2ρEX2, we can take δ>0 sufficiently small such that ϱ=:ε21/[(2+δ)EX2]>ρ. Hence, taking s≥α, we obtain by Lemmas 3.3–3.5 and (2.1) that
I2≤C∞∑n=1nr−1nτ1+τ2exp{−ε21nlogn(2+δ)∑ni=1EY2ni}+C∞∑n=1nr−1nτ1+τ2n∑i=1E|Yni|s(nlogn)s/2≤C∞∑n=1nr+τ1+τ2−1exp{−ε21nlogn(2+δ)EX2∑ni=1EA2ni}+C∞∑n=1nr+τ1+τ2−1(nlogn)−s/2n∑i=1E|AniXi|sI(|AniXi|≤√nlogn)+C∞∑n=1nr+τ1+τ2−1n∑i=1P(|AniXi|>√nlogn)≤C+C∞∑n=1nr+τ1+τ2−1exp{−ϱnlogn∑ni=1EA2ni}<∞. |
The proof is completed.
Proof of Theorem 2.2. We first estimate EWqni, where q>0. Without loss of generality, we only need to estimate EWqn1 for some n large enough. By some standard calculation, we obtain that
EWqn1=Γ(n)∫10wq1dw1∫1−w10dw2⋯∫1−∑n−2i=1wi0dwn−1=Γ(n)∫10wq1dw1∫1−w10dw2⋯∫1−∑n−3i=1wi0(1−n−2∑i=1wi)dwn−2=Γ(n)∫10wq1dw1∫1−w10dw2⋯∫10(1−n−3∑i=1wi)2(1−yn−2)dyn−2(by letting yn−2=wn−21−∑n−3i=1wi)=Γ(n)2!∫10wq1dw1∫1−w10dw2⋯∫1−∑n−4i=1wi0(1−n−3∑i=1wi)2dwn−3=⋯=Γ(n)(n−3)!∫10wq1dw1∫1−w10(1−w1−w2)n−3dw2=Γ(n)(n−3)!∫10wq1dw1∫10(1−w1)n−2(1−y2)n−3dy2(by letting y2=w21−w1)=Γ(n)Γ(1+q)Γ(n+q). |
By Stirling's approximation and the formula above, we have that for each 1≤i≤n, when n is large enough,
EWqni≈Γ(1+q)⋅√2π(n−1)(n−1e)n−1√2π(n+q−1)(n+q−1e)n+q−1≤C(n−1n+q−1)n−1n−q≤Cn−q. | (4.3) |
Note that for any ε>√2ρEX2, where ρ satisfies (2.1) with r=1 and τ2=0,
∞∑n=1P(|√nlogn(Hn−ˉXn)|>8ε)≤∞∑n=1P(|n∑i=1nWni(Xi−EX)|>4ε√nlogn)+∞∑n=1P(|n∑i=1(Xi−EX)|>4ε√nlogn). | (4.4) |
The second term in the righthand of the formula above converges by applying Corollary 2.1 with α>2(2+τ1) and Ani=1 for each 1≤i≤n. On the other hand, it follows from Example 4.3 of Hu [15] that (Wn1,Wn2,⋯,Wnn) are NSD, and hence, WOD with τ2=0. Taking Ani=nWni for each 1≤i≤n,n≥1 in Corollary 2.1, it is easy to obtain by (4.3) that for all α>2(2+τ1),
n∑i=1EAαni=nαn∑i=1EWαni=O(n). |
Therefore, the first series in (4.4) also converges and, thus, (4.4) has been proved to be finite. This completes the proof of the theorem.
In this paper, the law of the single logarithm for randomly weighted sums of widely orthant dependent random variables is investigated. The result improves and generalizes some existing ones. A new result on the rate of complete convergence for random weighting estimation with widely orthant dependent samples is further obtained as an application. Some simulation studies are provided to confirm the theoretical results. In future study, we will further investigate more meaningful outcomes and applications on the topic.
The authors declare they have not used Artificial Intelligence (AI) tools in the creation of this article.
This work was supported by the Outstanding Youth Research Project of Anhui Colleges (2022AH030156).
The authors declare there is no conflict of interest.
[1] |
P. L. Hsu, H. Robbins, Complete convergence and the law of large numbers, P. National Acad. Sci. USA, 33 (1947), 25–31. https://doi.org/10.1073/pnas.33.2.25 doi: 10.1073/pnas.33.2.25
![]() |
[2] |
L. E. Baum, M. Katz, Convergence rates in the law of large numbers, Trans. Amer. Math. Soc., 120 (1965), 108–123. https://doi.org/10.1090/S0002-9947-1965-0198524-1 doi: 10.1090/S0002-9947-1965-0198524-1
![]() |
[3] |
T. L. Lai, Limit theorems for delayed sums, Ann. Probab., 2 (1974), 432–440. https://doi.org/10.1214/aop/1176996658 doi: 10.1214/aop/1176996658
![]() |
[4] |
P. Y. Chen, D. C. Wang, Convergence rates for probabilities of moderate deviations for moving average processes, Acta Math. Sin. English Ser., 24 (2008), 611–622. https://doi.org/10.1007/s10114-007-6062-7 doi: 10.1007/s10114-007-6062-7
![]() |
[5] |
P. Y. Chen, S. H. Sung, A Bernstein type inequality for NOD random variables and applications, J. Math. Inequal., 11 (2017), 455–467. https://doi.org/10.7153/jmi-11-38 doi: 10.7153/jmi-11-38
![]() |
[6] | Z. D. Bai, P. E. Cheng, C. H. Zhang, An extension of the Hardy-Littlewood strong law, Stat. Sinica, 7 (1997), 923–928. |
[7] |
P. Y. Chen, S. X. Gan, Limiting behavior of weighted sums of i.i.d. random variables, Statist. Probab. Lett., 77 (2007), 1589–1599. https://doi.org/10.1016/j.spl.2007.03.038 doi: 10.1016/j.spl.2007.03.038
![]() |
[8] |
S. H. Sung, A law of the single logarithm for weighted sums of i.i.d. random elements, Statist. Probab. Lett., 79 (2009), 1351–1357. https://doi.org/10.1016/j.spl.2009.02.008 doi: 10.1016/j.spl.2009.02.008
![]() |
[9] |
P. Y. Chen, N. N. Kong, S. H. Sung, Complete convergence for weighted sums of i.i.d. random variables with applications in regression estimation and EV model, Commun. Stat. Theor. M., 46 (2017), 3599–3613. https://doi.org/10.1080/03610926.2015.1066817 doi: 10.1080/03610926.2015.1066817
![]() |
[10] |
X. D. Liu, L. H. Zhang, J. X. Zuo, The Lai law for weighted sums, J. Math. Inequal., 16 (2022), 1621–1632. https://doi.org/10.7153/jmi-2022-16-105 doi: 10.7153/jmi-2022-16-105
![]() |
[11] |
J. Cuzick, A strong law for weighted sums of i.i.d. random variables, J. Theor. Probab., 8 (1995), 625–641. https://doi.org/10.1007/BF02218047 doi: 10.1007/BF02218047
![]() |
[12] |
P. Y. Chen, T. Zhang, S. H. Sung, Strong laws for randomly weighted sums of random variables and applications in the bootstrap and random design regression, Stat. Sinica, 29 (2019), 1739–1749. https://doi.org/10.5705/SS.202017.0106 doi: 10.5705/SS.202017.0106
![]() |
[13] |
K. Y. Wang, Y. B. Wang, Q. W. Gao, Uniform asymptotics for the finite-time ruin probability of a new dependent risk model with a constant interest rate, Methodol. Comput. Appl. Probab., 15 (2013), 109–124. https://doi.org/10.1007/s11009-011-9226-y doi: 10.1007/s11009-011-9226-y
![]() |
[14] | K. Alam, K. M. L. Saxena, Positive dependence in multivariate distributions, Commun. Stat. Theor. M., 10 (12), 1183–1196. https://doi.org/10.1080/03610928108828102 |
[15] | T. Z. Hu, Negatively superadditive dependence of random variables with applications, Chinese J. Appl. Probab. Stat., 16 (2000), 133–144. |
[16] | E. L. Lehmann, Some concepts of dependence, Ann. Math. Statist., 37 (1966), 1137–1153. https://doi.org/10.1214/aoms/1177699260 |
[17] |
L. Liu, Precise large deviations for dependent random variables with heavy tails, Stat. Probab. Lett., 79 (2009), 1290–1298. https://doi.org/10.1016/j.spl.2009.02.001 doi: 10.1016/j.spl.2009.02.001
![]() |
[18] |
Z. G. Zheng, Random weighting method for T statistic, Acta Math. Sin., 5 (1989), 87–94. https://doi.org/10.1007/BF02107626 doi: 10.1007/BF02107626
![]() |
[19] |
S. S. Gao, J. M. Zhang, T. Zhou, Law of large numbers for sample mean of random weighting estimate, Inform. Sci., 155 (2003), 151–156. https://doi.org/10.1016/S0020-0255(03)00158-0 doi: 10.1016/S0020-0255(03)00158-0
![]() |
[20] |
L. G. Xue, L. X. Zhu, L1-norm estimation and random weighting method in a semiparametric model, Acta Math. Appl. Sin. English Ser., 21 (2005), 295–302. https://doi.org/10.1007/s10255-005-0237-8 doi: 10.1007/s10255-005-0237-8
![]() |
[21] |
S. S. Gao, Y. M. Zhong, Random weighting estimation of kernel density, J. Stat. Plan. Infer., 140 (2010), 2403–2407. https://doi.org/10.1016/j.jspi.2010.02.009 doi: 10.1016/j.jspi.2010.02.009
![]() |
[22] |
R. Roozegar, A. R. Soltani, On the asymptotic behavior of randomly weighted averages, Stat. Probab. Lett., 96 (2015), 269–272. https://doi.org/10.1016/j.spl.2014.10.003 doi: 10.1016/j.spl.2014.10.003
![]() |
[23] |
K. Joag-Dev, F. Proschan, Negative association of random variables with applications, Ann. Stat., 11 (1983), 286–295. https://doi.org/10.1214/aos/1176346079 doi: 10.1214/aos/1176346079
![]() |
[24] |
X. J. Wang, C. Xu, T. C. Hu, A. Volodin, S. H. Hu, On complete convergence for widely orthant-dependent random variables and its applications in nonparametric regression models, TEST, 23 (2014), 607–629. https://doi.org/10.1007/s11749-014-0365-7 doi: 10.1007/s11749-014-0365-7
![]() |
[25] |
Y. Wu, W. Wang, X. J. Wang, Sufficient and necessary conditions for the strong consistency of LS estimators in simple linear EV regression models, J. Stat. Comput. Simul., 93 (2023), 1244–1262. https://doi.org/10.1080/00949655.2022.2134377 doi: 10.1080/00949655.2022.2134377
![]() |
[26] |
X. Deng, X. J. Wang, An exponential inequality and its application to M estimators in multiple linear models, Stat. Papers, 61 (2020), 1607–1627. https://doi.org/10.1007/s00362-018-0994-0 doi: 10.1007/s00362-018-0994-0
![]() |