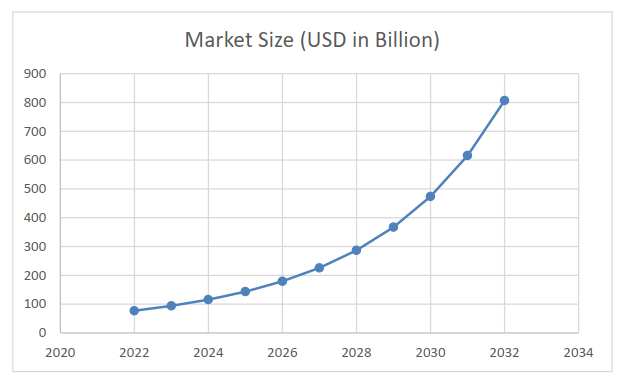
Due to their impact on transportation, Internet of Transportation Things (IoTT) devices have garnered attention recently. Their most notable use is in healthcare, where transportation has been significantly influenced by Internet of Things (IoT) devices. However, threats to infrastructure integrity, medical equipment vulnerabilities, encryption, data integrity threats, and various other security issues make these devices particularly vulnerable. They transmit a considerable amount of sensitive data via sensors and actuators. Given their susceptibility to various attacks, securing the application security of IoTT is crucial. Consequently, IoTT device-based applications must undergo thorough security screening before integration into the healthcare network. Additionally, the authentication technique employed must be robust and reliable. IoTT device evaluation should be impartial and take into account security risk issues. This study proposes an evaluation approach for IoTT devices that utilizes key security risk factors to ensure reliable and secure authentication. Employing hybrid multicriteria decision-making, the suggested strategy evaluates authentication features to select the optimal hospital information system. The hesitant fuzzy analytic hierarchy process-technique for order of preference by similarity to ideal solution (Hesitant Fuzzy AHP-TOPSIS) method is used to systematically examine security risks in a real-time case study with seven alternatives. Results indicate that mediXcel electronic medical records are the most viable, while the Caresoft hospital information system is the least viable, providing valuable insights for future studies and IoTT application professionals. This research addresses security issues to enhance patient data integrity and privacy, facilitating the seamless integration of IoTT applications into healthcare, particularly in emergency healthcare.
Citation: Waeal J. Obidallah. Enhancing healthcare security measures in IoTT applications through a Hesitant Fuzzy-Based integrated approach[J]. AIMS Mathematics, 2024, 9(4): 9020-9048. doi: 10.3934/math.2024439
[1] | Noura Omair Alshehri, Rania Saeed Alghamdi, Noura Awad Al Qarni . Development of novel distance measures for picture hesitant fuzzy sets and their application in medical diagnosis. AIMS Mathematics, 2025, 10(1): 270-288. doi: 10.3934/math.2025013 |
[2] | Attaullah, Shahzaib Ashraf, Noor Rehman, Asghar Khan, Muhammad Naeem, Choonkil Park . Improved VIKOR methodology based on $ q $-rung orthopair hesitant fuzzy rough aggregation information: application in multi expert decision making. AIMS Mathematics, 2022, 7(5): 9524-9548. doi: 10.3934/math.2022530 |
[3] | Wael Alosaimi, Abdullah Alharbi, Hashem Alyami, Bader Alouffi, Ahmed Almulihi, Mohd Nadeem, Rajeev Kumar, Alka Agrawal . Analyzing the impact of quantum computing on IoT security using computational based data analytics techniques. AIMS Mathematics, 2024, 9(3): 7017-7039. doi: 10.3934/math.2024342 |
[4] | Jia-Bao Liu, Rashad Ismail, Muhammad Kamran, Esmail Hassan Abdullatif Al-Sabri, Shahzaib Ashraf, Ismail Naci Cangul . An optimization strategy with SV-neutrosophic quaternion information and probabilistic hesitant fuzzy rough Einstein aggregation operator. AIMS Mathematics, 2023, 8(9): 20612-20653. doi: 10.3934/math.20231051 |
[5] | Misbah Rasheed, ElSayed Tag-Eldin, Nivin A. Ghamry, Muntazim Abbas Hashmi, Muhammad Kamran, Umber Rana . Decision-making algorithm based on Pythagorean fuzzy environment with probabilistic hesitant fuzzy set and Choquet integral. AIMS Mathematics, 2023, 8(5): 12422-12455. doi: 10.3934/math.2023624 |
[6] | Wenyi Zeng, Rong Ma, Deqing Li, Qian Yin, Zeshui Xu, Ahmed Mostafa Khalil . Novel operations of weighted hesitant fuzzy sets and their group decision making application. AIMS Mathematics, 2022, 7(8): 14117-14138. doi: 10.3934/math.2022778 |
[7] | Asit Dey, Tapan Senapati, Madhumangal Pal, Guiyun Chen . A novel approach to hesitant multi-fuzzy soft set based decision-making. AIMS Mathematics, 2020, 5(3): 1985-2008. doi: 10.3934/math.2020132 |
[8] | Wajid Ali, Tanzeela Shaheen, Iftikhar Ul Haq, Hamza Toor, Faraz Akram, Harish Garg, Md. Zia Uddin, Mohammad Mehedi Hassan . Aczel-Alsina-based aggregation operators for intuitionistic hesitant fuzzy set environment and their application to multiple attribute decision-making process. AIMS Mathematics, 2023, 8(8): 18021-18039. doi: 10.3934/math.2023916 |
[9] | Rajagopal Reddy N, Sharief Basha S . A cosine similarity measures between hesitancy fuzzy graphs and its application to decision making. AIMS Mathematics, 2023, 8(5): 11799-11821. doi: 10.3934/math.2023597 |
[10] | Feng Feng, Zhe Wan, José Carlos R. Alcantud, Harish Garg . Three-way decision based on canonical soft sets of hesitant fuzzy sets. AIMS Mathematics, 2022, 7(2): 2061-2083. doi: 10.3934/math.2022118 |
Due to their impact on transportation, Internet of Transportation Things (IoTT) devices have garnered attention recently. Their most notable use is in healthcare, where transportation has been significantly influenced by Internet of Things (IoT) devices. However, threats to infrastructure integrity, medical equipment vulnerabilities, encryption, data integrity threats, and various other security issues make these devices particularly vulnerable. They transmit a considerable amount of sensitive data via sensors and actuators. Given their susceptibility to various attacks, securing the application security of IoTT is crucial. Consequently, IoTT device-based applications must undergo thorough security screening before integration into the healthcare network. Additionally, the authentication technique employed must be robust and reliable. IoTT device evaluation should be impartial and take into account security risk issues. This study proposes an evaluation approach for IoTT devices that utilizes key security risk factors to ensure reliable and secure authentication. Employing hybrid multicriteria decision-making, the suggested strategy evaluates authentication features to select the optimal hospital information system. The hesitant fuzzy analytic hierarchy process-technique for order of preference by similarity to ideal solution (Hesitant Fuzzy AHP-TOPSIS) method is used to systematically examine security risks in a real-time case study with seven alternatives. Results indicate that mediXcel electronic medical records are the most viable, while the Caresoft hospital information system is the least viable, providing valuable insights for future studies and IoTT application professionals. This research addresses security issues to enhance patient data integrity and privacy, facilitating the seamless integration of IoTT applications into healthcare, particularly in emergency healthcare.
In the rapidly evolving landscape of digital transformation, the Internet of Things (IoT) has emerged as a transformative force, reshaping industries and sectors across the globe. One particularly dynamic facet of the IoT is the Internet of Transportation Things (IoTT), which plays an increasingly pivotal role in various domains, including healthcare emergency services [1,2]. IoTT applications have enabled the seamless monitoring of critical assets, real-time tracking of resources, and the efficient management of transportation networks during healthcare emergencies such as the COVID-19 outbreak. However, as the adoption of IoTT applications accelerates, so do the associated security risks. The paramount concern in this rapidly evolving landscape is safeguarding sensitive data and ensuring the integrity of critical services, especially when human lives are at stake [3,4]. Healthcare emergency services, in particular, rely on the uninterrupted functionality of IoTT applications to ensure timely and effective responses to emergencies. Any breach in security could lead to catastrophic consequences.
In 2019, the healthcare sector showed a strong inclination toward embracing IoT technology, with 86% of healthcare organizations incorporating it in various operations, recognizing IoT's potential to enhance healthcare services. The IoT healthcare market projected a worth of $158.1 billion in 2022, driven by a remarkable compound annual growth rate (CAGR) of 28.6% during 2021 [3,4,5]. The surge in data generation, doubling every 73 days on average, underscored the need for robust IoT security measures. Projections indicate that the global IoT healthcare market is expected to reach $534.3 billion by 2025, with a CAGR of 19.9% over the next five years. IoT technology has significantly improved patient care through innovations like EarlySense, FreeStyle Libre, coagulation systems, and disease monitoring devices. However, IoT security became a prominent concern in 2021, with privacy issues and vulnerabilities in IoT devices requiring urgent attention [5,6].
The Internet of Things (IoT) has applications in transportation that go beyond the realm of transportation itself [7,8,9]. These applications include better safety, environmental sustainability, and convenience in mobility [10,11,12,13,14]. For example, a smart car can perform multiple tasks simultaneously, including functions such as navigation, communication, entertainment, and ensuring that transportation is both efficient and reliable. It is now possible for travelers to maintain a continuous connection to all modes of transportation thanks to the Internet of Things (IoT). The automobile is outfitted with a wide variety of wireless connectivity options that allow for access to the internet. These options include Bluetooth, Wi-Fi, 3G, 4G, intelligent traffic systems, and inter-vehicle communication. In the field of logistics, the use of Internet of Things (IoT) technology might be beneficial. One example of this would be the installation of sensors in ambulances, which would allow for continuous monitoring of the patients and prediction of their arrival time in hospital in public healthcare emergency situations [15,16,17,18,19]. Through the optimization of the number of vehicles on the road and the routes that they travel, the Internet of Things (IoT) has the potential to increase savings on both fuel and maintenance costs. These statistics underscore the significant role IoT plays in transportation, healthcare, and various industries, reflecting its growth, challenges, and potential impact on the global economy. Further, Figure 1 shows the future market of IoT in the transportation market [3].
Every country in the world is committed to providing high-quality healthcare emergency services, which is underscored by extensive networks of healthcare facilities [5,6,7]. During emergencies, the rapid and secure transportation of patients and medical resources is of paramount importance, as Figure 2 shows [6]. Assessing security risks in IoTT applications ensures the uninterrupted functioning of healthcare services, enhancing the country's resilience in the face of healthcare crises. Furthermore, many countries place a strong emphasis on data privacy and compliance. The protection of sensitive medical data, in alignment with local regulations, such as the Health Insurance Portability and Accountability Act (HIPAA), is a critical consideration [20,21,22,23,24,25]. A robust security risk assessment ensures that patient data remains confidential and compliant with relevant laws.
The evolving nature of cyber threats requires continuous assessment and adaptation of security measures. Not a single country is completely immune to the evolving tactics of cybercriminals [26,27,28,29]. Conducting security risk assessments allows for the identification and mitigation of emerging threats specific to the IoTT applications used in healthcare emergency services [30]. In addition, efficient resource allocation and management are central to effective healthcare emergency services [31,32,33,34]. Security risks, if not properly managed, can disrupt the optimal utilization of transportation assets and medical resources. An assessment helps in identifying vulnerabilities that may hinder resource optimization.
Public trust is essential in healthcare emergency services. Ensuring the security and reliability of IoTT applications reinforces trust in the healthcare system [35,36,37,38,39]. Every country and its commitment to delivering world-class healthcare services relies, in part, on the trust it instills in its citizens [40,41,42,43]. The security risk assessment of IoTT applications encompasses various Stages, and developers of IoTT applications and software categorize development tasks as either extensive, large, or small applications based on factors such as project team size, duration, and lines of code.
The points of contribution to this research effort are highlighted in the following:
● Our literature review revealed that several academics have proposed a plethora of evaluation approaches to aid in the process of healthcare system security risk assessment. However, no substantial research assessing the risk components of security for IoT applications in transportation could be located. Contrarily, current healthcare assessment methods are designed to evaluate electronic medical records (EMR) and EHR database security.
● Through the presentation of an assessment method that makes use of a hesitant fuzzy AHP TOPSIS methodology, this is the first effort to solve security risk issues that are associated with Internet of Transportation Things applications deployed in the healthcare industry. In this research endeavor, the author's overarching goal is to enhance the security of IoTT applications in the critical domain of healthcare emergency services. In the real world, it is always hard and complicated to deal with knowledge that is not clear. The idea of hesitant fuzzy sets along with AHP and TOPSIS makes it easier to deal with the confusion that comes from hesitating in decisions [41]. By leveraging the synergistic power of hesitant fuzzy AHP-TOPSIS, researcher aims to provide actionable insights and recommendations that empower stakeholders to make informed decisions, fortify security measures, and ultimately ensure the reliability and effectiveness of IoTT applications when they matter most—during healthcare emergencies such as COVID outbreak.
● The security risk attributes that have been taken into consideration in this work have not been identified and assessed before for the sake of security in IoTT applications.
The motivations to conduct this research are mentioned below:
● In addition, secure IoTT applications enable the safe storage of patient data as records and facilitate easy data access while also addressing concerns related to data theft and loss [10,11]. When building IoTT applications, the researcher requires input from multiple teams, and every expert may have a different opinion. Hence, multiple criteria decision-making (MCDM) techniques help establish a unified goal based on multiple expert opinions [12]. In this context, as described in this paper on security risk assessment goals for IoTT applications for healthcare emergency services, the author adopted a hybrid technique involving hesitant fuzzy set theory, AHP, and TOPSIS.
● The hybrid technique of hesitant fuzzy AHP-TOPSIS offers a comprehensive approach to security risk assessment. It allows for a nuanced evaluation of security risks, considering multiple factors and their relative importances. The hybrid technique enables tailored risk mitigation strategies. In the context of IoTT applications for healthcare emergency services, customization is vital, as risks can vary widely based on specific applications and local conditions. Hesitant fuzzy AHP-TOPSIS provides decision-makers with actionable insights [7,10,11].
This research paper is structured as follows: Section 2 reviews related work in security risk assessment in the healthcare sector, explores various security concerns associated with IoTT applications healthcare emergencies, and presents an overview of the hesitant fuzzy AHP-TOPSIS research approach employed. Section 3 provides detailed empirical data analysis and conducts a comparative analysis with conventional approaches. Section 4 discusses this study's conclusions and key insights. Section 5 provides a concise summary of the research's significance in enhancing the security of IoT applications for healthcare emergency services.
In the ever-evolving landscape of IoT applications for transportation within the realm of healthcare emergency services, the paramount concern is ensuring the security and reliability of these systems [12]. Theoretical and empirical efforts are underway to strike a balance between assurance and security and practicality and usability [13,14,15]. This section builds upon and extends the foundation laid by previous studies in this field, offering a comprehensive overview of the research efforts that have paved the way for this investigation into security risk assessment in IoTT applications in healthcare emergency services.
The studies cited herein provide a solid foundation for this work, and the incorporation of hesitant fuzzy AHP and TOPSIS methodologies allows us to systematically assess and rank security risks, advancing the field's understanding and preparedness in this critical domain. Further, Table 1 shows the comparative overviews of the security risk assessment research studies and the applications of hesitant fuzzy AHP and hesitant fuzzy-TOPSIS methodologies.
S. No. | Study | Approach/Methodology | Application Focus | Key Contributions |
1 | Hussain, A., Ali, T., et al. [16] | Knowledge-Based Security | Vulnerability detection in IoTT Applications | Introduces knowledge-based security approach. |
2 | Q. Li [17] | Learning-Based Security | Web-based emergency clinic plans | Addresses functional and security requirements. |
3 | Shahid, J., et al. [18] | Hesitant Fuzzy Multi-Criteria Decision-Making | IoTT applications network | Provides a mathematical framework for risk assessment. |
4 | Rejeb, A., et al. [19] | Mitigate System | Protection hazard assessment | Systematically measures security risks. |
5 | Alsaadi, M. R., et al. [20] | Hazard Assessment Process | Security feature prioritization | Highlights the importance of alignment with development. |
6 | Alqahtani, M. et al. [21 | IoT Digital Risk Assessment | Digital risk assessment for IoT | Identifies gaps in digital risk guidelines. |
7 | Binsawad, M., et al. [22] | Cloud Supply Chain Cyber Risk | CRM application security risk | Quantitative risk assessment supported by survey model. |
8 | L. W. Lee et al. [23] | Hesitant Fuzzy-TOPSIS | Supplier selection for car parts | Offers a clear ranking of suppliers for sourcing decisions. |
9 | Quasim, M. T. [24] | IoT Risk Assessment | Multi-criteria decision making | Challenges and applications of internet of things (IoT) |
10 | Büyüközkan, G., et al. [25] | Hesitant Fuzzy AHP-TOPSIS | Internet finance risk assessment | Identifies and ranks financial risk factors. |
11 | (Cubukcu, C., et al. [26] | Hesitant Fuzzy AHP-TOPSIS | Sustainable energy planning | Integrates AHP, and Hesitant Fuzzy-TOPSIS for energy planning. |
12 | A. Memari, et al. [27] | Hesitant Fuzzy ANP-TOPSIS | Sustainable environmental conflict ranking | Ranks environmental conflicts arising from supplier selection. |
13 | Gündoğdu, F. K., et. al. [28] | ANP, and F-TOPSIS | Technology adoption barriers | Identifies and ranks obstacles to technology adoption. |
After a systematic literature review of the above studies, it has been established that there are very few works in IoT for transportation applications in healthcare emergency services. Hence, an assessment for security risk in IoTT applications for healthcare is an important concern for reaserch. This work therefore focuses on such assessment using a hybird method of MCDM, the hesitant fuzzy AHP TOPSIS method. The next section describes the attributes that affect security risk in emergency healthcare services in IoTT.
Understanding the IoTT is crucial, as it involves the networking of interconnected devices and sensors that play a pivotal role in emergency healthcare transportation delivery. According to Ericsson, the IoT is projected to encompass 22 billion devices by the end of 2022, with experts from Business Insider anticipating a further increase to 30.9 billion by 2025 [3,4,5]. However, this rapid expansion of IoT devices in transportation also widens the potential attack surface for cybersecurity vulnerabilities within healthcare emergency services. In recent years, there has been a sudden and alarming surge in security incidents, including cyberattacks, data breaches, and compromising patients' data confidentiality and integrity. These incidents can be associated with cyberattacks on patients, healthcare workers, and healthcare facilities. The digital threats facing healthcare facilities are not limited to any one country; they are a global concern. Cyberattacks have impacted various healthcare facilities, including in high-income countries like the United States and Saudi Arabia [2,4].
Transportation applications that are based on Internet of Things devices are especially susceptible to network assaults, such as data theft, phishing, spoofing, and denial of service assaults (DDoS) [1,2]. These applications are essential for patient monitoring and logistics during times of emergency. These assaults have the potential to evolve into more severe cybersecurity risks, such as ransomware outbreaks and data breaches, which can impose significant financial and operational difficulties on healthcare institutions. Given these challenges, it becomes imperative to secure IoTT devices and networks in healthcare emergency services against cyberattacks. Measures should include robust encryption, secure communication protocols, and the elimination of default passwords widely known to hackers. Addressing personal information leaks and managing risks associated with automation and artificial intelligence (AI) technologies is vital to prevent unauthorized access and protect critical healthcare data.
Several illustrative cyberattacks underscore the vulnerabilities of IoTT devices within healthcare emergency services [3,4]. For instance, the Mirai botnet executed a massive DDoS attack in 2016 [5], disrupting various online services, demonstrating the potential for similar attacks on healthcare infrastructure. The Verkada hack in 2021 exposed the importance of stringent access control for security camera feeds in healthcare facilities [5,7]. Moreover, the Finland incident illustrated the real-world impact of IoT attacks by disrupting essential services, emphasizing the need for resilience. The Jeep Hack and Stuxnet serve as cautionary tales, illustrating the potential consequences of IoT vulnerabilities in healthcare, from compromised medical devices to espionage [6].
These examples underscore the critical need for robust IoTT security measures tailored to the unique challenges of healthcare emergency services. Protecting against the growing range of threats and vulnerabilities is paramount to ensuring patient safety, data integrity, and the seamless operation of healthcare services in an interconnected world. Worldwide, healthcare institutions are increasingly reliant on their information systems for a wide range of administrative, financial, and medical functions; this trend is causing concern due to the increasing usage of connected medical devices, cloud storage services, and the expanding network infrastructure. To provide security to these IoTT devices, it is imperative to find the security risks associated with these applications. Hence, in the context of IoTT applications, various security risks have been identified, as depicted in Table 2. It is worth noting that each security risk is interconnected with every attribute. In summary, the rising threat landscape in healthcare emergency services, exacerbated by cyberattacks and data breaches, underscores the critical importance of addressing security risks associated with IoTT applications [7,8,9,10,13,14,15]. These risks extend beyond national borders, affecting healthcare facilities worldwide and necessitating comprehensive security measures to protect patients' well-being and data integrity. Following are the definitions for each of the provided categories of security risks [8,9,12,13,14,17,18,19,20,21,22,23,24,25,26,29].
Stage 1 | Stage 2 | Stage 3 |
Transportation (C1) | Physical Infrastructure Risks (C11) | Threats to Infrastructure Integrity (C111) |
Sabotage of Transportation Facilities (C112) | ||
Unauthorized Access to Critical Transportation Locations (C113) | ||
Cybersecurity Threats (C12) | Cyberattacks on Transportation Networks (C121) | |
Data Breaches in Ticketing and Reservation Systems (C122) | ||
Malware Targeting Transportation Infrastructure (C123) | ||
Safety and Compliance Risks (C13) | Non-Compliance with Safety Regulations (C131) | |
Accidents Resulting from Non-Adherence to Safety Protocols (C132) | ||
Failure to Meet Transportation Security Standards (C133) | ||
Healthcare (C2) | Medical Data Privacy Risks (C21) | Unauthorized Access to Patient Medical Records (C211) |
Data Leakage from Medical Devices during Transit (C212) | ||
Non-Compliance with Healthcare Data Privacy Regulations (C213) | ||
Medical Device Security Risks (C22) | Vulnerabilities in Medical Equipment (C221) | |
Unauthorized Access to Medical Devices (C222) | ||
Tampering with Medical Sensors and Monitoring Systems (C223) | ||
Compliance with Healthcare Regulations (C23) | Non-Compliance with HIPAA Regulations (C231) | |
Violations of Medical Transportation Standards (C232) | ||
Legal Consequences of Non-Compliance (C233) | ||
IoT (C3) | IoT Device Vulnerabilities (C31) | Weaknesses in IoT Device Security (C311) |
Unauthorized Access to IoT Devices (C312) | ||
IoT Device Tampering (C313) | ||
Data Security in Transportation IoT (C32) | Data Breaches in Transportation IoT (C321) | |
Data Manipulation in Transit (C322) | ||
Encryption and Data Integrity Risks (C323) | ||
Interconnected Systems Risks (C33) | Cross-Sector Data Leakage (C331) | |
Interference with Healthcare IoT Devices (C332) | ||
Regulatory Challenges in Cross-Sector Integration (C333) |
● Threats to Infrastructure Integrity: Risks associated with physical threats to transportation infrastructure, including damage or harm to the structural components.
● Sabotage of Transportation Facilities: Deliberate actions intended to disrupt or destroy transportation facilities, such as bridges, roads, or railways.
● Unauthorized Access to Critical Transportation Locations: Instances where individuals gain entry to secure transportation areas without proper authorization.
● Cyberattacks on Transportation Networks: Malicious digital actions targeting the information technology systems and networks within transportation.
● Data Breaches in Ticketing and Reservation Systems: Unauthorized access to and exposure of sensitive data related to ticketing and reservation systems.
● Malware Targeting Transportation Infrastructure: Malicious software designed to compromise the security and functionality of transportation infrastructure.
● Non-Compliance with Safety Regulations: Failure to adhere to safety regulations and guidelines in transportation operations.
● Accidents Resulting from Non-Adherence to Safety Protocols: Incidents or mishaps that occur due to a lack of compliance with safety protocols.
● Failure to Meet Transportation Security Standards: Inadequacy in meeting established security standards in the transportation sector.
● Unauthorized Access to Patient Medical Records: Unauthorized viewing or retrieval of patient medical records.
● Data Leakage from Medical Devices during Transit: Unauthorized disclosure or loss of data from medical devices during transportation.
● Non-Compliance with Healthcare Data Privacy Regulations: Failure to adhere to regulations and standards governing the privacy of healthcare data.
● Vulnerabilities in Medical Equipment: Weaknesses or security gaps in medical equipment that could be exploited by malicious actors.
● Unauthorized Access to Medical Devices: Unauthorized entry or interaction with medical devices.
● Tampering with Medical Sensors and Monitoring Systems: Malicious interference or tampering with sensors and monitoring systems used in healthcare.
● Non-Compliance with HIPAA Regulations: Failure to comply with the HIPAA regulations related to patient data privacy.
● Violations of Medical Transportation Standards: Breach of standards and protocols related to the transportation of medical equipment and supplies.
● Legal Consequences of Non-Compliance: Legal and regulatory repercussions resulting from non-compliance with healthcare regulations.
● Weaknesses in IoT Device Security: Inherent security flaws or weaknesses in IoT devices.
● Unauthorized Access to IoT Devices: Unauthorized entry or control of IoT devices.
● IoT Device Tampering: Malicious interference or tampering with IoT devices.
● Data Breaches in Transportation IoT: Unauthorized access and exposure of data within transportation IoT systems.
● Data Manipulation in Transit: Unauthorized alteration or manipulation of data in transit within IoT systems.
● Encryption and Data Integrity Risks: Risks related to the security and integrity of data due to encryption vulnerabilities.
● Cross-Sector Data Leakage: Unauthorized sharing or leakage of data between interconnected systems in different sectors.
● Interference with Healthcare IoT Devices: Deliberate interference or disruption of IoT devices used in healthcare.
● Regulatory Challenges in Cross-Sector Integration: Difficulties and challenges related to integrating IoT systems across different sectors while complying with regulatory requirements.
In the realm of healthcare emergency services, the need to address security risks associated with IoTT applications cannot be overstated.
In this interconnected landscape, compliance with healthcare regulations, such as HIPAA, is not just a legal obligation but a cornerstone for preserving data security and patient privacy during transportation. In sum, addressing these security risks is indispensable for healthcare emergency services to maintain the highest standards of patient care, data integrity, and operational efficiency in an increasingly digital and interconnected world.
Concurrently, the healthcare sector is undergoing profound transformations characterized by dynamic changes, particularly in data collection and security measures [30,31]. As a consequence, security attributes in both the healthcare sector and the design of IoTT applications have undergone enhancements across various dimensions, encompassing aspects such as risk assessment and authorization. Over the past few years, different types of methods have been introduced to assess the security risks associated with IoT devices utilized in the healthcare sector. Still, the increase in cyberattacks on the healthcare sector has given rise to challenges in making decisions concerning the implementation of the most appropriate assessment scheme. Hence, the primary aim of this research methodology is to evaluate and select the most optimal IoTT application or healthcare emergency services. At the outset, characteristics pertaining to security risk were ascertained from scholarly literature and subsequently exhibited during a consultation with panel of experts. In light of the objectives outlined in this paper, the present study introduces hesitant fuzzy AHP-TOPSIS as a comprehensive approach for assessing security risks within IoTT applications. This study delves into the evaluation of security risks based on IoTT applications, considering three, nine, and twenty-seven factors.
Subsequently, the study proceeds to determine the weights associated with these factors, thereby evaluating their influence on IoTT applications through the utilization of hesitant fuzzy-AHP. Following the derivation of these weights, an in-depth assessment of the impact of alternative solutions is conducted employing the hesitant fuzzy-TOPSIS methodology.
In this context, an extensive review of pertinent literature serves as the foundation for identifying various security risks and establishing criteria to scrutinize these risks effectively. Hesitant fuzzy-AHP emerges as the tool of choice for making multi-criteria decisions among alternative solutions. The procedure comprises four crucial steps:
● Creating a security matrix and conducting pairwise comparisons.
● Verification of further assessment for the presence of security attributes post pairwise comparisons.
● Assignment of access privileges post hesitant fuzzy-AHP analysis.
● Aggregation of ratings and subsequent calculation of security risk weights, culminating in their ranking.
The interconnections of different attributes form a hierarchical structure, as illustrated in Table 2. These attributes represent various security risks, with their respective weights determined by assessing their influence on factors and alternative solutions. These rankings are derived in accordance with this methodology employing hesitant fuzzy AHP-TOPSIS. The security risks associated with IoTT application planning frameworks assume a pivotal role as a precise mechanism and an indispensable foundation for prospective research initiatives.
Furthermore, the consistent emergence of decision-making challenges in achieving client objectives and handling sensitive information necessitates the exploration of various approaches and algorithms available in the literature to address these complexities. While multiple methods exist for assessing security risks, hesitant fuzzy-AHP emerges as a particularly suitable technique in comparison to other multi-criteria methods. Hesitant fuzzy sets proposed by V. Torra [41] are a different way to think about fuzzy sets. The goal is to model the uncertainty that comes from not being sure how to give membership degrees to elements in a fuzzy set. However, it is essential to acknowledge that hesitant fuzzy-AHP, while robust, cannot fully mitigate the inherent vagueness and imprecision associated with decision-making processes. Moreover, the hesitant fuzzy-AHP system heavily relies on subjective judgments and carries certain inherent limitations [19,25,26,27]. In order to effectively assess the effects of potential solutions in the IoTT application domain, a novel strategy that combines hesitant fuzzy sets with the AHP-TOPSIS method stands out.
Hesitant Fuzzy-AHP
The majority of problems that occur in the real world require decision-making solutions that include multiple criteria in order to address them and produce an informed choice. From the perspective of the MCDM methodology, the AHP is seen as being well-organized due to the fact that it offers professionals an effective solution [29,30,31,32,33]. The assessment of outcomes in this approach is linked to the notion of paired matrix sets. If there are several potential solutions, the expert judgements will have a major influence on these pair-wise comparisons. An integrated technique consists of two different MCDM approaches, wherein AHP provides the findings linked to prioritization, and TOPSIS evaluates the evaluated results on a variety of selected projects as trials.
Within the scope of this work, the hesitant-fuzzy approach is utilized in order to accomplish more accurate checks. Despite the fact that there are a variety of difficult approaches to the decision-making process for numerous criteria, TOPSIS is considered to be the most effective approach in this league. In order to provide a good framework for it, it takes into consideration optimal, positive and negative results [11]. This kind of circumstance calls for an additional standard for measuring the problems and circumstances that occur in the actual world; the reluctant factor of the technique that was taken provides this additional space to specialists during the measurement process. The most recent developments in hesitant fuzzy set theories have a strong belief in the expansion of complexity [22].
This tentative idea was proposed by V. Torra [41], but it has subsequently been refined by several researchers [24] to discuss membership functions. Research has broadened HFS use. According to K. Sahu [42], TOP-SIS is used in several fields, including cloud computing security. The suggested technique addresses contextual definition ambiguity and fuzziness quickly. Sun et al. [26] compared the results to other multi-factor decision-making processes, using a descriptive account of stock selection to validate the suggested method. They then utilized this to inform their prediction theory model. P. Singh [44,45,46] used automated transportation things for railways transportation. They reviewed recent trends of automation and future works in train transportation system during and after the COVID-19 pandemic.
For the purpose of assessing the ordering of various selected elements in hierarchy, this research offered the hesitant-fuzzy AHP. Subsequently, the hesitant fuzzy TOPSIS approach was utilized in order to test the results of these evaluated considerations in a variety of projects [47,48,49]. How the methodology that was chosen operates is as follows:
The first step is to create a tree-based structure by connecting a number of different aspects that are pertinent.
The second step involves doing pair-wise comparisons between these characteristics with the assistance of the linguistic words that are listed in Table 3. In order for professionals to acquire more precise results, a scale that is far higher has been defined. Within the context of this MCDM procedure, the dependent alternatives are identified.
Rank | Abbreviation | Linguistic Term | Triangular Hesitant-Fuzzy Number |
10 | EHI | Extremely High Importance | (7, 9, 9) |
9 | VHS | Very High Significance | (5, 7, 9) |
8 | RHS | Really High Significance | (3, 5, 7) |
7 | WHI | Weakly High Importance | (1, 3, 5) |
6 | EHI | Equally High Importance | (1, 1, 3) |
5 | EE | Exactly Equal | (1, 1, 1) |
4 | ELI | Equally Low Importance | (0.33, 1, 1) |
3 | WLI | Weakly Low Important | (0.2, 0.33, 1) |
2 | RLS | Really Low Significance | (0.14, 0.2, 0.33) |
1 | VLS | Very Low Significance | (0.11, 0.14, 0.2) |
0 | ELI | Extremely Low Importance | (0.11, 0.11, 0.14) |
The next step is to use hesitant based fuzzy set [24,34] for transformed numerical analyses. Consider C0 as the lowest significance and CK to be the uppermost importance in the given scale of linguistics, and the statistical analyses are among Ci and Cj such that C0≤ Ci ≤ Cj ≤ CK. Calculate weights as given in Eq 1.
OWA(a1,a2,…an)=∑nj=1Wjbj | (1) |
where W=(ω1,ω2,….ωn)Srepresents the weight average as∑ni=1W=1, and bj takes implication equivalency to the maximum of a1,a2,…..an. Now, for assessing T=(a,b,c,d), the following Eqs 2–5 are used:
a=min{aiL,aiM,ai+1M,……ajM,ajR}=aiL | (2) |
d=max{aiL,aiM,ai+1M,……ajM,ajR}=ajR | (3) |
b={aiM,ifi+1=jOWAw2(ajm,…..ai+j2m),ifi+jisevenOWAw2(ajm,…..ai+j+12m),ifi+jisodd} | (4) |
c={ai+1M,ifi+1=jOWAw2(ajmaj−1m,…..a(i+j)2m),ifi+jisevenOWAw2(ajm,aj−1m…..a(i+j+1)2m),ifi+jisodd} | (5) |
In this work, the author employed Eqs 6 and 7 for assessing first and second type weights.
The equation for first type weights is given as
(W1 = (ω11,ω12,……..ω1n)):
ω11=η2,ω12=η2(1−η2),…….ω1nη2(1−η2)n−2 | (6) |
The equation for second type weights is given as
(W2 = (ω21,ω22,……..ω2n)):
ω21=ηn−11,ω22=(1−η1)ηn−11 | (7) |
From the equation η1=κ−(j−1)κ−1s, and η2=κ−(j−1)κ−1where K represents the maximum priority attribute, and i and j represent the lowest and average valued attributes.
Step 4: For calculating the results of ( A), the following Eqs 8 and 9 were employed.
A=[1⋯t1n⋮⋱⋮ tn1…1] | (8) |
tji=(1tiju,1tijm2,1tijm1,1tij1) | (9) |
Step 5: It is now time to defuzzify the evaluated weights, which are represented by Eq 10, d = (l, m1, m2, h), by applying the formulas that are listed below.
μx=l+2m1+2m2+h6 | (10) |
Following that, it is necessary to have an understanding of the consistency ratio by applying the equations that are shown in Eqs 11 and 12:
CI=γmax−nn−1 | (11) |
CRatio=CIRI | (12) |
The coefficient index (CI) represents the ratio of consistency, while the vector is represented by λmax. Additionally, the numerical weights for evaluation are displayed by n. Furthermore, the random number is displayed by RI in the analysis section. At this point, the value of the consistency ratio must be less than 0.1.
Step 6: The following Eq 13 is now used to assess geometric mean.
ri=( ti1⨂ ti2……⨂ tin)1/n | (13) |
Step 7: At this point, it is time to examine the factor weight that has been ranked the highest by applying the algorithm stated in Eq 14.
. ωi= r1⨂( r1⨁ r2……. rn)−1 | (14) |
Step 8: As the eighth phase, the values will now be defuzzified using Eq 15.
μx=l+2m1+2m2+h6 | (15) |
Step 9: All of the weights that have been defuzzified need to be normalized in form using Eq 16.
ωi∑i∑j ωj | (16) |
Utilizing hesitant-fuzzy TOPSIS, the subsequent step is to ascertain which solution is the most suitable. As an approach to MADM that is frequently utilized, TOPSIS enables specialists to determine the most effective answer for problems that occur in the actual world. With their very first proposal, R. M. Rodríguez [43] presented TOPSIS to the world. The findings that are positive are the most significant and effective ones, while the outcomes that are negative are the ones that are the least effective. Hesitant-fuzzy TOPSIS is used to demonstrate the recommended analysis study of security estimations in healthcare perspectives. We prioritize certain components of these standards to do this. TOPSIS uses an envelope algorithm [38,39,40,41,42,43] to calculate distances of H1s and H2s.
env(H1s) = [Cp, Cq], and env(H2s)=[C∗p,C∗q]. The distance is defined as Eq (17):
d(H1s,H2s)=|q∗−q|+|p∗−p| | (17) |
Detailed steps are described as follows:
Step 10: Let us consider that their M experiments are selected as alternatives(T={T1,T2,…..TM})as well as N factors for layer(T={T1,T2,…..TN}).
ex describes the expertise of experts E.
Xl=[HlSij]M×Nis considered as a fuzzy matrix associating hesitant theory, andHlSijportrays the investigational and attribute-based results produced by expertsex.
The different linguistic scale for the TOPSIS methodology [35,36,37] is described as follows:
The scale consists of {nothing, very bad, bad, medium, good, very good, perfect}.
r11 = between medium and good (bt M & G)
r12 = at most medium (am M)
r21 = at least good (al G)
r22 = between very bad and medium (bt VB & M)
The numerical analysis of theory is computed using equations as given in [42]:
envF(EGH (btM & G)) = T (0.33, 0.5, 0.67, 0.83)
envF(EGH (amM)) = T (0, 0, 0.35, 0.67)
envF(EGH (alG)) = T (0.5, 0.85, 1, 1)
envF(EGH (btVB & M)) = T (0, 0.3, 0.37, 0.66)
Step 11: Subordinate the quantitative analysis of results ( X1, X2…… XK), helping the author to portray a matrix (Eq 18) as xij = [Cpij, Cqij].
Cpij=min{minKi=1(maxHxtij),maxKi=1(minHxtij)} |
Cqij=max{minKi=1(maxHxtij),maxKi=1(minHxtij)} | (18) |
Step 12: αb portray the lower effective attributes set as well as αc portray the lowest affective experiments.
Taking into consideration the positive HF set (Eq (19–22)), which is indicated by T+as well as equation is described as T+=( V+1, V+2,… V+n) where V+j=[V+pj,V+qj](j=1,2,3….n)similarly the negative value is displayed as T−and equation is denoted as T−=( V−1, V−2,… V−n) where V−j=[V−pj,V−qj](j=1,2,3….n).
Define V+pj, V+qj, V−pj and V−qj as
V+pj=maxKi=1(maxi(minHxSij))j∈αb |
and minKi=1(mini(minHxSij))j∈αc) (19)
V+qj=maxKi=1(maxi(minHxSij))j∈αb |
and minKi=1(mini(minHxSij))j∈αc) (20)
V−pj=maxKi=1(maxi(minHxSij))j∈αc |
andminKi=1(mini(minHxSij))j∈αb) (21)
V−qj=maxKi=1(maxi(minHxSij))j∈αc |
andminKi=1(mini(minHxSij))j∈αb) (22)
Step 13: The authors adopted the following Eq (23, 24) by explaining ND− and respectively, in order to demonstrate positive and negative examples of research findings.
PD+=[d(x11, V+1)+d(x12, V+2)+…d(x21, V+1)+d(x22, V+2)+…d(xm1, V+1)+d(xm2, V+1)+…+d(x1n, V+n)+d(x21, V+n)+d(xmn, V+n)] | (23) |
ND−=[d(x11, V−1)+d(x12, V−2)+…d(x21, V−1)+d(x22, V−2)+…d(xm1, V−1)+d(xm2, V−1)+…+d(x1n, V−n)+d(x21, V−n)+d(xmn, V−n)] | (24) |
Step 14: Coefficient of Closeness is evaluated by following Eqs 25 and 26.
CS(Ai)=PD+iPD+i+ND−i,i=1,2,….m | (25) |
where
PD+i=∑nj=1d(xij,V+j)and ND−i=∑nj=1d(xij,V−j) | (26) |
Step 15: At the very end of the process, we grouped the options according to the values of their proximities to one another. The real evaluation of the approach is given in the next section.
The hesitant fuzzy AHP-TOPSIS methodology involves assigning weights to security risk factors mentioned in Eqs 1–17, corresponding to various risks listed in Table 2. The hesitant fuzzy-TOPSIS process determines the ranks of these security risks. Once a researcher has obtained both the weights and ranks for these security risks, the researcher assesses the degree of closeness and analyzes whether these results should be applied to IoTT applications across various hospitals. While subjective estimation is suitable for evaluating security risks, quantitatively assessing IoTT applications can be challenging. However, with the assistance of the hesitant fuzzy AHP-TOPSIS method, the researcher can quantitatively evaluate security risks. The security risk factors have been thoroughly discussed at various Stages in the previous sections.
As shown in Table 2, the characteristic of one Stage can influence other properties at a higher Stage, although the impact may not be the same; it can vary. To facilitate the assessment, authors have converted these grouped properties into a hierarchy, as indicated in Table 2. For the sake of clarity in the assessment, security risk factors of IoTT applications at Stage 1 are labeled as C1, C2, and C3. Similarly, other security risks are denoted as discussed in the previous sections. Furthermore, the definitions of all selected security risks have also been provided in the earlier sections. To assess the security risks of IoTT applications for healthcare services, the authors employed Eqs 1–26 of the hesitant fuzzy AHP-TOPSIS method as follows:
With the support of Table 3 and Eqs 1–9, the researcher in this work interpreted textual terms into numerical values and integrated them into triangular fuzzy numeral values. Using Eqs 3–6, crisp estimated standards were converted into triangular fuzzy numbers. The Stage 1 comparative analysis matrices were then computed pair-wise, as shown in Table 4. Subsequently, the consistency index and random index (RI) were calculated using Eqs 10 and 11. The RI value for these pair-wise assessments was below 0.1, indicating the consistency of the matrices in pair-wise comparisons. To defuzzify a matrix of pair-sided measurements at tier two, the formulation provided in Eqs 12–17 was employed, and the results are presented in Table 4. Similar pair-wise matrix comparative assessment matrices were determined for Stage 2 and Stage 3 factors, and systematic findings were extracted from all these respective matrices. A matrix was developed representing criteria (factor) weights relative to their comparables, as shown in Table 5. Attribute rankings based on their weights are also presented in Table 5.
Characteristic A/ Characteristic B | Fuzzy Pair-Wise Comparisons Matrices | Defuzzified Pair-Wise Comparison Matrices |
C1/C2 | 0.6352, 0.9143, 1.3430 | 0.3720 |
C2/C3 | 1.0000, 1.5200, 1.9300 | 0.4561 |
C11/C12 | 0.8206, 1.1118, 1.6150 | 0.3720 |
C12/C13 | 0.6600, 1.1700, 1.6900 | 0.8920 |
C21/C22 | 0.6900, 0.8900, 1.1000 | 0.6910 |
C22/C23 | 0.9710, 1.2475, 1.6094 | 0.3720 |
C31/C32 | 0.3230, 0.4480, 0.6051 | 0.6910 |
C32/C33 | 1.0592, 1.5849, 2.2206 | 0.3720 |
C111/C112 | 1.1500, 1.4400, 1.7000 | 1.1720 |
C112/C113 | 0.3000, 0.4400, 0.8000 | 0.6910 |
C121/C122 | 0.2300, 0.2800, 0.3600 | 0.3720 |
C122/C123 | 0.6600, 1.1700, 1.6900 | 0.6910 |
C131/C132 | 0.6900, 0.8900, 1.1000 | 0.3720 |
C132/C133 | 0.9710, 1.2475, 1.6094 | 0.3720 |
C211/C212 | 0.3230, 0.4480, 0.6051 | 0.6910 |
C212/C213 | 0.9710, 1.2475, 1.6094 | 0.3720 |
C221/C222 | 0.3230, 0.4480, 0.6051 | 1.1720 |
C222/C223 | 1.1500, 1.4400, 1.7000 | 0.6910 |
C231/C232 | 0.3000, 0.4400, 0.8000 | 0.3720 |
C232/C233 | 0.2300, 0.2800, 0.3600 | 1.1720 |
C311/C312 | 0.6600, 1.1700, 1.6900 | 0.6910 |
C312/C313 | 0.6900, 0.8900, 1.1000 | 0.3720 |
C321/C322 | 0.9710, 1.2475, 1.6094 | 0.6910 |
C322/C323 | 0.3230, 0.4480, 0.6051 | 0.3720 |
C331/C332 | 0.9710, 1.2475, 1.6094 | 0.3720 |
C332/C333 | 0.3230, 0.4480, 0.6051 | 0.6910 |
Characteristic | Symbols | Independent Weight of the Groups | Overall Weights through Network | Percentage |
Priority at Stage 1 | ||||
Transportation | C1 | 0.472329 | 0.472329 | 47.23% |
Healthcare | C2 | 0.328972 | 0.328972 | 32.90% |
IoT | C3 | 0.198699 | 0.198699 | 19.87% |
Priority at Stage 2 | ||||
Physical Infrastructure Risks | C11 | 0.178541 | 0.084758 | 8.48% |
Cybersecurity Threats | C12 | 0.311521 | 0.147886 | 14.79% |
Safety and Compliance Risks | C13 | 0.210311 | 0.09984 | 9.98% |
Medical Data Privacy Risks | C21 | 0.166168 | 0.078884 | 7.89% |
Medical Device Security Risks | C22 | 0.300120 | 0.142474 | 14.25% |
Compliance with Healthcare Regulations | C23 | 0.220006 | 0.104442 | 10.45% |
IoT Device Vulnerabilities | C31 | 0.322513 | 0.153105 | 15.31% |
Data Security in Transportation IoT | C32 | 0.177183 | 0.084113 | 8.41% |
Interconnected Systems Risks | C33 | 0.220126 | 0.104499 | 10.45% |
Priority at Stage 3 | ||||
Threats to Infrastructure Integrity | C111 | 0.2200 | 0.02825 | 2.83% |
Sabotage of Transportation Facilities | C112 | 0.3225 | 0.04930 | 4.93% |
Unauthorized Access to Critical Transportation Locations | C113 | 0.1772 | 0.03328 | 3.33% |
Cyberattacks on Transportation Networks | C121 | 0.2201 | 0.02629 | 2.63% |
Data Breaches in Ticketing and Reservation Systems | C122 | 0.1785 | 0.04749 | 4.75% |
Malware Targeting Transportation Infrastructure | C123 | 0.3115 | 0.03481 | 3.48% |
Non-Compliance with Safety Regulations | C131 | 0.2103 | 0.05103 | 5.10% |
Accidents Resulting from Non-Adherence to Safety Protocols | C132 | 0.1662 | 0.02804 | 2.80% |
Failure to Meet Transportation Security Standards | C133 | 0.3001 | 0.03483 | 3.48% |
Unauthorized Access to Patient Medical Records | C211 | 0.2200 | 0.02825 | 2.83% |
Data Leakage from Medical Devices during Transit | C212 | 0.3225 | 0.04930 | 4.93% |
Non-Compliance with Healthcare Data Privacy Regulations | C213 | 0.1772 | 0.03328 | 3.33% |
Vulnerabilities in Medical Equipment | C221 | 0.2201 | 0.02629 | 2.63% |
Unauthorized Access to Medical Devices | C222 | 0.1785 | 0.04749 | 4.75% |
Tampering with Medical Sensors and Monitoring Systems | C223 | 0.3115 | 0.03481 | 3.48% |
Non-Compliance with HIPAA Regulations | C231 | 0.2103 | 0.05103 | 5.10% |
Violations of Medical Transportation Standards | C232 | 0.1662 | 0.02804 | 2.80% |
Legal Consequences of Non-Compliance | C233 | 0.3001 | 0.03483 | 3.48% |
Weaknesses in IoT Device Security | C311 | 0.2200 | 0.02825 | 2.83% |
Unauthorized Access to IoT Devices | C312 | 0.3225 | 0.04930 | 4.93% |
IoT Device Tampering | C313 | 0.1772 | 0.03328 | 3.33% |
Data Breaches in Transportation IoT | C321 | 0.2201 | 0.02629 | 2.63% |
Data Manipulation in Transit | C322 | 0.2200 | 0.04749 | 4.75% |
Encryption and Data Integrity Risks | C323 | 0.3225 | 0.03481 | 3.48% |
Cross-Sector Data Leakage | C331 | 0.1772 | 0.05103 | 5.10% |
Interference with Healthcare IoT Devices | C332 | 0.2201 | 0.02804 | 2.80% |
Regulatory Challenges in Cross-Sector Integration | C333 | 0.1785 | 0.03483 | 3.48% |
After collecting the weights of variables using the hesitant fuzzy-AHP procedure, the TOPSIS system employs these weights as input and provides rankings for each alternative solution. For this research, seven different alternatives representing healthcare software systems were considered. These alternatives were chosen for their specific features and capabilities. They are referred to as follows: mediXcel Electronic Medical Records (EMR) [32], Trio Hospital Information System (HIS) [33], Caresoft HIS [34], GeniPulse [35], LiveHealth for diagnostic [36], Visual Hospital Management [37], and NextGen [38], denoted as IoTTHA1, IoTTHA2, IoTTHA3, IoTTHA4, IoTTHA5, IoTTHA6, and IoTTHA7.
mediXcel EMR, Trio HIS, Caresoft HIS, GeniPulse, LiveHealth (diagnostic), Visual Hospital Management, and NextGen are diverse healthcare software systems that cater to the unique needs of healthcare providers. mediXcel EMR specializes in EMR, digitizing patient data to enhance care delivery efficiency. Trio HIS offers a comprehensive HIS, managing patient records, billing, appointments, and inventory. Caresoft HIS streamlines hospital management tasks, integrating patient data and billing. GeniPulse focuses on EMR and practice management. LiveHealth supports diagnostics by managing samples and results. Visual Hospital Management aids in visualizing and coordinating hospital operations. NextGen provides EMR, practice management, and revenue cycle management solutions to enhance patient care and provider operations.
Hesitant fuzzy AHP-TOPSIS requires performance ratings over standardized variables for each alternative choice. Eqs 18 and 19 and Table 3 are used to standardize the decision matrix, which is developed for m requirements and n alternatives. Each cell in the standardized decision matrix represents the normalized performance value, multiplied by the weights of each set of criteria (Table 6). A fuzzy weighted standardized decision matrix was calculated using Eqs 20–22, and the results are presented in Table 7. The FPIS and FNIS were calculated with Eqs 23. The range of each option's value from the FPIS matrix and FNIS matrix was determined using Eqs 24 and 25. Finally, the parameter's success score was determined using Eq 26, and the ratings of the alternatives were calculated based on the estimated performance ratings, as shown in Table 8 and Figure 3. Figure 3 shows that IoTTHA1 has the highest rating among all alternatives. The alternatives are ranked as follows: IoTTHA1, IoTTHA2, IoTTHA4, IoTTHA7, IoTTHA6, IoTTHA5, and IoTTHA3, respectively.
Factors at Final Stage | IoTTHA1 | IoTTHA2 | IoTTHA3 | IoTTHA4 | IoTTHA5 | IoTTHA6 | IoTTHA7 |
C111 | 0.7642 | 0.7576 | 0.7656 | 0.7642 | 0.7576 | 0.7656 | 0.5333 |
C112 | 0.8832 | 0.9193 | 0.9051 | 0.8832 | 0.9193 | 0.9051 | 0.7336 |
C113 | 0.9172 | 0.9051 | 0.7336 | 0.9172 | 0.9051 | 0.7336 | 0.7571 |
C121 | 0.9681 | 0.6492 | 0.6578 | 0.9681 | 0.6492 | 0.6578 | 0.9191 |
C122 | 0.7642 | 0.7576 | 0.7656 | 0.7642 | 0.7576 | 0.7656 | 0.5333 |
C123 | 0.8832 | 0.9193 | 0.9051 | 0.8832 | 0.9193 | 0.9051 | 0.7336 |
C131 | 0.9172 | 0.9051 | 0.7336 | 0.9172 | 0.9051 | 0.7336 | 0.7571 |
C132 | 0.9193 | 0.9051 | 0.8832 | 0.9193 | 0.9051 | 0.8832 | 0.9193 |
C133 | 0.9051 | 0.7336 | 0.9172 | 0.9051 | 0.7336 | 0.9172 | 0.9051 |
C211 | 0.6492 | 0.6578 | 0.9681 | 0.6492 | 0.6578 | 0.9681 | 0.6492 |
C212 | 0.7576 | 0.7656 | 0.7642 | 0.7576 | 0.7656 | 0.7642 | 0.7576 |
C213 | 0.9193 | 0.9051 | 0.8832 | 0.9193 | 0.9051 | 0.8832 | 0.9193 |
C221 | 0.9051 | 0.9051 | 0.7336 | 0.9172 | 0.9051 | 0.7336 | 0.7571 |
C222 | 0.6492 | 0.6492 | 0.6578 | 0.9681 | 0.6492 | 0.6578 | 0.9191 |
C223 | 0.7576 | 0.7576 | 0.7656 | 0.7642 | 0.7576 | 0.7656 | 0.5333 |
C231 | 0.9193 | 0.9193 | 0.9051 | 0.8832 | 0.9193 | 0.9051 | 0.7336 |
C232 | 0.9051 | 0.9051 | 0.7336 | 0.9172 | 0.9051 | 0.7336 | 0.7571 |
C233 | 0.9051 | 0.7336 | 0.9172 | 0.9051 | 0.7336 | 0.7571 | 0.9193 |
C311 | 0.6492 | 0.6578 | 0.9681 | 0.6492 | 0.6578 | 0.9191 | 0.9051 |
C312 | 0.7576 | 0.7656 | 0.7642 | 0.7576 | 0.7656 | 0.5333 | 0.6492 |
C313 | 0.9193 | 0.9051 | 0.9051 | 0.7336 | 0.9172 | 0.9051 | 0.7336 |
C321 | 0.9051 | 0.7336 | 0.6492 | 0.6578 | 0.9681 | 0.6492 | 0.6578 |
C322 | 0.9051 | 0.8832 | 0.7576 | 0.7656 | 0.7642 | 0.7576 | 0.7656 |
C323 | 0.7336 | 0.9172 | 0.9193 | 0.9051 | 0.8832 | 0.9193 | 0.9051 |
C331 | 0.6578 | 0.9681 | 0.9051 | 0.7336 | 0.9172 | 0.9051 | 0.7336 |
C332 | 0.7656 | 0.7642 | 0.9051 | 0.8832 | 0.9193 | 0.9051 | 0.8832 |
C333 | 0.9051 | 0.8832 | 0.7336 | 0.9172 | 0.9051 | 0.7336 | 0.9172 |
Factors at Final Stage | IoTTHA1 | IoTTHA2 | IoTTHA3 | IoTTHA4 | IoTTHA5 | IoTTHA6 | IoTTHA7 |
C111 | 0.0630 | 0.0230 | 0.0370 | 0.1310 | 0.1220 | 0.1310 | 0.1220 |
C112 | 0.0979 | 0.0370 | 0.1220 | 0.0630 | 0.0230 | 0.0630 | 0.0230 |
C113 | 0.1310 | 0.1220 | 0.0230 | 0.0979 | 0.0370 | 0.0979 | 0.0516 |
C121 | 0.0630 | 0.0370 | 0.0370 | 0.1310 | 0.1220 | 0.1310 | 0.1220 |
C122 | 0.0979 | 0.1220 | 0.1220 | 0.0630 | 0.0230 | 0.0630 | 0.0230 |
C123 | 0.0370 | 0.0230 | 0.0230 | 0.0979 | 0.0370 | 0.0979 | 0.0516 |
C131 | 0.0370 | 0.1220 | 0.0630 | 0.0230 | 0.0630 | 0.0230 | 0.0230 |
C132 | 0.1220 | 0.0230 | 0.0979 | 0.0370 | 0.0979 | 0.0516 | 0.0516 |
C133 | 0.0370 | 0.0370 | 0.1310 | 0.1220 | 0.1310 | 0.1220 | 0.1220 |
C211 | 0.0370 | 0.1220 | 0.0630 | 0.0230 | 0.0630 | 0.0230 | 0.0230 |
C212 | 0.1220 | 0.0230 | 0.0979 | 0.0370 | 0.0979 | 0.0516 | 0.0516 |
C213 | 0.0370 | 0.0370 | 0.1220 | 0.0630 | 0.0230 | 0.0630 | 0.0230 |
C221 | 0.1220 | 0.1220 | 0.0230 | 0.0979 | 0.0370 | 0.0979 | 0.0516 |
C222 | 0.0230 | 0.0370 | 0.0370 | 0.1310 | 0.1220 | 0.1310 | 0.1220 |
C223 | 0.1220 | 0.1220 | 0.1220 | 0.0630 | 0.0230 | 0.0630 | 0.0230 |
C231 | 0.0230 | 0.0230 | 0.0230 | 0.0979 | 0.0370 | 0.0979 | 0.0516 |
C232 | 0.1220 | 0.1220 | 0.1220 | 0.0630 | 0.0230 | 0.0630 | 0.0230 |
C233 | 0.0230 | 0.0230 | 0.0230 | 0.0979 | 0.0370 | 0.0979 | 0.0516 |
C311 | 0.1220 | 0.1220 | 0.0630 | 0.0230 | 0.0630 | 0.0230 | 0.0230 |
C312 | 0.0230 | 0.0230 | 0.0979 | 0.0370 | 0.0979 | 0.0516 | 0.0516 |
C313 | 0.0370 | 0.0370 | 0.1310 | 0.1220 | 0.1310 | 0.1220 | 0.1220 |
C321 | 0.1220 | 0.1220 | 0.0630 | 0.0230 | 0.0630 | 0.0230 | 0.0230 |
C322 | 0.0230 | 0.0230 | 0.0979 | 0.0370 | 0.0979 | 0.0516 | 0.0516 |
C323 | 0.0370 | 0.0370 | 0.1220 | 0.0630 | 0.0230 | 0.0630 | 0.0230 |
C331 | 0.1220 | 0.1220 | 0.0230 | 0.0979 | 0.0370 | 0.0979 | 0.0516 |
C332 | 0.0370 | 0.0370 | 0.0370 | 0.1310 | 0.1220 | 0.1310 | 0.1220 |
C333 | 0.1220 | 0.1220 | 0.1220 | 0.0630 | 0.0230 | 0.0630 | 0.0230 |
S. No. | Alternatives | Closeness Coefficients | Ranks |
1 | IoTTHA1 | 0.599854 | 1 |
2 | IoTTHA2 | 0.556365 | 2 |
3 | IoTTHA3 | 0.485547 | 7 |
4 | IoTTHA4 | 0.552658 | 3 |
5 | IoTTHA5 | 0.512227 | 6 |
6 | IoTTHA6 | 0.521145 | 5 |
7 | IoTTHA7 | 0.545887 | 4 |
To ensure the validity of this findings for each variable, the researcher conducted a rigorous sensitivity analysis, meticulously considering the weights assigned to these variables [25,26,27,28]. In the context of this research focused on IoTT applications for healthcare services, this sensitivity analysis underwent thorough validation through a series of multiple experiments, each targeting a specific factor. The outcome of this comprehensive analysis is presented in Table 9, revealing a diverse spectrum of results. The satisfaction degree, often referred to as closeness coefficient, plays a pivotal role in this analysis. It is meticulously calculated based on the weight assigned to each individual factor, particularly at the final evaluation stage, leveraging the robust hesitant fuzzy AHP-TOPSIS methodology. These intricate calculations and their corresponding results are thoughtfully visualized in Table 9 and Figure 4.
Experiments | Weights/ Alternatives | IoTTHA1 | IoTTHA2 | IoTTHA3 | IoTTHA4 | IoTTHA5 | IoTTHA6 | IoTTHA7 |
Exp-0 | Original Weights | 0.599854 | 0.554515 | 0.485540 | 0.552656 | 0.517587 | 0.521789 | 0.545237 |
Exp-1 | C111 | 0.594154 | 0.555765 | 0.485568 | 0.552656 | 0.512857 | 0.521745 | 0.545997 |
Exp-2 | C112 | 0.54154 | 0.555565 | 0.485582 | 0.552677 | 0.512286 | 0.523564 | 0.545777 |
Exp-3 | C113 | 0.594154 | 0.555565 | 0.485554 | 0.552689 | 0.512285 | 0.521745 | 0.543567 |
Exp-4 | C121 | 0.591254 | 0.557455 | 0.485547 | 0.552698 | 0.512278 | 0.585687 | 0.542367 |
Exp-5 | C122 | 0.51254 | 0.555875 | 0.485583 | 0.552686 | 0.512275 | 0.521778 | 0.542217 |
Exp-6 | C123 | 0.565854 | 0.556365 | 0.485587 | 0.552656 | 0.512285 | 0.528569 | 0.545237 |
Exp-7 | C131 | 0.597454 | 0.557455 | 0.485589 | 0.552658 | 0.512275 | 0.521145 | 0.548757 |
Exp-8 | C132 | 0.555244 | 0.557465 | 0.485589 | 0.552656 | 0.512278 | 0.526587 | 0.548967 |
Exp-9 | C133 | 0.574454 | 0.555665 | 0.485578 | 0.552658 | 0.512296 | 0.521145 | 0.548567 |
Exp-10 | C211 | 0.545214 | 0.554755 | 0.485556 | 0.552685 | 0.512285 | 0.525678 | 0.546597 |
Exp-11 | C212 | 0.544544 | 0.554565 | 0.485574 | 0.552600 | 0.512587 | 0.521458 | 0.548567 |
Exp-12 | C213 | 0.594524 | 0.557465 | 0.485563 | 0.552674 | 0.512279 | 0.525623 | 0.547897 |
Exp-13 | C221 | 0.599854 | 0.554565 | 0.485596 | 0.552641 | 0.512229 | 0.525556 | 0.546587 |
Exp-14 | C222 | 0.54424 | 0.556525 | 0.485582 | 0.552645 | 0.512297 | 0.524477 | 0.545987 |
Exp-15 | C223 | 0.594124 | 0.553255 | 0.485558 | 0.552656 | 0.512291 | 0.528899 | 0.545897 |
Exp-16 | C231 | 0.595214 | 0.553365 | 0.485579 | 0.552658 | 0.512238 | 0.527711 | 0.545787 |
Exp-17 | C232 | 0.51454 | 0.558985 | 0.485578 | 0.552669 | 0.512274 | 0.523355 | 0.545597 |
Exp-18 | C233 | 0.599854 | 0.557895 | 0.485575 | 0.552697 | 0.512282 | 0.526666 | 0.552587 |
Exp-19 | C311 | 0.545344 | 0.555235 | 0.485546 | 0.552689 | 0.512264 | 0.527777 | 0.548987 |
Exp-20 | C312 | 0.594154 | 0.552355 | 0.485548 | 0.552654 | 0.512275 | 0.521758 | 0.548787 |
Exp-21 | C313 | 0.584254 | 0.552545 | 0.485546 | 0.552656 | 0.512237 | 0.521145 | 0.548887 |
Exp-22 | C321 | 0.591254 | 0.554785 | 0.485548 | 0.552653 | 0.512286 | 0.528569 | 0.585678 |
Exp-23 | C322 | 0.592254 | 0.552565 | 0.485777 | 0.552655 | 0.512275 | 0.524257 | 0.545887 |
Exp-24 | C323 | 0.594454 | 0.553255 | 0.485787 | 0.552657 | 0.512253 | 0.525632 | 0.547857 |
Exp-25 | C331 | 0.588854 | 0.552545 | 0.487747 | 0.552685 | 0.512287 | 0.522356 | 0.548967 |
Exp-26 | C332 | 0.595654 | 0.557895 | 0.487457 | 0.552652 | 0.512252 | 0.521254 | 0.547857 |
Exp-27 | C333 | 0.594564 | 0.554755 | 0.484757 | 0.552635 | 0.512256 | 0.522563 | 0.545878 |
In the comprehensive tableau of Table 9, the initial row impeccably captures the original weights, providing a crucial reference point for the subsequent analyses. Simultaneously, Figure 4 offers an insightful visualization of the initial dataset. The initial insights drawn from these original weights reveal that IoTTHA1 consistently exhibits a remarkably high satisfaction degree. However, the essence of this investigation unfolds when the researcher delves into the outcomes of the 28 experiments conducted, ranging from Exp-0 to Exp-27. Throughout this extensive experimentation process, a compelling pattern emerges—Exp-1 steadfastly maintains its position with a high satisfaction degree. In contrast, Exp-3 consistently emerges as the least weighted alternative in each of these experiments. These intriguing variations observed across the spectrum of experiments underscore the paramount importance of the weights assigned to the factors. It becomes evident that alternative rankings are acutely sensitive to the specific weight allocations, shedding light on the intricacies of this analytical framework.
In a comprehensive comparative analysis, it becomes evident that varying methodologies yield distinct data outputs. The reliability and efficiency of any given technique can only be effectively ascertained when subjected to a battery of different methods for validation [19,20,21,22]. In the context of this research, the researcher has employed the hesitant fuzzy AHP-TOPSIS methodology to meticulously assess the efficiency, as well as the degree of closeness or accuracy of the obtained results. It is worth noting that in other methodologies such as fuzzy AHP-TOPSIS [23,24,25], hesitant fuzzy AHP-VIKOR [26,27], and hesitant fuzzy AHP-ELECTRE [31,39], the process of data compilation and estimation remains consistent with that of the hesitant fuzzy AHP-TOPSIS method. These methodologies share a common foundation in terms of data handling and estimation.
However, the key distinction comes to light when the researcher examines the disparities in the results achieved through hesitant fuzzy and conventional AHP-TOPSIS methodologies, as exemplified in Table 10 and Figure 5. Notably, the outcomes generated by the hesitant fuzzy AHP-TOPSIS method exhibit a remarkable degree of consistency and correlation with those obtained through the fuzzy AHP-TOPSIS, hesitant fuzzy AHP-VIKOR, and hesitant fuzzy AHP-ELECTRE methodologies. This high degree of interrelatedness is quantified by a Pearson correlation coefficient of 0.99785. Ultimately, this comparative analysis underscores the enhanced reliability and efficiency of the hesitant fuzzy AHP-TOPSIS methodology. It emerges as the superior approach when juxtaposed with the fuzzy AHP-TOPSIS, hesitant fuzzy AHP-VIKOR, and hesitant fuzzy AHP-ELECTRE techniques. This robust methodology not only demonstrates its effectiveness but also reaffirms its status as the preferred choice for achieving precise and dependable results.
Methods/ Alternatives | IoTTHA1 | IoTTHA2 | IoTTHA3 | IoTTHA4 | IoTTHA5 | IoTTHA6 | IoTTHA7 |
Hesitant Fuzzy-AHP-TOPSIS | 0.599854 | 0.554515 | 0.485540 | 0.552656 | 0.517587 | 0.521789 | 0.545237 |
Fuzzy-AHP-TOPSIS | 0.594124 | 0.553255 | 0.485558 | 0.552656 | 0.512291 | 0.528899 | 0.545897 |
Hesitant Fuzzy AHP-VIKOR | 0.588854 | 0.552545 | 0.487747 | 0.552685 | 0.512287 | 0.522356 | 0.548967 |
Hesitant Fuzzy AHP-ELECTRE | 0.594124 | 0.553255 | 0.485558 | 0.552656 | 0.512291 | 0.528899 | 0.545897 |
Hesitant fuzzy AHP-TOPSIS is a crucial security risk assessment tool for web-based healthcare applications. This study investigated local hospitals to prove the developer's framework's importance in IoTT application security. The developer framework is more important when security concerns shift to sustainable and secure website design. After investigating these issues, this research produced a hierarchical framework that identifies key components in a hospital's sustainable security plan within the developer framework.
A smart city represents the most commonly covered area of the Internet of Things (IoT). In these smart cities, blockchain technology is utilized to enhance real-time data sharing, electricity trading, and other related activities. Furthermore, it has been demonstrated that, despite the numerous advantages offered by blockchain technology in the healthcare industry, authors often employ it for managing medicine supply chains and data management. This is done to prevent counterfeiting and to empower patients regarding their data, respectively [47,48,49]. As IoTT applications gain increasing importance, their usage and complexity continue to escalate. The exponential growth in security assessments emphasizes the need for a digital hospital developer framework that prioritizes robust security and effective sustainability.
The evaluation and assessment of security risks are pivotal in achieving sustainable security. This research seamlessly integrates security and sustainability factors, systematically evaluating sustainable security. The outcomes of this study will facilitate developers in seamlessly integrating sustainable security into the development life cycle of IoTT applications. This research engaged in the scrutiny of diverse IoTT applications across different hospital settings, extracting insights from experts concerning the causative factors related to sustainability, design, and security of specific IoTT applications. This expert-derived data was meticulously analyzed using the hesitant fuzzy AHP-TOPSIS methodology.
This comprehensive research framework is centered around the development and evaluation of secure IoTT applications. The paper expounds upon security factors and their alternatives, drawing insights from case studies conducted in various hospitals employing different hospital management system applications. This research is poised to guide engineers in enhancing IoTT application development across their life cycle.
While numerous security assessment models and techniques exist in the literature for independently evaluating security, models or techniques seamlessly incorporating security into the hesitant fuzzy-AHP approach remain relatively scarce. Within this work, the researcher scrutinized 27 core security risks and seven alternatives pertaining to web-based applications. These alternatives were meticulously selected following consultations with experts and the aggregation of their opinions regarding risk planning, mitigation and security attributes specific to web-based applications.
The salient findings of this work can be distilled as follows:
● Quantitative results obtained through hesitant fuzzy AHP-TOPSIS will aid professionals in categorizing the highest-ranked components of an electronic hospital management system.
● The hesitant fuzzy-AHP method assigns weights to risk attributes, while hesitant fuzzy-TOPSIS provides rankings for these attributes.
● A comparative analysis of hesitant fuzzy AHP-TOPSIS with conventional AHP-TOPSIS underlines the superiority of the former methodologies.
● Sensitivity analysis quantifies the satisfaction degree for IoTT applications.
● Prioritizing the web-based hospital management system is imperative for both future research and ongoing endeavors aimed at optimizing IoTT application efficiency. This evaluation will provide engineers with invaluable insights into the security framework.
● Recommendations for enhancements can be extrapolated from this assessment to guide engineers in refining security structures, leveraging highly structured components. However, it is essential to acknowledge certain limitations:
● The data collected for web design, while significant, remains limited in scope. Results may exhibit variations with a larger dataset.
● There may exist additional security design factors beyond those identified within this work.
This paper has conducted an exhaustive examination of security risks affecting IoTT applications, particularly within the healthcare sector. Furthermore, it has explored various security countermeasures for these risks. To mitigate the risk of data breaches in IoTT applications, this study conducted a risk assessment and harnessed the innovative AHP-TOPSIS methodology to craft a bespoke technique tailored to the unique healthcare industry requirements. In addition, there are a few restrictions that apply to this study. These restrictions are as follows:
● Due to the study's limitation to the collected data, there may be additional factors related to healthcare transportation services that could not be included in the investigation.
● The AHP-TOPSIS methodology is employed to assign distinct security risk factors for evaluation. Furthermore, this research article presents rankings and weights for various security variables.
In addition, this study has the potential to be expanded in a number of different areas, including the following:
● Authors can conduct a series of interviews with a diverse group of healthcare drivers, such as ambulance drivers of different genders, ages, nationalities, and so on. This approach aims to gain a better understanding of all the roles and duties that drivers are expected to perform to ensure the success and security of healthcare operations.
● Identify potential alternatives for the new designs of safe and risk-free transportation. Subsequently, model those possibilities to address the impending issues posed by the pandemic.
● Develop a comprehensive cost-benefit model that can be used to account for the initial investment and operational expenditures associated with the deployment of healthcare technology. Additionally, consider the benefits that could be obtained.
Thus, the rigorously validated and highly conclusive results emanating from this study will provide software and IoTT application developers with invaluable insights when embarking on web-based application development.
The authors declare they have not used artificial intelligence (AI) tools in the creation of this article.
The authors extend their appreciation to the Deanship of Scientific Research, Imam Mohammad Ibn Saud Islamic University (IMSIU), Saudi Arabia, for funding this research work through Grant No. (221409015).
The author declares no conflict of interest.
[1] | Cyber Management Alliance, IoT Security: 5 Cyber Attacks Caused by IoT Security Vulnerabilities, 2022. Available from: https://www.cm-alliance.com/cybersecurity-blog/iot-security-5-cyber-attacks-caused-by-iot-security-vulnerabilities |
[2] | Developing National Information Security Strategy for the Kingdom of Saudi Arabia, National Information Security Strategy of Saudi Arabia, 2024. Available from: https://www.itu.int/en/ITU-D/Cybersecurity/Documents/National_Strategies_Repository/SaudiArabia_NISS_Draft_7_EN.pdf |
[3] | Saudi Arabia Internet of Things (IoT) Market, Research and Markets, 2022. Available from: https://www.researchandmarkets.com/reports/5562151/saudi-arabia-internet-of-things-iot-market-by |
[4] | Saudi Arabia-Country Commercial Guide, U.S. Department of Commerce, 2024. Available from: https://www.trade.gov/country-commercial-guides/saudi-arabia-information-and-communications-technology |
[5] | Healthcare, Unified National Platform, Government of Saudi Arabia, 2024. Available from: https://www.my.gov.sa/wps/portal/snp/aboutksa/HealthCareInKSA/?lang = en |
[6] | S. T. U. Shah, H. Yar, I. Khan, M. Ikram, H. Khan, Internet of things-based healthcare: recent advances and challenges, in Applications of Intelligent Technologies in Healthcare, (2019), 153–162. https://doi.org/10.1007/978-3-319-96139-2_15 |
[7] | Internet of Things, IoT Demand in Saudi Arabia, A Survey-Based Study, Communications and Information Technology Commission, 2021. IoT Demand in Saudi Arabia. Available from: https://www.cst.gov.sa/en/researchs-studies/research-innovation/Documents/CITC-IoT_Demand.pdf |
[8] | Cyber Security Framework, Saudi Arabian Monetary Authority, Riyadh, Saudi Arabia, 2017. Available from: https://www.sama.gov.sa/en-US/Laws/FinanceRules/SAMA%20Cyber%20Security%20Framework%20v1.0%20final_updated.pdf |
[9] |
X. Huang, S. Nazir, Evaluating security of internet of medical things using the analytic network process method, Secur. Commun. Netw., 2020, 1–14. https://doi.org/10.1155/2020/8829595 doi: 10.1155/2020/8829595
![]() |
[10] |
K. Kim, I. M. Alshenaifi, S. Ramachandran, J. Kim, T. Zia, A. Almorjan, Cybersecurity and cyber forensics for smart cities: A comprehensive literature review and survey, Sensors, 23 (22023), 3681. https://doi.org/10.3390/s23073681 doi: 10.3390/s23073681
![]() |
[11] |
S. Nasiri, F. Sadoughi, M. H. Tadayon, A. Dehnad, Security requirements of Internet of Things-based healthcare system: A survey study, Acta Informatica Medica, 27 (2019), 253. https://doi.org/10.5455/aim.2019.27.253-258 doi: 10.5455/aim.2019.27.253-258
![]() |
[12] | M. R. Mokhtar, M. P. Abdullah, M. Y. Hassan, F. Hussin, Combination of AHP-PROMETHEE and TOPSIS for selecting the best demand side management (DSM) options, In IEEE Student Conference on Research and Development (SCOReD), (2015), 367–372. https://doi.org/10.1109/SCORED.2015.7449357 |
[13] |
A. Salamai, O. K. Hussain, M. Saberi, E. Chang, F. K. Hussain, Highlighting the Importance of Considering the Impacts of Both External and Internal Risk Factors on Operational Parameters to Improve Supply Chain Risk Management, IEEE Access, 7 (2019), 49297–49315. https://doi.org/10.1109/ACCESS.2019.2902191 doi: 10.1109/ACCESS.2019.2902191
![]() |
[14] |
P. Radanliev, D. C. D. Roure, R. Nicolescu, M. Huth, R. M. Montalvo, S. Cannady, et al., Future developments in cyber risk assessment for the Internet of Things, Comput. Ind., 102 (2018), 14–22. https://doi.org/10.1016/j.compind.2018.08.002 doi: 10.1016/j.compind.2018.08.002
![]() |
[15] |
M. Humayun, N. Z. Jhanjhi, A. Almotilag, Real-time security health and privacy monitoring for Saudi highways using cutting-edge technologies, Appl. Sci., 12 (2022), 1–19. https://doi.org/10.3390/app12042177 doi: 10.3390/app12042177
![]() |
[16] |
A. Hussain, T. Ali, F. Althobiani, U. Draz, M. Irfan, S. Yasin, et al., Security framework for IoT-based real-time health applications, Electronics, 10 (2021), 1–21. https://doi.org/10.3390/electronics10060719 doi: 10.3390/electronics10060719
![]() |
[17] | Q. Li, An improved fuzzy AHP approach to evaluating conductor joint alternatives, In Seventh International Conference on Fuzzy Systems and Knowledge Discovery, (2010), 811–814. https://doi.org/10.1109/FSKD.2010.5569216 |
[18] |
J. Shahid, R. Ahmad, A. K. Kiani, T. Ahmad, S. Saeed, A. M. Almuhaideb, Data protection and privacy of the Internet of Healthcare Things (IoHTs), Appl. Sci., 12 (2022), 1–18. https://doi.org/10.3390/app12041927 doi: 10.3390/app12041927
![]() |
[19] |
A. Rejeb, K. Rejeb, H. Treiblmaier, A. Appolloni, S. Alghamdi, Y. Alhasawi, et al., The Internet of Things (IoT) in healthcare: Taking stock and moving forward, Internet Things, (2023), 1–20. https://doi.org/10.1016/j.iot.2023.100721 doi: 10.1016/j.iot.2023.100721
![]() |
[20] |
M. R. Alsaadi, S. Z. Ahmad, A quality function deployment strategy for improving mobile-government service quality in the Gulf Cooperation Council countries, Benchmarking, 25 (2018), 3276–3295. https://doi.org/10.1108/BIJ-12-2017-0333 doi: 10.1108/BIJ-12-2017-0333
![]() |
[21] | M. Alqahtani, IoT within the Saudi healthcare industry during Covid-19, In Proceedings of International Conference on Emerging Technologies and Intelligent Systems: ICETIS 2021, 1 M. (2022), 469–483. https://doi.org/10.1007/978-3-030-82616-1_40 |
[22] |
M. Binsawad, M. Albahar, A technology survey on IoT applications serving umrah and hajj, Appl. Comput. Intell. Soft Comput., (2022) 1–15. https://doi.org/10.1155/2022/1919152 doi: 10.1155/2022/1919152
![]() |
[23] |
L. Lee, S. Chen, Fuzzy multiple attributes group decision-making based on the extension of TOPSIS method and interval type-2 fuzzy sets, In International Conference on Machine Learning and Cybernetics, 8 (2008), 3260–3265. https://doi.org/10.1109/ICMLC.2008.4620968 doi: 10.1109/ICMLC.2008.4620968
![]() |
[24] | M. T. Quasim, Challenges and applications of Internet of Things (IoT) in Saudi Arabia, (2021). Available from: https://easychair.org/publications/preprint_download/r2W4 |
[25] |
G. Büyüközkan, G. Çifçi, A combined fuzzy AHP and fuzzy TOPSIS based strategic analysis of electronic service quality in the healthcare industry, Expert Syst. Appl., 39 (2015), 2341–2354. https://doi.org/10.1016/j.eswa.2011.08.061 doi: 10.1016/j.eswa.2011.08.061
![]() |
[26] |
C. Cubukcu, C. Cantekin, Using a combined fuzzy-AHP and TOPSIS decision model for selecting the best firewall alternative, J. Fuzzy Extension Appl., 3 (2022), 192–200. https://doi.org/10.22105/jfea.2021.313606.1167 doi: 10.22105/jfea.2021.313606.1167
![]() |
[27] |
A. Memari, A. Dargi, M. R. A. Jokar, R. Ahmad, A. Rahman, Sustainable supplier selection: A multi-criteria intuitionistic fuzzy TOPSIS method, J. Manuf. Syst., 50 (2019), 9–24. https://doi.org/10.1016/j.jmsy.2018.11.002 doi: 10.1016/j.jmsy.2018.11.002
![]() |
[28] |
F. K. Gündoğdu, S. Duleba, S. Moslem, S. Aydın, Evaluating public transport service quality using picture fuzzy analytic hierarchy process and linear assignment model, Appl. Soft Comput., 100 (2021), 106920. https://doi.org/10.1016/j.asoc.2020.106920 doi: 10.1016/j.asoc.2020.106920
![]() |
[29] |
A. S. Anvari, The applications of MCDM methods in COVID-19 pandemic: A state of the art review, Appl. Soft Comput., (2022), 109238. https://doi.org/10.1016/j.asoc.2022.109238 doi: 10.1016/j.asoc.2022.109238
![]() |
[30] | S. Chen, S. Cheng, T. Lan, A new multicriteria decision making method based on the TOPSIS method and similarity measures between intuitionistic fuzzy sets, In International Conference on Machine Learning and Cybernetics (ICMLC), Jeju, (2016), 692–696. https://doi.org/10.1109/ICMLC.2016.7872972 |
[31] |
O. Dogan, M. Deveci, F. Canıtez, A corridor selection for locating autonomous vehicles using an interval-valued intuitionistic fuzzy AHP and TOPSIS method, Soft Comput., 24 (2020), 8937–8953. https://doi.org/10.1007/s00500-019-04421-5 doi: 10.1007/s00500-019-04421-5
![]() |
[32] | Medixcel, 2024. Available from: https://www.medixcel.in/ |
[33] | TRIO Corporation, 2024. Available from: https://triocorporation.in/ |
[34] | CareSoft, 2024. Available from: https://caresoft.co.in/ |
[35] | Genipulse, 2024. Available from: http://www.genipulse.com/ |
[36] | LiveHealth, 2024. Available from: https://livehealth.solutions/ |
[37] | Visual Infosoft, 2024. Available from: https://www.visualinfosoft.com/ |
[38] | NextGen, 2024. Available from: https://www.nextgen.com/ |
[39] |
T. Kaya, C. Kahraman, An integrated fuzzy AHP–ELECTRE methodology for environmental impact assessment, Expert Syst. Appl., 38 (2011), 8553–8562. https://doi.org/10.1016/j.eswa.2011.01.057 doi: 10.1016/j.eswa.2011.01.057
![]() |
[40] | Saudi Arabia Internet of Things (IoT) Market, TechSci Research, 2023. Available from: https://www.techsciresearch.com/report/saudi-arabia-internet-of-things-iot-market/7663.html |
[41] |
V. Torra, Y. Narukawa, On hesitant fuzzy sets and decision, In Proc. IEEE Int. Conf. Fuzzy Syst., (2009), 1378–1382. https://doi.org/10.1109/FUZZY.2009.5276884 doi: 10.1109/FUZZY.2009.5276884
![]() |
[42] |
K. Sahu, R. K. Srivastava, S. Kumar, M. Saxena, B. K. Gupta, Integrated hesitant fuzzy-based decision-making framework for evaluating sustainable and renewable energy, Int. J. Data Sci. Anal., 16 (2023), 371–390. https://doi.org/10.1007/s41060-023-00426-4 doi: 10.1007/s41060-023-00426-4
![]() |
[43] |
R. M. Rodríguez, L. Martínez, V. Torra, Z. S. Xu, F. Herrera, Hesitant fuzzy sets: State of the art and future directions, Int. J. Intell. Syst., 29 (2014), 495–524. https://doi.org/10.1002/int.21654 doi: 10.1002/int.21654
![]() |
[44] |
P. Singh, Z. Elmi, V. K. Meriga, Internet of Things for sustainable railway transportation: Past, present, and future, Cleaner Logistics Supply Chain, 4 (2022), 100065. https://doi.org/10.1016/j.clscn.2022.100065 doi: 10.1016/j.clscn.2022.100065
![]() |
[45] |
P. Singh, Z. Elmi, Blockchain and AI technology convergence: Applications in transportation systems, Veh. Commun., 100521 (2022). https://doi.org/10.1016/j.vehcom.2022.100521 doi: 10.1016/j.vehcom.2022.100521
![]() |
[46] | P. Singh, M. A. Dulebenets, Deployment of autonomous trains in rail transportation: Current trends and existing challenges, IEEE Access, 9 (2021), 91427–91461. 10.1109/ACCESS.2021.3091550 |
[47] |
E. M. Adere, Blockchain in healthcare and IoT: A systematic literature review, Array, 14 (2022), 100139. https://doi.org/10.1016/j.array.2022.100139 doi: 10.1016/j.array.2022.100139
![]() |
[48] |
T. Wang, H. Hua, Challenges of blockchain in new generation energy systems and future outlooks, Int. J. Elec. Power, 135 (2021), 107499. https://doi.org/10.1016/j.ijepes.2021.107499 doi: 10.1016/j.ijepes.2021.107499
![]() |
[49] |
M. S. Sangari, A. Mashatan, A data-driven, comparative review of the academic literature and news media on blockchain-enabled supply chain management: Trends, gaps, and research needs, Comput. Ind., 143 (2022), 103769. https://doi.org/10.1016/j.compind.2022.103769 doi: 10.1016/j.compind.2022.103769
![]() |
1. | Habib Ullah Khan, Yasir Ali, Modeling security evaluation framework for IoHT-driven systems using integrated decision-making methodology, 2024, 14, 2045-2322, 10.1038/s41598-024-62066-3 | |
2. | Shouhuai Xu, Sandeep Pirbhulal, Habtamu Abie, 2025, Chapter 1, 978-3-031-85557-3, 3, 10.1007/978-3-031-85558-0_1 | |
3. | Merve Güler, Gülçin Büyüközkan, Cybersecurity maturity assessment using an incomplete hesitant fuzzy AHP method and Bonferroni means operator, 2025, 09574174, 127268, 10.1016/j.eswa.2025.127268 |
S. No. | Study | Approach/Methodology | Application Focus | Key Contributions |
1 | Hussain, A., Ali, T., et al. [16] | Knowledge-Based Security | Vulnerability detection in IoTT Applications | Introduces knowledge-based security approach. |
2 | Q. Li [17] | Learning-Based Security | Web-based emergency clinic plans | Addresses functional and security requirements. |
3 | Shahid, J., et al. [18] | Hesitant Fuzzy Multi-Criteria Decision-Making | IoTT applications network | Provides a mathematical framework for risk assessment. |
4 | Rejeb, A., et al. [19] | Mitigate System | Protection hazard assessment | Systematically measures security risks. |
5 | Alsaadi, M. R., et al. [20] | Hazard Assessment Process | Security feature prioritization | Highlights the importance of alignment with development. |
6 | Alqahtani, M. et al. [21 | IoT Digital Risk Assessment | Digital risk assessment for IoT | Identifies gaps in digital risk guidelines. |
7 | Binsawad, M., et al. [22] | Cloud Supply Chain Cyber Risk | CRM application security risk | Quantitative risk assessment supported by survey model. |
8 | L. W. Lee et al. [23] | Hesitant Fuzzy-TOPSIS | Supplier selection for car parts | Offers a clear ranking of suppliers for sourcing decisions. |
9 | Quasim, M. T. [24] | IoT Risk Assessment | Multi-criteria decision making | Challenges and applications of internet of things (IoT) |
10 | Büyüközkan, G., et al. [25] | Hesitant Fuzzy AHP-TOPSIS | Internet finance risk assessment | Identifies and ranks financial risk factors. |
11 | (Cubukcu, C., et al. [26] | Hesitant Fuzzy AHP-TOPSIS | Sustainable energy planning | Integrates AHP, and Hesitant Fuzzy-TOPSIS for energy planning. |
12 | A. Memari, et al. [27] | Hesitant Fuzzy ANP-TOPSIS | Sustainable environmental conflict ranking | Ranks environmental conflicts arising from supplier selection. |
13 | Gündoğdu, F. K., et. al. [28] | ANP, and F-TOPSIS | Technology adoption barriers | Identifies and ranks obstacles to technology adoption. |
Stage 1 | Stage 2 | Stage 3 |
Transportation (C1) | Physical Infrastructure Risks (C11) | Threats to Infrastructure Integrity (C111) |
Sabotage of Transportation Facilities (C112) | ||
Unauthorized Access to Critical Transportation Locations (C113) | ||
Cybersecurity Threats (C12) | Cyberattacks on Transportation Networks (C121) | |
Data Breaches in Ticketing and Reservation Systems (C122) | ||
Malware Targeting Transportation Infrastructure (C123) | ||
Safety and Compliance Risks (C13) | Non-Compliance with Safety Regulations (C131) | |
Accidents Resulting from Non-Adherence to Safety Protocols (C132) | ||
Failure to Meet Transportation Security Standards (C133) | ||
Healthcare (C2) | Medical Data Privacy Risks (C21) | Unauthorized Access to Patient Medical Records (C211) |
Data Leakage from Medical Devices during Transit (C212) | ||
Non-Compliance with Healthcare Data Privacy Regulations (C213) | ||
Medical Device Security Risks (C22) | Vulnerabilities in Medical Equipment (C221) | |
Unauthorized Access to Medical Devices (C222) | ||
Tampering with Medical Sensors and Monitoring Systems (C223) | ||
Compliance with Healthcare Regulations (C23) | Non-Compliance with HIPAA Regulations (C231) | |
Violations of Medical Transportation Standards (C232) | ||
Legal Consequences of Non-Compliance (C233) | ||
IoT (C3) | IoT Device Vulnerabilities (C31) | Weaknesses in IoT Device Security (C311) |
Unauthorized Access to IoT Devices (C312) | ||
IoT Device Tampering (C313) | ||
Data Security in Transportation IoT (C32) | Data Breaches in Transportation IoT (C321) | |
Data Manipulation in Transit (C322) | ||
Encryption and Data Integrity Risks (C323) | ||
Interconnected Systems Risks (C33) | Cross-Sector Data Leakage (C331) | |
Interference with Healthcare IoT Devices (C332) | ||
Regulatory Challenges in Cross-Sector Integration (C333) |
Rank | Abbreviation | Linguistic Term | Triangular Hesitant-Fuzzy Number |
10 | EHI | Extremely High Importance | (7, 9, 9) |
9 | VHS | Very High Significance | (5, 7, 9) |
8 | RHS | Really High Significance | (3, 5, 7) |
7 | WHI | Weakly High Importance | (1, 3, 5) |
6 | EHI | Equally High Importance | (1, 1, 3) |
5 | EE | Exactly Equal | (1, 1, 1) |
4 | ELI | Equally Low Importance | (0.33, 1, 1) |
3 | WLI | Weakly Low Important | (0.2, 0.33, 1) |
2 | RLS | Really Low Significance | (0.14, 0.2, 0.33) |
1 | VLS | Very Low Significance | (0.11, 0.14, 0.2) |
0 | ELI | Extremely Low Importance | (0.11, 0.11, 0.14) |
Characteristic A/ Characteristic B | Fuzzy Pair-Wise Comparisons Matrices | Defuzzified Pair-Wise Comparison Matrices |
C1/C2 | 0.6352, 0.9143, 1.3430 | 0.3720 |
C2/C3 | 1.0000, 1.5200, 1.9300 | 0.4561 |
C11/C12 | 0.8206, 1.1118, 1.6150 | 0.3720 |
C12/C13 | 0.6600, 1.1700, 1.6900 | 0.8920 |
C21/C22 | 0.6900, 0.8900, 1.1000 | 0.6910 |
C22/C23 | 0.9710, 1.2475, 1.6094 | 0.3720 |
C31/C32 | 0.3230, 0.4480, 0.6051 | 0.6910 |
C32/C33 | 1.0592, 1.5849, 2.2206 | 0.3720 |
C111/C112 | 1.1500, 1.4400, 1.7000 | 1.1720 |
C112/C113 | 0.3000, 0.4400, 0.8000 | 0.6910 |
C121/C122 | 0.2300, 0.2800, 0.3600 | 0.3720 |
C122/C123 | 0.6600, 1.1700, 1.6900 | 0.6910 |
C131/C132 | 0.6900, 0.8900, 1.1000 | 0.3720 |
C132/C133 | 0.9710, 1.2475, 1.6094 | 0.3720 |
C211/C212 | 0.3230, 0.4480, 0.6051 | 0.6910 |
C212/C213 | 0.9710, 1.2475, 1.6094 | 0.3720 |
C221/C222 | 0.3230, 0.4480, 0.6051 | 1.1720 |
C222/C223 | 1.1500, 1.4400, 1.7000 | 0.6910 |
C231/C232 | 0.3000, 0.4400, 0.8000 | 0.3720 |
C232/C233 | 0.2300, 0.2800, 0.3600 | 1.1720 |
C311/C312 | 0.6600, 1.1700, 1.6900 | 0.6910 |
C312/C313 | 0.6900, 0.8900, 1.1000 | 0.3720 |
C321/C322 | 0.9710, 1.2475, 1.6094 | 0.6910 |
C322/C323 | 0.3230, 0.4480, 0.6051 | 0.3720 |
C331/C332 | 0.9710, 1.2475, 1.6094 | 0.3720 |
C332/C333 | 0.3230, 0.4480, 0.6051 | 0.6910 |
Characteristic | Symbols | Independent Weight of the Groups | Overall Weights through Network | Percentage |
Priority at Stage 1 | ||||
Transportation | C1 | 0.472329 | 0.472329 | 47.23% |
Healthcare | C2 | 0.328972 | 0.328972 | 32.90% |
IoT | C3 | 0.198699 | 0.198699 | 19.87% |
Priority at Stage 2 | ||||
Physical Infrastructure Risks | C11 | 0.178541 | 0.084758 | 8.48% |
Cybersecurity Threats | C12 | 0.311521 | 0.147886 | 14.79% |
Safety and Compliance Risks | C13 | 0.210311 | 0.09984 | 9.98% |
Medical Data Privacy Risks | C21 | 0.166168 | 0.078884 | 7.89% |
Medical Device Security Risks | C22 | 0.300120 | 0.142474 | 14.25% |
Compliance with Healthcare Regulations | C23 | 0.220006 | 0.104442 | 10.45% |
IoT Device Vulnerabilities | C31 | 0.322513 | 0.153105 | 15.31% |
Data Security in Transportation IoT | C32 | 0.177183 | 0.084113 | 8.41% |
Interconnected Systems Risks | C33 | 0.220126 | 0.104499 | 10.45% |
Priority at Stage 3 | ||||
Threats to Infrastructure Integrity | C111 | 0.2200 | 0.02825 | 2.83% |
Sabotage of Transportation Facilities | C112 | 0.3225 | 0.04930 | 4.93% |
Unauthorized Access to Critical Transportation Locations | C113 | 0.1772 | 0.03328 | 3.33% |
Cyberattacks on Transportation Networks | C121 | 0.2201 | 0.02629 | 2.63% |
Data Breaches in Ticketing and Reservation Systems | C122 | 0.1785 | 0.04749 | 4.75% |
Malware Targeting Transportation Infrastructure | C123 | 0.3115 | 0.03481 | 3.48% |
Non-Compliance with Safety Regulations | C131 | 0.2103 | 0.05103 | 5.10% |
Accidents Resulting from Non-Adherence to Safety Protocols | C132 | 0.1662 | 0.02804 | 2.80% |
Failure to Meet Transportation Security Standards | C133 | 0.3001 | 0.03483 | 3.48% |
Unauthorized Access to Patient Medical Records | C211 | 0.2200 | 0.02825 | 2.83% |
Data Leakage from Medical Devices during Transit | C212 | 0.3225 | 0.04930 | 4.93% |
Non-Compliance with Healthcare Data Privacy Regulations | C213 | 0.1772 | 0.03328 | 3.33% |
Vulnerabilities in Medical Equipment | C221 | 0.2201 | 0.02629 | 2.63% |
Unauthorized Access to Medical Devices | C222 | 0.1785 | 0.04749 | 4.75% |
Tampering with Medical Sensors and Monitoring Systems | C223 | 0.3115 | 0.03481 | 3.48% |
Non-Compliance with HIPAA Regulations | C231 | 0.2103 | 0.05103 | 5.10% |
Violations of Medical Transportation Standards | C232 | 0.1662 | 0.02804 | 2.80% |
Legal Consequences of Non-Compliance | C233 | 0.3001 | 0.03483 | 3.48% |
Weaknesses in IoT Device Security | C311 | 0.2200 | 0.02825 | 2.83% |
Unauthorized Access to IoT Devices | C312 | 0.3225 | 0.04930 | 4.93% |
IoT Device Tampering | C313 | 0.1772 | 0.03328 | 3.33% |
Data Breaches in Transportation IoT | C321 | 0.2201 | 0.02629 | 2.63% |
Data Manipulation in Transit | C322 | 0.2200 | 0.04749 | 4.75% |
Encryption and Data Integrity Risks | C323 | 0.3225 | 0.03481 | 3.48% |
Cross-Sector Data Leakage | C331 | 0.1772 | 0.05103 | 5.10% |
Interference with Healthcare IoT Devices | C332 | 0.2201 | 0.02804 | 2.80% |
Regulatory Challenges in Cross-Sector Integration | C333 | 0.1785 | 0.03483 | 3.48% |
Factors at Final Stage | IoTTHA1 | IoTTHA2 | IoTTHA3 | IoTTHA4 | IoTTHA5 | IoTTHA6 | IoTTHA7 |
C111 | 0.7642 | 0.7576 | 0.7656 | 0.7642 | 0.7576 | 0.7656 | 0.5333 |
C112 | 0.8832 | 0.9193 | 0.9051 | 0.8832 | 0.9193 | 0.9051 | 0.7336 |
C113 | 0.9172 | 0.9051 | 0.7336 | 0.9172 | 0.9051 | 0.7336 | 0.7571 |
C121 | 0.9681 | 0.6492 | 0.6578 | 0.9681 | 0.6492 | 0.6578 | 0.9191 |
C122 | 0.7642 | 0.7576 | 0.7656 | 0.7642 | 0.7576 | 0.7656 | 0.5333 |
C123 | 0.8832 | 0.9193 | 0.9051 | 0.8832 | 0.9193 | 0.9051 | 0.7336 |
C131 | 0.9172 | 0.9051 | 0.7336 | 0.9172 | 0.9051 | 0.7336 | 0.7571 |
C132 | 0.9193 | 0.9051 | 0.8832 | 0.9193 | 0.9051 | 0.8832 | 0.9193 |
C133 | 0.9051 | 0.7336 | 0.9172 | 0.9051 | 0.7336 | 0.9172 | 0.9051 |
C211 | 0.6492 | 0.6578 | 0.9681 | 0.6492 | 0.6578 | 0.9681 | 0.6492 |
C212 | 0.7576 | 0.7656 | 0.7642 | 0.7576 | 0.7656 | 0.7642 | 0.7576 |
C213 | 0.9193 | 0.9051 | 0.8832 | 0.9193 | 0.9051 | 0.8832 | 0.9193 |
C221 | 0.9051 | 0.9051 | 0.7336 | 0.9172 | 0.9051 | 0.7336 | 0.7571 |
C222 | 0.6492 | 0.6492 | 0.6578 | 0.9681 | 0.6492 | 0.6578 | 0.9191 |
C223 | 0.7576 | 0.7576 | 0.7656 | 0.7642 | 0.7576 | 0.7656 | 0.5333 |
C231 | 0.9193 | 0.9193 | 0.9051 | 0.8832 | 0.9193 | 0.9051 | 0.7336 |
C232 | 0.9051 | 0.9051 | 0.7336 | 0.9172 | 0.9051 | 0.7336 | 0.7571 |
C233 | 0.9051 | 0.7336 | 0.9172 | 0.9051 | 0.7336 | 0.7571 | 0.9193 |
C311 | 0.6492 | 0.6578 | 0.9681 | 0.6492 | 0.6578 | 0.9191 | 0.9051 |
C312 | 0.7576 | 0.7656 | 0.7642 | 0.7576 | 0.7656 | 0.5333 | 0.6492 |
C313 | 0.9193 | 0.9051 | 0.9051 | 0.7336 | 0.9172 | 0.9051 | 0.7336 |
C321 | 0.9051 | 0.7336 | 0.6492 | 0.6578 | 0.9681 | 0.6492 | 0.6578 |
C322 | 0.9051 | 0.8832 | 0.7576 | 0.7656 | 0.7642 | 0.7576 | 0.7656 |
C323 | 0.7336 | 0.9172 | 0.9193 | 0.9051 | 0.8832 | 0.9193 | 0.9051 |
C331 | 0.6578 | 0.9681 | 0.9051 | 0.7336 | 0.9172 | 0.9051 | 0.7336 |
C332 | 0.7656 | 0.7642 | 0.9051 | 0.8832 | 0.9193 | 0.9051 | 0.8832 |
C333 | 0.9051 | 0.8832 | 0.7336 | 0.9172 | 0.9051 | 0.7336 | 0.9172 |
Factors at Final Stage | IoTTHA1 | IoTTHA2 | IoTTHA3 | IoTTHA4 | IoTTHA5 | IoTTHA6 | IoTTHA7 |
C111 | 0.0630 | 0.0230 | 0.0370 | 0.1310 | 0.1220 | 0.1310 | 0.1220 |
C112 | 0.0979 | 0.0370 | 0.1220 | 0.0630 | 0.0230 | 0.0630 | 0.0230 |
C113 | 0.1310 | 0.1220 | 0.0230 | 0.0979 | 0.0370 | 0.0979 | 0.0516 |
C121 | 0.0630 | 0.0370 | 0.0370 | 0.1310 | 0.1220 | 0.1310 | 0.1220 |
C122 | 0.0979 | 0.1220 | 0.1220 | 0.0630 | 0.0230 | 0.0630 | 0.0230 |
C123 | 0.0370 | 0.0230 | 0.0230 | 0.0979 | 0.0370 | 0.0979 | 0.0516 |
C131 | 0.0370 | 0.1220 | 0.0630 | 0.0230 | 0.0630 | 0.0230 | 0.0230 |
C132 | 0.1220 | 0.0230 | 0.0979 | 0.0370 | 0.0979 | 0.0516 | 0.0516 |
C133 | 0.0370 | 0.0370 | 0.1310 | 0.1220 | 0.1310 | 0.1220 | 0.1220 |
C211 | 0.0370 | 0.1220 | 0.0630 | 0.0230 | 0.0630 | 0.0230 | 0.0230 |
C212 | 0.1220 | 0.0230 | 0.0979 | 0.0370 | 0.0979 | 0.0516 | 0.0516 |
C213 | 0.0370 | 0.0370 | 0.1220 | 0.0630 | 0.0230 | 0.0630 | 0.0230 |
C221 | 0.1220 | 0.1220 | 0.0230 | 0.0979 | 0.0370 | 0.0979 | 0.0516 |
C222 | 0.0230 | 0.0370 | 0.0370 | 0.1310 | 0.1220 | 0.1310 | 0.1220 |
C223 | 0.1220 | 0.1220 | 0.1220 | 0.0630 | 0.0230 | 0.0630 | 0.0230 |
C231 | 0.0230 | 0.0230 | 0.0230 | 0.0979 | 0.0370 | 0.0979 | 0.0516 |
C232 | 0.1220 | 0.1220 | 0.1220 | 0.0630 | 0.0230 | 0.0630 | 0.0230 |
C233 | 0.0230 | 0.0230 | 0.0230 | 0.0979 | 0.0370 | 0.0979 | 0.0516 |
C311 | 0.1220 | 0.1220 | 0.0630 | 0.0230 | 0.0630 | 0.0230 | 0.0230 |
C312 | 0.0230 | 0.0230 | 0.0979 | 0.0370 | 0.0979 | 0.0516 | 0.0516 |
C313 | 0.0370 | 0.0370 | 0.1310 | 0.1220 | 0.1310 | 0.1220 | 0.1220 |
C321 | 0.1220 | 0.1220 | 0.0630 | 0.0230 | 0.0630 | 0.0230 | 0.0230 |
C322 | 0.0230 | 0.0230 | 0.0979 | 0.0370 | 0.0979 | 0.0516 | 0.0516 |
C323 | 0.0370 | 0.0370 | 0.1220 | 0.0630 | 0.0230 | 0.0630 | 0.0230 |
C331 | 0.1220 | 0.1220 | 0.0230 | 0.0979 | 0.0370 | 0.0979 | 0.0516 |
C332 | 0.0370 | 0.0370 | 0.0370 | 0.1310 | 0.1220 | 0.1310 | 0.1220 |
C333 | 0.1220 | 0.1220 | 0.1220 | 0.0630 | 0.0230 | 0.0630 | 0.0230 |
S. No. | Alternatives | Closeness Coefficients | Ranks |
1 | IoTTHA1 | 0.599854 | 1 |
2 | IoTTHA2 | 0.556365 | 2 |
3 | IoTTHA3 | 0.485547 | 7 |
4 | IoTTHA4 | 0.552658 | 3 |
5 | IoTTHA5 | 0.512227 | 6 |
6 | IoTTHA6 | 0.521145 | 5 |
7 | IoTTHA7 | 0.545887 | 4 |
Experiments | Weights/ Alternatives | IoTTHA1 | IoTTHA2 | IoTTHA3 | IoTTHA4 | IoTTHA5 | IoTTHA6 | IoTTHA7 |
Exp-0 | Original Weights | 0.599854 | 0.554515 | 0.485540 | 0.552656 | 0.517587 | 0.521789 | 0.545237 |
Exp-1 | C111 | 0.594154 | 0.555765 | 0.485568 | 0.552656 | 0.512857 | 0.521745 | 0.545997 |
Exp-2 | C112 | 0.54154 | 0.555565 | 0.485582 | 0.552677 | 0.512286 | 0.523564 | 0.545777 |
Exp-3 | C113 | 0.594154 | 0.555565 | 0.485554 | 0.552689 | 0.512285 | 0.521745 | 0.543567 |
Exp-4 | C121 | 0.591254 | 0.557455 | 0.485547 | 0.552698 | 0.512278 | 0.585687 | 0.542367 |
Exp-5 | C122 | 0.51254 | 0.555875 | 0.485583 | 0.552686 | 0.512275 | 0.521778 | 0.542217 |
Exp-6 | C123 | 0.565854 | 0.556365 | 0.485587 | 0.552656 | 0.512285 | 0.528569 | 0.545237 |
Exp-7 | C131 | 0.597454 | 0.557455 | 0.485589 | 0.552658 | 0.512275 | 0.521145 | 0.548757 |
Exp-8 | C132 | 0.555244 | 0.557465 | 0.485589 | 0.552656 | 0.512278 | 0.526587 | 0.548967 |
Exp-9 | C133 | 0.574454 | 0.555665 | 0.485578 | 0.552658 | 0.512296 | 0.521145 | 0.548567 |
Exp-10 | C211 | 0.545214 | 0.554755 | 0.485556 | 0.552685 | 0.512285 | 0.525678 | 0.546597 |
Exp-11 | C212 | 0.544544 | 0.554565 | 0.485574 | 0.552600 | 0.512587 | 0.521458 | 0.548567 |
Exp-12 | C213 | 0.594524 | 0.557465 | 0.485563 | 0.552674 | 0.512279 | 0.525623 | 0.547897 |
Exp-13 | C221 | 0.599854 | 0.554565 | 0.485596 | 0.552641 | 0.512229 | 0.525556 | 0.546587 |
Exp-14 | C222 | 0.54424 | 0.556525 | 0.485582 | 0.552645 | 0.512297 | 0.524477 | 0.545987 |
Exp-15 | C223 | 0.594124 | 0.553255 | 0.485558 | 0.552656 | 0.512291 | 0.528899 | 0.545897 |
Exp-16 | C231 | 0.595214 | 0.553365 | 0.485579 | 0.552658 | 0.512238 | 0.527711 | 0.545787 |
Exp-17 | C232 | 0.51454 | 0.558985 | 0.485578 | 0.552669 | 0.512274 | 0.523355 | 0.545597 |
Exp-18 | C233 | 0.599854 | 0.557895 | 0.485575 | 0.552697 | 0.512282 | 0.526666 | 0.552587 |
Exp-19 | C311 | 0.545344 | 0.555235 | 0.485546 | 0.552689 | 0.512264 | 0.527777 | 0.548987 |
Exp-20 | C312 | 0.594154 | 0.552355 | 0.485548 | 0.552654 | 0.512275 | 0.521758 | 0.548787 |
Exp-21 | C313 | 0.584254 | 0.552545 | 0.485546 | 0.552656 | 0.512237 | 0.521145 | 0.548887 |
Exp-22 | C321 | 0.591254 | 0.554785 | 0.485548 | 0.552653 | 0.512286 | 0.528569 | 0.585678 |
Exp-23 | C322 | 0.592254 | 0.552565 | 0.485777 | 0.552655 | 0.512275 | 0.524257 | 0.545887 |
Exp-24 | C323 | 0.594454 | 0.553255 | 0.485787 | 0.552657 | 0.512253 | 0.525632 | 0.547857 |
Exp-25 | C331 | 0.588854 | 0.552545 | 0.487747 | 0.552685 | 0.512287 | 0.522356 | 0.548967 |
Exp-26 | C332 | 0.595654 | 0.557895 | 0.487457 | 0.552652 | 0.512252 | 0.521254 | 0.547857 |
Exp-27 | C333 | 0.594564 | 0.554755 | 0.484757 | 0.552635 | 0.512256 | 0.522563 | 0.545878 |
Methods/ Alternatives | IoTTHA1 | IoTTHA2 | IoTTHA3 | IoTTHA4 | IoTTHA5 | IoTTHA6 | IoTTHA7 |
Hesitant Fuzzy-AHP-TOPSIS | 0.599854 | 0.554515 | 0.485540 | 0.552656 | 0.517587 | 0.521789 | 0.545237 |
Fuzzy-AHP-TOPSIS | 0.594124 | 0.553255 | 0.485558 | 0.552656 | 0.512291 | 0.528899 | 0.545897 |
Hesitant Fuzzy AHP-VIKOR | 0.588854 | 0.552545 | 0.487747 | 0.552685 | 0.512287 | 0.522356 | 0.548967 |
Hesitant Fuzzy AHP-ELECTRE | 0.594124 | 0.553255 | 0.485558 | 0.552656 | 0.512291 | 0.528899 | 0.545897 |
S. No. | Study | Approach/Methodology | Application Focus | Key Contributions |
1 | Hussain, A., Ali, T., et al. [16] | Knowledge-Based Security | Vulnerability detection in IoTT Applications | Introduces knowledge-based security approach. |
2 | Q. Li [17] | Learning-Based Security | Web-based emergency clinic plans | Addresses functional and security requirements. |
3 | Shahid, J., et al. [18] | Hesitant Fuzzy Multi-Criteria Decision-Making | IoTT applications network | Provides a mathematical framework for risk assessment. |
4 | Rejeb, A., et al. [19] | Mitigate System | Protection hazard assessment | Systematically measures security risks. |
5 | Alsaadi, M. R., et al. [20] | Hazard Assessment Process | Security feature prioritization | Highlights the importance of alignment with development. |
6 | Alqahtani, M. et al. [21 | IoT Digital Risk Assessment | Digital risk assessment for IoT | Identifies gaps in digital risk guidelines. |
7 | Binsawad, M., et al. [22] | Cloud Supply Chain Cyber Risk | CRM application security risk | Quantitative risk assessment supported by survey model. |
8 | L. W. Lee et al. [23] | Hesitant Fuzzy-TOPSIS | Supplier selection for car parts | Offers a clear ranking of suppliers for sourcing decisions. |
9 | Quasim, M. T. [24] | IoT Risk Assessment | Multi-criteria decision making | Challenges and applications of internet of things (IoT) |
10 | Büyüközkan, G., et al. [25] | Hesitant Fuzzy AHP-TOPSIS | Internet finance risk assessment | Identifies and ranks financial risk factors. |
11 | (Cubukcu, C., et al. [26] | Hesitant Fuzzy AHP-TOPSIS | Sustainable energy planning | Integrates AHP, and Hesitant Fuzzy-TOPSIS for energy planning. |
12 | A. Memari, et al. [27] | Hesitant Fuzzy ANP-TOPSIS | Sustainable environmental conflict ranking | Ranks environmental conflicts arising from supplier selection. |
13 | Gündoğdu, F. K., et. al. [28] | ANP, and F-TOPSIS | Technology adoption barriers | Identifies and ranks obstacles to technology adoption. |
Stage 1 | Stage 2 | Stage 3 |
Transportation (C1) | Physical Infrastructure Risks (C11) | Threats to Infrastructure Integrity (C111) |
Sabotage of Transportation Facilities (C112) | ||
Unauthorized Access to Critical Transportation Locations (C113) | ||
Cybersecurity Threats (C12) | Cyberattacks on Transportation Networks (C121) | |
Data Breaches in Ticketing and Reservation Systems (C122) | ||
Malware Targeting Transportation Infrastructure (C123) | ||
Safety and Compliance Risks (C13) | Non-Compliance with Safety Regulations (C131) | |
Accidents Resulting from Non-Adherence to Safety Protocols (C132) | ||
Failure to Meet Transportation Security Standards (C133) | ||
Healthcare (C2) | Medical Data Privacy Risks (C21) | Unauthorized Access to Patient Medical Records (C211) |
Data Leakage from Medical Devices during Transit (C212) | ||
Non-Compliance with Healthcare Data Privacy Regulations (C213) | ||
Medical Device Security Risks (C22) | Vulnerabilities in Medical Equipment (C221) | |
Unauthorized Access to Medical Devices (C222) | ||
Tampering with Medical Sensors and Monitoring Systems (C223) | ||
Compliance with Healthcare Regulations (C23) | Non-Compliance with HIPAA Regulations (C231) | |
Violations of Medical Transportation Standards (C232) | ||
Legal Consequences of Non-Compliance (C233) | ||
IoT (C3) | IoT Device Vulnerabilities (C31) | Weaknesses in IoT Device Security (C311) |
Unauthorized Access to IoT Devices (C312) | ||
IoT Device Tampering (C313) | ||
Data Security in Transportation IoT (C32) | Data Breaches in Transportation IoT (C321) | |
Data Manipulation in Transit (C322) | ||
Encryption and Data Integrity Risks (C323) | ||
Interconnected Systems Risks (C33) | Cross-Sector Data Leakage (C331) | |
Interference with Healthcare IoT Devices (C332) | ||
Regulatory Challenges in Cross-Sector Integration (C333) |
Rank | Abbreviation | Linguistic Term | Triangular Hesitant-Fuzzy Number |
10 | EHI | Extremely High Importance | (7, 9, 9) |
9 | VHS | Very High Significance | (5, 7, 9) |
8 | RHS | Really High Significance | (3, 5, 7) |
7 | WHI | Weakly High Importance | (1, 3, 5) |
6 | EHI | Equally High Importance | (1, 1, 3) |
5 | EE | Exactly Equal | (1, 1, 1) |
4 | ELI | Equally Low Importance | (0.33, 1, 1) |
3 | WLI | Weakly Low Important | (0.2, 0.33, 1) |
2 | RLS | Really Low Significance | (0.14, 0.2, 0.33) |
1 | VLS | Very Low Significance | (0.11, 0.14, 0.2) |
0 | ELI | Extremely Low Importance | (0.11, 0.11, 0.14) |
Characteristic A/ Characteristic B | Fuzzy Pair-Wise Comparisons Matrices | Defuzzified Pair-Wise Comparison Matrices |
C1/C2 | 0.6352, 0.9143, 1.3430 | 0.3720 |
C2/C3 | 1.0000, 1.5200, 1.9300 | 0.4561 |
C11/C12 | 0.8206, 1.1118, 1.6150 | 0.3720 |
C12/C13 | 0.6600, 1.1700, 1.6900 | 0.8920 |
C21/C22 | 0.6900, 0.8900, 1.1000 | 0.6910 |
C22/C23 | 0.9710, 1.2475, 1.6094 | 0.3720 |
C31/C32 | 0.3230, 0.4480, 0.6051 | 0.6910 |
C32/C33 | 1.0592, 1.5849, 2.2206 | 0.3720 |
C111/C112 | 1.1500, 1.4400, 1.7000 | 1.1720 |
C112/C113 | 0.3000, 0.4400, 0.8000 | 0.6910 |
C121/C122 | 0.2300, 0.2800, 0.3600 | 0.3720 |
C122/C123 | 0.6600, 1.1700, 1.6900 | 0.6910 |
C131/C132 | 0.6900, 0.8900, 1.1000 | 0.3720 |
C132/C133 | 0.9710, 1.2475, 1.6094 | 0.3720 |
C211/C212 | 0.3230, 0.4480, 0.6051 | 0.6910 |
C212/C213 | 0.9710, 1.2475, 1.6094 | 0.3720 |
C221/C222 | 0.3230, 0.4480, 0.6051 | 1.1720 |
C222/C223 | 1.1500, 1.4400, 1.7000 | 0.6910 |
C231/C232 | 0.3000, 0.4400, 0.8000 | 0.3720 |
C232/C233 | 0.2300, 0.2800, 0.3600 | 1.1720 |
C311/C312 | 0.6600, 1.1700, 1.6900 | 0.6910 |
C312/C313 | 0.6900, 0.8900, 1.1000 | 0.3720 |
C321/C322 | 0.9710, 1.2475, 1.6094 | 0.6910 |
C322/C323 | 0.3230, 0.4480, 0.6051 | 0.3720 |
C331/C332 | 0.9710, 1.2475, 1.6094 | 0.3720 |
C332/C333 | 0.3230, 0.4480, 0.6051 | 0.6910 |
Characteristic | Symbols | Independent Weight of the Groups | Overall Weights through Network | Percentage |
Priority at Stage 1 | ||||
Transportation | C1 | 0.472329 | 0.472329 | 47.23% |
Healthcare | C2 | 0.328972 | 0.328972 | 32.90% |
IoT | C3 | 0.198699 | 0.198699 | 19.87% |
Priority at Stage 2 | ||||
Physical Infrastructure Risks | C11 | 0.178541 | 0.084758 | 8.48% |
Cybersecurity Threats | C12 | 0.311521 | 0.147886 | 14.79% |
Safety and Compliance Risks | C13 | 0.210311 | 0.09984 | 9.98% |
Medical Data Privacy Risks | C21 | 0.166168 | 0.078884 | 7.89% |
Medical Device Security Risks | C22 | 0.300120 | 0.142474 | 14.25% |
Compliance with Healthcare Regulations | C23 | 0.220006 | 0.104442 | 10.45% |
IoT Device Vulnerabilities | C31 | 0.322513 | 0.153105 | 15.31% |
Data Security in Transportation IoT | C32 | 0.177183 | 0.084113 | 8.41% |
Interconnected Systems Risks | C33 | 0.220126 | 0.104499 | 10.45% |
Priority at Stage 3 | ||||
Threats to Infrastructure Integrity | C111 | 0.2200 | 0.02825 | 2.83% |
Sabotage of Transportation Facilities | C112 | 0.3225 | 0.04930 | 4.93% |
Unauthorized Access to Critical Transportation Locations | C113 | 0.1772 | 0.03328 | 3.33% |
Cyberattacks on Transportation Networks | C121 | 0.2201 | 0.02629 | 2.63% |
Data Breaches in Ticketing and Reservation Systems | C122 | 0.1785 | 0.04749 | 4.75% |
Malware Targeting Transportation Infrastructure | C123 | 0.3115 | 0.03481 | 3.48% |
Non-Compliance with Safety Regulations | C131 | 0.2103 | 0.05103 | 5.10% |
Accidents Resulting from Non-Adherence to Safety Protocols | C132 | 0.1662 | 0.02804 | 2.80% |
Failure to Meet Transportation Security Standards | C133 | 0.3001 | 0.03483 | 3.48% |
Unauthorized Access to Patient Medical Records | C211 | 0.2200 | 0.02825 | 2.83% |
Data Leakage from Medical Devices during Transit | C212 | 0.3225 | 0.04930 | 4.93% |
Non-Compliance with Healthcare Data Privacy Regulations | C213 | 0.1772 | 0.03328 | 3.33% |
Vulnerabilities in Medical Equipment | C221 | 0.2201 | 0.02629 | 2.63% |
Unauthorized Access to Medical Devices | C222 | 0.1785 | 0.04749 | 4.75% |
Tampering with Medical Sensors and Monitoring Systems | C223 | 0.3115 | 0.03481 | 3.48% |
Non-Compliance with HIPAA Regulations | C231 | 0.2103 | 0.05103 | 5.10% |
Violations of Medical Transportation Standards | C232 | 0.1662 | 0.02804 | 2.80% |
Legal Consequences of Non-Compliance | C233 | 0.3001 | 0.03483 | 3.48% |
Weaknesses in IoT Device Security | C311 | 0.2200 | 0.02825 | 2.83% |
Unauthorized Access to IoT Devices | C312 | 0.3225 | 0.04930 | 4.93% |
IoT Device Tampering | C313 | 0.1772 | 0.03328 | 3.33% |
Data Breaches in Transportation IoT | C321 | 0.2201 | 0.02629 | 2.63% |
Data Manipulation in Transit | C322 | 0.2200 | 0.04749 | 4.75% |
Encryption and Data Integrity Risks | C323 | 0.3225 | 0.03481 | 3.48% |
Cross-Sector Data Leakage | C331 | 0.1772 | 0.05103 | 5.10% |
Interference with Healthcare IoT Devices | C332 | 0.2201 | 0.02804 | 2.80% |
Regulatory Challenges in Cross-Sector Integration | C333 | 0.1785 | 0.03483 | 3.48% |
Factors at Final Stage | IoTTHA1 | IoTTHA2 | IoTTHA3 | IoTTHA4 | IoTTHA5 | IoTTHA6 | IoTTHA7 |
C111 | 0.7642 | 0.7576 | 0.7656 | 0.7642 | 0.7576 | 0.7656 | 0.5333 |
C112 | 0.8832 | 0.9193 | 0.9051 | 0.8832 | 0.9193 | 0.9051 | 0.7336 |
C113 | 0.9172 | 0.9051 | 0.7336 | 0.9172 | 0.9051 | 0.7336 | 0.7571 |
C121 | 0.9681 | 0.6492 | 0.6578 | 0.9681 | 0.6492 | 0.6578 | 0.9191 |
C122 | 0.7642 | 0.7576 | 0.7656 | 0.7642 | 0.7576 | 0.7656 | 0.5333 |
C123 | 0.8832 | 0.9193 | 0.9051 | 0.8832 | 0.9193 | 0.9051 | 0.7336 |
C131 | 0.9172 | 0.9051 | 0.7336 | 0.9172 | 0.9051 | 0.7336 | 0.7571 |
C132 | 0.9193 | 0.9051 | 0.8832 | 0.9193 | 0.9051 | 0.8832 | 0.9193 |
C133 | 0.9051 | 0.7336 | 0.9172 | 0.9051 | 0.7336 | 0.9172 | 0.9051 |
C211 | 0.6492 | 0.6578 | 0.9681 | 0.6492 | 0.6578 | 0.9681 | 0.6492 |
C212 | 0.7576 | 0.7656 | 0.7642 | 0.7576 | 0.7656 | 0.7642 | 0.7576 |
C213 | 0.9193 | 0.9051 | 0.8832 | 0.9193 | 0.9051 | 0.8832 | 0.9193 |
C221 | 0.9051 | 0.9051 | 0.7336 | 0.9172 | 0.9051 | 0.7336 | 0.7571 |
C222 | 0.6492 | 0.6492 | 0.6578 | 0.9681 | 0.6492 | 0.6578 | 0.9191 |
C223 | 0.7576 | 0.7576 | 0.7656 | 0.7642 | 0.7576 | 0.7656 | 0.5333 |
C231 | 0.9193 | 0.9193 | 0.9051 | 0.8832 | 0.9193 | 0.9051 | 0.7336 |
C232 | 0.9051 | 0.9051 | 0.7336 | 0.9172 | 0.9051 | 0.7336 | 0.7571 |
C233 | 0.9051 | 0.7336 | 0.9172 | 0.9051 | 0.7336 | 0.7571 | 0.9193 |
C311 | 0.6492 | 0.6578 | 0.9681 | 0.6492 | 0.6578 | 0.9191 | 0.9051 |
C312 | 0.7576 | 0.7656 | 0.7642 | 0.7576 | 0.7656 | 0.5333 | 0.6492 |
C313 | 0.9193 | 0.9051 | 0.9051 | 0.7336 | 0.9172 | 0.9051 | 0.7336 |
C321 | 0.9051 | 0.7336 | 0.6492 | 0.6578 | 0.9681 | 0.6492 | 0.6578 |
C322 | 0.9051 | 0.8832 | 0.7576 | 0.7656 | 0.7642 | 0.7576 | 0.7656 |
C323 | 0.7336 | 0.9172 | 0.9193 | 0.9051 | 0.8832 | 0.9193 | 0.9051 |
C331 | 0.6578 | 0.9681 | 0.9051 | 0.7336 | 0.9172 | 0.9051 | 0.7336 |
C332 | 0.7656 | 0.7642 | 0.9051 | 0.8832 | 0.9193 | 0.9051 | 0.8832 |
C333 | 0.9051 | 0.8832 | 0.7336 | 0.9172 | 0.9051 | 0.7336 | 0.9172 |
Factors at Final Stage | IoTTHA1 | IoTTHA2 | IoTTHA3 | IoTTHA4 | IoTTHA5 | IoTTHA6 | IoTTHA7 |
C111 | 0.0630 | 0.0230 | 0.0370 | 0.1310 | 0.1220 | 0.1310 | 0.1220 |
C112 | 0.0979 | 0.0370 | 0.1220 | 0.0630 | 0.0230 | 0.0630 | 0.0230 |
C113 | 0.1310 | 0.1220 | 0.0230 | 0.0979 | 0.0370 | 0.0979 | 0.0516 |
C121 | 0.0630 | 0.0370 | 0.0370 | 0.1310 | 0.1220 | 0.1310 | 0.1220 |
C122 | 0.0979 | 0.1220 | 0.1220 | 0.0630 | 0.0230 | 0.0630 | 0.0230 |
C123 | 0.0370 | 0.0230 | 0.0230 | 0.0979 | 0.0370 | 0.0979 | 0.0516 |
C131 | 0.0370 | 0.1220 | 0.0630 | 0.0230 | 0.0630 | 0.0230 | 0.0230 |
C132 | 0.1220 | 0.0230 | 0.0979 | 0.0370 | 0.0979 | 0.0516 | 0.0516 |
C133 | 0.0370 | 0.0370 | 0.1310 | 0.1220 | 0.1310 | 0.1220 | 0.1220 |
C211 | 0.0370 | 0.1220 | 0.0630 | 0.0230 | 0.0630 | 0.0230 | 0.0230 |
C212 | 0.1220 | 0.0230 | 0.0979 | 0.0370 | 0.0979 | 0.0516 | 0.0516 |
C213 | 0.0370 | 0.0370 | 0.1220 | 0.0630 | 0.0230 | 0.0630 | 0.0230 |
C221 | 0.1220 | 0.1220 | 0.0230 | 0.0979 | 0.0370 | 0.0979 | 0.0516 |
C222 | 0.0230 | 0.0370 | 0.0370 | 0.1310 | 0.1220 | 0.1310 | 0.1220 |
C223 | 0.1220 | 0.1220 | 0.1220 | 0.0630 | 0.0230 | 0.0630 | 0.0230 |
C231 | 0.0230 | 0.0230 | 0.0230 | 0.0979 | 0.0370 | 0.0979 | 0.0516 |
C232 | 0.1220 | 0.1220 | 0.1220 | 0.0630 | 0.0230 | 0.0630 | 0.0230 |
C233 | 0.0230 | 0.0230 | 0.0230 | 0.0979 | 0.0370 | 0.0979 | 0.0516 |
C311 | 0.1220 | 0.1220 | 0.0630 | 0.0230 | 0.0630 | 0.0230 | 0.0230 |
C312 | 0.0230 | 0.0230 | 0.0979 | 0.0370 | 0.0979 | 0.0516 | 0.0516 |
C313 | 0.0370 | 0.0370 | 0.1310 | 0.1220 | 0.1310 | 0.1220 | 0.1220 |
C321 | 0.1220 | 0.1220 | 0.0630 | 0.0230 | 0.0630 | 0.0230 | 0.0230 |
C322 | 0.0230 | 0.0230 | 0.0979 | 0.0370 | 0.0979 | 0.0516 | 0.0516 |
C323 | 0.0370 | 0.0370 | 0.1220 | 0.0630 | 0.0230 | 0.0630 | 0.0230 |
C331 | 0.1220 | 0.1220 | 0.0230 | 0.0979 | 0.0370 | 0.0979 | 0.0516 |
C332 | 0.0370 | 0.0370 | 0.0370 | 0.1310 | 0.1220 | 0.1310 | 0.1220 |
C333 | 0.1220 | 0.1220 | 0.1220 | 0.0630 | 0.0230 | 0.0630 | 0.0230 |
S. No. | Alternatives | Closeness Coefficients | Ranks |
1 | IoTTHA1 | 0.599854 | 1 |
2 | IoTTHA2 | 0.556365 | 2 |
3 | IoTTHA3 | 0.485547 | 7 |
4 | IoTTHA4 | 0.552658 | 3 |
5 | IoTTHA5 | 0.512227 | 6 |
6 | IoTTHA6 | 0.521145 | 5 |
7 | IoTTHA7 | 0.545887 | 4 |
Experiments | Weights/ Alternatives | IoTTHA1 | IoTTHA2 | IoTTHA3 | IoTTHA4 | IoTTHA5 | IoTTHA6 | IoTTHA7 |
Exp-0 | Original Weights | 0.599854 | 0.554515 | 0.485540 | 0.552656 | 0.517587 | 0.521789 | 0.545237 |
Exp-1 | C111 | 0.594154 | 0.555765 | 0.485568 | 0.552656 | 0.512857 | 0.521745 | 0.545997 |
Exp-2 | C112 | 0.54154 | 0.555565 | 0.485582 | 0.552677 | 0.512286 | 0.523564 | 0.545777 |
Exp-3 | C113 | 0.594154 | 0.555565 | 0.485554 | 0.552689 | 0.512285 | 0.521745 | 0.543567 |
Exp-4 | C121 | 0.591254 | 0.557455 | 0.485547 | 0.552698 | 0.512278 | 0.585687 | 0.542367 |
Exp-5 | C122 | 0.51254 | 0.555875 | 0.485583 | 0.552686 | 0.512275 | 0.521778 | 0.542217 |
Exp-6 | C123 | 0.565854 | 0.556365 | 0.485587 | 0.552656 | 0.512285 | 0.528569 | 0.545237 |
Exp-7 | C131 | 0.597454 | 0.557455 | 0.485589 | 0.552658 | 0.512275 | 0.521145 | 0.548757 |
Exp-8 | C132 | 0.555244 | 0.557465 | 0.485589 | 0.552656 | 0.512278 | 0.526587 | 0.548967 |
Exp-9 | C133 | 0.574454 | 0.555665 | 0.485578 | 0.552658 | 0.512296 | 0.521145 | 0.548567 |
Exp-10 | C211 | 0.545214 | 0.554755 | 0.485556 | 0.552685 | 0.512285 | 0.525678 | 0.546597 |
Exp-11 | C212 | 0.544544 | 0.554565 | 0.485574 | 0.552600 | 0.512587 | 0.521458 | 0.548567 |
Exp-12 | C213 | 0.594524 | 0.557465 | 0.485563 | 0.552674 | 0.512279 | 0.525623 | 0.547897 |
Exp-13 | C221 | 0.599854 | 0.554565 | 0.485596 | 0.552641 | 0.512229 | 0.525556 | 0.546587 |
Exp-14 | C222 | 0.54424 | 0.556525 | 0.485582 | 0.552645 | 0.512297 | 0.524477 | 0.545987 |
Exp-15 | C223 | 0.594124 | 0.553255 | 0.485558 | 0.552656 | 0.512291 | 0.528899 | 0.545897 |
Exp-16 | C231 | 0.595214 | 0.553365 | 0.485579 | 0.552658 | 0.512238 | 0.527711 | 0.545787 |
Exp-17 | C232 | 0.51454 | 0.558985 | 0.485578 | 0.552669 | 0.512274 | 0.523355 | 0.545597 |
Exp-18 | C233 | 0.599854 | 0.557895 | 0.485575 | 0.552697 | 0.512282 | 0.526666 | 0.552587 |
Exp-19 | C311 | 0.545344 | 0.555235 | 0.485546 | 0.552689 | 0.512264 | 0.527777 | 0.548987 |
Exp-20 | C312 | 0.594154 | 0.552355 | 0.485548 | 0.552654 | 0.512275 | 0.521758 | 0.548787 |
Exp-21 | C313 | 0.584254 | 0.552545 | 0.485546 | 0.552656 | 0.512237 | 0.521145 | 0.548887 |
Exp-22 | C321 | 0.591254 | 0.554785 | 0.485548 | 0.552653 | 0.512286 | 0.528569 | 0.585678 |
Exp-23 | C322 | 0.592254 | 0.552565 | 0.485777 | 0.552655 | 0.512275 | 0.524257 | 0.545887 |
Exp-24 | C323 | 0.594454 | 0.553255 | 0.485787 | 0.552657 | 0.512253 | 0.525632 | 0.547857 |
Exp-25 | C331 | 0.588854 | 0.552545 | 0.487747 | 0.552685 | 0.512287 | 0.522356 | 0.548967 |
Exp-26 | C332 | 0.595654 | 0.557895 | 0.487457 | 0.552652 | 0.512252 | 0.521254 | 0.547857 |
Exp-27 | C333 | 0.594564 | 0.554755 | 0.484757 | 0.552635 | 0.512256 | 0.522563 | 0.545878 |
Methods/ Alternatives | IoTTHA1 | IoTTHA2 | IoTTHA3 | IoTTHA4 | IoTTHA5 | IoTTHA6 | IoTTHA7 |
Hesitant Fuzzy-AHP-TOPSIS | 0.599854 | 0.554515 | 0.485540 | 0.552656 | 0.517587 | 0.521789 | 0.545237 |
Fuzzy-AHP-TOPSIS | 0.594124 | 0.553255 | 0.485558 | 0.552656 | 0.512291 | 0.528899 | 0.545897 |
Hesitant Fuzzy AHP-VIKOR | 0.588854 | 0.552545 | 0.487747 | 0.552685 | 0.512287 | 0.522356 | 0.548967 |
Hesitant Fuzzy AHP-ELECTRE | 0.594124 | 0.553255 | 0.485558 | 0.552656 | 0.512291 | 0.528899 | 0.545897 |