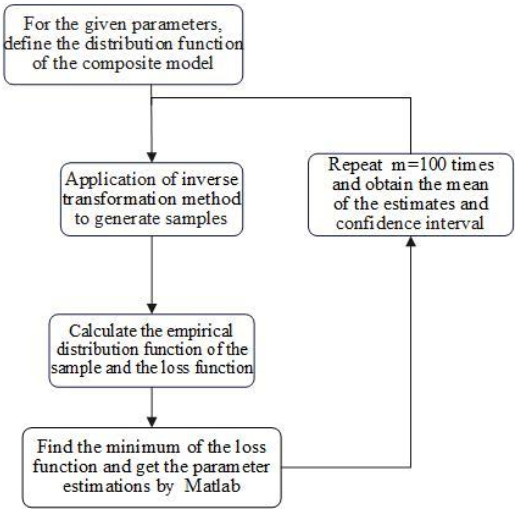
Threshold selection is challenging when analyzing tail data with a generalized Pareto distribution. Data below the threshold was not used in the model, resulting in incomplete characterization of the whole data. This paper applied the Gamma distribution, Weibull distribution, and lognormal distribution to fit the central data separately, and a generalized Pareto distribution (GPD) was used to analyze the tail data. In such composite models, the thresholds are estimated directly as parameters. We proposed an empirical distribution function-based parameter estimation method. The absolute value of the difference between the empirical distribution function and the composite distribution function was used as a loss function to obtain an estimate of the parameter. This parameter estimation method is suitable for complex multiparameter distributions. The estimation method based on the empirical distribution function was verified to be feasible through simulation studies. The composite model and the estimation method based on the empirical distribution function were applied to study the earthquake magnitude data to provide a reference for earthquake hazard analysis.
Citation: Yanfang Zhang, Fuchang Wang, Yibin Zhao. Statistical characteristics of earthquake magnitude based on the composite model[J]. AIMS Mathematics, 2024, 9(1): 607-624. doi: 10.3934/math.2024032
[1] | Riza Andrian Ibrahim, Sukono, Herlina Napitupulu, Rose Irnawaty Ibrahim . Modeling earthquake bond prices with correlated dual trigger indices and the approximate solution using the Monte Carlo algorithm. AIMS Mathematics, 2025, 10(2): 2223-2253. doi: 10.3934/math.2025103 |
[2] | Aisha Fayomi, Ehab M. Almetwally, Maha E. Qura . A novel bivariate Lomax-G family of distributions: Properties, inference, and applications to environmental, medical, and computer science data. AIMS Mathematics, 2023, 8(8): 17539-17584. doi: 10.3934/math.2023896 |
[3] | H. M. Barakat, M. A. Alawady, I. A. Husseiny, M. Nagy, A. H. Mansi, M. O. Mohamed . Bivariate Epanechnikov-exponential distribution: statistical properties, reliability measures, and applications to computer science data. AIMS Mathematics, 2024, 9(11): 32299-32327. doi: 10.3934/math.20241550 |
[4] | Tie Li, Zhengcheng Zhang . Generalized conditional spacings and their stochastic properties. AIMS Mathematics, 2024, 9(9): 23909-23923. doi: 10.3934/math.20241162 |
[5] | Qin Gong, Laijun Luo, Haiping Ren . Unit compound Rayleigh model: Statistical characteristics, estimation and application. AIMS Mathematics, 2024, 9(8): 22813-22841. doi: 10.3934/math.20241110 |
[6] | Mohamed Said Mohamed, Haroon M. Barakat, Aned Al Mutairi, Manahil SidAhmed Mustafa . Further properties of Tsallis extropy and some of its related measures. AIMS Mathematics, 2023, 8(12): 28219-28245. doi: 10.3934/math.20231445 |
[7] | Rashad M. EL-Sagheer, Mohamed S. Eliwa, Khaled M. Alqahtani, Mahmoud El-Morshedy . Bayesian and non-Bayesian inferential approaches under lower-recorded data with application to model COVID-19 data. AIMS Mathematics, 2022, 7(9): 15965-15981. doi: 10.3934/math.2022873 |
[8] | Nora Nader, Dina A. Ramadan, Hanan Haj Ahmad, M. A. El-Damcese, B. S. El-Desouky . Optimizing analgesic pain relief time analysis through Bayesian and non-Bayesian approaches to new right truncated Fréchet-inverted Weibull distribution. AIMS Mathematics, 2023, 8(12): 31217-31245. doi: 10.3934/math.20231598 |
[9] | Yong Tao . Generalized Pareto distribution and income inequality: an extension of Gibrat's law. AIMS Mathematics, 2024, 9(6): 15060-15075. doi: 10.3934/math.2024730 |
[10] | Ahmed M. Gemeay, Najwan Alsadat, Christophe Chesneau, Mohammed Elgarhy . Power unit inverse Lindley distribution with different measures of uncertainty, estimation and applications. AIMS Mathematics, 2024, 9(8): 20976-21024. doi: 10.3934/math.20241021 |
Threshold selection is challenging when analyzing tail data with a generalized Pareto distribution. Data below the threshold was not used in the model, resulting in incomplete characterization of the whole data. This paper applied the Gamma distribution, Weibull distribution, and lognormal distribution to fit the central data separately, and a generalized Pareto distribution (GPD) was used to analyze the tail data. In such composite models, the thresholds are estimated directly as parameters. We proposed an empirical distribution function-based parameter estimation method. The absolute value of the difference between the empirical distribution function and the composite distribution function was used as a loss function to obtain an estimate of the parameter. This parameter estimation method is suitable for complex multiparameter distributions. The estimation method based on the empirical distribution function was verified to be feasible through simulation studies. The composite model and the estimation method based on the empirical distribution function were applied to study the earthquake magnitude data to provide a reference for earthquake hazard analysis.
Earthquakes with a magnitude greater than five cause many casualties, and destructive earthquakes are a threat to human life and property. Many small and medium-sized earthquakes cause more disasters than people expect, even affecting social stability, and are of great concern to the government and the public. Therefore, small and medium-magnitude seismic data study is also of practical importance. The Peaks Over Threshold (POT) model based on the generalized Pareto distribution (GPD) is widely used in data analysis in various industries because it can fully use the information of extreme value contained in the data [1]. In recent years, there have been some findings of earthquake hazards, especially in the analysis of large-magnitude earthquakes [2]. The classical fixed threshold modeling approach uses graphical diagnostics. Coles [3] outlined the common graphical diagnostics for threshold choice. Scarrott and MacDonald [4] gave a long review of tail and non-tail estimation methods. However, this model falls short in practical data processing in two ways as data analysis with such a model is generally done in two steps. In the first, the threshold u is chosen graphically by looking at the mean excess plot [5] or simply setting it as some high percentile of the data [6]. Both methods are subjective and the parameter estimates depend on the threshold. In such areas, the main problem is the scarcity of data or, more specifically, modeling with a fairly small amount of observations.
To address this issue, Arnoldo et al. [7] proposed a new dynamically weighted mixture model, where one of the terms is the GPD and the other is a light-tailed density function. Mendes and Lopes [8] proposed a procedure to fit a mixture model by maximum likelihood where the tails are GPD and the distribution center is normal. The normal spliced with GPD tail developed by Carreau and Bengio [9] named the 'hybrid Pareto' model was further developed to include constraints on parameters to ensure continuity up to the first derivative of the density. Behrens [10] proposed a model to analyze data characterized by extreme events where a threshold is estimated directly. Nadarajah and Bakar [11] proposed new composite models based on the lognormal distribution. Caladerin-Ojeda [12,13] analyzed claim data and all French settlements from 1962 to 2012 using the composite Weibull-Burr model and the composite lognormal-Pareto distribution separately. Extreme likelihood estimation is widely used in composite models [14]. Carreau & Bengio [15] and Carreau et al. [16] implemented a neural network learning approach in nonstationary and bivariate modeling situations. Bayesian inference was used by Behrens et al. [10] with sensible prior forms for the bulk, tail and threshold parameters. Tancredi et al. [17] was the first to propose an extreme value mixture model that combined a nonparametric estimator for the bulk distribution spliced with an extreme value tail model. Li et al. [18] extended the model by fitting the tails with GPD and the central part with gamma, lognormal, mixed gamma and Weibull distributions to build four combined models for the evaluation of post-earthquake loss in Yunnan Province, respectively. Due to the complexity of the combined models, the Bayes estimates of the parameters were calculated using the Markov chain Monte Carlo (MCMC) method used in the previous papers. There are other methods to estimate the parameters. Dupuis [19] proposed a robust procedure for fitting GPD, including statistics to guide threshold choice. Thompson et al. [20] developed an automated procedure for threshold estimation and uncertainty quantification. They set a uniformly spaced grid of possible threshold values (between the median and the 98% empirical quantile). For each potential threshold, the GPD is fitted (using maximum likelihood (ML) estimation) and the differences in the modified scale parameters for neighboring thresholds are calculated.
The composite model allows fitting all the data and considering the thresholds as parameters for direct estimation. We propose an empirical distribution function-based parameter estimation method to estimate the parameters in the composite model. The correctness of the parameter estimation method is tested by generating simulated data. Finally, the model and parameter estimation method is used to analyze the seismic data of the fracture zone with the composite model.
Consider that X1,X2,⋯,Xn are independent and identically distributed observations and u is the threshold over which these observations are exceedances.
Then, (Xi|Xi⩾u)∼G(ξ,σ,u), where
G(ξ,σ,u)={1−(1+ξ(x−u)σ)−1/ξ, ξ≠01−exp{−(x−μ)/σ},ξ=0 | (1) |
is the distribution function of the GPD and the probability density function of the GPD is
g(x|ξ,σ,u)={1σ(1+ξx−uσ)(−1/ξ)−1,x⩾u,1+ξ(x−u)/σ>01σexp(−(x−u)/σ),ξ=0 . | (2) |
Observations below the threshold obey the H distribution, which can be estimated.
Assume that is any distribution such as a Weibull, Gamma or Lognormal distribution. The distribution function of the composite distribution is as follows:
![]() |
(3) |
Composite model one Gamma-GPD composite model:
In formula (3), if ,
, where
h1(x|α,β)=βαΓ(α)exp(−βx)xα−1,x>0 | (4) |
is the probability density function of the Gamma distribution. The distribution function of the composite model is
F(x|α,β,ξ,σ,u)={H1(x|α,β), x<uH1(u|α,β)+[1−H1(u|α,β)]G(x|ξ,σ,u),x⩾u. | (5) |
Composite model two Weibull-GPD composite model:
In formula (3), if ,
, where
h2(x|λ,k)={kλ(xλ)k−1e−(xλ)k,x⩾00, x<0 | (6) |
is the probability density function of the Weibull distribution. The distribution function of the composite model is
F(x|λ,k,ξ,σ,u)={H2(x|λ,k), x<uH2(u|λ,k)+[1−H2(u|λ,k)]G(x|ξ,σ,u),x⩾u. | (7) |
Composite model three Lognormal-GPD composite model:
In formula (3), if ,
, where
h3(x|μ,σL)={1√2πσLxexp{−(lnx−μ)22σL2},x>00, x⩽0 | (8) |
is the probability density function of the Weibull distribution. The distribution function of the composite model is
F(x|μ,σ,ξ,σ,u)={H3(x|μ,σL), x<uH3(u|μ,σL)+[1−H3(u|μ,σL)]G(x|ξ,σ,u),x⩾u. | (9) |
Definition 1. A statistical estimation of F(x) based on a random sample X1,X2,⋯,Xn is the empirical distribution function defined by
Fn(x)=1nn∑i=1I(Xi⩽x),−∞<x<∞, | (10) |
where I(⋅) denotes the indicator function of the event in the brackets.
From the Glivenko-Cantelli theorem [21], it follows
P{limn→∞sup−∞<x<∞|Fn(x)−F(x)|=0}=1. | (11) |
Therefore, when the sample size n is sufficiently large, Fn(x) approximates well the distribution function , where
is the parameter of the distribution. Based on the above conclusions, we devise the following method for estimating the unknown parameters of the distribution. Define the loss function as
![]() |
where Fn(x) is the empirical distribution function.
Find that satisfies
and
is an estimate of the parameter
. This method allows for the numerical estimation of complex distributions that contain several parameters. For the composite model in this paper, which contains several parameters and cannot be solved analytically, we use the above empirical distribution function-based method to estimate the parameters.
To illustrate the feasibility of the method, we compare the traditional maximum likelihood estimation with the estimation based on the empirical distribution function.
The likelihood function of the composite model is
![]() |
(12) |
where
g((xi−u)|ξ,σ)={σ−1(1+(xi−u)ξ/σ)−(1+ξ)/ξ,(1+(xi−u)ξ/σ))>0σ−1exp(−(xi−u)/σ), ξ=0 | (13) |
is the probability density function of GPD. Find that satisfies
and
is the maximum likelihood estimate of the parameter
. Since there is no analytical solution to the maximum likelihood estimate, the numerical solution is solved here using the optimization algorithm.
The numerical simulation process of parameter estimations based on the empirical distribution functions is as follows and the flowchart is shown in Figure 1.
(1) For the given parameters, define the distribution function of the composite model and generate random numbers of [0, 1]. The distribution functions of the three composite models are given in section two. Apply the inverse transformation method to obtain samples obeying the composite models.
(2) Calculate the empirical distribution function Fn(x) of the samples. Define the loss function as
![]() |
(3) Apply the Nelder-Mead simplex algorithm in Matlab to find the minimum of the loss function and get the parameter estimations.
(4) The number of samples generated is n = 100, n = 200, n = 300, n = 500 and n = 1000 and the mean of the estimates obtained by repeating m = 100 times, respectively, is the parameter estimate.
(5) Apply nonparametric bootstrap methods to obtain confidence intervals for parameters.
The numerical simulation process of parameter estimations based on the maximum likelihood method is similar to the empirical distribution function based method.
In the composite model x=(x1,x2,⋯,xn) for the given parameters θθ, the numerical simulation results are given in Tables 1–3 as follows. The histograms of the parameters of each model are shown in Figures 2–4.
Means of Parameter estimations |
Number of random numbers |
Composite model one ![]() α=5,β=2,u=3,ξ=−0.3,σ=1.5 |
Empirical distribution functions-based estimation | 100 | ˆα=4.6242,ˆβ=1.8387,ˆu=2.6312,ˆξ=−0.1217,ˆσ=1.1875 |
200 | ˆα=4.8517,ˆβ=1.9235,ˆu=2.4942,ˆξ=−0.1310,ˆσ=1.2402 | |
300 | ˆα=4.7897,ˆβ=1.8964,ˆu=2.6707,ˆξ=−0.1347,ˆσ=1.2642 | |
500 | ˆα=4.7226,ˆβ=1.8623,ˆu=2.4255,ˆξ=−0.1690,ˆσ=1.2757 | |
1000 | ˆα=4.9295,ˆβ=1.9630,ˆu=2.5380,ˆξ=−0.2087,ˆσ=1.4185 | |
Maximum likelihood estimation | 100 | ˆα=4.8392,ˆβ=1.9280,ˆu=3.0209,ˆξ=−0.2249,ˆσ=1.3420 |
200 | ˆα=4.5448,ˆβ=1.8052,ˆu=2.1849,ˆξ=−0.2161,ˆσ=1.1923 | |
300 | ˆα=4.6571,ˆβ=1.8489,ˆu=2.1895,ˆξ=−0.1903,ˆσ=1.1988 | |
500 | ˆα=4.7507,ˆβ=1.8935,ˆu=2.2595,ˆξ=−0.2175,ˆσ=1.2391 | |
1000 | ˆα=4.9420,ˆβ=1.9709,ˆu=2.4197,ˆξ=−0.2438,ˆσ=1.4156 |
Means of Parameter estimations | Number of random numbers |
Composite model two ![]() λ=5,k=2,u=3,ξ=−0.3,σ=1.5 |
Empirical distribution functions-based estimation | 100 | ˆλ=4.2335,ˆk=1.8201,ˆu=3.0727,ˆξ=−0.2960,ˆσ=1.4363 |
200 | ˆλ=4.1684,ˆk=1.6556,ˆu=3.0153,ˆξ=−0.2976,ˆσ=1.4851 | |
300 | ˆλ=4.0384,ˆk=1.5171,ˆu=3.0160,ˆξ=−0.2868,ˆσ=1.4703 | |
500 | ˆλ=4.8354,ˆk=1.4530,ˆu=3.0156,ˆξ=−0.2983,ˆσ=1.4978 | |
1000 | ˆλ=4.9770,ˆk=2.0320,ˆu=3.0093,ˆξ=−0.2927,ˆσ=1.4844 | |
Maximum likelihood estimation | 100 | ˆλ=4.3512,ˆk=1.7322,ˆu=2.9528,ˆξ=−0.3853,ˆσ=1.4463 |
200 | ˆλ=4.7620,ˆk=1.9973,ˆu=2.9354,ˆξ=−0.3948,ˆσ=1.4745 | |
300 | ˆλ=4.6439,ˆk=1.9146,ˆu=2.9607,ˆξ=−0.3828,ˆσ=1.4298 | |
500 | ˆλ=4.7451,ˆk=1.9671,ˆu=2.9772,ˆξ=−0.3837,ˆσ=1.4175 | |
1000 | ˆλ=4.5761,ˆk=2.2758,ˆu=2.7070,ˆξ=−0.4154,ˆσ=1.3960 |
Means of Parameter estimations | Number of random numbers |
Composite model three ![]() μ=5,σL=2,u=3,ξ=−0.3,σ=1.5 |
Empirical distribution functions-based estimation | 100 | ˆμ=3.8481,ˆσL=1.5243,ˆu=3.0643,ˆξ=−0.2516,ˆσ=1.3874 |
200 | ˆμ=4.0775,ˆσL=1.6193,ˆu=3.0249,ˆξ=−0.2922,ˆσ=1.4906 | |
300 | ˆμ=3.9829,ˆσL=1.5393,ˆu=3.0123,ˆξ=−0.3026,ˆσ=1.5040 | |
500 | ˆμ=3.9249,ˆσL=1.5245,ˆu=3.0226,ˆξ=−0.2833,ˆσ=1.4555 | |
1000 | ˆμ=3.9233,ˆσL=1.4751,ˆu=3.0072,ˆξ=−0.2967,ˆσ=1.4962 | |
Maximum likelihood estimation | 100 | ˆμ=4.5894,ˆσL=1.9642,ˆu=2.8494,ˆξ=−0.3467,ˆσ=1.3204 |
200 | ˆμ=4.6049,ˆσL=1.9401,ˆu=2.9521,ˆξ=−0.3623,ˆσ=1.4088 | |
300 | ˆμ=4.6161,ˆσL=1.8971,ˆu=2.9614,ˆξ=−0.3830,ˆσ=1.4167 | |
500 | ˆμ=4.6565,ˆσL=1.9063,ˆu=2.9623,ˆξ=−0.3684,ˆσ=1.3782 | |
1000 | ˆμ=4.8945,ˆσL=1.8788,ˆu=2.9927,ˆξ=−0.3627,ˆσ=1.3885 |
From Tables 1–3, it can be seen that for all three composite models, the proposed method based on empirical distribution functions is similar to the maximum likelihood estimation and is a feasible parameter estimation method. Both parameter estimation methods are relatively robust. Except for the parameter ξ, the deviation of each parameter estimate from the true value is small. The standard deviation and 95% confidence intervals for the parameters are shown in Tables 4–8.
Model | Meannnn | Standard deviation | Confidence interval |
Composite model one | 4.9295 | 0.3486 | [4.3261, 5.5389] |
Composite model two | 4.9770 | 0.2573 | [4.5044, 5.4832] |
Composite model three | 3.9233 | 0.9264 | [2.8943, 5.9720] |
Model | Meannnn | Standard deviation | Confidence interval |
Composite model one | 1.9630 | 0.1616 | [1.6596, 2.2588] |
Composite model two | 2.0320 | 0.1400 | [1.7210, 2.2723] |
Composite model three | 1.4751 | 0.4708 | [1.0014, 2.6472] |
Model | Mean of u | Standard of u | Confidence interval of u |
Composite model one | 2.5380 | 0.0682 | [2.0057, 3.3380] |
Composite model two | 3.0093 | 0.0394 | [2.9299, 3.0811] |
Composite model three | 3.0072 | 0.0186 | [2.9709, 3.0495] |
Model | Mean of ξ | Standard of ξ | Confidence interval of ξ |
Composite model one | −0.2087 | 0.1258 | [−0.4928, −0.0943] |
Composite model two | −0.2927 | 0.0722 | [−0.4489, −0.1643] |
Composite model three | −0.2967 | 0.0594 | [−0.4843, −0.1864] |
Model | Mean of σ | Standard of σ | Confidence interval of σ |
Composite model one | 1.4185 | 0.1563 | [1.0188, 1.6509] |
Composite model two | 1.4844 | 0.1044 | [1.2182, 1.6936] |
Composite model three | 1.4962 | 0.0785 | [1.3475, 1.6431] |
The standard deviations of the parameters λ are smaller in model 2, which is consistent with Figure 3. The standard deviation of the parameters ξ is greater in model one. The method appears to have a variability with ξ but is robust for the other parameters.
A comparison of the empirical distribution functions with the distribution function of the three composite models is shown in Figures 5–7. The two curves are very close to each other in each figure. The confidence interval for the empirical distribution function is also shown in each figure. The distribution function falls between the upper and lower confidence levels of the empirical distribution function.
GPD in the composite model can describe the tail data. The quantile is important for extreme value analysis. Scholars [22,23,24] proved that if the distribution function F belongs to the attraction domain of a generalized extreme value distribution (GEV), the limiting distribution of excesses is the GDP. The distribution function of the excess is
Fu(x)=P{X−u⩽x|X>u}=F(y+u)−F(u)1−F(u)→Gp(x,ξ,σ), | (14) |
where,
Gp(x,ξ,σ)=1−(1+ξxσ)−1ξ,x⩾0,1+ξxσ⩾0. | (15) |
¯Fu(x)=1−Fu(x)=1−F(x+u)−F(u)1−F(u) =1−F(u)−F(x+u)+F(u)1−F(u) |
and
¯F(u+x)=¯Fu(x)¯F(u). |
From Eq (15) ˆ¯Fu(x)=(1+ξxσ)−1ξ, the frequency Nu/n above the threshold u is the estimation of ¯F(u), where Nu is the number of samples above the threshold, then ˆ¯F(u)=Nun. We can get the estimation of ¯F(u+x) as follows:
ˆ¯F(u+x)=Nun(1+ˆξxˆσ)−1ˆξ, | (16) |
then we can get the estimation of F(x):
ˆF(x)=1−Nun(1+ˆξx−ˆuˆσ)−1/ˆξ. | (17) |
From Eq (17), we get the estimation of the p-quantile xp:
ˆxp=ˆu+ˆσˆξ{[nNu(1−p)]−ˆξ−1}, | (18) |
where F(xp)=p,0<p<1.
If ξ<0 and p→1, the estimation of the upper limit point x∗ of the support F is
ˆx∗=ˆu−ˆσˆξ. | (19) |
P{X>xp}=1−F(xp)=1/T, T=1/¯F(xp) is the theoretical return period with the return level xp and F(xp)=p (0<p<1). The return period estimate can be obtained:
ˆT=n365Nu(1+ˆξxp−ˆuˆσ)1/ˆξ. | (20) |
If the given return period is T years, bring p=1−1/(365T) into Eq (18), and we can estimate the return level
ˆx1−1365T=ˆu+ˆσˆξ((nNu(1−p))−ˆξ−1)=ˆu+ˆσˆξ((365T⋅Nun)ˆξ−1). | (21) |
In the following part, the earthquake magnitude data is analyzed using the above three composite models to study the seismic risk.
The data in this paper is from the National Seismic Science Data Sharing Center (https://data.earthquake.cn/). 19,221 records before December 2019 are the research samples in the scope of reference [25]. The annual magnitudes of the earthquake are shown in Figure 8.
The magnitude data is analyzed with the above three composite models. The final results of the parameters obtained by the empirical distribution function-based method are summarized in Tables 9–11.
Parameters | AIC | α | β | u | ξ | σ |
Parameter estimations |
10.388 | 5.7666 | 1.4296 | 2.9632 | −0.1296 | 0.7051 |
Parameters | AIC | λ | k | u | ξ | σ |
Parameter estimations |
311.518 | 8.8975 | 0.5459 | 2.9473 | −0.1103 | 0.7033 |
Parameters | AIC | μ | σL | u | ξ | σ |
Parameter estimations |
14.265 | 2.2692 | 0.4855 | 2.0800 | −0.1545 | 0.7182 |
Although the distributions describing the central part are different, the parameter estimations of the GPD that describe the tail are very close to each other in the three composite models. The values of the loss functions are 1.0212, 1.4330, 1.5874 in the three models, respectively, and the residual of composite model one is the smallest. From Figure 9 we can see that the Gamma distribution function is closer with the first half of the empirical distribution function, which is the same as the loss function value. Akaike information criterion (AIC) values are also reported in Tables 9–11 to compare the performance of different models. AIC values are 10.388 in composite model one and 14.265 in composite model three. The AIC value of 311.5178 for model two is much bigger than that for models one and three. Thus, the performance of models one and three are comparable, and model two performs the least. The value of the loss function for model one is the smallest; hence, model one is selected as the reasonable model.
Nowadays, there are non-parametric and semi-parametric approaches for the distribution of the non-tail, which make modeling more flexible. Mohd et al. [26] used the semi-parametric for measuring income inequality in Malaysia. Based on this, we developed a semi-parametric model for comparison with the composite model.
Assume that p denotes the proportion of top earthquake magnitude data. In the semi-parametric model, a generalized Pareto distribution is fitted to 100p% of the upper tail data.
The 100(1−p)% lower tail data is modeled by an empirical distribution. The empirical distribution is given by
Fn−k(x)=1n−kn∑i=1I(Xi⩽x),−∞<x<∞, |
where I(⋅) denotes the indicator function of the event in the brackets, and k is the number of observations in the upper tail of the earthquake magnitude.
The full semi-parametric distribution can be written as
F(x|ξ,σ,u)={Fn−k(x),x<uG(x|ξ,σ,u),x⩾u, |
where G(ξ,σ,u) is the distribution function of the GPD.
We used the same empirical-distribution function-based method to calculate parameters. The result is in Table 12 below.
Parameter | u | ξ | σ |
Parameter estiamtion | 7.0469 | −0.1841 | 0.0202 |
The parameter u is the threshold of earthquake magnitude; therefore, the estimation value of 7.0469 is too large and unreasonable. The data above the threshold is only 15. The sample size is reduced and, consequently, the variance of the parameter estimates increases.
Therefore, model one can be chosen to study the distribution of earthquake magnitude. The earthquake magnitude data that is smaller than the threshold obeys the Gamma distribution with parameters α=5.7666,β=1.4296, while the tail data larger than the threshold obeys a GPD. This conclusion can be used as a complement to probabilistic seismic hazard analysis (PSHA).
The estimation of ˆξ in Tables 9–11 is less than zero, indicating that the return level of magnitude predicted by the model has a theoretical upper limit. The theoretical maximum magnitude is about Ms=8.4 according to Eq (19) in composite model one.
In the Pareto distribution, the most important part of the tail analysis is the estimation of the quantile. The quantile is the return level of the magnitude of earthquake. We obtain the 1−p quantile of the distribution according to Eq (18). Bring the estimates of u,σ and ξ into Eq (21), and the return level for the given return period can be summarized in Table 13.
return period(year) | return level(Ms) xp | |
Composite model two | 3 | 6.33 |
5 | 6.64 | |
10 | 7.05 | |
30 | 7.68 | |
50 | 7.98 | |
100 | 8.24 |
The 100-year return level is Ms=8.24, indicating that earthquakes with a magnitude of about Ms=8.2 will occur in the east of Bayan Har every 100 years. This result is consistent with the fact that there was an earthquake of Ms=8.0 in 2008. It shows that the estimated return levels are all within the confidence intervals of the return level in Figure 10. The model is reasonable.
In response to the difficulty of selecting a threshold when analyzing data using the GPD and the fact that the model can only study data above the threshold, this article studied all the data using composite models. Composite models were developed by applying the Gamma distribution, the Weibull distribution, and the lognormal distribution combined with the GPD separately. A parameter estimation method was proposed based on the empirical distribution function. The parameter estimation method based on the empirical distribution function is suitable for the loss function, which is complex and can not be derived to optimize the function. The method appears robust for most of the measurements in the three composite models in this paper. For some parameters, the method appears to have some variability. This method is suitable for solving numerical solutions of parameter estimates in complex distributions. The composite models and the empirical distribution function-based method are applied to earthquake magnitude data to provide a reference for earthquake hazard analysis. The composite model can analyze all the data and the threshold can be directly estimated as a parameter. Composite models in seismic magnitude analysis expand the scope of their use and provide new ideas for magnitude analysis. Composite models and parameter estimation methods can also be used in other industries.
The authors declare they have not used Artificial Intelligence (AI) tools in the creation of this article.
This research was funded by the Fundamental Research Funds for Central Universities, Grant number ZY20215140 and Self-Financing Project of Scientific Research and Development Plan of Lang Fang Science and Technology Bureau, Grant number 2022011019.
The authors thank all the reviewers for their helpful comments.
The authors declare no conflict of interest.
[1] | E. Castillo, Extreme value theory in engineering, 1 Eds., New York: Academic Press, 1988. https://doi.org/10.2307/1269867 |
[2] |
V. F. Pisarenko, A. Sornette, D. Sornette, Characterization of the tail of the distribution of earthquake magnitudes by combining the GEV and GPD descriptions of extreme value theory, Pure Appl. Geophys., 171 (2014), 1599–1624. https://doi.org/10.1007/s00024-014-0882-z doi: 10.1007/s00024-014-0882-z
![]() |
[3] | S. Coles, An introduction to statistical modeling of extreme values, 1 Eds., Springer Series in Statistics, London: Springer-Verlag, 2001. Available from: https://www.doc88.com/p-9089129087291.html. |
[4] |
C. Scarrott, A. Macdonald, A review of extreme value threshold estimation and uncertainty quantification authors, Revstat-Stat. J., 10 (2012), 33–60. https://doi.org/10.1111/j.1467-842X.2012.00658.x doi: 10.1111/j.1467-842X.2012.00658.x
![]() |
[5] | P. Embrechts, C. Klüppelberg, T. Mikosch, Modelling extremal events for insurance and finance, New York: Springer, 1997. https://doi.org/10.1007/978-3-642-33483-2 |
[6] |
W. Dumouchel, G. Duncan, Using sample survey weights in multiple regression analyses of stratified samples, J. Am. Stat. Assoc., 78 (1983), 535–54. https://doi.org/10.1080/01621459.1983.10478006 doi: 10.1080/01621459.1983.10478006
![]() |
[7] |
A. Frigessi, O. Haug, H. Rue, A dynamic mixture model for unsupervised tail estimation without threshold selection, Extremes, 5 (2002), 219–235. https://doi.org/10.1023/A:1024072610684 doi: 10.1023/A:1024072610684
![]() |
[8] |
B. D. M. Mendes, H. F. Lopes, Data driven estimates for mixtures, Comput. Stat. Data An., 47 (2004), 583–598. https://doi.org/10.1016/j.csda.2003.12.006 doi: 10.1016/j.csda.2003.12.006
![]() |
[9] |
J. Carreau, Y. Bengio, A hybrid Pareto model for asymmetric fat-tailed data: the univariate case, Extremes, 12 (2009), 53–76. https://doi.org/10.1007/s10687-008-0068-0 doi: 10.1007/s10687-008-0068-0
![]() |
[10] |
C. N. Behrens, H. F. Lopes, D. Gamerman, Bayesian analysis of extreme events with threshold estimation, Stat. Model. Int. J., 4 (2003), 227–244. https://doi.org/10.1191/1471082X04st075oa doi: 10.1191/1471082X04st075oa
![]() |
[11] |
S. Nadarajah, S. A. A. Bakar, New composite models for the Danish fire insurance data, Scand. Actuar. J., 2014 (2014), 180–187. https://doi.org/10.1080/03461238.2012.695748 doi: 10.1080/03461238.2012.695748
![]() |
[12] |
E. C. Ojeda, On the composite Weibull-Burr model to describe claim data, Communications in Statistics: Case Studies, Data Anal. Appl., 1 (2015), 59–69. https://doi.org/10.1080/23737484.2015.1066661 doi: 10.1080/23737484.2015.1066661
![]() |
[13] |
E. C. Ojeda, The distribution of all French communes: A composite parametric approach, Physica A, 450 (2016), 385–394. https://doi.org/10.1016/j.physa.2016.01.018 doi: 10.1016/j.physa.2016.01.018
![]() |
[14] |
S. Wang, W. Chen, M. Chen, Y. W. Zhou, Maximum likelihood estimation of the parameters of the inverse Gaussian distribution using maximum rank set sampling with unequal samples, Math. Popul. Stud., 30 (2023), 1–21. https://doi.org/10.1080/08898480.2021.1996822 doi: 10.1080/08898480.2021.1996822
![]() |
[15] |
J. Carreau, Y. Bengio, A hybrid Pareto mixture for conditional asymmetric fat-tailed distributions, IEEE T. Neur. Networ., 20 (2009), 1087–1101. https://doi.org/10.1109/TNN.2009.2016339 doi: 10.1109/TNN.2009.2016339
![]() |
[16] |
J. Carreau, P. Naveau, E. Sauquet, A statistical rainfall-runoff mixture model with heavy-tailed components, Water Resour. Res., 45 (2009). https://doi.org/10.1029/2009wr007880 doi: 10.1029/2009wr007880
![]() |
[17] |
A. Tancredi, C. Anderson, A. O'Hagan, Accounting for threshold uncertainty in extreme value estimation, Extremes, 9 (2006), 87–106. https://doi.org/10.1007/s10687-006-0009-8 doi: 10.1007/s10687-006-0009-8
![]() |
[18] |
Y. X. Li, N. Tang, X. Jiang, Bayesian approaches for analyzing earthquake catastrophic risk, Insur. Math. Econ., 68 (2016), 110–119. https://doi.org/10.1016/j.insmatheco.2016.02.004 doi: 10.1016/j.insmatheco.2016.02.004
![]() |
[19] |
D. J. Dupuis, Exceedances over high thresholds: A guide to threshold selection, Extremes, 1 (1999), 251–261. https://doi.org/10.1023/A:1009914915709 doi: 10.1023/A:1009914915709
![]() |
[20] |
Y. Cai, D. Reeve, J. Stander, Automated threshold selection methods for extreme wave analysis, Coast. Eng., 56 (2009), 1013–1021. https://doi.org/10.1016/j.coastaleng.2009.06.003 doi: 10.1016/j.coastaleng.2009.06.003
![]() |
[21] | C. Forbes, M. Evans, N. Hastings, B. Peacock, Statistical distributions, 4 Eds., New Jersey: John Wiley & Sons, Inc., Hoboken, 2011. https://doi.org/10.1177/14614448100120051102 |
[22] |
J. P. Iii, Statistical inference using extreme order statistics, Ann. Stat., 3 (1975), 119–131. https://doi.org/10.1214/aos/1176343003 doi: 10.1214/aos/1176343003
![]() |
[23] |
A. A. Balkema, L. D. Haan, Residual life time at great age, Ann. Probab., 2 (1974), 792–804. https://doi.org/10.1214/aop/1176996548 doi: 10.1214/aop/1176996548
![]() |
[24] | M. R. Leadbetter, G. Lindgren, H. Rootzén, Extremes and related properties of random sequences and processes, New York: Springer Science Business Media, LLC Springer Verlag, 1984. |
[25] |
V. F. P. Sornette, Characterization of the frequency of extreme earthquake events by the generalized Pareto distribution, Pure Appl. Geophys., 160 (2003), 2343–2364. https://doi.org/10.1007/s00024-003-2397-x doi: 10.1007/s00024-003-2397-x
![]() |
[26] |
S. M. A. Mohd, M. Nurulkamal, I. Kamarulzaman, A robust semi-parametric approach for measuring income inequality in Malaysia, Physica A, 2018. https://doi.org/10.1016/j.physa.2018.08.029 doi: 10.1016/j.physa.2018.08.029
![]() |
Means of Parameter estimations |
Number of random numbers |
Composite model one ![]() α=5,β=2,u=3,ξ=−0.3,σ=1.5 |
Empirical distribution functions-based estimation | 100 | ˆα=4.6242,ˆβ=1.8387,ˆu=2.6312,ˆξ=−0.1217,ˆσ=1.1875 |
200 | ˆα=4.8517,ˆβ=1.9235,ˆu=2.4942,ˆξ=−0.1310,ˆσ=1.2402 | |
300 | ˆα=4.7897,ˆβ=1.8964,ˆu=2.6707,ˆξ=−0.1347,ˆσ=1.2642 | |
500 | ˆα=4.7226,ˆβ=1.8623,ˆu=2.4255,ˆξ=−0.1690,ˆσ=1.2757 | |
1000 | ˆα=4.9295,ˆβ=1.9630,ˆu=2.5380,ˆξ=−0.2087,ˆσ=1.4185 | |
Maximum likelihood estimation | 100 | ˆα=4.8392,ˆβ=1.9280,ˆu=3.0209,ˆξ=−0.2249,ˆσ=1.3420 |
200 | ˆα=4.5448,ˆβ=1.8052,ˆu=2.1849,ˆξ=−0.2161,ˆσ=1.1923 | |
300 | ˆα=4.6571,ˆβ=1.8489,ˆu=2.1895,ˆξ=−0.1903,ˆσ=1.1988 | |
500 | ˆα=4.7507,ˆβ=1.8935,ˆu=2.2595,ˆξ=−0.2175,ˆσ=1.2391 | |
1000 | ˆα=4.9420,ˆβ=1.9709,ˆu=2.4197,ˆξ=−0.2438,ˆσ=1.4156 |
Means of Parameter estimations | Number of random numbers |
Composite model two ![]() λ=5,k=2,u=3,ξ=−0.3,σ=1.5 |
Empirical distribution functions-based estimation | 100 | ˆλ=4.2335,ˆk=1.8201,ˆu=3.0727,ˆξ=−0.2960,ˆσ=1.4363 |
200 | ˆλ=4.1684,ˆk=1.6556,ˆu=3.0153,ˆξ=−0.2976,ˆσ=1.4851 | |
300 | ˆλ=4.0384,ˆk=1.5171,ˆu=3.0160,ˆξ=−0.2868,ˆσ=1.4703 | |
500 | ˆλ=4.8354,ˆk=1.4530,ˆu=3.0156,ˆξ=−0.2983,ˆσ=1.4978 | |
1000 | ˆλ=4.9770,ˆk=2.0320,ˆu=3.0093,ˆξ=−0.2927,ˆσ=1.4844 | |
Maximum likelihood estimation | 100 | ˆλ=4.3512,ˆk=1.7322,ˆu=2.9528,ˆξ=−0.3853,ˆσ=1.4463 |
200 | ˆλ=4.7620,ˆk=1.9973,ˆu=2.9354,ˆξ=−0.3948,ˆσ=1.4745 | |
300 | ˆλ=4.6439,ˆk=1.9146,ˆu=2.9607,ˆξ=−0.3828,ˆσ=1.4298 | |
500 | ˆλ=4.7451,ˆk=1.9671,ˆu=2.9772,ˆξ=−0.3837,ˆσ=1.4175 | |
1000 | ˆλ=4.5761,ˆk=2.2758,ˆu=2.7070,ˆξ=−0.4154,ˆσ=1.3960 |
Means of Parameter estimations | Number of random numbers |
Composite model three ![]() μ=5,σL=2,u=3,ξ=−0.3,σ=1.5 |
Empirical distribution functions-based estimation | 100 | ˆμ=3.8481,ˆσL=1.5243,ˆu=3.0643,ˆξ=−0.2516,ˆσ=1.3874 |
200 | ˆμ=4.0775,ˆσL=1.6193,ˆu=3.0249,ˆξ=−0.2922,ˆσ=1.4906 | |
300 | ˆμ=3.9829,ˆσL=1.5393,ˆu=3.0123,ˆξ=−0.3026,ˆσ=1.5040 | |
500 | ˆμ=3.9249,ˆσL=1.5245,ˆu=3.0226,ˆξ=−0.2833,ˆσ=1.4555 | |
1000 | ˆμ=3.9233,ˆσL=1.4751,ˆu=3.0072,ˆξ=−0.2967,ˆσ=1.4962 | |
Maximum likelihood estimation | 100 | ˆμ=4.5894,ˆσL=1.9642,ˆu=2.8494,ˆξ=−0.3467,ˆσ=1.3204 |
200 | ˆμ=4.6049,ˆσL=1.9401,ˆu=2.9521,ˆξ=−0.3623,ˆσ=1.4088 | |
300 | ˆμ=4.6161,ˆσL=1.8971,ˆu=2.9614,ˆξ=−0.3830,ˆσ=1.4167 | |
500 | ˆμ=4.6565,ˆσL=1.9063,ˆu=2.9623,ˆξ=−0.3684,ˆσ=1.3782 | |
1000 | ˆμ=4.8945,ˆσL=1.8788,ˆu=2.9927,ˆξ=−0.3627,ˆσ=1.3885 |
Model | Meannnn | Standard deviation | Confidence interval |
Composite model one | 4.9295 | 0.3486 | [4.3261, 5.5389] |
Composite model two | 4.9770 | 0.2573 | [4.5044, 5.4832] |
Composite model three | 3.9233 | 0.9264 | [2.8943, 5.9720] |
Model | Meannnn | Standard deviation | Confidence interval |
Composite model one | 1.9630 | 0.1616 | [1.6596, 2.2588] |
Composite model two | 2.0320 | 0.1400 | [1.7210, 2.2723] |
Composite model three | 1.4751 | 0.4708 | [1.0014, 2.6472] |
Model | Mean of u | Standard of u | Confidence interval of u |
Composite model one | 2.5380 | 0.0682 | [2.0057, 3.3380] |
Composite model two | 3.0093 | 0.0394 | [2.9299, 3.0811] |
Composite model three | 3.0072 | 0.0186 | [2.9709, 3.0495] |
Model | Mean of ξ | Standard of ξ | Confidence interval of ξ |
Composite model one | −0.2087 | 0.1258 | [−0.4928, −0.0943] |
Composite model two | −0.2927 | 0.0722 | [−0.4489, −0.1643] |
Composite model three | −0.2967 | 0.0594 | [−0.4843, −0.1864] |
Model | Mean of σ | Standard of σ | Confidence interval of σ |
Composite model one | 1.4185 | 0.1563 | [1.0188, 1.6509] |
Composite model two | 1.4844 | 0.1044 | [1.2182, 1.6936] |
Composite model three | 1.4962 | 0.0785 | [1.3475, 1.6431] |
Parameters | AIC | α | β | u | ξ | σ |
Parameter estimations |
10.388 | 5.7666 | 1.4296 | 2.9632 | −0.1296 | 0.7051 |
Parameters | AIC | λ | k | u | ξ | σ |
Parameter estimations |
311.518 | 8.8975 | 0.5459 | 2.9473 | −0.1103 | 0.7033 |
Parameters | AIC | μ | σL | u | ξ | σ |
Parameter estimations |
14.265 | 2.2692 | 0.4855 | 2.0800 | −0.1545 | 0.7182 |
Parameter | u | ξ | σ |
Parameter estiamtion | 7.0469 | −0.1841 | 0.0202 |
return period(year) | return level(Ms) xp | |
Composite model two | 3 | 6.33 |
5 | 6.64 | |
10 | 7.05 | |
30 | 7.68 | |
50 | 7.98 | |
100 | 8.24 |
Means of Parameter estimations |
Number of random numbers |
Composite model one ![]() α=5,β=2,u=3,ξ=−0.3,σ=1.5 |
Empirical distribution functions-based estimation | 100 | ˆα=4.6242,ˆβ=1.8387,ˆu=2.6312,ˆξ=−0.1217,ˆσ=1.1875 |
200 | ˆα=4.8517,ˆβ=1.9235,ˆu=2.4942,ˆξ=−0.1310,ˆσ=1.2402 | |
300 | ˆα=4.7897,ˆβ=1.8964,ˆu=2.6707,ˆξ=−0.1347,ˆσ=1.2642 | |
500 | ˆα=4.7226,ˆβ=1.8623,ˆu=2.4255,ˆξ=−0.1690,ˆσ=1.2757 | |
1000 | ˆα=4.9295,ˆβ=1.9630,ˆu=2.5380,ˆξ=−0.2087,ˆσ=1.4185 | |
Maximum likelihood estimation | 100 | ˆα=4.8392,ˆβ=1.9280,ˆu=3.0209,ˆξ=−0.2249,ˆσ=1.3420 |
200 | ˆα=4.5448,ˆβ=1.8052,ˆu=2.1849,ˆξ=−0.2161,ˆσ=1.1923 | |
300 | ˆα=4.6571,ˆβ=1.8489,ˆu=2.1895,ˆξ=−0.1903,ˆσ=1.1988 | |
500 | ˆα=4.7507,ˆβ=1.8935,ˆu=2.2595,ˆξ=−0.2175,ˆσ=1.2391 | |
1000 | ˆα=4.9420,ˆβ=1.9709,ˆu=2.4197,ˆξ=−0.2438,ˆσ=1.4156 |
Means of Parameter estimations | Number of random numbers |
Composite model two ![]() λ=5,k=2,u=3,ξ=−0.3,σ=1.5 |
Empirical distribution functions-based estimation | 100 | ˆλ=4.2335,ˆk=1.8201,ˆu=3.0727,ˆξ=−0.2960,ˆσ=1.4363 |
200 | ˆλ=4.1684,ˆk=1.6556,ˆu=3.0153,ˆξ=−0.2976,ˆσ=1.4851 | |
300 | ˆλ=4.0384,ˆk=1.5171,ˆu=3.0160,ˆξ=−0.2868,ˆσ=1.4703 | |
500 | ˆλ=4.8354,ˆk=1.4530,ˆu=3.0156,ˆξ=−0.2983,ˆσ=1.4978 | |
1000 | ˆλ=4.9770,ˆk=2.0320,ˆu=3.0093,ˆξ=−0.2927,ˆσ=1.4844 | |
Maximum likelihood estimation | 100 | ˆλ=4.3512,ˆk=1.7322,ˆu=2.9528,ˆξ=−0.3853,ˆσ=1.4463 |
200 | ˆλ=4.7620,ˆk=1.9973,ˆu=2.9354,ˆξ=−0.3948,ˆσ=1.4745 | |
300 | ˆλ=4.6439,ˆk=1.9146,ˆu=2.9607,ˆξ=−0.3828,ˆσ=1.4298 | |
500 | ˆλ=4.7451,ˆk=1.9671,ˆu=2.9772,ˆξ=−0.3837,ˆσ=1.4175 | |
1000 | ˆλ=4.5761,ˆk=2.2758,ˆu=2.7070,ˆξ=−0.4154,ˆσ=1.3960 |
Means of Parameter estimations | Number of random numbers |
Composite model three ![]() μ=5,σL=2,u=3,ξ=−0.3,σ=1.5 |
Empirical distribution functions-based estimation | 100 | ˆμ=3.8481,ˆσL=1.5243,ˆu=3.0643,ˆξ=−0.2516,ˆσ=1.3874 |
200 | ˆμ=4.0775,ˆσL=1.6193,ˆu=3.0249,ˆξ=−0.2922,ˆσ=1.4906 | |
300 | ˆμ=3.9829,ˆσL=1.5393,ˆu=3.0123,ˆξ=−0.3026,ˆσ=1.5040 | |
500 | ˆμ=3.9249,ˆσL=1.5245,ˆu=3.0226,ˆξ=−0.2833,ˆσ=1.4555 | |
1000 | ˆμ=3.9233,ˆσL=1.4751,ˆu=3.0072,ˆξ=−0.2967,ˆσ=1.4962 | |
Maximum likelihood estimation | 100 | ˆμ=4.5894,ˆσL=1.9642,ˆu=2.8494,ˆξ=−0.3467,ˆσ=1.3204 |
200 | ˆμ=4.6049,ˆσL=1.9401,ˆu=2.9521,ˆξ=−0.3623,ˆσ=1.4088 | |
300 | ˆμ=4.6161,ˆσL=1.8971,ˆu=2.9614,ˆξ=−0.3830,ˆσ=1.4167 | |
500 | ˆμ=4.6565,ˆσL=1.9063,ˆu=2.9623,ˆξ=−0.3684,ˆσ=1.3782 | |
1000 | ˆμ=4.8945,ˆσL=1.8788,ˆu=2.9927,ˆξ=−0.3627,ˆσ=1.3885 |
Model | Meannnn | Standard deviation | Confidence interval |
Composite model one | 4.9295 | 0.3486 | [4.3261, 5.5389] |
Composite model two | 4.9770 | 0.2573 | [4.5044, 5.4832] |
Composite model three | 3.9233 | 0.9264 | [2.8943, 5.9720] |
Model | Meannnn | Standard deviation | Confidence interval |
Composite model one | 1.9630 | 0.1616 | [1.6596, 2.2588] |
Composite model two | 2.0320 | 0.1400 | [1.7210, 2.2723] |
Composite model three | 1.4751 | 0.4708 | [1.0014, 2.6472] |
Model | Mean of u | Standard of u | Confidence interval of u |
Composite model one | 2.5380 | 0.0682 | [2.0057, 3.3380] |
Composite model two | 3.0093 | 0.0394 | [2.9299, 3.0811] |
Composite model three | 3.0072 | 0.0186 | [2.9709, 3.0495] |
Model | Mean of ξ | Standard of ξ | Confidence interval of ξ |
Composite model one | −0.2087 | 0.1258 | [−0.4928, −0.0943] |
Composite model two | −0.2927 | 0.0722 | [−0.4489, −0.1643] |
Composite model three | −0.2967 | 0.0594 | [−0.4843, −0.1864] |
Model | Mean of σ | Standard of σ | Confidence interval of σ |
Composite model one | 1.4185 | 0.1563 | [1.0188, 1.6509] |
Composite model two | 1.4844 | 0.1044 | [1.2182, 1.6936] |
Composite model three | 1.4962 | 0.0785 | [1.3475, 1.6431] |
Parameters | AIC | α | β | u | ξ | σ |
Parameter estimations |
10.388 | 5.7666 | 1.4296 | 2.9632 | −0.1296 | 0.7051 |
Parameters | AIC | λ | k | u | ξ | σ |
Parameter estimations |
311.518 | 8.8975 | 0.5459 | 2.9473 | −0.1103 | 0.7033 |
Parameters | AIC | μ | σL | u | ξ | σ |
Parameter estimations |
14.265 | 2.2692 | 0.4855 | 2.0800 | −0.1545 | 0.7182 |
Parameter | u | ξ | σ |
Parameter estiamtion | 7.0469 | −0.1841 | 0.0202 |
return period(year) | return level(Ms) xp | |
Composite model two | 3 | 6.33 |
5 | 6.64 | |
10 | 7.05 | |
30 | 7.68 | |
50 | 7.98 | |
100 | 8.24 |