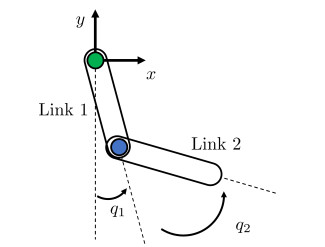
This paper considers a model-free control approach to Euler-Lagrange equations and proposes a new quantitative performance measure with its Lyapunov-based computation method. More precisely, this paper aims to solve a trajectory tracking problem for uncertain Euler-Lagrange equations by using a model-free controller with a proportional-integral-derivative (PID) control form. The L∞-gain is evaluated for the closed-loop systems obtained through the feedback connection between the Euler-Lagrange equation and the model-free controller. To this end, the input-to-state stability (ISS) for the closed-loop systems is first established by deriving an appropriate Lyapunov function. The study further extends these arguments to develop a computational approach to determine the L∞-gain. Finally, the theoretical validity and effectiveness of the proposed quantitative performance measure are demonstrated through a simulation of a 2-degree-of-freedom (2-DOF) robot manipulator, which is one of the most representative examples of Euler-Lagrange equations.
Citation: Hae Yeon Park, Jung Hoon Kim. Model-free control approach to uncertain Euler-Lagrange equations with a Lyapunov-based L∞-gain analysis[J]. AIMS Mathematics, 2023, 8(8): 17666-17686. doi: 10.3934/math.2023902
[1] | Taewan Kim, Jung Hoon Kim . A new optimal control approach to uncertain Euler-Lagrange equations: H∞ disturbance estimator and generalized H2 tracking controller. AIMS Mathematics, 2024, 9(12): 34466-34487. doi: 10.3934/math.20241642 |
[2] | Hyung Tae Choi, Jung Hoon Kim . An L∞ performance control for time-delay systems with time-varying delays: delay-independent approach via ellipsoidal D-invariance. AIMS Mathematics, 2024, 9(11): 30384-30405. doi: 10.3934/math.20241466 |
[3] | Chunjuan Hou, Zuliang Lu, Xuejiao Chen, Fei Huang . Error estimates of variational discretization for semilinear parabolic optimal control problems. AIMS Mathematics, 2021, 6(1): 772-793. doi: 10.3934/math.2021047 |
[4] | Huijuan Li . Input-to-state stability for discrete-time switched systems by using Lyapunov functions with relaxed constraints. AIMS Mathematics, 2023, 8(12): 30827-30845. doi: 10.3934/math.20231576 |
[5] | Yuxin Lou, Mengzhuo Luo, Jun Cheng, Xin Wang, Kaibo Shi . Double-quantized-based H∞ tracking control of T-S fuzzy semi-Markovian jump systems with adaptive event-triggered. AIMS Mathematics, 2023, 8(3): 6942-6969. doi: 10.3934/math.2023351 |
[6] | Miao Xiao, Zhe Lin, Qian Jiang, Dingcheng Yang, Xiongfeng Deng . Neural network-based adaptive finite-time tracking control for multiple inputs uncertain nonlinear systems with positive odd integer powers and unknown multiple faults. AIMS Mathematics, 2025, 10(3): 4819-4841. doi: 10.3934/math.2025221 |
[7] | Xiao Yu, Yan Hua, Yanrong Lu . Observer-based robust preview tracking control for a class of continuous-time Lipschitz nonlinear systems. AIMS Mathematics, 2024, 9(10): 26741-26764. doi: 10.3934/math.20241301 |
[8] | Linni Li, Jin-E Zhang . Input-to-state stability of nonlinear systems with delayed impulse based on event-triggered impulse control. AIMS Mathematics, 2024, 9(10): 26446-26461. doi: 10.3934/math.20241287 |
[9] | Chuang Liu, Jinxia Wu, Weidong Yang . Robust H∞ output feedback finite-time control for interval type-2 fuzzy systems with actuator saturation. AIMS Mathematics, 2022, 7(3): 4614-4635. doi: 10.3934/math.2022257 |
[10] | Xinyu Li, Wei Wang, Jinming Liang . Improved results on sampled-data synchronization control for chaotic Lur'e systems. AIMS Mathematics, 2025, 10(3): 7355-7369. doi: 10.3934/math.2025337 |
This paper considers a model-free control approach to Euler-Lagrange equations and proposes a new quantitative performance measure with its Lyapunov-based computation method. More precisely, this paper aims to solve a trajectory tracking problem for uncertain Euler-Lagrange equations by using a model-free controller with a proportional-integral-derivative (PID) control form. The L∞-gain is evaluated for the closed-loop systems obtained through the feedback connection between the Euler-Lagrange equation and the model-free controller. To this end, the input-to-state stability (ISS) for the closed-loop systems is first established by deriving an appropriate Lyapunov function. The study further extends these arguments to develop a computational approach to determine the L∞-gain. Finally, the theoretical validity and effectiveness of the proposed quantitative performance measure are demonstrated through a simulation of a 2-degree-of-freedom (2-DOF) robot manipulator, which is one of the most representative examples of Euler-Lagrange equations.
Motion control is an essential aspect of industrial systems, wherein achieving accurate trajectory tracking represents a primary objective. In order to achieve high accuracy in motion control, a number of methods have been introduced in the context of industrial systems [1] by noting that the dynamics of physical systems are generally described by Euler-Lagrange equations. The control approaches discussed in that study can be interpreted as providing various model-based methods based on the position control mode, in which position, velocity and torque controllers are connected in series so that torque set-point and velocity set-point is generated by the velocity and position controllers, respectively. Motivated by the success in achieving high tracking performances via the control methods in [1], a number of model-based approaches have been developed subsequently to tackle various control problems in Euler-Lagrange equations, e.g., adaptive control [2,3], disturbance observer [4], sliding mode control [5,6], neural network control [7] and so on. More interestingly, the adaptive control approach is also significantly extended in [8] by taking a leakage-type adaptive law tailored to solving input saturation problems in Euler-Lagrange systems.
Even though some important properties in control systems such as stability and tracking performance could be often achieved by using the aforementioned model-based control approaches, it should be required for taking these approaches to derive accurate mathematical models of the control systems. However, obtaining exact dynamic information of real control systems becomes more difficult as the size and complexity of the control systems become larger. For such large-scale systems, there should exist inescapable errors between the nominal model and the real dynamics, and they often lead to instability and/or performance deterioration for the feedback systems between the real system and a controller obtained through a model-based approach. To solve this difficulty in taking model-based control approaches, there have been various studies on robust control [9,10]. However, the relevant model uncertainties should be characterized for employing these studies in terms of norm-boundness, structurality and so on. Hence, this requirement makes it difficult to directly apply the arguments in robust control [9,10] to real systems.
As an intrinsic method for alleviating the difficulty occurring from the above model-based control approaches, one could take the model-free control approaches as in [11,12]. Some dynamic characteristics for real systems, such as a positive definiteness of the inertia matrix and a skew-symmetric property relevant to the inertia matrix and Coriolis matrix in Euler-Lagrange equations, are taken in those studies for ensuring the convergence of the associated state trajectory and/or the stability and robustness of the corresponding closed-loop systems, although the overall model information is not employed in the model-free control approaches. More interestingly, the control inputs in the model-free control architectures are usually generated in a heuristic fashion, based on the online or offline input/output data from the real systems. However, it is also a non-trivial task to establish an immediately applicable control law as a model-free structure from such input/output data due to a number of processes of trial and error.
In connection with this, one of the most representative and readily applicable model-free control methods is the proportional-integral-derivative (PID) control, which is also often applied to a number of control systems, irrespective of the associated system models. For instance, two-layer structure optimal setting control systems with exogenous disturbances are considered with PID control law for a bottom loop in [13], the nonlocal boundary condition for parabolic reaction-diffusion equations with PID controllers is tackled in [14], a PID control law for affine-nonlinear uncertain dynamical systems is considered in [15], an observer-based adaptive PID controller subject to cyberattacks is discussed in [16] and an event-triggered PID controller with respect to quadrotors is introduced in [17].
To clarify some theoretical effectiveness of applying the PID controller to Euler-Lagrange equations, the global asymptotic stability (GAS) for Euler-Lagrange equations equipped with a PID controller is discussed [18,19,20,21], in which there is no consideration of external disturbances. A concept of input-to-state stability (ISS) with the consideration of external disturbances is introduced in [22] for the first time, and this issue is further theoretically tackled in [23]. More precisely, the notion of ISS means that the states of the systems are bounded even if unknown bounded disturbances, such as parameter perturbations and model uncertainties, exist. Those studies [22,23] further shed new light on the arguments relevant to the disturbance ISS for nonlinear systems [24,25,26,27]. Roughly speaking, the stability of nonlinear systems could be established by constructing adequate Lyapunov functions in the employment of the ISS or disturbance ISS, in which the arguments on Lyapunov stability [28,29] play significant roles in deriving the relevant assertions.
Furthermore, the Lyapunov arguments with respect to the stability for Euler-Lagrange equations could be more sophisticated by using the arguments on passivity [28,29], in which it is intrinsically assumed that the signals considered in systems have finite energy, i.e., those of finite L2 norms. In this sense, the applications of passivity-based arguments to Euler-Lagrange equations are confined to themselves to the treatment of decaying signals in the time-domain. However, it is quite practically meaningful to take the corresponding performance specifications on the time-domain bound for the relevant error signals in Euler-Lagrange equations, i.e., the L∞ norm, rather than taking the L2 norm of those signals. More importantly, there is no discussion on the L∞-gain with respect to Euler-Lagrange equations in aforementioned studies because it is quite difficult to lead to an analytic form for the L∞-gain with respect to nonlinear systems, while the L∞-induced norm for linear equations is deeply studied in [30,31,32,33].
With this in mind, this paper aims at deriving sophisticated arguments on the L∞-gain of the closed-loop systems obtained by connecting the Euler-Lagrange equation and a PID control law. To do this, we first consider a trajectory tracking problem of Euler-Lagrange equations and develop a sort of PID control law for achieving a desired tracking performance. By noting the dynamical properties of the Euler-Lagrange equation, we also deal with the disturbance ISS associated with the provided PID controller by constructing a relevant Lyapunov function. At the same line, we propose a new quantitative performance measure for Euler-Lagrange equations by re-interpreting the corresponding Lyapunov function through the L∞ norm of signals. To put it concisely, the L∞-gain is taken as the quantitative performance measure for dealing with the corresponding trajectory tracking errors caused by unknown elements in the time-domain bounds. More importantly, the quantitative performance measure (i.e., the L∞-gain) of the closed-loop systems could be obtained by computing the minimum eigenvalue of an adequately constructed positive definite matrix. A computational approach to taking the corresponding control parameters is also derived based on the quantitative performance measure.
To summarize, the contributions of this paper on a model-free control approach to Euler-Lagrange equations are as follows.
● Synthesis of an effective PID control law: A readily applicable model-free controller for the Euler-Lagrange equations is introduced.
● Disturbance ISS: The disturbance ISS for the closed-loop systems obtained through the feedback connection between the Euler-Lagrange equations and the PID controller is ensured by deriving an adequate Lyapunov function.
● Quantitative performance measure: With respect to the characteristics of desired control objectives in Euler-Lagrange equations, the L∞-gain for the aforementioned closed-loop systems is defined.
● Performance analysis: The explicit method for computing the above L∞ gain is established.
● Computational approach to taking parameters: We introduce a computational approach to taking the corresponding control parameters based on the quantitative performance measure together with its computation method.
Finally, it should be remarked that some earlier results of this paper were partially presented at the conference [34], but they are limited to the PD control law and no computational approach to taking the relevant parameters was provided in that study. In this sense, the arguments derived in this paper can be regarded as generalized and extended versions of those in [34].
This paper is organized as follows. We first introduce the underlying mathematical notations used in this paper in Section 2. We next formulate the problem definition relevant to a trajectory tracking problem of Euler-Lagrange equations in Section 3. A new quantitative performance measure (i.e., the L∞-gain) for Euler-Lagrange equations together with its computation method is given in Section 4. We then verify in Section 5 the effectiveness and validity of the main results through a simulation result associated with a trajectory tracking problem of a 2-degree-of-freedom (2-DOF) robot manipulator, whose dynamics are one of the most representative examples of Euler-Lagrange equations. Finally, conclusion remarks are given in Section 6.
This section provides the underlying mathematical notations used for establishing the main results in this paper.
We denote the sets of ν-dimensional real numbers, ν-dimensional nonnegative real numbers and ν-dimensional symmetric matrices by Rν, Rν+ and Sν, respectively. The notation ≻(⪰) is used for M1,M2∈Sν to mean the binary relation such that
M1≻M2(M1⪰M2)⇔xT(M1−M2)x>0 (xT(M1−M2)x≥0),∀x∈Rν(x≠0). |
In other words, M1≻(⪰)M2 implies that M1−M2 is a positive (semi-)definite matrix. Furthermore, we denote the minimum eigenvalue of a matrix (⋅) and the ν-dimensional identity matrix by λmin(⋅) and Iν, respectively.
We denote the Euclidean-norm of a vector f(t)∈Rν by |f(t)|2, i.e.,
|f(t)|2:=(ν∑i=0f2i(t))1/2=(fT(t)f(t))1/2 |
while the ∞-norm of a vector f(t)∈Rν is denoted by |⋅|∞, i.e.,
|f(t)|∞:=max1≤i≤ν|fi(t)| |
where fi(t) implies the ith element of the vector f(t). With respect to this, we use the notation ‖⋅‖∞ for the L∞ norm of a real-valued vector function, i.e.,
‖f‖∞:=esssup0≤t<∞max1≤i≤ν|fi(t)|=esssup0≤t<∞|f(t)|∞. |
We next introduce the classes of functions considered in this paper as follows [28,29]. A function β(⋅):R+→R+ is said to be of class K, if it is continuous, strictly increasing and β(0)=0. A function β(⋅):R+→R+ is said to be of class K∞, if it satisfies all the conditions of class K as well as the condition that β(t)→∞ as t→∞. A function ξ(⋅,⋅):R+×R+→R+ is said to be of class KL, if ξ(⋅,t) is of class K for each fixed t, ξ(s,⋅) is monotonically decreasing and converges to 0 as t→∞ for each fixed s, i.e., ξ(s,t)→0 (t→∞).
By using these notations, we also introduce some fundamental properties of the disturbance input-to-stability (ISS) as follows [22,23,24,25].
Definition 1. Let us consider the system
˙x(t)=A(x,t)x(t)+B1(x,t)w(t)+B2(x,t)u(t) | (2.1) |
where x(t)∈Rn is the state vector, w(t)∈Rnw is the exogenous input vector and u(t)∈Rnu is the control input vector. The disturbance ISS is defined for this system when there exist a class K function β and a class KL function ξ such that the solution for (2.1) exists for all t≥0 and satisfies
|x(t)|2≤β(sup0≤s≤t|w(s)|2)+ξ(|x(0)|2,t) | (2.2) |
for an arbitrary x(0)∈Rn and for a bounded exogenous input function w(⋅).
Remark 1. The disturbance ISS can be also regarded as a generalized version of the global asymptotic stability (GAS) since the inequality (2.2) is essentially equivalent to the GAS for the case of w≡0.
Because a direct application of the arguments in Definition 1 in establishing the disturbance ISS for Euler-Lagrange equations is quite difficult, we introduce the following necessary and sufficient condition, by which the difficulty in the employment of disturbance ISS would be alleviated.
Lemma 1. The system described by (2.1) is said to be disturbance input-to-state stable, if and only if there exist a positive definite and radially unbounded function V(x,t), a class K function β1, and a class K∞ function β2 such that the following inequality holds:
dV(x,t)dt≤β1(|w(t)|2)−β2(|x(t)|2). | (2.3) |
Furthermore, this radially unbounded function V(x,t) is called also an ISS-Lyapunov function for the system given by (2.1).
Remark 2. The implication of taking (2.3) could be also interpreted as ensuring the fact that all trajectories with respect to nonlinear systems satisfying the disturbance ISS should converge to a specific ball centered at the origin x=0, in which the radius is determined depending on the L∞ norm of w. In other words, such a ball establishes also the attractive property [29] for systems with the disturbance ISS property.
Based on Lemma 1, the disturbance ISS for Euler-Lagrange equations equipped with a PID control law would be dealt with in the subsequent sections.
The problem definition to be tackled in this paper is formulated in this section. Let us first take the n-dimensional Euler-Lagrange equation given by
M(q(t))¨q(t)+C(q(t),˙q(t))˙q(t)+g(q(t))=τ(t)+d(t) | (3.1) |
where M(q(t))∈Rn×n is the Inertia matrix, C(q(t),˙q(t))∈Rn×n is the Coriolis and centrifugal torques matrix, g(q(t))∈Rn is the gravitational torque vector, q(t)∈Rn is the n-dimensional generalized coordinates, τ(t)∈Rn is the control input torque vector and d(t)∈Rn is the unknown disturbance vector.
For this Euler-Lagrange equation (3.1), we aim to achieve the trajectory tracking performance described by
q(t)→qd(t),(t→∞) | (3.2) |
where qd(t) is the reference trajectory determined depending on the desired control objectives. With respect to quantitative interpretations of the above trajectory tracking performance, we also define the tracking error as e(t):=qd(t)−q(t). Then, we say the Euler-Lagrange equation of (3.1) satisfies the tracking performance of (3.2) if the L∞ norm ‖e‖∞ is bounded. Subsequently, the tracking performance of the Euler-Lagrange equation (3.1) is said to be improved when the maximum of the L∞ norm ‖e‖∞ becomes smaller.
As a preliminary step to establishing methods for tackling the above performance tracking problem, we introduce the following properties of Euler-Lagrange equations (3.1) [35]:
(i) q(⋅),˙q(⋅),¨q(⋅),qd(⋅),˙qd(⋅),¨qd(⋅),d(⋅) are assumed to be continuous and bounded functions of t with the zero initial condition.
(ii) 0≺M and there exist constants m∗,m∗∈R+ such that
m∗In⪯M(q(t))⪯m∗In, ∀q(t)∈Rn. | (3.3) |
(iii) There exist constants c∗,g∗∈R+ such that
|C(q(t),x)y|2≤c∗|x|2|y|2,∀q(t),x,y∈Rn, | (3.4) |
|g(q(t))|2≤g∗, ∀q(t)∈Rn. | (3.5) |
(iv) ˙M=C+CT and all the initial conditions C(q(0),˙q(0)),g(q(0)) are zeros.
This section provides the main results of this paper, i.e., a PID control law, which is readily applicable to Euler-Lagrange equations, together with the relevant arguments on the disturbance ISS and the L∞-gain analysis. These results could be further extended to deriving a computational approach to determining the corresponding control parameters. To this end, this section is divided into two subsections, the arguments associated with the PID control law and the computational approach to determining control parameters, respectively.
In this subsection, we are in a position to consider the PID control law given by
˙ϵ(t)=e(t), | (4.1) |
τ(t)=α(KIϵ(t)+KPe(t)+˙e(t))=:αh(t) | (4.2) |
where α>0 is a scalar parameter, KI and KP are diagonal positive definite matrices such that K2P≻2KI. Because the PID control law given by (4.2) is used in this paper, it is natural to consider the behavior of the signals ϵ(t), e(t) and ˙e(t) when we proceed to construct the corresponding mathematical proofs.
As a preliminary step to prove the disturbance ISS associated with the PID control law described by (4.2), we define the exogenous input vector w(t)∈Rn as
w(t):=M(q(t))˙s(t)+C(q(t),˙q(t))s(t)+g(q(t))−d(t) | (4.3) |
where
s(t):=˙qd(t)+KPe(t)+KIϵ(t). | (4.4) |
Then, defining the state vector x(t)∈R3n as
x(t):=[ϵT(t)eT(t)˙eT(t)]T | (4.5) |
leads to the state-space equation described by
˙x(t)=A(x,t)x(t)+B(x,t)w(t)−B(x,t)τ(t) | (4.6) |
where
A(x,t):=[0I000I−M−1CKI−M−1CKP−KI−M−1C−KP], | (4.7) |
B(x,t):=[00M−1]. | (4.8) |
One of the main contributions in this paper is to show that the closed-form equation obtained by connecting the state-space equation (4.6) with the PID control law (4.2) satisfies the disturbance ISS. With respect to this, let us consider the Lyapunov function V(x,t) defined as
V(x,t)=12xTPx | (4.9) |
where
P:=[KIMKI+αKIKPKIMKP+αKIKIMKPMKI+αKIKPMKP+αKPKPMMKIMKPM]. |
Here, V(x,t) is a obviously positive function for all x∈R3n, because it can also be represented by
V(x,t)=12hTMh+12[ϵe]T[αKIKPαKIαKIαKP][ϵe]. | (4.10) |
Based on this representation, we give the following theorem relevant to the disturbance ISS.
Theorem 1. The closed-form equation obtained by connecting the state-space equation (4.6) and the PID control law (4.2) satisfies disturbance ISS.
Proof. Let us first note from (4.2) and (4.4) that
s(t)=h(t)+˙q(t). | (4.11) |
Substituting this into (4.3) derives that
w(t)=M(q(t))(˙h(t)+¨q(t))+C(q(t),˙q(t))(h(t)+˙q(t))+g(q(t))−d(t)=M(q(t))¨q(t)+C(q(t),˙q(t))˙q(t)+g(q(t))−d(t)+M(q(t))˙h(t)+C(q(t),˙q(t))h(t). | (4.12) |
This admits from (3.1) the representation
M(q(t))˙h(t)+C(q(t),˙q(t))h(t)=w(t)−τ(t). | (4.13) |
Combining (4.13) with (4.2) also leads to
M(q(t))˙h(t)+C(q(t),˙q(t))h(t)=w(t)−αh(t). | (4.14) |
On the other hand, we can obtain from (4.10) that
˙V(x,t)=12(˙hTMh+hTM˙h+hT˙Mh+[e˙e]T[αKIKPαKIαKIαKP][ϵe]+[ϵe]T[αKIKPαKIαKIαKP][e˙e])=hTM˙h+12hT˙Mh+[ϵe]T[αKIKPαKIαKIαKP][e˙e], | (4.15) |
since KI and KP are diagonal matrices. This further admits from the assertion (iv) that
˙V(x,t)=hTM˙h+12hT˙Mh+[ϵe]T[αKIKPαKIαKIαKP][e˙e]=hTM˙h+12hT(C+CT)h+[ϵe]T[αKIKPαKIαKIαKP][e˙e]=hT(M˙h+Ch)+[ϵe]T[αKIKPαKIαKIαKP][e˙e]. | (4.16) |
Then, substituting (4.14) into (4.16) leads to
˙V(x,t)=hT(M˙h+Ch)+[ϵe]T[αKIKPαKIαKIαKP][e˙e]=hTw−αhTh+[ϵe]T[αKIKPαKIαKIαKP][e˙e]=hTw−αhTh+α2xT[0KIKPKIKIKP2KIKPKIKP0]x=hTw−α2hTh−α2hTh+α2xT[0KIKPKIKIKP2KIKPKIKP0]x=hTw−α2hTh−α2xT[KIKPIn]T[KIKPIn]x+α2xT[0KIKPKIKIKP2KIKPKIKP0]x=hTw−α2hTh−α2xT[K2I000(K2P−2KI)000In]x. | (4.17) |
Furthermore, if we note from the AM–GM inequality that
hTw≤α2hTh+12αwTw, | (4.18) |
then (4.17) can be further represented by
˙V(x,t)=hTw−α2hTh−α2xT[K2I000(K2P−2KI)000In]x≤12αwTw−α2xT[K2I000(K2P−2KI)000In]x≤12α|w(t)|22−α2η⋅|x(t)|22 | (4.19) |
where
η:=min{λmin(K2I),λmin((K2P−2KI)),λmin(In)}. | (4.20) |
Because 12α|w(t)|22 and α2η|x(t)|22 are class K∞ functions of |w(t)|2 and |x(t)|2, respectively, it is obvious from (4.19) and Lemma 1 that the closed-form equation obtained by (4.6) and (4.2) satisfies the disturbance ISS. This completes the proof.
The disturbance ISS discussed in Theorem 1 clearly implies that the state vector signal x(⋅) is bounded if the exogenous vector signal w(⋅) is bounded, i.e.,
‖w‖∞<∞⇒‖x‖∞<∞. | (4.21) |
Next, we are concerned with computing the L∞-gain of the closed-form equation. With this regard, the performance index function γ(t) is first defined as the ∞-norm of the state vector x(t) when ˙V(x,t)≥0. Otherwise, γ(t) is defined as 0 (i.e., when ˙V(x,t)<0). Based on the performance index function, we introduce the following theorem relevant to the L∞-gain of the closed-form equation.
Theorem 2. Denote a lower bound of α by α0(>0) and decompose α as α=α0+α1 with α1>0. We also define the matrix T as
T:=[2K2IKIKPKIKPKI2(K2P−KI)KPKIKP2In]. |
Then, the following inequality holds:
‖γ‖∞≤√nα0⋅√1α1λmin(T)‖w‖∞. | (4.22) |
Proof. Similarly for the proof of Theorem 1, let us first note that
hTw≤α02hTh+12α0wTw. | (4.23) |
Then, we could see from (4.17) that
˙V(x,t)=hTw−α2hTh−α2xT[K2I000(K2P−2KI)000In]x≤12α0wTw−α12hTh−α12xT[K2I000(K2P−2KI)000In]x=12α0wTw−α12xT([KIKPIn]T[KIKPIn]+[K2I000(K2P−2KI)000In])x=12α0wTw−α12xTTx≤12α0wTw−α12λmin(T)xTx. | (4.24) |
From the fact that |w(t)|22≤n|w(t)|2∞ and −|x(t)|22≤−|x(t)|2∞, (4.24) can be further simplified to
˙V(x,t)≤n2α0|w(t)|2∞−α12λmin(T)|x(t)|2∞≤n2α0‖w‖2∞−α12λmin(T)|x(t)|2∞. | (4.25) |
Since (4.25) corresponds to deriving an upper bound of ˙V(x,t), the performance index vector γ(t) does not exceed the ∞-norm |x(t)|∞, which makes the right-hand side (RHS) of (4.25) zero. This completes the proof.
This paper is in a position to take the RHS of (4.22) as the Lyapunov-based quantitative performance measure associated with suppressing the maximum amplitude of the trajectory tracking error x, i.e., ‖x‖∞. Furthermore, it can be concluded from Theorem 2 that the L∞ norm ‖γ‖∞ is decreasing within a convergence order, which is not slower than 1/√α1λmin(T). This implication is expected to establish that ‖x‖∞ is also decreasing in a convergence order no smaller than 1/√α1λmin(T). Such an expectation together with the validity of the Lyapunov-based quantitative performance measure for reducing ‖x‖∞ will be demonstrated through simulation results in Section 5.
This subsection is concerned with introducing a computational approach to determining the corresponding control parameters in (4.2). We can see from (4.2) that the parameters KP and KI could be interpreted as weighting constants of e and ϵ, respectively, while the scalar parameter α>0 plays the role of a common weighting constant of KPe,KIϵ and ˙e. Here, it should be remarked from the proof of Theorem 1 that it is sufficient to take the scalar parameter α larger than 0 as well as the positive diagonal matrices KP and KI when we are only interested in the disturbance ISS.
With respect to more constructive methods for determining the control parameters rather than the above arguments, the parameters α,KP and KI could be determined as follows. Based on the fact that the scalar parameter α is decomposed as α=α0+α1 where α0 denotes an lower bound of α, a relatively small α0>0 is first selected to guarantee the disturbance ISS for the corresponding closed-loop system. Once α0 is fixed, the next step is to select a relatively small initial value of α1, for instance, α1=1 or α1=2. If the initial value of α1 is also selected, it is required to take KP and KI by which KI‖ϵ‖∞,KP‖e‖∞ and ‖˙e‖∞ have a similar order of accuracy. After KP and KI are also fixed, the value of α1 would be modulated by using the Lyapunov-based performance measure (4.22) such that max{‖ϵ‖∞,‖e‖∞,‖˙e‖∞}=‖x‖∞ becomes small corresponding to a control specification desired by the users. This might be achieved by taking larger α1 for fixed α0,KP and KI.
This section verifies the validity and effectiveness of the main results in this paper, i.e., the arguments of Theorems 1 and 2, through a simulation result for the 2-degree-of-freedom (2-DOF) planar robot manipulator Σ as shown in Figure 1, whose dynamics is one of the most representative examples of Euler-Lagrange equations.
Let us assume that the dynamics of Σ is described by
M(q(t))¨q(t)+C(q(t),˙q(t))˙q(t)+g(q(t))=τ(t)+d(t) |
with
M(q(t))=[7.96+2.4cos(q2(t))0.96+1.2cos(q2(t))0.96+1.2cos(q2(t))5.96],C(q(t),˙q(t))=[−1.2sin(q2(t))˙q2(t)−1.2sin(q2(t))(˙q1(t)+˙q2(t))1.2sin(q2(t))˙q1(t)0],g(q(t))=[11.77sin(q1(t)+q2(t))+19.62sin(q1(t))11.77sin(q1(t)+q2(t))] |
where qi(t) (i=1,2) is the ith joint position and the values of model parameters are obtained from [8,36]. We also assume that the disturbance vector as shown in Figure 2 is applied to this 2-DOF planar robot manipulator and the reference trajectories are described by the the periodic functions
qd1(t)={−1π2sin(π2t)+12πt0≤t≤4,1π2sin(π2t)−12π(t−8)4≤t≤8,,qd2(t)={−1π2sin(πt)+1πt0≤t≤2,1π2sin(πt)−1π(t−4)2≤t≤4. |
To take into account a practical situation relevant to uncertain elements, the velocity measurements are also assumed to be obtained from tachometers in each link with noise, i.e.,
y(t)=[q(t)˙q(t)]+[0n(t)] |
with the normal distribution given by n∼N(0,5⋅10−3).
This section provides some simulation results to examine the effectiveness of Theorems 1 and 2. More precisely, the arguments with respect to the disturbance ISS and the arguments with respect to the convergence rate for ‖x‖∞ are verified through the simulation results.
Based on the computational approach introduced in Subsection 4.2, we are concerned with the PID control law given by
τ=(α0+α1)(˙e+KPe+KIϵ) | (5.1) |
where the corresponding control parameters are determined to be α0=2, KP=15I2 and KI=15I2 with the initial value of α1 as 4. With these control parameter values, the feedback system obtained by connecting (4.6) and (4.2) is expected to satisfy the disturbance ISS by the arguments in Theorem 1. With respect to the arguments in Theorem 2, the L∞ norm ‖x‖∞ is further expected to be smaller as α1 increases because taking α1 larger obviously makes α1λmin(T) in (4.22) become larger. In this respect, we also take α1=8, α1=16 and α1=32 to examine the validity of the convergence rate for ‖x‖∞ corresponding to taking larger α1 mentioned above.
The simulation results for the real position vector q(t)∈R2 and the reference position vector qd(t)∈R2 are shown in Figure 3, while the results for the accumulated position error vector ϵ(t)∈R2, the position error vector e(t)∈R2, the velocity error vector ˙e(t)∈R2 and the control input torque vector τ(t)∈R2 are shown in Figures 4–7, respectively. Furthermore, the simulation results for the L∞ norm ‖x‖∞ and √1α1λmin(T) are shown in Table 1.
α1 (α0=2) | 4 | 8 | 16 | 32 |
‖x‖∞ | 0.9483 | 0.3451 | 0.1622 | 0.0702 |
√1α1λmin(T) | 0.4359 | 0.3082 | 0.2180 | 0.1541 |
We can observe from Figure 3 that the arguments in Theorem 1 with respect to the disturbance ISS are verified for all the taken control parameter values since the corresponding tracking errors are bounded. More importantly, we can also see from Figure 3 that making α1 larger leads to an improved accuracy for the considered trajectory tracking problem. Furthermore, it can be observed from Figures 4–6 that all the peak magnitudes of the accumulated position error ϵ(t), the position error e(t) and the velocity error ˙e(t) are reduced by making α1 larger. The aforementioned intuitive implication of the results in Theorem 2 with respect to the convergence rate for ‖x‖∞ might be verified through these observations. Regarding a more sophisticated interpretation of the simulation results in a theoretical side, we note from the results in Table 1 that the L∞ norm ‖x‖∞ decreases within a order no slower than 1/√α1λmin(T). This clearly demonstrates the effectiveness of the arguments in Theorem 2.
This section provides some simulation results to examine the superiority of the proposed method over the conventional method obtained by combining the PID control and the computed-torque control (CTC) [37]. This conventional method is called the PID-CDC throughout the paper, and it is described by
˙ϵ=e(t), | (5.2) |
τ=ˆM(q)(¨qd+K[c]D˙e+K[c]Pe+K[c]Iϵ)+ˆC(q(t),˙q(t))˙q+ˆg(q(t)) | (5.3) |
where ˆM,ˆC and ˆg are the estimated (i.e., nominal) values of M,C and g, respectively, and the control parameters K[c]d,K[c]p and K[c]I are diagonal positive definite matrices. Assuming that the accurate dynamic model cannot be derived due to some model uncertainties, we take the estimated values of M,C and g respectively as
ˆM(q(t))=[9.52+2.88cos(q2(t))1.15+1.44cos(q2(t))1.15+1.44cos(q2(t))7.15],ˆC(q(t),˙q(t))=[−1.44sin(q2(t))˙q2(t)−1.44sin(q2(t))(˙q1(t)+˙q2(t))1.44sin(q2(t))˙q1(t)0],ˆg(q(t))=[14.12sin(q1(t)+q2(t))+23.54sin(q1(t))14.12sin(q1(t)+q2(t))]. |
Finally, the control parameters are taken by K[c]d=2ωcI2, K[c]p=ω2cI2 and K[c]I=2ω3cI2 with the cut-off frequency ωc.
We first take the same parameter values for the proposed controller as in the last case of the previous subsection, i.e., α0=2,α1=32,KP=15I2 and KI=15I2. To make a comparison between the proposed and conventional controllers fair, we then determine the control parameters for the latter, by which both the tracking performances relevant to the two controllers become similar to each other. In other words, we take the cut-off frequency ωc in K[c]d,K[c]p and K[c]I to make the L∞ norm of the state x to be close to that of the case of α1=32 in Table 1, i.e., ‖x‖∞=0.07. The simulation results for the real position vector q(t)∈R2 and the reference position vector qd(t)∈R2 are shown in Figure 8(a), while the control input torque vector τ(t)∈R2 are shown in Figure 8(b). In a quantitative comparison between the proposed and conventional controllers, the simulation results for ‖x‖∞, ‖Δτ‖∞ and ‖τ‖∞ are shown in Table 2, where Δτ is the input difference in a unit time defined as Δτ:=τ(t+δ)−τ(t) with the operating period δ in the controllers.
‖x‖∞ | ‖Δτ‖∞ | ‖τ‖∞ | |
Proposed control law | 0.0702 | 14.00 | 32.38 |
Conventional control law | 0.0701 | 26.42 | 40.52 |
It could be observed from Figure 7(a) and Figure 8(b) that the torque input from the conventional controller is more turbulent than that from the proposed controller, although both controllers achieve similar tracking performances as observed from Figure 3(a) and Figure 8(a) as well as Table 2.
This tendency can be more obviously observed from Table 2 by showing that the peak values of the input difference and the torque input in the conventional controller are quite larger than those of the proposed controller; the peak values of the proposed controller are about 53 and 80 of those of the conventional controller, respectively.
We can conclude from these observations that the proposed controller would lead to more smooth control actions even for similar tracking performances to the conventional controller. More importantly, obtaining the exact values of M,C and g becomes more difficult as the dimension of Euler-Lagrange equations increases, and thus the proposed controller can be expected to be used more effectively for such large-scale Euler-Lagrange equations than the conventional controller. This superiority of the proposed controller over the conventional controller in the effectiveness of torque input might be arising from the fact that the parameter ˆM(q)K[c]D relevant to the derivative term in the latter is usually much larger than the similar parameter α in the former as seen from (5.3) and (4.2).
This paper was concerned with introducing a new Lyapunov-based quantitative performance measure associated with suppressing the peak magnitude of trajectory tracking errors for an Euler-Lagrange equation. We proposed a PID control law readily applicable to the Euler-Lagrange equation and evaluated the effect of disturbances on the system states by showing that the feedback system obtained through the connection between the Euler-Lagrange equation and the proposed PID control law satisfies the disturbance ISS. The main idea was to introduce an adequate Lyapunov function with respect to the disturbance ISS, and the relevant arguments could be further effectively employed for the L∞-gain analysis with respect to the corresponding trajectory tracking problem. More precisely, the relation between the associated control parameters and the L∞-gain was shown to be described in terms of the minimum eigenvalue of a suitably constructed positive definite matrix. The theoretical validity and the practical effectiveness of the overall arguments developed in this paper were demonstrated through some simulation results for a 2-DOF robot manipulator.
On the other hand, we would like to note that the tracking accuracy might be improved through an additional implementation of a friction compensator. Furthermore, an auto-tuning technique for the proposed PID controller undoubtedly contributes to wider applications of this paper to practical Euler-Lagrange equations. In this sense, it is quite meaningful to extend the arguments for deriving (4.22) tailored to a friction compensator and/or an auto-tuning algorithm for the proposed PID controller, but this issue seems to be quite difficult and is left as an interesting future work.
The authors declare they have not used Artificial Intelligence (AI) tools in the creation of this article.
This work was supported by project for Smart Manufacturing Innovation R & D funded Korea Ministry of SMEs and Startups in 2022 (Project No. RS–202200141122).
All authors declare no conflicts of interest in this paper.
[1] |
T. Brogårdh, Present and future robot control development–An industrial perspective, Annu. Rev. Control, 31 (2007), 69–79. https://doi.org/10.1016/j.arcontrol.2007.01.002 doi: 10.1016/j.arcontrol.2007.01.002
![]() |
[2] |
C. J. Chien, A. Tayebi, Further results on adaptive iterative learning control of robot manipulators, Automatica, 44 (2008), 830–837. https://doi.org/10.1016/j.automatica.2007.06.023 doi: 10.1016/j.automatica.2007.06.023
![]() |
[3] |
K. K. Tan, S. Huang, T. H. Lee, Decentralized adaptive controller design of large-scale uncertain robotic systems, Automatica, 45 (2009), 161–166. https://doi.org/10.1016/j.automatica.2008.06.005 doi: 10.1016/j.automatica.2008.06.005
![]() |
[4] |
T. Sun, L. Cheng, W. Wang, Y. Pan, Semiglobal exponential control of Euler-Lagrange systems using a sliding-mode disturbance observer, Automatica, 112 (2020), 108677. https://doi.org/10.1016/j.automatica.2019.108677 doi: 10.1016/j.automatica.2019.108677
![]() |
[5] |
P. Yang, Y. Su, Proximate fixed-time prescribed performance tracking control of uncertain robot manipulators, IEEE/ASME Trans. Mech., 27 (2022), 3275–3285. https://doi.org/10.1109/TMECH.2021.3107150 doi: 10.1109/TMECH.2021.3107150
![]() |
[6] |
S. K. Kommuri, S. Han, S. Lee, External torque estimation using higher order sliding-mode observer for robot manipulators, IEEE/ASME Trans. Mech., 27 (2022), 513–523. https://doi.org/10.1109/TMECH.2021.3067443 doi: 10.1109/TMECH.2021.3067443
![]() |
[7] |
H. Gao, W. He, C. Zhou, C. Sun, Neural network control of a two-link flexible robotic manipulator using assumed mode method, IEEE Trans. Ind. Inform., 15 (2019), 755–765. https://doi.org/10.1109/TII.2018.2818120 doi: 10.1109/TII.2018.2818120
![]() |
[8] |
K. Shao, R. Tang, F. Xu, X. Wang, J. Zheng, Adaptive sliding mode control for uncertain Euler-Lagrange systems with input saturation, J. Franklin Inst., 358 (2021), 8356–8376. https://doi.org/10.1016/j.jfranklin.2021.08.027 doi: 10.1016/j.jfranklin.2021.08.027
![]() |
[9] | M. A. Dahleh, I. J. Diaz-Bobillo, Control of uncertain systems: a linear programming approach, Prentice-Hall, 1994. |
[10] | I. S. Khalil, J. C. Doyle, K. Glover, Robust and optimal control, Prentice-Hall, 1996. |
[11] | Z. Hou, S. Jin, Model free adaptive control: theory and applications, CRC Press, 2013. |
[12] | R. E. Precup, R. C. Roman, A. Safaei, Data-driven model-free controllers, CRC Press, 2021. |
[13] |
L. Yin, Y. Zhu, Y. Xu, T. Li, Dynamic optimal operational control for complex systems with nonlinear external loop disturbances, AIMS Math., 7 (2022), 16673–16691. https://doi.org/10.3934/math.2022914 doi: 10.3934/math.2022914
![]() |
[14] |
F. Ivanauskas, V. Laurinavičius, M. Sapagovas, A. Neciporenko, Reaction-diffusion equation with nonlocal boundary condition subject to PID-controlled bioreactor, Nonlinear Anal.: Model. Control, 22 (2017), 261–272. https://doi.org/10.15388/NA.2017.2.8 doi: 10.15388/NA.2017.2.8
![]() |
[15] |
C. Zhao, L. Guo, Control of nonlinear uncertain systems by extended PID, IEEE Trans. Automat Control, 66 (2021), 3840–3847. https://doi.org/10.1109/TAC.2020.3030876 doi: 10.1109/TAC.2020.3030876
![]() |
[16] |
D. Zhao, Z. Wang. G. Wei, Q. L. Han, A dynamic event-triggered approach to observer-based PID security control subject to deception attacks, Automatica, 120 (2020), 109128. https://doi.org/10.1016/j.automatica.2020.109128 doi: 10.1016/j.automatica.2020.109128
![]() |
[17] |
X. Z. Zhu, P. Casau, C. Silvestre, Event-triggered global trajectory tracking control of a quadrotor: synthesis, simulations, and experiments, Int. J. Robust Nonlinear Control, 31 (2021), 6144–6165. https://doi.org/10.1002/rnc.5565 doi: 10.1002/rnc.5565
![]() |
[18] |
J. Alvarez-Ramirez, I. Cervantes, R. Kelly, PID regulation of robot manipulators: stability and performance, Syst. Control Lett., 41 (2000), 73–83. https://doi.org/10.1016/S0167-6911(00)00038-4 doi: 10.1016/S0167-6911(00)00038-4
![]() |
[19] |
R. Kelly, Global positioning of robot manipulators via PD control plus a class of nonlinear integral actions, IEEE Trans. Automatic Control, 43 (1998), 934–938. https://doi.org/10.1109/9.701091 doi: 10.1109/9.701091
![]() |
[20] | R. Ortega, A. Loria, R. Kelly, A semiglobally stable output feedback PI2D regulator for robot manipulators IEEE Trans. Automatic Control, 40 (1995), 1432–1436. https://doi.org/10.1109/9.402235 |
[21] |
M. Takegaki, S. Arimoto, A new feedback method for dynamic control of manipulators, ASME J. Dyn. Syst., Meas., Control, 103 (1981), 119–125. https://doi.org/10.1115/1.3139651 doi: 10.1115/1.3139651
![]() |
[22] |
E. D. Sontag, Smooth stabilization implies coprime factorization, IEEE Trans. Automatic Control, 34 (1989), 435–443. https://doi.org/10.1109/9.28018 doi: 10.1109/9.28018
![]() |
[23] |
E. D. Sontag, Y. Wang, On characterizations of the input-to-state stability property, Syst. Control Lett., 24 (1995), 351–359. https://doi.org/10.1016/0167-6911(94)00050-6 doi: 10.1016/0167-6911(94)00050-6
![]() |
[24] |
D. Angeli, Input-to-state stability of PD-controlled robotic systems, Automatica, 35 (1999), 1285–1290. https://doi.org/10.1016/S0005-1098(99)00037-0 doi: 10.1016/S0005-1098(99)00037-0
![]() |
[25] |
M. Krstic, Z. Li, Inverse optimal design of input-to-state stabilizing nonlinear controllers, IEEE Trans. Automatic Control, 43 (1998), 336–350. https://doi.org/10.1109/9.661589 doi: 10.1109/9.661589
![]() |
[26] |
R. Rao, X. Li, Input-to-state stability in the meaning of switching for delayed feedback switched stochastic financial system, AIMS Math., 6 (2021), 1040–1064. https://doi.org/10.3934/math.2021062 doi: 10.3934/math.2021062
![]() |
[27] |
T. Wei, X. Xie, X. Li, Input-to-state stability of delayed reaction-diffusion neural networks with multiple impulses, AIMS Math., 6 (2021), 5786–5800. https://doi.org/10.3934/math.2021342 doi: 10.3934/math.2021342
![]() |
[28] | H. K. Khalil, Lyapunov stability, In: Nonlinear systems, Prentice-Hall, 2002,111–194. |
[29] | M. Vidyasagar, Lyapunov stability, In: Nonlinear systems analysis, Prentice-Hall, 1993,135–269. |
[30] |
J. H. Kim, T. Hagiwara, Computing the L∞-induced norm of linear time-invariant systems via Kernel approximation and its comparison with input approximation, IET Control Theory Appl., 9 (2015), 700–709. https://doi.org/10.1049/iet-cta.2014.0453 doi: 10.1049/iet-cta.2014.0453
![]() |
[31] |
J. H. Kim, T. Hagiwara, L1 discretization for sampled-data controller synthesis via piecewise linear approximation, IEEE Trans. Automatic Control, 61 (2016), 1143–1157. https://doi.org/10.1109/TAC.2015.2452815 doi: 10.1109/TAC.2015.2452815
![]() |
[32] |
J. H. Kim, T. Hagiwara, L1 optimal controller synthesis for sampled-data systems via piecewise linear kernel approximation, Int. J. Robust Nonlinear Control, 31 (2021), 4933–4950. https://doi.org/10.1002/rnc.5513 doi: 10.1002/rnc.5513
![]() |
[33] |
M. Vidyasagar, Optimal rejection of persistent bounded disturbances, IEEE Trans. Automatic Control, 31 (1986), 527–534. https://doi.org/10.1109/TAC.1986.1104315 doi: 10.1109/TAC.1986.1104315
![]() |
[34] | J. H. Kim, S. Hur, Y. Oh, Maximum tracking errors in PD-controlled robotic manipulators, 2016 IEEE/SICE International Symposium on System Integration, 2016,676–681. https://doi.org/10.1109/SII.2016.7844077 |
[35] | M. W. Spong, S. Hutchinson, M. Vidyasagar, Dynamics, In: Robot modeling and control, John Wiley & Sons, 2020,165–214. |
[36] |
Y. Feng, X. Yu, Z. Man, Non-singular terminal sliding mode control of rigid manipulators, Automatica, 38 (2002), 2159–2167. https://doi.org/10.1016/S0005-1098(02)00147-4 doi: 10.1016/S0005-1098(02)00147-4
![]() |
[37] | F. L. Lewis, D. M. Dawson, C. T. Abdallah, Computed-torque control, In: Robot manipulator control: theory and practice, CRC Press, 2003,169–260. |
1. | Hyung Tae Choi, Jung Hoon Kim, An L∞ performance control for time-delay systems with time-varying delays: delay-independent approach via ellipsoidal D-invariance, 2024, 9, 2473-6988, 30384, 10.3934/math.20241466 | |
2. | Wei Lu, Optimization and design of electromechanical control automation based on dual motor control algorithm, 2024, 10, 2297-3079, 10.3389/fmech.2024.1485041 | |
3. | Taewan Kim, Jung Hoon Kim, A new optimal control approach to uncertain Euler-Lagrange equations: H∞ disturbance estimator and generalized H2 tracking controller, 2024, 9, 2473-6988, 34466, 10.3934/math.20241642 | |
4. | Geun Il Song, Hae Yeon Park, Jung Hoon Kim, The H∞ Robust Stability and Performance Conditions for Uncertain Robot Manipulators, 2025, 12, 2329-9266, 270, 10.1109/JAS.2024.124701 |
α1 (α0=2) | 4 | 8 | 16 | 32 |
‖x‖∞ | 0.9483 | 0.3451 | 0.1622 | 0.0702 |
√1α1λmin(T) | 0.4359 | 0.3082 | 0.2180 | 0.1541 |
‖x‖∞ | ‖Δτ‖∞ | ‖τ‖∞ | |
Proposed control law | 0.0702 | 14.00 | 32.38 |
Conventional control law | 0.0701 | 26.42 | 40.52 |