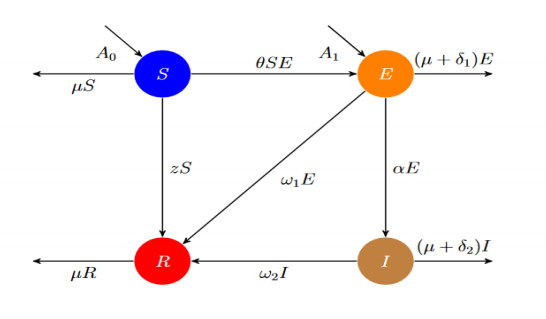
COVID-19 has become a serious pandemic affecting many countries around the world since it was discovered in 2019. In this research, we present a compartmental model in ordinary differential equations for COVID-19 with vaccination, inflow of infected and a generalized contact rate. Existence of a unique global positive solution of the model is proved, followed by stability analysis of the equilibrium points. A control problem is presented, with vaccination as well as reduction of the contact rate by way of education, law enforcement or lockdown. In the last section, we use numerical simulations with data applicable to South Africa, for supporting our theoretical results. The model and application illustrate the interesting manner in which a diseased population can be perturbed from within itself.
Citation: Peter Joseph Witbooi, Sibaliwe Maku Vyambwera, Mozart Umba Nsuami. Control and elimination in an SEIR model for the disease dynamics of COVID-19 with vaccination[J]. AIMS Mathematics, 2023, 8(4): 8144-8161. doi: 10.3934/math.2023411
[1] | Xiaoying Pan, Longkun Tang . A new model for COVID-19 in the post-pandemic era. AIMS Mathematics, 2024, 9(8): 21255-21272. doi: 10.3934/math.20241032 |
[2] | Moh. Mashum Mujur Ihsanjaya, Nanang Susyanto . A mathematical model for policy of vaccinating recovered people in controlling the spread of COVID-19 outbreak. AIMS Mathematics, 2023, 8(6): 14508-14521. doi: 10.3934/math.2023741 |
[3] | C. W. Chukwu, Fatmawati . Modelling fractional-order dynamics of COVID-19 with environmental transmission and vaccination: A case study of Indonesia. AIMS Mathematics, 2022, 7(3): 4416-4438. doi: 10.3934/math.2022246 |
[4] | Mahmoud A. Ibrahim . Threshold dynamics in a periodic epidemic model with imperfect quarantine, isolation and vaccination. AIMS Mathematics, 2024, 9(8): 21972-22001. doi: 10.3934/math.20241068 |
[5] | Chandan Maji, Fahad Al Basir, Debasis Mukherjee, Kottakkaran Sooppy Nisar, Chokkalingam Ravichandran . COVID-19 propagation and the usefulness of awareness-based control measures: A mathematical model with delay. AIMS Mathematics, 2022, 7(7): 12091-12105. doi: 10.3934/math.2022672 |
[6] | Asma Hanif, Azhar Iqbal Kashif Butt, Tariq Ismaeel . Fractional optimal control analysis of Covid-19 and dengue fever co-infection model with Atangana-Baleanu derivative. AIMS Mathematics, 2024, 9(3): 5171-5203. doi: 10.3934/math.2024251 |
[7] | Ishtiaq Ali, Sami Ullah Khan . Dynamics and simulations of stochastic COVID-19 epidemic model using Legendre spectral collocation method. AIMS Mathematics, 2023, 8(2): 4220-4236. doi: 10.3934/math.2023210 |
[8] | Chontita Rattanakul, Inthira Chaiya . A mathematical model for predicting and controlling COVID-19 transmission with impulsive vaccination. AIMS Mathematics, 2024, 9(3): 6281-6304. doi: 10.3934/math.2024306 |
[9] | I. A. Moneim, G. A. Mosa . A realistic model for the periodic dynamics of the hand-foot-and-mouth disease. AIMS Mathematics, 2022, 7(2): 2585-2601. doi: 10.3934/math.2022145 |
[10] | Xinjie Zhu, Hua Liu, Xiaofen Lin, Qibin Zhang, Yumei Wei . Global stability and optimal vaccination control of SVIR models. AIMS Mathematics, 2024, 9(2): 3453-3482. doi: 10.3934/math.2024170 |
COVID-19 has become a serious pandemic affecting many countries around the world since it was discovered in 2019. In this research, we present a compartmental model in ordinary differential equations for COVID-19 with vaccination, inflow of infected and a generalized contact rate. Existence of a unique global positive solution of the model is proved, followed by stability analysis of the equilibrium points. A control problem is presented, with vaccination as well as reduction of the contact rate by way of education, law enforcement or lockdown. In the last section, we use numerical simulations with data applicable to South Africa, for supporting our theoretical results. The model and application illustrate the interesting manner in which a diseased population can be perturbed from within itself.
The COVID-19 pandemic has imposed a massive global challenge in public health for many countries, following its discovery early in December 2019 in Wuhan, China. Since then, the virus has rapidly spread to other provinces in China and the rest of the world. The first case of the COVID-19 disease in South Africa was reported in March 2020, [29]. Due to the increase in COVID-19 incidence, the South African government introduced strategies to control the spread of the pandemic, such as social distancing to limit close contact among individuals, contact tracing, testing and screening. On 26 March 2020, in order to curb the spread of the corona virus, the South African government enforced a national lockdown to restrict contact of individuals [7,12,17].
Prior to COVID-19, the world has already seen some serious outbreaks of disease from corona viruses, see, for instance, [23]. Mathematical models of COVID-19 disease dynamics, e.g., [6,14,18,36], have been far more complex than, for instance, the 4-compartment model in [23]. Recent published work, by way of mathematical models have shown that short term interventions such as physical distancing, hand washing and mask wearing are useful in reducing the COVID-19 case incidence [14,18,22,30]. Such non-pharmaceutical intervention were implemented world-wide during the heavy impact of COVID-19, see, for instance, in [2,3,25].
However, long term interventions including vaccination are highly desirable, given the socio-economic costs of lockdowns and physical distancing [11]. Since, vaccine was introduced, there has been much research on its effect in the control of COVID-19 in the population. Mathematical modeling studies in this regard can be found in [1,4,5,6,18,26,36]. The current paper is another contribution. We here present a compartmental model in ordinary differential equations (ODEs) for COVID-19 with a generalized contact rate and vaccination. Our paper focuses on the intervention that took place in South Africa for the reduction of the COVID-19 cases, which includes education, lockdown, law enforcement, etc., and vaccination. A detailed analysis of the model is included, and we also include an optimal control problem.
Regarding the form of the model, the closest work to ours seems to be [5]. The model in [5] has a nonlinear recovery rate. In the current paper the contact rate is more general. Our more general contact rate is inspired by the work of [10,13,24] and others. Our results have a slightly different focus from that in [5], and we also study an optimal control problem. Into the model we introduce two control functions: a control on the rate of vaccination of susceptibles and another control on the contact rate. The latter control amounts to introduction and enforcement of measures such as lockdown, social distancing, mask-wearing, sanitizing of hands and of objects and education on these preventive measures. Optimal control theory determines the best scheduling of the two strategies for the most effective results in combating COVID-19, balanced against the cost of these interventions. Our paper is one of only a few papers on optimal control of COVID-19 in South Africa [8,18,25]. These papers consider control on only non-pharmaceutical interventions. The current paper is the only one that includes vaccination in the model.
The paper is set up as follows. In Section 2 we present the model, and we prove existence of the global positive solutions. Section 3 focuses on the disease-free equilibrium point and its stability, and Section 4 deals with the endemic equilibrium point. The optimal control problem is presented in Section 5. In Section 6 we illustrate our theoretical results by way of numerical simulations with reference to South Africa. The paper is concluded in Section 7.
We consider a population of size N(t) at time t, which is subdivided into four classes. These classes are the susceptibles S(t), the infectious but asymptomatic cases E(t), the symptomatic infectious cases I(t), and recovered individuals R(t). Therefore, we have the following equation:
N(t)=S(t)+E(t)+I(t)+R(t). |
Members of the symptomatic COVID-19 class I are indeed infectious. However, they are assumed to be so effectively isolated that eventually they do not contribute significantly to the transmission of the disease. The model is displayed in the flow diagram below, as Figure 1:
We use the following notation for the (mostly constant) parameters:
P0 | The size of the population at the disease-free state |
A0 | Rate of birth of newborns, assumed to be susceptibles; Note that A0>0 |
A1 | Rate of inflow into the E-class; A1≥0 |
θ(I) | Contact rate, a function of I |
δ1 and δ2 | Disease-induced mortality rate, for E and I classes, respectively |
z | Vaccination rate |
α | Transfer rate of from E-class to I-class |
ω1 | Transfer rate of from E-class to R-class |
ω2 | Transfer rate of from I-class to R-class |
μ | The average mortality rate by natural causes. |
The contact rate is a function θ(I), of the infection class I. The vaccination rate is a non-negative constant. The rest of the parameters listed above are assumed to be positive constants. The function θ(I) is assumed to be positive-valued, and to have a continuous derivative θ′(I)≤0. We shall write:
θ0:=θ(0) and θ1:=θ′(0).
The motivation for such a contact rate is that it reflects the response of the susceptible population with respect to lower or higher values of I. The idea is that as the susceptibles become aware of the magnitude of the higher numbers of symptomatic infections, they become more cautious in terms of their contact with people, especially those suspected of being infected. This leads to a reduction in the contact rate. The model is described by the system of ODEs (2.1).
˙S=A0−θSE−(μ+z)S˙E=A1+θSE−(μ+δ1+α+ω1)E˙I=αE−(μ+δ2+ω2)I˙R=zS+ω1E+ω2I−μR. | (2.1) |
The initial conditions are:
S(0)>0,E(0)>0,I(0)>0,R(0)>0. |
We write N(t) as the human population size at time t, i.e.,
S(t)+E(t)+I(t)+R(t)=N(t).
We shall adopt the following notation:
μ0=μ+z,μ1=μ+δ1+α+ω1, μ2=μ+δ2+ω2 and (A0+A1)/μ=P,
and we introduce the set Γ,
Γ={x∈R4| xi>0, i=1,2,3,4 and x1+x2+x3+x4<P}. | (2.2) |
The following theorem is proved by contradiction, following an argument that has been popularly used, for instance, in [19,20].
Theorem 2. Given any y∈Γ, then there exists a unique solution X(t) of the system (2.1) with X(0)=y and with X(s)∈Γ for each s∈[0,∞).
Proof. Consider any point y∈Γ. Then, there exists a local solution X(t) in Γ which is a C1 function and with initial value X(0)=y. Suppose that t1 is the time of exit from Γ. Since X(t) is continuous, and Γ is an open set, it follows that t1>0. We shall show by contradiction that t1=∞. Thus, suppose that t1 is finite.
Let us define the following functions L0(X(t)) for 0≤t≤t1 (and note that for notational simplicity, we suppress the function's dependence on t).
L0(X(t))=lnPS(t)+lnPE(t)+lnPI(t)+lnPR(t)+ln(PP−N(t)). | (2.3) |
Each term on the right-hand side of equation (2.3) is a non-negative valued function of time t for 0≤t<t1. We note that for each of the first four terms on the right-hand side of equation (2.3) above, if any of the Xi(t) tends to 0 as t→t1, then L0(X(t))→∞. We also note that for a positive constant c and a variable z with 0<z<c,
limz→0+{ln[c/z]}=+∞. |
Therefore, if the explosion time is finite, then
limt→t−1{ln[L0(X(t))]}=+∞. | (2.4) |
Now, we calculate the derivative (and we suppress the time-argument (t)):
dL0dt=−1S[A0−θSE−(μ+z)S]−1E[A1+θSE−(μ+δ1+α+ω1)E]−1I[αE−(μ+δ2+ω2)I]−1R[zS+ω1E+ω2I−μR]+1P−N[μ(P−N)−δ1E−δ2I] | (2.5) |
Note that θE<θ0P. After cancellation of some terms which are negative, we obtain an inequality:
dL0dt≤θ0P+5μ+z+δ1+α+ω1+δ2+ω2. |
Thus, dL0dt≤B0, with B0 being the constant
B0=θ0P+5μ+z+δ1+α+ω1+δ2+ω2. | (2.6) |
Therefore, over the bounded interval [0,t1), L0(t)≤B0t1, and so L0 is bounded over the interval [0,t1). This is a contradiction to the statement of equation (2.4). Therefore, we can conclude that X(t) never exits the set Γ. This concludes the proof.
Following the methodology as in [31], we obtain the basic reproduction number as follows:
R0=θ0A0μ0μ1. |
We note that the variable R does not appear in the first three equations of the system (2.1). Therefore, we can consider the reduced system (2.7) below, derived from the system (2.1) by ignoring the R-equation, i.e., the fourth equation.
˙S=A0−θSE−(μ+z)S˙E=A1+θSE−(μ+δ1+α+ω1)E˙I=αE−(μ+δ2+ω2)I. | (2.7) |
In this section we focus on the case A1=0. In this case the model (2.7) permits a disease-free equilibrium
X0=(A0μ+z,0,0). |
Theorem 3. If A1=0 and R0<1, then the disease-free equilibrium of the model (2.7) is globally asymptotically stable.
Proof. Suppose that R0<1. Then, θA0μ0−μ1<0. There exists c>0 such that
θA0μ0−μ1+cα<0. | (3.1) |
Fix such a number c and define a function L1(X(t)) as:
L1=S−A0μ0+A0μ0ln(A0μ0S)+E+cI. |
The time derivative of L1 is computed as
dL1dt=(1−A0μ0S)[A0−θSE−(μ+z)S]+[A1+θSE−(μ+δ1+α+ω1)E]+c[αE−(μ+δ2+ω2)I]=1μ0S(μ0S−A0)(A0−μ0S)−θSE+A0θEμ0+θSE−μ1E+c(αE−μ2I)=−1μ0S(μ0S−A0)2+A0θEμ0−μ1E+cαE−cμ2I=−1μ0S(μ0S−A0)2+E[A0θμ0−μ1+cα]−cμ2I≤−1μ0S(μ0S−A0)2+EQ−cμ2I, |
where Q=A0θ0μ0−μ1+cα. Thus, by Equation (3.1) we have Q<0. So, L1 is Lyapunov and the proof is complete.
In this section we investigate endemic equilibrium points, X∗=(S∗,E∗,I∗). The general technique of the proof of Theorem 4.1 is similar as in the papers [10,13,24,27] and others.
Theorem 4.1. The system (2.7) has an endemic equilibrium point X∗=(S∗,E∗,I∗) if and only if one of the following conditions (a) or (b) holds, and then the endemic equilibrium point is unique.
(a) A1>0,
(b) A1=0 and R0>1.
Proof. For an equilibrium point X∗, the following relations hold.
E∗=μ2αI∗, S∗=μ1E∗−A1θE, S∗=A0μ0+θE. |
These identities give rise to the equation that must be satisfied by I∗ if I∗ exists:
θ(I∗)=αμ0[μ1μ2I∗−αA1]μ2I∗[α(A0+A1)−μ1μ2I∗]. | (4.1) |
(a) Suppose that A1>0. Let us introduce the constant
ζ:=α(A0+A1)/(μ1μ2). |
Let F:(0,ζ)→R be the function defined as
F:x↦αμ0[μ1μ2x−αA1]μ2x[α(A0+A1)−μ1μ2x]. | (4.2) |
Now, since A1>0, we note that
limx→0+F(x)=−∞ and limx→ζ−F(x)=+∞. | (4.3) |
A routine calculation gives
F′(x)=αμ0μ2Q(x)μ22x2[α(A0+A1)−μ1μ2x]2 |
with
Q(x)=α2A1(A0+A1)−2αμ1μ2A1x+(μ1μ2x)2, |
and the discriminant of Q(x) is −4α2μ1μ2A0<0. Therefore, Q(x)>0 for all x∈(0,ζ), and consequently F′(x)>0 for all x∈(0,ζ).
Since θ and F are continuous, and their domains are connected (and overlapping), boundedness of θ together with Eq (4.3) implies that there exists x0∈(0,ζ) such that θ(x0)=F(x0). Such x0 is unique since θ is a decreasing function, and F is an increasing function. The number x0 happens to be I∗. This completes the proof of part (a) of the theorem.
(b) Suppose that A1=0. Similarly as above, let ξ=αA0/(μ1μ2) and let G:[0,ξ)→R be the function
G:x↦αμ0μ1αA0−μ1μ2x. |
Now, G is an increasing continuous function on the interval [0,ξ) with
limx→ξ−G(x)=+∞. |
Since θ is continuous and decreasing, there exists x1∈(0,ξ) such that θ(x1)=G(x1) if and only if θ(0)>G(0). The latter condition is equivalent to θ0>μ0μ1/A0, which is equivalent to the condition R0>1. Such x1 is unique, and I∗=x1. This proves (b).
Theorem 4.2. If an endemic equilibrium X∗=(S∗,E∗,I∗) for the model (2.7) exists, then X∗ is locally asymptotically stable.
Proof. Let us write θ(I∗)=θ∗ and θ′(I∗)=θ3. At the point X∗ we have the Jacobian matrix:
J(X∗)=(−θ∗E∗−μ0−θ∗S∗−θ3E∗S∗θ∗E∗θ∗S∗−μ1θ3E∗S∗0α−μ2)
and then
−det(λI3−J(X∗)=λ3+g1λ2+g2λ+g3, |
where
g1=μ0+μ1+μ2+θ∗E∗−θ∗S∗,g2=μ2θ∗E∗+μ2μ0+μ2(μ1−θ∗S∗)+(μ1−θ∗S∗)(μ0+θ∗E∗)−αθ3E∗S∗+θ∗2E∗S∗,g3=μ1μ2θ∗E∗+μ0μ2(μ1−θ∗S∗)−μ0αθ3E∗S∗. |
We note that μ1−θ∗S∗=A1/E∗>0. Therefore, g1>0. Also, θ3≤0. Therefore, g2>0 and g3>0. Now, let us test g1g2−g3 for positivity. We note that:
g1g2>(μ1−θ∗S∗+μ2+μ0)(μ0μ2+μ2θ∗E∗+θ∗2E∗S∗−αθ3E∗S∗)>(μ1−θ∗S∗)(μ0μ2+μ2θ∗E∗)+(μ2)(θ∗2E∗S∗)+(μ0)(αθ3E∗S∗)=g3, |
which means that g1g2−g3>0. Therefore, det(λI3−J(X∗) satisfies the Routh-Hurwitz criterion. Thus, the endemic equilibrium point X∗ is locally asymptotically stable.
This section is devoted to formulating and solving the optimal control problem. The idea is to design a vaccination schedule and another intervention by way of education, law enforcement, etc., which aim to minimize the number of COVID-19 cases on the one hand and the overall cost of these two strategies on the other hand, over a certain time horizon [0,T].
We introduce two control functions u1(t) and u2(t) in such a way that the vaccination rate will be replaced by the function z0u1(t), with z0 being the maximum affordable rate of vaccination, and the contact rate θ is replaced by θ[1−u2(t)]. For a finite time horizon [0,T], we have
u1:[0,T]→[0,1] and u2:[0,T]→[0,ρ], with 0<ρ<1. |
Thus, u2 is an intervention, by way of education, law enforcement, lockdown, etc., towards reducing the contact rate, while u1 is proportional to the vaccination rate.
The optimal control problem has an objective functional, as in Eq (5.1) below, over a fixed time interval [0,T].
J(u1,u2)=∫T0(C1u21(t)+C2u22(t)+C3I(t))dt, | (5.1) |
which is to be minimized subject to model system (5.2), below.
˙S(t)=A0−[1−u2(t)]θ(I(t))S(t)E(t)−[μ+z0u1(t)]S(t)˙E(t)=A1+[1−u2(t)]θ(I(t))S(t)E(t)−(μ+δ1+α+ω1)E(t)˙I(t)=αE(t)−(μ+δ2+ω2)I(t). | (5.2) |
We use squares in the first two terms of the objective functional for convexity. The constants C1 and C2 represent unit cost. The last term of the integrand in equation (5.1) represents the compartment I(t) that we wish to decrease, and C3 is a weight constant. The control variables u1(t) and u2(t) are bounded, and are assumed to be measurable functions of time. We solve our optimization problem by using well-established control theory, such as in [15] or the very detailed presentation in [16]. We construct the Hamiltonian function, and to this end we introduce Lagrange multipliers λ1(t),λ2(t) and λ3(t), also referred to as the costate variables. In the theorem below, the control variable, the state variables and the costate variables are functions of time, but this dependence is suppressed except where required explicitly.
H(t,S,E,I,R,u1,u2)=C1u21(t)+C2u22(t)+C3I(t)+λ1(t)k1(t)+λ2(t)k2(t)+λ3(t)k3(t) |
where
k1(t)=[A0−[1−u2(t)]θ(I(t))S(t)E(t)−[μ+z0u1(t)]S(t)]k2(t)=[A1+[1−u2(t)]θ(I(t))S(t)E(t)−(μ+δ1+α+ω1)E(t)]k3(t)=[αE(t)−(μ+δ2+ω2)I(t)] |
Theorem 5. An optimal solution for the problem of minimizing the objective functional in Eq (5.1), subject to the system (5.2), does exist, and it satisfies the following system of differential equations:
˙λ1=λ1[(1−u2)θ(I)E+(μ+z0u1)]−λ2[(1−u2)θ(I)E+(μ+z0u1)]˙λ2=λ1(1−u2)θ(I)S−λ2(1−u2)θ(I)S+λ2(μ+δ1+α+ω1)−λ3α˙λ3=(1−u2)θ′(I)SEλ1−(1−u2)θ′(I)SEλ2+λ3(μ+δ2+ω2)−C3, | (5.3) |
with transversality conditions
λ1(T)=λ2(T)=λ3(T)=0. |
Furthermore, the optimal controls are given by
u∗1=max{0,min(1,z0S∗λ12C1)} |
and
u∗2=max{0,min(ρ,θ∗S∗E∗(λ2−λ1)2C2)}. |
Proof. Sufficient conditions for existence of an optimal control, such as, for instance, in [9], can readily be checked, and we omit the detail. We continue by applying the Pontryagin maximum principle. The partial derivatives of the Hamiltonian with respect to the different state variables are calculated, and then we obtain the time derivatives of the costate variables as in Eq (5.3).
The functions u∗1 and u∗2 must optimize J. Therefore, we start our search for u∗1 and u∗2 by making:
∂H∂u1=0 and ∂H∂u2=0, |
and out of this, u∗1 and u∗2 emerge in the stated forms.
For a specific application we must declare the form of the function θ, and in the current application we choose a function of the form
θ(I)=β1+bI, for constants β>0 and b>0. |
The model is applied to South Africa, over time periods when there are (assumed to be) no internal or external disturbances on the model. Let us assume that for South Africa, such a time horizon is the period from 1 February to 1 June of 2021. Another such period would be from 1 August until 30 November of each the two years 2021 and 2022. Parameter values for the model are declared in Table 1. These are mostly obtained from the published literature. Some values are roughly estimated, when information or data is not available. The last parameter to be valuated is β, which is the most important one in the contact rate. We choose β so as to fit the COVID-19 incidence data as recorded at Worldometers, [35].
Parameters | Numerical values | Source |
P0 | 69281690 | cf. [33] |
μ | 8.43×10−5 | [33] |
A0 | μP0 | (standard) |
A1 | 0 | nominal |
β | 0.39/P0 | fitted |
b | 7×10−5 | fitted |
α | 1/9 | [32] |
z | 0 | see Remark 6.2.1 |
δ1 | 0.05δ2 | estimate |
δ2 | 0.0015 | [12] |
ω1 | 0.05ω2 | estimate |
ω2 | 0.006 | [28] |
For South Africa, the active infections show peaks at June/July and December/January for 2020 and 2021, see, for instance, [35]. One would attribute these peaks to people clustering densely together, more so than during other times of the year. Such unusual behavior, if it persists for a significant period of time, is not accounted for in a simple model with constant parameters such as the model that we have presented here. When the conditions and assumptions of the model are disturbed, the model with parameters constant and fixed, is no longer accurate or is not applicable. Such disturbances can take the form of external factors such as regulations by governments restricting the movement of people, it can be in the form of influx of carriers of the disease, or it can even be internally produced, for instance, when people tend to have much closer contact than usual, such as at a party, feast or another form of gathering. A disturbance may be such that the model will be able to detect it. When such disturbances occur, it may be possible to modify the model by allowing for seasonality or by some other intervention on the model [21,27]. An instance, where ideas from the model were utilized for studying a system during the period of the disturbance, is found in [34].
We utilize the model over the following periods,
Period 1: 24 July 2020 – 05 November 2020,
Period 2: 08 January 2021 – 29 April 2021.
Initial state: The coordinates of the initial point are determined as follows. The daily incidence (i.e., the number of daily new cases) and I0 (active cases) are obtained from [35]. From these we can get an estimate for E0, using the equation
Daily New Cases = αE0.
Then, we make an estimate for S0<P0−(E0+I0). These values were adjusted slightly to get a better fit on the rest of the daily new cases. We then take our initial state to be
I0=135110, E0=11500, S0=0.998P0.
Remark 6.2.1. During Period 1, there were no vaccines in circulation in South Africa. Vaccination of the public only started during the course of Period 2. Thus, the effect of vaccination during the periods in point is regarded as insignificant.
Let us first consider Period 1. The observed incidence of COVID-19 is obtained from the source [35]. We note that the model with parameters as in Table 1 makes a good fit, see, Figure 2, on the incidence cases over the first 35 days of Period 1. Then, for the rest of that period, we note that the model under-estimates the observed data.
Our explanation for this under-estimation is as follows. During the extraordinary rise of daily cases, there was awareness and caution. After the peak of incidence, when incidence dropped below a certain level, human behaviour became more risky. If the same alertness level had been maintained at least for the given period, the daily new cases would have dropped below 9 000, versus the observed 19 000. Now, quite remarkably, exactly the same model also fitted the data for Period 2, see, Figure 3. We note that the model output shows an increase towards the end of the period, for both Period 1 and Period 2. Now, if we observe the model output for a few more weeks as in Figure 4, we find that it would stabilize at the average of the observed values in the latter half of the observed period. Unfortunately, in reality, the system suffered another serious perturbation (which our model is not meant to handle), which led to the next wave of the epidemic in South Africa.
We run a numerical approximation using hypothetical values for the parameters that were introduced in the control problem. We choose C1=1, equal to 1 as a reference value. Then, we argue that the economic costs of lockdown and distancing at the workplace, in businesses and schools are very costly, compared to the cost of vaccination. On that basis, we choose C2=2. Finally, the balancing weight C3 is chosen as C3=2×10−5. Our time horizon for the problem is 180 days. The optimal controls obtained for this particular instance of the control problem are shown in Figures 5 and 6.
These are quite informative. They tell us that the vaccination should be executed at maximum level for most of the time. They also tell us that the control on the contact rate parameter, θ, must be run at maximum intensity over a period of some 60 days, after which it can be relaxed, at approximately 90% of the maximum intensity for another 60 days, and then it will dwindle away towards zero more or less linearly. The graphs of the E-class are shown in Figure 7. Here, we have a comparison between the system without control (the broken line) and the optimally controlled situation (the unbroken line). For these two scenarios we have computed the new cases that arise over the 180 days, and they are, respectively, 334970 and 288370. We observe a difference of 46600. We get a more impressive performance of the optimal control if we consider the same parameters, but starting at a different initial state. In this case the intervention averts more than 102 000 new cases. The graphs of the E-classes and I-classes are shown in Figures 8 and 9, respectively. An explanation for this is that the transmission rate is lower when the I-numbers are higher, and this is due to the form of the contact rate function, θ.
We have introduced a new disease model which allows for vaccination and inflow of infected. The model is mathematically sound with respect to existence of a unique global positive solution, and its equilibrium points have been shown to be stable. This model with general contact rate can be considered as additional to the class of SEI models that was presented in the paper [24] of Sigdel and McCluskey and subsequent contributions by [10,27] and others. We have also considered a control problem covering a two-fold intervention: vaccination and reduction of the contact rate. As a sample application we have considered the COVID-19 epidemic in South Africa. Our paper is one of a handful of optimal control works applicable to COVID-19 in South Africa, of which the closest to ours is [18]. These papers consider control on only non-pharmaceutical interventions. The current paper is the only one that includes vaccination in the model. Other papers have been more concerned with the initial rise of COVID-19 in the earlier part of 2020, whereas we compare our model output with observed data over two periods subsequent to peaks of COVID-19 incidence. The model was able to highlight an important phenomenon. It revealed how behavior change in terms of social distancing and contact shortly after a peak of COVID-19 incidence can be detrimental to the fight against the disease. More generally, it illustrates how a system can experience a significant perturbation from within itself. Of course with only four compartments, this SEIR model is limited in its accuracy. Furthermore, it may be necessary to introduce more classes if we wish to study the effect of asymptomatic infectives more thoroughly.
The authors wish to thank the reviewers for valuable comments which led to an improved manuscript.
The authors declare that there is no conflict of interest.
[1] |
S. Y. Tchoumi, M. L. Diagne, H. Rwezaura, J. M. Tchuenche, Malaria and COVID-19 co-dynamics: A mathematical model and optimal control, Appl. Math. Model., 99 (2021), 294–327. https://doi.org/10.1016/j.apm.2021.06.016 doi: 10.1016/j.apm.2021.06.016
![]() |
[2] |
T. A. Perkins, G. Espana, Optimal Control of the COVID-19 Pandemic with Non-pharmaceutical Interventions, Math. Biol., 82 (2020). https://doi.org/10.1007/s11538-020-00795-y doi: 10.1007/s11538-020-00795-y
![]() |
[3] |
M. Kantner, T. Koprucki, Beyond just "flattening the curve": Optimal control of epidemics with purely non-pharmaceutical interventions, J. Ind. Math., 10 (2020). https://doi.org/10.1186/s13362-020-00091-3 doi: 10.1186/s13362-020-00091-3
![]() |
[4] |
Y. Yuan, N. Li, Optimal control and cost-effectiveness analysis for a COVID-19 model with individual protection awareness, Phys. A, 603 (2022), 127804. https://doi.org/10.1016/j.physa.2022.127804 doi: 10.1016/j.physa.2022.127804
![]() |
[5] |
R. T. Alqahtani, A. Ajbar, Study of dynamics of a COVID-19 model for saudi arabia with vaccination rate, saturated treatment function and saturated incidence rate, Mathematics, 9 (2021), 3134. https://doi.org/10.3390/math9233134 doi: 10.3390/math9233134
![]() |
[6] |
B. Boukanjime, T. Caraballo, M. El Fatini, M. El Khalifi, Dynamics of a stochastic coronavirus (COVID-19) epidemic model with Markovian switching, Chaos Solit. Fractals, 141 (2020), 110361. https://doi.org/10.1016/j.chaos.2020.110361 doi: 10.1016/j.chaos.2020.110361
![]() |
[7] | Disaster Management Act. Regulations to address, prevent and combat the spread of coronavirus COVID-19: amendment. https://www.gov.za/documents/disaster-management-act-regulations-address-prevent-and-combat-spread-coronavirus-covid-19. (Accessed June 24, 2020). |
[8] |
L. E. Olivier, S. Botha, I. K. Craig, Optimized Lockdown Strategies for Curbing the Spread of COVID-19: A South African Case Study, IEEE Access : Practical Innovations, Open Solutions, 8 (2020), 205755–205765. https://doi.org/10.1109/ACCESS.2020.3037415 doi: 10.1109/ACCESS.2020.3037415
![]() |
[9] | W. H. Fleming, H. M. Soner, Controlled Markov processes and viscosity solutions. Second edition, Stoch. Model. Appl. Probab., 25. Springer, New York, 2006. XVII, 429 pages. https://doi.org/10.1007/0-387-31071-1 |
[10] |
M. Cerón Gómez, E. I. Mondragon, P. L. Molano, Global stability analysis for a model with carriers and non-linear incidence rate, J. Biol. Dyn., 14 (2020), 409–420. https://doi.org/10.1080/17513758.2020.1772998 doi: 10.1080/17513758.2020.1772998
![]() |
[11] |
P. C. Jentsch, M. Anand, C. T. Bauch, Prioritising COVID-19 vaccination in changing social and epidemiological landscapes: a mathematical modelling study, Lancet Infect Dis., 21 (2021), 1097–1106. https://doi.org/10.1101/2020.09.25.20201889 doi: 10.1101/2020.09.25.20201889
![]() |
[12] |
M. A. Khan, A. Atangana, Mathematical modeling and analysis of COVID-19: A study of new variant Omicron, Phys. A, 599 (2022), 127452. https://doi.org/10.1016/j.physa.2022.127452 doi: 10.1016/j.physa.2022.127452
![]() |
[13] |
Z. A. Khan, A. L. Alaoui, A. Zeb, M. Tilioua, S. Djilali, Global dynamics of a SEI epidemic model with immigration and generalized nonlinear incidence functional, Results Phys., 27 (2021), 104477. https://doi.org/10.1016/j.rinp.2021.104477 doi: 10.1016/j.rinp.2021.104477
![]() |
[14] |
M. Kinyili, J. B. Munyakazi, A. Y. A. Mukhtar, Assessing the impact of vaccination on COVID-19 in South Africa using mathematical modeling, Appl. Math. Inf. Sci., 15 (2021), 701–716. http://dx.doi.org/10.18576/amis/150604 doi: 10.18576/amis/150604
![]() |
[15] | S. Lenhart, J. T. Workman, Optimal Control Applied to Biological Models, (1st Ed.). Chapman and Hall/CRC. (2007). https://doi.org/10.1201/9781420011418 |
[16] |
A. K. Mengistu, P. J. Witbooi, Tuberculosis in Ethiopia: Optimal Intervention Strategies and Cost-Effectiveness Analysis, Axioms, 11 (2022), 343. https://doi.org/10.3390/axioms11070343 doi: 10.3390/axioms11070343
![]() |
[17] |
S. Mushayabasa, E. T. Ngarakana-Gwasira, J. Mushanyu, On the role of governmental action and individual reaction on COVID-19 dynamics in South Africa: A mathematical modelling study, Inform. Med. Unlocked., 20 (2020), 100387. https://doi.org/10.1016/j.imu.2020.100387 doi: 10.1016/j.imu.2020.100387
![]() |
[18] |
S. P. Gatyeni, C. W. Chukwu, F. Chirove, Fatmawati, F. Nyabadza, Application of Optimal Control to Long Term Dynamics of COVID-19 Disease in South Africa, Sci. Afr., 16 (2020), e01268. https://doi.org/10.1016/j.sciaf.2022.e01268 doi: 10.1016/j.sciaf.2022.e01268
![]() |
[19] |
E. Tornatore, P. Vetro, S. M. Buccellato, SIVR epidemic model with stochastic perturbation, Neural Comput. Appl., 24 (2014), 309–315. https://doi.org/10.1007/s00521-012-1225-6 doi: 10.1007/s00521-012-1225-6
![]() |
[20] |
N. Dalal, D. Greenhalgh, X. Mao, A stochastic model of AIDS and condom use, J. Math. Anal. Appl., 325 (2007), 36–53. ISSN 0022-247X. https://doi.org/10.1016/j.jmaa.2006.01.055 doi: 10.1016/j.jmaa.2006.01.055
![]() |
[21] |
O. S. Obabiyi, A. Onifade, Mathematical model for Lassa fever transmission dynamics with variable human and reservoir population, Int. J. Differ. Equ., 16 (2017), 67–91. http://dx.doi.org/10.12732/ijdea.v16i1.4703 doi: 10.12732/ijdea.v16i1.4703
![]() |
[22] |
C. M. Peak, R. Kahn, Y. H. Grad, L. M. Childs, R. Li, M. Lipsitch, et al., Individual quarantine versus active monitoring of contacts for the mitigation of COVID-19: a modelling study, Lancet Infect Dis., 20 (2020), 1025–1033. https://doi.org/10.1016/S1473-3099(20)30361-3 doi: 10.1016/S1473-3099(20)30361-3
![]() |
[23] | J. Lamwong, P. Pongsumpun, I. M. Tang, N. Wongvanich, The Lyapunov Analyses of MERS-Cov Transmission in Thailand, Curr. Appl. Sci. Technol., 19 (2019), 112–122. https://li01.tci-thaijo.org/index.php/cast/article/view/182299 |
[24] |
R. P. Sigdel, C. C. McCluskey, Global stability for an SEI model of infectious disease with immigration, Appl. Math. Comput., 243 (2014), 684–689. https://doi.org/10.1016/j.amc.2014.06.020 doi: 10.1016/j.amc.2014.06.020
![]() |
[25] |
A. Atangana, S. Iǧret Araz, Mathematical model of COVID-19 spread in Turkey and South Africa: theory, methods, and applications, Adv. Differ. Equ., 2020 (2020). https://doi.org/10.1186/s13662-020-03095-w doi: 10.1186/s13662-020-03095-w
![]() |
[26] |
G. T. Tilahun, H. T. Alemneh, Mathematical modeling and optimal control analysis of COVID-19 in Ethiopia, J. Interdiscip. Math., 24 (2021), 2101–2120. https://doi.org/10.1080/09720502.2021.1874086 doi: 10.1080/09720502.2021.1874086
![]() |
[27] |
B. Traore, O. Koutou, B. Sangare, Global dynamics of a seasonal mathematical model of schistosomiasis transmission with general incidence function J. Biol. Syst., 27 (2019), 19–49. https://doi.org/10.1142/S0218339019500025 doi: 10.1142/S0218339019500025
![]() |
[28] |
A. Rahmani, G. Dini, V. Leso, A. Montecucco, B. Kusznir Vitturi, I. Iavicoli, et al., Duration of SARS-CoV-2 shedding and infectivity in the working age population: A systematic review and meta-analysis, Med. Lav., 113 (2022), e2022014. https://doi.org/10.23749/mdl.v113i2.12724 doi: 10.23749/mdl.v113i2.12724
![]() |
[29] | Western Cape Department of Health in collaboration with the National Institute for Communicable Diseases, South Africa. "Risk factors for coronavirus disease 2019 (COVID-19) death in a population cohort study from the Western Cape Province, South Africa". Clin. Infect. Dis., 73 (2021), e2005–e2015. 10.1093/cid/ciaa1198 |
[30] |
A. R. Tuite, D. N. Fisman, A. L. Greer, Mathematical modelling of COVID-19 transmission and mitigation strategies in the population of Ontario, Canada, CMAJ, 192 (2020), E497–E505. https://doi.org/10.1503/cmaj.200476 doi: 10.1503/cmaj.200476
![]() |
[31] |
P. Van Den Driessche, J. Watmough, Reproduction numbers and sub-threshold endemic equilibria for compartmental model of disease transmission, Math. Biosci., 180 (2002), 29–48. https://doi.org/10.1016/S0025-5564(02)00108-6 doi: 10.1016/S0025-5564(02)00108-6
![]() |
[32] |
C. R. Wells, J. P. Townsend, A. Pandey, S. M. Moghadas, G. Krieger, B. Singer, et al., Optimal COVID-19 quarantine and testing strategies, Nat. Commun., 212, (2021). https://doi.org/10.1038/s41467-020-20742-8 doi: 10.1038/s41467-020-20742-8
![]() |
[33] |
P. J. Witbooi, An SEIR model with infected immigrants and recovered emigrants, Adv. Differ. Equ., 2021, (2021). https://doi.org/10.1186/s13662-021-03488-5 doi: 10.1186/s13662-021-03488-5
![]() |
[34] |
P. J. Witbooi, C. Africa, A. Christoffels, I. H. I. Ahmed, A population model for the 2017/18 listeriosis outbreak in South Africa, Plos One, 15 (2020), e0229901. https://doi.org/10.1371/journal.pone.0229901 doi: 10.1371/journal.pone.0229901
![]() |
[35] | Worldometers. Available from: https://covid19.who.int/region/afro/country/za |
[36] | H. Zine, E. M. Lotfi, M. Mahrouf, A. Boukhouima, Y. Aqachmar, K. Hattaf, et al., Modeling the spread of COVID-19 pandemic in Morocco. In; Analysis of Infectious Disease Problems (Covid-19) and Their Global Impact, Springer, Singapore, (2021), 599–615. https://doi.org/10.1007/978-981-16-2450-6_28 |
1. | F. Mortaji, A. Abta, H. Laarabi, M. Rachik, Global stability analysis, stabilization and synchronization of an SEIR epidemic model, 2024, 0020-7179, 1, 10.1080/00207179.2024.2391923 | |
2. | Nabila Beqqali, Khalid Hattaf, Naceur Achtaich, Vaccination against COVID-19 and Other Infectious Diseases in the Moroccan Education System, 2024, 59, 0258-2724, 10.35741/issn.0258-2724.59.5.7 |