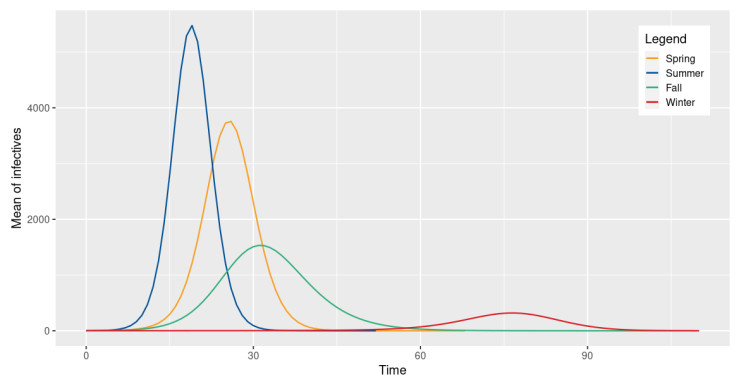
To better describe the spread of a disease, we extend a discrete time stochastic SIR-type epidemic model of Tuckwell and Williams. We assume the dependence on time of the number of daily encounters and include a parameter to represent a possible quarantine of the infectious individuals. We provide an analytic description of this Markovian model and investigate its dynamics. Both a diffusion approximation and the basic reproduction number are derived. Through several simulations, we show how the evolution of a disease is affected by the distribution of the number of daily encounters and its dependence on time. Finally, we show how the appropriate choice of this parameter allows a suitable application of our model to two real diseases.
Citation: Mireia Besalú, Giulia Binotto. Time-dependent non-homogeneous stochastic epidemic model of SIR type[J]. AIMS Mathematics, 2023, 8(10): 23218-23246. doi: 10.3934/math.20231181
[1] | Xavier Bardina, Marco Ferrante, Carles Rovira . A stochastic epidemic model of COVID-19 disease. AIMS Mathematics, 2020, 5(6): 7661-7677. doi: 10.3934/math.2020490 |
[2] | Lin Xu, Linlin Wang, Hao Wang, Liming Zhang . Optimal investment game for two regulated players with regime switching. AIMS Mathematics, 2024, 9(12): 34674-34704. doi: 10.3934/math.20241651 |
[3] | Shufan Wang, Zhihui Ma, Xiaohua Li, Ting Qi . A generalized delay-induced SIRS epidemic model with relapse. AIMS Mathematics, 2022, 7(4): 6600-6618. doi: 10.3934/math.2022368 |
[4] | Kalpana Umapathy, Balaganesan Palanivelu, Renuka Jayaraj, Dumitru Baleanu, Prasantha Bharathi Dhandapani . On the decomposition and analysis of novel simultaneous SEIQR epidemic model. AIMS Mathematics, 2023, 8(3): 5918-5933. doi: 10.3934/math.2023298 |
[5] | Jinsheng Guo, Shuang-Ming Wang . Threshold dynamics of a time-periodic two-strain SIRS epidemic model with distributed delay. AIMS Mathematics, 2022, 7(4): 6331-6355. doi: 10.3934/math.2022352 |
[6] | Zhengwen Yin, Yuanshun Tan . Threshold dynamics of stochastic SIRSW infectious disease model with multiparameter perturbation. AIMS Mathematics, 2024, 9(12): 33467-33492. doi: 10.3934/math.20241597 |
[7] | Muhammad Altaf Khan, Muhammad Ismail, Saif Ullah, Muhammad Farhan . Fractional order SIR model with generalized incidence rate. AIMS Mathematics, 2020, 5(3): 1856-1880. doi: 10.3934/math.2020124 |
[8] | Windarto, Muhammad Altaf Khan, Fatmawati . Parameter estimation and fractional derivatives of dengue transmission model. AIMS Mathematics, 2020, 5(3): 2758-2779. doi: 10.3934/math.2020178 |
[9] | Hui Sun, Zhongyang Sun, Ya Huang . Equilibrium investment and risk control for an insurer with non-Markovian regime-switching and no-shorting constraints. AIMS Mathematics, 2020, 5(6): 6996-7013. doi: 10.3934/math.2020449 |
[10] | Shah Hussain, Naveed Iqbal, Elissa Nadia Madi, Mohsen Bakouri, Ilyas Khan, Wei Sin Koh . On the stochastic modeling and forecasting of the SVIR epidemic dynamic model under environmental white noise. AIMS Mathematics, 2025, 10(2): 3983-3999. doi: 10.3934/math.2025186 |
To better describe the spread of a disease, we extend a discrete time stochastic SIR-type epidemic model of Tuckwell and Williams. We assume the dependence on time of the number of daily encounters and include a parameter to represent a possible quarantine of the infectious individuals. We provide an analytic description of this Markovian model and investigate its dynamics. Both a diffusion approximation and the basic reproduction number are derived. Through several simulations, we show how the evolution of a disease is affected by the distribution of the number of daily encounters and its dependence on time. Finally, we show how the appropriate choice of this parameter allows a suitable application of our model to two real diseases.
The interest of mathematical models to describe the spread of a disease has a long history. It dates back to the eighteenth-century study of Bernoulli on smallpox inoculation [1]. Both deterministic and stochastic models have been considered and many factors have been taken into account: infectious agents, mode of transmission, latent periods, temporary or partial immunity, quarantine periods, etc. (see, for example, [2,3,4,5,6,7]). The main advantage of the deterministic approach lies in its more manageable (even if not necessarily simple) analysis. However, the most natural way to describe the spread of a disease is stochastic. This is due to several facts. On one hand, some phenomena are genuinely stochastic or present random features. On the other hand, the infective agent is introduced into the population only through a few hosts. Deterministic models cannot capture this component, given that they apply only when a small fraction (not a small number) of the large population is infected. To solve this contrast, demographic stochasticity needs to be taken into account (see [4] for an exhaustive explanation).
An important role in mathematical epidemiology is played by the class of models that divide the population into different categories and study the transitions of state between them. One of the most classical are the SIR models, where the letters stand for Susceptible, Infectious and Removed, respectively. The first SIR stochastic model was proposed by McKendrick [8] as a continuous-time version of the deterministic model of Kermack and McKendrick [9], although more attention was given to the chain-binomial model of Reed and Frost (later published by Abbey [10]). From that moment on, many other texts and models have been developed.
Stochastic models can be divided into three major categories: discrete time models, continuous time Markov chain models and diffusion models (see [11]). The literature that deals with these three categories is extensive. To name a few, Tuckwell and Williams [12] consider a simple discrete time Markovian model in which the total population is constant and individuals meet a random number of other individuals at each time step. Ferrante et al. [13] generalize this model by adding two new classes. They assume that the number of encounters at each time step is constant for all individuals and time. Their results are more suitable for diseases with an initial latency period and the presence of asymptomatic individuals. On the other hand, Gray et al. [14] directly propose a system of equations to model the dynamics of the population. Discrete time models are mathematically less complex than those employed in the other two categories. However, they grant a simplified vision of the evolution of a disease which highlights its main characteristics. They also provide a good guide for constructing more complex models. Besides, they do not generally present specific constraints or assumptions that could be in contrast with empirical evidence as do, for example, continuous time Markov chain models.
In the present paper, we consider the SIR-type model proposed by Tuckwell and Williams [12]. We expand their results by weakening the homogeneity in time, that is, assuming the dependence on time in some parameters and by including factors that have not been taken into account. We deal with a discrete time, discrete state space stochastic model built on a generation basis. Time unit is of one-day length. The population is assumed to be closed, homogeneous and homogeneously mixing and it is divided into the three classes defined above. We assume that every day any susceptible individual meets a certain number of different individuals. If one of them is infective, the disease is transmitted with a given probability. While in [12] the number of daily encounters is time-homogeneous, in our case it can change at any time. Moreover, for the main computations and almost all simulations they fix this quantity for simplicity while we maintain it random all over the paper. With simulations and applications to real diseases, we will show that this randomness and dependence on time play an important role and can generate different scenarios. After being infected, an individual remains infectious for a fixed number of consecutive epochs. After this period, he or she recovers and becomes immune. We also add a parameter to the model to consider the case of a possible quarantine for infectious individuals.
We describe this type of model analytically using the methodologies of [12] and [13]. We derive an explicit structure for the underlying discrete time Markov chain and deduce the probability that a susceptible will become infected at the following epoch. This is the content of Section 2. Section 3 is dedicated to describing the dynamics of the model. Two possible situations are taken into account: when the duration of the disease is constant and when infectious individuals remain infectious throughout their lives. For both cases, we describe the distribution of new infected and derive a diffusion approximation and the basic reproduction number R0. This is the expected number of secondary cases produced by an infectious individual during its infectious period in a virgin population. This value is used to measure the potential for transmission of a disease. Section 4 contains some simulations of the SIR-type model we have described. After fixing some parameters, we compare the evolution of the disease for different distributions of the number of daily contacts. We see how the different behaviors and the dependence on time of this parameter affect the course of the disease. Section 5 is devoted to the application of our model to real diseases. We consider the cases of influenza and meningococcal infection. The appropriate choice of the distribution of the number of daily encounters, both random and time-dependent, ensures that our model adjusts remarkably well to real data.
Throughout the paper, we use the term infective to refer to an individual who has contracted the disease and is infectious (he or she belongs to class I) while we connote with infected an individual who is either infectious or removed, i.e., no longer susceptible (he or she belongs to class I or R).
This section is dedicated to describing the basic properties of the SIR model we consider. It is an extension of the one proposed by Tuckwell and Williams [12]. The main difference regards the number of daily contacts. While Tuckwell and Williams assume that this quantity is time-homogeneous, in our case it can change at any time step allowing us to differentiate the number of contacts, for example, between weekdays and weekends, between different seasons, etc. Moreover, they assume it is a random variable but they fix it to be a constant in the main computations. On the contrary, we maintain the random character of this parameter throughout the paper. We also add a parameter to the model to consider the case that the infective individuals adopt some kind of quarantine. This can range from a perfect quarantine (there are no contacts) to no quarantine at all (the infective individuals have the same contacts as a susceptible one).
We assume:
(1) Total population size: It is fixed at n. The population is closed.
(2) Time: Time is discrete. In epidemics, the natural unit for the duration of an epoch is one day, although in some applications the time step is bigger (see, for example, [15]).
(3) Definition of a sick individual: Given any individual i, with i=1,…,n, we define a stochastic process Yi={Yi(t),t=0,1,2,…} such that
Yi(t)={1if the individualiis infective (and infectious) at timet0otherwise. |
Then, the total number of infective and hence infectious individuals at time t is
Y(t)=n∑i=1Yi(t). |
(4) Daily encounters: Over (t,t+1] each individual i meets a random number Ni(t) of other individuals. For all i and t, the variables Ni(t) are mutually independent and independent of the state of the population. Furthermore, for all i and t, Ni(t) can take only a finite number of values nk, each with probability pk(t). The values are fixed, that is, they depend neither on individuals nor on time while their probability varies with respect to time but not to individuals. This is, for k=1,…,m,
P(Ni(t)=nk)=pk(t)∀i,t |
with ∑mk=1pk(t)=1 for all t. Observe that the set of all possible values of Ni(t) that we define M={n1,…,nm} is an ordered subset of N≥0. As we consider models where, for a fixed t, the distribution of the number of daily encounters is the same for all individuals, throughout the paper we will use the simplified notation N(t) instead of Ni(t) when appropriate.
(5) Duration of the disease: Any individual remains infectious for r consecutive epochs where r is a positive integer. After this period, the individual recovers and becomes immune. The case without recovery, that is when r=∞, is also considered.
(6) Contagion probability: If an individual who has never been diseased up to and including time t meets an individual in (t,t+1] who is diseased at time t, then independently of the results of other encounters, this encounter results in transmission of the disease with probability p. Thus, if this individual becomes infected, it will be counted as such at epoch t+1.
(7) Encounter probability: The population is homogeneously distributed. This means that, given Y(t)=y, the probability that a randomly chosen individual is infectious at time t is given by y/n. We will multiply this probability by a constant λ∈[0,1] to characterize a possible quarantine of the infectious individuals. If λ=0 the infectious individuals do a rigorous quarantine and for values of λ close to 1 they will do a mild or non-existent quarantine.
As mentioned before, the variables Ni(t) are independent for all i and t. Assuming that for a fixed t they are also identically distributed, the model can be seen as an (r+1)-dimensional Markov chain. Observe that only if Ni(t) are identically distributed with respect to time, this model is a homogeneous Markov chain.
Let
● Yℓ(t) be the number of individuals who are infective at time t and have been infective for exactly ℓ time units, with ℓ=0,1,…,r−1;
● X(t) be the number of susceptible individuals at time t;
● Z(t) be the number of individuals who were previously infective and are recovered at time t.
We assume that all of the individuals who are infective at t=0 have just become infected so that Y(0)=Y0(0) and Yℓ(0)=0 for ℓ=1,…,r−1. Furthermore, there are no recovered individuals at t=0, so that Z(0)=0 and the population is made up only of susceptible and just infected individuals. So, Y0(0)+X(0)=n.
Regardless of the initial conditions, the process
M(t)=(X(t),Y0(t),Y1(t),…,Yr−1(t))t=0,1,2,… |
is a Markov chain with state space
S(n,r)={(x,y0,…,yr−1)∈Nr+1 s.t x+r−1∑ℓ=0yℓ≤n}. |
The cardinality of S(n,r) is (n+r+1n). Observe that the values of Z(t) are determined if all the components of M(t) are known.
The total number of infectives at time t is given by
Y(t)=r−1∑ℓ=0Yℓ(t), |
so the set (X(t),Y(t),Z(t)) gives the traditional SIR description.
In addition to the process Yi={Yi(t),t=0,1,2,…}, we can define in a similar manner the process Xi={Xi(t),t=0,1,2,…}, for i=1,…,n, which indicates whether individual i is susceptible or not and the variable Zi(t)=1−Xi(t)−Yi(t) which indicates if the individual i has recovered from the disease and is no longer infectious. For all t, we get
X(t)=n∑i=1Xi(t),Y(t)=n∑i=1Yi(t)andZ(t)=n∑i=1Zi(t). |
Furthermore, for i=1,…,n, we can consider the processes Yiℓ={Yiℓ(t)}t≥0 for ℓ=0,…,r−1 where
Yiℓ(t)={1if the individuali at timetis infective forℓ days0otherwise. |
Hence,
Yi(t)=r−1∑ℓ=0Yiℓ(t)andYℓ(t)=n∑i=1Yiℓ(t). |
Then, we can consider another non-homogeneous Markov chain
˜M(t)=(Xi(t),Yi0(t),Yi1(t),…,Yir−1(t),i=1,…,n)t=0,1,2,… |
with state space
˜S(n,r)={(x1,y10,…,y1r−1,…,xn,yn0,…,ynr−1)∈{0,1}n(r+1) s.t αi=xi+r−1∑ℓ=0yiℓ≤1 for i=1,…,n and n∑i=1αi≤n}. |
The cardinality of ˜S(n,r) is equal to (r+2)n. Even if the cardinality is bigger than the one of S(n,r), this Markovian model is more simple for computation purposes.
For a fixed individual i, consider the process
˜Mi(t)=(Xi(t),Yi0(t),Yi1(t),…,Yir−1(t))t=0,1,2,… |
If one of the variables Yi0(t),Yi1(t),…,Yir−1(t) is equal to 1 then the process at times bigger than t is certain since the transition in this case is sure. The only interesting case is when the individual is susceptible at time t, that is, Xi(t)=1.
Now we are interested in calculating the probability that an individual i susceptible at t becomes infected for the first time at t+1. This probability depends on the total number Y(t)=y of diseased individuals together with the probability p of transmission per contact and the parameter λ∈[0,1] which characterizes a possible quarantine. It is calculated taking into account all possible values of Ni(t) and its probabilities. Recall that the variables Ni(t) are identically distributed for all individuals but not with respect to time, so we will use the notion N(t) instead. Assuming n is much greater than N(t), so that the binomial approximation may be used, the probability of meeting exactly j infectives if N(t)=nk individuals are met is
pij(y,nk;n)≈(nkj)(λyn−1)j(1−λyn−1)nk−j, | (2.1) |
when y<n−1, while pink(y,nk;n)=1 and pij(y,nk;n)=0 for j=0,…,nk−1 when y=n−1. The probability pj of becoming infected if j infectives are met is
pj=1−(1−p)j. | (2.2) |
Then, using (2.1) as an equality the probability that an individual i susceptible at t becomes infected for the first time at t+1 is
p(t,y)=P(Yi0(t+1)=1|Xi(t)=1,Y(t)=y)=m∑k=1P(Yi0(t+1)=1|Xi(t)=1,Y(t)=y,N(t)=nk)P(N(t)=nk)=m∑k=1nk∑j=0pij(y,nk;n)pjpk(t)=m∑k=1nk∑j=0(nkj)(λyn−1)j(1−λyn−1)nk−j[1−(1−p)j]pk(t)=m∑k=1pk(t)nk∑j=0(nkj)(λyn−1)j(1−λyn−1)nk−j+m∑k=1pk(t)nk∑j=0(nkj)((1−p)λyn−1)j(1−λyn−1)nk−j=1−m∑k=1(1−pλyn−1)nkpk(t). | (2.3) |
when y<n−1, while p(t,n−1)=1−∑mk=1(1−p)nkpk(t).
Note that, as commonly used, this model contains a simplification regarding the encounters between individuals: the meeting relationship is not symmetric because if the group randomly chosen to meet individual i contains individual j, the group chosen to meet individual j does not necessarily contain individual i.
After presenting our model, we are interested in describing its dynamics. We study the distribution of new infective individuals and the basic reproduction number. Then, we prove that our model can approximate a diffusion process. We consider two cases. The first is a general one where any individual remains infectious for r<∞ consecutive epochs. Here r is a fixed positive constant. We refer to this model as the one with recovery. The second is the particular case without recovery that is when r=∞.
Recall that the processes X(t), Y(t) and Z(t) represent the number of susceptible, infective and (previously infected and) recovered individuals at time t, respectively. As their sum is fixed and corresponds to the size of the population, to study the number of new infective individuals it is sufficient to know just two of these quantities. We define V(t) as the number of non susceptible individuals at time t, that is V(t)=Y(t)+Z(t). It is useful to observe that the processes Y(t) and Z(t) can be expressed in terms of V(t):
Y(t)=V(t)−V(t−r)andZ(t)=V(t−r). |
The number of new infectives at time t+1 is given by V(t+1)−V(t). So, the total number of infectives at time t+1 is given by the number of new infectives and the number of infectives at t who have not yet recovered at t+1.
Then, following the previous computations we have
P(V(t+1)=w+y+z|Y(t)=y,Z(t)=z)=P(V(t+1)=w+y+z|V(t)−V(t−r)=y,V(t−r)=z)=(n−y−zw)p(t,y)w(1−p(t,y))n−y−z−w. |
Therefore, the distribution of the increment in the number of infectives follows a binomial law:
V(t+1)−V(t)|Y(t)=y,Z(t)=z∼Binom(n−y−z,p(t,y)). |
When y<n−1, its mean and variance are
E[V(t+1)−V(t)|Y(t)=y,Z(t)=z]=(n−y−z)[1−m∑k=1(1−pλyn−1)nkpk(t)] |
and
Var[V(t+1)−V(t)|Y(t)=y,Z(t)=z]=(n−y−z)[1−m∑k=1(1−pλyn−1)nkpk(t)][m∑k=1(1−pλyn−1)nkpk(t)]. |
In the extreme case when y=n−1, z can take only two possible values. If z=1, it means that there are no more susceptible individuals in the population and the number of new infectives is constantly zero. If z=0, all individuals are infective except one. Then, the distribution of the increment in the number of infectives corresponds to the infection of the unique susceptible and follows a Bernoulli law with parameter p(t,n−1). Its means and variance are easily deduced.
The basic reproduction number R0 is the expected number of secondary cases produced by an infective individual during its period of infectiousness in a population where all individuals are susceptible to infection. R0 excludes new cases produced by the secondary cases.
From a theoretical point of view, it plays a vital role in the analysis of infectious disease models. Given a population in a stable demographic state with no history of a given infection, it provides insight as to whether the introduction of the infectious agent will cause an outbreak. In this sense, the basic reproduction number is used to measure the transmission potential of a disease as an epidemic occurs in a susceptible population only if R0>1 (see [4] for additional details).
Our idea consists of estimating the basic reproduction number by observing the role of the infective individual and determining how many people he or she infects at any time step. A different approach has been used, for example, in [13] where the estimation is based on the probability of a susceptible individual being infected by the tagged one. In other cases, it can be estimated computing other related metrics such as the effective reproductive number (see, for example, [16]). We first consider the case without quarantine and then deduce the basic reproduction number when a quarantine for infectious individuals has been established.
In accordance with the definition of the basic reproduction number, we assume that at time t=0 the number of susceptible individuals is X(0)=n−1 and that the number of infected ones is Y(0)=1. Clearly, Z(0)=0.
Suppose first that there is no quarantine, that is λ=1. We denote by I(t) the number of individuals infected by our tagged individual during only the t-th period and by S(t) the number of susceptible individuals met by one person at time t. We assume that a susceptible individual, met and infected by another (for example, the tagged one) at time t, is considered infective since time t+1. Since our tagged individual remains infectious for r consecutive days (from t=0 to t=r−1), the basic reproduction number is given by
R0=r∑t=1E[I(t)]. |
For all t, I(t) depends on the number S(t−1) of susceptible individuals met by the tagged one at time t−1 and the probability of transmission p.
Since at time t=0 all individuals except the tagged one are susceptible, S(0)=N(0) and the distribution of I(1) is quite simple to determine:
I(1)|N(0)=nk∼Binom(nk,p). |
Consequently,
E[I(1)]=E[E[I(1)|N(0)]]=pE[N(0)]. |
For all t∈{1,…,r−1}, the variable S(t) may not coincide with N(t) and the distribution of I(t+1) is given by
I(t+1)|S(t)=l∼Binom(l,p). | (3.1) |
Moreover, for all t∈{1,…,r−1} S(t) depends on the number of individuals met by a single person and on the number of infective in the total population. Observe that during this period the number of removed individuals is always zero since our tagged one will be the first removed individual at time t=r. S(t) follows a hypergeometric distribution:
S(t)|N(t)=nk,Y(t)=y∼HGeom(n−1,n−y,nk). |
Observe that we have the following parameters: n−1 is the size of the population without counting the tagged individual; n−y represents the number of susceptible individuals in the population; and nk is the number of daily contacts for one individual. As n is supposed to be much greater than N(t), we use the binomial approximation
S(t)|N(t)=nk,Y(t)=y≈Binom(nk,p(y)), | (3.2) |
where
p(y)=1−y−1n−1 | (3.3) |
is the proportion of susceptible individuals in the population (without counting the tagged individual).
Observe that the total number of infectives at a time step is given by the total number of infectives of the previous generation and the new infectives they have produced. That leads to:
E[Y(t)]=E[E[Y(t)|Y(t−1),S(t−1)]]=E[Y(t−1)+pS(t−1)Y(t−1)]=E[(1+pS(t−1))Y(t−1)]. |
With inductive arguments, we obtain
E[Y(t)]=E[t−1∏s=0(1+pS(s))]. | (3.4) |
We can find another expression for the last term that is more manageable for our purpose. Before giving it explicitly, we present a basic example to better understand the underlying idea. With the notation employed in previous sections, using that, for t∈{1,…,r−1}, Y(t)=∑r−1ℓ=0Yℓ(t)=∑tℓ=0Yℓ(t) since Yℓ(t)=0 for ℓ>t and also that Yt(t)=1 refers to the tagged individual, for t=3 we have
E[Y(3)]=E[Y3(3)+Y2(3)+Y1(3)+Y0(3)]=E[1+pS(0)+pS(1)(1+pS(0))+pS(2)(1+pS(0)+pS(1)(1+pS(0)))]=E[1+pS(0)+pS(1)+pS(2)+p2S(0)S(1)+p2S(0)S(2)+p2S(1)S(2)+p3S(0)S(1)S(2)] |
that can be shortly expressed as
E[Y(t)]=E[∑js∈{0,1}s=0,1,2pj0+j1+j22∏s=0S(s)js]. |
Induction on the general case leads to the following expression equivalent to (3.4):
E[Y(t)]=E[∑js∈{0,1}s=0,…,t−1pj0+⋯+jt−1t−1∏s=0S(s)js]. | (3.5) |
If we define ψ(t)=∏ts=0(1+pS(s)), the induction is based on the decomposition
ψ(t)=ψ(t−1)(1+pS(t)) |
and the fact that the last term can be written as
(1+pS(t))=∑jt∈{0,1}pjtS(s)jt. |
Equations (3.3) and (3.5) lead to
E[p(Y(t))]=1+O(1n−1). | (3.6) |
Then, from (3.1), (3.2) and (3.6) for all t∈{1,…,r−1} we have
E[I(t+1)]=E[E[I(t+1)|S(t)]]=pE[S(t)]=pE[E[S(t)|N(t),Y(t)]]=pE[N(t)⋅p(Y(t))]=pE[N(t)]+O(1n−1). |
In the last step, we use the assumption that the number of daily contacts does not depend on the state of the population which implies that N(t) is independent of Y(t).
Finally, we get
R0=r∑t=1E[I(t)]=pr−1∑t=0E[N(t)]+O(1n−1). |
If we assume that there is a quarantine, recall that the probability that a randomly chosen individual is infectious is multiplied by a parameter λ∈[0,1]. Thinking about the role of this parameter, we see that it also affects the contagiousness of an infective individual which is also multiplied by the same factor. This means that the basic reproduction number is given by
R0=λr∑t=1E[I(t)]. |
The same calculations used for the case without quarantine lead to the following expression for R0:
R0=λpr−1∑t=0E[N(t)]+O(1n−1). | (3.7) |
As the number of susceptibles met by the tagged individual is always less or equal to the number of his or her encounters, we can deduce an upper bound for the expected value of R0:
R0≤λpr−1∑t=0E[N(t)]. |
From (3.7) it follows that, since R0≈1 for λp∑r−1t=0E[N(t)]=1, this is a threshold for the epidemic. This means that it grows for larger values and dies soon for smaller ones.
Finally, note that R0 is closely related to the number of daily encounters that occur during the first r days of the spread of the disease. Since this parameter depends on time, its value could change significantly if the disease arises in a different period as will be shown in Section 4.1.1.
Following the ideas of Tuckwell and Williams [12], the study of the mean and variance of the one-step increments of V indicates that for a large population size n and a small probability transition p such that npE[N([nt])] is of moderate size for all t, we can approximate a rescaled version of V by a diffusion process.
More accurately, if we speed up time and rescale state we can define a process
ˆVn(t)=V([nt])nforallt≥0, |
where [⋅] denotes the greatest integer part. ˆVn(t) can be interpreted as the fraction of the population that has been infected by the time [nt] in the original time scale of V. Then, for n large and p small such that θ(t)=npE[N([nt])] is of moderate size for all t, we see that with Δt=1n and t=0,1n,2n,…
E[ˆVn(t+Δt)−ˆVn(t)|ˆVn(t)−ˆVn(t−rn)=ˆy,ˆVn(t−rn)=ˆz]=1nE[V([nt]+1)−V([nt])|V([nt])−V([nt]−r)=nˆy,V([nt]−r)=nˆz]=1n(n−nˆy−nˆz)[1−m∑k=1(1−λnpˆyn−1)nkpk([nt])]≈(1−ˆy−ˆz)λnpˆyn−1E[N([nt])]≈λθ(t)ˆy(1−ˆy−ˆz)Δt |
and
Var[ˆVn(t+Δt)−ˆVn(t)|ˆVn(t)−ˆVn(t−rn)=ˆy,ˆVn(t−rn)=ˆz]=1n2Var[V([nt]+1)−V([nt])|V([nt])−V([nt]−r)=nˆy,V([nt]−r)=nˆz]=1n2(n−nˆy−nˆz)[1−m∑k=1(1−λnpˆyn−1)nkpk([nt])][m∑k=1(1−λnpˆyn−1)nkpk([nt])]≈1n(1−ˆy−ˆz)[λnpˆyn−1E[N([nt])]][1−λnpˆyn−1E[N([nt])]]≈1n(1−ˆy−ˆz)λnpˆyn−1E[N([nt])]≈θ(t)λnˆy(1−ˆy−ˆz)Δt. |
In both calculation we use the approximation 1−(1−x)a≈ax for small x. In the calculation of the variance we also use the approximation x(1−x)≈x for small x.
Moreover, we recall that E[N([nt])] depends on the values n1,n2,…,nm and its probabilities pk(t),k=1,…,m. In fact, the time dependence on E[N([nt])] rely on pk(t)∈[0,1],k=1,…,m. So, we can assume for all n and t that E[N([nt])] is of order of a constant N, so
θ(t)=npE[N([nt])]≈npN:=θ. |
With the previous results and approximation methods for continuous time Markov chains using diffusion processes (similar results to [13] and [12]), we can approximate ˆVn by a diffusion process ˆV in [0,1] that satisfies the stochastic delay differential equation (SDDE)
dˆV(t)=λθ(ˆV(t)−ˆV(t−τ))(1−ˆV(t))dt+[λθn(ˆV(t)−ˆV(t−τ))(1−ˆV(t))]12dW(t), | (3.8) |
where τ=rn and W={W(t),t≥0} is a standard Brownian motion. This equation has a unique solution for a given initial condition ˆV0 which is a non-decreasing continuous function ˆV0:[−τ,0]→[0,1] (see [17] for more references in SDDE).
Moreover, if we can assume that the variability is small then the noise term in (3.8) has little effect. So, it seems natural to conjecture that the mean of ˆV(t) can be approximated by ˆm(t) where ˆm satisfies the deterministic equation
dˆm(t)=λθ(ˆm(t)−ˆm(t−τ))(1−ˆm(t))dt. |
We can interpret the solution of the equation ˆm(t) as the proportion of the number of infective and recovered individuals. The general solution of this equation cannot be obtained using the classic methods of delay equations. However, it is easy to check that all constants c∈[0,1] are solutions. Also, this equation presents two fixed points. One is ˆm(t)=1, when the whole population is already infective or recovered. The other is ˆm(t)=ˆm(t−τ), meaning that we do not have new infected in an interval of length τ. Returning to the initial process without rescaling, it infers that for a period of length r the number of infective and recovered remains constant. That implies there will be no more infective individuals and the distribution of the population in the three classes will be invariant.
Consider now the case in which the infectious individuals remain infectious throughout the course of the epidemic. Such a situation can arise when a disease causing agent has a long life, as with tuberculosis in deer [18], or when life-prolonging drug therapies have been found, as with HIV in humans [19]. As recovered individuals are not presented in this case, the model reduces to one of SI type rather than SIR.
Here, only two classes of individuals are present: the susceptibles and the infectives. Their amount at time t is given by the variables X(t) and Y(t), respectively.
The distribution of new infective individuals only depends on the number of infectives of the previous generation. Assuming that at time t there are Y(t)=y infectives implies that there are n−y susceptibles since the population size is n and there are no recovered individuals. Recall that the distribution of daily encounters at time t, given by the variables N(t), is the same for all individuals. Moreover, N(t) are independent for all i and t. In this case, λ parameter will be fixed λ=1 (no quarantine).
To study the distribution of new infectives, we follow the same ideas of the case with recovery with the difference that here it is not necessary to define a new variable that represents the number of non-susceptible individuals, as this is given by Y(t). Given Y(t)=y, the number of new infectives at t+1 follows a binomial distribution whose parameters are the number of susceptibles at t and the probability that an individual susceptible at t becomes infected for the first time at t+1. This is
Y(t+1)−Y(t)|Y(t)=y∼Binom(n−y,p(t,y)), |
where
p(t,y)=1−m∑k=1(1−pyn−1)nkpk(t) |
is given by (2.3). This implies that the distribution of the increment in the number of infectives is given by
P(Y(t+1)=y+w|Y(t)=y)=(n−yw)[1−m∑k=1(1−pyn−1)nkpk(t)]w[m∑k=1(1−pyn−1)nkpk(t)]n−y−w | (3.9) |
for w=0,…,n−y. Its mean is
E[Y(t+1)−Y(t)|Y(t)=y]=(n−y)[1−m∑k=1(1−pyn−1)nkpk(t)] |
and its variance is
Var[Y(t+1)−Y(t)|Y(t)=y]=(n−y)[1−m∑k=1(1−pyn−1)nkpk(t)][m∑k=1(1−pyn−1)nkpk(t)]. |
In this section we calculate the value of the basic reproduction number in the case without recovery which we denote by R(∞)0. The computation for the case with recovery can be adapted to this model.
Here, our tagged individual is infectious all his or her life but we can suppose that he or she lives only for a certain amount T of days after being infected. Thus, the calculation of the basic reproduction number is the same as that of the case with recovery, changing the number of terms from r to T:
R(∞)0=T∑t=1E[I(t)]=pT−1∑t=0E[N(t)]+O(1n−1). |
Recall that I(t) denotes the number of individuals infected by our tagged individual during only the t-th period and observe that in this case no quarantine is established for infective individuals.
The considerations about an upper bound for R(∞)0 and a threshold for the epidemic can be done also in the case without recovery. On one hand, as the number of susceptibles met by the tagged one is always less or equal to the number of his or her encounters, an upper bound for R(∞)0 is:
R(∞)0≤pT−1∑t=1E[N(t)]. |
On the other hand, since R(∞)0≈1 for p∑T−1t=1E[N(t)]=1, this is a threshold for the epidemic.
Following the ideas of the case with recovery, the study of the mean and variance of the one-step increments of Y indicates that for a large population size n and a small probability transition p such that npE[N([nt])] is of moderate size for all t fixed, we can approximate a rescaled version of Y by a diffusion process.
More accurately, if we speed up time and rescale state we can define a process
ˆYn(t)=Y([nt])nforallt≥0 |
where [⋅] denotes the greatest integer part. Then, we can interpret ˆYn(t) as the fraction of the population that has been infected by the time [nt] in the original time scale of Y. Then, for n large and p small such that θ(t)=npE[N([nt])] is of moderate size for all t we see that with Δt=1n and t=0,1n,2n,…
E[ˆYn(t+Δt)−ˆYn(t)|ˆYn(t)=ˆy]=1n(n−nˆy)[1−m∑k=1(1−npˆyn−1)nkpk(t)]≈1n(n−nˆy)npˆyn−1E[N([nt])]≈pˆy(1−ˆy)E[N([nt])]=θ(t)ˆy(1−ˆy)Δt |
using the approximation 1−(1−x)a≈ax for small x. With the same procedure, we have
Var[ˆYn(t+Δt)−ˆYn(t)|ˆYn(t)=ˆy]=1n2Var[Y([nt]+1)−Y([nt])|Y([nt])=nˆy]=1n2(n−nˆy)[1−m∑k=1(1−npˆyn−1)nkpk(t)][m∑k=1(1−npˆyn−1)nkpk(t)]≈1n(1−ˆy)[1−npˆyn−1E[N([nt])]]npˆyn−1E[N([nt])]≈1np(1−ˆy)ˆyE[N([nt])]=θ(t)nˆy(1−ˆy)Δt. |
Using as before the approximation 1−(1−x)a≈ax for small x and p(1−p)≈p for small p. As in the case with recovery (r<∞), following the same steps we can assume
θ(t)=npE[N([nt])]≈npN:=θ |
for a constant N.
With this results and approximation methods for continuous time Markov chains using diffusion processes we can approximate ˆYn by a diffusion process ˆY in [0,1] that satisfies the stochastic differential equation
dˆY(t)=θˆY(t)(1−ˆY(t))dt+√θnˆY(t)(1−ˆY(t))dW(t) | (3.10) |
where W={W(t),t≥0} is a standard Brownian motion. The approximation of diffusion processes by Markovian chains is well explained in [20] (Chapter 10).
If the variability is assumed to be small then the noise term of the stochastic differential equation (3.10) will have little effect. In that case, we can presume that the mean of ˆY(t) can be approximated by ˆm(t) where ˆm satisfies the deterministic equation
dˆm(t)=θˆm(t)(1−ˆm(t))dt |
with explicit solution
ˆm(t)=11+1−ˆm0ˆm0e−θt,t≥0 |
where ˆm0=E[ˆYn(0)]=1nE[Y(0)].
This suggests that for t=0,1,2,… (with attendant scaling up of error terms),
E[Y(t)]=nE[ˆYn(tn)]≈nˆm(tn)=n1+n−y0y0e−pNt |
where y0=E[Y(0)].
The aim of this chapter is to simulate the SIR epidemic model we have presented in the previous sections, comparing the evolution of the disease for different distributions of the number of daily contacts N(t). Particularly, we study the cases when N(t) follows two distributions: binomial and truncated Poisson. We will compare the results for different values of their parameters.
Since we are interested in showing the different evolution of the SIR epidemic model as the number of daily contacts changes, we fix the other parameters. We consider a population of n=10000 individuals with a unique initial infective individual: Y(0)=1. The probability of transmission derived from an encounter is p=0.1 and the duration of the disease is r=5. We assume the quarantine is nonexistent, that is, λ=1.
For each case, we present the average of the duration of the disease (Total time), the total proportion of the infected population when the disease ends (Population infected) and the number of individuals infected by the first infective (~R0). Also, R∗0=λp∑r−1t=0E(N(t)) is computed. The theoric R0 will be R∗0 with an error of O(1n−1).
The simulations are made using R programming environment. All of them are over 10,000 trials and run for a maximum of 365 days.
Here, the number of daily contacts N(t) has a binomial distribution with probability depending on time. This is N(t)∼Binom(N,p(t)). With this distribution, the set of all possible values of N(t) is M={0,…,N}.
We consider three cases. In all of them, the function p(t) is periodic with yearly, monthly and weekly periods, respectively. In the first case, we fix a value for N and compare the spread of the disease for four different functions of p(t). In the other two cases, we choose a function for p(t) and observe how the disease evolves when the maximum number of daily contacts changes.
It is a natural assumption that the number of daily contacts varies all over the year and changes according to the season. We suppose that the mean of daily encounters arrives at its maximum in summer, decreases in fall until arriving at its minimum in winter and then increases in spring and summer again. Moreover, we assume that p(t) has a sinusoidal shape where the peak coincides with summer and the valley with winter and we analyze the spread of the disease depending on the epoch of the year it starts. The nomenclature we use refers to this epoch. The following plot suggests the idea:
![]() |
The four functions we consider are:
Spring:p(t)=0.3⋅sin(2πt365)+0.5Summer:p(t)=0.3⋅cos(2πt365)+0.5Fall:p(t)=−0.3⋅sin(2πt365)+0.5Winter:p(t)=−0.3⋅cos(2πt365)+0.5 |
All of them oscillate between 0.2 and 0.8. We fix the maximum number of daily contacts N at 10.
Figure 1 shows the evolution of the mean number of infectives per day. The graphic has been truncated at 110 days, as the number of cases of the following days is almost irrelevant. We have a fast spread of the disease in the Summer case which coincides with the time when the number of encounters is higher. By reducing this number the disease presents a lower mean number of infectives per day but it lasts longer. Observe that in Spring and Fall p(t) has the same initial value p(0)=0.5, but the model evolves differently depending on whether this probability increases or decreases.
All these facts are underlined in Figure 2 which represents the histogram of the duration of the disease (Left) and the histogram of the total number of infected in the population when the disease ends (Right). Moreover, it is interesting to notice that, while in Spring and Summer almost the entire population gets infected in most trials, in Winter the epidemic develops only in a limited number of cases.
The first two rows of Table 1 help to better understand Figure 2 (Left and Right). The course of the disease is on average longer in Fall but it has a big variation and it is on average shorter in Winter with an even bigger dispersion. This is evident in Figure 2 (Left) where in Winter we notice two different behaviors: in most of the trials the disease lasts for a short time and does not exceed 50 days but in the other cases it lasts for more than 100 days. As remarked before, almost the entire population is infected in Spring and Summer but this proportion decreases drastically in Fall and Winter.
Spring | Summer | Fall | Winter | |
Total time | 43.54 (12.77) | 35.33 (5.05) | 60.83 (21.92) | 29.50 (42.08) |
Population infected (%) | 86% (28%) | 96% (14%) | 61% (23%) | 14% (31%) |
~R0 | 2.53 (1.26) | 3.97 (1.43) | 2.44 (1.25) | 1.00 (0.90) |
R∗0 | 2.55 | 4.00 | 2.45 | 1.00 |
Table 1 also contains information regarding the number of individuals infected by the first infective (~R0). If compared with R∗0 (last row) very similar values are observed. Recall that R∗0 is an approximation of the theoretical value R0. In the first three cases, ~R0 exceeds the threshold value 1 implying that the disease turns into an epidemic. In the last case, the mean of ~R0 coincides with the threshold itself but its variability makes it unclear if an epidemic occurs on average or not. Moreover, observe that ~R0 is very similar in Spring and Fall.
Finally, notice that the dispersion is smaller in Summer and bigger in Winter when we refer to the duration of the disease and the total proportion of infected but it is reversed when we consider ~R0.
We assume that the number of daily contacts changes periodically over the course of the month. This behavior could be due to economic reasons. For example, it might catch the tendency to go out more when a salary is perceived. The periodicity is reflected in p(t), that is the sinusoidal function
p(t)=0.3⋅sin(2πt30)+0.5. |
This probability oscillates between 0.2 and 0.8. We simulate and then compare the SIR epidemic model for three different values of the maximum number of daily contacts: N=7, N=10 and N=12.
Figure 3(Left) shows the evolution of the mean number of infectives per day in the three cases. The graphic has been truncated at 100 days. We see that the evolution of the disease behaves differently with respect to the maximum number of daily encounters. Particularly, the number and position of the peaks vary. When N=7, there are three peaks, the central one big enough and the others very small. When the maximum number of daily contacts is set to N=10, two similar peaks are displayed. Finally, when this parameter is increased to N=12 a tall peak is followed by a small one.
It might be interesting to investigate how the mean number of infectives presents two peaks in the case N=10. Figure 3(Right) shows the evolution of the number of infectives per day for some selected simulations. Observe that the trials behave quite differently: in some cases there are two (similar or not) peaks and in others only one. However, it is also important to point out that all peaks occur at similar times as those of the mean.
It appears that on average the disease lasts longer when the number of daily contacts is smaller. This is noted in Figure 4 (Left) and Table 2. In addition, here we observe a bigger variation for smaller values of N. For example, the standard deviation of the case N=7 is more than double that of the case N=10. This is also due to the fact that the disease does not spread in more simulations of the case N=7 than of the others, as pointed out in Figure 4 (Left).
N=7 | N=10 | N=12 | |
Total time | 67.14 (27.62) | 58.40 (13.41) | 49.18 (11.84) |
Population infected (%) | 63% (26%) | 81% (19%) | 85% (14%) |
~R0 | 2.17 (1.20) | 3.07 (1.33) | 3.70 (1.40) |
R∗0 | 2.16 | 3.08 | 3.70 |
Figure 4 (Right) shows the distribution of the total number of infected over all simulations. When the number of contacts increases, this quantity is on average bigger but its variation decreases, as indicated in Table 2. Note that the cases in which the total population is infected are very few.
Finally, Table 2 reveals that the mean number of individuals infected by the first infective increases as the maximum number of daily encounters does. Its variation follows the same tendency although the differences are quite small.
Now, we assume that the number of daily contacts changes periodically with a week period. For example, this could mark the different behavior of the population at the weekend with respect to the weekdays. The periodicity is reflected in p(t), that is the sinusoidal function
p(t)=0.3⋅cos(2πt7)+0.5. |
This probability oscillates between 0.2 and 0.8. As in the monthly-periodic study, we simulate and then compare the SIR epidemic model for three different values of the maximum number of daily contacts: N=7, N=10 and N=12.
Figure 5 (Left) plots the evolution of the mean number of infectives in the three cases. The graphic has been truncated at 100 days. One could observe that the results are very different depending on the values of N. When N=7, the mean number of infectives oscillates many times presenting several peaks but none of them is particularly high. Some selected simulations are displayed in Figure 5 (Right). They seem to reflect the same tendency as the mean. When N=10 and N=12, only one peak is displayed but the curves are not similar to those of a normal distribution as they were for the yearly and monthly periodic studies. Furthermore, the different shapes the two of them presented make their behavior quite distinct.
The histograms in Figure 6 (Left and Right) also highlight the differences that the change in the number of daily contacts generates. We observe two features also reported in the monthly-periodic study. On one hand, the disease lasts longer on average when the number of daily contacts is smaller. On the other hand, the magnitude of the spread, intended as the part of the total population infected, decreases. Furthermore, note that when N=7 the number of trials in which the disease does not spread is much higher than in the other two cases. This increases the variability of the results, as can be observed in Table 3.
N=7 | N=10 | N=12 | |
Total time | 63.01 (40.58) | 50.71 (17.87) | 44.45 (11.70) |
Population infected (%) | 45% (32%) | 76% (29%) | 86% (25%) |
~R0 | 1.67 (1.13) | 2.39 (1.28) | 2.85 (1.36) |
R∗0 | 1.67 | 2.38 | 2.86 |
The dispersion of the mean number of individuals infected by the first infective is also very high in all cases (see Table 3). Particularly, when N=7 and N=10 it is difficult to know if the spread of the disease will result in an epidemic or not.
Now, we assume that the number of daily contacts N(t) follows a truncated Poisson distribution. This is the distribution of a Poisson random variable conditional on the fact that it takes values only in an interval. In our case, we fix a maximum number of daily contacts. We set this upper bound at 15 encounters.
We consider three different functions for the parameter λ depending on t:
λ1(t)=101+log(t+1)λ2(t)=505+2√tλ3(t)=−5sin(2πt15+k)+7withk=arcsin(−35) |
where k is an adjustment constant so that all functions start at 10 when t=0. Over the course of a year (365 days), λ1(t) takes values between 1.45 and 10, λ2(t) between 1.16 and 10 and λ3(t) between 2 and 12 (all these values are rounded to two decimals). We can see in Figure 7 (Right) the behavior of the three functions we have considered.
Figure 7 (Left) shows the evolution of the mean number of infectives per day. We notice important differences between the first two cases although the two parameters λ1(t) and λ2(t) present a similar evolution in time. The third case goes fast to zero despite the periodic behavior of the parameter λ3(t).
From Figure 8 (Left) and Table 4 we can see that for the case λ1(t) there is a lot of dispersion of the total duration of the disease which takes values from 0 to 125 days. For the other two cases, the dispersion is much smaller and the duration of the case λ1(t) is the smallest one. Concerning the total number of infected, the biggest dispersion is presented by the case λ3(t) since there are almost 1, 000 simulations that end without infecteds and much more with almost the entire population infected. The case λ1(t) is the one that presents a less infected population and also the one with less dispersion. In all three cases, ~R0 exceeds the threshold value 1 so the disease turns into an epidemic. Moreover, its values are similar to the expected ones given by R∗0.
λ1(t) | λ2(t) | λ3(t) | |
Total time | 91.71 (39.92) | 68.57 (16.87) | 42.45 (11.85) |
Population infected (%) | 9% (7%) | 59% (14%) | 86% (28%) |
~R0 | 2.83 (1.36) | 3.45 (1.42) | 3.05 (1.36) |
R∗0 | 2.83 | 3.46 | 3.05 |
In this chapter, we investigate how the SIR model we have proposed in the previous sections can approximate the spread of a real disease. For this purpose, we consider two diseases: influenza and meningococcal infection. The real data we study are part of the data frame InfluMen contained in the package surveillance of R programming, focused on modeling and monitoring epidemic phenomena. The data frame contains the cases observed in Germany between 2001 and 2006. We intentionally analyze only one year for each disease (2001 for influenza and 2006 for meningococcal infection), as the methods we employ can be applied to other periods. In the case of influenza, the development of the disease follows a similar pattern each year. The curve presents the same shape, so an adjusted model can be obtained by slightly varying the parameters. In the case of meningococcal disease, the behavior of the number of infectives is quite rough. The model has to be adjusted more carefully, but equally good results can be achieved with a suitable choice of the number of daily encounters.
We consider the cases of influenza registered in Germany in 2001. We compare this data with our SIR model. In that year, the German population was 82.35 million, so we fix n accordingly. The duration of the disease is estimated between 3 and 7 days, thus we choose r=5. The probability of transmission is fixed at p=0.062. Since we do not know the level of quarantine, we set λ=1. We assume that the number of daily encounters follows a truncated Poisson distribution with a parameter λ(t) that is a slight variation of the function λ2(t) used in Section 4.2:
λ(t)=201+√t. |
We run our simulation for a year (365 days). The results obtained are contained in Figure 9.
In Figure 9 (Left) real data are compared to the mean number of infectives per day. In Figure 9 (Right) three single simulations are plotted. In both cases, our model adjusts remarkably well to real data.
Using the parameters set for the model, we obtain the approximation of the basic reproduction number R∗0=λp∑r−1t=0E(N(t))=2.8. This value suggests that an outbreak could occur. However, remember that it is computed only considering the number of daily encounters during the first r days which means that the subsequent development of the disease may be different than expected.
Meningococcal disease refers to any illness caused by bacteria called Neisseria meningitidis. About 10% of people have these bacteria without being ill. Sometimes the bacteria invade the body and cause certain illnesses known as meningococcal disease. Meningococcal bacteria are spread by respiratory and throat secretions. Generally, it takes close or prolonged contact to spread them. Fortunately, they are not as contagious as the germs that cause the common cold or flu. This disease is often severe and can be deadly.
We consider the cases of meningococcal disease registered in Germany in 2006. These data are compared with our SIR model. In 2006, the German population was 82.38 million so n is fixed to this quantity. The duration of meningococcal infection is very variable. Even if it is typically 3 to 4 days, it can last a range of 1 to 10 days. Under these assumptions, we find it opportune to set r=5. As the probability of transmission is quite low, we set p=0.02. Since we do not know the level of quarantine, we set λ=1. In this case, we assume that the number of daily encounters follows a binomial distribution with parameters N and p(t). N is fixed to 12 and p(t) is a step function that varies from 0.55 to 1. We run our simulation for a year (365 days). The results obtained are shown in Figure 10.
The figure compares real data with the mean number of infectives per day simulated using our SIR model. In the early days, the simulation does not adjust much to the real data. This can be explained because contrary to our model not all infected at time t=0 belong to the class Y0(0). Apart from that, our model adjusts rather well to real data. This result could not have been achieved without taking into account the dependence on time of the number of daily encounters.
As for the influenza, we can estimate the basic reproduction number by computing its approximation R∗0=λp∑r−1t=0E(N(t))=0.96. However, its value is closely related to the number of daily encounters in the first r days which corresponds to the time when our simulation does not fit the real data accurately, as explained above.
In this paper, we study a stochastic SIR-type epidemic model that is an extension of the one proposed by Tuckwell and Williams. We assume that the number of daily encounters of each individual depends on time and we add a parameter to control a possible quarantine of the infectious individuals. Two cases are taken into consideration: when the duration of the disease is constant and when infectious individuals remain infectious throughout their lives. In both situations, we describe analytically the underlying model and its dynamics, deriving a diffusion process and the basic reproduction number.
Several simulations are made to show how differently the disease evolves with respect to the distribution of the number of daily encounters. We consider two laws for this parameter: binomial and truncated Poisson. They will both be used in the application of our model to real diseases. We show how not only the variation of distribution but also the change of their parameters could generate a great variability of results. In particular, the evolution of the mean number of infectives can present different shapes and peaks. Also, the total duration of the disease (intended as the total time before the disease ends) and the total proportion of population infected behave differently. Moreover, we check that in all simulations the mean number of individuals infected by the first infective ~R0 adjusts to R∗0, which is an approximation of the basic reproduction number.
The importance of time dependence stands out when we apply our model to real diseases. We consider two cases: influenza and meningococcal infection. In both situations, the flexibility given by the time dependence allows our model to adjust exceptionally well to real data.
These results can be the springboard for constructing more complex models to investigate diseases that cannot be adequately described by only the three classes S, I and R. For example, diseases with an initial latency period or the presence of asymptomatic individuals. In these cases, it could be interesting to explore how different distributions and the dependence on time of some parameters such as the number of daily encounters can affect the evolution of the disease.
Another future work could be to consider other parameters as random quantities. In a real-life situation, the duration of the disease r is generally not fixed and can vary from one individual to another. Therefore, an interesting case would be to study a model that deals with this parameter as a random variable and investigate how the disease behaves when its distribution varies.
The authors declare they have not used Artificial Intelligence (AI) tools in the creation of this article.
Mireia Besalú is supported by Departament de Recerca i Universitats de la Generalitat de Catalunya (Spain) with the grant 2021 SGR 01421 (GRBIO).
Giulia Binotto is supported by Ministerio de Ciencia e Innovación (Spain) with the grant PID2021‐123733NB‐I00.
All authors declare no conflicts of interest in this paper.
[1] | D. Bernoulli, Essai d'une nouvelle analyse de la mortalité causée par la petite vérole, et des avantages de l'Inoculation pour la prévenir, Hist. Acad. R. Sci. Paris, 1760, 1–45. |
[2] | N. T. Bailey, The mathematical theory of infectious diseases and its applications, London: Charles Griffin & Company Limited, 1975. |
[3] | R. M. Anderson, R. M. May, Infectious diseases of humans. Dynamics and control, Oxford: Oxford University Press, 1991. https://doi.org/10.1002/hep.1840150131 |
[4] | O. Diekmann, H. Heesterbeek, T. Britton, Mathematical tools for understanding infectious disease dynamics, Princeton: Princeton University Press, 2013. |
[5] |
Y. Cai, Y. Kang, W. Wang, A stochastic SIRS epidemic model with nonlinear incidence rate, Appl. Math. Comput., 305 (2017), 221–240. https://doi.org/10.1016/j.amc.2017.02.003 doi: 10.1016/j.amc.2017.02.003
![]() |
[6] |
X. Bardina, M. Ferrante, C. Rovira, A stochastic epidemic model of COVID-19 disease, AIMS Math., 5 (2020), 7661–7677. https://doi.org/10.3934/math.2020490 doi: 10.3934/math.2020490
![]() |
[7] |
F. Flandoli, E. La Fauci, M. Riva, Individual-based Markov model of virus diffusion: Comparison with COVID-19 incubation period, serial interval and regional time series, Math. Mod. Meth. Appl. Sci., 31 (2021), 907–939. https://doi.org/10.1142/S0218202521500226 doi: 10.1142/S0218202521500226
![]() |
[8] |
A. McKendrick, Application of mathematics to medical problems, Proc. Edinburgh Math. Soc., 14 (1926), 98–130. https://doi.org/10.1017/S0013091500034428 doi: 10.1017/S0013091500034428
![]() |
[9] |
W. Kermack, A. McKendrick, A contribution to the mathematical theory of epidemics, Proc. Roy. Soc. Lond., 115 (1927), 700–721. https://doi.org/10.1098/rspa.1927.0118 doi: 10.1098/rspa.1927.0118
![]() |
[10] | H. Abbey, An examination of the Reed-Frost theory of epidemics, Hum. Biol., 24 (1952), 201–233. |
[11] | L. J. S. Allen, An introduction to stochastic epidemic models, In: Mathematical Epidemiology, Berlin, Heidelberg: Springer-Verlag, 2008, 81–130. https://doi.org/10.1007/978-3-540-78911-6 |
[12] |
H. C. Tuckwell, R. J. Williams, Some properties of a simple stochastic epidemic model of SIR type, Math. Biosci., 208 (2007), 76–97. https://doi.org/10.1016/j.mbs.2006.09.018 doi: 10.1016/j.mbs.2006.09.018
![]() |
[13] |
M. Ferrante, E. Ferraris, C. Rovira, On a stochastic epidemic SEIHR model and its diffusion approximation, Test, 25 (2016), 482–502. https://doi.org/10.1007/s11749-015-0465-z doi: 10.1007/s11749-015-0465-z
![]() |
[14] |
A. Gray, D. Greenhalgh, L. Hu, X. Mao, J. Pan, A stochastic differential equation SIS epidemic model, SIAM J. Appl. Math., 71 (2011), 876–902. https://doi.org/10.1137/10081856X doi: 10.1137/10081856X
![]() |
[15] |
T. Tsutsui, N. Minamib, M. Koiwai, T. Hamaokaa, I. Yamanea, K. Shimura, A stochastic-modeling evaluation of the foot-and-mouth-disease survey conducted after the outbreak in Miyazaki, Japan in 2000, Prev. Vet. Med., 61 (2003), 45–58. https://doi.org/10.1016/s0167-5877(03)00160-0 doi: 10.1016/s0167-5877(03)00160-0
![]() |
[16] |
S. Z. Huang, A new SEIR epidemic model with applications to the theory of eradication and control of diseases, and to the calculation of R0, Math. Biosci., 215 (2008), 84–104. https://doi.org/10.1016/j.mbs.2008.06.005 doi: 10.1016/j.mbs.2008.06.005
![]() |
[17] | S. E. A. Mohammed, Stochastic differential systems with memory: Theory, examples and applications, In: Stochastic Analysis and related topics Ⅵ, Boston: Birkhaüser, 1998. https://doi.org/10.1007/978-1-4612-2022-0_1 |
[18] |
H. Wahlstrom, L. Englund, T. Carpenter, U. Emanuelson, A. Engvall, I. Vagsholm, A Reed-Frost model of the spread of tuberculosis within seven Swedish extensive farmed fallow deer herds, Prev. Vet. Med., 35 (1998), 181–193. https://doi.org/10.1016/s0167-5877(98)00061-0 doi: 10.1016/s0167-5877(98)00061-0
![]() |
[19] |
J. Ng, E. J. Orav, A generalized chain-binomial model with application to HIV infection, Math. Biosci., 101 (1990), 99–119. https://doi.org/10.1016/0025-5564(90)90104-7 doi: 10.1016/0025-5564(90)90104-7
![]() |
[20] | O. C. Ibe, Markov processes for stochastic modeling, Elsevier, 2013. |
Spring | Summer | Fall | Winter | |
Total time | 43.54 (12.77) | 35.33 (5.05) | 60.83 (21.92) | 29.50 (42.08) |
Population infected (%) | 86% (28%) | 96% (14%) | 61% (23%) | 14% (31%) |
~R0 | 2.53 (1.26) | 3.97 (1.43) | 2.44 (1.25) | 1.00 (0.90) |
R∗0 | 2.55 | 4.00 | 2.45 | 1.00 |
N=7 | N=10 | N=12 | |
Total time | 67.14 (27.62) | 58.40 (13.41) | 49.18 (11.84) |
Population infected (%) | 63% (26%) | 81% (19%) | 85% (14%) |
~R0 | 2.17 (1.20) | 3.07 (1.33) | 3.70 (1.40) |
R∗0 | 2.16 | 3.08 | 3.70 |
N=7 | N=10 | N=12 | |
Total time | 63.01 (40.58) | 50.71 (17.87) | 44.45 (11.70) |
Population infected (%) | 45% (32%) | 76% (29%) | 86% (25%) |
~R0 | 1.67 (1.13) | 2.39 (1.28) | 2.85 (1.36) |
R∗0 | 1.67 | 2.38 | 2.86 |
λ1(t) | λ2(t) | λ3(t) | |
Total time | 91.71 (39.92) | 68.57 (16.87) | 42.45 (11.85) |
Population infected (%) | 9% (7%) | 59% (14%) | 86% (28%) |
~R0 | 2.83 (1.36) | 3.45 (1.42) | 3.05 (1.36) |
R∗0 | 2.83 | 3.46 | 3.05 |
Spring | Summer | Fall | Winter | |
Total time | 43.54 (12.77) | 35.33 (5.05) | 60.83 (21.92) | 29.50 (42.08) |
Population infected (%) | 86% (28%) | 96% (14%) | 61% (23%) | 14% (31%) |
~R0 | 2.53 (1.26) | 3.97 (1.43) | 2.44 (1.25) | 1.00 (0.90) |
R∗0 | 2.55 | 4.00 | 2.45 | 1.00 |
N=7 | N=10 | N=12 | |
Total time | 67.14 (27.62) | 58.40 (13.41) | 49.18 (11.84) |
Population infected (%) | 63% (26%) | 81% (19%) | 85% (14%) |
~R0 | 2.17 (1.20) | 3.07 (1.33) | 3.70 (1.40) |
R∗0 | 2.16 | 3.08 | 3.70 |
N=7 | N=10 | N=12 | |
Total time | 63.01 (40.58) | 50.71 (17.87) | 44.45 (11.70) |
Population infected (%) | 45% (32%) | 76% (29%) | 86% (25%) |
~R0 | 1.67 (1.13) | 2.39 (1.28) | 2.85 (1.36) |
R∗0 | 1.67 | 2.38 | 2.86 |
λ1(t) | λ2(t) | λ3(t) | |
Total time | 91.71 (39.92) | 68.57 (16.87) | 42.45 (11.85) |
Population infected (%) | 9% (7%) | 59% (14%) | 86% (28%) |
~R0 | 2.83 (1.36) | 3.45 (1.42) | 3.05 (1.36) |
R∗0 | 2.83 | 3.46 | 3.05 |