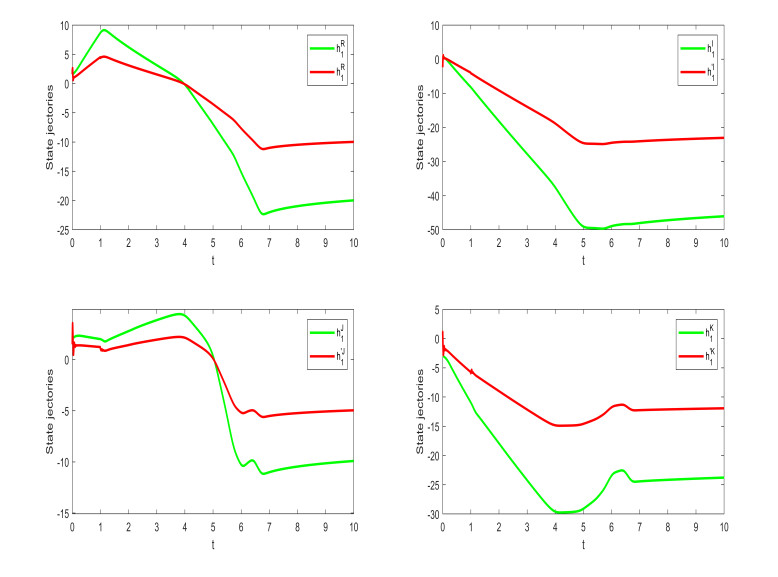
This paper is devoted to discussing the globally projective synchronization of Caputo fractional-order quaternion-valued neural networks (FOQVNNs) with discrete and distributed delays. Without decomposing the FOQVNNs into several subsystems, by employing the Lyapunov direct method and inequality techniques, the algebraic criterion for the globally projective synchronization is derived. The effectiveness of the proposed result is illustrated by the MATLAB toolboxes and numerical simulation.
Citation: Chen Wang, Hai Zhang, Hongmei Zhang, Weiwei Zhang. Globally projective synchronization for Caputo fractional quaternion-valued neural networks with discrete and distributed delays[J]. AIMS Mathematics, 2021, 6(12): 14000-14012. doi: 10.3934/math.2021809
[1] | Zhifeng Lu, Fei Wang, Yujuan Tian, Yaping Li . Lag synchronization of complex-valued interval neural networks via distributed delayed impulsive control. AIMS Mathematics, 2023, 8(3): 5502-5521. doi: 10.3934/math.2023277 |
[2] | Rakkiet Srisuntorn, Wajaree Weera, Thongchai Botmart . Modified function projective synchronization of master-slave neural networks with mixed interval time-varying delays via intermittent feedback control. AIMS Mathematics, 2022, 7(10): 18632-18661. doi: 10.3934/math.20221025 |
[3] | Dong Pan, Huizhen Qu . Finite-time boundary synchronization of space-time discretized stochastic fuzzy genetic regulatory networks with time delays. AIMS Mathematics, 2025, 10(2): 2163-2190. doi: 10.3934/math.2025101 |
[4] | Shuang Li, Xiao-mei Wang, Hong-ying Qin, Shou-ming Zhong . Synchronization criteria for neutral-type quaternion-valued neural networks with mixed delays. AIMS Mathematics, 2021, 6(8): 8044-8063. doi: 10.3934/math.2021467 |
[5] | Jin Gao, Lihua Dai . Anti-periodic synchronization of quaternion-valued high-order Hopfield neural networks with delays. AIMS Mathematics, 2022, 7(8): 14051-14075. doi: 10.3934/math.2022775 |
[6] | Chengqiang Wang, Xiangqing Zhao, Yang Wang . Finite-time stochastic synchronization of fuzzy bi-directional associative memory neural networks with Markovian switching and mixed time delays via intermittent quantized control. AIMS Mathematics, 2023, 8(2): 4098-4125. doi: 10.3934/math.2023204 |
[7] | Călin-Adrian Popa . Synchronization of Clifford-valued neural networks with leakage, time-varying, and infinite distributed delays on time scales. AIMS Mathematics, 2024, 9(7): 18796-18823. doi: 10.3934/math.2024915 |
[8] | Hongmei Zhang, Xiangnian Yin, Hai Zhang, Weiwei Zhang . New criteria on global Mittag-Leffler synchronization for Caputo-type delayed Cohen-Grossberg Inertial Neural Networks. AIMS Mathematics, 2023, 8(12): 29239-29259. doi: 10.3934/math.20231497 |
[9] | Shuo Ma, Jiangman Li, Qiang Li, Ruonan Liu . Adaptive exponential synchronization of impulsive coupled neutral stochastic neural networks with Lévy noise and probabilistic delays under non-Lipschitz conditions. AIMS Mathematics, 2024, 9(9): 24912-24933. doi: 10.3934/math.20241214 |
[10] | Yang Xu, Zhouping Yin, Yuanzhi Wang, Qi Liu, Anwarud Din . Mittag-Leffler projective synchronization of uncertain fractional-order fuzzy complex valued neural networks with distributed and time-varying delays. AIMS Mathematics, 2024, 9(9): 25577-25602. doi: 10.3934/math.20241249 |
This paper is devoted to discussing the globally projective synchronization of Caputo fractional-order quaternion-valued neural networks (FOQVNNs) with discrete and distributed delays. Without decomposing the FOQVNNs into several subsystems, by employing the Lyapunov direct method and inequality techniques, the algebraic criterion for the globally projective synchronization is derived. The effectiveness of the proposed result is illustrated by the MATLAB toolboxes and numerical simulation.
In recent decades, the integer-order real-valued neural networks (RVNNs) and integer-order complex-valued neural networks (CVNNs) have been extensively studied and applied in pattern recognition, signal processing, associative memory [1,2,3] and so on. Nonetheless, the multidimensional data processing cannot be solved through RVNNs or CVNNs such as body images and color images [4]. As the quaternion-valued neurons processing is more efficiently and compactly, more and more scholars have combined the quaternion valued with the conventional neural network to study the QVNNs. It is widely known that three feasible methods are generally used to discuss the dynamic behaviors of integer-order QVNNs: the decomposition of integer-order QVNNs into four RVNNs [5]; the decomposition of integer-order QVNNs into two CVNNs [6]; the discussion of integer-order QVNNs as a whole [7].
Time delay is a common phenomenon in neural networks (NNs), which will produce oscillation, chaos and instability. The limited transmission speed and traffic congestion of neural networks make discrete delays inevitable [8]. Furthermore, the large number of parallel paths of varying axon size and length make a certain spatial extent frequently present in the NNs, which is the distribution delay [9]. Recently, a flurry of research has been done on the stabilization and synchronization of integer-order NNs with mixed delays. In [10], Yang et al. studied the finite-time stability of NNs with mixed delays. In [11], Song and Wen investigated the exponential synchronization of memristor-based NNs with random mixed delays. The study of synchronization with mixed delays still attracts a large number of scholars' attention.
Synchronization of NNs is an interesting and crucial dynamic behaviors that is extensive applied in information science, signal processing, secure communication. Researchers have proposed several synchronization patterns including phase synchronization [12], projective synchronization [13], exponential synchronization [14], Mittag-Leffler synchronization [15], as well as plentiful results have been obtained. The projective synchronization means that the slave system synchronizes with the master system by a specific scale factor under the appropriate controller.
Note that the fractional order model can provide more accurate description of the memory and genetic characteristics than the integer order model. To our knowledge, many results have been achieved in analysing the dynamics of FOQVNNs [16,17,18,19,20]. In [17], Yang et al. investigated the global Mittag-Leffler synchronization of FOQVNNs by applying a linear feedback controller with transforming the system into four equivalent real-valued systems. In [19], Li et al. studied the global asymptotic synchronization of FOQVNNs by choosing an appropriate controller and Lyapunov function. However, the results of FOQVNNs with discrete delay and distributed delay are very rare. Based on these considerations, the aim of this paper focuses on the projective synchronization of FOQVNNs with discrete and distributed delays. The highlights of this paper are shown as below.
∙ The paper is concerned with the FOQVNNs including the discrete delay and distributed delay, and the considered models are more general and less conservative.
∙ The method of this paper is the direct quaternion approach rather than decomposing FOQVNNs into subsystems, which significantly reduces the complexity of the calculation.
∙ The synchronisation conditions are described by the algebraic inequality forms, which are easy to check in practical applications.
In this section, we will describe the related notations and basic concepts with regard to fractional calculation. Moreover, the FOQVNNs model and several lemmas are introduced.
Notations: The quaternion valued function hp(⋅) is defined as hp=hRp+ihIp+jhJp+khKp, where hRp,hIp,hJp,hKp∈R, i,j,k satisfy the Hamilton rules: ij=−ji=k,jk=−kj=i,ki=−ik=j,i2=j2=k2=−1;¯hp=hRp−ihIp−jhJp−khKp is the conjugate of hp, |hp|=√¯hphp=√hp¯hp denotes the module of hp. For h=(h1,h1,...,hn)T∈Qn,||h||=(n∑p=1|hp|2)12 represents the norm of h.
Definition 1. ([21]) The fractional integral of function φ(t) with order q>0 is defined as:
I−qφ(t)=1Γ(q)∫t0(t−s)q−1φ(s)ds, q>0. | (2.1) |
Definition 2. ([21]) The Caputo derivative of fractional 0<q<1 of function φ(t) is defined as:
Dqφ(t)=1Γ(1−q)∫t0φ′(s)(t−s)qds, t>0. | (2.2) |
Remark 1. Compared with the Riemann-Liouville derivative in [21], the Caputo type fractional-order derivative of a constant is identically equal to zero, that is not the case for the Riemann-Liouville type fractional-order derivative. Moreover, the Caputo type fractional-order derivative is a formal generalization of the integer-order derivative in the sense of the Laplace transformation. Based on the above considerations, the Caputo derivative has been adopted in this paper.
Lemma 1. ([22,23]) If the function φ(⋅)∈Q is differentiable, then
Dq(¯φ(t)φ(t))⩽¯φ(t)Dqφ(t)+¯(Dqφ(t))φ(t), t⩾0, 0<q<1. | (2.3) |
Lemma 2. ([24]) For any η,μ∈Q, the following inequality holds:
η¯μ+¯ημ⩽η¯η+μ¯μ. | (2.4) |
Lemma 3. ([25]) Assuming that V(⋅)∈R be bounded and continuous. If there exist χ>φ>0 such that
DqV(t)⩽−χV(t)+φsupt1⩽ω⩽t2V(t+ω), 0<q<1, | (2.5) |
then limt→+∞V(t)=0.
Next, consider the FOQVNNs model including discrete and distributed delays as follows:
Dqhp(t)=−aphp(t)+n∑q=1bpqfq(hq(t))+n∑q=1cpqfq(hq(t−τ1))+n∑q=1dpq∫tt−τ2fq(hq(s))ds+Ip, | (2.6) |
where 0<q<1,hp(⋅)∈Q denotes the state vector of pth; ap∈R stand for the self-regulating coefficient; respectively, bpq,cpq,dpq∈Q are the connection weights; τ1,τ2 represent the delay; Ip∈Q is the external input; f(⋅)∈Q denotes the activation function. For any hq,h′q∈Q, the activation function fq(⋅) satisfies the following Lipschitz condition:
|fq(hq)−fq(h′q)|⩽lq|hq−h′q|, | (2.7) |
where lq is a positive constant.
Consider system (2.6) as master system, and the controlled slave model is depicted below
Dqh′p(t)=−aph′p(t)+n∑q=1bpqfq(h′q(t))+n∑q=1cpqfq(h′q(t−τ1))+n∑q=1dpq∫tt−τ2fq(h′q(s))ds+Ip+Up(t), | (3.1) |
where Up(⋅)∈Q is the controller.
The error is ep(t)=h′p(t)−ζhp(t), and the hybrid quaternion-valued controller Up(⋅) is defined as follows:
Up(t)=n∑q=1bpq[ζfq(h′q(t))−fq(ζhq(t))]+n∑q=1cpq[ζfq(h′q(t−τ1))−fq(ζhq(t−τ1))]+n∑q=1dpq∫tt−τ2[ζfq(h′q(s))−fq(ζhq(s))]ds+[ζ−1]Ip. | (3.2) |
Combining (2.6), (3.1) and (3.2), the error system is
Dqep(t)=−apep(t)+n∑q=1bpq[fq(h′q(t))−fq(ζhq(t))]+n∑q=1cpq[fq(h′q(t−τ1))−fq(ζhq(t−τ1))]+n∑q=1dpq∫tt−τ2[fq(h′q(s))−fq(ζhq(s))]ds. | (3.3) |
Theorem 1. Suppose that the activation function fq(⋅) satisfies the Lipschitz condition (2.7), for the controller Up(⋅) in (3.2), if there exist μ1>0, μ2>0, μ3>0, such that for some α>0, the following LMI holds:
Φ=(2(ap−32n)−μ1−n∑q=1l21|b2pq|⋆⋆⋆⋆μ2−n∑q=1l22|c2pq|⋆⋆⋆⋆μ3−n∑q=1l23|d2pq|τ22⋆⋆⋆⋆μ1−μ2α)>0, | (3.4) |
then system (2.6) and (3.1) can realize globally projective synchronization.
{Proof.} Choosing a Lyapunov function
V(t)=n∑p=1¯ep(t)ep(t). | (3.5) |
From Lemma 1, we can get that
DqV(t)⩽ n∑p=1[ep(t)Dq¯ep(t)+¯ep(t)Dqep(t)]=n∑p=1ep(t){−ap¯ep(t)+n∑q=1[¯fq(h′q(t))−fq(ζhq(t))]¯bpq+n∑q=1[¯fq(h′q(t−τ1))−fq(ζhq(t−τ1))]¯cpq+n∑q=1∫tt−τ2[¯fq(h′q(s))−fq(ζhq(s))]ds¯dpq}+n∑q=1¯ep(t){−apep(t)+n∑q=1bpq[fq(h′q(t))−fq(ζhq(t))]+n∑q=1cpq[fq(h′q(t−τ1))−fq(ζhq(t−τ1))]+n∑q=1dpq∫tt−τ2[fq(h′q(s))−fq(ζhq(s))]ds}=−n∑q=1ap[¯ep(t)ep(t)+ep(t)¯ep(t)]+n∑p=1n∑q=1{ep(t)[¯fq(h′q(t))−fq(ζhq(t))]¯bpq+¯ep(t)bpq[fq(h′q(t))−fq(ζhq(t))]}+n∑p=1n∑q=1{ep(t)[¯fq(h′q(t−τ1))−fq(ζhq(t−τ1))]¯cpq+¯ep(t)cpq[fq(h′q(t−τ1))−fq(ζhq(t−τ1))]}+n∑p=1n∑q=1{ep(t)∫tt−τ2¯[fq(h′q(s))−fq(ζhq(s))]ds¯dpq+¯ep(t)dpq∫tt−τ2[fq(h′q(s))−fq(ζhq(s))]ds}. | (3.6) |
According to the Lipschitz condition (2.7) and Lemma 2, we have
n∑p=1n∑q=1{ep(t)[¯fq(h′q(t))−fq(ζhq(t))]¯bpq+¯ep(t)bpq[fq(h′q(t))−fq(ζhq(t))]}⩽ n∑p=1n∑q=1{¯ep(t)ep(t)+[¯fq(h′q(t))−fq(ζhq(t))]¯bpqbpq[fq(h′q(t))−fq(ζhq(t))]}⩽ nn∑p=1¯ep(t)ep(t)+n∑p=1n∑q=1l21|bpq|2¯eq(t)eq(t). | (3.7) |
Similarly,
n∑p=1n∑q=1{ep(t)[¯fq(h′q(t−τ1))−fq(ζhq(t−τ1))]¯cpq+¯ep(t)cpq[fq(h′q(t−τ1))−fq(ζhq(t−τ1))]}⩽ n∑p=1n∑q=1{¯ep(t)ep(t)+[¯fq(h′q(t−τ1))−fq(ζhq(t−τ1))]¯cpqcpq[fq(h′q(t−τ1))−fq(ζhq(t−τ1))]}⩽ nn∑p=1¯ep(t)ep(t)+n∑p=1n∑q=1l22|cpq|2¯eq(t−τ1)eq(t−τ1), | (3.8) |
n∑p=1n∑q=1{ep(t)∫tt−τ2[¯fq(h′q(s))−fq(ζhq(s))]ds¯dpq+¯ep(t)dpq∫tt−τ2[fq(h′q(s))−fq(ζhq(s))]ds}⩽ n∑p=1n∑q=1{¯ep(t)ep(t)+∫tt−τ2[¯fq(h′q(s))−fq(ζhq(s))]ds¯dpqdpq∫tt−τ2[fq(h′q(s))−fq(ζhq(s))]ds}⩽ nn∑p=1¯ep(t)ep(t)+n∑p=1n∑q=1l23|dpq|2∫tt−τ2¯eq(s)ds∫tt−τ2eq(s)ds⩽ nn∑p=1¯ep(t)ep(t)+n∑p=1n∑q=1l23|dpq|2τ22supt−τ2⩽ω⩽t|eq(ω)|2. | (3.9) |
Combining (3.7)–(3.9) with (3.6), one can get
DqV(t)⩽n∑p=1{−2(ap−32n)+n∑q=1l21|bpq|2}|ep(t)|2+n∑p=1n∑q=1l22|cpq|2|ep(t−τ1)|2+n∑p=1n∑q=1l23|dpq|2τ22supt−τ2⩽ω⩽t|eq(ω)|2⩽−μ1V(t)+μ2V(t−τ1)+μ3supt−τ2⩽ω⩽tV(ω). | (3.10) |
It follows from fractional-order Razumikhin theorem [26] that
DqV(t)⩽−(μ1−μ2α)V(t)+μ3supt−τ2⩽ω⩽tV(ω). | (3.11) |
From Lemma 3, we have limt→+∞V(t)=0. Therefore, systems (2.6) and (3.1) can realize the globally projective synchronization.
Remark 2. If dpq=0, then system (2.6) was considered without the distributed delay in [27]. If dpq=0, τ1=τ2=0, then system (2.6) without delays was discussed in [5]. Note that the distributed delay can affect the dynamics of NNs, comparing with the models [5,6,9,23,27], the considered model including discrete and distributed delays in this paper is more general.
Remark 3. Compared with the real decomposition method [5] and plural decomposition method [6], without decomposing the FOQVNNs into several subsystems in this paper, the globally projective synchronization criterion (3.4) of FOQVNN is obtained by the algebraic inequality forms, which is easy to check in practical applications and reduces the complexity of the calculation.
Example 1. Consider the FOQVNNs with discrete and distributed delays described by:
Dqhp(t)=−aphp(t)+2∑q=1bpqfq(hq(t))+2∑q=1cpqfq(hq(t−τ1))+2∑q=1dpq∫tt−τ2fq(hq(s))ds+Ip, | (4.1) |
where a1=a2=1,I1=I2=0, l1=l2=l3=1, b11=2+2i+2j+2k,b12=−0.1−0.1i−0.1j−0.1k, b21=2−3i+2j−3k, b22=1+i+j+k, c11=−2−1.5i−2j−1.5k, c12=−0.2+0.5i−0.2j+0.5k, c21=6.5−i+6.5j−k, c22=−3.5+2.5i−3.5j+2.5k, d11=2.5−4.5i+2.5j−4.5k, d12=−0.3+0.2i−0.3j+0.2k, d21=−3−2i−3j−2k, d22=−1+i−j+k,τ1=τ2=1.
The corresponding slave system is described by:
Dqh′p(t)=−aph′p(t)+2∑q=1bpqfq(h′q(t))+2∑q=1cpqfq(h′q(t−τ1))+2∑q=1dpq∫tt−τ2fq(h′q(s))ds+Ip+Up(t). | (4.2) |
By using the MATLAB toolbox, Figures 1 and 2 shows the state trajectories in the absence of a controller with the order q=0.93.
In controller (3.2), if we choose the coefficients μ1=190, μ2=125, μ3=55,α=1, then the conditions of Theorem 1 hold. The initial values are selected as h10=1.5+i+2j−3k, h20=−1.4−i−2j+3k, h′10=−4−3.5i−2.5j+k, h′20=4+3.5i+2.5j−k. The projective parameters is chosen as ζ=0.5. Thus, Figures 3 and 5 shows the state trajectories with the different orders q=0.83 and q=0.93 respectively. The synchronization error norms are depicted with the different orders q=0.83 and q=0.93 in Figures 4 and 6. The numerical simulation shows that the figures are consistent with the theoretical results.
Remark 4. In order to further characterize the influence of the system order q on the performance of projective synchronization, we have chosen the different orders q = 0.83 and q = 0.93 in Example 1 to present the state trajectories and error norms by Figures 3–6. It can be seen that the speed of projective synchronization becomes faster and faster along with the increase of the order q .
The globally projective synchronization for a class of FOQVNNs with discrete and distributed delays is investigated through the direct quaternion approach rather than decomposing the QVNNs into several subsystems. By constructing an appropriate controller, the synchronization conditions were obtained by using fractional differential inequality techniques and fraction-order Razumikhin theorem. Finally, an example are performed to show the effectiveness of the method. The proposed results are easy to check in practical applications and reduces the complexity of the calculation.
This work was jointly supported the Natural Science Foundation of Anhui Province of China (No. 1908085MA01), the Natural Science Foundation of the Higher Education Institutions of Anhui Province (No. KJ2019A0557) and the Excellent Young Talents Fund Program of Higher Education Institutions of Anhui Province (No. gxyq2019048).
The authors declare that they have no competing interests.
[1] | Q. Song, Q. Yu, Z. Zhao, Y. Liu, F. Alsaadi, Boundedness and global robust stability analysis of delayed complex-valued neural networks with interval parameter uncertainties, Neural Networks, 103 (2018), 55–62. |
[2] | Z. Zhao, Z. Wang, L. Zou, G. Guo, Finite-time state estimation for delayed neural networks with redundant delayed channels, IEEE T. Syst. Man Cy-s., 51 (2018), 441–451. |
[3] |
D. Ding, Z. Wang, Q. Han, Neural-network-based output-feedback control with stochastic communication protocols, Automatica, 106 (2019), 221–229. doi: 10.1016/j.automatica.2019.04.025
![]() |
[4] |
C. Zou, K. Kou, Y. Wang, Quaternion collaborative and sparse representation with application to color face recognition, IEEE T. Image Process., 25 (2016), 3287–3302. doi: 10.1109/TIP.2016.2567077
![]() |
[5] |
J. Xiao, J. Cao, J. Cheng, S. Zhong, S. Wen, Novel methods to finite-time Mittag-Leffler synchronization problem of fractional-order quaternion-valued neural networks, Inform. Sciences, 526 (2020), 221–244. doi: 10.1016/j.ins.2020.03.101
![]() |
[6] | X. Chen, Q. Song, Z. Li, Z. Zhao, Y. Liu, Stability analysis of continuous-time and discrete-time quaternion-valued neural networks with linear threshold neurons, IEEE T. Neur. Lear., 29 (2017), 2769–2781. |
[7] | W. Zhang, H. Zhao, C. Sha, Y. Wang, Finite time synchronization of delayed quaternion valued neural networks with fractional order, Neural Process. Lett., 2021 (2021), 1–12. |
[8] |
Q. Song, Synchronization analysis of coupled connected neural networks with mixed time delays, Neurocomputing, 72 (2009), 3907–3914. doi: 10.1016/j.neucom.2009.04.009
![]() |
[9] | X. Yang, Can neural networks with arbitrary delays be finite-timely synchronized? Neurocomputing, 143 (2014), 275–281. |
[10] |
X. Yang, Q. Song, J. Liang, B. He, Finite-time synchronization of coupled discontinuous neural networks with mixed delays and nonidentical perturbations, J. Franklin I., 352 (2015), 4382–4406. doi: 10.1016/j.jfranklin.2015.07.001
![]() |
[11] | Y. Song, S. Wen, Synchronization control of stochastic memristor-based neural networks with mixed delays, Neurocomputing, 156 (2015), 121–128. |
[12] | B. Boaretto, R. Budzinski, T. Prado, J. Kurths, S. Lopes, Neuron dynamics variability and anomalous phase synchronization of neural networks, Chaos, 28 (2018), 106304. |
[13] |
Z. Ding, Y. Shen, Projective synchronization of nonidentical fractional-order neural networks based on sliding mode controller, Neural Networks, 76 (2016), 97–105. doi: 10.1016/j.neunet.2016.01.006
![]() |
[14] |
L. Ke, W. Li, Exponential synchronization in inertial neural networks with time delays, Electronics, 8 (2019), 356. doi: 10.3390/electronics8030356
![]() |
[15] |
R. Ye, X. Liu, H. Zhang, J. Cao, Global Mittag-Leffler synchronization for fractional-order BAM neural networks with impulses and multiple variable delays via delayed-feedback control strategy, Neural Process. Lett., 49 (2019), 1–18. doi: 10.1007/s11063-018-9801-0
![]() |
[16] |
A. Pratap, R. Raja, J. Alzabut, J. Cao, G. Rajchakit, C. Huang, Mittag-Leffler stability and adaptive impulsive synchronization of fractional order neural networks in quaternion field, Math. Method. Appl. Sci., 43 (2020), 6223–6253. doi: 10.1002/mma.6367
![]() |
[17] |
X. Yang, C. Li, Q. Song, J. Chen, J. Huang, Global Mittag-Leffler stability and synchronization analysis of fractional-order quaternion-valued neural networks with linear threshold neurons, Neural Networks, 105 (2018), 88–103. doi: 10.1016/j.neunet.2018.04.015
![]() |
[18] |
J. Xiao, S. Zhong, Synchronization and stability of delayed fractional-order memristive quaternion-valued neural networks with parameter uncertainties, Neurocomputing, 363 (2019), 321–338. doi: 10.1016/j.neucom.2019.06.044
![]() |
[19] |
H. Li, H. Jiang, J. Cao, Global synchronization of fractional-order quaternion-valued neural networks with leakage and discrete delays, Neurocomputing, 385 (2020), 211–219. doi: 10.1016/j.neucom.2019.12.018
![]() |
[20] |
J. Xiao, S. Wen, X. Yang, S. Zhong, New approach to global Mittag-Leffler synchronization problem of fractional-order quaternion-valued BAM neural networks based on a new inequality, Neural Networks, 122 (2020), 320–337. doi: 10.1016/j.neunet.2019.10.017
![]() |
[21] | I. Podlubny, Fractional differential equations, New York: Academic Press, 1998. |
[22] |
J. Yu, C. Hu, H. Jiang, X. Fan, Projective synchronization for fractional neural networks, Neural Networks, 49 (2014), 87–95. doi: 10.1016/j.neunet.2013.10.002
![]() |
[23] | R. Li, J. Cao, T. Huang, C. Xue, R. Manivannan, Quasi-stability and quasi-synchronization control of quaternion-valued fractional-order discrete-time memristive neural networks, Appl. Math. Comput., 395 (2021), 125851. |
[24] |
Q. Song, Y. Chen, Z. Zhao, Y. Liu, F. Alsaadi, Robust stability of fractional-order quaternion-valued neural networks with neutral delays and parameter uncertainties, Neurocomputing, 420 (2021), 70–81. doi: 10.1016/j.neucom.2020.08.059
![]() |
[25] | P. Liu, M. Kong, Z. Zeng, Projective synchronization analysis of fractional-order neural networks with mixed time delays, IEEE T. Cybernetics, 2020. |
[26] | D. Baleanu, S. Sadati, R. Ghaderi, A. Ranjbar, T. Abdeljawad (Maraaba), F. Jarad, Razumikhin stability theorem for fractional systems with delay, Abstract Appl. Anal., 9 (2010), 124812. |
[27] | W. Zhang, H. Zhang, J. Cao, H. Zhang, A. Alsaedi, Global projective synchronization in fractional-order quaternion valued neural networks, Asian J. Control, 2020. |
1. | Renyu Ye, Chen Wang, Axiu Shu, Hai Zhang, Quasi-Synchronization and Quasi-Uniform Synchronization of Caputo Fractional Variable-Parameter Neural Networks with Probabilistic Time-Varying Delays, 2022, 14, 2073-8994, 1035, 10.3390/sym14051035 | |
2. | Isra Al-Shbeil, Abdelkader Benali, Houari Bouzid, Najla Aloraini, Existence of solutions for multi-point nonlinear differential system equations of fractional orders with integral boundary conditions, 2022, 7, 2473-6988, 18142, 10.3934/math.2022998 | |
3. | Chen Wang, Hai Zhang, Ivanka Stamova, Jinde Cao, Global synchronization for BAM delayed reaction-diffusion neural networks with fractional partial differential operator, 2023, 360, 00160032, 635, 10.1016/j.jfranklin.2022.08.038 | |
4. | Yuhong Cheng, Hai Zhang, Weiwei Zhang, Hongmei Zhang, Novel algebraic criteria on global Mittag–Leffler synchronization for FOINNs with the Caputo derivative and delay, 2022, 68, 1598-5865, 3527, 10.1007/s12190-021-01672-0 | |
5. | Ravi Agarwal, Snezhana Hristova, Donal O'Regan, Integral presentations of the solution of a boundary value problem for impulsive fractional integro-differential equations with Riemann-Liouville derivatives, 2022, 7, 2473-6988, 2973, 10.3934/math.2022164 | |
6. | Muhammad Bilal Khan, Omar Mutab Alsalami, Savin Treanțǎ, Tareq Saeed, Kamsing Nonlaopon, New class of convex interval-valued functions and Riemann Liouville fractional integral inequalities, 2022, 7, 2473-6988, 15497, 10.3934/math.2022849 | |
7. | Tao Yan, Ghulam Farid, Sidra Bibi, Kamsing Nonlaopon, On Caputo fractional derivative inequalities by using strongly $ (\alpha, h-m) $-convexity, 2022, 7, 2473-6988, 10165, 10.3934/math.2022565 | |
8. | Muhammad Imran Asjad, Waqas Ali Faridi, Mohammed M. Al-Shomrani, Abdullahi Yusuf, The generalization of Hermite-Hadamard type Inequality with exp-convexity involving non-singular fractional operator, 2022, 7, 2473-6988, 7040, 10.3934/math.2022392 | |
9. | Saima Rashid, Sobia Sultana, Bushra Kanwal, Fahd Jarad, Aasma Khalid, Fuzzy fractional estimates of Swift-Hohenberg model obtained using the Atangana-Baleanu fractional derivative operator, 2022, 7, 2473-6988, 16067, 10.3934/math.2022880 | |
10. | Jin Gao, Lihua Dai, Asymptotic synchronization analysis of fractional-order octonion-valued neural networks with impulsive effects, 2023, 8, 2473-6988, 1975, 10.3934/math.2023102 | |
11. | Md Samshad Hussain Ansari, Muslim Malik, Projective synchronization of fractional order quaternion valued uncertain competitive neural networks, 2024, 88, 05779073, 740, 10.1016/j.cjph.2024.02.032 | |
12. | Md Samshad Hussain Ansari, Muslim Malik, Juan J. Nieto, Projective synchronization of fractional-order quaternion-valued neural networks with Mittag-Leffler kernel, 2025, 0308-1079, 1, 10.1080/03081079.2025.2457550 |