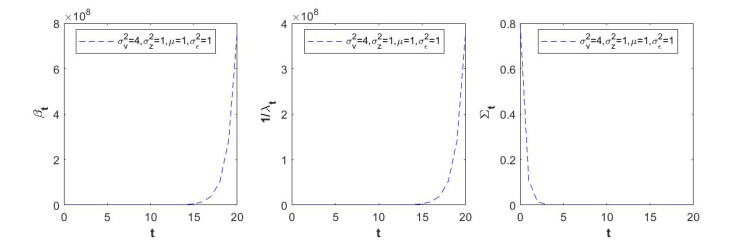
In this paper, the insider trading model of Xiao and Zhou (Acta Mathematicae Applicatae, 2021) is further studied, in which market makers receive partial information about a static risky asset and an insider stops trading at a random time. With the help of dynamic programming principle, we obtain a unique linear Bayesian equilibrium consisting of insider's trading intensity and market liquidity parameter, instead of none Bayesian equilibrium as before. It shows that (i) as time goes by, both trading intensity and market depth increase exponentially, while residual information decreases exponentially; (ii) with average trading time increasing, trading intensity decrease, but both residual information and insider's expected profit increase, while market depth is a unimodal function with a unique minimum with respect to average trading time; (iii) the less information observed by market makers, the weaker trading intensity and market depth are, but the more both expect profit and residual information are, which is in accord with our economic intuition.
Citation: Kai Xiao, Yonghui Zhou. Linear Bayesian equilibrium in insider trading with a random time under partial observations[J]. AIMS Mathematics, 2021, 6(12): 13347-13357. doi: 10.3934/math.2021772
[1] | Jixiu Qiu, Yonghui Zhou . Insider trading with dynamic asset under market makers' partial observations. AIMS Mathematics, 2023, 8(10): 25017-25036. doi: 10.3934/math.20231277 |
[2] | Kai Xiao . Risk-seeking insider trading with partial observation in continuous time. AIMS Mathematics, 2023, 8(11): 28143-28152. doi: 10.3934/math.20231440 |
[3] | Baria A. Helmy, Amal S. Hassan, Ahmed K. El-Kholy, Rashad A. R. Bantan, Mohammed Elgarhy . Analysis of information measures using generalized type-Ⅰ hybrid censored data. AIMS Mathematics, 2023, 8(9): 20283-20304. doi: 10.3934/math.20231034 |
[4] | Bing Long, Zaifu Jiang . Estimation and prediction for two-parameter Pareto distribution based on progressively double Type-II hybrid censored data. AIMS Mathematics, 2023, 8(7): 15332-15351. doi: 10.3934/math.2023784 |
[5] | Abdulhakim A. Al-Babtain, Amal S. Hassan, Ahmed N. Zaky, Ibrahim Elbatal, Mohammed Elgarhy . Dynamic cumulative residual Rényi entropy for Lomax distribution: Bayesian and non-Bayesian methods. AIMS Mathematics, 2021, 6(4): 3889-3914. doi: 10.3934/math.2021231 |
[6] | Tomás Caraballo, Javier López-de-la-Cruz . Bounded random fluctuations on the input flow in chemostat models with wall growth and non-monotonic kinetics. AIMS Mathematics, 2021, 6(4): 4025-4052. doi: 10.3934/math.2021239 |
[7] | Mohamed S. Eliwa, Essam A. Ahmed . Reliability analysis of constant partially accelerated life tests under progressive first failure type-II censored data from Lomax model: EM and MCMC algorithms. AIMS Mathematics, 2023, 8(1): 29-60. doi: 10.3934/math.2023002 |
[8] | Bin Zhou, Xiujuan Ma, Fuxiang Ma, Shujie Gao . Robustness analysis of random hyper-networks based on the internal structure of hyper-edges. AIMS Mathematics, 2023, 8(2): 4814-4829. doi: 10.3934/math.2023239 |
[9] | Xue Hu, Haiping Ren . Statistical inference of the stress-strength reliability for inverse Weibull distribution under an adaptive progressive type-Ⅱ censored sample. AIMS Mathematics, 2023, 8(12): 28465-28487. doi: 10.3934/math.20231457 |
[10] | Chunwei Wang, Shaohua Li, Jiaen Xu, Shujing Wang . Dividend problem of an investment risk model under random observation. AIMS Mathematics, 2024, 9(9): 24039-24057. doi: 10.3934/math.20241169 |
In this paper, the insider trading model of Xiao and Zhou (Acta Mathematicae Applicatae, 2021) is further studied, in which market makers receive partial information about a static risky asset and an insider stops trading at a random time. With the help of dynamic programming principle, we obtain a unique linear Bayesian equilibrium consisting of insider's trading intensity and market liquidity parameter, instead of none Bayesian equilibrium as before. It shows that (i) as time goes by, both trading intensity and market depth increase exponentially, while residual information decreases exponentially; (ii) with average trading time increasing, trading intensity decrease, but both residual information and insider's expected profit increase, while market depth is a unimodal function with a unique minimum with respect to average trading time; (iii) the less information observed by market makers, the weaker trading intensity and market depth are, but the more both expect profit and residual information are, which is in accord with our economic intuition.
In Kyle's [11] seminal contribution, a continuous auction model of insider trading on a risky asset is proposed, in which an insider is assumed to possess complete information about the asset value with normal distribution, noise traders without any information on the asset randomly submit orders and market makers excavate the insider's private information based on the total market order flow to make the market efficient. It shows that there is a unique linear Bayesian equilibrium consisting of insider's trading intensity and market liquidity parameter such that the intensity is increasing to infinity and the market liquidity keeps constant as time goes to the final trading time.
Later, Kyle's model [11] is extended from different perspectives. For a class of continuous-time insider trading on a risky asset with general distributions, Back [2] obtained a closed form of Bayesian equilibrium. In Cho's [8] view, he believed that there is risk behavior for the insider, and found that in the Bayesian equilibrium, the price pressure with risk aversion converges to the risk-neutral price pressure as the insider becomes less and less risk-averse. Furthermore, Caldentey and Stacchetti [5] considered a setting of insider trading when an insider may trade at a random deadline and also established its Bayesian equilibrium. Note that all of the above results are deduced by the principle of dynamic programming. Recently, Aase, Bjuland and Øksendal[1] applied the maximum principle to solve continuous-time insider trading problem to establish a closed form of none Bayesian equilibrium. And Zhou [18] also obtained a unique none Bayesian equilibrium when market makers observe some information about the risky asset. There is much literature on this topic, see [3,4,6,7,9,10,14,15] and so on.
In fact, there may be multifactorial to influence a financial market. Based on [5,18], Xiao and Zhou [16] studied an insider trading model, in which partial information on a risky asset with value v as
v+ϵ+∫t0σusdBus | (1.1) |
is observed by market makers and the trading will be ended at a random time τ. And they obtained a closed form of none Bayesian equilibrium of the model by the maximum principle method. However, the model [16] can be simplified: for the market makers, only the initial information
v+ϵ | (1.2) |
in the formula (1.1) is useful to make market efficient, since the standard Brownian motion But is independent of v and ϵ for t>0. That is to say, only the information v+ϵ at the beginning of trading is useful while But is not for market makers to set the asset's price, therefore, we think that But is redundant and can be omitted. In this paper, applying dynamic programming principle, we further establish Bayesian equilibrium of this simplified model, and clearly deduce the none Bayesian equilibrium in [16]. To illustrate some characteristics of the Bayesian equilibrium, simulations are given.
The rest of the paper is organized as follows. In Section 2, our model of insider trading with the definition of linear Bayesian equilibrium will be introduced. In Section 3, a necessary condition of market efficiency is given. Section 4 includes the important HJB equation. In Section 5, the existence and uniqueness of linear equilibrium is established. In Section 6, we give some simulations to describe properties about the equilibrium. Conclusions are drawn in the last section. The model with linear Baysian equilibrium
The model of insider trading here is a new version of the model in [16]. Basically, There is a risky asset whose value v is a normal distribution with mean 0 and variance σ2v trading until a random time τ when the value v becomes public knowledge, which has a geometric distribution with probability of failure e−μt for some μ>0 and is independent of the history of transactions and prices [5]. And there are three types of traders: (1) An insider, who knows the risky asset value v with its current market price and submits her/his order xt at time t; (2) Noise traders, who have no information about the underlying asset and submit total order zt=σzBzt [8], where the number σz>0 and Bzt is a standard Brownian motion; (3) Market makers, who observe not only the total traded volume yt=xt+zt (can not discriminate xt and zt respectively) but also another signal of the asset value as (1.2): v+ϵ, where ϵ is normally distributed with mean 0 and variance σ2ϵ and is independent of v and Bzt, and then set the market price of the underlying asset in a semi-strong way as in [11]:
pt=E[v|FMt] |
where FMt=σ{ys,0≤s≤t,}∨σ{v+ϵ}.
As in [1,2,11,18], the dynamic of the insider's strategy xt and the dynamic of the market pricing pt are assumed local linear respectively as follows:
dxt=βt(v−pt)dt, dpt=λtdyt | (2.1) |
where x0=0 and p0=E[v|v+ϵ]=σ2vσ2v+σ2ϵ(v+ϵ).
Roughly speak, the insider's expected profit [5] is written as
E∫τ0(v−ps)dxs=E∫+∞0e−μsβs(v−ps)2ds | (2.2) |
where both βt>0 and λt>0 are two deterministic, smooth function on [0,∞); technically, E[∫∞0e−μtβt(v−pt)2dt]<∞ should be satisfied. Note that βt and λt (or 1λt) are called trading intensity of the insider and market liquidity parameter (or market depth) respectively, and Σt=E[v−pt]2 is called residual information [11].
The collection of all these functions β and the collection of all these functions λ are denoted by Θ and Λ respectively. Clearly, given a pair (β,λ)∈(Θ,Λ), there is a unique insider trading market; and sometimes we also call it market (β,λ).
Definition 2.1 A linear Bayesian equilibrium is a pair (β,λ)∈(Θ,Λ) such that
(i) (maximization of profit) given λ, β maximizes
E[∫∞0exp−μsβs(v−ps)2ds|FIt] | (2.3) |
where FIt=σ{ps,0≤s≤t}∨σ{v};
(ii) (market efficiency) given β, λ satisfies
p0+∫t0λsdys=E[v|FMt]. | (2.4) |
Before establishing the existence of linear equilibria in our insider trading model, we first give some necessary conditions for market efficiency.
Lemma 1. Let (β,λ)∈(Θ,Λ) be a market with λ satisfying the market efficiency condition (2.4). Then
λt=Σtβtσ2z, dΣtdt=−β2tΣ2tσ2z |
where Σ0=σ2ϵσ2vσ2v+σ2ϵ.
Proof. At first, the total traded volume
yt=xt+zt | (3.1) |
and (1.2) observed by market makers can be written as a vector dynamic equation
dξt=(A0+A1v)dt+A2dBt |
where
ξt=(ytut), ξ0=(0v+ϵ), A0=(−ptβt0), A1=(βt0), A2=(σz000), Bt=(Bzt0).
Therefore at time t, the market makers' information
FMt=Fξt=σ{ξs,0≤s≤t}. |
Note that the matrix A2 is irreversible, we need to construct a new dynamic equation which must be equivalent to ξt for market makers setting price such that a new version of A2 is reversible.
For convenience, we consider the following signal-observation dynamic system
{dv=0dηt=(A0+A1v)dt+A∗2dB∗t | (3.2) |
where ηt=(ytut), η0=(0v+ϵ), A∗2=(σz00σ), B∗t=(BztWt), ut=v+ϵ+σWt, and σ is a positive real number and W is a standard Brownian motion independent of v, ϵ and Bz. Clearly, for any time t,
FMt=Fξt⊂Fηt=σ{ηs,0≤s≤t}. |
Denote p∗t=E[v|Fηt] and Σ∗t=E[(v−p∗t)2]. Then by Theorems 12.7 and 12.9 of optimal filtering equations in [12,13] or Lemma 3.3 in [18], we have
p∗t=p∗0+∫t0Σ∗tβtσ2zdyt, dΣ∗tdt=−β2tΣ∗2tσ2z | (3.3) |
with
p∗0=σ2vσ2v+σ2ϵ(v+ϵ), Σ∗0=σ2ϵσ2vσ2v+σ2ϵ. |
Then, by the expression of p∗t above, we have that p∗t is Fξt measurable. And by tower law of conditional expectation,
E[v|FMt]=E[v|Fξt]=E[E[v|Fηt]|Fξt]=E[p∗t|Fξt]=p∗t. |
Note that λ satisfies the market efficiency condition (2.4):
pt=p0+∫t0λsdys=E[v|FMt], |
then we have
pt=p∗t, Σt=Σ∗t. |
Therefore, by the equations in (3.3), we have
λt=Σtβtσ2z, dΣtdt=−β2tΣ2tσ2z |
with Σ0=σ2ϵσ2vσ2v+σ2ϵ, and the proof is complete.
Remark 1. In our model, for the risky value v, the observation of market makers is v+ϵ at any time t≥0. However, to obtain our desired result, we take ut=v+ϵ+σWt as the observation of market makers at time t>0 where σ>0, since the standard Brownian motion W is independent of v, Bz and ϵ and then only the initial information v+ϵ is useful for market makers to make market efficient. In fact, the information v+ϵ in the signal ut for any t>0 has been integrated into Σ0, which is verified by the expression of λt, βt and Σt independent of σ and Wut in Lemma 1.
Given a market liquidity parameter λ∈Λ, the object of the insider is to take her/his information advantage and find an optimal strategy β∈Θ to maximize the profit [5]:
E[∫∞0exp−μsβs(v−ps)2ds|FIt] |
under the following stochastic control system, the second dynamic in (2.1):
dpt=λtβt(v−pt)dt+λtσzdBzt, p0=E[v|v+ϵ]. | (4.1) |
This is a classical stochastic control problem, which can be solved by the dynamic programming principle [17].
Since the random continuing time τ obeys a life distribution which has no memory, the insider's value function at time t can be written as
π(t,pt)=maxβ′∈ΘE[∫∞texp−u(s−t)β′s(v−ps)2ds|FIt]. | (4.2) |
Lemma 2. The value function π(t,pt) satisfies the HJB equation below:
−μπ(t,pt)+∂π(t,pt)∂t+12λ2tσ2z∂2π(t,pt)∂p2+maxβ(λtβ(v−pt)∂π(t,pt)∂p+β(v−pt)2)=0. | (4.3) |
Proof. According to the equality (4.2), we can denote
˜π(t,pt)=exp−μtπ(t,pt)=maxβ′∈ΘE[∫∞texp−μsβ′s(v−ps)2ds|FIt]. | (4.4) |
Note that pt evolves the dynamic (4.1). Then by Proposition 3.5 in [17], the value function ˜π(t,pt) satisfies
∂˜π(t,pt)∂t+maxβ∈R{12λ2tσ2z∂2˜π(t,pt)∂p2+λtβ(v−pt)∂˜π(t,pt)∂p+exp−μtβ(v−pt)2}=0, |
which can be easily simplified as the HJB (4.3), and the proof is complete.
Now the existence and uniqueness of linear equilibrium in our model can be given below.
Theorem 1. There is a unique linear equilibrium (β,λ)∈(Θ,Λ) satisfying
βt=√2μσ2zΣ0expμt, λt=√2μΣ0σ2zexp−μt; |
and at the equilibrium, the remained information
Σt=Σ0exp−2μt |
where Σ0=σ2ϵσ2vσ2v+σ2ϵ; the insider's profit from t onwards
π(t,pt)=(v−pt)2−v2+σ2v2λt+σ2zλt4μ |
with the whole expect profit
E(π)=E(π(0,p0))=√σ2zΣ02μ. |
Proof. Let (β,λ)∈(Θ,Λ) be a linear equilibrium in the insider trading market. Then by Theorem 3.4 in [17], the value function at any time t is equal to the optimal profit from t onward, that is,
π(t,pt)=E[∫∞texp−μ(s−t)βs(v−ps)2ds|FIt]. |
Note that the value function π(t,pt) satisfies the HJB (4.3), which is equivalent to the following system
{−μπ(t,pt)+∂π(t,pt)∂t+12λ2tσ2z∂2π(t,pt)∂p2=0λt(v−pt)∂π(t,pt)∂p+(v−pt)2)=0 | (5.1) |
with boundary condition: limt→∞π(t,pt)=0.
By Lemma 1, we have dΣtdt=−β2tΣ2tσ2z<0. Since Σt=E(v−pt)2, then
v≠pt a.s. |
Then by the second equation of (5.1), we obtain that
π(t,pt)=p2t2λt−vptλt+gt | (5.2) |
where gt is some smooth, deterministic function respect to t.
Then taking (5.2) into the first equation of (5.1), we obtain the equation
(ddt(1λt)−μλt)(p2t2−vpt)+dgdt−μgt+12λtσ2z=0. |
Since pt can be taken any real number, then
{ddt(1λt)−μλt=0;dgdt−μgt+12λtσ2z=0, | (5.3) |
which can be easily solved as follows:
λt=λ0exp−ut, gt=g0expμt+λ0σ2z4μ(exp−μt−expμt) | (5.4) |
where both λ0 and g0 are some positive real numbers.
In the following, let us determine the values of λ0 and g0. By Lemma 1, we also have
Σt=Σ0−∫t0σ2zλ2tdt. | (5.5) |
Then bring the value of λt in (5.4) into (5.5),
Σt=Σ0+σ2zλ202μ(exp−2μt−1). |
By the lemma 1, we can assume that limt→∞Σt=a, where a is a real number, and so
λ0=√2μ(Σ0−a)σ2z. | (5.6) |
So we have
Σt=Σ0exp−2μt+a2μ(1−exp−2μt), λt=√2μ(Σ0−a)σ2zexp−μt. |
And according to (5.2), (5.4), (5.6) and the above two equations, we have
π(t,pt)=((v−pt)2−v22λ0+g0−λ0σ2z4μ)expμt+λ0σ2z4μexp−μt. |
So by the boundary condition limt→∞π(t,pt)=0, by Fatou Lemma, there must be
limt→∞(Σt−σ2v2λ0+g0−λ0σ2z4μ)=0. |
So we have
g0=σ2v−a2λ0+λ0σ2z4μ, |
and so
π(t,pt)=((v−pt)2−v2+σ2v−a2λ0−λ0σ2z4μ)expμt+λ0σ2z4μexp−μt |
or
π(t,pt)=(v−pt)2−v2+σ2v−a2λt+σ2zλt4μ. |
Finally, by Lemma 1,
βt=√2μσ2z(Σ0−a)exp−μtΣ0exp−2μt+a/2μ(1−exp(−2μt)) |
where Σ0=σ2ϵσ2vσ2v+σ2ϵ.
Since the random time τ is life distributed with parameter μ, E(τ)=1μ. And the optimal profit from time t onward of the insider is
π(t,pt)=(v−pt)2−v2+σ2v−a2λt+σ2zλt4μ. |
Then
E(π)=E(π(0,p0))=Σ0−a2λ0+σ2zλ04μ. |
Since the insider's expected profit is maximal, there must be a=0. That is to say
limt→∞Σt=0. |
Then
E(π)=√σ2zΣ02μ. |
and other conclusions hold.
Remark 2. Clearly, the linear Bayesian equilibrium in Theorem 5.1 here obtained by dynamic programming principle is the same as the linear equilibrium in Theorem 4.1 obtained by maximal principle in [16]. However, the former equilibrium can tell us the insider's profit from any trading time t onwards under her/his current information, but the latter can not do that; that is our motivation of this paper. Of course, the former equilibrium has the same properties of the latter in Corollary 4.1 in [16]. For readers' convenience, we restate them bellow (and give simulations in Section 6 as supplement):
(i) As time goes by, both trading intensity βt and market depth 1λt increase exponentially, while residual information Σt decreases exponentially even approaching zero as trading time going to ∞;
(ii)
∂βt∂E(τ)<0, ∂(1λt)∂E(τ)>0 if and only if E(τ)>2t, ∂Σt∂E(τ)>0, ∂E(π)∂E(τ)>0; |
(iii)
∂βt∂σ2ϵ<0, ∂(1λt)∂σ2ϵ<0, ∂E(π)∂σ2ϵ>0,∂Σt∂σ2ϵ>0. |
To see more clearly those properties of linear Bayesian equilibrium about Theorem 1 and Remark 2, some simulations are illustrated, where we always assume that σ2z=1 and Σ2v=4.
According to those expressions in Theorem 1, as trading time t increasing, both trading intensity β and market depth 1λ increase exponentially, but residual information Σ decreases exponentially, see Figure 1.
Figure 2 tells us that (1) with the average trading time E(τ) increasing, trading intensity β decrease but residual information Σ increase, while market depth 1λ is a unimodal function of average trading time with a unique minimum value; and (2) if the average trading time E(τ) is longer, the insider will make more expect profit E(π).
Figure 3 shows that if partial information on the risky value observed by market makers is less and less (σ2ϵ→∞), both trading intensity β and market depth 1/λ is decreasing while both residual information Σ and the whole expect profit E(π) earned by the insider is more and more.
In this paper, the insider trading model in [16] is revisited, in which market makers observe partial information about a risky asset and an insider stops trading at a random time. By dynamic programming principle, we obtain a unique linear Bayesian equilibrium consisting of insider's trading intensity and market liquidity parameter which is the same as that deduced by maximal principle in [16], including the insider's profit from any trading time onwards.
It shows that trading time, average trading time and partial information on the risky asset value observed by market makers have some impacts on the linear Bayesian equilibrium: (1) As trading time goes by, both trading intensity and market depth increase, while residual information decreases even closing to zero; (2) With average trading time increasing, trading intensity decrease but residual information increase, while market depth is a unimodal function with respect to average trading time with a unique minimum, and if average trading time is longer, the insider will make more expect profit; (3) As partial information on the risky value observed by market makers is less and less, both trading intensity and market depth is decreasing while both residual information and the whole expect profit earned by the insider is more and more. All of these results are in accord with our economic intuition.
The work is supported by NSF China (11861025), Guizhou QKHPTRC[2018]5769-07, Guizhou EDKY[2016]027, Guizhou QKZYD[2016]4006 and Guizhou ZDXK[2016]8.
The authors declare no conflict of interest in this paper.
[1] |
K. Aase, T. Bjuland, B. Øksendal, Strategic insider trading equilibrium: A filter theory approach, Afr. Mat., 23 (2012), 145–162. doi: 10.1007/s13370-011-0026-x
![]() |
[2] |
K. Back, Insider trading in continuous time, Rev. Financ. Stud., 5 (1992), 387–409. doi: 10.1093/rfs/5.3.387
![]() |
[3] |
K. Back, H. Pedersen, Long-lived information and intraday patterns, J. Financ. Mark., 1 (1998), 385–402. doi: 10.1016/S1386-4181(97)00003-7
![]() |
[4] |
F. Biagini, Y. Hu, T. Myer-Brandis, B. Øksendal, Insider trading equilibrium in a market with memory, Math. Finan. Econ., 6 (2012), 229–247. doi: 10.1007/s11579-012-0065-6
![]() |
[5] | R. Caldentey, E. Stacchetti, Insider trading with a random deadline, Econometrica, 1 (2010), 245–283. |
[6] |
L. Campi, U. Cetin, A. Danilova, Dynamic Markov bridges motivated by models of insider trading, Stoch. Proc. Appl., 121 (2011), 534–567. doi: 10.1016/j.spa.2010.11.004
![]() |
[7] |
L. Campi, U. Cetin, Insider trading in an equilibrium model with default: A passage from reduced-form to structral modelling, Financ. Stoch., 11 (2007), 591–602. doi: 10.1007/s00780-007-0038-4
![]() |
[8] |
K. Cho, Continuous auctions and insider trading, Financ. Stoch., 7 (2003), 47–71. doi: 10.1007/s007800200078
![]() |
[9] | P. Collins-Dufresne, V. Fos, Insider trading, stochastic liquidity and equilibrium prices, Econometrica, 84 (2016), 1451–1475. |
[10] |
F. Fostor, S. Viswanathan, Strategic trading when agents forecast the forcasts of others, J. Finance, 51 (1996), 1437–1478. doi: 10.1111/j.1540-6261.1996.tb04075.x
![]() |
[11] |
A. Kyle, Continuous auctions and insider trading, Econometrica, 53 (1985), 1315–1335. doi: 10.2307/1913210
![]() |
[12] | R. Liptser, A. Shiryaev, Statistics of Random Processes: General Theory, Springer, 2001. |
[13] | R. Liptser, A. Shiryaev, Statistics of Random Processes II. Applications, Springer, 2001. |
[14] |
S. Luo, The impact of public information on insider trading, Econ. Lett., 70 (2001), 59–68. doi: 10.1016/S0165-1765(00)00347-5
![]() |
[15] |
J. Ma, R. Sun, Y. Zhou, Kyle-Back equilibrium models and linear conditional mean-field SDEs, SIAM J. Control Optim., 56 (2018), 1154–1180. doi: 10.1137/15M102558X
![]() |
[16] | K. Xiao, Y. Zhou, Insider trading with a random deadline under partial observations: Maximal principle method, Accepted by Acta Math. Appl. Sin-E., 2021. |
[17] | J. Yong, X. Zhou, Stochastic Controls, Springer, 2012. |
[18] |
Y. Zhou, Existence of linear strategy equilibrium in insider trading with partial observations, J. Sys. Sci. Complex., 29 (2016), 1–12. doi: 10.1007/s11424-015-4074-4
![]() |
1. | Real-Time AWS Resource Surveillance with Reporting and Dashboard Solution, 2024, 2582-8045, 202, 10.48175/IJARSCT-15936 | |
2. | Kai Xiao, Risk-seeking insider trading with partial observation in continuous time, 2023, 8, 2473-6988, 28143, 10.3934/math.20231440 |