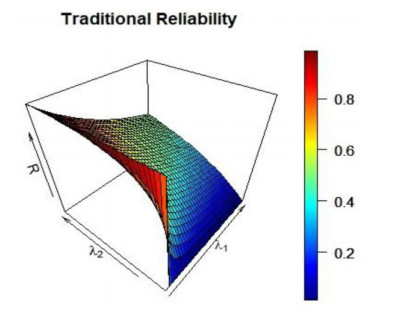
In this paper, the question of inference of the reliability parameter of fuzzy stress strength RF=P(Y<X) is attached to the difference between stress and strength values when X and Y are independently distributed from inverse Rayleigh random variables. Including fuzziness in the stress-strength interference enables researchers to make more sensitive and precise analyses about the underlying systems. The maximum product of the spacing method for the reliability of fuzzy stress intensity inference has been introduced. As classical estimation methods and Bayesian estimation methods are used to estimate the reliability parameter RF, the maximum product of spacing and maximum likelihood estimation methods is used. The maximum product of spacing under fuzzy reliability of stress strength model is introducing in this paper. Markov Chain Monte Carlo approach is used to obtain Bayesian estimators of traditional and fuzzy reliability of stress strength for inverse Rayleigh model by using the Metropolis-Hastings algorithm. Using an extensive Monte Carlo simulation analysis, the outputs of the fuzzy reliability and traditional reliability estimators are contrasted. Finally, for example, and to verify the efficiency of the proposed estimators, a genuine data application is used.
Citation: Mohamed A. H. Sabry, Ehab M. Almetwally, Osama Abdulaziz Alamri, M. Yusuf, Hisham M. Almongy, Ahmed Sedky Eldeeb. Inference of fuzzy reliability model for inverse Rayleigh distribution[J]. AIMS Mathematics, 2021, 6(9): 9770-9785. doi: 10.3934/math.2021568
[1] | Xue Hu, Haiping Ren . Statistical inference of the stress-strength reliability for inverse Weibull distribution under an adaptive progressive type-Ⅱ censored sample. AIMS Mathematics, 2023, 8(12): 28465-28487. doi: 10.3934/math.20231457 |
[2] | Hanan Haj Ahmad, Ehab M. Almetwally, Dina A. Ramadan . A comparative inference on reliability estimation for a multi-component stress-strength model under power Lomax distribution with applications. AIMS Mathematics, 2022, 7(10): 18050-18079. doi: 10.3934/math.2022994 |
[3] | Abdulaziz S. Alghamdi, Muhammad Ahsan-ul-Haq, Ayesha Babar, Hassan M. Aljohani, Ahmed Z. Afify . The discrete power-Ailamujia distribution: properties, inference, and applications. AIMS Mathematics, 2022, 7(5): 8344-8360. doi: 10.3934/math.2022465 |
[4] | Neama Salah Youssef Temraz . Analysis of stress-strength reliability with m-step strength levels under type I censoring and Gompertz distribution. AIMS Mathematics, 2024, 9(11): 30728-30744. doi: 10.3934/math.20241484 |
[5] | Haiping Ren, Xue Hu . Estimation for inverse Weibull distribution under progressive type-Ⅱ censoring scheme. AIMS Mathematics, 2023, 8(10): 22808-22829. doi: 10.3934/math.20231162 |
[6] | Hatim Solayman Migdadi, Nesreen M. Al-Olaimat, Maryam Mohiuddin, Omar Meqdadi . Statistical inference for the Power Rayleigh distribution based on adaptive progressive Type-II censored data. AIMS Mathematics, 2023, 8(10): 22553-22576. doi: 10.3934/math.20231149 |
[7] | Refah Alotaibi, Mazen Nassar, Zareen A. Khan, Ahmed Elshahhat . Statistical analysis of stress–strength in a newly inverted Chen model from adaptive progressive type-Ⅱ censoring and modelling on light-emitting diodes and pump motors. AIMS Mathematics, 2024, 9(12): 34311-34355. doi: 10.3934/math.20241635 |
[8] | Alanazi Talal Abdulrahman, Khudhayr A. Rashedi, Tariq S. Alshammari, Eslam Hussam, Amirah Saeed Alharthi, Ramlah H Albayyat . A new extension of the Rayleigh distribution: Methodology, classical, and Bayes estimation, with application to industrial data. AIMS Mathematics, 2025, 10(2): 3710-3733. doi: 10.3934/math.2025172 |
[9] | Mashail M. Al Sobhi . The modified Kies-Fréchet distribution: Properties, inference and application. AIMS Mathematics, 2021, 6(5): 4691-4714. doi: 10.3934/math.2021276 |
[10] | Refah Alotaibi, Mazen Nassar, Zareen A. Khan, Ahmed Elshahhat . Analysis of reliability index $ \mathfrak{R} = P(Y < X) $ for newly extended xgamma progressively first-failure censored samples with applications. AIMS Mathematics, 2024, 9(11): 32200-32231. doi: 10.3934/math.20241546 |
In this paper, the question of inference of the reliability parameter of fuzzy stress strength RF=P(Y<X) is attached to the difference between stress and strength values when X and Y are independently distributed from inverse Rayleigh random variables. Including fuzziness in the stress-strength interference enables researchers to make more sensitive and precise analyses about the underlying systems. The maximum product of the spacing method for the reliability of fuzzy stress intensity inference has been introduced. As classical estimation methods and Bayesian estimation methods are used to estimate the reliability parameter RF, the maximum product of spacing and maximum likelihood estimation methods is used. The maximum product of spacing under fuzzy reliability of stress strength model is introducing in this paper. Markov Chain Monte Carlo approach is used to obtain Bayesian estimators of traditional and fuzzy reliability of stress strength for inverse Rayleigh model by using the Metropolis-Hastings algorithm. Using an extensive Monte Carlo simulation analysis, the outputs of the fuzzy reliability and traditional reliability estimators are contrasted. Finally, for example, and to verify the efficiency of the proposed estimators, a genuine data application is used.
The stress-strength reliability model is significant in reliability literature, medical, economic, and engineering applications where systems or specialized units may be exposed to randomly occurring environmental stresses such as pressure, temperature, and humidity. In this case, the survival of the system depends on its resistance. During World War Ⅱ, it was found that some of the equipment such as radar and communication systems failed to work efficiently when they were used in an environment different from the environment for which they were designed. For that, experts started to consider the effects of environmental conditions while evaluating the reliability of equipment.
The computations of fuzzy reliability have been addressed by [1], where if X and Y are independent but not identical random variables in distribution. The idea behind fuzzy reliability is that including fuzziness in the stress-strength interference enables researchers to make more sensitive and precise analyses about the underlying systems of life reliability and the system becomes more stable and reliable when the difference (x−y) gets larger. The advantages of the fuzzy stress-strength reliability model over the traditional stress-strength reliability model are in considering the randomness in reliability engineering and the fuzziness of operating time. For more information see [1,2,3,4,5].
The fuzzy reliability RF=P(Y<X) is defined as
RF=∬y<xμA(y)(x)dFY(y)dFX(x) | (1) |
where A(y)={x:y<x} is a fuzzy set and μA(y)(x) is an appropriate membership function on A(y); that is assumed increasing on the difference (x−y) (readers are encouraged to read [5,6] who used the definition of the fuzzy stress-strength model to estimate RF=(Y<X), when X and Y were independent inverse exponential random variables).
The probability that the system is strong enough to overcome the stress imposed on it is defined as system reliability. Traditional reliability R=P(Y<X) may be equally explained as the region under the receiver operating characteristic (ROC) for diagnostic test or biomarkers, see reference [7]. The ROC curve is exceedingly used in medical, biological, economic, and health service research, to evaluate the reliability and distinguish between two groups of subjects, generally non-satisfied and satisfied subjects. The research conducted on the traditional stress-strength reliability model focuses on computing, calculating, and estimating the reliability of different stress and strength distributions. For example, reference [8] estimated the traditional reliability of the stress-strength model for a generalized exponential distribution with three parameters. Confidence intervals estimation of traditional reliability of stress-strength model for generalized Pareto distribution has been discussed by [9]. The stress-strength model of a generalized logistic distribution has been studied by [10]. Reference [11] estimated the R when X and Y independent Lindley populations. In 2020, [12] and others discussed the estimation of R when X and Y are independent exponentiated Pareto random variables when samples are selected using some ranked set sampling designs. Reference [13] presented a comprehensive review of the traditional reliability of the stress-strength model. In 2021, [14] estimated the traditional stress strength reliability by the use of the MPS estimation method.
In our study, we used failure times in insulating fluid between two electrodes subjected to a voltage of 34 kV and 36 kV as an application and for illustrative purposes. These failure times were randomly observed and there was no reliable information available. Moreover, the failure times model may be difficult to measure due to the complexity of the action of electrodes. So, we used stress-strength reliability model in the presence of fuzziness.
In this article, estimation of fuzzy stress-strength reliability model RF=P(Y<X), when X and Y are independent but not symmetrically distributed inverse Rayleigh random variables, is discussed. The product of the spacing method was presented to infer the reliability of fuzzy stress strength by using different methods. The proposed estimators are obtained using the maximum likelihood estimation method (MLE) and the maximum product of the spacing estimation method (MPS) as well as Bayesian estimation when prior distributions are assumed exponential. Besides, a Monte Carlo simulation study is made to analyze and compare the performance of the different estimators. A real data application is conducted for illustration purposes and to test the estimated functions of the reliability parameter RF. Finally, the paper is concluded.
An increase in the values of x−y can be thought of equivalently as the increase in the difference of 1y2−1x2. With such consideration, the membership function can be redefined as
μ⋆A(y)(x)=M⋆(1y2−1x2)=1−exp[−k(1y2−1x2)],x>y, | (2) |
where k>0.
Let X and Y be two independent inverse Rayleigh random variables with scale parameters λ1 and λ2, respectively. The inverted Rayleigh [IR(λ)] distribution has the following cumulative distribution function (CDF) and probability density function (PDF) for x>0:
F(x)=exp[−λx2]andf(x)=2λx3exp[−λx2] | (3) |
respectively, where λ>0 is a scale parameter. The traditional reliability of the stress-strength model for inverse Rayleigh distribution was studied and calculated to be R=λ2/(λ1+λ2) (see Kotz et al. [9]). Therefore, the fuzzy reliability of stress-strength RF=P(Y≺X) is given by
RF=P(Y<X)=∫∞0∫∞y(1−exp[−k(1y2−1x2)])(2λ1x3exp[−λ1x2])(2λ2y3exp[−λ2y2])dxdy=(1−λ1λ1+k)(λ2λ1+λ2)=(kλ1+k)R | (4) |
The traditional reliability R is always greater than the fuzzy reliability RF, and as k→∞,RF→R. Figure 1 shows different values for R when λ1 and λ2 changes simultaneously, Figure 2 shows fuzzy reliably values for different values of the constant k and when also λ1 and λ2 changes simultaneously.
In this section, the two methods (MLE and MPS) of estimation are used to estimate the fuzzy reliability parameter RF. Let (X1,X2,…,Xn) and (Y1,Y2,…,Ym) be two independent random samples from IR (λ1) and IR (λ2), respectively.
The joint likelihood function of the IR distribution for the stress-strength model can be written as
L(λ1,λ2)=n∏i=1f(xi;λ1)m∏j=1f(yj;λ2), | (5) |
and the log-likelihood function is given as
l(Ω)=lnL(λ1,λ2)=nln(2λ1)−3n∑i=1ln(xi)−λ1n∑i=1x2i+mln(2λ2)−3m∑i=1ln(yi)−λ2m∑i=1y2i. | (6) |
The normal equations for unknown parameters λ1,λ2, are obtained by differentiating (6) partially concerning the parameters λ1,λ2, and equate them to zero. The estimators ˆλ1,ˆλ2 can be obtained as a solution to the following equation:
∂l(λ1,λ2)∂λj=ℵjλj−ℵj∑i=1g2ji;j=1,2, |
where gji=(xi,yi), ℵj=(n,m). The estimate by using MLE is ˆλj=ℵj∑ℵji=1g2ji. By using the invariance property of MLE, traditional reliability R and the fuzzy reliability RF of IR distribution for the stress-strength model are obtained by using MLE's as following
ˆR=ˆλ2ˆλ1+ˆλ2,ˆRF=kˆλ1+kˆR. |
The maximum product spacing for stress-strength model is denoted as following.
GS(λ1,λ2)=(n+1∏i=1Di(xi;λ1))1n+1(m+1∏i=1Di(yj;λ2))1m+1, | (7) |
such that ∑iDi(gji;λj)=1, where
Di(gji;λj)={D1=F(gj1;λj)Di=F(gji;λj)−F(gj(i−1);λj)Dn+1=1−F(gjn;λj)); i=2…sj,j=1,2. | (8) |
The natural logarithm of the product spacing function of the exponential distribution for stress-strength model is denoted as following
gs(Ω)=lnGS(λ1,λ2)=1n+1[−λ1x21+ln(1−e−λ1x2n)+n∑i=2ln(e−λ1x2i−e−λ1x2i−1)]+1m+1[−λ2y21+ln(1−e−λ2y2m)+∑mi=2ln(e−λ2y2i−e−λ2y2i−1)] | (9) |
To obtain the normal equations for the unknown parameters, we partially differentiate Eq (8) concerning the parameters λ1,λ2, and equate them with zero. The estimators ˆλ1,ˆλ2 can be obtained as a solution of the following equations.
∂gs(λ1,λ2)∂λj=1ℵj+1[−1g2j1+1g2jne−λjg2jn1−e−λjg2jn+ℵj∑i=21g2jie−λjg2ji−1g2j(i−1)e−λjg2j(i−1)e−λjg2ji−e−λjg2j(i−1)]. |
The above nonlinear equations can't be solved analytically to find ˆλ1,ˆλ2 of λ1,λ2. So, by using optimization algorism as conjugate-gradient or Newton-Raphson optimization methods, the estimators of λ1,λ2 are obtained. Using the invariance property of MPS estimators of the MPS's which have been discussed by [13,14,15,16,17,18] and have concluded that it is the same as that of MLE, traditional reliability R and fuzzy reliability RF for IR distribution for the stress-strength model can be computed.
Assume that the parameters λ1 and λ2 are random variables with exponential prior distributions [11] with density function given by
π1(λ1)∝e−b1λ1andπ2(λ2)∝e−b2λ2, |
where b1>0 and b2>0 are hyperparameters of the prior distributions of λ1 and λ2. The posterior distributions of λ1 and λ2 are obtained by combining the likelihood function with the prior distributions of both λ1 and λ2 and are found to be
π1(λ1|x)∝λn1exp[−λ1(b1+n∑i=1x−2i)]∼gamma(n+1,(b1+n∑i=1x−2i)), |
and
π1(λ1|x)=(b1+∑ni=1x−2i)n+1Γ(n+1)λn1exp[−λ1(b1+n∑i=1x−2i)]. |
Also,
π2(λ2|y)∝λm2exp[−λ2(b2+m∑i=1y−2i)]∼gamma(m+1,(b2+m∑i=1y−2i)), |
and
π2(λ2|y)=(b2+∑mi=1y−2i)m+1Γ(m+1)λm2exp[−λ2(b2+m∑i=1y−2i)]. |
Now, let T1=b1+∑ni=1x−2i and T2=b2+∑mi=1y−2i. After some manipulations and simplifications, we have that π1(λ1∖u)∼Gamma(n+1,T1) and π2(λ2∖v)∼Gamma(m+1,T2) respectively. The joint posterior distribution of λ1 and λ2 is then given by
π(λ1,λ2|x,y)=Tn+11Tm+12Γ(n+1)Γ(m+1)λn1λm2exp[−(λ1T1+λ2T2)]. |
Let S=λ1+λ2 and since R=λ2λ1+λ2, then using standard transformation techniques, the joint posterior pdf of R and S will be
π(r,s|x,y)=Tn+11Tm+12Γ(n+1)Γ(m+1)rm(1−r)nsn+m+2exp[−s((1−r)T1+rT2)]. |
Under squared error loss function, the Bayes estimate ˜RF is the expected value of RF and is given by,
˜RF=E(RF|x,y)=∫10∫∞0RFπ(r,s|x,y)dsdr˜RF=A∫10∫∞0krs(1−r)+krm+1(1−r)nsn+m+3exp[−s((1−r)T1+rT2)]dsdr, | (10) |
where A=Tn+11Tm+12Γ(n+1)Γ(m+1).
The above integrals are hard to obtain, so numerical technique is used to evaluate the Bayes estimate. Markov Chain Monte Carlo (MCMC) approach is used to obtain Bayesian estimators. An important sub-class of the MCMC techniques is Gibbs's sampling and more general Metropolis within Gibbs samplers see [24,25]. The Metropolis-Hastings algorithm, together with the Gibbs sampling, are the two most popular examples of an MCMC method. It's similar to acceptance-rejection sampling, and the Metropolis-Hastings algorithm considers that to each iteration of the algorithm, a candidate value can be generated from the IR distributions. We use the Metropolis-Hastings within Gibbs sampling steps to generate random samples from conditional posterior densities of λ1, λ2, andRF. For more information, see [19,20,21,22,23].
In this section, we provide a numerical comparison using the Monte Carlo simulation algorithm. We explain our algorithm through an application of fuzzy and traditional stress-strength models by different estimation methods. In this current simulation, we will compare MLE, MPS, and Bayesian estimation methods based on traditional and fuzzy stress-strength measures for estimating the parameter of IR distribution. The comparison is made through bias and mean squared errors (MSE) of the different measures. The simulations are made using the R program for several combinations of the parameters and m,n, and k.
Simulation Algorithm:
We build our model by creating all simulation controls. At this point, we must follow the following steps in order:
Step 1: Suppose different values of the parameters vector of IR distribution.
Step 2: Choose the different sample size of strength
n=30,50,80,100,150200 and different sample size of stress
m=20,40,90,110,120and150 respectively.
Step 3: Generate the sample random values of IR distribution by using quantile function in equation
xi=λ1√−1ln(ui);0<u<1 and yi=λ2√−1ln(vi);0<v<1. |
Step 4: Solve differential equations for each estimation method. To obtain the estimators of the parameters for IR distribution, we calculate ˆλ1,ˆλ2, RF1 when k = 1; and RF2 when k = 5.
Step 5: Repeat this experiment (L-1) iterations. In each experiment, the parameter values are the same. The generated random values are certainly varying from experiment to experiment even though the sample size is not changed. In the end, we have L-values of mean and MSE, and we restricted the number of repeats in this experiment to 10,000. Take the averages of these values and call them Monte Carlo estimates.
After completing the treatment stage, simulated outcomes are listed in Tables 1–4, Figure 3, and the following observations were observed:
MLE | MPS | Bayesian | |||||
n,m | Bias | MSE | Bias | MSE | Bias | MSE | |
30, 20 | λ1 | 0.01688 | 0.009853 | -0.01175 | 0.008694 | 0.01667 | 0.009923 |
λ2 | 0.02254 | 0.012176 | -0.01042 | 0.010402 | 0.02298 | 0.012269 | |
R | 0.00191 | 0.004646 | -0.00010 | 0.004668 | 0.00222 | 0.004652 | |
RF1 | 0.00082 | 0.003707 | 0.00574 | 0.003819 | 0.00108 | 0.003709 | |
RF2 | 0.00123 | 0.004530 | 0.00173 | 0.004564 | 0.00152 | 0.004536 | |
50, 40 | λ1 | 0.01460 | 0.005687 | -0.00528 | 0.005089 | 0.01421 | 0.005724 |
λ2 | 0.01605 | 0.006444 | -0.00743 | 0.005703 | 0.01554 | 0.006470 | |
R | 0.00037 | 0.002765 | -0.00140 | 0.002770 | 0.00032 | 0.002777 | |
RF1 | -0.00101 | 0.002248 | 0.00214 | 0.002275 | -0.00096 | 0.002259 | |
RF2 | -0.00034 | 0.002712 | -0.00034 | 0.002716 | -0.00036 | 0.002724 | |
80, 90 | λ1 | 0.00467 | 0.003394 | -0.00915 | 0.003286 | 0.00454 | 0.003400 |
λ2 | 0.00465 | 0.002857 | -0.00793 | 0.002753 | 0.00475 | 0.002900 | |
R | 0.00025 | 0.001457 | 0.00088 | 0.001462 | 0.00035 | 0.001479 | |
RF1 | 0.00032 | 0.001266 | 0.00382 | 0.001296 | 0.00043 | 0.001280 | |
RF2 | 0.00015 | 0.001461 | 0.00187 | 0.001470 | 0.00026 | 0.001482 | |
100,110 | λ1 | 0.00709 | 0.002738 | -0.00456 | 0.002591 | 0.00702 | 0.002759 |
λ2 | 0.00432 | 0.002318 | -0.00647 | 0.002250 | 0.00435 | 0.002311 | |
R | -0.00120 | 0.001109 | -0.00079 | 0.001115 | -0.00113 | 0.001116 | |
RF1 | -0.00144 | 0.000968 | 0.00140 | 0.000980 | -0.00138 | 0.000976 | |
RF2 | -0.00143 | 0.001113 | -0.00011 | 0.001118 | -0.00136 | 0.001121 | |
150,120 | λ1 | 0.00495 | 0.001869 | -0.00344 | 0.001793 | 0.00502 | 0.001872 |
λ2 | 0.00500 | 0.002185 | -0.00504 | 0.002103 | 0.00476 | 0.002177 | |
R | -0.00012 | 0.000925 | -0.00095 | 0.000927 | -0.00026 | 0.000930 | |
RF1 | -0.00052 | 0.000753 | 0.00078 | 0.000756 | -0.00063 | 0.000757 | |
RF2 | -0.00034 | 0.000906 | -0.00041 | 0.000906 | -0.00048 | 0.000911 | |
200,150 | λ1 | 0.00143 | 0.001289 | -0.00518 | 0.001280 | 0.00146 | 0.001289 |
λ2 | -0.00026 | 0.001603 | -0.00862 | 0.001624 | -0.00023 | 0.001609 | |
R | -0.00101 | 0.000743 | -0.00190 | 0.000745 | -0.00101 | 0.000745 | |
RF1 | -0.00051 | 0.000586 | 0.00036 | 0.000587 | -0.00052 | 0.000586 | |
RF2 | -0.00091 | 0.000721 | -0.00118 | 0.000721 | -0.00091 | 0.000722 |
MLE | MPS | Bayesian | |||||
n,m | Bias | MSE | Bias | MSE | Bias | MSE | |
30, 20 | λ1 | 0.01722 | 0.009963 | -0.01153 | 0.008799 | 0.01663 | 0.009996 |
λ2 | 0.09455 | 0.209672 | -0.03808 | 0.178814 | 0.08884 | 0.200672 | |
R | -0.00244 | 0.002011 | -0.00378 | 0.002049 | -0.00251 | 0.001967 | |
RF1 | -0.00430 | 0.003224 | 0.00483 | 0.003249 | -0.00415 | 0.003192 | |
RF2 | -0.00377 | 0.002483 | -0.00125 | 0.002477 | -0.00377 | 0.002441 | |
50, 40 | λ1 | 0.00929 | 0.005053 | -0.01045 | 0.004713 | 0.00944 | 0.005122 |
λ2 | 0.02895 | 0.104113 | -0.06328 | 0.098289 | 0.02630 | 0.101853 | |
R | -0.00321 | 0.001175 | -0.00435 | 0.001196 | -0.00342 | 0.001176 | |
RF1 | -0.00357 | 0.001841 | 0.00262 | 0.001843 | -0.00374 | 0.001854 | |
RF2 | -0.00376 | 0.001436 | -0.00220 | 0.001430 | -0.00396 | 0.001442 | |
80, 90 | λ1 | 0.00908 | 0.003693 | -0.00466 | 0.003437 | 0.00905 | 0.003681 |
λ2 | 0.02371 | 0.046319 | -0.02677 | 0.044176 | 0.02369 | 0.046968 | |
R | -0.00193 | 0.000621 | -0.00159 | 0.000619 | -0.00193 | 0.000622 | |
RF1 | -0.00318 | 0.001212 | 0.00187 | 0.001202 | -0.00318 | 0.001210 | |
RF2 | -0.00268 | 0.000834 | -0.00057 | 0.000821 | -0.00269 | 0.000834 | |
100,110 | λ1 | 0.00456 | 0.002584 | -0.00704 | 0.002496 | 0.00453 | 0.002569 |
λ2 | 0.01642 | 0.034435 | -0.02646 | 0.033520 | 0.01529 | 0.034530 | |
R | -0.00092 | 0.000489 | -0.00064 | 0.000486 | -0.00102 | 0.000495 | |
RF1 | -0.00127 | 0.000913 | 0.00303 | 0.000916 | -0.00132 | 0.000915 | |
RF2 | -0.00124 | 0.000645 | 0.00055 | 0.000637 | -0.00132 | 0.000651 | |
150,120 | λ1 | 0.00318 | 0.001967 | -0.00528 | 0.001923 | 0.00302 | 0.001941 |
λ2 | 0.01613 | 0.035520 | -0.02435 | 0.034584 | 0.01556 | 0.035056 | |
R | -0.00057 | 0.000409 | -0.00110 | 0.000412 | -0.00056 | 0.000406 | |
RF1 | -0.00079 | 0.000700 | 0.00186 | 0.000704 | -0.00073 | 0.000694 | |
RF2 | -0.00079 | 0.000515 | -0.00016 | 0.000515 | -0.00076 | 0.000511 | |
200,150 | λ1 | 0.00140 | 0.001126 | -0.00524 | 0.001124 | 0.00142 | 0.001134 |
λ2 | 0.02209 | 0.028304 | -0.01175 | 0.027012 | 0.02163 | 0.028342 | |
R | 0.00057 | 0.000293 | 0.00001 | 0.000294 | 0.00053 | 0.000295 | |
RF1 | 0.00031 | 0.000442 | 0.00230 | 0.000448 | 0.00027 | 0.000445 | |
RF2 | 0.00042 | 0.000349 | 0.00079 | 0.000350 | 0.00038 | 0.000351 |
MLE | MPS | Bayesian | |||||
n,m | Bias | MSE | Bias | MSE | Bias | MSE | |
30, 20 | λ1 | 0.06763 | 0.155282 | -0.04768 | 0.137440 | 0.06555 | 0.156610 |
λ2 | 0.02046 | 0.011668 | -0.01262 | 0.010028 | 0.02030 | 0.011591 | |
R | 0.00402 | 0.001864 | 0.00273 | 0.001846 | 0.00420 | 0.001887 | |
RF1 | 0.00203 | 0.000444 | 0.00421 | 0.000483 | 0.00216 | 0.000452 | |
RF2 | 0.00298 | 0.001319 | 0.00439 | 0.001348 | 0.00317 | 0.001340 | |
50, 40 | λ1 | 0.04891 | 0.094691 | -0.02992 | 0.086752 | 0.04807 | 0.094583 |
λ2 | 0.01049 | 0.006881 | -0.01254 | 0.006335 | 0.01044 | 0.006899 | |
R | 0.00135 | 0.001199 | 0.00026 | 0.001189 | 0.00140 | 0.001200 | |
RF1 | 0.00076 | 0.000280 | 0.00215 | 0.000295 | 0.00079 | 0.000280 | |
RF2 | 0.00092 | 0.000848 | 0.00173 | 0.000856 | 0.00097 | 0.000847 | |
80, 90 | λ1 | 0.03999 | 0.054062 | -0.01579 | 0.050003 | 0.04016 | 0.053777 |
λ2 | 0.00592 | 0.002720 | -0.00667 | 0.002597 | 0.00561 | 0.002704 | |
R | -0.00002 | 0.000582 | 0.00040 | 0.000586 | -0.00013 | 0.000580 | |
RF1 | -0.00007 | 0.000147 | 0.00130 | 0.000155 | -0.00012 | 0.000147 | |
RF2 | -0.00027 | 0.000431 | 0.00116 | 0.000440 | -0.00035 | 0.000430 | |
100,110 | λ1 | 0.00977 | 0.042263 | -0.03626 | 0.041625 | 0.01012 | 0.042315 |
λ2 | 0.00263 | 0.002330 | -0.00810 | 0.002294 | 0.00291 | 0.002340 | |
R | 0.00112 | 0.000520 | 0.00137 | 0.000522 | 0.00118 | 0.000522 | |
RF1 | 0.00084 | 0.000130 | 0.00197 | 0.000137 | 0.00085 | 0.000131 | |
RF2 | 0.00107 | 0.000383 | 0.00219 | 0.000391 | 0.00111 | 0.000385 | |
150,120 | λ1 | 0.00724 | 0.028570 | -0.02618 | 0.028321 | 0.00687 | 0.028620 |
λ2 | 0.00732 | 0.002145 | -0.00283 | 0.002016 | 0.00731 | 0.002161 | |
R | 0.00236 | 0.000382 | 0.00182 | 0.000379 | 0.00240 | 0.000386 | |
RF1 | 0.00108 | 0.000090 | 0.00165 | 0.000093 | 0.00110 | 0.000091 | |
RF2 | 0.00184 | 0.000271 | 0.00214 | 0.000274 | 0.00188 | 0.000274 | |
200,150 | λ1 | 0.00595 | 0.020483 | -0.02065 | 0.020369 | 0.00619 | 0.020443 |
λ2 | 0.00547 | 0.001682 | -0.00295 | 0.001608 | 0.00545 | 0.001685 | |
R | 0.00171 | 0.000301 | 0.00116 | 0.000300 | 0.00168 | 0.000302 | |
RF1 | 0.00077 | 0.000068 | 0.00118 | 0.000070 | 0.00075 | 0.000068 | |
RF2 | 0.00133 | 0.000210 | 0.00148 | 0.000212 | 0.00130 | 0.000210 |
MLE | MPS | Bayesian | |||||
n, m | Bias | MSE | Bias | MSE | Bias | MSE | |
30, 20 | λ1 | 0.06224 | 0.176286 | -0.05263 | 0.157645 | 0.06459 | 0.177161 |
λ2 | 0.09727 | 0.203480 | -0.03537 | 0.172121 | 0.09340 | 0.199105 | |
R | 0.00357 | 0.004826 | 0.00162 | 0.004858 | 0.00292 | 0.004771 | |
RF1 | 0.00261 | 0.001646 | 0.00843 | 0.001815 | 0.00227 | 0.001636 | |
RF2 | 0.00246 | 0.004133 | 0.00686 | 0.004254 | 0.00188 | 0.004101 | |
50, 40 | λ1 | 0.03017 | 0.082788 | -0.04820 | 0.078270 | 0.03007 | 0.083022 |
λ2 | 0.06224 | 0.113595 | -0.03145 | 0.101059 | 0.06146 | 0.113486 | |
R | 0.00315 | 0.002556 | 0.00139 | 0.002559 | 0.00306 | 0.002556 | |
RF1 | 0.00181 | 0.000863 | 0.00564 | 0.000929 | 0.00178 | 0.000862 | |
RF2 | 0.00220 | 0.002158 | 0.00494 | 0.002204 | 0.00214 | 0.002157 | |
80, 90 | λ1 | 0.02923 | 0.051774 | -0.02658 | 0.048799 | 0.03008 | 0.052007 |
λ2 | 0.01866 | 0.047300 | -0.03152 | 0.045476 | 0.01861 | 0.047487 | |
R | -0.00118 | 0.001537 | -0.00050 | 0.001532 | -0.00129 | 0.001538 | |
RF1 | -0.00039 | 0.000552 | 0.00294 | 0.000576 | -0.00047 | 0.000553 | |
RF2 | -0.00131 | 0.001370 | 0.00200 | 0.001378 | -0.00142 | 0.001371 | |
100,110 | λ1 | 0.02007 | 0.043336 | -0.02620 | 0.041573 | 0.02040 | 0.043196 |
λ2 | 0.01477 | 0.035742 | -0.02818 | 0.034901 | 0.01559 | 0.035898 | |
R | -0.00043 | 0.001201 | -0.00003 | 0.001200 | -0.00038 | 0.001204 | |
RF1 | 0.00010 | 0.000455 | 0.00281 | 0.000473 | 0.00010 | 0.000455 | |
RF2 | -0.00048 | 0.001100 | 0.00215 | 0.001108 | -0.00046 | 0.001101 | |
150,120 | λ1 | 0.02229 | 0.027613 | -0.01152 | 0.026372 | 0.02199 | 0.027322 |
λ2 | 0.01364 | 0.036194 | -0.02658 | 0.035347 | 0.01402 | 0.036164 | |
R | -0.00135 | 0.000946 | -0.00218 | 0.000952 | -0.00127 | 0.000944 | |
RF1 | -0.00084 | 0.000300 | 0.00075 | 0.000305 | -0.00080 | 0.000298 | |
RF2 | -0.00157 | 0.000781 | -0.00045 | 0.000783 | -0.00151 | 0.000778 | |
200,150 | λ1 | 0.01240 | 0.020863 | -0.01433 | 0.020372 | 0.01221 | 0.020684 |
λ2 | 0.01492 | 0.025875 | -0.01865 | 0.025209 | 0.01504 | 0.025844 | |
R | 0.00016 | 0.000705 | -0.00069 | 0.000707 | 0.00020 | 0.000703 | |
RF1 | 0.00002 | 0.000232 | 0.00122 | 0.000237 | 0.00004 | 0.000231 | |
RF2 | -0.00011 | 0.000594 | 0.00064 | 0.000597 | -0.00007 | 0.000592 |
● The Bias and MSE decrease as sample sizes increase for all estimates.
● For fixed values of λ1, the biases and MSE's of estimates of parameters are increasing with λ2 increasing.
● For fixed values of λ1, the biases and MSE's of estimates of parameters are increasing with λ2 increasing, but the estimate of R for fuzzy stress strength and tradition are decreasing in approximately most situations.
● For fixed values of λ2, the bias and MSE of estimates of λ1, R for fuzzy stress strength and tradition are decreasing with λ1 increasing, but the estimates of λ2 are increasing, in approximately most situations.
● The MPS method is found to be superior to the MLE and Bayesian methods in most cases.
● From the observed results of reliability, we note the efficiency of the fuzzy stress strength is over traditional stress strength in most situations according to Bias and MSE.
● In fuzzy stress strength, the efficiency is better with decreased values of k according to Bias and MSE.
The numerical results of tradition and fuzzy stress-strength reliability estimation of the IR distribution for real data are presented in this section.
Two real stress and strength data sets contained times to breakdown down an insulating fluid between electrodes recorded at different voltages; these data have been discussed by [26]. Data Ⅰ and data Ⅱ as presented in Table 4, are the failure times (in minutes) are presented, which are for an insulating fluid between two electrodes subject to a voltage of 34 kV (data set Ⅰ) and 36 kV (data set Ⅱ). Table 6 provides information about the estimated parameters of the IR model and the corresponding traditional and fuzzy reliability measures
Data Ⅰ | 0.19 | 0.78 | 0.96 | 1.31 | 2.78 | 3.16 | 4.15 | 4.67 | 4.85 | 6.5 |
7.35 | 8.01 | 8.27 | 12.06 | 31.75 | 32.52 | 33.91 | 36.71 | 72.89 | - | |
Data Ⅱ | 0.35 | 0.59 | 0.96 | 0.99 | 1.69 | 1.97 | 2.07 | 2.58 | 2.71 | 2.9 |
3.67 | 3.99 | 5.35 | 13.77 | 25.50 | - | - | - | - | - |
λ1 | λ2 | R | RF1 | RF2 | |
MLE | 0.60363 | 1.02982 | 0.63046 | 0.39315 | 0.56254 |
MPS | 0.55815 | 0.89220 | 0.61516 | 0.39480 | 0.55339 |
Bayesian | 0.64494 | 1.12664 | 0.63595 | 0.38661 | 0.56330 |
The graph of MCMC estimates for λ1 and λ2 using the MH algorithm are the plotting of estimates, histogram of estimates, and convergence of estimates are shown in Figure 3. In Figure 4, we note the convergence of MCMC estimates for λ1 and λ2 in the first quartile iteration.
The new approach of estimating fuzzy stress-strength reliability RF=P(Y<X) is getting much attention because of the properties of RF, which makes the analysis more sensitive and more reliable. Also, when the study results are not known completely, the use of traditional methods may be misleading, and the need for new approaches that can handle such situations is very important. In this paper, the stress and strength variables were distributed as inverted Rayleigh distribution. It can be noted that different membership functions will provide different measures of RF. It is also noted that the MPS method is superior to the MLE and Bayesian methods in most cases.
Thanks a lot for everyone's help.
The authors declare that they have no conflict of interest to report regarding the present study.
[1] |
H. Z. Huang, Reliability analysis method in the presence of fuzziness attached to operating time, Microelectro. Reliab., 35 (1995), 1483-1487. doi: 10.1016/0026-2714(94)00173-L
![]() |
[2] |
H. Z. Huang, M. J. Zuo, Z. Q. Sun, Bayesian reliability analysis for fuzzy lifetime data, Fuzzy Sets Syst., 157 (2006), 1674-1686. doi: 10.1016/j.fss.2005.11.009
![]() |
[3] |
H. C. Wu, Fuzzy reliability estimation using Bayesian approach, Comput. Ind. Eng., 46 (2004), 467-493. doi: 10.1016/j.cie.2004.01.009
![]() |
[4] |
H. C. Wu, Fuzzy Bayesian system reliability assessment based on exponential distribution, Appl. Math. Model., 30 (2006), 509-530. doi: 10.1016/j.apm.2005.05.014
![]() |
[5] |
S. Eryilmaz, G. Y. Tütüncüb, Stress strength reliability in the presence of fuzziness, J. Comput. Appl. Math., 282 (2015), 262-267. doi: 10.1016/j.cam.2014.12.047
![]() |
[6] |
M. A. Hussian, E. Amin, A. Fuzzy, Reliability estimation for exponential distribution using ranked set sampling, IJCMS, 12 (2017), 31-42. doi: 10.12988/ijcms.2017.612158
![]() |
[7] |
D. Bamber, The area above the ordinal dominance graph and the area below the receiver operating graph, J. Math. Psychol., 12 (1975), 387-415. doi: 10.1016/0022-2496(75)90001-2
![]() |
[8] |
M. Z. Raqab, M. T. Madi, D. Kundu, Estimation of P (Y < X) for a 3-parameter generalized exponential distribution, Com. Stat. Theory Methods, 37 (2008), 2854-2864. doi: 10.1080/03610920802162664
![]() |
[9] |
A. Wong, Interval estimation of P (Y < X) for generalized Pareto distribution, J. Stat. Plan. Infer., 142 (2012), 601-607. doi: 10.1016/j.jspi.2011.04.024
![]() |
[10] |
A. Asgharzadeh, R. Valiollahi, M. Z. Raqab, Estimation of the stress-strength reliability for the generalized logistic distribution, Stat. Method, 15 (2013), 73-94. doi: 10.1016/j.stamet.2013.05.002
![]() |
[11] |
F. G. Akgül, B. Şenoğlu, Estimation of P (X < Y) using some modifications of ranked set sampling for Weibull distribution, Pak. J. Stat. Oper. Res., 13 (2017), 931-958. doi: 10.18187/pjsor.v13i4.2056
![]() |
[12] | A. I. Al-Omari, I. M. Almanjahie, A. S. Hassan, H. F. Nagy, Estimation of the stress-strength reliability for exponentiated Pareto distribution using median and ranked set sampling methods, CMC, 64 (2020), 835-857. |
[13] | F. P. A. Coolen, M. J. Newby, A note on the use of the product of spacings in Bayesian inference, Memorandum COSOR, Vol. 9035, Technische Universiteit Eindhoven, 1990. |
[14] |
R. Alshenawy, M. A. H. Sabry, E. M. Almetwally, H. M. Elomngy, Product spacing of stress-strength under progressive hybrid censored for exponentiated-gumbel distribution, Comput. Mater. Continua, 66 (2021), 2973-2995. doi: 10.32604/cmc.2021.014289
![]() |
[15] | R. K. Singh, S. K. Singh, U. Singh, Maximum product spacings method for the estimation of parameters of generalized inverted exponential distribution under Progressive Type Ⅱ Censoring, J. Stat. Manage. Syst., 19 (2016), 219-245. |
[16] |
H. M. Almongy, F. Y. Alshenawy, E. M. Almetwally, D. A. Abdo, Applying transformer insulation using Weibull extended distribution based on progressive censoring scheme, Axioms, 10 (2021), 100. doi: 10.3390/axioms10020100
![]() |
[17] |
E. S. A. El-Sherpieny, E. M. Almetwally, H. Z. Muhammed, Progressive Type-Ⅱ hybrid censored schemes based on maximum product spacing with application to Power Lomax distribution, Phys. A: Stat. Mech. Appl., 553 (2020), 124251. doi: 10.1016/j.physa.2020.124251
![]() |
[18] | E. M. Almetwally, H. M. Almongy, M. K. Rastogi, M. Ibrahim, Maximum product spacing estimation of Weibull distribution under adaptive type-Ⅱ progressive censoring schemes, Ann. Data Sci., 7 (2020), 257-279. |
[19] |
K. Krishnamoorthy, S. Mukherjee, H. Guo, Inference on reliability in two-parameter exponential stress-strength model, Metrika, 65 (2007), 261-273. doi: 10.1007/s00184-006-0074-7
![]() |
[20] | A. Gelman, J. B. Carlin, H. S. Stern, D. B. Dunson, A. Vehtari, D. B. Rubin, Bayesian data analysis, CRC press, 2013. |
[21] | E. M. Almetwally, H. M. Almongy, Parameter estimation and stress-strength model of power Lomax distribution, classical methods and Bayesian estimation, J. Data Sci., 18 (2020), 718-738. |
[22] | E. M. Almetwally, H. M. Almongy, A. El sayed Mubarak, Bayesian and maximum likelihood estimation for the Weibull generalized exponential distribution parameters using progressive censoring schemes, Pak. J. Stat. Oper. Res., 14 (2018), 853-868. |
[23] | H. M. Almongy, E. M. Almetwally, R. Alharbi, D. Alnagar, E. H. Hafez, M. M. Mohie El-Din, The Weibull generalized exponential distribution with censored sample: estimation and application on real data. Complexity, 2021 (2021), 1-15. |
[24] |
W. R. Gilks, P. Wild, Adaptive rejection sampling for Gibbs sampling, Appl. Stat., 41 (1992), 337-348. doi: 10.2307/2347565
![]() |
[25] |
W. R. Gilks, N. G. Best, K. K. C. Tan, Adaptive rejection Metropolis sampling within Gibbs sampling, Appl. Stat., 44 (1995), 455-472. doi: 10.2307/2986138
![]() |
[26] | L. Martino, J. Read, D. Luengo, Independent doubly adaptive rejection Metropolis sampling within Gibbs sampling, IEEE Trans. Signal Process., 63 (2015), 3132-3138. |
1. | Saurabh L. Raikar, Dr. Rajesh S. Prabhu Gaonkar, Jaya algorithm in estimation of P[X > Y] for two parameter Weibull distribution, 2021, 7, 2473-6988, 2820, 10.3934/math.2022156 | |
2. | Ahmed Sayed M. Metwally, Amal S. Hassan, Ehab M. Almetwally, B M Golam Kibria, Hisham M. Almongy, Reliability Analysis of the New Exponential Inverted Topp–Leone Distribution with Applications, 2021, 23, 1099-4300, 1662, 10.3390/e23121662 | |
3. | Majd Alslman, Amal Helu, Lili Yu, Estimation of the stress-strength reliability for the inverse Weibull distribution under adaptive type-II progressive hybrid censoring, 2022, 17, 1932-6203, e0277514, 10.1371/journal.pone.0277514 | |
4. | Wael S. Abu El Azm, Ehab M. Almetwally, Abdulaziz S. Alghamdi, Hassan M. Aljohani, Abdisalam Hassan Muse, O. E. Abo-Kasem, Ahmed Mostafa Khalil, Stress-Strength Reliability for Exponentiated Inverted Weibull Distribution with Application on Breaking of Jute Fiber and Carbon Fibers, 2021, 2021, 1687-5273, 1, 10.1155/2021/4227346 | |
5. | Jiju Gillariose, Oluwafemi Samson Balogun, Ehab M. Almetwally, Rehan Ahmad Khan Sherwani, Farrukh Jamal, Joshin Joseph, On the Discrete Weibull Marshall–Olkin Family of Distributions: Properties, Characterizations, and Applications, 2021, 10, 2075-1680, 287, 10.3390/axioms10040287 | |
6. | Md. Awwal Islam Khan, Amirah Saeed Alharthi, Hassan M. Aljohani, Taghreed M. Jawa, Neveen Sayed-Ahmed, Md. Shohel Rana, Md. Mahabubur Rahman, Saima K Khosa, Classical Inference of the Cubic Transmuted Lindley Distribution under Type-II Censored Sample, 2022, 2022, 1563-5147, 1, 10.1155/2022/3543517 | |
7. | Osama Abdulaziz Alamri, Abdulrahman H Alessa, Eslam Hussam, Marwan H. Alhelali, Mutua Kilai, Statistical modelling for the Covid-19 mortality rate in the Kingdom of Saudi Arabia, 2023, 68, 11100168, 517, 10.1016/j.aej.2023.01.024 | |
8. | Ehab M. Almetwally, Refah Alotaibi, Aned Al Mutairi, Chanseok Park, Hoda Rezk, Optimal Plan of Multi-Stress–Strength Reliability Bayesian and Non-Bayesian Methods for the Alpha Power Exponential Model Using Progressive First Failure, 2022, 14, 2073-8994, 1306, 10.3390/sym14071306 | |
9. | Rania A. H. Mohamed, Ahlam H. Tolba, Ehab M. Almetwally, Dina A. Ramadan, Inference of Reliability Analysis for Type II Half Logistic Weibull Distribution with Application of Bladder Cancer, 2022, 11, 2075-1680, 386, 10.3390/axioms11080386 | |
10. | Manal M. Yousef, Amal S. Hassan, Huda M. Alshanbari, Abdal-Aziz H. El-Bagoury, Ehab M. Almetwally, Bayesian and Non-Bayesian Analysis of Exponentiated Exponential Stress–Strength Model Based on Generalized Progressive Hybrid Censoring Process, 2022, 11, 2075-1680, 455, 10.3390/axioms11090455 | |
11. | Manal M. Yousef, Ehab M. Almetwally, Multi Stress-Strength Reliability Based on Progressive First Failure for Kumaraswamy Model: Bayesian and Non-Bayesian Estimation, 2021, 13, 2073-8994, 2120, 10.3390/sym13112120 | |
12. | Akram Kohansal, Carlos J. Pérez-González, Arturo J. Fernández, Multi-component Reliability Inference in Modified Weibull Extension Distribution and Progressive Censoring Scheme, 2023, 46, 0126-6705, 10.1007/s40840-022-01453-3 | |
13. | Rania A. H. Mohamed, Ibrahim Elbatal, Ehab M. ALmetwally, Mohammed Elgarhy, Hisham M. Almongy, Bayesian Estimation of a Transmuted Topp-Leone Length Biased Exponential Model Based on Competing Risk with the Application of Electrical Appliances, 2022, 10, 2227-7390, 4042, 10.3390/math10214042 | |
14. | Hanan Haj Ahmad, Ehab M. Almetwally, Dina A. Ramadan, A comparative inference on reliability estimation for a multi-component stress-strength model under power Lomax distribution with applications, 2022, 7, 2473-6988, 18050, 10.3934/math.2022994 | |
15. | Ahlam Tolba, Dina Ramadan, Ehab Almetwally, Taghreed Jawa, Neveen Sayed-Ahmed, Statistical inference for stress-strength reliability using inverse Lomax lifetime distribution with mechanical engineering applications, 2022, 26, 0354-9836, 303, 10.2298/TSCI22S1303T | |
16. | Abdulhakim A. Al-Babtain, I. Elbatal, Ehab M. Almetwally, Ahmed Mostafa Khalil, Bayesian and Non-Bayesian Reliability Estimation of Stress-Strength Model for Power-Modified Lindley Distribution, 2022, 2022, 1687-5273, 1, 10.1155/2022/1154705 | |
17. | Bouhadjar Meriem, Ahmed M. Gemeay, Ehab M. Almetwally, Zeghdoudi Halim, Etaf Alshawarbeh, Alanazi Talal Abdulrahman, M. M. Abd El-Raouf, Eslam Hussam, Muhammad Gulzar, The Power XLindley Distribution: Statistical Inference, Fuzzy Reliability, and COVID-19 Application, 2022, 2022, 2314-8888, 1, 10.1155/2022/9094078 | |
18. | El-Sayed A. El-Sherpieny, Ehab M. Almetwally, Abdisalam Hassan Muse, Eslam Hussam, Qichun Zhang, Data analysis for COVID-19 deaths using a novel statistical model: Simulation and fuzzy application, 2023, 18, 1932-6203, e0283618, 10.1371/journal.pone.0283618 | |
19. | Marwa K. H. Hassan, Abdisalam Hassan Muse, Sania Qureshi, Fuzzy Stress‐Strength Model and Mean Remaining Strength for Lindley Distribution: Estimation and Application in Cancer of Benign Endocrine, 2023, 2023, 1748-670X, 10.1155/2023/8952946 | |
20. | Dina A. Ramadan, Ehab M. Almetwally, Ahlam H. Tolba, Statistical inference for multi stress–strength reliability based on progressive first failure with lifetime inverse Lomax distribution and analysis of transformer insulation data, 2023, 39, 0748-8017, 2558, 10.1002/qre.3362 | |
21. | Tingting Shan, 2023, Fuzzy Mathematical Reliability Modeling Based on Robustness Analysis, 979-8-3503-1646-9, 1, 10.1109/AIKIIE60097.2023.10390109 | |
22. | Sunita Sharma, Vinod Kumar, Bayesian reliability estimation of weighted exponential-lindley distribution with intuitionistic fuzzy lifetime data, 2024, 13, 2520-1352, 399, 10.1007/s41872-024-00274-6 | |
23. | Eslam Hussam, Mohamed A. Sabry, M. M. Abd El-Raouf, Ehab M. Almetwally, Fuzzy vs. Traditional Reliability Model for Inverse Weibull Distribution, 2023, 12, 2075-1680, 582, 10.3390/axioms12060582 | |
24. | Neama Salah Youssef Temraz, Fuzzy multicomponent stress-strength reliability in presence of partially accelerated life testing under generalized progressive hybrid censoring scheme subject to inverse Weibull model, 2024, 12, 22150161, 102586, 10.1016/j.mex.2024.102586 |
MLE | MPS | Bayesian | |||||
n,m | Bias | MSE | Bias | MSE | Bias | MSE | |
30, 20 | λ1 | 0.01688 | 0.009853 | -0.01175 | 0.008694 | 0.01667 | 0.009923 |
λ2 | 0.02254 | 0.012176 | -0.01042 | 0.010402 | 0.02298 | 0.012269 | |
R | 0.00191 | 0.004646 | -0.00010 | 0.004668 | 0.00222 | 0.004652 | |
RF1 | 0.00082 | 0.003707 | 0.00574 | 0.003819 | 0.00108 | 0.003709 | |
RF2 | 0.00123 | 0.004530 | 0.00173 | 0.004564 | 0.00152 | 0.004536 | |
50, 40 | λ1 | 0.01460 | 0.005687 | -0.00528 | 0.005089 | 0.01421 | 0.005724 |
λ2 | 0.01605 | 0.006444 | -0.00743 | 0.005703 | 0.01554 | 0.006470 | |
R | 0.00037 | 0.002765 | -0.00140 | 0.002770 | 0.00032 | 0.002777 | |
RF1 | -0.00101 | 0.002248 | 0.00214 | 0.002275 | -0.00096 | 0.002259 | |
RF2 | -0.00034 | 0.002712 | -0.00034 | 0.002716 | -0.00036 | 0.002724 | |
80, 90 | λ1 | 0.00467 | 0.003394 | -0.00915 | 0.003286 | 0.00454 | 0.003400 |
λ2 | 0.00465 | 0.002857 | -0.00793 | 0.002753 | 0.00475 | 0.002900 | |
R | 0.00025 | 0.001457 | 0.00088 | 0.001462 | 0.00035 | 0.001479 | |
RF1 | 0.00032 | 0.001266 | 0.00382 | 0.001296 | 0.00043 | 0.001280 | |
RF2 | 0.00015 | 0.001461 | 0.00187 | 0.001470 | 0.00026 | 0.001482 | |
100,110 | λ1 | 0.00709 | 0.002738 | -0.00456 | 0.002591 | 0.00702 | 0.002759 |
λ2 | 0.00432 | 0.002318 | -0.00647 | 0.002250 | 0.00435 | 0.002311 | |
R | -0.00120 | 0.001109 | -0.00079 | 0.001115 | -0.00113 | 0.001116 | |
RF1 | -0.00144 | 0.000968 | 0.00140 | 0.000980 | -0.00138 | 0.000976 | |
RF2 | -0.00143 | 0.001113 | -0.00011 | 0.001118 | -0.00136 | 0.001121 | |
150,120 | λ1 | 0.00495 | 0.001869 | -0.00344 | 0.001793 | 0.00502 | 0.001872 |
λ2 | 0.00500 | 0.002185 | -0.00504 | 0.002103 | 0.00476 | 0.002177 | |
R | -0.00012 | 0.000925 | -0.00095 | 0.000927 | -0.00026 | 0.000930 | |
RF1 | -0.00052 | 0.000753 | 0.00078 | 0.000756 | -0.00063 | 0.000757 | |
RF2 | -0.00034 | 0.000906 | -0.00041 | 0.000906 | -0.00048 | 0.000911 | |
200,150 | λ1 | 0.00143 | 0.001289 | -0.00518 | 0.001280 | 0.00146 | 0.001289 |
λ2 | -0.00026 | 0.001603 | -0.00862 | 0.001624 | -0.00023 | 0.001609 | |
R | -0.00101 | 0.000743 | -0.00190 | 0.000745 | -0.00101 | 0.000745 | |
RF1 | -0.00051 | 0.000586 | 0.00036 | 0.000587 | -0.00052 | 0.000586 | |
RF2 | -0.00091 | 0.000721 | -0.00118 | 0.000721 | -0.00091 | 0.000722 |
MLE | MPS | Bayesian | |||||
n,m | Bias | MSE | Bias | MSE | Bias | MSE | |
30, 20 | λ1 | 0.01722 | 0.009963 | -0.01153 | 0.008799 | 0.01663 | 0.009996 |
λ2 | 0.09455 | 0.209672 | -0.03808 | 0.178814 | 0.08884 | 0.200672 | |
R | -0.00244 | 0.002011 | -0.00378 | 0.002049 | -0.00251 | 0.001967 | |
RF1 | -0.00430 | 0.003224 | 0.00483 | 0.003249 | -0.00415 | 0.003192 | |
RF2 | -0.00377 | 0.002483 | -0.00125 | 0.002477 | -0.00377 | 0.002441 | |
50, 40 | λ1 | 0.00929 | 0.005053 | -0.01045 | 0.004713 | 0.00944 | 0.005122 |
λ2 | 0.02895 | 0.104113 | -0.06328 | 0.098289 | 0.02630 | 0.101853 | |
R | -0.00321 | 0.001175 | -0.00435 | 0.001196 | -0.00342 | 0.001176 | |
RF1 | -0.00357 | 0.001841 | 0.00262 | 0.001843 | -0.00374 | 0.001854 | |
RF2 | -0.00376 | 0.001436 | -0.00220 | 0.001430 | -0.00396 | 0.001442 | |
80, 90 | λ1 | 0.00908 | 0.003693 | -0.00466 | 0.003437 | 0.00905 | 0.003681 |
λ2 | 0.02371 | 0.046319 | -0.02677 | 0.044176 | 0.02369 | 0.046968 | |
R | -0.00193 | 0.000621 | -0.00159 | 0.000619 | -0.00193 | 0.000622 | |
RF1 | -0.00318 | 0.001212 | 0.00187 | 0.001202 | -0.00318 | 0.001210 | |
RF2 | -0.00268 | 0.000834 | -0.00057 | 0.000821 | -0.00269 | 0.000834 | |
100,110 | λ1 | 0.00456 | 0.002584 | -0.00704 | 0.002496 | 0.00453 | 0.002569 |
λ2 | 0.01642 | 0.034435 | -0.02646 | 0.033520 | 0.01529 | 0.034530 | |
R | -0.00092 | 0.000489 | -0.00064 | 0.000486 | -0.00102 | 0.000495 | |
RF1 | -0.00127 | 0.000913 | 0.00303 | 0.000916 | -0.00132 | 0.000915 | |
RF2 | -0.00124 | 0.000645 | 0.00055 | 0.000637 | -0.00132 | 0.000651 | |
150,120 | λ1 | 0.00318 | 0.001967 | -0.00528 | 0.001923 | 0.00302 | 0.001941 |
λ2 | 0.01613 | 0.035520 | -0.02435 | 0.034584 | 0.01556 | 0.035056 | |
R | -0.00057 | 0.000409 | -0.00110 | 0.000412 | -0.00056 | 0.000406 | |
RF1 | -0.00079 | 0.000700 | 0.00186 | 0.000704 | -0.00073 | 0.000694 | |
RF2 | -0.00079 | 0.000515 | -0.00016 | 0.000515 | -0.00076 | 0.000511 | |
200,150 | λ1 | 0.00140 | 0.001126 | -0.00524 | 0.001124 | 0.00142 | 0.001134 |
λ2 | 0.02209 | 0.028304 | -0.01175 | 0.027012 | 0.02163 | 0.028342 | |
R | 0.00057 | 0.000293 | 0.00001 | 0.000294 | 0.00053 | 0.000295 | |
RF1 | 0.00031 | 0.000442 | 0.00230 | 0.000448 | 0.00027 | 0.000445 | |
RF2 | 0.00042 | 0.000349 | 0.00079 | 0.000350 | 0.00038 | 0.000351 |
MLE | MPS | Bayesian | |||||
n,m | Bias | MSE | Bias | MSE | Bias | MSE | |
30, 20 | λ1 | 0.06763 | 0.155282 | -0.04768 | 0.137440 | 0.06555 | 0.156610 |
λ2 | 0.02046 | 0.011668 | -0.01262 | 0.010028 | 0.02030 | 0.011591 | |
R | 0.00402 | 0.001864 | 0.00273 | 0.001846 | 0.00420 | 0.001887 | |
RF1 | 0.00203 | 0.000444 | 0.00421 | 0.000483 | 0.00216 | 0.000452 | |
RF2 | 0.00298 | 0.001319 | 0.00439 | 0.001348 | 0.00317 | 0.001340 | |
50, 40 | λ1 | 0.04891 | 0.094691 | -0.02992 | 0.086752 | 0.04807 | 0.094583 |
λ2 | 0.01049 | 0.006881 | -0.01254 | 0.006335 | 0.01044 | 0.006899 | |
R | 0.00135 | 0.001199 | 0.00026 | 0.001189 | 0.00140 | 0.001200 | |
RF1 | 0.00076 | 0.000280 | 0.00215 | 0.000295 | 0.00079 | 0.000280 | |
RF2 | 0.00092 | 0.000848 | 0.00173 | 0.000856 | 0.00097 | 0.000847 | |
80, 90 | λ1 | 0.03999 | 0.054062 | -0.01579 | 0.050003 | 0.04016 | 0.053777 |
λ2 | 0.00592 | 0.002720 | -0.00667 | 0.002597 | 0.00561 | 0.002704 | |
R | -0.00002 | 0.000582 | 0.00040 | 0.000586 | -0.00013 | 0.000580 | |
RF1 | -0.00007 | 0.000147 | 0.00130 | 0.000155 | -0.00012 | 0.000147 | |
RF2 | -0.00027 | 0.000431 | 0.00116 | 0.000440 | -0.00035 | 0.000430 | |
100,110 | λ1 | 0.00977 | 0.042263 | -0.03626 | 0.041625 | 0.01012 | 0.042315 |
λ2 | 0.00263 | 0.002330 | -0.00810 | 0.002294 | 0.00291 | 0.002340 | |
R | 0.00112 | 0.000520 | 0.00137 | 0.000522 | 0.00118 | 0.000522 | |
RF1 | 0.00084 | 0.000130 | 0.00197 | 0.000137 | 0.00085 | 0.000131 | |
RF2 | 0.00107 | 0.000383 | 0.00219 | 0.000391 | 0.00111 | 0.000385 | |
150,120 | λ1 | 0.00724 | 0.028570 | -0.02618 | 0.028321 | 0.00687 | 0.028620 |
λ2 | 0.00732 | 0.002145 | -0.00283 | 0.002016 | 0.00731 | 0.002161 | |
R | 0.00236 | 0.000382 | 0.00182 | 0.000379 | 0.00240 | 0.000386 | |
RF1 | 0.00108 | 0.000090 | 0.00165 | 0.000093 | 0.00110 | 0.000091 | |
RF2 | 0.00184 | 0.000271 | 0.00214 | 0.000274 | 0.00188 | 0.000274 | |
200,150 | λ1 | 0.00595 | 0.020483 | -0.02065 | 0.020369 | 0.00619 | 0.020443 |
λ2 | 0.00547 | 0.001682 | -0.00295 | 0.001608 | 0.00545 | 0.001685 | |
R | 0.00171 | 0.000301 | 0.00116 | 0.000300 | 0.00168 | 0.000302 | |
RF1 | 0.00077 | 0.000068 | 0.00118 | 0.000070 | 0.00075 | 0.000068 | |
RF2 | 0.00133 | 0.000210 | 0.00148 | 0.000212 | 0.00130 | 0.000210 |
MLE | MPS | Bayesian | |||||
n, m | Bias | MSE | Bias | MSE | Bias | MSE | |
30, 20 | λ1 | 0.06224 | 0.176286 | -0.05263 | 0.157645 | 0.06459 | 0.177161 |
λ2 | 0.09727 | 0.203480 | -0.03537 | 0.172121 | 0.09340 | 0.199105 | |
R | 0.00357 | 0.004826 | 0.00162 | 0.004858 | 0.00292 | 0.004771 | |
RF1 | 0.00261 | 0.001646 | 0.00843 | 0.001815 | 0.00227 | 0.001636 | |
RF2 | 0.00246 | 0.004133 | 0.00686 | 0.004254 | 0.00188 | 0.004101 | |
50, 40 | λ1 | 0.03017 | 0.082788 | -0.04820 | 0.078270 | 0.03007 | 0.083022 |
λ2 | 0.06224 | 0.113595 | -0.03145 | 0.101059 | 0.06146 | 0.113486 | |
R | 0.00315 | 0.002556 | 0.00139 | 0.002559 | 0.00306 | 0.002556 | |
RF1 | 0.00181 | 0.000863 | 0.00564 | 0.000929 | 0.00178 | 0.000862 | |
RF2 | 0.00220 | 0.002158 | 0.00494 | 0.002204 | 0.00214 | 0.002157 | |
80, 90 | λ1 | 0.02923 | 0.051774 | -0.02658 | 0.048799 | 0.03008 | 0.052007 |
λ2 | 0.01866 | 0.047300 | -0.03152 | 0.045476 | 0.01861 | 0.047487 | |
R | -0.00118 | 0.001537 | -0.00050 | 0.001532 | -0.00129 | 0.001538 | |
RF1 | -0.00039 | 0.000552 | 0.00294 | 0.000576 | -0.00047 | 0.000553 | |
RF2 | -0.00131 | 0.001370 | 0.00200 | 0.001378 | -0.00142 | 0.001371 | |
100,110 | λ1 | 0.02007 | 0.043336 | -0.02620 | 0.041573 | 0.02040 | 0.043196 |
λ2 | 0.01477 | 0.035742 | -0.02818 | 0.034901 | 0.01559 | 0.035898 | |
R | -0.00043 | 0.001201 | -0.00003 | 0.001200 | -0.00038 | 0.001204 | |
RF1 | 0.00010 | 0.000455 | 0.00281 | 0.000473 | 0.00010 | 0.000455 | |
RF2 | -0.00048 | 0.001100 | 0.00215 | 0.001108 | -0.00046 | 0.001101 | |
150,120 | λ1 | 0.02229 | 0.027613 | -0.01152 | 0.026372 | 0.02199 | 0.027322 |
λ2 | 0.01364 | 0.036194 | -0.02658 | 0.035347 | 0.01402 | 0.036164 | |
R | -0.00135 | 0.000946 | -0.00218 | 0.000952 | -0.00127 | 0.000944 | |
RF1 | -0.00084 | 0.000300 | 0.00075 | 0.000305 | -0.00080 | 0.000298 | |
RF2 | -0.00157 | 0.000781 | -0.00045 | 0.000783 | -0.00151 | 0.000778 | |
200,150 | λ1 | 0.01240 | 0.020863 | -0.01433 | 0.020372 | 0.01221 | 0.020684 |
λ2 | 0.01492 | 0.025875 | -0.01865 | 0.025209 | 0.01504 | 0.025844 | |
R | 0.00016 | 0.000705 | -0.00069 | 0.000707 | 0.00020 | 0.000703 | |
RF1 | 0.00002 | 0.000232 | 0.00122 | 0.000237 | 0.00004 | 0.000231 | |
RF2 | -0.00011 | 0.000594 | 0.00064 | 0.000597 | -0.00007 | 0.000592 |
Data Ⅰ | 0.19 | 0.78 | 0.96 | 1.31 | 2.78 | 3.16 | 4.15 | 4.67 | 4.85 | 6.5 |
7.35 | 8.01 | 8.27 | 12.06 | 31.75 | 32.52 | 33.91 | 36.71 | 72.89 | - | |
Data Ⅱ | 0.35 | 0.59 | 0.96 | 0.99 | 1.69 | 1.97 | 2.07 | 2.58 | 2.71 | 2.9 |
3.67 | 3.99 | 5.35 | 13.77 | 25.50 | - | - | - | - | - |
λ1 | λ2 | R | RF1 | RF2 | |
MLE | 0.60363 | 1.02982 | 0.63046 | 0.39315 | 0.56254 |
MPS | 0.55815 | 0.89220 | 0.61516 | 0.39480 | 0.55339 |
Bayesian | 0.64494 | 1.12664 | 0.63595 | 0.38661 | 0.56330 |
MLE | MPS | Bayesian | |||||
n,m | Bias | MSE | Bias | MSE | Bias | MSE | |
30, 20 | λ1 | 0.01688 | 0.009853 | -0.01175 | 0.008694 | 0.01667 | 0.009923 |
λ2 | 0.02254 | 0.012176 | -0.01042 | 0.010402 | 0.02298 | 0.012269 | |
R | 0.00191 | 0.004646 | -0.00010 | 0.004668 | 0.00222 | 0.004652 | |
RF1 | 0.00082 | 0.003707 | 0.00574 | 0.003819 | 0.00108 | 0.003709 | |
RF2 | 0.00123 | 0.004530 | 0.00173 | 0.004564 | 0.00152 | 0.004536 | |
50, 40 | λ1 | 0.01460 | 0.005687 | -0.00528 | 0.005089 | 0.01421 | 0.005724 |
λ2 | 0.01605 | 0.006444 | -0.00743 | 0.005703 | 0.01554 | 0.006470 | |
R | 0.00037 | 0.002765 | -0.00140 | 0.002770 | 0.00032 | 0.002777 | |
RF1 | -0.00101 | 0.002248 | 0.00214 | 0.002275 | -0.00096 | 0.002259 | |
RF2 | -0.00034 | 0.002712 | -0.00034 | 0.002716 | -0.00036 | 0.002724 | |
80, 90 | λ1 | 0.00467 | 0.003394 | -0.00915 | 0.003286 | 0.00454 | 0.003400 |
λ2 | 0.00465 | 0.002857 | -0.00793 | 0.002753 | 0.00475 | 0.002900 | |
R | 0.00025 | 0.001457 | 0.00088 | 0.001462 | 0.00035 | 0.001479 | |
RF1 | 0.00032 | 0.001266 | 0.00382 | 0.001296 | 0.00043 | 0.001280 | |
RF2 | 0.00015 | 0.001461 | 0.00187 | 0.001470 | 0.00026 | 0.001482 | |
100,110 | λ1 | 0.00709 | 0.002738 | -0.00456 | 0.002591 | 0.00702 | 0.002759 |
λ2 | 0.00432 | 0.002318 | -0.00647 | 0.002250 | 0.00435 | 0.002311 | |
R | -0.00120 | 0.001109 | -0.00079 | 0.001115 | -0.00113 | 0.001116 | |
RF1 | -0.00144 | 0.000968 | 0.00140 | 0.000980 | -0.00138 | 0.000976 | |
RF2 | -0.00143 | 0.001113 | -0.00011 | 0.001118 | -0.00136 | 0.001121 | |
150,120 | λ1 | 0.00495 | 0.001869 | -0.00344 | 0.001793 | 0.00502 | 0.001872 |
λ2 | 0.00500 | 0.002185 | -0.00504 | 0.002103 | 0.00476 | 0.002177 | |
R | -0.00012 | 0.000925 | -0.00095 | 0.000927 | -0.00026 | 0.000930 | |
RF1 | -0.00052 | 0.000753 | 0.00078 | 0.000756 | -0.00063 | 0.000757 | |
RF2 | -0.00034 | 0.000906 | -0.00041 | 0.000906 | -0.00048 | 0.000911 | |
200,150 | λ1 | 0.00143 | 0.001289 | -0.00518 | 0.001280 | 0.00146 | 0.001289 |
λ2 | -0.00026 | 0.001603 | -0.00862 | 0.001624 | -0.00023 | 0.001609 | |
R | -0.00101 | 0.000743 | -0.00190 | 0.000745 | -0.00101 | 0.000745 | |
RF1 | -0.00051 | 0.000586 | 0.00036 | 0.000587 | -0.00052 | 0.000586 | |
RF2 | -0.00091 | 0.000721 | -0.00118 | 0.000721 | -0.00091 | 0.000722 |
MLE | MPS | Bayesian | |||||
n,m | Bias | MSE | Bias | MSE | Bias | MSE | |
30, 20 | λ1 | 0.01722 | 0.009963 | -0.01153 | 0.008799 | 0.01663 | 0.009996 |
λ2 | 0.09455 | 0.209672 | -0.03808 | 0.178814 | 0.08884 | 0.200672 | |
R | -0.00244 | 0.002011 | -0.00378 | 0.002049 | -0.00251 | 0.001967 | |
RF1 | -0.00430 | 0.003224 | 0.00483 | 0.003249 | -0.00415 | 0.003192 | |
RF2 | -0.00377 | 0.002483 | -0.00125 | 0.002477 | -0.00377 | 0.002441 | |
50, 40 | λ1 | 0.00929 | 0.005053 | -0.01045 | 0.004713 | 0.00944 | 0.005122 |
λ2 | 0.02895 | 0.104113 | -0.06328 | 0.098289 | 0.02630 | 0.101853 | |
R | -0.00321 | 0.001175 | -0.00435 | 0.001196 | -0.00342 | 0.001176 | |
RF1 | -0.00357 | 0.001841 | 0.00262 | 0.001843 | -0.00374 | 0.001854 | |
RF2 | -0.00376 | 0.001436 | -0.00220 | 0.001430 | -0.00396 | 0.001442 | |
80, 90 | λ1 | 0.00908 | 0.003693 | -0.00466 | 0.003437 | 0.00905 | 0.003681 |
λ2 | 0.02371 | 0.046319 | -0.02677 | 0.044176 | 0.02369 | 0.046968 | |
R | -0.00193 | 0.000621 | -0.00159 | 0.000619 | -0.00193 | 0.000622 | |
RF1 | -0.00318 | 0.001212 | 0.00187 | 0.001202 | -0.00318 | 0.001210 | |
RF2 | -0.00268 | 0.000834 | -0.00057 | 0.000821 | -0.00269 | 0.000834 | |
100,110 | λ1 | 0.00456 | 0.002584 | -0.00704 | 0.002496 | 0.00453 | 0.002569 |
λ2 | 0.01642 | 0.034435 | -0.02646 | 0.033520 | 0.01529 | 0.034530 | |
R | -0.00092 | 0.000489 | -0.00064 | 0.000486 | -0.00102 | 0.000495 | |
RF1 | -0.00127 | 0.000913 | 0.00303 | 0.000916 | -0.00132 | 0.000915 | |
RF2 | -0.00124 | 0.000645 | 0.00055 | 0.000637 | -0.00132 | 0.000651 | |
150,120 | λ1 | 0.00318 | 0.001967 | -0.00528 | 0.001923 | 0.00302 | 0.001941 |
λ2 | 0.01613 | 0.035520 | -0.02435 | 0.034584 | 0.01556 | 0.035056 | |
R | -0.00057 | 0.000409 | -0.00110 | 0.000412 | -0.00056 | 0.000406 | |
RF1 | -0.00079 | 0.000700 | 0.00186 | 0.000704 | -0.00073 | 0.000694 | |
RF2 | -0.00079 | 0.000515 | -0.00016 | 0.000515 | -0.00076 | 0.000511 | |
200,150 | λ1 | 0.00140 | 0.001126 | -0.00524 | 0.001124 | 0.00142 | 0.001134 |
λ2 | 0.02209 | 0.028304 | -0.01175 | 0.027012 | 0.02163 | 0.028342 | |
R | 0.00057 | 0.000293 | 0.00001 | 0.000294 | 0.00053 | 0.000295 | |
RF1 | 0.00031 | 0.000442 | 0.00230 | 0.000448 | 0.00027 | 0.000445 | |
RF2 | 0.00042 | 0.000349 | 0.00079 | 0.000350 | 0.00038 | 0.000351 |
MLE | MPS | Bayesian | |||||
n,m | Bias | MSE | Bias | MSE | Bias | MSE | |
30, 20 | λ1 | 0.06763 | 0.155282 | -0.04768 | 0.137440 | 0.06555 | 0.156610 |
λ2 | 0.02046 | 0.011668 | -0.01262 | 0.010028 | 0.02030 | 0.011591 | |
R | 0.00402 | 0.001864 | 0.00273 | 0.001846 | 0.00420 | 0.001887 | |
RF1 | 0.00203 | 0.000444 | 0.00421 | 0.000483 | 0.00216 | 0.000452 | |
RF2 | 0.00298 | 0.001319 | 0.00439 | 0.001348 | 0.00317 | 0.001340 | |
50, 40 | λ1 | 0.04891 | 0.094691 | -0.02992 | 0.086752 | 0.04807 | 0.094583 |
λ2 | 0.01049 | 0.006881 | -0.01254 | 0.006335 | 0.01044 | 0.006899 | |
R | 0.00135 | 0.001199 | 0.00026 | 0.001189 | 0.00140 | 0.001200 | |
RF1 | 0.00076 | 0.000280 | 0.00215 | 0.000295 | 0.00079 | 0.000280 | |
RF2 | 0.00092 | 0.000848 | 0.00173 | 0.000856 | 0.00097 | 0.000847 | |
80, 90 | λ1 | 0.03999 | 0.054062 | -0.01579 | 0.050003 | 0.04016 | 0.053777 |
λ2 | 0.00592 | 0.002720 | -0.00667 | 0.002597 | 0.00561 | 0.002704 | |
R | -0.00002 | 0.000582 | 0.00040 | 0.000586 | -0.00013 | 0.000580 | |
RF1 | -0.00007 | 0.000147 | 0.00130 | 0.000155 | -0.00012 | 0.000147 | |
RF2 | -0.00027 | 0.000431 | 0.00116 | 0.000440 | -0.00035 | 0.000430 | |
100,110 | λ1 | 0.00977 | 0.042263 | -0.03626 | 0.041625 | 0.01012 | 0.042315 |
λ2 | 0.00263 | 0.002330 | -0.00810 | 0.002294 | 0.00291 | 0.002340 | |
R | 0.00112 | 0.000520 | 0.00137 | 0.000522 | 0.00118 | 0.000522 | |
RF1 | 0.00084 | 0.000130 | 0.00197 | 0.000137 | 0.00085 | 0.000131 | |
RF2 | 0.00107 | 0.000383 | 0.00219 | 0.000391 | 0.00111 | 0.000385 | |
150,120 | λ1 | 0.00724 | 0.028570 | -0.02618 | 0.028321 | 0.00687 | 0.028620 |
λ2 | 0.00732 | 0.002145 | -0.00283 | 0.002016 | 0.00731 | 0.002161 | |
R | 0.00236 | 0.000382 | 0.00182 | 0.000379 | 0.00240 | 0.000386 | |
RF1 | 0.00108 | 0.000090 | 0.00165 | 0.000093 | 0.00110 | 0.000091 | |
RF2 | 0.00184 | 0.000271 | 0.00214 | 0.000274 | 0.00188 | 0.000274 | |
200,150 | λ1 | 0.00595 | 0.020483 | -0.02065 | 0.020369 | 0.00619 | 0.020443 |
λ2 | 0.00547 | 0.001682 | -0.00295 | 0.001608 | 0.00545 | 0.001685 | |
R | 0.00171 | 0.000301 | 0.00116 | 0.000300 | 0.00168 | 0.000302 | |
RF1 | 0.00077 | 0.000068 | 0.00118 | 0.000070 | 0.00075 | 0.000068 | |
RF2 | 0.00133 | 0.000210 | 0.00148 | 0.000212 | 0.00130 | 0.000210 |
MLE | MPS | Bayesian | |||||
n, m | Bias | MSE | Bias | MSE | Bias | MSE | |
30, 20 | λ1 | 0.06224 | 0.176286 | -0.05263 | 0.157645 | 0.06459 | 0.177161 |
λ2 | 0.09727 | 0.203480 | -0.03537 | 0.172121 | 0.09340 | 0.199105 | |
R | 0.00357 | 0.004826 | 0.00162 | 0.004858 | 0.00292 | 0.004771 | |
RF1 | 0.00261 | 0.001646 | 0.00843 | 0.001815 | 0.00227 | 0.001636 | |
RF2 | 0.00246 | 0.004133 | 0.00686 | 0.004254 | 0.00188 | 0.004101 | |
50, 40 | λ1 | 0.03017 | 0.082788 | -0.04820 | 0.078270 | 0.03007 | 0.083022 |
λ2 | 0.06224 | 0.113595 | -0.03145 | 0.101059 | 0.06146 | 0.113486 | |
R | 0.00315 | 0.002556 | 0.00139 | 0.002559 | 0.00306 | 0.002556 | |
RF1 | 0.00181 | 0.000863 | 0.00564 | 0.000929 | 0.00178 | 0.000862 | |
RF2 | 0.00220 | 0.002158 | 0.00494 | 0.002204 | 0.00214 | 0.002157 | |
80, 90 | λ1 | 0.02923 | 0.051774 | -0.02658 | 0.048799 | 0.03008 | 0.052007 |
λ2 | 0.01866 | 0.047300 | -0.03152 | 0.045476 | 0.01861 | 0.047487 | |
R | -0.00118 | 0.001537 | -0.00050 | 0.001532 | -0.00129 | 0.001538 | |
RF1 | -0.00039 | 0.000552 | 0.00294 | 0.000576 | -0.00047 | 0.000553 | |
RF2 | -0.00131 | 0.001370 | 0.00200 | 0.001378 | -0.00142 | 0.001371 | |
100,110 | λ1 | 0.02007 | 0.043336 | -0.02620 | 0.041573 | 0.02040 | 0.043196 |
λ2 | 0.01477 | 0.035742 | -0.02818 | 0.034901 | 0.01559 | 0.035898 | |
R | -0.00043 | 0.001201 | -0.00003 | 0.001200 | -0.00038 | 0.001204 | |
RF1 | 0.00010 | 0.000455 | 0.00281 | 0.000473 | 0.00010 | 0.000455 | |
RF2 | -0.00048 | 0.001100 | 0.00215 | 0.001108 | -0.00046 | 0.001101 | |
150,120 | λ1 | 0.02229 | 0.027613 | -0.01152 | 0.026372 | 0.02199 | 0.027322 |
λ2 | 0.01364 | 0.036194 | -0.02658 | 0.035347 | 0.01402 | 0.036164 | |
R | -0.00135 | 0.000946 | -0.00218 | 0.000952 | -0.00127 | 0.000944 | |
RF1 | -0.00084 | 0.000300 | 0.00075 | 0.000305 | -0.00080 | 0.000298 | |
RF2 | -0.00157 | 0.000781 | -0.00045 | 0.000783 | -0.00151 | 0.000778 | |
200,150 | λ1 | 0.01240 | 0.020863 | -0.01433 | 0.020372 | 0.01221 | 0.020684 |
λ2 | 0.01492 | 0.025875 | -0.01865 | 0.025209 | 0.01504 | 0.025844 | |
R | 0.00016 | 0.000705 | -0.00069 | 0.000707 | 0.00020 | 0.000703 | |
RF1 | 0.00002 | 0.000232 | 0.00122 | 0.000237 | 0.00004 | 0.000231 | |
RF2 | -0.00011 | 0.000594 | 0.00064 | 0.000597 | -0.00007 | 0.000592 |
Data Ⅰ | 0.19 | 0.78 | 0.96 | 1.31 | 2.78 | 3.16 | 4.15 | 4.67 | 4.85 | 6.5 |
7.35 | 8.01 | 8.27 | 12.06 | 31.75 | 32.52 | 33.91 | 36.71 | 72.89 | - | |
Data Ⅱ | 0.35 | 0.59 | 0.96 | 0.99 | 1.69 | 1.97 | 2.07 | 2.58 | 2.71 | 2.9 |
3.67 | 3.99 | 5.35 | 13.77 | 25.50 | - | - | - | - | - |
λ1 | λ2 | R | RF1 | RF2 | |
MLE | 0.60363 | 1.02982 | 0.63046 | 0.39315 | 0.56254 |
MPS | 0.55815 | 0.89220 | 0.61516 | 0.39480 | 0.55339 |
Bayesian | 0.64494 | 1.12664 | 0.63595 | 0.38661 | 0.56330 |