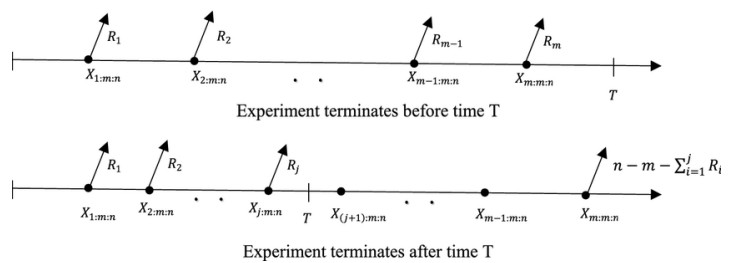
The Power Rayleigh distribution (PRD) is a new extension of the standard one-parameter Rayleigh distribution. To employ this distribution as a life model in the analysis of reliability and survival data, we focused on the statistical inference for the parameters of the PRD under the adaptive Type-II censored scheme. Point and interval estimates for the model parameters and the corresponding reliability function at a given time are obtained using likelihood, Bootstrap and Bayesian estimation methods. A simulation study is conducted in different settings of the life testing experiment to compare and evaluate the performance of the estimates obtained. In addition, the estimation procedure is also investigated in real lifetimes data. The results indicated that the obtained estimates gave an accurate and efficient estimation of the model parameters. The Bootstrap estimates are better than the estimates obtained by the likelihood estimation approach, and estimates obtained using the Markov Chain Monte Carlo method by the Bayesian approach under both the squared error and the general entropy loss functions have priority over other point and interval estimates. Under the adaptive Type-II censoring scheme, concluding results confirmed that the PRD can be effectively used to model the lifetimes in survival and reliability analysis.
Citation: Hatim Solayman Migdadi, Nesreen M. Al-Olaimat, Maryam Mohiuddin, Omar Meqdadi. Statistical inference for the Power Rayleigh distribution based on adaptive progressive Type-II censored data[J]. AIMS Mathematics, 2023, 8(10): 22553-22576. doi: 10.3934/math.20231149
[1] | Bing Long, Zaifu Jiang . Estimation and prediction for two-parameter Pareto distribution based on progressively double Type-II hybrid censored data. AIMS Mathematics, 2023, 8(7): 15332-15351. doi: 10.3934/math.2023784 |
[2] | Samah M. Ahmed, Abdelfattah Mustafa . Estimation of the coefficients of variation for inverse power Lomax distribution. AIMS Mathematics, 2024, 9(12): 33423-33441. doi: 10.3934/math.20241595 |
[3] | Mustafa M. Hasaballah, Oluwafemi Samson Balogun, M. E. Bakr . Frequentist and Bayesian approach for the generalized logistic lifetime model with applications to air-conditioning system failure times under joint progressive censoring data. AIMS Mathematics, 2024, 9(10): 29346-29369. doi: 10.3934/math.20241422 |
[4] | Hassan Okasha, Mazen Nassar, Saeed A. Dobbah . E-Bayesian estimation of Burr Type XII model based on adaptive Type-Ⅱ progressive hybrid censored data. AIMS Mathematics, 2021, 6(4): 4173-4196. doi: 10.3934/math.2021247 |
[5] | Ahmed R. El-Saeed, Ahmed T. Ramadan, Najwan Alsadat, Hanan Alohali, Ahlam H. Tolba . Analysis of progressive Type-Ⅱ censoring schemes for generalized power unit half-logistic geometric distribution. AIMS Mathematics, 2023, 8(12): 30846-30874. doi: 10.3934/math.20231577 |
[6] | Hanan Haj Ahmad, Ehab M. Almetwally, Dina A. Ramadan . A comparative inference on reliability estimation for a multi-component stress-strength model under power Lomax distribution with applications. AIMS Mathematics, 2022, 7(10): 18050-18079. doi: 10.3934/math.2022994 |
[7] | Young Eun Jeon, Suk-Bok Kang, Jung-In Seo . Pivotal-based inference for a Pareto distribution under the adaptive progressive Type-II censoring scheme. AIMS Mathematics, 2024, 9(3): 6041-6059. doi: 10.3934/math.2024295 |
[8] | Naif Alotaibi, A. S. Al-Moisheer, Ibrahim Elbatal, Salem A. Alyami, Ahmed M. Gemeay, Ehab M. Almetwally . Bivariate step-stress accelerated life test for a new three-parameter model under progressive censored schemes with application in medical. AIMS Mathematics, 2024, 9(2): 3521-3558. doi: 10.3934/math.2024173 |
[9] | Xue Hu, Haiping Ren . Statistical inference of the stress-strength reliability for inverse Weibull distribution under an adaptive progressive type-Ⅱ censored sample. AIMS Mathematics, 2023, 8(12): 28465-28487. doi: 10.3934/math.20231457 |
[10] | A. M. Abd El-Raheem, Ehab M. Almetwally, M. S. Mohamed, E. H. Hafez . Accelerated life tests for modified Kies exponential lifetime distribution: binomial removal, transformers turn insulation application and numerical results. AIMS Mathematics, 2021, 6(5): 5222-5255. doi: 10.3934/math.2021310 |
The Power Rayleigh distribution (PRD) is a new extension of the standard one-parameter Rayleigh distribution. To employ this distribution as a life model in the analysis of reliability and survival data, we focused on the statistical inference for the parameters of the PRD under the adaptive Type-II censored scheme. Point and interval estimates for the model parameters and the corresponding reliability function at a given time are obtained using likelihood, Bootstrap and Bayesian estimation methods. A simulation study is conducted in different settings of the life testing experiment to compare and evaluate the performance of the estimates obtained. In addition, the estimation procedure is also investigated in real lifetimes data. The results indicated that the obtained estimates gave an accurate and efficient estimation of the model parameters. The Bootstrap estimates are better than the estimates obtained by the likelihood estimation approach, and estimates obtained using the Markov Chain Monte Carlo method by the Bayesian approach under both the squared error and the general entropy loss functions have priority over other point and interval estimates. Under the adaptive Type-II censoring scheme, concluding results confirmed that the PRD can be effectively used to model the lifetimes in survival and reliability analysis.
In the analysis of survival and reliability data, different engineering and medical applications arise. Due to the limitations of cost and time, life-testing experiments are frequently performed under censoring schemes. In the literature contexts, Type-I and Type-II censoring schemes are initially used. In Type-I censoring, the life testing experiment is terminated at a pre-determined time T and the total number of failures is considered a random variable, while in Type-II censoring, the total number of failures is pre-fixed and in this case, the termination time is considered a random variable. The weakness of the traditional Type–I and Type-II censoring schemes is that they do not have the flexibility to remove some of the surviving units at different stages of the life-testing experiment. Recently, a generalization of Type-II censoring scheme called progressive Type-II censoring is the most commonly used. Suppose that n units are put on the life test at time zero for failure and the experimenter initially specified the number m of units that will be failed during the life test with the proposed censored scheme: R=(R1,R2,...,Rm) of random removals. The mechanism to perform the life test under the progressive Type-II censoring scheme is carried out as follows: At the time of the first failure, x1:m:n, R1 of the remaining n−1 surviving units are randomly removed from the test. Continuing on, at the second failure time x2:m:n, R2 of the remaining n−R1−1 surviving units are randomly removed from the test and so on. This experiment is terminated at the time xm:m:n of the mth failure and at this time, the remaining n−m−∑ni=m+1Ri surviving units are all removed.
Balakrishnan [1] and Aggarwala and Balakrishnan [2] have introduced the concept of Type-II progressive censoring and an algorithm to simulate progressively Type-II censored samples from any continuous distribution. Recent statistical inferences for many lifetime distributions are also included in [3,4,5,6,7,8,9,10].
In many real practical settings, experimenters need to control the termination time of the life test and to change the number of removed units during the test. For these reasons, Ng et al. [11] have proposed the adaptive progressive Type-II censoring scheme (APT-II). In this scheme, the expected value of the ideal time T>θ for terminating the life test is initially determined. Now, if (xi:m:n,i=1,2,…,m) are the m completely observed failure times and(Ri,i=1,2,…,m) are the removed units at the times xi:m:n,i=1,2,…,m, respectively. APT-II censoring scheme is carried out as follows: if xm:m:n<T, the test is terminated at xm:m:n and we will have the standard progressive Type-II censoring, in this case: Rm=n−m−∑mi=1Ri, otherwise, if xj:m:n<T<xj+1:m:n, where j+1<m and xj:m:n is the jth failure before the ideal time T, then any surviving units will be removed from the test by placing Rj+1=Rj+2=…=Rm=0, and at the time xm:m:n all the remaining units are removed, in this case: Rm=n−m−∑ji=1Ri. This scheme provides a design in which the experimenter is assured of getting m observed failure times for efficiency of statistical inference and the total test time will be closest to the ideal time T. Figure 1 illustrates a schematic presentation of the life testing experiment based on the APT-II censoring scheme.
Many authors have considered classical and Bayesian estimations for the parameters of different distributions based on APT-II. Examples of many others, under the APT-II censoring scheme, are: Chen and Gui [12] discussed the estimation problem of a lifetime distribution with a bathtub-shaped failure rate function, Abu-Moussa et al. [13] discussed the maximum likelihood estimation for the Gompertz distribution. Mahmoud et al. [14] studied the Bayes estimation of the Pareto distribution. Asadi et al. [15] investigated the accelerated life test in Gompertz distribution for the evaluation of virus-containing micro-droplets data. AL Alotaibi et al. [16] considered the estimation of parameters of the XLindley distribution, Almalki et al. [17] have explored the partially constant-stress accelerated life tests model for estimation of the parameters of the Kumaraswamy distribution and Ahmad et al. [18] adopted both Bayesian and non-Bayesian inference for the exponentiated power Lindley distribution.
Using the power transformation method (see Shukala and Shanker [19]), Bhat and Ahmad [20] introduced a new form of Rayleigh distribution called the Power Rayleigh distribution (PRD) with probability density (PDF), cumulative distribution (CDF) and the reliability functions given, respectively, by:
f(x;θ,β)=βθ2x2β−1e−x2β2θ2;x>0,θ,β>0, | (1.1) |
F(x;θ,β)=1−e−x2β2θ2;x>0,θ,β>0, | (1.2) |
R(x;θ,β)=e−x2β2θ2;x>0,θ,β>0. | (1.3) |
The hazard rate function (HRF) of the PRD is given by
h(x;θ,β)=βθ2x2β−1;x>0,θ,β>0. | (1.4) |
As a generalization of the standard Rayleigh distribution, which can be used in modeling the lifetimes of many pieces of equipment like resistors, transformers and capacitors (see Kundu and Raqab [21] and Ateeq et al. [22]), the hazard rate function of PRD is constant when β=0.5, increasing when β<0.5 and decreasing when β>0.5. For this flexibility, PRD can be utilized for more reliability and survival data analysis. Particularly, as shown by Bhat and Ahmad [20], this new distribution has superiority for modeling the stress and strength data. The PDF and the HRF of the PRD with different combinations of (θ,β) are presented in Figure 2.
Under the APT-II censoring scheme. Many authors have adopted statistical inference for the Rayleigh distribution and some of its generalizations. Recent studies were completed by Mahmoud and Ghazal [23] for the Exponentiated Rayleigh distribution, Almetwally et al. [24] for the Generalized Rayleigh distribution, Panahi and Moradi [25], Gao and Gao [26] and Fan and Gui [27] for the Inverted Exponentiated Rayleigh distribution. To the best of our knowledge, there exists no statistical inference about the parameters of the PRD based on any censoring scheme. The aim of this paper is to focus on classical and Bayesian point and interval estimation of the unknown parameters of the PRD using the APT-II censored data. This will extend the application of PRD as a life model to include more reliability and survival data.
The rest of this paper is organized as follows: Section 2 is devoted to point and interval estimation using the likelihood approach. Bayesian estimation under the squared error (SE) and the general entropy (GE) loss functions is explored in Section 3. In Section 4, both point and interval estimates are presented and the Bootstrap sampling method is also implemented. To evaluate the performance of the obtained point and interval estimates, a Monte Carlo simulation study is conducted in Section 5. Section 6 illustrates the application of the developed estimation procedure to a set of real-life data. Finally, a conclusion about this work is summarized in Section 7.
Based on an APT-II observed censored sampled data from a continuous distribution with PDF f(x;φ) and CDF F(x;φ), Ng et al. [11] derived the likelihood function for a vector of parameter φ in the form:
l(φ )=C m∏i=1f(xi;φ) [j∏i=1(1−F(xi;φ))Ri ](1−F(xm;φ))cj, | (2.1) |
where: xi=xi,m,n cj=n−m−∑ji=1Ri and C is a constant that is independent of the parameters φ. Substituting the corresponding formulas for f(xi;θ,β), F(xi;θ,β) of PRD from Eqs (1.1) and (1.2) in Eq (2.1). This implies that the corresponding likelihood function of the parameters θ and β using APT-II data is given by:
l(θ, β )∝βmθ2m (m∏i=1( xi))2β−1(e−12θ2(m∑i=1x2βi+j∑i=1Rix2βi+cjx2βm)). | (2.2) |
Therefore, the natural logarithmic function of the likelihood function is
ln(l(θ, β ))∝mln(βθ2)+(2β−1)m∑i=1ln(xi)−12θ2(m∑i=1x2βi+j∑i=1Rix2βi+cjx2βm). | (2.3) |
Differentiating both sides of Eq (2.2) with respect to θ and β and equating the derived equations to zero, we get the likelihood equations:
∂ln(l(θ, β))∂θ=−2mθ+1θ3(m∑i=1x2βi+j∑i=1Rix2βi+cjx2βm)=0. | (2.4) |
∂ln(l(θ, β))∂β=mβ+2m∑i=1ln(xi)−1θ2(m∑i=1ln(xi)x2βi+j∑i=1Riln(xi)x2βi+cjln(xm)x2βm)=0. | (2.5) |
When the shape parameter β is known, solving Eq (2.4) with respect to θ, the maximum likelihood estimate (MLE) of θ exists in an explicit form given by
θmle=√∑mi=1x2βi+∑ji=1Rix2βi+cjx2βm2m. | (2.6) |
When the two parameters are both unknown, numerical methods should be used to solve the system of the nonlinear equations in Eqs (2.4) and (2.5). In this paper, a multivariate Newton-Raphson numerical method is implemented to obtain the approximated values for the MLEs (ˆθ, ˆβ) of θ and β. Utilizing the invariance property of the MLEs, the MLE of the reliability function at a given time t is given by:
ˆR(t)=e−t2ˆβ2ˆθ2 ,t>0. |
Under the APT-II censoring scheme, another desired statistical inference for the PRD is to find confidence interval estimates for the unknown parameters θ and β. It is mathematically difficult to construct exact confidence intervals because the distributions of the MLEs of θ and β are not in closed forms. The observed Fisher information matrix of (θ, β) can also be used to find approximated confidence intervals for the two unknown parameters (see Lawless [28]) which are defined as:
I(ˆθ,ˆβ)=[−∂2ln(l(θ, β))∂θ2−∂2ln(l(θ, β))∂θ∂β−∂2ln(l(θ, β))∂β∂θ−∂2ln(l(θ, β))∂β2](ˆθmle,ˆβmle), | (2.7) |
where:
∂2ln(l(θ, β))∂θ2=2mθ2−3θ4(m∑i=1x2βi+j∑i=1Rix2βi+cjx2βm), |
∂2ln(l(θ, β))∂β2=−mθ2−2θ2(m∑i=1(ln(xi))2x2βi+j∑i=1Ri(ln(xi))2x2βi+cj(ln(xm))2x2βm), |
∂2ln(l(θ, β))∂θ∂β=∂2ln(l(θ, β))∂β∂θ=−6θ4 (m∑i=1ln(xi)x2βi+j∑i=1Riln(xi)x2βi+cjln(xm)x2βm). |
Hence, the approximated variance-covariance matrix of (ˆθ, ˆβ) is simplified by
ˆv=[ˆv11ˆv12ˆv21ˆv22]=I−1(ˆθ,ˆβ). | (2.8) |
Consequently, based on the large sample theory of the MLEs, the asymptotic 100(1−α)% confidence intervals (ACIs) for θ and β are constructed respectively as:
ˆθ±zα2√ˆv11, ˆβ±zα2√ˆv22, |
where zα2 is the (1−α)% quantile of the standard Normal distribution, and ˆv11, ˆv22 are the diagonal elements of ˆv. Accordingly, the approximate 100(1−α)% confidence intervals for the reliability function at a given time t is
ˆR(t)±zα2e−t2√ˆv112ˆv22 . |
In statistical inference, the Bayesian estimation approach is a complement part to the conventional likelihood estimation approach. To apply the estimation procedures using the Bayesian approach, prior information of the parameters θ and β should be determined first. When no previous information is available about these parameters, it is convenient to specify their priors in such a way that their joint prior is proportional to their likelihood function. In this research, the joint prior distribution of the parameters θ and θ is proposed to take the form:
π(θ,β)=π1(θ)π2(β|θ), | (3.1) |
where π1(θ) is the Inverse Rayleigh distribution with scale parameter γ and π2(β|θ) is the Exponential distribution with scale parameter (λθ2) with prior densities given, respectively, by:
π1(θ)=2γ2θ3e−γ2θ2 , γ>0, | (3.2) |
π2(β|θ)=λθ2e−λβθ2 ,λ>0. | (3.3) |
Substituting for π1(θ) and π2(β|θ) from Eqs (3.2) and (3.3) in Eq (3.1), the joint prior of θ and β has the form
π(θ,β)=2λγ2θ5 e−(γ2+λβ)θ2 ,γ>0,λ>0. | (3.4) |
Now, combining Eq (2.1) and (3.3), the posterior functions of θ and β is:
π∗(θ,β|x)=λγ2βmθ2m+5 (∏mi=1( xi))2β−1 e−(u2+γ2+λβ)θ2∫∞0∫∞0λγ2βmθ2m+5 (∏mi=1( xi))2β−1 e−(u2+γ2+λβ)θ2∂θ∂β, | (3.5) |
where: u=∑mi=1x2βi+∑ji=1Rix2βi+cjx2βm. Clearly, the joint prior given in Eq (3.4) is proportional to the posterior function of (θ,β) in Eq (3.5) and thus, it can be considered a conjugate prior. The conditional posteriors of θ and β can be obtained, respectively, as:
π∗1(θ|β,x)=∫∞0π∗(θ,β|x)∂β, | (3.6) |
π∗2(β|θ,x)=∫∞0π∗(θ,β|x)∂θ. | (3.7) |
When the shape parameter β is known (As in the case of the standard Rayleigh distribution). The posterior function π∗(θ,β|x) given in Eq (3.4) becomes a function of θ only given by
π∗(θ|β,x)= θ−(2m+5) e−(u2+γ2+λβ)θ2∫∞0θ−(2m+5)e−(u2+γ2+λβ)θ2∂θ. | (3.8) |
Consequently, the Bayes point estimate of θ under the squared error loss function (SE) is obtained as the mean of the posterior, which is given by:
θ1S=∫∞0θπ∗(θ|β,x)∂θ=Γ(m+1.5)Γ(m+2)√u2+γ2+λβ. | (3.9) |
Similarly, the Bayes point estimate of θ under the general entropy loss function (GE) indexed with parameter α>0 is obtained as:
θ1G=(∫∞0θ−aπ∗(θ|β,x)∂θ)−1a=(Γ(m+2)Γ(m+0.5a+2))1a√u2+γ2+λβ. | (3.10) |
When the shape parameter is known, another common Bayesian inference is to find a credible interval in the form [ll,lu] for the parameters θ. The (1−α)% credible interval for the parameter θ can be obtained as
∫lullπ∗(θ|β,x)∂θ=(1−α)100%. | (3.11) |
Substituting for π∗(θ|β,x) from Eq (3.5) in Eq (3.10) and simplifying the integral in the above equation, the lower and the upper bounds of the (1−α)% credible interval for θ can be obtained from the following equation:
Ig((zll)2,m+2)−Ig((zlu)2,m+2)=(1−α)%, | (3.12) |
where: z=(u2+γ2+λβ),andIg(x,y)=1Γ(y)∫x0ty−1e−t∂t is the incomplete Gamma function.
In addition to the constraint Eq (3.12), a (1−α)% highest posterior credible intervals (HPD) credible interval for θ can also be obtained by adding the constraint:
(lllu)−(2m+5)=e−z(1l2u−1l2l). | (3.13) |
When the parameters θ and β are both unknown, it is difficult to obtain explicit forms of their Bayes point estimates under SE or GE loss functions because, in this case, the corresponding integrals:
∫∞0θaπ∗(θ,β|x)∂θ, ∫∞0βaπ∗(θ,β|x)∂θ |
cannot be found in closed forms for any number α∈R.
To overcome this problem, the Markov Chain Monte Carlo (MCMC) method can be used to approximate the above integrals. In this paper, we use the common type of MCMC, which is called the Metropolis-Hasting (M-H) developed by Metropolis et al. [29] and extended by Hasting [30] with Gibbs sampling to find Bayes point and interval estimates for the parameters θ and β. Assuming normal is the target distribution, the procedure of implementing the MCMC is performed through the following steps:
Step 1. Set the initial values of θ and β, (θ(0),β(0)).
Step 2. Put j = 1.
Step 3. Simulate θ∗, β∗ from the normal distributions N(θ(j−1),ˆv11), N(β(j−1),ˆv22) respectively, where ˆv11 and ˆv22 are the diagonal elements of the variance-covariance matrix computed given the values of θ(j−1),β(j−1).
Step 4. Compute the acceptance ratios:
rθ∗=min[1,π∗1(θ∗|β(j−1),x)π∗1(θ(j−1)|β(j−1),x)],rβ∗=min[ 1,π∗2(β∗|θ(j−1),x)π∗2(β(j−1)|θ(j−1),x)]. |
Step 5. Generate u1, u2 from the uniform distribution U(0,1).
Step 6. If u1≤rθ∗, u2≤rβ∗, set: θ(j)=θ∗,β(j)=β∗, else: θ(j)=θ(j−1),β(j)=β(j−1).
Step 7. Use the generated sample to obtain an estimate of the reliability function: R(j)(t).
Step 8. Set j=j+1.
Step 9. Repeat Step 3- Step 8 N times to have (θ(1),θ(2),…,θ(N)),(β(1),β(2),…,β(N)) Then the Bayesian estimates of θ and β under SE are given, respectively, by
ˆθS=1N−MN∑j=M+1θ(j),ˆβS=1N−MN∑j=M+1β(j). |
And under the GE, the Bayesian estimates of θ and β are given, respectively, by
ˆθG=(1N−MN∑j=M+1(θ(j))−α)−1α,ˆβG=(1N−MN∑j=M+1(β(j))−α)−1α, |
where α is the parameter of the GE function and M is the number of iterations before the stationary distribution is achieved (the burn in-period) from N (the total number of iterations).
Based on the (M-H) algorithm, the Bayesian estimations of the reliability function under SE and GE at a given time t are:
ˆR(t)S=1N−MN∑j=M+1R(j)(t),ˆR(t)G=(1N−MN∑j=M+1R(j)(t))−α)−1α. |
Ascending order the values of θ(i), β(i) and R(i)(t), i=M+1,…,N generated by the M-H algorithm. The 100(1−α)% symmetric two-sided credible intervals of θ, β and the reliability function R(t) are given, respectively, by:
(θ(N−M)α2,θ(N−M)(1−α2)),(β(N−M)α2,β(N−M)(1−α2)),(R∗(t)(N−M)α2,R∗(t)(N−M)(1−α2)). |
Using the method of Chen and Shao [31]. The symmetric 100(1−α)% two sided HPD credible intervals of θ, β and the reliability function R(t) are given, respectively, by:
(θ(i∗),θ(i∗+(1−α)(N−M))),(β(i∗),β(i∗+(1−α)(N−M))),(R∗(t)(i∗),R∗(t)(i∗+(1−α)(N−M))), |
where R∗(t) is the estimated R(t) by the (M-H) algorithm and i∗ is chosen such that:
θ(i∗+[(1−α)(N−M)])−θ(i∗)=min1≤i≤α(N−M){θ(i+(1−α)(N−M))−θ(i)}, |
β(i∗+[(1−α)(N−M)])−β(i∗)=min1≤i≤α(N−M){β(i+(1−α)(N−M))−β(i)}, |
R∗(t)(i∗+[(1−α)(N−M)])−R∗(t)(i∗)=min1≤i≤α(N−M){R∗(t)(i+(1−α)(N−M))−R∗(t)(i)}, |
where [y] denotes the greatest integer less than or equal y.
One common method to estimate the parameters of the underlying distribution is the Bootstrap resampling technique. This method gives better estimates of the parameters than the approximate Bayes and likelihood estimates when the effective sample m is small enough. Details about the Bootstrap estimation method are explored in Hall [32] and Efron and Tibshirani [33]. The procedure to obtain point and interval estimates for the parameters θ and β by this method is described in the following steps:
Step 1. Based on the specified values of n, m, T, R, ncompute ˆθmle and ˆβmle.
Step 2. Using ˆθmle and ˆβmle generate a Bootstrap resample.
Step 3. Calculate the Bootstrap estimates ˆθ1p and ˆβ1p.
Step 4. Repeat the steps 2 and 3 up to k times to have ˆθ1p,ˆθ2p,…,ˆθkp,ˆβ1p,ˆβ2p,…,ˆβkp.
Step 5. Use the generated sample to obtain an estimate of the reliability function: R(t)(1)p,R(t)(2)p,…, R(t)(k)p.
Step 6. Compute the Bootstrap point estimates
ˆθp=1kk∑i=1ˆθip, ˆβp=1kk∑i=1ˆβip, ˆR(t)p=1kk∑i=1R(t)(i)p. |
Step 7. Rearrange the Bootstrap estimates in ascending order to have 100(1−α)% percentile confidence intervals for θ, β and the reliability function R(t), respectively, as:
(θ[kα2]p,θ[k(1−α2)]p),(β[kα2]p,β[k(1−α2)]p),(ˆR(t)[kα2]p,ˆR(t)[k(1−α2)]p). |
We evaluate the performance of the point and interval estimates obtained in the previous sections. Thus, Monte Carlo simulations are performed by generating 1000 APT-II samples from the PRD with different settings of sample size, affective sample size, true parameter values and ideal termination time with the following censoring schemes:
SC.I:R1=n−m,Ri=0 for i≠1, |
SC.II:Rm=n−m,Ri=0 for i≠m, |
SC.III:Rm2=n−m,Ri=0 for i≠m2. |
SC.I presents left censoring at the beginning of the experiment, SC.II presents ordinary Type-II censoring and SC.III presents progressive APT-II at the expected mid-termination time of the experiment.
The simulation process is implemented through the following steps:
1) Determine the values of n, m, R, T and set the true parameter values (θ, β).
2) Generate a random sample of size n from the uniform distribution and apply the Balakrishnan and Sandho [34] algorithm to generate a random sample of size m from the PRD using the relation:
x=(2θ2lin(1−u)−1)12β. |
3) Determine the value of j and remove all observations greater than xj.
4) Generate (m − j − 1) Type-II censored sample from [f(xj)1 − F(xj+1)] where f(x) and F(x) are the PDF and the CDF of the PRD, and stop the experiment at xm.
5) Obtain point and interval estimates of the parameters θ, β and the reliability function at time t=1.5.
6) Repeat steps 2–5 and compute the average absolute biases (ABs), the mean squared errors (MSEs) of the point estimates, the average lengths (ALs) and the coverage probabilities (CPs) of the interval estimates.
For the case where the shape parameter β is assumed to be known, the true parameter values are taken to be (θ=1, β=0.8), the values of n and m are determined such that:
(n,m)={(40,15),(40,20),(60,20),(60,40)}, |
with the ideal terminations T=1 and T=2.
When both parameters are assumed to be unknown, the true parameter values are considered to be {(θ,β)=(2,0.4) and (2,0.8)} with ideal termination times (T=200 for (θ=2,β=0.4)) and (T=20 for (θ=2,β=0.8)), the sample sizes are proposed to be n=80, n = 50 and the effective sample size m is indicated such that the proportion of observed failures r=(mn) are: 0.80, 0.60 and 0.40.
In the Bayesian estimation, the values of the hyperparameters (γ, λ) are chosen in a way such that the prior mean is equal to the expected value of the unknown parameter; see Kundu [35]. The shape parameter of the GE loss is fixed to be equal to α=1.1. Bayesian point estimates and associated credible intervals are computed by generating 12000 samples using the M-H algorithm and discarding the first 2000 corresponding values of (θ, β) as burn-in periods. Simulation results are listed in Tables 1–7. Tables 1–7 manifest the following conclusions:
MLE | Boot | Bayes-SE | Bayes-GE | ||||||
(n, m) | sc | AB | MSE | AB | MSE | AB | MSE | AB | MSE |
T= 1 | |||||||||
(40, 15) | I | 0.2741 | 0.1801 | 0.2283 | 0.1334 | 0.2321 | 0.1201 | 0.2172 | 0.1183 |
II | 0.1918 | 0.1310 | 0.1403 | 0.1078 | 0.1306 | 0.1042 | 0.1300 | 0.1026 | |
III | 0.2631 | 0.1655 | 0.2202 | 0.1263 | 0.2019 | 0.1184 | 0.1987 | 0.1109 | |
(40, 20) | I | 0.2543 | 0.1415 | 0.2142 | 0.1278 | 0.2115 | 0.1106 | 0.2064 | 0.1095 |
II | 0.1831 | 0.1296 | 0.1392 | 0.1001 | 0.1295 | 0.0995 | 0.1143 | 0.0912 | |
III | 0.2428 | 0.1401 | 0.2137 | 0.1206 | 0.2001 | 0.1100 | 0.1988 | 0.1066 | |
(60, 20) | I | 0.1778 | 0.0982 | 0.1587 | 0.0943 | 0.1213 | 0.0864 | 0.1171 | 0.0776 |
II | 0.1412 | 0.0876 | 0.1116 | 0.0851 | 0.1017 | 0.0811 | 0.0958 | 0.0658 | |
III | 0.1711 | 0.0986 | 0.1521 | 0.0952 | 0.1201 | 0.0843 | 0.1104 | 0.1104 | |
(60, 40) | I | 0.1471 | 0.0978 | 0.1332 | 0.0924 | 0.1132 | 0.0802 | 0.1089 | 0.0704 |
II | 0.1213 | 0.0774 | 0.1103 | 0.0742 | 0.1012 | 0.0763 | 0.0933 | 0.0643 | |
III | 0.1441 | 0.0976 | 0.1321 | 0.0902 | 0.1123 | 0.0792 | 0.1118 | 0.0703 | |
T=2 | |||||||||
(40, 15) | I | 0.2077 | 0.1106 | 0.1821 | 0.0817 | 0.1631 | 0.0763 | 0.1022 | 0.0632 |
II | 0.1309 | 0.0958 | 0.1122 | 0.0784 | 0.0997 | 0.0743 | 0.0881 | 0.0639 | |
III | 0.2042 | 0.1057 | 0.1678 | 0.0806 | 0.1578 | 0.0773 | 0.1101 | 0.0655 | |
(40, 20) | I | 0.1876 | 0.0888 | 0.1579 | 0.0781 | 0.1532 | 0.0697 | 0.1003 | 0.0615 |
II | 0.1232 | 0.0803 | 0.1054 | 0.0731 | 0.0943 | 0.0699 | 0.0805 | 00629 | |
III | 0.1774 | 0.0816 | 0.1476 | 0.0775 | 0.1460 | 0.0691 | 0.1022 | 0.0637 | |
(60, 20) | I | 0.1544 | 0.0767 | 0.1353 | 0.0728 | 0.0979 | 0.0649 | 0.0925 | 0.0608 |
II | 0.1178 | 0.0661 | 0.0882 | 0.0636 | 0.0783 | 0.0596 | 0.0766 | 0.0588 | |
III | 0.1477 | 0.0771 | 0.1287 | 0.0737 | 0.0967 | 0.0628 | 0.0931 | 0.0622 | |
(60, 40) | I | 0.1237 | 0.0763 | 0.1098 | 0.0709 | 0.0898 | 0.0587 | 0.0832 | 0.0580 |
II | 0.0979 | 0.0559 | 0.0869 | 0.0527 | 0.0778 | 0.0548 | 0.0751 | 0.0510 | |
III | 0.1207 | 0.0761 | 0.1087 | 0.0687 | 0.0889 | 0.0577 | 0.0812 | 0. |
Asymptotic | Bootstrap | Credible | HPD Credible | ||||||
(n, m) | sc | AL | CP | AL | CP | AL | CP | AL | CP |
T= 1 | |||||||||
(40, 15) | I | 0.2107 | 0.9684 | 0.2006 | 0.9624 | 0.1906 | 0.9618 | 0.1892 | 0.9541 |
II | 0.1978 | 0.9634 | 0.1917 | 0.9593 | 0.1893 | 0.9582 | 0.1831 | 0.9516 | |
I | 0.1764 | 0.9581 | 0.1723 | 0.9580 | 0.1702 | 0.9567 | 0.1694 | 0.9508 | |
(40, 20) | I | 0.2064 | 0.9625 | 0.1901 | 0.9624 | 0.1876 | 0.9610 | 0.1807 | 0.9538 |
II | 0.1908 | 0.9612 | 0.1829 | 0.9584 | 0.1751 | 0.9564 | 0.1688 | 0.9512 | |
I | 0.1732 | 0.9572 | 0.1705 | 0.9579 | 0.1693 | 0.9541 | 0.1604 | 0.9506 | |
(60, 20) | I | 0.1902 | 0.9615 | 0.1823 | 0.9619 | 0.1811 | 0.9540 | 0.1773 | 0.9533 |
II | 0.1764 | 0.9545 | 0.1631 | 0.9546 | 0.1605 | 0.9519 | 0.1587 | 0.9507 | |
I | 0.1578 | 0.9550 | 0.1509 | 0.9570 | 0.1491 | 0.9508 | 0.1432 | 0.9501 | |
(60, 40) | I | 0.1812 | 0.9614 | 0.1720 | 0.9604 | 0.1704 | 0.9536 | 0.1701 | 0.9512 |
II | 0.1721 | 0.9601 | 0.1584 | 0.9531 | 0.1515 | 0.9511 | 0.1508 | 0.9503 | |
I | 0.1354 | 0.9544 | 0.1417 | 0.9514 | 0.1401 | 0.9502 | 0.1372 | 0.9492 | |
T=2 | |||||||||
(40, 15) | I | 0.1859 | 0.9512 | 0.1658 | 0.9452 | 0.1859 | 0.9446 | 0.1698 | 0.9431 |
II | 0.1730 | 0.9462 | 0.1645 | 0.9421 | 0.1733 | 0.9412 | 0.1496 | 0.9410 | |
I | 0.1516 | 0.9409 | 0.1454 | 0.9408 | 0.1516 | 0.9395 | 0.1321 | 0.9352 | |
(40, 20) | I | 0.1816 | 0.9453 | 0.1628 | 0.9452 | 0.1816 | 0.9438 | 0.1605 | 0.9428 |
II | 0.1662 | 0.9444 | 0.1503 | 0.9412 | 0.1662 | 0.9392 | 0.1433 | 0.9367 | |
I | 0.1484 | 0.9438 | 0.1445 | 0.9407 | 0.1484 | 0.9369 | 0.1289 | 0.9301 | |
(60, 20) | I | 0.1654 | 0.9443 | 0.1563 | 0.9447 | 0.1654 | 0.9368 | 0.1573 | 0.9307 |
II | 0.1516 | 0.9373 | 0.1357 | 0.9374 | 0.1516 | 0.9347 | 0.1411 | 0.9289 | |
I | 0.1332 | 0.9378 | 0.1243 | 0.9398 | 0.1332 | 0.9336 | 0.1275 | 0.9208 | |
(60, 40) | I | 0.1564 | 0.9442 | 0.1456 | 0.9432 | 0.1564 | 0.9364 | 0.1493 | 0.9110 |
II | 0.1473 | 0.9429 | 0.1267 | 0.9359 | 0.1473 | 0.9339 | 0.1388 | 0.9108 | |
I | 0.1106 | 0.9372 | 0.1153 | 0.9342 | 0.1106 | 0.9331 | 0.1010 | 0.9072 |
MLE | Boot | Bayes-SE | Bayes-GE | ||||||||
n | r | CS | θ, β | AB | MSE | AB | MSE | AB | MSE | AB | MSE |
0.40 | θ | 0.2372 | 0.1372 | 0.2253 | 0.1318 | 0.2078 | 0.1086 | 0.1926 | 0.0984 | ||
β | 0.2175 | 0.1214 | 0.2041 | 0.1162 | 0.1883 | 0.1485 | 0.1723 | 0.0835 | |||
0.60 | I | θ | 0.2253 | 0.1341 | 0.2124 | 0.1289 | 0.1949 | 0.1016 | 0.1808 | 0.0957 | |
β | 0.2051 | 0.1136 | 0.1922 | 0.1084 | 0.1797 | 0.1352 | 0.1604 | 0.0842 | |||
0.80 | θ | 0.2052 | 0.1071 | 0.1923 | 0.1019 | 0.1768 | 0.0979 | 0.1705 | 0.0893 | ||
β | 0.1853 | 0.0919 | 0.1721 | 0.0867 | 0.1555 | 0.1152 | 0.1503 | 0.0748 | |||
0.40 | θ | 0.1906 | 0.1246 | 0.1777 | 0.1194 | 0.1816 | 0.1114 | 0.1748 | 0.0965 | ||
β | 0.1357 | 0.1086 | 0.1228 | 0.1034 | 0.1131 | 0.1647 | 0.1022 | 0.0818 | |||
50 | 0.60 | II | θ | 0.1885 | 0.1177 | 0.1756 | 0.1125 | 0.1778 | 0.1099 | 0.1723 | 0.0946 |
β | 0.1283 | 0.0995 | 0.1154 | 0.0943 | 0.1098 | 0.1129 | 0.1027 | 0.0786 | |||
0.80 | θ | 0.1866 | 0.1161 | 0.1737 | 0.1109 | 0.1759 | 0.1094 | 0.1705 | 0.0919 | ||
β | 0.1206 | 0.0833 | 0.1077 | 0.0781 | 0.1035 | 0.1418 | 0.0913 | 0.0744 | |||
θ | 0.2347 | 0.1303 | 0.2218 | 0.1251 | 0.1966 | 0.0121 | 0.1784 | 0.0988 | |||
0.40 | β | 0.2144 | 0.1149 | 0.2015 | 0.1097 | 0.1767 | 0.1002 | 0.1582 | 0.0816 | ||
θ | 0.2218 | 0.1268 | 0.2089 | 0.1216 | 0.1931 | 0.1155 | 0.1747 | 0.0957 | |||
0.60 | III | β | 0.2016 | 0.1087 | 0.1887 | 0.1028 | 0.1659 | 0.0956 | 0.1555 | 0.0816 | |
θ | 0.2076 | 0.1256 | 0.1947 | 0.1204 | 0.1871 | 0.0947 | 0.1706 | 0.0924 | |||
0.80 | β | 0.1744 | 0.0846 | 0.1615 | 0.0794 | 0.1452 | 0.0942 | 0.1433 | 0.0734 | ||
θ | 0.1739 | 0.0983 | 0.166 | 0.1581 | 0.1667 | 0.0942 | 0.1268 | 0.0884 | |||
0.40 | β | 0.1537 | 0.0834 | 0.1458 | 0.1379 | 0.1469 | 0.0791 | 0.1074 | 0.0731 | ||
θ | 0.1647 | 0.0968 | 0.1568 | 0.1489 | 0.1522 | 0.0927 | 0.1149 | 0.0847 | |||
0.60 | I | β | 0.1512 | 0.0813 | 0.1433 | 0.1354 | 0.1325 | 0.0769 | 0.0947 | 0.0694 | |
θ | 0.1407 | 0.0962 | 0.1328 | 0.1249 | 0.1267 | 0.0908 | 0.1068 | 0.0785 | |||
0.80 | β | 0.1205 | 0.0809 | 0.1126 | 0.1047 | 0.1072 | 0.0729 | 0.0874 | 0.0632 | ||
θ | 0.1477 | 0.0922 | 0.1398 | 0.1319 | 0.1259 | 0.0896 | 0.1164 | 0.0856 | |||
0.40 | β | 0.1275 | 0.0769 | 0.1196 | 0.1117 | 0.1057 | 0.0747 | 0.0958 | 0.0721 | ||
θ | 0.1153 | 0.0707 | 0.1074 | 0.0995 | 0.0849 | 0.0685 | 0.0751 | 0.0643 | |||
80 | 0.60 | II | β | 0.1153 | 0.0707 | 0.1074 | 0.0995 | 0.0849 | 0.0709 | 0.0751 | 0.0641 |
θ | 0.1149 | 0.0758 | 0.1076 | 0.0991 | 0.1038 | 0.0726 | 0.0948 | 0.0746 | |||
0.80 | β | 0.0947 | 0.0665 | 0.0868 | 0.0789 | 0.0836 | 0.0605 | 0.0752 | 0.0598 | ||
θ | 0.1668 | 0.0972 | 0.1589 | 0.1517 | 0.1589 | 0.0927 | 0.1359 | 0.0874 | |||
0.40 | β | 0.1473 | 0.0819 | 0.1394 | 0.1315 | 0.1387 | 0.0753 | 0.1157 | 0.0721 | ||
θ | 0.1647 | 0.0965 | 0.1568 | 0.1489 | 0.1456 | 0.0905 | 0.1323 | 0.0859 | |||
0.60 | III | β | 0.1445 | 0.0817 | 0.1366 | 0.1287 | 0.1254 | 0.0734 | 0.1074 | 0.0731 | |
θ | 0.1377 | 0.0794 | 0.1298 | 0.1219 | 0.1256 | 0.0886 | 0.1068 | 0.0775 | |||
0.80 | β | 0.1175 | 0.0808 | 0.1096 | 0.1017 | 0.1054 | 0.0716 | 0.0857 | 0.0694 |
MLE | Boot | Bayes-SE | Bayes-GE | ||||||||
n | r | CS | θ, β | AB | MSE | AB | MSE | AB | MSE | AB | MSE |
I | |||||||||||
50 | 0.40 | θ | 0.2430 | 0.1402 | 0.2310 | 0.1332 | 0.2134 | 0.1102 | 0.1985 | 0.1003 | |
β | 0.2228 | 0.1246 | 0.2098 | 0.1176 | 0.1936 | 0.1501 | 0.1782 | 0.0849 | |||
0.60 | θ | 0.2311 | 0.1373 | 0.2181 | 0.1303 | 0.2005 | 0.1032 | 0.1867 | 0.0976 | ||
β | 0.2109 | 0.1168 | 0.1979 | 0.1098 | 0.1853 | 0.1368 | 0.1663 | 0.0861 | |||
0.80 | θ | 0.2110 | 0.1103 | 0.1980 | 0.1033 | 0.1816 | 0.0995 | 0.1764 | 0.0912 | ||
II | β | 0.1908 | 0.0951 | 0.1778 | 0.0881 | 0.1611 | 0.1168 | 0.1562 | 0.0759 | ||
0.40 | θ | 0.1964 | 0.1278 | 0.1834 | 0.1208 | 0.1866 | 0.1126 | 0.1807 | 0.0984 | ||
β | 0.1415 | 0.1118 | 0.1285 | 0.1048 | 0.1187 | 0.1663 | 0.1081 | 0.0837 | |||
0.60 | θ | 0.1943 | 0.1209 | 0.1813 | 0.1139 | 0.1834 | 0.1115 | 0.1782 | 0.0965 | ||
β | 0.1341 | 0.1027 | 0.1211 | 0.0957 | 0.1154 | 0.1145 | 0.1079 | 0.0805 | |||
0.80 | θ | 0.1924 | 0.1193 | 0.1794 | 0.1123 | 0.1815 | 0.1106 | 0.1764 | 0.0938 | ||
β | 0.1264 | 0.0865 | 0.1134 | 0.0795 | 0.1086 | 0.1434 | 0.0972 | 0.0763 | |||
0.40 | III | θ | 0.2405 | 0.1335 | 0.2275 | 0.1265 | 0.2022 | 0.1101 | 0.1843 | 0.1007 | |
β | 0.2202 | 0.1181 | 0.2072 | 0.1111 | 0.1823 | 0.1135 | 0.1641 | 0.0835 | |||
0.60 | θ | 0.2276 | 0.1300 | 0.2146 | 0.123 | 0.1987 | 0.1018 | 0.1806 | 0.0976 | ||
β | 0.2074 | 0.1112 | 0.1944 | 0.1042 | 0.1715 | 0.1171 | 0.1614 | 0.0835 | |||
0.80 | θ | 0.2134 | 0.1288 | 0.2004 | 0.1218 | 0.1927 | 0.0972 | 0.1765 | 0.0943 | ||
β | 0.1802 | 0.0878 | 0.1672 | 0.0808 | 0.1508 | 0.0963 | 0.1492 | 0.0753 | |||
0.40 | θ | 0.1803 | 0.0999 | 0.1723 | 0.0965 | 0.1732 | 0.0956 | 0.1332 | 0.0901 | ||
β | 0.1601 | 0.0846 | 0.1521 | 0.0812 | 0.1534 | 0.0807 | 0.1138 | 0.0748 | |||
0.60 | θ | 0.1711 | 0.0984 | 0.1631 | 0.0957 | 0.1587 | 0.0943 | 0.1213 | 0.0864 | ||
β | 0.1576 | 0.0829 | 0.1496 | 0.0795 | 0.1385 | 0.0785 | 0.1011 | 0.0711 | |||
0.80 | I | θ | 0.1471 | 0.0978 | 0.1391 | 0.0944 | 0.1332 | 0.0924 | 0.1132 | 0.0802 | |
β | 0.1269 | 0.0825 | 0.1189 | 0.0791 | 0.1137 | 0.0745 | 0.0938 | 0.0649 | |||
0.40 | θ | 0.1541 | 0.0938 | 0.1461 | 0.0904 | 0.1324 | 0.0906 | 0.1224 | 0.0873 | ||
β | 0.1339 | 0.0785 | 0.1259 | 0.0751 | 0.1122 | 0.0763 | 0.1022 | 0.0738 | |||
0.60 | θ | 0.1217 | 0.0723 | 0.1137 | 0.0689 | 0.0914 | 0.0701 | 0.0815 | 0.0657 | ||
80 | II | β | 0.1217 | 0.0723 | 0.1137 | 0.0689 | 0.0914 | 0.0725 | 0.0815 | 0.0658 | |
0.80 | θ | 0.1213 | 0.0774 | 0.1133 | 0.0748 | 0.1103 | 0.0742 | 0.1012 | 0.0763 | ||
β | 0.1011 | 0.0681 | 0.0931 | 0.0647 | 0.0901 | 0.0621 | 0.0816 | 0.0615 | |||
0.40 | θ | 0.1732 | 0.0988 | 0.1652 | 0.0954 | 0.1654 | 0.0943 | 0.1423 | 0.0891 | ||
β | 0.1537 | 0.0835 | 0.1457 | 0.0808 | 0.1452 | 0.0769 | 0.1221 | 0.0738 | |||
0.60 | θ | 0.1711 | 0.0981 | 0.1631 | 0.0947 | 0.1521 | 0.0921 | 0.1387 | 0.0867 | ||
III | β | 0.1509 | 0.0833 | 0.1429 | 0.0799 | 0.1319 | 0.0750 | 0.1138 | 0.0748 | ||
0.80 | θ | 0.1441 | 0.0810 | 0.1361 | 0.0790 | 0.1321 | 0.0902 | 0.1132 | 0.0792 | ||
β | 0.1239 | 0.0824 | 0.1159 | 0.0802 | 0.1119 | 0.0732 | 0.0921 | 0.0711 |
(θ=1, β=0.4) | (θ=1, β=0.8) | |||||||||
Asymptotic | Bootstrap | Credible | Asymptotic | Bootstrap | Credible | |||||
n | r | CS | θ, β | |||||||
0.40 | θ | 0.1927(99) | 0.1838(98) | 0.1774(97) | 0.1938(99) | 0.1852(98) | 0.1796(97) | |||
β | 0.1601(96) | 0.1528(95) | 0.1514(95) | 0.1628(96) | 0.1543(95) | 0.1521(95) | ||||
0.60 | I | θ | 0.1897(98) | 0.1827(98) | 0.1759(97) | 0.1887(98) | 0.1832(98) | 0.1780(97) | ||
β | 0.1594(96) | 0.1501(95) | 0.1492(95) | 0.1603(96) | 0.1522(95) | 0.1518(95) | ||||
0.80 | θ | 0.1842(98) | 0.1809(98) | 0.1743(97) | 0.1856(98) | 0.1827(98) | 0.1769(97) | |||
β | 0.1491(95) | 0.1483(95) | 0.1470(95) | 0.1528(95) | 0.1507(95) | 0.1493(95) | ||||
0.40 | θ | 0.1673(96) | 0.1568(96) | 0.1535(95) | 0.1694(96) | 0.1639(96) | 0.1571(96) | |||
β | 0.1347(94) | 0.1258(93) | 0.1275(93) | 0.1384(94) | 0.1330(94) | 0.1295(93) | ||||
50 | 0.60 | θ | 0.1643(96) | 0.1557(96) | 0.1526(95) | 0.1643(96) | 0.1618(96) | 0.1554(95) | ||
II | β | 0.1348(94) | 0.1231(93) | 0.1253(93) | 0.1359(94) | 0.1305(94) | 0.1292(93) | |||
0.80 | θ | 0.1588(96) | 0.1539(95) | 0.1504(95) | 0.1612(96) | 0.1614(96) | 0.1543(95) | |||
β | 0.1237(93) | 0.1213(93) | 0.1231(93) | 0.1284(93) | 0.1293(93) | 0.1267(93) | ||||
0.40 | θ | 0.1575(96) | 0.1583(96) | 0.1557(96) | 0.1618(96) | 0.1502(95) | 0.1446(95) | |||
β | 0.1249(93) | 0.1257(93) | 0.1231(92) | 0.1308(94) | 0.1193(92) | 0.1171(92) | ||||
0.60 | θ | 0.1545(95) | 0.1553(95) | 0.1527(95) | 0.1567(95) | 0.1482(95) | 0.1436(95) | |||
III | β | 0.1242(93) | 0.1255(93) | 0.1224(93) | 0.1283(93) | 0.1172(92) | 0.1168(92) | |||
0.80 | θ | 0.1493(95) | 0.1498(95) | 0.1472(95) | 0.1536(95) | 0.1477(95) | 0.1419(95) | |||
β | 0.1139(92) | 0.1147(92) | 0.1121(92) | 0.1208(93) | 0.1157(92) | 0.1143(92) | ||||
0.40 | θ | 0.1717(97) | 0.1598(96) | 0.1584(96) | 0.1749(97) | 0.1662(96) | 0.1626(96) | |||
β | 0.1391(94) | 0.1288(93) | 0.1324(94) | 0.1439(95) | 0.1353(94) | 0.1351(94) | ||||
0.60 | θ | 0.1687(97) | 0.1587(95) | 0.1569(95) | 0.1698(97) | 0.1642(96) | 0.1619(96) | |||
I | β | 0.1384(94) | 0.1261(93) | 0.1302(94) | 0.1414(95) | 0.1332(94) | 0.1348(94) | |||
0.80 | θ | 0.1632(96) | 0.1569(95) | 0.1553(95) | 0.1667(97) | 0.1637(96) | 0.1599(96) | |||
β | 0.1281(93) | 0.1243(93) | 0.1287(93) | 0.1339(94) | 0.1317(94) | 0.1323(95) | ||||
0.40 | θ | 0.1463(95) | 0.1328(94) | 0.1345(94) | 0.1505(95) | 0.1449(95) | 0.1401(95) | |||
β | 0.1137(94) | 0.1018(92) | 0.1085(93) | 0.1195(92) | 0.1148(92) | 0.1125(93) | ||||
0.60 | θ | 0.1433(95) | 0.1317(94) | 0.1336(94) | 0.1454(95) | 0.1428(95) | 0.1384(94) | |||
80 | II | β | 0.1138(93) | 0.0991(92) | 0.1063(93) | 0.1176(93) | 0.1115(93) | 0.1122(92) | ||
0.80 | θ | 0.1378(94) | 0.1299(93) | 0.1314(94) | 0.1423(95) | 0.1424(95) | 0.1373(94) | |||
β | 0.1027(92) | 0.0973(92) | 0.1041(93) | 0.1095(92) | 0.1103(93) | 0.1097(93) | ||||
0.40 | θ | 0.1365(94) | 0.1343(94) | 0.1367(94) | 0.1429(95) | 0.1312(94) | 0.1276(93) | |||
β | 0.1039(92) | 0.1017(92) | 0.1041(92) | 0.1119(92) | 0.1003(92) | 0.1001(92) | ||||
0.60 | θ | 0.1335(94) | 0.1313(94) | 0.1337(94) | 0.1378(94) | 0.1292(94) | 0.1262(93) | |||
III | β | 0.1032(92) | 0.1015(92) | 0.1034(93) | 0.1094(93) | 0.0982(92) | 0.0998(92) | |||
0.80 | θ | 0.1284(93) | 0.1258(93) | 0.1282(93) | 0.1347(94) | 0.1287(93) | 0.1249(93) | |||
β | 0.0929(91) | 0.0907(91) | 0.0931(91) | 0.1019(92) | 0.0967(92) | 0.0973(92) |
MLE | Boot | Bayes-SE | Bayes-GE | |||||||
n | r | CS | AB | MSE | AB | MSE | AB | MSE | AB | MSE |
50 | 0.40 | I | 0.2105 | 0.1223 | 0.2073 | 0.1158 | 0.1913 | 0.1488 | 0.1738 | 0.0823 |
0.60 | 0.2077 | 0.1204 | 0.1968 | 0.1131 | 0.1892 | 0.1436 | 0.1718 | 0.0802 | ||
0.80 | 0.1968 | 0.1185 | 0.1934 | 0.1094 | 0.1827 | 0.1415 | 0.169 | 0.0792 | ||
0.40 | II | 0.1907 | 0.1176 | 0.1908 | 0.1066 | 0.1774 | 0.1403 | 0.1675 | 0.0787 | |
0.60 | 0.1899 | 0.1134 | 0.1874 | 0.1063 | 0.1742 | 0.1395 | 0.1651 | 0.0763 | ||
0.80 | 0.1873 | 0.1117 | 0.1856 | 0.1022 | 0.1721 | 0.1369 | 0.1628 | 0.0754 | ||
0.40 | III | 0.1865 | 0.1109 | 0.1832 | 0.1007 | 0.1711 | 0.1329 | 0.1602 | 0.073 | |
0.60 | 0.1842 | 0.1085 | 0.1818 | 0.1001 | 0.1702 | 0.1311 | 0.1589 | 0.0712 | ||
0.80 | 0.1827 | 0.1043 | 0.1806 | 0.0972 | 0.1685 | 0.1300 | 0.1571 | 0.0695 | ||
80 | 0.40 | I | 0.1812 | 0.1012 | 0.1799 | 0.0961 | 0.1642 | 0.1289 | 0.1547 | 0.0632 |
0.60 | 0.1793 | 0.1002 | 0.1749 | 0.0943 | 0.1638 | 0.1254 | 0.1518 | 0.0611 | ||
0.80 | 0.1772 | 0.0988 | 0.1727 | 0.0916 | 0.1587 | 0.1231 | 0.1500 | 0.0603 | ||
0.40 | II | 0.1744 | 0.0965 | 0.1708 | 0.0907 | 0.1548 | 0.1225 | 0.1478 | 0.0591 | |
0.60 | 0.1717 | 0.0938 | 0.1693 | 0.0874 | 0.1525 | 0.1206 | 0.1465 | 0.0532 | ||
0.80 | 0.1708 | 0.0916 | 0.1635 | 0.0844 | 0.1501 | 0.1191 | 0.1451 | 0.0521 | ||
0.40 | III | 0.1689 | 0.0901 | 0.1622 | 0.081 | 0.1492 | 0.1131 | 0.1438 | 0.0516 | |
0.60 | 0.1646 | 0.0883 | 0.1601 | 0.0792 | 0.1461 | 0.1115 | 0.1421 | 0.0500 | ||
0.80 | 0.1622 | 0.0861 | 0.1593 | 0.0774 | 0.1448 | 0.1109 | 0.1413 | 0.0492 |
Asymptotic | Bootstrap | Credible | HPD Credible | ||||||||||
n | r | CS | AIL | CP | AIL | CP | AIL | CP | AIL | CP | |||
50 | 0.40 | I | 0.1624 | 98% | 0.1539 | 97% | 0.1520 | 97% | 0.1492 | 96% | |||
0.60 | 0.1617 | 98% | 0.1523 | 96% | 0.1518 | 96% | 0.1467 | 96% | |||||
0.80 | 0.1596 | 97% | 0.1517 | 96% | 0.1502 | 96% | 0.1443 | 96% | |||||
0.40 | II | 0.1576 | 97% | 0.1508 | 96% | 0.1495 | 96% | 0.1437 | 95% | ||||
0.60 | 0.1549 | 97% | 0.1496 | 96% | 0.1472 | 96% | 0.1428 | 95% | |||||
0.80 | 0.1538 | 97% | 0.1477 | 96% | 0.1447 | 96% | 0.1419 | 95% | |||||
0.40 | III | 0.1521 | 96% | 0.1468 | 96% | 0.1432 | 96% | 0.1405 | 95% | ||||
0.60 | 0.1510 | 96% | 0.1443 | 96% | 0.1429 | 95% | 0.1388 | 95% | |||||
0.80 | 0.1502 | 96% | 0.1439 | 96% | 0.1406 | 95% | 0.1382 | 94% | |||||
80 | 0.40 | I | 0.1487 | 96% | 0.1421 | 95% | 0.1386 | 95% | 0.1374 | 94% | |||
0.60 | 0.1476 | 96% | 0.1414 | 95% | 0.1378 | 95% | 0.1367 | 94% | |||||
0.80 | 0.1468 | 96% | 0.1409 | 95% | 0.1363 | 95% | 0.1359 | 94% | |||||
0.40 | II | 0.1455 | 96% | 0.1396 | 95% | 0.1348 | 95% | 0.1345 | 94% | ||||
0.60 | 0.1447 | 96% | 0.1383 | 95% | 0.1339 | 95% | 0.1338 | 94% | |||||
0.80 | 0.1436 | 95% | 0.1375 | 95% | 0.1325 | 94% | 0.1329 | 93% | |||||
0.40 | III | 0.1424 | 95% | 0.1367 | 95% | 0.1320 | 94% | 0.1315 | 93% | ||||
0.60 | 0.1388 | 95% | 0.1359 | 94% | 0.1311 | 93% | 0.1306 | 92% | |||||
0.80 | 0.1374 | 95% | 0.1343 | 94% | 0.1308 | 93% | 0.1289 | 92% |
1) All the obtained (point and interval) estimates have satisfactory behavior and gave an efficient inference about the unknown parameters of the PRD and the reliability function.
2) All estimates perform better with an increase in sample size n, the proportion of effective sample size r and the ideal time T.
3) The point and interval estimates obtained by the Bootstrap sampling method are clearly better than their corresponding estimates obtained by the conventional likelihood method.
4) Under the same settings, the Bayes estimates obtained by the MCMC method are better than estimates obtained by the Likelihood and Bootstrap classical approaches.
5) We notice that the obtained estimates become better with a decrease in the value of the shape parameter.
6) It is noted that the CPs of the obtained interval estimates are almost close at a 95% confidence level, but when the lengths of (Asymptotic, Bootstrap or Credible) interval estimates become relatively small enough, the CPs of the interval estimates are strictly less than their nominal 95% confidence level.
7) In comparing schemes 1–3, we conclude that:
(i) Point estimates obtained under the progressive SC.II scheme are better than point estimates obtained under progressive (SC.I and SC.III) censoring schemes.
(ii) Interval estimates obtained under SC.III have smaller lengths than interval estimates obtained under progressive (SC.I and SC.II) schemes.
We illustrate the application of the developed estimation methods. The inferential procedure is investigated in a real data set from Al-Aqtash et al. [36] This data set consists of 66 observations representing the breaking stress of carbon fibers of 50 mm length (GPa). Bhat and Ahmad [20] have analyzed this data to confirm the priority fit of the PRD in real-life data as compared to other generalizations of Rayleigh and Weibull distributions. Assuming the PRD modeling for this data, the MLEs of the parameters θ and β using the complete data set (see Bhat and Ahmad [20]) are ˆθcom=4.850, ˆβcom=1.721, the asymptotic 95% confidence intervals for θ and β are (2.818,6.883) and (1.396,2.045). The Kolmogorov-Smirnov (K-S), the Akaike's information and the negative log-likelihood goodness of fit test statistics are 0.08, 172.14 and 176.33, respectively, with a p-value = 0.768. Figures 3–6 present the empirical and the theoretical CDF, the histogram plot, the empirical survival function and the empirical cumulative hazard function of these data.
To confirm the validity of the PRD for the APT-II censored data, we generate APT-II censored data from the original data with m=40 and T=3.5 using two different censoring schemes as presented in Table 8. Based on the APT-II censored data, the performance of point estimates ˆθ and ˆβ of θ and β, respectively, are measured in terms of their relative efficiencies defined as:
Rˆθ=1−|ˆθ−ˆθcom|ˆθcom,Rˆβ=1−|ˆβ−ˆβcom|ˆβcom. |
Scheme: (2, 0∗20, 2, 0∗20) | ||||||||||||||
0.39 | 1.08 | 1.25 | 1.47 | 1.57 | 1.61 | 1.69 | 1.80 | 1.84 | 1.87 | 1.89 | 2.03 | 2.03 | 2.05 | 2.12 |
2.35 | 2.41 | 2.43 | 2.48 | 2.50 | 2.53 | 2.55 | 2.55 | 2.59 | 2.67 | 2.74 | 2.79 | 2.81 | 2.82 | 2.85 |
2.87 | 2.88 | 2.93 | 2.95 | 2.96 | 2.97 | 3.09 | 3.11 | 3.11 | 3.15 | |||||
Scheme: (8, 0∗25, 8, 0∗15) | ||||||||||||||
0.39 | 0.85 | 1.25 | 1.47 | 1.61 | 1.80 | 1.87 | 2.03 | 2.12 | 2.35 | 2.41 | 2.43 | 2.48 | 2.50 | 2.53 |
2.55 | 2.55 | 2.56 | 2.59 | 2.67 | 2.73 | 2.74 | 2.79 | 2.81 | 2.82 | 2.93 | 2.95 | 2.97 | 3.11 | 3.15 |
3.19 | 3.22 | 3.28 | 3.31 | 3.31 | 3.33 | 3.39 | 3.39 | 3.56 | 3.60 |
The relative efficiencies of the point estimates and the ABLs of the interval estimates are listed in Table 9. Based on (2,0∗20,2,0∗20) and (8,0∗25,8,0∗15) APT-II censoring schemes, Figures 7 and 8 present the trace and the histogram plots for point estimates of (θ, β) using 12000 samples generated by the MCMC method and assuming M=2000 is the burn-in period. As clearly observed from Table 9, all point estimates have high relative efficiency and The Bayes point estimates under GE are the best among others as they have the highest relative efficiency. All estimates became better with a decrease in the sum of random removals and the interval estimates are also very close to the approximate intervals for (θ, β) obtained by the asymptotic distributions using the complete data set. It is clearly observed from Figures 7 and 8 that Bayesian estimates obtained by the MCMC converge to their means and thus the MCMC is recommended.
Relative efficiency | Absolute difference lengths(ABLs) | |||||||
parameter | CS | MLE | Bayes-SE | Bayes-GE | Bootstrap | Asymptotic | Credible | Bootstrap |
θ | (2,0∗20,2,0∗20) | 0.9832 | 0.9851 | 0.9876 | 0.9842 | 0.0964 | 0.0961 | 0.0955 |
(8,0∗25,8,0∗15) | 0.9706 | 0.9723 | 0.9735 | 0.9714 | 0.101 | 0.0985 | 0.0971 | |
β | (2,0∗20,2,0∗20) | 0.9864 | 0.9896 | 0.9902 | 0.9876 | 0.0935 | 0.0927 | 0.0919 |
(8,0∗25,8,0∗15) | 0.9726 | 0.9745 | 0.9813 | 0.9753 | 0.0982 | 0.0962 | 0.0945 |
In this paper, based on the Type-II censoring, we have considered point and interval estimation for the parameters and the reliability function of PRD via likelihood, Bayesian and Bootstrap estimation methods. The Bayesian estimation approach is carried out under the SE and GE loss functions. When the shape parameter is known, the ML and Bayesian point estimates of the scale parameter, as well as the corresponding asymptotic confidence, credible and HPD credible intervals, are obtained in explicit forms. When both parameters are unknown, the MLEs and the asymptotic confidence intervals of the unknown parameters are obtained using the Newton-Raphson numerical method, while Bayesian point estimators and associated credible and HPD credible intervals are obtained based on the MCMC method.
To evaluate the performance of the obtained point and interval estimates, a simulation study is conducted in different combinations of the life testing experiment, and the estimation procedure is also applied to a real-life data set. Concluding results indicated that both point and interval estimates are efficient and have good characteristics for estimating the model parameters with a specific preference for the Bayesian point and interval estimates obtained under the GE loss function.
As a generalization of the standard Rayleigh distribution, the main conclusions conducted from this work confirmed that the PRD is an appropriate lifetime model and can be effectively used with Type-II censored data. Future researchers may extend this work by employing PRD with other censoring schemes.
The authors declare they have not used Artificial Intelligence (AI) tools in the creation of this article.
The authors declare no conflict of interest.
[1] |
N. Balakrishnan, Progressive censoring methodology: An appraisal, TEST, 16 (2007), 211–259. https://doi.org/10.1007/s11749-007-0061-y doi: 10.1007/s11749-007-0061-y
![]() |
[2] |
R. Aggarwala, N. Balakrishnan, Some properties of progressive censored order statistics from arbitrary and uniform distributions with applications to inference and simulation, J. Stat. Plan. Infer., 70 (1998), 35–49. https://doi.org/10.1016/s0378-3758(97)00173-0 doi: 10.1016/s0378-3758(97)00173-0
![]() |
[3] |
H. Z. Muhammed, E. M. Almetwally, Bayesian and non-Bayesian estimation for the bivariate inverse weibull distribution under progressive type-II censoring, Ann. Data Sci., 10 (2023), 481–512. https://doi.org/10.1007/s40745-020-00316-7 doi: 10.1007/s40745-020-00316-7
![]() |
[4] |
R. Alshenawy, A. Al-Alwan, E. M. Almetwally, A. Z. Afify, H. M. Almongy, Progressive type-II censoring schemes of extended odd Weibull exponential distribution with applications in medicine and engineering, Mathematics, 8 (2020), 1679. https://doi.org/10.3390/math8101679 doi: 10.3390/math8101679
![]() |
[5] |
K. Maiti, S. Kayal, Estimation of parameters and reliability characteristics for a generalized Rayleigh distribution under progressive type-II censored sample, Commun. Stat.-Simul. Comput., 50 (2021), 3669–3698. https://doi.org/10.1080/03610918.2019.1630431 doi: 10.1080/03610918.2019.1630431
![]() |
[6] |
S. Dey, A. Elshahhat, Analysis of Wilson‐Hilferty distribution under progressive Type‐II censoring, Qual. Reliab. Eng. Int., 38 (2022), 3771–3796. https://doi.org/10.1002/qre.3173 doi: 10.1002/qre.3173
![]() |
[7] |
A. Elshahhat, A. H. Muse, O. M. Egeh, B. R. Elemary, Estimation for parameters of life of the Marshall-Olkin generalized-exponential distribution using progressive Type-II censored data, Complexity, 2022 (2022), 8155929. https://doi.org/10.1155/2022/8155929 doi: 10.1155/2022/8155929
![]() |
[8] |
E. M. Almetwally, T. M. Jawa, N. Sayed-Ahmed, C. Park, M. Zakarya, S. Dey, Analysis of unit-Weibull based on progressive type-II censored with optimal scheme, Alex. Eng. J., 63 (2023), 321–338. https://doi.org/10.1016/j.aej.2022.07.064 doi: 10.1016/j.aej.2022.07.064
![]() |
[9] |
Y. A. Tashkandy, E. M. Almetwally, R. Ragab, A. M. Gemeay, M. M. Abd El-Raouf, S. K. Khosa, et al., Statistical inferences for the extended inverse Weibull distribution under progressive type-II censored sample with applications, Alex. Eng. J., 65 (2023), 493–502. https://doi.org/10.1016/j.aej.2022.09.023 doi: 10.1016/j.aej.2022.09.023
![]() |
[10] |
S. M. A. Aljeddani, M. A. Mohammed, Estimating the power generalized Weibull Distribution's parameters using three methods under Type-II Censoring-Scheme, Alex. Eng. J., 67 (2023), 219–228. https://doi.org/10.1016/j.aej.2022.12.043 doi: 10.1016/j.aej.2022.12.043
![]() |
[11] |
H. K. T. Ng, D. Kundu, P. S. Chan, Statistical analysis of exponential lifetimes under an adaptive Type‐II progressive censoring scheme, Nav. Res. Log., 56 (2009), 687–698. https://doi.org/10.1002/nav.20371 doi: 10.1002/nav.20371
![]() |
[12] |
S. Chen, W. Gui, Statistical analysis of a lifetime distribution with a bathtub-shaped failure rate function under adaptive progressive type-II censoring, Mathematics, 8 (2020), 670. https://doi.org/10.3390/math8050670 doi: 10.3390/math8050670
![]() |
[13] |
M. H. Abu-Moussa, M. M. Mohie El-Din, M. A. Mosilhy, Statistical inference for Gompertz distribution using the adaptive-general progressive type-II censored samples, Amer. J. Math. Manage. Sci., 40 (2021), 189–211. https://doi.org/10.1080/01966324.2020.1835590 doi: 10.1080/01966324.2020.1835590
![]() |
[14] |
M. A. W. Mahmoud, A. A. Soliman, A. H. Abd Ellah, R. M. El-Sagheer, Estimation of generalized Pareto under an adaptive type-II progressive censoring, Intell. Inf. Manage., 5 (2013), 73–83. https://doi.org/10.4236/iim.2013.53008 doi: 10.4236/iim.2013.53008
![]() |
[15] |
S. Asadi, H. Panahi, C. Swarup, S. A. Lone, Inference on adaptive progressive hybrid censored accelerated life test for Gompertz distribution and its evaluation for virus-containing micro droplets data, Alex. Eng. J., 61 (2022), 10071–10084. https://doi.org/10.1016/j.aej.2022.02.061 doi: 10.1016/j.aej.2022.02.061
![]() |
[16] |
R. Alotaibi, M. Nassar, A. Elshahhat, Computational analysis of XLindley parameters using adaptive Type-II progressive hybrid censoring with applications in chemical engineering, Mathematics, 10 (2022), 3355. https://doi.org/10.3390/math10183355 doi: 10.3390/math10183355
![]() |
[17] |
S. J. Almalki, A. W. A. Farghal, M. K. Rastogi, G. A. Abd-Elmougod, Partially constant-stress accelerated life tests model for parameters estimation of Kumaraswamy distribution under adaptive Type-II progressive censoring, Alex. Eng. J., 61 (2022), 5133–5143. https://doi.org/10.1016/j.aej.2021.10.035 doi: 10.1016/j.aej.2021.10.035
![]() |
[18] |
H. H. Ahmad, M. M. Salah, M. S. Eliwa, Z. A. Alhussain, E. M. Almetwally, E. A. Ahmed, Bayesian and non-Bayesian inference under adaptive type-II progressive censored sample with exponentiated power Lindley distribution, J. Appl. Stat., 49 (2022), 2981–3001. https://doi.org/10.1080/02664763.2021.1931819 doi: 10.1080/02664763.2021.1931819
![]() |
[19] |
K. K. Shukla, R. Shanker, Power Ishita distribution and its application to model lifetime data, Stat. Transit. New Ser., 19 (2018), 135–148. https://doi.org/10.21307/stattrans-2018-008 doi: 10.21307/stattrans-2018-008
![]() |
[20] | A. A. Bhat, S. P. Ahmad, A new generalization of Rayleigh distribution: Properties and applications, Pak. J. Stat., 36 (2020), 225–250. |
[21] |
D. Kundu, M. Z. Raqab, Generalized Rayleigh distribution: Different methods of estimations, Comput. Stat. Data Anal., 49 (2005), 187–200. https://doi.org/10.1016/j.csda.2004.05.008 doi: 10.1016/j.csda.2004.05.008
![]() |
[22] |
K. Ateeq, B. T. Qasim, R. A. Alvi, An extension of Rayleigh distribution and applications, Cogent Math. Stat., 6 (2019), 1622191. https://doi.org/10.1080/25742558.2019.1622191 doi: 10.1080/25742558.2019.1622191
![]() |
[23] |
M. A. W. Mahmoud, M. G. M. Ghazal, Estimations from the exponentiated Rayleigh distribution based on generalized Type-II hybrid censored data, J. Egypt. Math. Soc., 25 (2017), 71–78. https://doi.org/10.1016/j.joems.2016.06.008 doi: 10.1016/j.joems.2016.06.008
![]() |
[24] |
E. M. Almetwally, H. M. Almongy, E. A. ElSherpieny, Adaptive type-II progressive censoring schemes based on maximum product spacing with application of generalized Rayleigh distribution, J. Data Sci., 17 (2019), 802–831. https://doi.org/10.6339/jds.201910\textunderscore17(4).0010 doi: 10.6339/jds.201910\textunderscore17(4).0010
![]() |
[25] |
H. Panahi, N. Moradi, Estimation of the inverted exponentiated Rayleigh distribution based on adaptive Type II progressive hybrid censored sample, J. Comput. Appl. Math., 364 (2020), 112345. https://doi.org/10.1016/j.cam.2019.112345 doi: 10.1016/j.cam.2019.112345
![]() |
[26] |
S. Gao, J. Yu, W. Gui, Pivotal inference for the inverted exponentiated Rayleigh distribution based on progressive type-II censored data, Amer. J. Math. Manage. Sci., 39 (2020), 315–328. https://doi.org/10.1080/01966324.2020.1762142 doi: 10.1080/01966324.2020.1762142
![]() |
[27] |
J. Fan, W. Gui, Statistical inference of inverted exponentiated Rayleigh distribution under joint progressively type-II censoring, Entropy, 24 (2022), 171. https://doi.org/10.3390/e24020171 doi: 10.3390/e24020171
![]() |
[28] | J. F. Lawless, Statistical models and methods for lifetime data, John Wiley & Sons, Inc. 2003. https://doi.org/10.1002/9781118033005 |
[29] |
N. Metropolis, A. W. Rosenbluth, M. N. Rosenbluth, A. H. Teller, E. Teller, Equation of state calculations by fast computing machines, J. Chem. Phys., 21 (1953), 1087–1092. https://doi.org/10.1063/1.1699114 doi: 10.1063/1.1699114
![]() |
[30] |
W. K. Hastings, Monte Carlo sampling methods using Markov chains and their applications, Biometrika, 57 (1970), 97–109. https://doi.org/10.1093/biomet/57.1.97 doi: 10.1093/biomet/57.1.97
![]() |
[31] |
M. H. Chen, Q. M. Shao, Monte Carlo estimation of Bayesian credible and HPD intervals, J. Comput. Graph. Stat., 8 (1999), 69–92. https://doi.org/10.2307/1390921 doi: 10.2307/1390921
![]() |
[32] |
P. Hall, Theoretical comparison of bootstrap confidence intervals, Ann. Statist., 16 (1988), 927–953. https://doi.org/10.1214/aos/1176350933 doi: 10.1214/aos/1176350933
![]() |
[33] | B. Efron, R. J. Tibshirani, An introduction to the bootstrap, CRC Press, 1994. |
[34] |
N. Balakrishnan, R. A. Sandhu, A simple simulational algorithm for generating progressive TypeII censored samples, Amer. Stat., 49 (1995), 229–230. https://doi.org/10.2307/2684646 doi: 10.2307/2684646
![]() |
[35] |
D. Kundu, Bayesian inference and life testing plan for the Weibull distribution in presence of progressive censoring, Technometrics, 50 (2008), 144–154. https://doi.org/10.1198/004017008000000217 doi: 10.1198/004017008000000217
![]() |
[36] |
R. Al-Aqtash, C. Lee, F. Famoye, Gumbel-Weibull distribution: Properties and applications, J. Mod. Appl. Stat. Meth., 13 (2014), 11. https://doi.org/10.22237/jmasm/1414815000 doi: 10.22237/jmasm/1414815000
![]() |
1. | Shixiao Xiao, Xue Hu, Haiping Ren, Estimation of Lifetime Performance Index for Generalized Inverse Lindley Distribution Under Adaptive Progressive Type-II Censored Lifetime Test, 2024, 13, 2075-1680, 727, 10.3390/axioms13100727 | |
2. | Hatim Solayman Migdadi, Nesreen M. Al-Olaimat, Omar Meqdadi, Inference and optimal design for the k-level step-stress accelerated life test based on progressive Type-I interval censored power Rayleigh data, 2023, 20, 1551-0018, 21407, 10.3934/mbe.2023947 | |
3. | Mustafa M Hasaballah, Oluwafemi Samson Balogun, M E Bakr, Point and interval estimation based on joint progressive censoring data from two Rayleigh-Weibull distribution with applications, 2024, 99, 0031-8949, 085239, 10.1088/1402-4896/ad6107 | |
4. | Mustafa M. Hasaballah, Yusra A. Tashkandy, Oluwafemi Samson Balogun, M. E. Bakr, Reliability analysis for two populations Nadarajah-Haghighi distribution under Joint progressive type-II censoring, 2024, 9, 2473-6988, 10333, 10.3934/math.2024505 | |
5. | Mustafa M Hasaballah, Oluwafemi Samson Balogun, M E Bakr, Bayesian estimation for the power rayleigh lifetime model with application under a unified hybrid censoring scheme, 2024, 99, 0031-8949, 105209, 10.1088/1402-4896/ad72b2 |
MLE | Boot | Bayes-SE | Bayes-GE | ||||||
(n, m) | sc | AB | MSE | AB | MSE | AB | MSE | AB | MSE |
T= 1 | |||||||||
(40, 15) | I | 0.2741 | 0.1801 | 0.2283 | 0.1334 | 0.2321 | 0.1201 | 0.2172 | 0.1183 |
II | 0.1918 | 0.1310 | 0.1403 | 0.1078 | 0.1306 | 0.1042 | 0.1300 | 0.1026 | |
III | 0.2631 | 0.1655 | 0.2202 | 0.1263 | 0.2019 | 0.1184 | 0.1987 | 0.1109 | |
(40, 20) | I | 0.2543 | 0.1415 | 0.2142 | 0.1278 | 0.2115 | 0.1106 | 0.2064 | 0.1095 |
II | 0.1831 | 0.1296 | 0.1392 | 0.1001 | 0.1295 | 0.0995 | 0.1143 | 0.0912 | |
III | 0.2428 | 0.1401 | 0.2137 | 0.1206 | 0.2001 | 0.1100 | 0.1988 | 0.1066 | |
(60, 20) | I | 0.1778 | 0.0982 | 0.1587 | 0.0943 | 0.1213 | 0.0864 | 0.1171 | 0.0776 |
II | 0.1412 | 0.0876 | 0.1116 | 0.0851 | 0.1017 | 0.0811 | 0.0958 | 0.0658 | |
III | 0.1711 | 0.0986 | 0.1521 | 0.0952 | 0.1201 | 0.0843 | 0.1104 | 0.1104 | |
(60, 40) | I | 0.1471 | 0.0978 | 0.1332 | 0.0924 | 0.1132 | 0.0802 | 0.1089 | 0.0704 |
II | 0.1213 | 0.0774 | 0.1103 | 0.0742 | 0.1012 | 0.0763 | 0.0933 | 0.0643 | |
III | 0.1441 | 0.0976 | 0.1321 | 0.0902 | 0.1123 | 0.0792 | 0.1118 | 0.0703 | |
T=2 | |||||||||
(40, 15) | I | 0.2077 | 0.1106 | 0.1821 | 0.0817 | 0.1631 | 0.0763 | 0.1022 | 0.0632 |
II | 0.1309 | 0.0958 | 0.1122 | 0.0784 | 0.0997 | 0.0743 | 0.0881 | 0.0639 | |
III | 0.2042 | 0.1057 | 0.1678 | 0.0806 | 0.1578 | 0.0773 | 0.1101 | 0.0655 | |
(40, 20) | I | 0.1876 | 0.0888 | 0.1579 | 0.0781 | 0.1532 | 0.0697 | 0.1003 | 0.0615 |
II | 0.1232 | 0.0803 | 0.1054 | 0.0731 | 0.0943 | 0.0699 | 0.0805 | 00629 | |
III | 0.1774 | 0.0816 | 0.1476 | 0.0775 | 0.1460 | 0.0691 | 0.1022 | 0.0637 | |
(60, 20) | I | 0.1544 | 0.0767 | 0.1353 | 0.0728 | 0.0979 | 0.0649 | 0.0925 | 0.0608 |
II | 0.1178 | 0.0661 | 0.0882 | 0.0636 | 0.0783 | 0.0596 | 0.0766 | 0.0588 | |
III | 0.1477 | 0.0771 | 0.1287 | 0.0737 | 0.0967 | 0.0628 | 0.0931 | 0.0622 | |
(60, 40) | I | 0.1237 | 0.0763 | 0.1098 | 0.0709 | 0.0898 | 0.0587 | 0.0832 | 0.0580 |
II | 0.0979 | 0.0559 | 0.0869 | 0.0527 | 0.0778 | 0.0548 | 0.0751 | 0.0510 | |
III | 0.1207 | 0.0761 | 0.1087 | 0.0687 | 0.0889 | 0.0577 | 0.0812 | 0. |
Asymptotic | Bootstrap | Credible | HPD Credible | ||||||
(n, m) | sc | AL | CP | AL | CP | AL | CP | AL | CP |
T= 1 | |||||||||
(40, 15) | I | 0.2107 | 0.9684 | 0.2006 | 0.9624 | 0.1906 | 0.9618 | 0.1892 | 0.9541 |
II | 0.1978 | 0.9634 | 0.1917 | 0.9593 | 0.1893 | 0.9582 | 0.1831 | 0.9516 | |
I | 0.1764 | 0.9581 | 0.1723 | 0.9580 | 0.1702 | 0.9567 | 0.1694 | 0.9508 | |
(40, 20) | I | 0.2064 | 0.9625 | 0.1901 | 0.9624 | 0.1876 | 0.9610 | 0.1807 | 0.9538 |
II | 0.1908 | 0.9612 | 0.1829 | 0.9584 | 0.1751 | 0.9564 | 0.1688 | 0.9512 | |
I | 0.1732 | 0.9572 | 0.1705 | 0.9579 | 0.1693 | 0.9541 | 0.1604 | 0.9506 | |
(60, 20) | I | 0.1902 | 0.9615 | 0.1823 | 0.9619 | 0.1811 | 0.9540 | 0.1773 | 0.9533 |
II | 0.1764 | 0.9545 | 0.1631 | 0.9546 | 0.1605 | 0.9519 | 0.1587 | 0.9507 | |
I | 0.1578 | 0.9550 | 0.1509 | 0.9570 | 0.1491 | 0.9508 | 0.1432 | 0.9501 | |
(60, 40) | I | 0.1812 | 0.9614 | 0.1720 | 0.9604 | 0.1704 | 0.9536 | 0.1701 | 0.9512 |
II | 0.1721 | 0.9601 | 0.1584 | 0.9531 | 0.1515 | 0.9511 | 0.1508 | 0.9503 | |
I | 0.1354 | 0.9544 | 0.1417 | 0.9514 | 0.1401 | 0.9502 | 0.1372 | 0.9492 | |
T=2 | |||||||||
(40, 15) | I | 0.1859 | 0.9512 | 0.1658 | 0.9452 | 0.1859 | 0.9446 | 0.1698 | 0.9431 |
II | 0.1730 | 0.9462 | 0.1645 | 0.9421 | 0.1733 | 0.9412 | 0.1496 | 0.9410 | |
I | 0.1516 | 0.9409 | 0.1454 | 0.9408 | 0.1516 | 0.9395 | 0.1321 | 0.9352 | |
(40, 20) | I | 0.1816 | 0.9453 | 0.1628 | 0.9452 | 0.1816 | 0.9438 | 0.1605 | 0.9428 |
II | 0.1662 | 0.9444 | 0.1503 | 0.9412 | 0.1662 | 0.9392 | 0.1433 | 0.9367 | |
I | 0.1484 | 0.9438 | 0.1445 | 0.9407 | 0.1484 | 0.9369 | 0.1289 | 0.9301 | |
(60, 20) | I | 0.1654 | 0.9443 | 0.1563 | 0.9447 | 0.1654 | 0.9368 | 0.1573 | 0.9307 |
II | 0.1516 | 0.9373 | 0.1357 | 0.9374 | 0.1516 | 0.9347 | 0.1411 | 0.9289 | |
I | 0.1332 | 0.9378 | 0.1243 | 0.9398 | 0.1332 | 0.9336 | 0.1275 | 0.9208 | |
(60, 40) | I | 0.1564 | 0.9442 | 0.1456 | 0.9432 | 0.1564 | 0.9364 | 0.1493 | 0.9110 |
II | 0.1473 | 0.9429 | 0.1267 | 0.9359 | 0.1473 | 0.9339 | 0.1388 | 0.9108 | |
I | 0.1106 | 0.9372 | 0.1153 | 0.9342 | 0.1106 | 0.9331 | 0.1010 | 0.9072 |
MLE | Boot | Bayes-SE | Bayes-GE | ||||||||
n | r | CS | θ, β | AB | MSE | AB | MSE | AB | MSE | AB | MSE |
0.40 | θ | 0.2372 | 0.1372 | 0.2253 | 0.1318 | 0.2078 | 0.1086 | 0.1926 | 0.0984 | ||
β | 0.2175 | 0.1214 | 0.2041 | 0.1162 | 0.1883 | 0.1485 | 0.1723 | 0.0835 | |||
0.60 | I | θ | 0.2253 | 0.1341 | 0.2124 | 0.1289 | 0.1949 | 0.1016 | 0.1808 | 0.0957 | |
β | 0.2051 | 0.1136 | 0.1922 | 0.1084 | 0.1797 | 0.1352 | 0.1604 | 0.0842 | |||
0.80 | θ | 0.2052 | 0.1071 | 0.1923 | 0.1019 | 0.1768 | 0.0979 | 0.1705 | 0.0893 | ||
β | 0.1853 | 0.0919 | 0.1721 | 0.0867 | 0.1555 | 0.1152 | 0.1503 | 0.0748 | |||
0.40 | θ | 0.1906 | 0.1246 | 0.1777 | 0.1194 | 0.1816 | 0.1114 | 0.1748 | 0.0965 | ||
β | 0.1357 | 0.1086 | 0.1228 | 0.1034 | 0.1131 | 0.1647 | 0.1022 | 0.0818 | |||
50 | 0.60 | II | θ | 0.1885 | 0.1177 | 0.1756 | 0.1125 | 0.1778 | 0.1099 | 0.1723 | 0.0946 |
β | 0.1283 | 0.0995 | 0.1154 | 0.0943 | 0.1098 | 0.1129 | 0.1027 | 0.0786 | |||
0.80 | θ | 0.1866 | 0.1161 | 0.1737 | 0.1109 | 0.1759 | 0.1094 | 0.1705 | 0.0919 | ||
β | 0.1206 | 0.0833 | 0.1077 | 0.0781 | 0.1035 | 0.1418 | 0.0913 | 0.0744 | |||
θ | 0.2347 | 0.1303 | 0.2218 | 0.1251 | 0.1966 | 0.0121 | 0.1784 | 0.0988 | |||
0.40 | β | 0.2144 | 0.1149 | 0.2015 | 0.1097 | 0.1767 | 0.1002 | 0.1582 | 0.0816 | ||
θ | 0.2218 | 0.1268 | 0.2089 | 0.1216 | 0.1931 | 0.1155 | 0.1747 | 0.0957 | |||
0.60 | III | β | 0.2016 | 0.1087 | 0.1887 | 0.1028 | 0.1659 | 0.0956 | 0.1555 | 0.0816 | |
θ | 0.2076 | 0.1256 | 0.1947 | 0.1204 | 0.1871 | 0.0947 | 0.1706 | 0.0924 | |||
0.80 | β | 0.1744 | 0.0846 | 0.1615 | 0.0794 | 0.1452 | 0.0942 | 0.1433 | 0.0734 | ||
θ | 0.1739 | 0.0983 | 0.166 | 0.1581 | 0.1667 | 0.0942 | 0.1268 | 0.0884 | |||
0.40 | β | 0.1537 | 0.0834 | 0.1458 | 0.1379 | 0.1469 | 0.0791 | 0.1074 | 0.0731 | ||
θ | 0.1647 | 0.0968 | 0.1568 | 0.1489 | 0.1522 | 0.0927 | 0.1149 | 0.0847 | |||
0.60 | I | β | 0.1512 | 0.0813 | 0.1433 | 0.1354 | 0.1325 | 0.0769 | 0.0947 | 0.0694 | |
θ | 0.1407 | 0.0962 | 0.1328 | 0.1249 | 0.1267 | 0.0908 | 0.1068 | 0.0785 | |||
0.80 | β | 0.1205 | 0.0809 | 0.1126 | 0.1047 | 0.1072 | 0.0729 | 0.0874 | 0.0632 | ||
θ | 0.1477 | 0.0922 | 0.1398 | 0.1319 | 0.1259 | 0.0896 | 0.1164 | 0.0856 | |||
0.40 | β | 0.1275 | 0.0769 | 0.1196 | 0.1117 | 0.1057 | 0.0747 | 0.0958 | 0.0721 | ||
θ | 0.1153 | 0.0707 | 0.1074 | 0.0995 | 0.0849 | 0.0685 | 0.0751 | 0.0643 | |||
80 | 0.60 | II | β | 0.1153 | 0.0707 | 0.1074 | 0.0995 | 0.0849 | 0.0709 | 0.0751 | 0.0641 |
θ | 0.1149 | 0.0758 | 0.1076 | 0.0991 | 0.1038 | 0.0726 | 0.0948 | 0.0746 | |||
0.80 | β | 0.0947 | 0.0665 | 0.0868 | 0.0789 | 0.0836 | 0.0605 | 0.0752 | 0.0598 | ||
θ | 0.1668 | 0.0972 | 0.1589 | 0.1517 | 0.1589 | 0.0927 | 0.1359 | 0.0874 | |||
0.40 | β | 0.1473 | 0.0819 | 0.1394 | 0.1315 | 0.1387 | 0.0753 | 0.1157 | 0.0721 | ||
θ | 0.1647 | 0.0965 | 0.1568 | 0.1489 | 0.1456 | 0.0905 | 0.1323 | 0.0859 | |||
0.60 | III | β | 0.1445 | 0.0817 | 0.1366 | 0.1287 | 0.1254 | 0.0734 | 0.1074 | 0.0731 | |
θ | 0.1377 | 0.0794 | 0.1298 | 0.1219 | 0.1256 | 0.0886 | 0.1068 | 0.0775 | |||
0.80 | β | 0.1175 | 0.0808 | 0.1096 | 0.1017 | 0.1054 | 0.0716 | 0.0857 | 0.0694 |
MLE | Boot | Bayes-SE | Bayes-GE | ||||||||
n | r | CS | θ, β | AB | MSE | AB | MSE | AB | MSE | AB | MSE |
I | |||||||||||
50 | 0.40 | θ | 0.2430 | 0.1402 | 0.2310 | 0.1332 | 0.2134 | 0.1102 | 0.1985 | 0.1003 | |
β | 0.2228 | 0.1246 | 0.2098 | 0.1176 | 0.1936 | 0.1501 | 0.1782 | 0.0849 | |||
0.60 | θ | 0.2311 | 0.1373 | 0.2181 | 0.1303 | 0.2005 | 0.1032 | 0.1867 | 0.0976 | ||
β | 0.2109 | 0.1168 | 0.1979 | 0.1098 | 0.1853 | 0.1368 | 0.1663 | 0.0861 | |||
0.80 | θ | 0.2110 | 0.1103 | 0.1980 | 0.1033 | 0.1816 | 0.0995 | 0.1764 | 0.0912 | ||
II | β | 0.1908 | 0.0951 | 0.1778 | 0.0881 | 0.1611 | 0.1168 | 0.1562 | 0.0759 | ||
0.40 | θ | 0.1964 | 0.1278 | 0.1834 | 0.1208 | 0.1866 | 0.1126 | 0.1807 | 0.0984 | ||
β | 0.1415 | 0.1118 | 0.1285 | 0.1048 | 0.1187 | 0.1663 | 0.1081 | 0.0837 | |||
0.60 | θ | 0.1943 | 0.1209 | 0.1813 | 0.1139 | 0.1834 | 0.1115 | 0.1782 | 0.0965 | ||
β | 0.1341 | 0.1027 | 0.1211 | 0.0957 | 0.1154 | 0.1145 | 0.1079 | 0.0805 | |||
0.80 | θ | 0.1924 | 0.1193 | 0.1794 | 0.1123 | 0.1815 | 0.1106 | 0.1764 | 0.0938 | ||
β | 0.1264 | 0.0865 | 0.1134 | 0.0795 | 0.1086 | 0.1434 | 0.0972 | 0.0763 | |||
0.40 | III | θ | 0.2405 | 0.1335 | 0.2275 | 0.1265 | 0.2022 | 0.1101 | 0.1843 | 0.1007 | |
β | 0.2202 | 0.1181 | 0.2072 | 0.1111 | 0.1823 | 0.1135 | 0.1641 | 0.0835 | |||
0.60 | θ | 0.2276 | 0.1300 | 0.2146 | 0.123 | 0.1987 | 0.1018 | 0.1806 | 0.0976 | ||
β | 0.2074 | 0.1112 | 0.1944 | 0.1042 | 0.1715 | 0.1171 | 0.1614 | 0.0835 | |||
0.80 | θ | 0.2134 | 0.1288 | 0.2004 | 0.1218 | 0.1927 | 0.0972 | 0.1765 | 0.0943 | ||
β | 0.1802 | 0.0878 | 0.1672 | 0.0808 | 0.1508 | 0.0963 | 0.1492 | 0.0753 | |||
0.40 | θ | 0.1803 | 0.0999 | 0.1723 | 0.0965 | 0.1732 | 0.0956 | 0.1332 | 0.0901 | ||
β | 0.1601 | 0.0846 | 0.1521 | 0.0812 | 0.1534 | 0.0807 | 0.1138 | 0.0748 | |||
0.60 | θ | 0.1711 | 0.0984 | 0.1631 | 0.0957 | 0.1587 | 0.0943 | 0.1213 | 0.0864 | ||
β | 0.1576 | 0.0829 | 0.1496 | 0.0795 | 0.1385 | 0.0785 | 0.1011 | 0.0711 | |||
0.80 | I | θ | 0.1471 | 0.0978 | 0.1391 | 0.0944 | 0.1332 | 0.0924 | 0.1132 | 0.0802 | |
β | 0.1269 | 0.0825 | 0.1189 | 0.0791 | 0.1137 | 0.0745 | 0.0938 | 0.0649 | |||
0.40 | θ | 0.1541 | 0.0938 | 0.1461 | 0.0904 | 0.1324 | 0.0906 | 0.1224 | 0.0873 | ||
β | 0.1339 | 0.0785 | 0.1259 | 0.0751 | 0.1122 | 0.0763 | 0.1022 | 0.0738 | |||
0.60 | θ | 0.1217 | 0.0723 | 0.1137 | 0.0689 | 0.0914 | 0.0701 | 0.0815 | 0.0657 | ||
80 | II | β | 0.1217 | 0.0723 | 0.1137 | 0.0689 | 0.0914 | 0.0725 | 0.0815 | 0.0658 | |
0.80 | θ | 0.1213 | 0.0774 | 0.1133 | 0.0748 | 0.1103 | 0.0742 | 0.1012 | 0.0763 | ||
β | 0.1011 | 0.0681 | 0.0931 | 0.0647 | 0.0901 | 0.0621 | 0.0816 | 0.0615 | |||
0.40 | θ | 0.1732 | 0.0988 | 0.1652 | 0.0954 | 0.1654 | 0.0943 | 0.1423 | 0.0891 | ||
β | 0.1537 | 0.0835 | 0.1457 | 0.0808 | 0.1452 | 0.0769 | 0.1221 | 0.0738 | |||
0.60 | θ | 0.1711 | 0.0981 | 0.1631 | 0.0947 | 0.1521 | 0.0921 | 0.1387 | 0.0867 | ||
III | β | 0.1509 | 0.0833 | 0.1429 | 0.0799 | 0.1319 | 0.0750 | 0.1138 | 0.0748 | ||
0.80 | θ | 0.1441 | 0.0810 | 0.1361 | 0.0790 | 0.1321 | 0.0902 | 0.1132 | 0.0792 | ||
β | 0.1239 | 0.0824 | 0.1159 | 0.0802 | 0.1119 | 0.0732 | 0.0921 | 0.0711 |
(θ=1, β=0.4) | (θ=1, β=0.8) | |||||||||
Asymptotic | Bootstrap | Credible | Asymptotic | Bootstrap | Credible | |||||
n | r | CS | θ, β | |||||||
0.40 | θ | 0.1927(99) | 0.1838(98) | 0.1774(97) | 0.1938(99) | 0.1852(98) | 0.1796(97) | |||
β | 0.1601(96) | 0.1528(95) | 0.1514(95) | 0.1628(96) | 0.1543(95) | 0.1521(95) | ||||
0.60 | I | θ | 0.1897(98) | 0.1827(98) | 0.1759(97) | 0.1887(98) | 0.1832(98) | 0.1780(97) | ||
β | 0.1594(96) | 0.1501(95) | 0.1492(95) | 0.1603(96) | 0.1522(95) | 0.1518(95) | ||||
0.80 | θ | 0.1842(98) | 0.1809(98) | 0.1743(97) | 0.1856(98) | 0.1827(98) | 0.1769(97) | |||
β | 0.1491(95) | 0.1483(95) | 0.1470(95) | 0.1528(95) | 0.1507(95) | 0.1493(95) | ||||
0.40 | θ | 0.1673(96) | 0.1568(96) | 0.1535(95) | 0.1694(96) | 0.1639(96) | 0.1571(96) | |||
β | 0.1347(94) | 0.1258(93) | 0.1275(93) | 0.1384(94) | 0.1330(94) | 0.1295(93) | ||||
50 | 0.60 | θ | 0.1643(96) | 0.1557(96) | 0.1526(95) | 0.1643(96) | 0.1618(96) | 0.1554(95) | ||
II | β | 0.1348(94) | 0.1231(93) | 0.1253(93) | 0.1359(94) | 0.1305(94) | 0.1292(93) | |||
0.80 | θ | 0.1588(96) | 0.1539(95) | 0.1504(95) | 0.1612(96) | 0.1614(96) | 0.1543(95) | |||
β | 0.1237(93) | 0.1213(93) | 0.1231(93) | 0.1284(93) | 0.1293(93) | 0.1267(93) | ||||
0.40 | θ | 0.1575(96) | 0.1583(96) | 0.1557(96) | 0.1618(96) | 0.1502(95) | 0.1446(95) | |||
β | 0.1249(93) | 0.1257(93) | 0.1231(92) | 0.1308(94) | 0.1193(92) | 0.1171(92) | ||||
0.60 | θ | 0.1545(95) | 0.1553(95) | 0.1527(95) | 0.1567(95) | 0.1482(95) | 0.1436(95) | |||
III | β | 0.1242(93) | 0.1255(93) | 0.1224(93) | 0.1283(93) | 0.1172(92) | 0.1168(92) | |||
0.80 | θ | 0.1493(95) | 0.1498(95) | 0.1472(95) | 0.1536(95) | 0.1477(95) | 0.1419(95) | |||
β | 0.1139(92) | 0.1147(92) | 0.1121(92) | 0.1208(93) | 0.1157(92) | 0.1143(92) | ||||
0.40 | θ | 0.1717(97) | 0.1598(96) | 0.1584(96) | 0.1749(97) | 0.1662(96) | 0.1626(96) | |||
β | 0.1391(94) | 0.1288(93) | 0.1324(94) | 0.1439(95) | 0.1353(94) | 0.1351(94) | ||||
0.60 | θ | 0.1687(97) | 0.1587(95) | 0.1569(95) | 0.1698(97) | 0.1642(96) | 0.1619(96) | |||
I | β | 0.1384(94) | 0.1261(93) | 0.1302(94) | 0.1414(95) | 0.1332(94) | 0.1348(94) | |||
0.80 | θ | 0.1632(96) | 0.1569(95) | 0.1553(95) | 0.1667(97) | 0.1637(96) | 0.1599(96) | |||
β | 0.1281(93) | 0.1243(93) | 0.1287(93) | 0.1339(94) | 0.1317(94) | 0.1323(95) | ||||
0.40 | θ | 0.1463(95) | 0.1328(94) | 0.1345(94) | 0.1505(95) | 0.1449(95) | 0.1401(95) | |||
β | 0.1137(94) | 0.1018(92) | 0.1085(93) | 0.1195(92) | 0.1148(92) | 0.1125(93) | ||||
0.60 | θ | 0.1433(95) | 0.1317(94) | 0.1336(94) | 0.1454(95) | 0.1428(95) | 0.1384(94) | |||
80 | II | β | 0.1138(93) | 0.0991(92) | 0.1063(93) | 0.1176(93) | 0.1115(93) | 0.1122(92) | ||
0.80 | θ | 0.1378(94) | 0.1299(93) | 0.1314(94) | 0.1423(95) | 0.1424(95) | 0.1373(94) | |||
β | 0.1027(92) | 0.0973(92) | 0.1041(93) | 0.1095(92) | 0.1103(93) | 0.1097(93) | ||||
0.40 | θ | 0.1365(94) | 0.1343(94) | 0.1367(94) | 0.1429(95) | 0.1312(94) | 0.1276(93) | |||
β | 0.1039(92) | 0.1017(92) | 0.1041(92) | 0.1119(92) | 0.1003(92) | 0.1001(92) | ||||
0.60 | θ | 0.1335(94) | 0.1313(94) | 0.1337(94) | 0.1378(94) | 0.1292(94) | 0.1262(93) | |||
III | β | 0.1032(92) | 0.1015(92) | 0.1034(93) | 0.1094(93) | 0.0982(92) | 0.0998(92) | |||
0.80 | θ | 0.1284(93) | 0.1258(93) | 0.1282(93) | 0.1347(94) | 0.1287(93) | 0.1249(93) | |||
β | 0.0929(91) | 0.0907(91) | 0.0931(91) | 0.1019(92) | 0.0967(92) | 0.0973(92) |
MLE | Boot | Bayes-SE | Bayes-GE | |||||||
n | r | CS | AB | MSE | AB | MSE | AB | MSE | AB | MSE |
50 | 0.40 | I | 0.2105 | 0.1223 | 0.2073 | 0.1158 | 0.1913 | 0.1488 | 0.1738 | 0.0823 |
0.60 | 0.2077 | 0.1204 | 0.1968 | 0.1131 | 0.1892 | 0.1436 | 0.1718 | 0.0802 | ||
0.80 | 0.1968 | 0.1185 | 0.1934 | 0.1094 | 0.1827 | 0.1415 | 0.169 | 0.0792 | ||
0.40 | II | 0.1907 | 0.1176 | 0.1908 | 0.1066 | 0.1774 | 0.1403 | 0.1675 | 0.0787 | |
0.60 | 0.1899 | 0.1134 | 0.1874 | 0.1063 | 0.1742 | 0.1395 | 0.1651 | 0.0763 | ||
0.80 | 0.1873 | 0.1117 | 0.1856 | 0.1022 | 0.1721 | 0.1369 | 0.1628 | 0.0754 | ||
0.40 | III | 0.1865 | 0.1109 | 0.1832 | 0.1007 | 0.1711 | 0.1329 | 0.1602 | 0.073 | |
0.60 | 0.1842 | 0.1085 | 0.1818 | 0.1001 | 0.1702 | 0.1311 | 0.1589 | 0.0712 | ||
0.80 | 0.1827 | 0.1043 | 0.1806 | 0.0972 | 0.1685 | 0.1300 | 0.1571 | 0.0695 | ||
80 | 0.40 | I | 0.1812 | 0.1012 | 0.1799 | 0.0961 | 0.1642 | 0.1289 | 0.1547 | 0.0632 |
0.60 | 0.1793 | 0.1002 | 0.1749 | 0.0943 | 0.1638 | 0.1254 | 0.1518 | 0.0611 | ||
0.80 | 0.1772 | 0.0988 | 0.1727 | 0.0916 | 0.1587 | 0.1231 | 0.1500 | 0.0603 | ||
0.40 | II | 0.1744 | 0.0965 | 0.1708 | 0.0907 | 0.1548 | 0.1225 | 0.1478 | 0.0591 | |
0.60 | 0.1717 | 0.0938 | 0.1693 | 0.0874 | 0.1525 | 0.1206 | 0.1465 | 0.0532 | ||
0.80 | 0.1708 | 0.0916 | 0.1635 | 0.0844 | 0.1501 | 0.1191 | 0.1451 | 0.0521 | ||
0.40 | III | 0.1689 | 0.0901 | 0.1622 | 0.081 | 0.1492 | 0.1131 | 0.1438 | 0.0516 | |
0.60 | 0.1646 | 0.0883 | 0.1601 | 0.0792 | 0.1461 | 0.1115 | 0.1421 | 0.0500 | ||
0.80 | 0.1622 | 0.0861 | 0.1593 | 0.0774 | 0.1448 | 0.1109 | 0.1413 | 0.0492 |
Asymptotic | Bootstrap | Credible | HPD Credible | ||||||||||
n | r | CS | AIL | CP | AIL | CP | AIL | CP | AIL | CP | |||
50 | 0.40 | I | 0.1624 | 98% | 0.1539 | 97% | 0.1520 | 97% | 0.1492 | 96% | |||
0.60 | 0.1617 | 98% | 0.1523 | 96% | 0.1518 | 96% | 0.1467 | 96% | |||||
0.80 | 0.1596 | 97% | 0.1517 | 96% | 0.1502 | 96% | 0.1443 | 96% | |||||
0.40 | II | 0.1576 | 97% | 0.1508 | 96% | 0.1495 | 96% | 0.1437 | 95% | ||||
0.60 | 0.1549 | 97% | 0.1496 | 96% | 0.1472 | 96% | 0.1428 | 95% | |||||
0.80 | 0.1538 | 97% | 0.1477 | 96% | 0.1447 | 96% | 0.1419 | 95% | |||||
0.40 | III | 0.1521 | 96% | 0.1468 | 96% | 0.1432 | 96% | 0.1405 | 95% | ||||
0.60 | 0.1510 | 96% | 0.1443 | 96% | 0.1429 | 95% | 0.1388 | 95% | |||||
0.80 | 0.1502 | 96% | 0.1439 | 96% | 0.1406 | 95% | 0.1382 | 94% | |||||
80 | 0.40 | I | 0.1487 | 96% | 0.1421 | 95% | 0.1386 | 95% | 0.1374 | 94% | |||
0.60 | 0.1476 | 96% | 0.1414 | 95% | 0.1378 | 95% | 0.1367 | 94% | |||||
0.80 | 0.1468 | 96% | 0.1409 | 95% | 0.1363 | 95% | 0.1359 | 94% | |||||
0.40 | II | 0.1455 | 96% | 0.1396 | 95% | 0.1348 | 95% | 0.1345 | 94% | ||||
0.60 | 0.1447 | 96% | 0.1383 | 95% | 0.1339 | 95% | 0.1338 | 94% | |||||
0.80 | 0.1436 | 95% | 0.1375 | 95% | 0.1325 | 94% | 0.1329 | 93% | |||||
0.40 | III | 0.1424 | 95% | 0.1367 | 95% | 0.1320 | 94% | 0.1315 | 93% | ||||
0.60 | 0.1388 | 95% | 0.1359 | 94% | 0.1311 | 93% | 0.1306 | 92% | |||||
0.80 | 0.1374 | 95% | 0.1343 | 94% | 0.1308 | 93% | 0.1289 | 92% |
Scheme: (2, 0∗20, 2, 0∗20) | ||||||||||||||
0.39 | 1.08 | 1.25 | 1.47 | 1.57 | 1.61 | 1.69 | 1.80 | 1.84 | 1.87 | 1.89 | 2.03 | 2.03 | 2.05 | 2.12 |
2.35 | 2.41 | 2.43 | 2.48 | 2.50 | 2.53 | 2.55 | 2.55 | 2.59 | 2.67 | 2.74 | 2.79 | 2.81 | 2.82 | 2.85 |
2.87 | 2.88 | 2.93 | 2.95 | 2.96 | 2.97 | 3.09 | 3.11 | 3.11 | 3.15 | |||||
Scheme: (8, 0∗25, 8, 0∗15) | ||||||||||||||
0.39 | 0.85 | 1.25 | 1.47 | 1.61 | 1.80 | 1.87 | 2.03 | 2.12 | 2.35 | 2.41 | 2.43 | 2.48 | 2.50 | 2.53 |
2.55 | 2.55 | 2.56 | 2.59 | 2.67 | 2.73 | 2.74 | 2.79 | 2.81 | 2.82 | 2.93 | 2.95 | 2.97 | 3.11 | 3.15 |
3.19 | 3.22 | 3.28 | 3.31 | 3.31 | 3.33 | 3.39 | 3.39 | 3.56 | 3.60 |
Relative efficiency | Absolute difference lengths(ABLs) | |||||||
parameter | CS | MLE | Bayes-SE | Bayes-GE | Bootstrap | Asymptotic | Credible | Bootstrap |
θ | (2,0∗20,2,0∗20) | 0.9832 | 0.9851 | 0.9876 | 0.9842 | 0.0964 | 0.0961 | 0.0955 |
(8,0∗25,8,0∗15) | 0.9706 | 0.9723 | 0.9735 | 0.9714 | 0.101 | 0.0985 | 0.0971 | |
β | (2,0∗20,2,0∗20) | 0.9864 | 0.9896 | 0.9902 | 0.9876 | 0.0935 | 0.0927 | 0.0919 |
(8,0∗25,8,0∗15) | 0.9726 | 0.9745 | 0.9813 | 0.9753 | 0.0982 | 0.0962 | 0.0945 |
MLE | Boot | Bayes-SE | Bayes-GE | ||||||
(n, m) | sc | AB | MSE | AB | MSE | AB | MSE | AB | MSE |
T= 1 | |||||||||
(40, 15) | I | 0.2741 | 0.1801 | 0.2283 | 0.1334 | 0.2321 | 0.1201 | 0.2172 | 0.1183 |
II | 0.1918 | 0.1310 | 0.1403 | 0.1078 | 0.1306 | 0.1042 | 0.1300 | 0.1026 | |
III | 0.2631 | 0.1655 | 0.2202 | 0.1263 | 0.2019 | 0.1184 | 0.1987 | 0.1109 | |
(40, 20) | I | 0.2543 | 0.1415 | 0.2142 | 0.1278 | 0.2115 | 0.1106 | 0.2064 | 0.1095 |
II | 0.1831 | 0.1296 | 0.1392 | 0.1001 | 0.1295 | 0.0995 | 0.1143 | 0.0912 | |
III | 0.2428 | 0.1401 | 0.2137 | 0.1206 | 0.2001 | 0.1100 | 0.1988 | 0.1066 | |
(60, 20) | I | 0.1778 | 0.0982 | 0.1587 | 0.0943 | 0.1213 | 0.0864 | 0.1171 | 0.0776 |
II | 0.1412 | 0.0876 | 0.1116 | 0.0851 | 0.1017 | 0.0811 | 0.0958 | 0.0658 | |
III | 0.1711 | 0.0986 | 0.1521 | 0.0952 | 0.1201 | 0.0843 | 0.1104 | 0.1104 | |
(60, 40) | I | 0.1471 | 0.0978 | 0.1332 | 0.0924 | 0.1132 | 0.0802 | 0.1089 | 0.0704 |
II | 0.1213 | 0.0774 | 0.1103 | 0.0742 | 0.1012 | 0.0763 | 0.0933 | 0.0643 | |
III | 0.1441 | 0.0976 | 0.1321 | 0.0902 | 0.1123 | 0.0792 | 0.1118 | 0.0703 | |
T=2 | |||||||||
(40, 15) | I | 0.2077 | 0.1106 | 0.1821 | 0.0817 | 0.1631 | 0.0763 | 0.1022 | 0.0632 |
II | 0.1309 | 0.0958 | 0.1122 | 0.0784 | 0.0997 | 0.0743 | 0.0881 | 0.0639 | |
III | 0.2042 | 0.1057 | 0.1678 | 0.0806 | 0.1578 | 0.0773 | 0.1101 | 0.0655 | |
(40, 20) | I | 0.1876 | 0.0888 | 0.1579 | 0.0781 | 0.1532 | 0.0697 | 0.1003 | 0.0615 |
II | 0.1232 | 0.0803 | 0.1054 | 0.0731 | 0.0943 | 0.0699 | 0.0805 | 00629 | |
III | 0.1774 | 0.0816 | 0.1476 | 0.0775 | 0.1460 | 0.0691 | 0.1022 | 0.0637 | |
(60, 20) | I | 0.1544 | 0.0767 | 0.1353 | 0.0728 | 0.0979 | 0.0649 | 0.0925 | 0.0608 |
II | 0.1178 | 0.0661 | 0.0882 | 0.0636 | 0.0783 | 0.0596 | 0.0766 | 0.0588 | |
III | 0.1477 | 0.0771 | 0.1287 | 0.0737 | 0.0967 | 0.0628 | 0.0931 | 0.0622 | |
(60, 40) | I | 0.1237 | 0.0763 | 0.1098 | 0.0709 | 0.0898 | 0.0587 | 0.0832 | 0.0580 |
II | 0.0979 | 0.0559 | 0.0869 | 0.0527 | 0.0778 | 0.0548 | 0.0751 | 0.0510 | |
III | 0.1207 | 0.0761 | 0.1087 | 0.0687 | 0.0889 | 0.0577 | 0.0812 | 0. |
Asymptotic | Bootstrap | Credible | HPD Credible | ||||||
(n, m) | sc | AL | CP | AL | CP | AL | CP | AL | CP |
T= 1 | |||||||||
(40, 15) | I | 0.2107 | 0.9684 | 0.2006 | 0.9624 | 0.1906 | 0.9618 | 0.1892 | 0.9541 |
II | 0.1978 | 0.9634 | 0.1917 | 0.9593 | 0.1893 | 0.9582 | 0.1831 | 0.9516 | |
I | 0.1764 | 0.9581 | 0.1723 | 0.9580 | 0.1702 | 0.9567 | 0.1694 | 0.9508 | |
(40, 20) | I | 0.2064 | 0.9625 | 0.1901 | 0.9624 | 0.1876 | 0.9610 | 0.1807 | 0.9538 |
II | 0.1908 | 0.9612 | 0.1829 | 0.9584 | 0.1751 | 0.9564 | 0.1688 | 0.9512 | |
I | 0.1732 | 0.9572 | 0.1705 | 0.9579 | 0.1693 | 0.9541 | 0.1604 | 0.9506 | |
(60, 20) | I | 0.1902 | 0.9615 | 0.1823 | 0.9619 | 0.1811 | 0.9540 | 0.1773 | 0.9533 |
II | 0.1764 | 0.9545 | 0.1631 | 0.9546 | 0.1605 | 0.9519 | 0.1587 | 0.9507 | |
I | 0.1578 | 0.9550 | 0.1509 | 0.9570 | 0.1491 | 0.9508 | 0.1432 | 0.9501 | |
(60, 40) | I | 0.1812 | 0.9614 | 0.1720 | 0.9604 | 0.1704 | 0.9536 | 0.1701 | 0.9512 |
II | 0.1721 | 0.9601 | 0.1584 | 0.9531 | 0.1515 | 0.9511 | 0.1508 | 0.9503 | |
I | 0.1354 | 0.9544 | 0.1417 | 0.9514 | 0.1401 | 0.9502 | 0.1372 | 0.9492 | |
T=2 | |||||||||
(40, 15) | I | 0.1859 | 0.9512 | 0.1658 | 0.9452 | 0.1859 | 0.9446 | 0.1698 | 0.9431 |
II | 0.1730 | 0.9462 | 0.1645 | 0.9421 | 0.1733 | 0.9412 | 0.1496 | 0.9410 | |
I | 0.1516 | 0.9409 | 0.1454 | 0.9408 | 0.1516 | 0.9395 | 0.1321 | 0.9352 | |
(40, 20) | I | 0.1816 | 0.9453 | 0.1628 | 0.9452 | 0.1816 | 0.9438 | 0.1605 | 0.9428 |
II | 0.1662 | 0.9444 | 0.1503 | 0.9412 | 0.1662 | 0.9392 | 0.1433 | 0.9367 | |
I | 0.1484 | 0.9438 | 0.1445 | 0.9407 | 0.1484 | 0.9369 | 0.1289 | 0.9301 | |
(60, 20) | I | 0.1654 | 0.9443 | 0.1563 | 0.9447 | 0.1654 | 0.9368 | 0.1573 | 0.9307 |
II | 0.1516 | 0.9373 | 0.1357 | 0.9374 | 0.1516 | 0.9347 | 0.1411 | 0.9289 | |
I | 0.1332 | 0.9378 | 0.1243 | 0.9398 | 0.1332 | 0.9336 | 0.1275 | 0.9208 | |
(60, 40) | I | 0.1564 | 0.9442 | 0.1456 | 0.9432 | 0.1564 | 0.9364 | 0.1493 | 0.9110 |
II | 0.1473 | 0.9429 | 0.1267 | 0.9359 | 0.1473 | 0.9339 | 0.1388 | 0.9108 | |
I | 0.1106 | 0.9372 | 0.1153 | 0.9342 | 0.1106 | 0.9331 | 0.1010 | 0.9072 |
MLE | Boot | Bayes-SE | Bayes-GE | ||||||||
n | r | CS | θ, β | AB | MSE | AB | MSE | AB | MSE | AB | MSE |
0.40 | θ | 0.2372 | 0.1372 | 0.2253 | 0.1318 | 0.2078 | 0.1086 | 0.1926 | 0.0984 | ||
β | 0.2175 | 0.1214 | 0.2041 | 0.1162 | 0.1883 | 0.1485 | 0.1723 | 0.0835 | |||
0.60 | I | θ | 0.2253 | 0.1341 | 0.2124 | 0.1289 | 0.1949 | 0.1016 | 0.1808 | 0.0957 | |
β | 0.2051 | 0.1136 | 0.1922 | 0.1084 | 0.1797 | 0.1352 | 0.1604 | 0.0842 | |||
0.80 | θ | 0.2052 | 0.1071 | 0.1923 | 0.1019 | 0.1768 | 0.0979 | 0.1705 | 0.0893 | ||
β | 0.1853 | 0.0919 | 0.1721 | 0.0867 | 0.1555 | 0.1152 | 0.1503 | 0.0748 | |||
0.40 | θ | 0.1906 | 0.1246 | 0.1777 | 0.1194 | 0.1816 | 0.1114 | 0.1748 | 0.0965 | ||
β | 0.1357 | 0.1086 | 0.1228 | 0.1034 | 0.1131 | 0.1647 | 0.1022 | 0.0818 | |||
50 | 0.60 | II | θ | 0.1885 | 0.1177 | 0.1756 | 0.1125 | 0.1778 | 0.1099 | 0.1723 | 0.0946 |
β | 0.1283 | 0.0995 | 0.1154 | 0.0943 | 0.1098 | 0.1129 | 0.1027 | 0.0786 | |||
0.80 | θ | 0.1866 | 0.1161 | 0.1737 | 0.1109 | 0.1759 | 0.1094 | 0.1705 | 0.0919 | ||
β | 0.1206 | 0.0833 | 0.1077 | 0.0781 | 0.1035 | 0.1418 | 0.0913 | 0.0744 | |||
θ | 0.2347 | 0.1303 | 0.2218 | 0.1251 | 0.1966 | 0.0121 | 0.1784 | 0.0988 | |||
0.40 | β | 0.2144 | 0.1149 | 0.2015 | 0.1097 | 0.1767 | 0.1002 | 0.1582 | 0.0816 | ||
θ | 0.2218 | 0.1268 | 0.2089 | 0.1216 | 0.1931 | 0.1155 | 0.1747 | 0.0957 | |||
0.60 | III | β | 0.2016 | 0.1087 | 0.1887 | 0.1028 | 0.1659 | 0.0956 | 0.1555 | 0.0816 | |
θ | 0.2076 | 0.1256 | 0.1947 | 0.1204 | 0.1871 | 0.0947 | 0.1706 | 0.0924 | |||
0.80 | β | 0.1744 | 0.0846 | 0.1615 | 0.0794 | 0.1452 | 0.0942 | 0.1433 | 0.0734 | ||
θ | 0.1739 | 0.0983 | 0.166 | 0.1581 | 0.1667 | 0.0942 | 0.1268 | 0.0884 | |||
0.40 | β | 0.1537 | 0.0834 | 0.1458 | 0.1379 | 0.1469 | 0.0791 | 0.1074 | 0.0731 | ||
θ | 0.1647 | 0.0968 | 0.1568 | 0.1489 | 0.1522 | 0.0927 | 0.1149 | 0.0847 | |||
0.60 | I | β | 0.1512 | 0.0813 | 0.1433 | 0.1354 | 0.1325 | 0.0769 | 0.0947 | 0.0694 | |
θ | 0.1407 | 0.0962 | 0.1328 | 0.1249 | 0.1267 | 0.0908 | 0.1068 | 0.0785 | |||
0.80 | β | 0.1205 | 0.0809 | 0.1126 | 0.1047 | 0.1072 | 0.0729 | 0.0874 | 0.0632 | ||
θ | 0.1477 | 0.0922 | 0.1398 | 0.1319 | 0.1259 | 0.0896 | 0.1164 | 0.0856 | |||
0.40 | β | 0.1275 | 0.0769 | 0.1196 | 0.1117 | 0.1057 | 0.0747 | 0.0958 | 0.0721 | ||
θ | 0.1153 | 0.0707 | 0.1074 | 0.0995 | 0.0849 | 0.0685 | 0.0751 | 0.0643 | |||
80 | 0.60 | II | β | 0.1153 | 0.0707 | 0.1074 | 0.0995 | 0.0849 | 0.0709 | 0.0751 | 0.0641 |
θ | 0.1149 | 0.0758 | 0.1076 | 0.0991 | 0.1038 | 0.0726 | 0.0948 | 0.0746 | |||
0.80 | β | 0.0947 | 0.0665 | 0.0868 | 0.0789 | 0.0836 | 0.0605 | 0.0752 | 0.0598 | ||
θ | 0.1668 | 0.0972 | 0.1589 | 0.1517 | 0.1589 | 0.0927 | 0.1359 | 0.0874 | |||
0.40 | β | 0.1473 | 0.0819 | 0.1394 | 0.1315 | 0.1387 | 0.0753 | 0.1157 | 0.0721 | ||
θ | 0.1647 | 0.0965 | 0.1568 | 0.1489 | 0.1456 | 0.0905 | 0.1323 | 0.0859 | |||
0.60 | III | β | 0.1445 | 0.0817 | 0.1366 | 0.1287 | 0.1254 | 0.0734 | 0.1074 | 0.0731 | |
θ | 0.1377 | 0.0794 | 0.1298 | 0.1219 | 0.1256 | 0.0886 | 0.1068 | 0.0775 | |||
0.80 | β | 0.1175 | 0.0808 | 0.1096 | 0.1017 | 0.1054 | 0.0716 | 0.0857 | 0.0694 |
MLE | Boot | Bayes-SE | Bayes-GE | ||||||||
n | r | CS | θ, β | AB | MSE | AB | MSE | AB | MSE | AB | MSE |
I | |||||||||||
50 | 0.40 | θ | 0.2430 | 0.1402 | 0.2310 | 0.1332 | 0.2134 | 0.1102 | 0.1985 | 0.1003 | |
β | 0.2228 | 0.1246 | 0.2098 | 0.1176 | 0.1936 | 0.1501 | 0.1782 | 0.0849 | |||
0.60 | θ | 0.2311 | 0.1373 | 0.2181 | 0.1303 | 0.2005 | 0.1032 | 0.1867 | 0.0976 | ||
β | 0.2109 | 0.1168 | 0.1979 | 0.1098 | 0.1853 | 0.1368 | 0.1663 | 0.0861 | |||
0.80 | θ | 0.2110 | 0.1103 | 0.1980 | 0.1033 | 0.1816 | 0.0995 | 0.1764 | 0.0912 | ||
II | β | 0.1908 | 0.0951 | 0.1778 | 0.0881 | 0.1611 | 0.1168 | 0.1562 | 0.0759 | ||
0.40 | θ | 0.1964 | 0.1278 | 0.1834 | 0.1208 | 0.1866 | 0.1126 | 0.1807 | 0.0984 | ||
β | 0.1415 | 0.1118 | 0.1285 | 0.1048 | 0.1187 | 0.1663 | 0.1081 | 0.0837 | |||
0.60 | θ | 0.1943 | 0.1209 | 0.1813 | 0.1139 | 0.1834 | 0.1115 | 0.1782 | 0.0965 | ||
β | 0.1341 | 0.1027 | 0.1211 | 0.0957 | 0.1154 | 0.1145 | 0.1079 | 0.0805 | |||
0.80 | θ | 0.1924 | 0.1193 | 0.1794 | 0.1123 | 0.1815 | 0.1106 | 0.1764 | 0.0938 | ||
β | 0.1264 | 0.0865 | 0.1134 | 0.0795 | 0.1086 | 0.1434 | 0.0972 | 0.0763 | |||
0.40 | III | θ | 0.2405 | 0.1335 | 0.2275 | 0.1265 | 0.2022 | 0.1101 | 0.1843 | 0.1007 | |
β | 0.2202 | 0.1181 | 0.2072 | 0.1111 | 0.1823 | 0.1135 | 0.1641 | 0.0835 | |||
0.60 | θ | 0.2276 | 0.1300 | 0.2146 | 0.123 | 0.1987 | 0.1018 | 0.1806 | 0.0976 | ||
β | 0.2074 | 0.1112 | 0.1944 | 0.1042 | 0.1715 | 0.1171 | 0.1614 | 0.0835 | |||
0.80 | θ | 0.2134 | 0.1288 | 0.2004 | 0.1218 | 0.1927 | 0.0972 | 0.1765 | 0.0943 | ||
β | 0.1802 | 0.0878 | 0.1672 | 0.0808 | 0.1508 | 0.0963 | 0.1492 | 0.0753 | |||
0.40 | θ | 0.1803 | 0.0999 | 0.1723 | 0.0965 | 0.1732 | 0.0956 | 0.1332 | 0.0901 | ||
β | 0.1601 | 0.0846 | 0.1521 | 0.0812 | 0.1534 | 0.0807 | 0.1138 | 0.0748 | |||
0.60 | θ | 0.1711 | 0.0984 | 0.1631 | 0.0957 | 0.1587 | 0.0943 | 0.1213 | 0.0864 | ||
β | 0.1576 | 0.0829 | 0.1496 | 0.0795 | 0.1385 | 0.0785 | 0.1011 | 0.0711 | |||
0.80 | I | θ | 0.1471 | 0.0978 | 0.1391 | 0.0944 | 0.1332 | 0.0924 | 0.1132 | 0.0802 | |
β | 0.1269 | 0.0825 | 0.1189 | 0.0791 | 0.1137 | 0.0745 | 0.0938 | 0.0649 | |||
0.40 | θ | 0.1541 | 0.0938 | 0.1461 | 0.0904 | 0.1324 | 0.0906 | 0.1224 | 0.0873 | ||
β | 0.1339 | 0.0785 | 0.1259 | 0.0751 | 0.1122 | 0.0763 | 0.1022 | 0.0738 | |||
0.60 | θ | 0.1217 | 0.0723 | 0.1137 | 0.0689 | 0.0914 | 0.0701 | 0.0815 | 0.0657 | ||
80 | II | β | 0.1217 | 0.0723 | 0.1137 | 0.0689 | 0.0914 | 0.0725 | 0.0815 | 0.0658 | |
0.80 | θ | 0.1213 | 0.0774 | 0.1133 | 0.0748 | 0.1103 | 0.0742 | 0.1012 | 0.0763 | ||
β | 0.1011 | 0.0681 | 0.0931 | 0.0647 | 0.0901 | 0.0621 | 0.0816 | 0.0615 | |||
0.40 | θ | 0.1732 | 0.0988 | 0.1652 | 0.0954 | 0.1654 | 0.0943 | 0.1423 | 0.0891 | ||
β | 0.1537 | 0.0835 | 0.1457 | 0.0808 | 0.1452 | 0.0769 | 0.1221 | 0.0738 | |||
0.60 | θ | 0.1711 | 0.0981 | 0.1631 | 0.0947 | 0.1521 | 0.0921 | 0.1387 | 0.0867 | ||
III | β | 0.1509 | 0.0833 | 0.1429 | 0.0799 | 0.1319 | 0.0750 | 0.1138 | 0.0748 | ||
0.80 | θ | 0.1441 | 0.0810 | 0.1361 | 0.0790 | 0.1321 | 0.0902 | 0.1132 | 0.0792 | ||
β | 0.1239 | 0.0824 | 0.1159 | 0.0802 | 0.1119 | 0.0732 | 0.0921 | 0.0711 |
(θ=1, β=0.4) | (θ=1, β=0.8) | |||||||||
Asymptotic | Bootstrap | Credible | Asymptotic | Bootstrap | Credible | |||||
n | r | CS | θ, β | |||||||
0.40 | θ | 0.1927(99) | 0.1838(98) | 0.1774(97) | 0.1938(99) | 0.1852(98) | 0.1796(97) | |||
β | 0.1601(96) | 0.1528(95) | 0.1514(95) | 0.1628(96) | 0.1543(95) | 0.1521(95) | ||||
0.60 | I | θ | 0.1897(98) | 0.1827(98) | 0.1759(97) | 0.1887(98) | 0.1832(98) | 0.1780(97) | ||
β | 0.1594(96) | 0.1501(95) | 0.1492(95) | 0.1603(96) | 0.1522(95) | 0.1518(95) | ||||
0.80 | θ | 0.1842(98) | 0.1809(98) | 0.1743(97) | 0.1856(98) | 0.1827(98) | 0.1769(97) | |||
β | 0.1491(95) | 0.1483(95) | 0.1470(95) | 0.1528(95) | 0.1507(95) | 0.1493(95) | ||||
0.40 | θ | 0.1673(96) | 0.1568(96) | 0.1535(95) | 0.1694(96) | 0.1639(96) | 0.1571(96) | |||
β | 0.1347(94) | 0.1258(93) | 0.1275(93) | 0.1384(94) | 0.1330(94) | 0.1295(93) | ||||
50 | 0.60 | θ | 0.1643(96) | 0.1557(96) | 0.1526(95) | 0.1643(96) | 0.1618(96) | 0.1554(95) | ||
II | β | 0.1348(94) | 0.1231(93) | 0.1253(93) | 0.1359(94) | 0.1305(94) | 0.1292(93) | |||
0.80 | θ | 0.1588(96) | 0.1539(95) | 0.1504(95) | 0.1612(96) | 0.1614(96) | 0.1543(95) | |||
β | 0.1237(93) | 0.1213(93) | 0.1231(93) | 0.1284(93) | 0.1293(93) | 0.1267(93) | ||||
0.40 | θ | 0.1575(96) | 0.1583(96) | 0.1557(96) | 0.1618(96) | 0.1502(95) | 0.1446(95) | |||
β | 0.1249(93) | 0.1257(93) | 0.1231(92) | 0.1308(94) | 0.1193(92) | 0.1171(92) | ||||
0.60 | θ | 0.1545(95) | 0.1553(95) | 0.1527(95) | 0.1567(95) | 0.1482(95) | 0.1436(95) | |||
III | β | 0.1242(93) | 0.1255(93) | 0.1224(93) | 0.1283(93) | 0.1172(92) | 0.1168(92) | |||
0.80 | θ | 0.1493(95) | 0.1498(95) | 0.1472(95) | 0.1536(95) | 0.1477(95) | 0.1419(95) | |||
β | 0.1139(92) | 0.1147(92) | 0.1121(92) | 0.1208(93) | 0.1157(92) | 0.1143(92) | ||||
0.40 | θ | 0.1717(97) | 0.1598(96) | 0.1584(96) | 0.1749(97) | 0.1662(96) | 0.1626(96) | |||
β | 0.1391(94) | 0.1288(93) | 0.1324(94) | 0.1439(95) | 0.1353(94) | 0.1351(94) | ||||
0.60 | θ | 0.1687(97) | 0.1587(95) | 0.1569(95) | 0.1698(97) | 0.1642(96) | 0.1619(96) | |||
I | β | 0.1384(94) | 0.1261(93) | 0.1302(94) | 0.1414(95) | 0.1332(94) | 0.1348(94) | |||
0.80 | θ | 0.1632(96) | 0.1569(95) | 0.1553(95) | 0.1667(97) | 0.1637(96) | 0.1599(96) | |||
β | 0.1281(93) | 0.1243(93) | 0.1287(93) | 0.1339(94) | 0.1317(94) | 0.1323(95) | ||||
0.40 | θ | 0.1463(95) | 0.1328(94) | 0.1345(94) | 0.1505(95) | 0.1449(95) | 0.1401(95) | |||
β | 0.1137(94) | 0.1018(92) | 0.1085(93) | 0.1195(92) | 0.1148(92) | 0.1125(93) | ||||
0.60 | θ | 0.1433(95) | 0.1317(94) | 0.1336(94) | 0.1454(95) | 0.1428(95) | 0.1384(94) | |||
80 | II | β | 0.1138(93) | 0.0991(92) | 0.1063(93) | 0.1176(93) | 0.1115(93) | 0.1122(92) | ||
0.80 | θ | 0.1378(94) | 0.1299(93) | 0.1314(94) | 0.1423(95) | 0.1424(95) | 0.1373(94) | |||
β | 0.1027(92) | 0.0973(92) | 0.1041(93) | 0.1095(92) | 0.1103(93) | 0.1097(93) | ||||
0.40 | θ | 0.1365(94) | 0.1343(94) | 0.1367(94) | 0.1429(95) | 0.1312(94) | 0.1276(93) | |||
β | 0.1039(92) | 0.1017(92) | 0.1041(92) | 0.1119(92) | 0.1003(92) | 0.1001(92) | ||||
0.60 | θ | 0.1335(94) | 0.1313(94) | 0.1337(94) | 0.1378(94) | 0.1292(94) | 0.1262(93) | |||
III | β | 0.1032(92) | 0.1015(92) | 0.1034(93) | 0.1094(93) | 0.0982(92) | 0.0998(92) | |||
0.80 | θ | 0.1284(93) | 0.1258(93) | 0.1282(93) | 0.1347(94) | 0.1287(93) | 0.1249(93) | |||
β | 0.0929(91) | 0.0907(91) | 0.0931(91) | 0.1019(92) | 0.0967(92) | 0.0973(92) |
MLE | Boot | Bayes-SE | Bayes-GE | |||||||
n | r | CS | AB | MSE | AB | MSE | AB | MSE | AB | MSE |
50 | 0.40 | I | 0.2105 | 0.1223 | 0.2073 | 0.1158 | 0.1913 | 0.1488 | 0.1738 | 0.0823 |
0.60 | 0.2077 | 0.1204 | 0.1968 | 0.1131 | 0.1892 | 0.1436 | 0.1718 | 0.0802 | ||
0.80 | 0.1968 | 0.1185 | 0.1934 | 0.1094 | 0.1827 | 0.1415 | 0.169 | 0.0792 | ||
0.40 | II | 0.1907 | 0.1176 | 0.1908 | 0.1066 | 0.1774 | 0.1403 | 0.1675 | 0.0787 | |
0.60 | 0.1899 | 0.1134 | 0.1874 | 0.1063 | 0.1742 | 0.1395 | 0.1651 | 0.0763 | ||
0.80 | 0.1873 | 0.1117 | 0.1856 | 0.1022 | 0.1721 | 0.1369 | 0.1628 | 0.0754 | ||
0.40 | III | 0.1865 | 0.1109 | 0.1832 | 0.1007 | 0.1711 | 0.1329 | 0.1602 | 0.073 | |
0.60 | 0.1842 | 0.1085 | 0.1818 | 0.1001 | 0.1702 | 0.1311 | 0.1589 | 0.0712 | ||
0.80 | 0.1827 | 0.1043 | 0.1806 | 0.0972 | 0.1685 | 0.1300 | 0.1571 | 0.0695 | ||
80 | 0.40 | I | 0.1812 | 0.1012 | 0.1799 | 0.0961 | 0.1642 | 0.1289 | 0.1547 | 0.0632 |
0.60 | 0.1793 | 0.1002 | 0.1749 | 0.0943 | 0.1638 | 0.1254 | 0.1518 | 0.0611 | ||
0.80 | 0.1772 | 0.0988 | 0.1727 | 0.0916 | 0.1587 | 0.1231 | 0.1500 | 0.0603 | ||
0.40 | II | 0.1744 | 0.0965 | 0.1708 | 0.0907 | 0.1548 | 0.1225 | 0.1478 | 0.0591 | |
0.60 | 0.1717 | 0.0938 | 0.1693 | 0.0874 | 0.1525 | 0.1206 | 0.1465 | 0.0532 | ||
0.80 | 0.1708 | 0.0916 | 0.1635 | 0.0844 | 0.1501 | 0.1191 | 0.1451 | 0.0521 | ||
0.40 | III | 0.1689 | 0.0901 | 0.1622 | 0.081 | 0.1492 | 0.1131 | 0.1438 | 0.0516 | |
0.60 | 0.1646 | 0.0883 | 0.1601 | 0.0792 | 0.1461 | 0.1115 | 0.1421 | 0.0500 | ||
0.80 | 0.1622 | 0.0861 | 0.1593 | 0.0774 | 0.1448 | 0.1109 | 0.1413 | 0.0492 |
Asymptotic | Bootstrap | Credible | HPD Credible | ||||||||||
n | r | CS | AIL | CP | AIL | CP | AIL | CP | AIL | CP | |||
50 | 0.40 | I | 0.1624 | 98% | 0.1539 | 97% | 0.1520 | 97% | 0.1492 | 96% | |||
0.60 | 0.1617 | 98% | 0.1523 | 96% | 0.1518 | 96% | 0.1467 | 96% | |||||
0.80 | 0.1596 | 97% | 0.1517 | 96% | 0.1502 | 96% | 0.1443 | 96% | |||||
0.40 | II | 0.1576 | 97% | 0.1508 | 96% | 0.1495 | 96% | 0.1437 | 95% | ||||
0.60 | 0.1549 | 97% | 0.1496 | 96% | 0.1472 | 96% | 0.1428 | 95% | |||||
0.80 | 0.1538 | 97% | 0.1477 | 96% | 0.1447 | 96% | 0.1419 | 95% | |||||
0.40 | III | 0.1521 | 96% | 0.1468 | 96% | 0.1432 | 96% | 0.1405 | 95% | ||||
0.60 | 0.1510 | 96% | 0.1443 | 96% | 0.1429 | 95% | 0.1388 | 95% | |||||
0.80 | 0.1502 | 96% | 0.1439 | 96% | 0.1406 | 95% | 0.1382 | 94% | |||||
80 | 0.40 | I | 0.1487 | 96% | 0.1421 | 95% | 0.1386 | 95% | 0.1374 | 94% | |||
0.60 | 0.1476 | 96% | 0.1414 | 95% | 0.1378 | 95% | 0.1367 | 94% | |||||
0.80 | 0.1468 | 96% | 0.1409 | 95% | 0.1363 | 95% | 0.1359 | 94% | |||||
0.40 | II | 0.1455 | 96% | 0.1396 | 95% | 0.1348 | 95% | 0.1345 | 94% | ||||
0.60 | 0.1447 | 96% | 0.1383 | 95% | 0.1339 | 95% | 0.1338 | 94% | |||||
0.80 | 0.1436 | 95% | 0.1375 | 95% | 0.1325 | 94% | 0.1329 | 93% | |||||
0.40 | III | 0.1424 | 95% | 0.1367 | 95% | 0.1320 | 94% | 0.1315 | 93% | ||||
0.60 | 0.1388 | 95% | 0.1359 | 94% | 0.1311 | 93% | 0.1306 | 92% | |||||
0.80 | 0.1374 | 95% | 0.1343 | 94% | 0.1308 | 93% | 0.1289 | 92% |
Scheme: (2, 0∗20, 2, 0∗20) | ||||||||||||||
0.39 | 1.08 | 1.25 | 1.47 | 1.57 | 1.61 | 1.69 | 1.80 | 1.84 | 1.87 | 1.89 | 2.03 | 2.03 | 2.05 | 2.12 |
2.35 | 2.41 | 2.43 | 2.48 | 2.50 | 2.53 | 2.55 | 2.55 | 2.59 | 2.67 | 2.74 | 2.79 | 2.81 | 2.82 | 2.85 |
2.87 | 2.88 | 2.93 | 2.95 | 2.96 | 2.97 | 3.09 | 3.11 | 3.11 | 3.15 | |||||
Scheme: (8, 0∗25, 8, 0∗15) | ||||||||||||||
0.39 | 0.85 | 1.25 | 1.47 | 1.61 | 1.80 | 1.87 | 2.03 | 2.12 | 2.35 | 2.41 | 2.43 | 2.48 | 2.50 | 2.53 |
2.55 | 2.55 | 2.56 | 2.59 | 2.67 | 2.73 | 2.74 | 2.79 | 2.81 | 2.82 | 2.93 | 2.95 | 2.97 | 3.11 | 3.15 |
3.19 | 3.22 | 3.28 | 3.31 | 3.31 | 3.33 | 3.39 | 3.39 | 3.56 | 3.60 |
Relative efficiency | Absolute difference lengths(ABLs) | |||||||
parameter | CS | MLE | Bayes-SE | Bayes-GE | Bootstrap | Asymptotic | Credible | Bootstrap |
θ | (2,0∗20,2,0∗20) | 0.9832 | 0.9851 | 0.9876 | 0.9842 | 0.0964 | 0.0961 | 0.0955 |
(8,0∗25,8,0∗15) | 0.9706 | 0.9723 | 0.9735 | 0.9714 | 0.101 | 0.0985 | 0.0971 | |
β | (2,0∗20,2,0∗20) | 0.9864 | 0.9896 | 0.9902 | 0.9876 | 0.0935 | 0.0927 | 0.0919 |
(8,0∗25,8,0∗15) | 0.9726 | 0.9745 | 0.9813 | 0.9753 | 0.0982 | 0.0962 | 0.0945 |