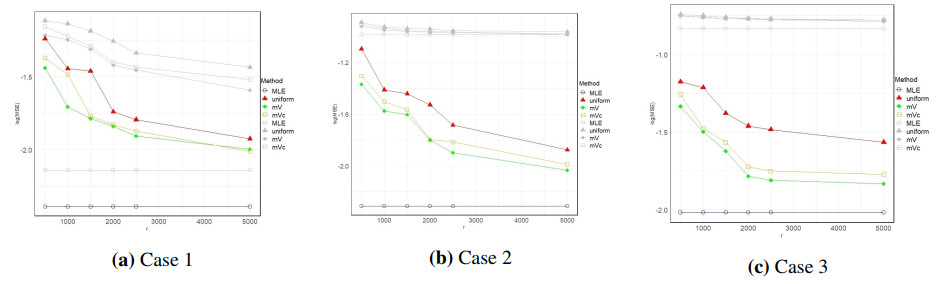
A semipositone fourth-order two-point boundary value problem is considered. In mechanics, the problem describes the deflection of an elastic beam rigidly fastened on the left and simply supported on the right. Under some conditions concerning the first eigenvalue corresponding to the relevant linear operator, the existence of nontrivial solutions and positive solutions to this boundary value problem is obtained. The main results are obtained by using the topological method and the fixed point theory of superlinear operators.
Citation: Haixia Lu, Li Sun. Positive solutions to a semipositone superlinear elastic beam equation[J]. AIMS Mathematics, 2021, 6(5): 4227-4237. doi: 10.3934/math.2021250
[1] | Taewan Kim, Jung Hoon Kim . A new optimal control approach to uncertain Euler-Lagrange equations: H∞ disturbance estimator and generalized H2 tracking controller. AIMS Mathematics, 2024, 9(12): 34466-34487. doi: 10.3934/math.20241642 |
[2] | Valérie Gauthier-Umaña, Henryk Gzyl, Enrique ter Horst . Decoding as a linear ill-posed problem: The entropy minimization approach. AIMS Mathematics, 2025, 10(2): 4139-4152. doi: 10.3934/math.2025192 |
[3] | Dayang Dai, Dabuxilatu Wang . A generalized Liu-type estimator for logistic partial linear regression model with multicollinearity. AIMS Mathematics, 2023, 8(5): 11851-11874. doi: 10.3934/math.2023600 |
[4] | Fei Yan, Junpeng Li, Haosheng Jiang, Chongqi Zhang . A-Optimal designs for mixture polynomial models with heteroscedastic errors. AIMS Mathematics, 2023, 8(11): 26745-26757. doi: 10.3934/math.20231369 |
[5] | Jiali Wu, Maoning Tang, Qingxin Meng . A stochastic linear-quadratic optimal control problem with jumps in an infinite horizon. AIMS Mathematics, 2023, 8(2): 4042-4078. doi: 10.3934/math.2023202 |
[6] | Xiaowei Zhang, Junliang Li . Model averaging with causal effects for partially linear models. AIMS Mathematics, 2024, 9(6): 16392-16421. doi: 10.3934/math.2024794 |
[7] | Zuliang Lu, Ruixiang Xu, Chunjuan Hou, Lu Xing . A priori error estimates of finite volume element method for bilinear parabolic optimal control problem. AIMS Mathematics, 2023, 8(8): 19374-19390. doi: 10.3934/math.2023988 |
[8] | Bo Jiang, Yongge Tian . Equivalent analysis of different estimations under a multivariate general linear model. AIMS Mathematics, 2024, 9(9): 23544-23563. doi: 10.3934/math.20241144 |
[9] | Chengjin Tang, Jiahao Guo, Yinghui Dong . Optimal investment based on performance measure with a stochastic benchmark. AIMS Mathematics, 2025, 10(2): 2750-2770. doi: 10.3934/math.2025129 |
[10] | Yang Liu, Ruihu Li, Qiang Fu, Hao Song . On the minimum distances of binary optimal LCD codes with dimension 5. AIMS Mathematics, 2024, 9(7): 19137-19153. doi: 10.3934/math.2024933 |
A semipositone fourth-order two-point boundary value problem is considered. In mechanics, the problem describes the deflection of an elastic beam rigidly fastened on the left and simply supported on the right. Under some conditions concerning the first eigenvalue corresponding to the relevant linear operator, the existence of nontrivial solutions and positive solutions to this boundary value problem is obtained. The main results are obtained by using the topological method and the fixed point theory of superlinear operators.
The generalized linear model (GLM), a generalization of the linear model with wide applications in many research areas, was proposed by Nelder and Wedderburn [1] in 1972 for discrete dependent variables, which cannot be dealt with by the ordinary linear regression model. The GLM allows the response variable to be nonnormal distributions, including binomial, Poisson, gamma, and inverse Gaussian distributions, whose means are linked with the predictors by a link function.
Nowadays, with the rapid development of science and technology, massive data is ubiquitous in many fields, including medicine, industry, and economics. Extracting effective information from massive data is the core challenge of big data analysis. However, the limited arithmetic power of computers tends to consume a lot of computing time. In order to deal with this challenge, parallel computing and distributed computing are commonly used, and subsampling techniques have emerged as a result, i.e., a small number of representative samples are extracted from massive data. Imberg et al. [2] proposed a theory on optimal design in the context of general data subsampling issues. It includes and extends most existing methods, works out optimality conditions, and offers algorithms for finding optimal subsampling scheme designs, which introduces a new class of invariant linear optimality criteria. Chao et al. [3] presented an optimal subsampling approach for modal regression with big data. The estimators are obtained by means of a two-step algorithm based on the modal expectation maximization when the bandwidth is not related to the subsample size.
There has been a great deal of research on subsampling algorithms of specific models. Wang et al. [4] devised a rapid subsampling algorithm to approximate the maximum likelihood estimators in the context of logistic regression. Based on the previous study, Wang [5] presented an enhanced estimation method for logistic regression, which has a higher estimation efficiency. In the case that data are usually distributed in multiple distributed sites for storage, Zuo et al. [6] developed a distributed subsampling procedure to effectively approximate the maximum likelihood estimators of logistic regression. Ai et al. [7] focused on the optimal subsampling method under the A-optimality criteria based on the method developed by Wang [4] for generalized linear models to quickly approximate maximum likelihood estimators from massive data. Yao and Wang [8] examined optimal subsampling methods for various models, including logistic regression models, softmax regression models, generalized linear models, quantile regression models, and quasi-likelihood estimators. Yu et al. [9] proposed an efficient subsampling procedure for online data streams with a multinomial logistic model. Yu et al. [10] studied the subsampling technique for the Akaike information criterion (AIC) and the smoothed AIC model-averaging framework for generalized linear models. Yu et al. [11] reviewed several subsampling methods for massive datasets from the viewpoint of statistical design.
To the best of our knowledge, all the existing methods above assume that the covariates are fully observable. However, in practice, this assumption is not realistic, and covariates may be inaccurately observed owing to measurement errors, which will lead to biases in the estimators of the regression coefficients. This means that we may incorrectly determine some unimportant variables as significant, which in turn affects the model selection and interpretation. Therefore, it is necessary to consider measurement errors. Liang et al. [12], Li and Xue [13], and Liang and Li [14] investigated the partial linear measurement error models. Stefanski [15] and Nakamura [16] obtained the corrected score functions of the GLM, such as linear regression, gamma regression, inverse gamma regression, and Poisson regression. Yang et al. [17] proposed an empirical likelihood method based on the moment identity of the corrected score function to perform statistical inference for a class of generalized linear measurement error models. Fuller [18] estimated the variable error model using the maximum likelihood method and studied statistical inference. Hu and Cui [19] proposed a corrected error variance method to accurately estimate the error variance, which can effectively reduce the influence of measurement error and false correlation at the same time. Carroll et al. [20] summarized the measurement errors in linear regression and described some simple and universally applicable measurement error analysis methods. Yi et al. [21] presented a regression calibration method, which is one of the first statistical methods introduced to address measurement errors in the covariates. In addition, they presented an overview of the conditional score and corrected score approaches for measurement error correction. Regarding the measurement errors in different situations existing in actual data, extensive research has been carried out, and a variety of methods have been proposed, see [22,23,24,25]. Recently, a class of variable selection procedures has been developed for measurement error models, see [26,27]. More recently, Ju et al. [28] studied the optimal subsampling algorithm and the random perturbation subsampling algorithm for big data linear models with measurement errors. The aim of this paper is to estimate the parameters using a subsampling algorithm for a class of generalized linear measurement error models in the massive data analysis.
In this paper, we study a class of the GLM with measurement errors, such as logistic regression models and Poisson regression models. We combine the corrected score function method with subsampling techniques to investigate subsampling algorithms. The consistency and asymptotic normality of the estimators obtained in the general subsampling algorithm are derived. We optimize the subsampling probabilities based on the design of A-optimality and L-optimality criteria and incorporate a truncation method in the optimal subsampling probabilities to obtain the optimal estimators. In addition, we develop an adaptive two-step algorithm and obtain the consistency and asymptotic normality of the final subsampling estimators. Finally, the effectiveness of the proposed method is demonstrated through numerical simulations and real data analysis.
The remainder of this paper is organized as follows: Section 2 introduces the corrected score function under different distributions and derives the general subsampling algorithm and the adaptive two-step algorithm. Sections 3 and 4 verify the effectiveness of the proposed method by generating simulated experimental data and two real data sets, respectively. Section 5 provides conclusions.
In the GLM, it is assumed that the conditional distribution of the response variable belongs to the exponential family
f(y;θ)=exp{θy−b(θ)a(ϕ)+c(y,ϕ)}, |
where a(⋅),b(⋅),c(⋅,⋅) are known functions, θ is called the natural parameter, and ϕ is called the dispersion parameter.
Let {(Xi,Yi)}Ni=1 be independent and identically distributed random samples, μi=E(Yi∣Xi),V(μi)=Var(Yi∣Xi), where the covariate Xi∈Rp and the response variable Yi∈R, V(⋅) is a known variance function. The conditional expectation of Yi given Xi is
g(μi)=XTiβ, | (2.1) |
where g(⋅) is the canonical link function, and β=(β1,…,βp)Tis a p-dimensional unknown regression parameter.
In practice, covariates are not always accurately observed, and there are measurement errors that cannot be ignored. Let Wi be an accurate observation of the covariate Xi. Assuming that the additive measurement error model is
Wi=Xi+Ui, | (2.2) |
where Ui∼Np(0,Σu), and it is independent of (Xi,Yi). Combining (2.1) and (2.2) yields a generalized linear model with measurement errors.
Define the log-likelihood function as ℓ(β;Yi)=N∑i=1logf(Yi;β). If Xi is observable, the score function for β in (2.1) is
N∑i=1ηi(β;Xi,Yi)=N∑i=1∂ℓ(β;Yi)∂β=N∑i=1Yi−μiV(μi)⋅∂μi∂β, |
and satisfies E[ηi(β;Xi,Yi)∣Xi]=0. However, when there is an error in Xi, directly replacing Xi with Wi to calculate ηi(β;Xi,Yi) causes a bias, i.e., E[ηi(β;Xi,Yi)]=0 will not always hold, hence a correction is needed. We define an unbiased score function η∗i(Σu,β;Wi,Yi) for β satisfying E[η∗i(Σu,β;Wi,Yi)∣Xi]=0 by the idea of [16]. The maximum likelihood estimator ˆβMLE of β is the solution of the estimating equation
Q(β):=N∑i=1η∗i(Σu,β;Wi,Yi)=0. | (2.3) |
Based on the following moment identities associated with the error model (2.2),
E(Wi∣Xi)=Xi, |
E(WiWTi∣Xi)=XiXTi+Σu, |
E(exp(WTiβ)∣Xi)=exp(XTiβ+12βTΣuβ), |
E[Wiexp(WTiβ)∣Xi]=(Xi+Σuβ)exp(XTiβ+12βTΣuβ), |
E[Wiexp(−WTiβ)∣Xi]=(Xi−Σuβ)exp[−XTiβ+12βTΣuβ], |
E[Wiexp(−2WTiβ)∣Xi]=(Xi−2Σuβ)exp[−2XTiβ+2βTΣuβ], |
then we can construct the unbiased score function for binary logistic measurement error regression models and Poisson measurement error regression models, which are widely used in practice.
(1) Binary logistic measurement error regression models.
We consider the logistic measurement error regression model
{P(Yi=1∣Xi)=11+exp(−XTiβ),Wi=Xi+Ui, |
with mean μi=[1+exp(−XTiβ)]−1 and variance Var(Yi∣Xi)=μi(1−μi). Followed by Huang and Wang [29], the corrected score function is
η∗i(Σu,β;Wi,Yi)=WiYi+(Wi+Σuβ)exp(−WTiβ−12βTΣuβ)Yi−Wi, |
and its first-order derivative is
Ω∗i(Σu,β;Wi,Yi)=∂η∗i(Σu,β;Wi,Yi)∂βT=[Σu−(Wi+Σuβ)(Wi+Σuβ)T]exp(−WTiβ−12βTΣuβ)Yi. |
(2) Poisson measurement error regression models.
Let Yi follow the Poisson distribution with mean μi, Var(Yi∣Xi)=μi. Consider the log linear measurement error model
{log(μi)=XTiβ,Wi=Xi+Ui, |
then we have the corrected score function
η∗i(Σu,β;Wi,Yi)=WiYi−(Wi−Σuβ)exp(WTiβ−12βTΣuβ), |
and its first-order derivative is
Ω∗i(Σu,β;Wi,Yi)=∂η∗i(Σu,β;Wi,Yi)∂βT=[Σu−(Wi−Σuβ)(Wi−Σuβ)T]exp(WTiβ−12βTΣuβ). |
It is assumed that πi is the probability of sampling the i-th sample (Wi,Yi), i=1,…,N. Let S be the set of the subsamples (˜Wi,~Yi) with corresponding sampling probabilities ~πi, i.e., S={(~Wi,~Yi,~πi)} with the subsample size r. The general subsampling algorithm is shown in Algorithm 1.
Algorithm 1 General subsampling algorithm. |
Step 1. Given the subsampling probabilities πi,i=1,…,N of all data points. Step 2. Perform repeated sampling with replacement r times to form the subsample set S={(˜Wi,˜Yi,˜πi)}, where ˜Wi, ~Yi and ~πi represent the covariate, response variable and subsampling probability in the subsample, respectively. Step 3. Based on the subsample set S, solve the weighted estimation equation Q∗(β) to obtain ⌣β, where |
Q∗(β):=1rr∑i=11~πi˜η∗i(Σu,β;˜Wi,~Yi)=0, (2.4) |
where ˜η∗i(Σu,β;˜Wi,~Yi) is the unbiased score function of i-th sample point in the subsample and its first order derivative is ˜Ω∗i(Σu,β;˜Wi,~Yi). |
To obtain the consistency and asymptotic normality of ⌣β, the following assumptions should be made. For simplicity, denote η∗i(Σu,β;Wi,Yi) and Ω∗i(Σu,β;Wi,Yi) as η∗i(Σu,β) and Ω∗i(Σu,β).
A1: It is assumed that WTiβ is almost necessarily in the interior of a closed set K∈Θ, Θ is a natural parameter space.
A2: The regression parameters are located in the ball Λ={β∈Rp:‖β‖1≤B},βt and ˆβMLE are true parameters and maximum likelihood estimators, which are interior points of Λ, and B is a constant, where ‖⋅‖1 denotes ℓ1-norm.
A3: As n→∞, the observed information matrix MX:=1NN∑i=1Ω∗i(Σu,ˆβMLE) is a positive definite matrix in probability.
A4: Assume that for all β∈Λ, 1NN∑i=1‖η∗i(Σu,β)‖4=OP(1), where ‖⋅‖ denotes the Euclidean norm.
A5: Suppose that the full sample covariates have finite 6th-order moments, i.e., E‖W1‖6≤∞.
A6: For any δ≥0, we assume that
1N2+δN∑i=1‖η∗i(Σu,ˆβMLE)‖2+δπ1+δi=OP(1),1N2+δN∑i=1|Ω∗(j1j2)i(Σu,ˆβMLE)|2+δπ1+δi=OP(1), |
where Ω∗(j1j2)i represents the elements of the j1-th row and j2-th column of the matrix Ω∗i.
A7: Assume that η∗i(Σu,β) and Ω∗i(Σu,β) are m(Wi)-Lipschitz continuous. For any β1,β2∈Λ, there exist functions m1(Wi) and m2(Wi) such that ‖η∗i(Σu,β1)−η∗i(Σu,β2)‖≤m1(Wi)‖β1−β2‖, ‖Ω∗i(Σu,β1)−Ω∗i(Σu,β2)‖S≤m2(Wi)‖β1−β2‖, where ‖A‖S denotes the spectral norm of matrix A. Further assume that E{m1(Wi)}≤∞ and E{m2(Wi)}≤∞.
Assumptions A1 and A2 are also used in Clémencon et al. [30]. The set Λ in Assumption A2 is also known as the admissible set and is a prerequisite for consistency estimation for the GLM with full data [31]. Assumption A3 imposes a condition on the covariates to ensure that the MLE based on the full dataset is consistent. In order to obtain the Bahadur representation of the subsampling estimators, Assumptions A4 and A5 are required. Assumption A6 is a moment condition for the subsampling probability and is also required for the Lindberg-Feller central limit theorem. Assumption A7 adds a restriction on smoothing, which can be found in [32].
The following theorems show the consistency and asymptotic normality of the subsampling estimators.
Theorem 2.1. If Assumptions A1–A7 hold, as r→∞ and N→∞, ⌣β converges to ˆβMLE in conditional probability given FN, and the convergence rate is r12. That is, for all ε>0, there exist constants Δε and rε such that
P(‖⌣β−ˆβMLE‖≥r−12Δε∣FN)<ε, | (2.5) |
for all r>rε.
Theorem 2.2. If Assumptions A1–A7 hold, as r→∞ and N→∞, conditional on FN, the estimator ⌣β obtained from Algorithm 1 satisfies
V−12(⌣β−ˆβMLE)d→Np(0,I), | (2.6) |
where V=M−1XVCM−1X=OP(r−1), and
VC=1N2rN∑i=1η∗i(Σu,ˆβMLE)η∗iT(Σu,ˆβMLE)πi. |
Remark 1. In order to get the standard error of the corresponding estimator, we estimate the variance-covariance matrix of ⌣β by
ˆV=ˆM−1XˆVCˆM−1X, |
where
ˆMX=1Nrr∑i=1˜Ω∗i(Σu,ˆβMLE)˜πi, |
ˆVC=1N2r2r∑i=1˜η∗i(Σu,ˆβMLE)˜η∗iT(Σu,ˆβMLE)˜π2i. |
Based on the A-optimality criteria in the optimal design language, the optimal subsampling probabilities are obtained by minimizing the asymptotic mean square error of ⌣β in Theorem 2.2.
However, Σu is usually unknown in practice. Therefore, we need to estimate the covariance matrix Σu as suggested by [12]. We observe that the consistent, unbiased moment estimator of Σu is
ˆΣu=N∑i=1mi∑j=1(Wij−¯Wi)(Wij−¯Wi)TN∑i=1(mi−1), |
where ¯Wi is the sample mean of the replicates, and mi is the number of repeated measurements of the i-th individual.
Theorem 2.3. Define gmVi=‖M−1Xη∗i(Σu,ˆβMLE)‖,i=1,…,N. The subsampling strategy is mV-optimal if the subsampling probability is chosen such that
πmVi=gmViN∑j=1gmVj, | (2.7) |
which is obtained by minimizing tr(V).
Theorem 2.4. Define gmVci=‖η∗i(Σu,ˆβMLE)‖,i=1,…,N. The subsampling strategy is mVc-optimal if the subsampling probability is chosen such that
πmVci=gmVciN∑j=1gmVcj, | (2.8) |
which is obtained by minimizing tr(VC).
Remark 2. MX and VC are non-negative definite matrices, and V=M−1XVCM−1X, then tr(V)=tr(M−1XVCM−1X)≤σmax(M−2X)tr(VC), where σmax(A) represents the maximum eigenvalue of square matrix A. As σmax(M−2X) does not depend on π, minimizing tr(VC) means minimizing the upper bound of tr(V). In fact, for two given subsampling probabilities π1 and π2, V(π1)≤V(π2) if and only if VC(π1)≤VC(π2). Therefore, minimizing tr(VC) reduces considerable computational time compared to minimizing tr(V), and tr(VC) does not take into account the structural information of the data.
The optimal subsampling probabilities are defined as {πopi}Ni=1={πmVi}Ni=1 or {πmVci}Ni=1. However, because πopi depends on ˆβMLE, it cannot be used directly in applications. To calculate πopi, it is necessary to use a prior estimator ˜β0, which is obtained by the prior subsample of size r0.
We know πopi is proportional to ‖η∗i(Σu,ˆβMLE)‖, however, in actual situations, there may be some data points that make η∗i(Σu,ˆβMLE)=0, which will never be included in a subsample, and some data points with η∗i(Σu,ˆβMLE)≈0 also have small probabilities of being sampled. If these special data points are excluded, some sample information will be missed, but if these data points are included, the variance of the subsampling estimator may increase.
To avoid Eq (2.4) from being inflated by these special data points, this paper adopts a truncation method, setting a threshold ω for ‖η∗i(Σu,ˆβMLE)‖, that is, replacing ‖η∗i(Σu,ˆβMLE)‖ with max{‖η∗i(Σu,ˆβMLE)‖,ω}, where ω is a very small positive number, for example, 10−4. In applications, the choice and design of the truncation weight function, which is a commonly used technique, are crucial to improving the robustness of the model and optimizing the performance.
We replace ˆβMLE in the matrix V with ˜β0, denoted as ˜V, then tr(˜V)≤tr(˜Vω)≤tr(˜V)+ω2N2rN∑i=1‖M−1X‖2πopi. Therefore, when ω is sufficiently small, tr(˜Vω) approaches tr(˜V). The threshold ω is set to make the subsample estimators more robust without sacrificing excessively estimation efficiency. ˜MX=1Nr0r0∑i=1Ω∗i(Σu,˜β0) based on the prior subsample can be used to approximate MX. The two-step algorithm is presented in Algorithm 2.
Algorithm 2 Optimal subsampling algorithm. |
Step 1. Extract a prior subsample set Sr0 with a subsample size of r0 from the full data, assuming that the subsampling probabilities of the prior subsample are πUNIF={πi:=1N}Ni=1. We use Algorithm 1 to obtain a prior estimator ˜β0, replace ˆβMLE with ˜β0 in Eqs (2.7) and (2.8) to get the optimal subsampling probabilities {πopti}Ni=1. Step 2. Use the optimal subsample probabilities {πopti}Ni=1 computed in Step 1 to extract a subsample size of r with replacement. According to the step in Algorithm 1, combining the subsamples from Step 1 and solving the estimating Eq (2.4) to get the estimator ˇβ based on a subsample of total size r0+r. |
Remark 3. In Algorithm 2, ˜β0 in Step 1 satisfies
Q∗0˜β0(β)=1r0r0∑i=1˜η∗i(Σu,β)πUNIFi=0 |
with the prior subsample set Sr0, and
M˜β0X=1Nr0r0∑i=1˜Ω∗i(Σu,˜β0)πUNIFi. |
In Step 2, the subsampling probabilities are {πopti}Ni=1={πmVti}Ni=1 or {πmVcti}Ni=1, let
gmVti={‖M−1Xη∗i(Σu,ˆβMLE)‖,if‖η∗i(Σu,ˆβMLE)‖>ωω‖M−1X‖,if‖η∗i(Σu,ˆβMLE)‖<ω,i=1,…,N, |
gmVcti=max{‖η∗i(Σu,ˆβMLE)‖,ω}, |
then
πmVti=gmVtiN∑j=1gmVtjandπmVcti=gmVctiN∑j=1gmVctj. |
The subsample set is Sr0∪{(˜Wi,˜Yi,˜πopti)∣i=1,…,r} with a subsample size of r+r0, and ˇβ is the solution to the corresponding estimating equation
Qtwo−step˜β0(β)=1r+r0r+r0∑i=1˜η∗i(Σu,β)˜πopti=rr+r0Q∗˜β0(β)+r0r+r0Q∗0˜β0(β)=0, |
where
Q∗˜β0(β)=1rr∑i=1˜η∗i(Σu,β)˜πopti. |
Theorem 2.5. If Assumptions A1–A7 hold, as r0r−1→0, r0→∞,r→∞ and N→∞, if ˜β0 exists, then the estimator ˇβ obtained from Algorithm 2 converges to ˆβMLE in conditional probability given FN, and its convergence rate is r12. For all ε>0, there exist finite Δε and rε such that
P(‖ˇβ−ˆβMLE‖≥r−12Δε∣FN)<ε, | (2.9) |
for all r>rε.
Theorem 2.6. If Assumptions A1–A7 hold, as r0r−1→0, r0→∞,r→∞ and N→∞, conditional on FN, the estimator ˇβ obtained from Algorithm 2 satisfies
V−12opt(ˇβ−ˆβMLE)d→Np(0,I), | (2.10) |
where Vopt=M−1XVoptCM−1X=OP(r−1), and
VoptC=1N2rN∑i=1η∗i(Σu,ˆβMLE)η∗iT(Σu,ˆβMLE)πopti. |
Remark 4. We estimate the variance-covariance matrix of ˇβ by
ˆVopt=ˆM−1XˆVoptCˆM−1X, |
where
ˆMX=1N(r0+r)[r0∑i=1˜Ω∗i(Σu,ˆβMLE)˜πUNIFi+r∑i=1˜Ω∗i(Σu,ˆβMLE)˜πopti], |
ˆVoptC=1N2(r0+r)2[r0∑i=1˜η∗i(Σu,ˆβMLE)˜η∗iT(Σu,ˆβMLE)˜πUNIF2i+r∑i=1˜η∗i(Σu,ˆβMLE)˜η∗iT(Σu,ˆβMLE)˜πopt2i]. |
In this section, we perform numerical simulations using synthetic data to evaluate the finite sample performance of the proposed method in Algorithm 2 (denoted as mV and mVc). For a fair comparison, we also give the results of the uniform subsampling method and set the size to be the same as that of Algorithm 2. The estimators of the above three subsampling methods, uniform—the uniform subsampling, mV—the mV probability subsampling, and mVc—the mVc probability subsampling, are compared with MLE—the maximum likelihood estimators for full data. In addition, we conduct simulation experiments using two models: the logistic regression model and the Poisson regression model.
Set the sample size N=100000, the true value βt=(0.5,−0.6,0.5)T, the covariate Xi∼N3(0,Σ), where Σ=0.5I+0.511T, I is an identity matrix. The response Yi follows a binomial distribution with P(Yi=1∣Xi)=(1+exp(−XTiβt))−1. We consider the following three cases to generate the measurement error term Ui.
● Case 1: Ui∼N3(0,0.42I);
● Case 2: Ui∼N3(0,0.52I);
● Case 3: Ui∼N3(0,0.62I).
The subsample size in Step 1 of Algorithm 2 is selected as r0=400. The subsample size r is set to be 500, 1000, 1500, 2000, 2500, and 5000. In order to verify that ˇβ can asymptotically approach βt, we repeat K=1000 and calculate MSE=1KK∑k=1‖ˇβ(k)−βt‖2, where ˇβ(k) is the parameter estimator of the subsample generated by the k-th repetition.
The simulation results are shown in Figure 1, which can be seen that both mV and mVc always have smaller MSEs than uniform subsampling. The MSEs of all the subsampling methods decrease as an increase of r, which confirms the theoretical results of the consistency of the subsampling methods. As the variance of the error term increases, the MSEs of uniform, mV, and mVc also increase. The mV is better than the mVc because the subsampling probabilities of mV take the structural information of the data into account. A comparison between the corrected and uncorrected methods shows that the MSEs of the corrected methods are much smaller than those of the uncorrected methods, and the difference between the corrected and uncorrected methods increases as the error variance increases.
Now, we evaluate the statistical inference performance of the optimal subsampling method for different r and variances of Ui. The parameter β1 is taken as an example, and a 95% confidence interval is constructed. Table 1 reports the empirical coverage probabilities and average lengths of three subsampling methods. It is evident that both mV and mVc have similar performance and consistently outperform the uniform subsampling method. As r increases, the length of the confidence interval uniformly decreases.
uniform | mV | mVc | |||||||
Case | r | Coverage | Length | Coverage | Length | Coverage | Length | ||
Case 1 | 500 | 0.958 | 0.565 | 0.932 | 0.331 | 0.942 | 0.457 | ||
1000 | 0.952 | 0.453 | 0.925 | 0.248 | 0.954 | 0.333 | |||
1500 | 0.960 | 0.387 | 0.920 | 0.206 | 0.964 | 0.274 | |||
2000 | 0.932 | 0.345 | 0.907 | 0.180 | 0.954 | 0.237 | |||
2500 | 0.938 | 0.313 | 0.910 | 0.160 | 0.956 | 0.211 | |||
5000 | 0.964 | 0.302 | 0.908 | 0.148 | 0.937 | 0.202 | |||
Case 2 | 500 | 0.956 | 0.634 | 0.946 | 0.602 | 0.962 | 0.613 | ||
1000 | 0.946 | 0.621 | 0.934 | 0.586 | 0.946 | 0.593 | |||
1500 | 0.927 | 0.597 | 0.954 | 0.551 | 0.962 | 0.561 | |||
2000 | 0.943 | 0.543 | 0.956 | 0.524 | 0.921 | 0.518 | |||
2500 | 0.970 | 0.475 | 0.958 | 0.453 | 0.944 | 0.462 | |||
5000 | 0.963 | 0.438 | 0.932 | 0.417 | 0.947 | 0.441 | |||
Case 3 | 500 | 0.958 | 0.706 | 0.956 | 0.432 | 0.968 | 0.550 | ||
1000 | 0.946 | 0.561 | 0.972 | 0.399 | 0.970 | 0.409 | |||
1500 | 0.944 | 0.479 | 0.968 | 0.321 | 0.960 | 0.329 | |||
2000 | 0.936 | 0.425 | 0.964 | 0.265 | 0.958 | 0.281 | |||
2500 | 0.926 | 0.389 | 0.966 | 0.249 | 0.954 | 0.250 | |||
5000 | 0.915 | 0.356 | 0.947 | 0.220 | 0.942 | 0.236 |
Let βt=(0.5,−0.6,0.5)T, the covariate Xi∼N3(0,Σ), where Σ=0.3I+0.511T, I is an identity matrix. We consider the following three cases to generate the measurement error term Ui.
● Case 1: Ui∼N3(0,0.32I);
● Case 2: Ui∼N3(0,0.42I);
● Case 3: Ui∼N3(0,0.52I).
We also generate a sample of N=100000 following Poisson(μi), where μi=exp(XTiβt). distribution, and summarize the MSEs with the number of simulations K=1000 in Figure 2. The other settings are the same as those in the logistic regression example.
In Figure 2, it can be seen that the MSEs of both the mV and mVc methods are smaller than those of the uniform subsampling, with the mV method being the optimal. In addition, the corrected method is obviously effective, which is consistent with Figure 1. Table 2 reports the empirical coverage probabilities and average lengths of 95% confidence interval of the parameter β3 for three subsampling methods. The conclusions of Table 2 are consistent with those of Table 1, but the average lengths of the intervals for Poisson regression are significantly longer than those for logistic regression.
uniform | mV | mVc | |||||||
Case | r | Coverage | Length | Coverage | Length | Coverage | Length | ||
Case 1 | 500 | 0.962 | 0.441 | 0.962 | 0.383 | 0.958 | 0.399 | ||
1000 | 0.944 | 0.352 | 0.964 | 0.291 | 0.964 | 0.304 | |||
1500 | 0.932 | 0.302 | 0.964 | 0.241 | 0.966 | 0.255 | |||
2000 | 0.952 | 0.268 | 0.930 | 0.210 | 0.944 | 0.223 | |||
2500 | 0.946 | 0.244 | 0.958 | 0.188 | 0.974 | 0.201 | |||
5000 | 0.952 | 0.234 | 0.961 | 0.173 | 0.943 | 0.185 | |||
Case 2 | 500 | 0.938 | 0.127 | 0.936 | 0.108 | 0.948 | 0.109 | ||
1000 | 0.936 | 0.102 | 0.946 | 0.082 | 0.934 | 0.082 | |||
1500 | 0.942 | 0.087 | 0.934 | 0.069 | 0.936 | 0.068 | |||
2000 | 0.952 | 0.078 | 0.956 | 0.060 | 0.952 | 0.059 | |||
2500 | 0.946 | 0.071 | 0.932 | 0.053 | 0.944 | 0.053 | |||
5000 | 0.935 | 0.068 | 0.965 | 0.045 | 0.971 | 0.047 | |||
Case 3 | 500 | 0.940 | 0.185 | 0.936 | 0.153 | 0.953 | 0.156 | ||
1000 | 0.950 | 0.148 | 0.954 | 0.113 | 0.958 | 0.118 | |||
1500 | 0.932 | 0.127 | 0.950 | 0.094 | 0.958 | 0.099 | |||
2000 | 0.946 | 0.113 | 0.952 | 0.082 | 0.960 | 0.086 | |||
2500 | 0.942 | 0.103 | 0.932 | 0.073 | 0.950 | 0.077 | |||
5000 | 0.937 | 0.096 | 0.956 | 0.065 | 0.964 | 0.061 |
In order to explore the influence of different subsample size allocated in the two-step algorithm, we calculate the MSEs for r0 at different proportions under the condition that the total subsample size remains constant. Set the total subsample size r0+r=3000, and the result is shown in Figure 3. It can be seen that the accuracy of the two-step algorithm will initially improve with the increase of r0. However, when r0 increases to a certain extent, the accuracy of the algorithm begins to decrease. There are two reasons: (1) if r0 is too small, the estimators in the first step will be biased, and it is difficult to ensure the accuracy; (2) if r0 are too large, then the performances of mV and mVc are similar to that of the uniform subsampling. When r0/(r0+r) is around 0.25, the two-step algorithm performs the best.
We use the Sys.time() function in R to calculate the running time of three subsampling methods and full data. We conduct 1000 repetitions, set r0=200, and consider different r values in Case 1. The results are shown in Tables 3 and 4. It is easy to find that the uniform subsampling algorithm requires the least computation time. Because there is no need to calculate the subsampling probabilities. In addition, the mV method takes longer than the mVc method, and this result is consistent with the theoretical analysis in Section 2.
r | ||||||
Method | 300 | 500 | 800 | 1200 | 1600 | 2000 |
uniform | 0.2993 | 0.3337 | 0.4985 | 0.5632 | 0.8547 | 0.5083 |
mV | 3.5461 | 3.6485 | 3.8623 | 4.1256 | 4.4325 | 5.2365 |
mVc | 3.2852 | 3.3658 | 3.5463 | 3.8562 | 4.0235 | 4.4235 |
Full | 45.9075 |
r | ||||||
Method | 300 | 500 | 800 | 1200 | 1600 | 2000 |
uniform | 0.4213 | 0.4868 | 0.5327 | 0.5932 | 0.7147 | 0.8883 |
mV | 4.6723 | 4.8963 | 5.2369 | 5.6524 | 6.0128 | 6.3567 |
mVc | 4.3521 | 4.6329 | 4.9658 | 5.2156 | 5.7652 | 5.9635 |
Full | 51.2603 |
In this section, we apply the proposed method to analyze the 1994 global census data, which contains 42 countries, from the Machine Learning Database [33]. There are 5 covariates in the data: x1 represents age; x2 represents the population weight value, which is assigned by the Population Division of the Census Bureau and is related to socioeconomic characteristics; x3 represents the highest level of education, that is, the highest level of education since primary school; x4 represents capital loss, which refers to the loss of income from bad investment, which is the difference between the lower selling price and the higher purchase price of an individual's investment; x5 represents weekly working hours. If an individual's annual income exceeds 50,000 dollars, it is expressed as yi=1 and yi=0 otherwise.
To verify the effectiveness of the proposed method, we add the measurement errors to the covariates x2, x4 and x5 in this dataset, and the covariance matrix of the measurement error is
Σu=[00.0400.040.04]. |
We split the full dataset into a training set of 32561 observations and a test set of 16281 observations in a 2:1 ratio. We apply the proposed method to the training set and evaluate the classification performance with the test set. We calculate LEMSE=log(1KK∑k=1‖ˇβ(k)−ˆβMLE‖2) based on 1000 bootstrap subsample estimators with r=500,1000,1500,2200,2500, and r0=500. The corrected MLE estimators for the training set are ˆβerrMLE, 0=−1.6121, ˆβerrMLE, 1=1.1992, ˆβerrMLE, 2=0.0103, ˆβerrMLE, 3=0.9142, ˆβerrMLE, 4=0.2617, ˆβerrMLE, 5=0.8694.
Table 5 shows the average estimators and the corresponding standard errors based on the proposed method (r0=500, r=2000). It can be seen that the estimators from three subsampling methods are close to the estimators from the full data. In general, the mV and mVc subsampling methods produce small standard errors.
uniform | mV | mVc | |
Intercept | -1.6084(0.069) | -1.5998(0.055) | -1.3122(0.052) |
ˇβerr1 | 1.2879(0.205) | 1.1880(0.103) | 1.2038(0.097) |
ˇβerr2 | 0.0105(0.106) | 0.0104(0.059) | 0.0111(0.046) |
ˇβerr3 | 1.0033(0.201) | 0.9217(0.067) | 0.9199(0.054) |
ˇβerr4 | 0.2636(0.094) | 0.2698(0.054) | 0.2555(0.063) |
ˇβerr5 | 0.9469(0.229) | 0.8741(0.083) | 0.8628(0.076) |
All subsampling methods show that each variable has a positive impact on income, with age, highest education level, and weekly working hours having significant impacts on income. Interestingly, capital losses have a significant positive impact on income because low-income people rarely invest. However, the population weight value has the smallest impact on income, the reason should be more inclined to reflect the overall distribution characteristics among groups rather than the specific economic performance of individuals. Income is a highly volatile variable, and the income gap between different groups may be large. Even under the same socioeconomic characteristics, the income distribution may have a large variance. This high variability weakens the overall impact of the population weight on income.
Fix r0=500, Figure 4(a) shows the LEMSEs calculated for the subsample with measurement errors. We can see that the LEMSEs of the corrected methods are much smaller than those of the uncorrected methods. As r increases, the LEMSEs become increasingly small. The estimators of the subsampling methods are consistent and the mV method is the best. Figure 4(b) shows the proportion of responses in the test set being correctly classified for different subsample sizes. The mV performs slightly better than the mVc. It can also be seen that the prediction accuracy of the corrected subsampling methods is slightly greater compared with the correspondingly uncorrected methods.
This subsection applies the corrected subsampling method to creditcard fraud detection dataset from Kaggle *, and the dependent variable is whether an individual has committed creditcard fraud. There are 284,807 pieces of data in the dataset, with a total of 492 fraud cases. Since the data involves sensitive information, the covariates have all been processed by principal component analysis with a total of 28 principal components. Amount represents the consumption amount, class is the dependent variable, 1 represents fraud, and 0 means normal. The first four principal components and the consumption amount are selected as independent variables.
*https://www.kaggle.com/datasets/creepycrap/creditcard-fraud-dataset
To verify the effectiveness of the proposed method, we add the measurement errors to the covariates, and the covariance matrix of the measurement error is Σu=0.16I. We split the dataset into the training set and the test set in a 3:1 ratio and summarize the LEMSEs based on the number of simulations K=1000 with r=500,1000,1500,2200,2500,5000, and r0=500.
The MLE estimators for the training set are ˆβerrMLE, 0=−8.8016, ˆβerrMLE, 1=−0.6070, ˆβerrMLE, 2=0.0737, ˆβerrMLE, 3=−0.9056, ˆβerrMLE, 4=1.4553, ˆβerrMLE, 5=−0.1329. Table 6 shows the average estimators and the corresponding standard errors (r0=500, r=2000). It can be seen that the estimators from three subsampling methods are close to the estimators from the full data. In general, the mV and mVc subsampling methods produce small standard errors. From Figure 5, we can obtain similar results as in Figure 4.
uniform | mV | mVc | |
Intercept | -8.7934(0.0678) | -8.8105(0.0562) | -8.8135(0.0543) |
ˇβerr1 | -0.6123(0.341) | -0.6047(0.142) | -0.6035(0.105) |
ˇβerr2 | 0.0712(0.125) | 0.0730(0.064) | 0.0798(0.088) |
ˇβerr3 | -0.9321(0.245) | -0.9087(0.067) | -0.9123(0.057) |
ˇβerr4 | 1.4618(0.198) | 1.4580(0.054) | 1.4603(0.075) |
ˇβerr5 | -0.1435(0.531) | -0.1347(0.242) | -0.1408(0.225) |
In this paper, we not only combine the corrected score method with the subsampling technique, but also theoretically derive the consistency and asymptotic normality of the subsampling estimators. In addition, an adaptive two-step algorithm is developed based on optimal subsampling probabilities using A-optimality and L-optimality criteria and the truncation method. The theoretical results of the proposed method are tested with simulated and two real datasets, and the experimental results demonstrate the effectiveness and good performance of the proposed method.
This paper merely assumes that the covariates are affected by the measurement error. However, in practical applications, the response variables can be influenced by measurement errors. The optimal subsampling probabilities are obtained by minimizing tr(V) or tr(VC) using the design ideas of the A-optimality and L-optimality criteria. In the future, the other optimality criteria for subsampling can be considered to develop more efficient algorithms.
Ruiyuan Chang: Furnished the algorithms and numerical results presented in the manuscript and composed the original draft of the manuscript; Xiuli Wang: Rendered explicit guidance regarding the proof of the theorem and refined the language of the entire manuscript; Mingqiu Wang: Rendered explicit guidance regarding the proof of theorems and the writing of codes and refined the language of the entire manuscript. All authors have read and consented to the published version of the manuscript.
The authors declare that they have not used Artificial Intelligence (AI) tools in the creation of this article.
This research was supported by the National Natural Science Foundation of China (12271294) and the Natural Science Foundation of Shandong Province (ZR2024MA089).
The authors declare no conflict of interest.
The proofs of the following lemmas and theorems are primarily based on Wang et al. [5], Ai et al. [7] and Yu et al. [34].
Lemma 1. If Assumptions A1–A4 and A6 hold, as r→∞ and N→∞, conditional on FN, we have
⌣MX−MX=OP∣FN(r−12), | (A.1) |
1NQ∗(ˆβMLE)−1NQ(ˆβMLE)=OP∣FN(r−12), | (A.2) |
1NV−12CQ∗(ˆβMLE)d→Np(0,I), | (A.3) |
where
⌣MX=1Nrr∑i=1˜Ω∗i(Σu,ˆβMLE)˜πi, |
and
VC=1N2rN∑i=1η∗i(Σu,ˆβMLE)η∗iT(Σu,ˆβMLE). |
Proof.
E(⌣MX|FN)=E(1Nrr∑i=1˜Ω∗i(Σu,ˆβMLE)˜πi|FN)=1Nrr∑i=1N∑j=1πjΩ∗j(Σu,ˆβMLE)πj=1NN∑i=1Ω∗i(Σu,ˆβMLE)=MX. |
By Assumption A6, we have
E[(⌣Mj1j2X−Mj1j2X)2|FN]=E[(1Nrr∑i=1˜Ω∗(j1j2)i(Σu,ˆβMLE)˜πi−1NN∑i=1Ω∗(j1j2)i(Σu,ˆβMLE))2|FN]=1rN∑i=1πi(Ω∗(j1j2)i(Σu,ˆβMLE)Nπi−Mj1j2X)2=1rN∑i=1πi(Ω∗(j1j2)i(Σu,ˆβMLE)Nπi)2−1r(Mj1j2X)2≤1rN∑i=1πi(Ω∗(j1j2)i(Σu,ˆβMLE)Nπi)2=OP(r−1). |
It follows from Chebyshev's inequality that (A.1) holds.
E(1NQ∗(ˆβMLE)|FN)=E(1N1rr∑i=1˜η∗i(Σu,ˆβMLE)˜πi|FN)=1Nrr∑i=1N∑j=1πjη∗j(Σu,ˆβMLE)πj=1NN∑i=1η∗i(Σu,ˆβMLE)=0. |
By Assumption A4, we have
Var(1NQ∗(ˆβMLE)|FN)=Var[(1N1rr∑i=1˜η∗i(Σu,ˆβMLE)˜πi)|FN]=1N2r2r∑i=1N∑j=1πjη∗i(Σu,ˆβMLE)η∗Ti(Σu,ˆβMLE)π2j=1N2rN∑i=1η∗i(Σu,ˆβMLE)η∗Ti(Σu,ˆβMLE)πi=OP(r−1). |
Now (A.2) follows from Markov's Inequality.
Let γ∗i=(Nπi)−1˜η∗i(Σu,ˆβMLE), then N−1Q∗(ˆβMLE)=r−1r∑i=1γ∗i holds. Based on Assumption A6, for all ε>0, we have
r∑i=1E{‖r−12γ∗i‖2I(‖γ∗i‖>r12ε)|FN}=1rr∑i=1E{‖γ∗i‖2I(‖γ∗i‖>r12ε)|FN}≤1r32εr∑i=1E{‖γ∗i‖3|FN}=1r12ε1N3N∑i=1‖γ∗i‖3π2i=OP(r−12)=oP(1). |
This shows that the Lindeberg-Feller conditions are satisfied in probability. Therefore (A.3) is true.
Lemma 2. Assumptions A1–A7 hold, as r→∞ and N→∞, conditional on FN, for all sr→0, we have
1NrN∑i=1˜Ω∗i(Σu,ˆβMLE+sr)˜πi−1NN∑i=1Ω∗i(Σu,ˆβMLE)=oP∣FN(1). | (A.4) |
Proof. The Eq (A.4) can be written as
1NrN∑i=1˜Ω∗i(Σu,ˆβMLE+sr)˜πi−1NrN∑i=1˜Ω∗i(Σu,ˆβMLE)˜πi+1NrN∑i=1˜Ω∗i(Σu,ˆβMLE)˜πi−1NN∑i=1Ω∗i(Σu,ˆβMLE). |
Let
τ1:=1NrN∑i=1˜Ω∗i(Σu,ˆβMLE+sr)˜πi−1NrN∑i=1˜Ω∗i(Σu,ˆβMLE)˜πi, |
then by Assumption A7, we have
E(‖τ1‖S|FN)=E{1NrN∑i=11˜πi‖˜Ω∗i(Σu,ˆβMLE+sr)−˜Ω∗i(Σu,ˆβMLE)‖S|FN}=1Nrr∑i=1N∑j=1πj1πj‖Ω∗i(Σu,ˆβMLE+sr)−Ω∗i(Σu,ˆβMLE)‖S≤1NN∑i=1m2(Wi)‖sr‖=oP(1). |
It follows from Markov's inequality that {{\mathit{\boldsymbol{\tau}}} _1} = {o_{P\mid\mathcal{F}_N}}(1) .
Let
{{\mathit{\boldsymbol{\tau}} }_2} : = \frac{1}{Nr}\sum\limits_{i = 1}^N {\frac{{{\widetilde{\bf{\Omega}}}_i^*({{\mathit{\boldsymbol{\Sigma}}}_u},{{\hat{\mathit{\boldsymbol{\beta}}}}_{\text {MLE}}})}}{{\widetilde \pi }_i} - \frac{1}{N}\sum\limits_{i = 1}^N {{\bf{\Omega}}_i^*}({{\mathit{\boldsymbol{\Sigma}}}_u},{{\hat{\mathit{\boldsymbol{\beta}}}}_{\text {MLE}}})}, |
then
E\left\{ \frac{1}{Nr} \sum\limits_{i = 1}^N \frac{\widetilde{\bf{{\Omega}}}_i^*(\mathit{\boldsymbol{\Sigma}}_u, {\hat{\mathit{\boldsymbol{\beta}}}}_{\text{MLE}})}{\widetilde{\pi}_i} \,\middle\vert\, \mathcal{F}_N \right\} = \frac{1}{N} \sum\limits_{i = 1}^N {\bf{\Omega}}_i^*(\mathit{\boldsymbol{\Sigma}}_u, {\hat{\mathit{\boldsymbol{\beta}}}}_{\text{MLE}}). |
From the proof of Lemma 1, it follows that
E\left[ \left( \overset{\smile}{\bf{M}}_X^{j_1 j_2} - {\bf{M}}_X^{j_1 j_2} \right)^2 \,\middle\vert\, \mathcal{F}_N \right] = {O_P}({r^{ - 1}}) = {o_P}(1). |
Therefore {{\mathit{\boldsymbol{\tau}}} _2} = {o_{P\mid\mathcal{F}_N}}(1) , and (A.4) holds
Next, we will prove Theorems 2.1 and 2.2.
Proof of Theorem 2.1. \overset{\smile}{\mathit{\boldsymbol{\beta}}} is the solution of {\mathit{\boldsymbol{Q}}^{*}}(\mathit{\boldsymbol{\beta}}) = \frac{1}{r}\sum\limits_{i = 1}^r {\frac{1}{{{{\widetilde \pi }_i}}}}{{\tilde{\mathit{\boldsymbol{\eta}}}}_{_i}^*({{\mathit{\boldsymbol{\Sigma}}}_u}, \mathit{\boldsymbol{\beta}})} = {\mathbf{0}} , then
E\left( \frac{1}{N} \mathit{\boldsymbol{Q}}^{*}(\mathit{\boldsymbol{\beta}}) \,\middle\vert\, \mathcal{F}_N \right) = \frac{1}{Nr} \sum\limits_{i = 1}^r \sum\limits_{j = 1}^N \pi_j \frac{{\mathit{\boldsymbol{\eta}}}_{j}^*(\mathit{\boldsymbol{\Sigma}}_u, \mathit{\boldsymbol{\beta}})}{\pi_j} = \frac{1}{N} \sum\limits_{i = 1}^N {\mathit{\boldsymbol{\eta}}}_{i}^*(\mathit{\boldsymbol{\Sigma}}_u, \mathit{\boldsymbol{\beta}}) = \frac{1}{N} \mathit{\boldsymbol{Q}}(\mathit{\boldsymbol{\beta}}). |
By Assumption A6, we have
\begin{aligned} \text{Var}\left( \frac{1}{N} \mathit{\boldsymbol{Q}}^{*}(\mathit{\boldsymbol{\beta}}) \,\middle\vert\, \mathcal{F}_N \right) & = \text{Var}\left( \frac{1}{N} \frac{1}{r} \sum\limits_{i = 1}^r \frac{{\tilde{\mathit{\boldsymbol{\eta}}}}_i^*(\mathit{\boldsymbol{\Sigma}}_u, \mathit{\boldsymbol{\beta}})}{\widetilde{\pi}_i} \,\middle\vert\, \mathcal{F}_N \right) \\ & = \frac{1}{N^2 r^2} \sum\limits_{i = 1}^r \sum\limits_{j = 1}^N \pi_j \frac{\mathit{\boldsymbol{\eta}}_i^*(\mathit{\boldsymbol{\Sigma}}_u, \mathit{\boldsymbol{\beta}}) {\mathit{\boldsymbol{\eta}}}_i^{*{\rm T}}(\mathit{\boldsymbol{\Sigma}}_u, \mathit{\boldsymbol{\beta}})}{\pi_j^2} \\ & = \frac{1}{N^2 r} \sum\limits_{i = 1}^N \frac{{\mathit{\boldsymbol{\eta}}}_i^*(\mathit{\boldsymbol{\Sigma}}_u, \mathit{\boldsymbol{\beta}}) {\mathit{\boldsymbol{\eta}}}_i^{*{\rm T}}(\mathit{\boldsymbol{\Sigma}}_u, \mathit{\boldsymbol{\beta}})}{\pi_i} \\ & = O_P(r^{-1}). \end{aligned} |
Therefore, as r \to \infty , {N}^{-1} \mathit{\boldsymbol{Q}}^{*}(\mathit{\boldsymbol{\beta}}) - {N}^{-1} \mathit{\boldsymbol{Q}}(\mathit{\boldsymbol{\beta}}) \xrightarrow{} 0 for all {\boldsymbol \beta} \in \Lambda in conditional probability given \mathcal{F}_N . Thus, from Theorem 5.9 in [32], we have \left\| \overset{\smile}{\mathit{\boldsymbol{\beta}}} - \hat{\mathit{\boldsymbol{\beta}}}_{\text{MLE}} \right\| = o_{P\mid\mathcal{F}_N}(1) . By Taylor expansion,
\begin{aligned} \frac{1}{N} \mathit{\boldsymbol{Q}}^{*}(\overset{\smile}{\mathit{\boldsymbol{\beta}}}) = {\mathbf{0}} & = \frac{1}{N} \mathit{\boldsymbol{Q}}^{*}({\hat{\mathit{\boldsymbol{\beta}}}}_{\text{MLE}}) + \frac{1}{Nr} \sum\limits_{i = 1}^r \frac{{\widetilde{\bf{\Omega}}}_i^*(\mathit{\boldsymbol{\Sigma}}_u, {\hat{\mathit{\boldsymbol{\beta}}}}_{\text{MLE}} + {{\boldsymbol{s}}}_r)}{\widetilde{\pi}_i} (\overset{\smile}{\mathit{\boldsymbol{\beta}}} - {\hat{\mathit{\boldsymbol{\beta}}}}_{\text{MLE}}). \end{aligned} |
By Lemma 2, it follows that
\frac{1}{Nr} \sum\limits_{i = 1}^N \frac{\widetilde{\bf{\Omega}}_i^*(\mathit{\boldsymbol{\Sigma}}_u, {\hat{\mathit{\boldsymbol{\beta}}}}_{\text{MLE}} + {{\boldsymbol{s}}}_r)}{\widetilde{\pi}_i} - \frac{1}{N} \sum\limits_{i = 1}^N {\bf{\Omega}}_i^*(\mathit{\boldsymbol{\Sigma}}_u, {\hat{\mathit{\boldsymbol{\beta}}}}_{\text{MLE}}) = o_{P\mid\mathcal{F}_N}(1), |
then
{\mathbf{0}} = \frac{1}{N} \mathit{\boldsymbol{Q}}^{*}({\hat{\mathit{\boldsymbol{\beta}}}}_{\text{MLE}}) + \frac{1}{N} \sum\limits_{i = 1}^N {\bf{\Omega}}_i^*(\mathit{\boldsymbol{\Sigma}}_u, {\hat{\mathit{\boldsymbol{\beta}}}}_{\text{MLE}}) (\overset{\smile}{\mathit{\boldsymbol{\beta}}} - {\hat{\mathit{\boldsymbol{\beta}}}}_{\text{MLE}}) + o_{P\mid\mathcal{F}_N}(1)(\overset{\smile}{\mathit{\boldsymbol{\beta}}} - {\hat{\mathit{\boldsymbol{\beta}}}}_{\text{MLE}}). |
Here is
\frac{1}{N}{\mathit{\boldsymbol{Q}}^{*}}({\mathit{\boldsymbol{\hat \beta}}_{\text {MLE}}}) + {{\bf{M}}_X}(\overset{\smile}{\mathit{\boldsymbol{\beta}}} - \hat{\mathit{\boldsymbol{\beta}}}_{\text{MLE}}) + {o_{P\mid\mathcal{F}_N}}\left( {\left\| {\overset{\smile}{\mathit{\boldsymbol{\beta}}} - \hat{\mathit{\boldsymbol{\beta}}}_{\text{MLE}}} \right\|} \right) = {\mathbf{0}}, |
we have
\begin{equation} \begin{aligned} \overset{\smile}{\mathit{\boldsymbol{\beta}}} - {\hat{\mathit{\boldsymbol{\beta}}}}_{\text{MLE}} & = - {\bf{M}}_X^{-1} \left\{ \frac{1}{N} \mathit{\boldsymbol{Q}}^{*}({\hat{\mathit{\boldsymbol{\beta}}}}_{\text{MLE}}) + o_{P\mid\mathcal{F}_N} \left( \left\| \overset{\smile}{\mathit{\boldsymbol{\beta}}} - {\hat{\mathit{\boldsymbol{\beta}}}}_{\text{MLE}} \right\| \right) \right\} \\ & = - {\bf{M}}_X^{-1} \mathit{\boldsymbol{V}}_{\text {C}}^{\frac{1}{2}} {\bf{V}}_{\text {C}}^{-\frac{1}{2}} \frac{1}{N} \mathit{\boldsymbol{Q}}^{*}({\hat{\mathit{\boldsymbol{\beta}}}}_{\text{MLE}}) + {\bf{M}}_X^{-1} o_{P\mid\mathcal{F}_N} \left( \left\| \overset{\smile}{\mathit{\boldsymbol{\beta}}} - {\hat{\mathit{\boldsymbol{\beta}}}}_{\text{MLE}} \right\| \right) \\ & = O_{P\mid\mathcal{F}_N} \left( r^{-\frac{1}{2}} \right) + o_{P\mid\mathcal{F}_N} \left( \left\| \overset{\smile}{\mathit{\boldsymbol{\beta}}} - {\hat{\mathit{\boldsymbol{\beta}}}}_{\text{MLE}} \right\| \right). \end{aligned} \end{equation} | (A.5) |
By Lemma 1 and Assumption A3, {\bf{M}}_X^{ - 1} = {O_{P\mid\mathcal{F}_N}}\left(1 \right) , we have \overset{\smile}{\mathit{\boldsymbol{\beta}}} - \hat{\mathit{\boldsymbol{\beta}}}_{\text{MLE}} = {O_{P\mid\mathcal{F}_N}}\left({{r^{ - \frac{1}{2}}}} \right) .
Proof of Theorem 2.2. By Lemma 1 and (A.5), as r \to \infty , conditional on \mathcal{F}_N , it holds that
\begin{aligned} {\bf{V}}^{-\frac{1}{2}}(\overset{\smile}{\mathit{\boldsymbol{\beta}}} - {\hat{\mathit{\boldsymbol{\beta}}}}_{\text{MLE}}) = - {\bf{V}}^{-\frac{1}{2}}{{\bf{M}}}_X^{-1}{\bf{V}}_{\text {C}}^{\frac{1}{2}}{\bf{V}}_{\text {C}}^{-\frac{1}{2}}\frac{1}{N}{\mathit{\boldsymbol{Q}}^{*}}({\hat{\mathit{\boldsymbol{\beta}}}}_{\text{MLE}}) + o_{P|{\mathcal{F}_N}}\left(1\right). \end{aligned} |
By Lemma 1 and Slutsky's theorem, it follows that
{{\bf{V}}^{ - \frac{1}{2}}}(\overset{\smile}{\mathit{\boldsymbol{\beta}}} - {\hat{\mathit{\boldsymbol{\beta}}}}_{\text{MLE}})\mathop \to \limits^d N_p({{\mathbf{0}}},{\bf I}). |
Proof of Theorem 2.3. To minimize the asymptotic variance tr({\bf{V}}) of \overset{\smile}{\mathit{\boldsymbol{\beta}}} , the optimization problem is
\begin{equation} \left\{\begin{array}{l} \min tr({\bf{V}}) = \min \frac{1}{N^2 r} \sum\limits_{i = 1}^N \left[\frac{1}{\pi_i} \left\|{{\bf{M}}_X^{-1}} {\mathit{\boldsymbol{\eta}}}_i^*\left(\mathit{\boldsymbol{\Sigma}}_u, \hat{\mathit{\boldsymbol{\beta}}}_{\text {MLE}}\right)\right\|^2\right], \\ \text { s.t. } \sum\limits_{i = 1}^N \pi_i = 1, \quad 0 \leq \pi_i \leq 1, \quad i = 1, \ldots, N. \end{array}\right. \end{equation} | (A.6) |
Define g_i^{\text{mV}} = \left\|{{\bf{M}}_X^{-1}} {\mathit{\boldsymbol{\eta}}}_i^*\left(\mathit{\boldsymbol{\Sigma}}_u, \hat{\mathit{\boldsymbol{\beta}}}_{\text {MLE}}\right)\right\|, \; i = 1, \ldots, N , it follows from Cauchy's inequality that
\begin{aligned} {tr}({\bf{V}}) & = \frac{1}{N^2 r} \sum\limits_{i = 1}^N \left[ \frac{1}{\pi_i} \left\|{{\bf{M}}_X^{-1}} {\mathit{\boldsymbol{\eta}}}_i^*\left(\mathit{\boldsymbol{\Sigma}}_u, \hat{\mathit{\boldsymbol{\beta}}}_{\text {MLE}}\right)\right\|^2 \right] \\ & = \frac{1}{N^2 r} \left( \sum\limits_{i = 1}^N \pi_i \right) \left\{ \sum\limits_{i = 1}^N \left[ \frac{1}{\pi_i} \left\|{{\bf{M}}_X^{-1}} {\mathit{\boldsymbol{\eta}}}_i^*\left(\mathit{\boldsymbol{\Sigma}}_u, \hat{\mathit{\boldsymbol{\beta}}}_{\text {MLE}}\right)\right\|^2 \right] \right\} \\ &\ge \frac{1}{N^2 r} \left[ \sum\limits_{i = 1}^N \left\|{{\bf{M}}_X^{-1}} {\mathit{\boldsymbol{\eta}}}_i^*\left(\mathit{\boldsymbol{\Sigma}}_u, \hat{\mathit{\boldsymbol{\beta}}}_{\text {MLE}}\right)\right\| \right]^2 \\ & = \frac{1}{N^2 r} \left[ \sum\limits_{i = 1}^N g_i^{\text{mV}} \right]^2. \end{aligned} |
The equality sign holds if and only if {\pi _i} \propto g_i^{\text{mV}} , therefore
\pi_i^{\text{mV}} = \frac{g_i^{\text{mV}}}{\sum\limits_{j = 1}^N g_j^{\text{mV}}} |
is the optimal solution.
The proof of Theorem 2.4 is similar to Theorem 2.3.
Lemma 3. If Assumptions A1–A4 and A6 hold, as r_0 \to \infty , r \to \infty and N \to \infty , conditional on \mathcal{F}_N , we have
\begin{equation} {\overset{\smile}{\bf{M}}_X^{{\tilde{\mathit{\boldsymbol{\beta}}}}_0}} - {{\bf{M}}_X} = {O_{P|{\mathcal{F}_N}}(r^{-\frac{1}{2}})}, \end{equation} | (A.7) |
\begin{equation} {\bf{M}}_X^0 - {{\bf{M}}_X} = {O_{P|{\mathcal{F}_N}}}({r_0}^{ - \frac{1}{2}}), \end{equation} | (A.8) |
\begin{equation} \frac{1}{N}\mathit{\boldsymbol{Q}}_{{{\tilde{\mathit{\boldsymbol{\beta}}}}_0}}^{*}({{\hat{\mathit{\boldsymbol{\beta}}}}_{\mathit{\text{MLE}}}}) = {O_{P|{\mathcal{F}_N}}}({r^{ - \frac{1}{2}}}), \end{equation} | (A.9) |
\begin{equation} \frac{1}{N}\mathit{\boldsymbol{Q}}_{{{\tilde{\mathit{\boldsymbol{\beta}}}}_0}}^{*0}({{\hat{\mathit{\boldsymbol{\beta}}}}_{\mathit{\text{MLE}}}}) = {O_{P|{\mathcal{F}_N}}}({{r_0}^{ - \frac{1}{2}}}), \end{equation} | (A.10) |
\begin{equation} \frac{1}{N}{\bf{V}}_{\mathit{\text{C}}}^{\mathit{\text{opt}}- \frac{1}{2}}\mathit{\boldsymbol{Q}}_{{{\tilde{\mathit{\boldsymbol{\beta}}}}_0}}^{*}({{\hat{\mathit{\boldsymbol{\beta}}}}_{\mathit{\text{MLE}}}})\mathop \to \limits^d N_p({{\mathbf{0}}},{\bf I}), \end{equation} | (A.11) |
where
\overset{\smile}{\bf{M}}_X^{\tilde{\boldsymbol \beta}_0} = \frac{1}{{Nr}}\sum\limits_{i = 1}^r {\frac{{{\widetilde{\bf{\Omega}}}_{_i}^*({{\mathit{\boldsymbol{\Sigma}}}_u},{{\hat{\mathit{\boldsymbol{\beta}}}}_{\mathit{\text{MLE}}}})}}{{\widetilde \pi _i^{\mathit{\text{opt}}}}}}, |
{\bf{M}}_X^0 = \frac{1}{{N{r_0}}}\sum\limits_{i = 1}^{{r_0}} {\frac{{{\widetilde{\bf{ \Omega}}}_{_i}^*({{\mathit{\boldsymbol{\Sigma}}}_u},{{\hat{\mathit{\boldsymbol{\beta}}}}_{\mathit{\text{MLE}}}})}}{{\widetilde \pi _i^{\mathit{\text{UNIF}}}}}}. |
Proof.
\begin{aligned} E\left( \overset{\smile}{\bf{M}}_X^{\tilde{\boldsymbol \beta}_0} \,\middle\vert\, \mathcal{F}_N \right) & = E_{{\tilde{\boldsymbol \beta}}_0} \left[ E\left(\overset{\smile}{\bf{M}}_X^{{\tilde{\boldsymbol \beta}}_0} \,\middle\vert\, \mathcal{F}_N, {\tilde{\boldsymbol \beta}}_0\right) \right] \\ & = E_{{\tilde{\boldsymbol \beta}}_0} \left[ E \left( \frac{1}{Nr} \sum\limits_{i = 1}^r \frac{{\widetilde{\bf{{\Omega}}}}_{i}^*(\mathit{\boldsymbol{\Sigma}}_u, {\hat{\mathit{\boldsymbol{\beta}}}}_{\text{MLE}})}{\widetilde{\pi}_i^{\text{opt}}} \,\middle\vert\, \mathcal{F}_N, {\tilde{\boldsymbol \beta}}_0 \right) \right] \\ & = E_{{\tilde{\boldsymbol \beta}}_0} \left[ E\left({\bf{M}}_X \,\middle\vert\, \mathcal{F}_N, {\tilde{\boldsymbol \beta}}_0\right) \right] \\ & = {\bf{M}}_X. \end{aligned} |
By Assumption A6, we have
\begin{aligned} &E\left[ \left( \overset{\smile}{\bf{M}}_X^{{\tilde{\boldsymbol \beta}}_0, j_1 j_2} - {{\bf{M}}_X}^{j_1 j_2} \right)^2 \,\middle\vert\, \mathcal{F}_N \right] \\ = & E_{{\tilde{\mathit{\boldsymbol{\beta}}}}_0} \left\{ E \left[ \left( \overset{\smile}{\bf{M}}_X^{\tilde{\mathit{\boldsymbol{\beta}}}_0, j_1 j_2} - {\bf{M}}_X^{j_1 j_2}\right)^2 \,\middle\vert\, \mathcal{F}_N, \tilde{\beta}_0 \right]\right\} \\ = & E_{{\tilde{\mathit{\boldsymbol{\beta}}}}_0} \left[ \frac{1}{r} \sum\limits_{i = 1}^N {\pi}_i^{\text{opt}} \left( \frac{{\widetilde{\bf{{\Omega}}}}_{i}^{*j_1 j_2}(\mathit{\boldsymbol{\Sigma}}_u, {\hat{\boldsymbol \beta}}_{\text{MLE}})}{N {\pi}_i^{\text{opt}}} - {\bf{M}}_X^{j_1 j_2} \right)^2 \,\middle\vert\, \mathcal{F}_N, \tilde{\boldsymbol \beta}_0 \right] \\ = & E_{{\tilde{\mathit{\boldsymbol{\beta}}}}_0} \left[ \frac{1}{r} \sum\limits_{i = 1}^N {\pi}_i^{\text{opt}} \left( \frac{{\widetilde{\bf{{\Omega}}}}_{i}^{*j_1 j_2}(\mathit{\boldsymbol{\Sigma}}_u, {\hat{\boldsymbol \beta}}_{\text{MLE}})}{N {\pi}_i^{\text{opt}}} \right)^2 - \frac{1}{r} \left( {\bf{M}}_X^{j_1 j_2} \right)^2 \,\middle\vert\, \mathcal{F}_N, {\widetilde{\boldsymbol \beta}}_0 \right] \\ \le& E_{{\tilde{\mathit{\boldsymbol{\beta}}}}_0} \left[ \frac{1}{r} \sum\limits_{i = 1}^N {\pi}_i^{\text{opt}} \left( \frac{{\widetilde{\bf{{\Omega}}}}_{i}^{*j_1 j_2}(\mathit{\boldsymbol{\Sigma}}_u, {\hat{\boldsymbol \beta}}_{\text{MLE}})}{N {\pi}_i^{\text{opt}}} \right)^2 \,\middle\vert\, \mathcal{F}_N, \tilde{\boldsymbol \beta}_0 \right] \\ = & \frac{1}{r} \sum\limits_{i = 1}^N \frac{\left( {\bf{\Omega}}_{i}^{*j_1 j_2}(\mathit{\boldsymbol{\Sigma}}_u, {\hat{\boldsymbol \beta}}_{\text{MLE}}) \right)^2}{N^2 {{\pi}}_i^{\text{opt}}} \\ = & O_P(r^{-1}). \end{aligned} |
It follows from Chebyshev's inequality that (A.7) holds. Similarly, (A.8) also holds.
E\left( \frac{1}{N} \mathit{\boldsymbol{Q}}_{\tilde{\boldsymbol \beta}_0}^{*}({\hat{\mathit{\boldsymbol{\beta}}}}_{\text{MLE}}) \,\middle\vert\, \mathcal{F}_N \right) = E_{\tilde{\boldsymbol \beta}_0} \left[ E\left( \frac{1}{N} \frac{1}{r} \sum\limits_{i = 1}^r \frac{{\tilde{\mathit{\boldsymbol{{\eta}}}}}_{i}^*(\mathit{\boldsymbol{\Sigma}}_u, {\hat{\mathit{\boldsymbol{\beta}}}}_{\text{MLE}})}{\widetilde{\pi}_i^{\text{opt}}} \,\middle\vert\, \mathcal{F}_N, {\tilde{\boldsymbol \beta}}_0 \right) \right] = \frac{1}{N} \sum\limits_{i = 1}^N \mathit{\boldsymbol{\eta}}_{i}^*(\mathit{\boldsymbol{\Sigma}}_u, {\hat{\mathit{\boldsymbol{\beta}}}}_{\text{MLE}}) = {{\mathbf{0}}}. |
By Assumption A6, we have
\begin{aligned} \text{Var}\left( \frac{1}{N} \mathit{\boldsymbol{Q}}_{\tilde{\boldsymbol \beta}_0}^{*}({\hat{\mathit{\boldsymbol{\beta}}}}_{\text{MLE}}) \,\middle\vert\, \mathcal{F}_N \right) & = E_{{\tilde{\boldsymbol \beta}}_0} \left\{\text{Var} \left[ \left( \frac{1}{N} \frac{1}{r} \sum\limits_{i = 1}^r \frac{{\tilde{\mathit{\boldsymbol{{\eta}}}}}_{i}^*(\mathit{\boldsymbol{\Sigma}}_u, {\hat{\mathit{\boldsymbol{\beta}}}}_{\text{MLE}})}{\widetilde{\pi}_i^{\text{opt}}}\right) \,\middle\vert\, \mathcal{F}_N, \tilde{\boldsymbol \beta}_0 \right] \right\}\\ & = \frac{1}{N^2 r} \sum\limits_{i = 1}^N \frac{\mathit{\boldsymbol{\eta}}_{i}^*(\mathit{\boldsymbol{\Sigma}}_u, {\hat{\mathit{\boldsymbol{\beta}}}}_{\text{MLE}}) {\mathit{\boldsymbol{\eta}}}_{i}^{*{\rm T}}(\mathit{\boldsymbol{\Sigma}}_u, {\hat{\mathit{\boldsymbol{\beta}}}}_{\text{MLE}})}{{\pi}_i^{\text{opt}}} \\ & = O_P(r^{-1}). \end{aligned} |
Therefore, the (A.9) and (A.10) follow from Markov's Inequality.
Let
\mathit{\boldsymbol{\gamma}} _{i,{{\mathit{\boldsymbol{\tilde \beta}}}_0}}^{*} = \frac{{\tilde{\mathit{\boldsymbol{\eta}}}_{_i}^*({{\mathit{\boldsymbol{\Sigma}}}_u},{{\hat{\mathit{\boldsymbol{\beta}}}}_{\text{MLE}}})}}{{N\widetilde \pi _i^{\text {opt}}}}, |
for all \varepsilon > 0 , it follows that {N}^{-1}\mathit{\boldsymbol{Q}}_{{{\tilde{\mathit{\boldsymbol{\beta}}}}_0}}^{*}({{\hat{\mathit{\boldsymbol{\beta}}}}_{\text{MLE}}}) = {r}^{-1}\sum\limits_{i = 1}^r {\mathit{\boldsymbol{\gamma}} _{i, {{\tilde{\mathit{\boldsymbol{\beta}}}}_0}}^{*}} ,
\begin{aligned} &\sum\limits_{i = 1}^r E_{\tilde{\boldsymbol \beta}_0} \left\{E\left[ \left\| r^{-\frac{1}{2}} \mathit{\boldsymbol{\gamma}}_{i,\tilde{\boldsymbol \beta}_0}^{*} \right\|^2 I \left( \left\| \mathit{\boldsymbol{\gamma}}_{i,\tilde{\boldsymbol \beta}_0}^{*} \right\| > r^{\frac{1}{2}} \varepsilon \right) \,\middle\vert\, \mathcal{F}_N, {\tilde{\boldsymbol \beta}}_0 \right] \right\} \\ = & \frac{1}{r} \sum\limits_{i = 1}^r E_{{\tilde{\boldsymbol \beta}}_0} \left\{ E \left[ \left\| \mathit{\boldsymbol{\gamma}}_{i,{\tilde{\boldsymbol \beta}_0}}^{*} \right\|^2 I \left( \left\| \mathit{\boldsymbol{\gamma}}_{i,\tilde{\boldsymbol \beta}_0}^{*} \right\| > r^{\frac{1}{2}} \varepsilon \right) \,\middle\vert\, \mathcal{F}_N, \tilde{\boldsymbol \beta}_0 \right] \right\} \\ \le & \frac{1}{r^{\frac{3}{2}} \varepsilon} \sum\limits_{i = 1}^r E_{\tilde{\boldsymbol \beta}_0} \left[ E \left( \left\| \mathit{\boldsymbol{\gamma}}_{i,{\tilde{\boldsymbol \beta}}_0}^{*} \right\|^3 \,\middle\vert\, \mathcal{F}_N, {\tilde{\boldsymbol \beta}}_0 \right) \right] \\ = & \frac{1}{r^{\frac{1}{2}} \varepsilon} \frac{1}{N^3} \sum\limits_{i = 1}^N \frac{\left\| {\tilde{\mathit{\boldsymbol{\eta}}}_{_i}^*({{\mathit{\boldsymbol{\Sigma}}}_u},{{\hat{\mathit{\boldsymbol{\beta}}}}_{\text{MLE}}})} \right\|^3}{\pi_i^{\text{opt}^2}} \\ = & O_P(r^{-\frac{1}{2}}) = o_P(1). \end{aligned} |
This shows that the Lindeberg-Feller conditions are satisfied in probability. Therefore (A.11) is true.
Lemma 4. If Assumptions A1–A7 hold, as r_0 \to \infty , r \to \infty and N \to \infty , for all {{{\boldsymbol{s}}}_{r_0}} \to \boldsymbol0 and {{{\boldsymbol{s}}}_r} \to {\mathbf{0}} , conditional on \mathcal{F}_N , we have
\begin{equation} \frac{1}{{N{r_0}}}\sum\limits_{i = 1}^{{r_0}} {\frac{{{\widetilde{\bf{ \Omega}}}_i^*({{\mathit{\boldsymbol{\Sigma}}}_u},{{\hat{\mathit{\boldsymbol{\beta}}}}_{\mathit{\text{MLE}}}} + {{{\boldsymbol{s}}}_{r_0}})}}{{\widetilde \pi _i^{\mathit{\text{opt}}}}}} - \frac{1}{N}\sum\limits_{i = 1}^N {{\bf{\Omega}}_i^*({{\mathit{\boldsymbol{\Sigma}}}_u},{{\hat{\mathit{\boldsymbol{\beta}}}}_{\mathit{\text{MLE}}}})} = {o_{P\mid\mathcal{F}_N}}(1), \end{equation} | (A.12) |
\begin{equation} \frac{1}{{N{r}}}\sum\limits_{i = 1}^{{r}} {\frac{{{\widetilde{\bf{ \Omega}}}_i^*({{\mathit{\boldsymbol{\Sigma}}}_u},{{\hat{\mathit{\boldsymbol{\beta}}}}_{\mathit{\text{MLE}}}} + {{{\boldsymbol{s}}}_r})}}{{\widetilde \pi _i^\mathit{\text{opt}}}}} - \frac{1}{N}\sum\limits_{i = 1}^N {{\bf{\Omega}}_i^*({{\mathit{\boldsymbol{\Sigma}}}_u},{{\hat{\mathit{\boldsymbol{\beta}}}}_{\mathit{\text{MLE}}}})} = {o_{P\mid\mathcal{F}_N}}(1). \end{equation} | (A.13) |
Proof. The Eq (A.12) can be written as
\frac{1}{{N{r_0}}}\sum\limits_{i = 1}^{{r_0}} {\frac{{{\widetilde{\bf{\Omega}}}_i^*({{\mathit{\boldsymbol{\Sigma}}}_u},{{\hat{\mathit{\boldsymbol{\beta}}}}_{\text{MLE}}} + {\mathit{\boldsymbol{s}}_{r_0}})}}{{{{\widetilde \pi }_i^{\text {opt}}}}}} - \frac{1}{{N{r_0}}}\sum\limits_{i = 1}^{{r_0}} {\frac{{{\widetilde{\bf{\Omega}}}_i^*({{\mathit{\boldsymbol{\Sigma}}}_u},{{\hat{\mathit{\boldsymbol{\beta}}}}_{\text{MLE}}})}}{{\widetilde \pi _i^{\text{opt}}}}} + \frac{1}{{N{r_0}}}\sum\limits_{i = 1}^{{r_0}} {\frac{{{\widetilde{\bf{\Omega}}}_i^*({{\mathit{\boldsymbol{\Sigma}}}_u},{{\hat{\mathit{\boldsymbol{\beta}}}}_{\text{MLE}}})}}{{\widetilde \pi _i^{\text{opt}}}}} - \frac{1}{N}\sum\limits_{i = 1}^N {{\bf{\Omega}}_i^*({{\mathit{\boldsymbol{\Sigma}}}_u},{{\hat{\mathit{\boldsymbol{\beta}}}}_{\text{MLE}}})}. |
Let
\mathit{\boldsymbol{\tau}}_1^0 : = \frac{1}{{N{r_0}}}\sum\limits_{i = 1}^{{r_0}} {\frac{{{\widetilde{\bf{\Omega}}}_i^*({{\mathit{\boldsymbol{\Sigma}}}_u},{{\hat{\mathit{\boldsymbol{\beta}}}}_{\text{MLE}}} + {\mathit{\boldsymbol{s}}_{r_0}})}}{{\widetilde \pi _i^{\text{opt}}}}} - \frac{1}{{N{r_0}}}\sum\limits_{i = 1}^{{r_0}} {\frac{{{\widetilde{\bf{ \Omega}}}_i^*({{\mathit{\boldsymbol{\Sigma}}}_u},{{\hat{\mathit{\boldsymbol{\beta}}}}_{\text{MLE}}})}}{{\widetilde \pi _i^{\text{opt}}}}}, |
then by Assumption A7, we have
\begin{aligned} E \left( \left\| \mathit{\boldsymbol{\tau}}_1^0 \right\|_S \,\middle\vert\, \mathcal{F}_N \right) = & E_{\tilde{\mathit{\boldsymbol{\beta}}}_0} \left\{ E \left[ \frac{1}{N r_0} \sum\limits_{i = 1}^{r_0} \frac{1}{\tilde{\pi}_i^{\text{opt}}} \left\| {\widetilde{\bf{\Omega}}}_{i}^*(\mathit{\boldsymbol{\Sigma}}_u, {\hat{\mathit{\boldsymbol{\beta}}}}_{\text{MLE}} +{\mathit{\boldsymbol{s}}_{r_0}}) - {\widetilde{\bf{\Omega}}}_{i}^*(\mathit{\boldsymbol{\Sigma}}_u, {\hat{\mathit{\boldsymbol{\beta}}}}_{\text{MLE}}) \right\|_S \,\middle\vert\, \mathcal{F}_N, {\tilde{\mathit{\boldsymbol{\beta}}}}_0 \right] \right\} \\ = & \frac{1}{N} \sum\limits_{i = 1}^N \left\|{\bf{\Omega}}_{i}^*(\mathit{\boldsymbol{\Sigma}}_u, {\hat{\mathit{\boldsymbol{\beta}}}}_{\text{MLE}} + {\mathit{\boldsymbol{s}}_{r_0}}) - {\bf{\Omega}}_{i}^*(\mathit{\boldsymbol{\Sigma}}_u, {\hat{\mathit{\boldsymbol{\beta}}}}_{\text{MLE}})\right\|_S \\ \le& \frac{1}{N} \sum\limits_{i = 1}^N m_2(\mathit{\boldsymbol{W}}_i) \left\| {\mathit{\boldsymbol{s}}_{r_0}} \right\| \\ = & o_P(1). \end{aligned} |
It follows from Markov's inequality that \mathit{\boldsymbol{\tau}}_1^0 = {o_{P\mid\mathcal{F}_N}}(1) .
Let
\mathit{\boldsymbol{\tau}}_2^0 : = \frac{1}{{N{r_0}}}\sum\limits_{i = 1}^{{r_0}} {\frac{{{\widetilde{\bf{\Omega}}}_i^*({{\mathit{\boldsymbol{\Sigma}}}_u},{{\hat{\mathit{\boldsymbol{\beta}}}}_{\text{MLE}}})}}{{\widetilde \pi _i^{\text{opt}}}}} - \frac{1}{N}\sum\limits_{i = 1}^N {{\bf{\Omega}}_i^*({{\mathit{\boldsymbol{\Sigma}}}_u},{{\hat{\mathit{\boldsymbol{\beta}}}}_{\text{MLE}}})}, |
then
\begin{aligned} E_{\tilde{\boldsymbol \beta}_0} \left\{ E \left[ \frac{1}{N r_0} \sum\limits_{i = 1}^{r_0} \frac{{\widetilde{\bf{\Omega}}}_{i}^*\left(\mathit{\boldsymbol{\Sigma}}_u, {\hat{\mathit{\boldsymbol{\beta}}}}_{\text{MLE}} + {\mathit{\boldsymbol{s}}_{r_0}}\right)}{\widetilde{\pi}_i^{\text {opt}}} \,\middle\vert\, \mathcal{F}_N, {\tilde{\boldsymbol \beta}}_0 \right] \right\} & = \frac{1}{N} \sum\limits_{i = 1}^N {\bf{\Omega}}_{i}^*(\mathit{\boldsymbol{\Sigma}}_u, {\hat{\mathit{\boldsymbol{\beta}}}}_{\text{MLE}}). \end{aligned} |
By the proof of Lemma 3, it follows that
E\left[ \left(\overset{\smile}{\bf{M}}_X^{\tilde{\boldsymbol \beta}_0, j_1 j_2} - {{\bf{M}}}_X^{j_1 j_2} \right)^2 \,\middle\vert\, \mathcal{F}_N \right] = {O_P}({r_0}^{-1}) = {o_P}(1), |
we have \mathit{\boldsymbol{\tau}}_2^0 = {o_{P\mid\mathcal{F}_N}}(1) . Therefore (A.12) holds. Similarly, (A.13) is also true.
Next, we will prove Theorems 2.5 and 2.6.
Proof of Theorem 2.5.
E\left( \frac{1}{N} \mathit{\boldsymbol{Q}}_{{\tilde{\boldsymbol \beta}}_0}^{*}(\mathit{\boldsymbol{\beta}}) \,\middle\vert\, \mathcal{F}_N \right) = E_{\tilde{\boldsymbol \beta}_0} \left[ E\left( \frac{1}{N} \frac{1}{r} \sum\limits_{i = 1}^r \frac{{\tilde{\mathit{\boldsymbol{\eta}}}}_{i}^*(\mathit{\boldsymbol{\Sigma}}_u, \mathit{\boldsymbol{\beta}})}{\widetilde{\pi}_i^{\text{opt}}} \,\middle\vert\, \mathcal{F}_N, \tilde{\boldsymbol \beta}_0 \right) \right] = \frac{1}{N} \sum\limits_{i = 1}^N \mathit{\boldsymbol{\eta}}_{i}^*(\mathit{\boldsymbol{\Sigma}}_u, \mathit{\boldsymbol{\beta}}) = \frac{1}{N} \mathit{\boldsymbol{Q}}(\mathit{\boldsymbol{\beta}}). |
By Assumption A6, we have
{\text{Var}}\left( \frac{1}{N} {\mathit{\boldsymbol{Q}}}_{{\tilde{\mathit{\boldsymbol{\beta}}}}_0}^{*}({\mathit{\boldsymbol{\beta}}}) \,\middle\vert\, \mathcal{F}_N \right) = E_{\tilde{\mathit{\boldsymbol{\beta}}}_0} \left\{ \text {Var} \left( \frac{1}{N} \frac{1}{r} \sum\limits_{i = 1}^r \frac{{\tilde{\mathit{\boldsymbol{\eta}}}}_{i}^*(\mathit{\boldsymbol{\Sigma}}_u, \mathit{\boldsymbol{\beta}})}{\widetilde{\pi}_i^{\text{opt}}} \,\middle\vert\, \mathcal{F}_N, \tilde{\mathit{\boldsymbol{\beta}}}_0 \right) \right\} = \frac{1}{N^2 r} \sum\limits_{i = 1}^N \frac{\mathit{\boldsymbol{\eta}}_{i}^*(\mathit{\boldsymbol{\Sigma}}_u, \mathit{\boldsymbol{\beta}}) \mathit{\boldsymbol{\eta}}_{i}^{*{\rm T}}(\mathit{\boldsymbol{\Sigma}}_u, \mathit{\boldsymbol{\beta}})}{\pi_i^{\text{opt}}} = O_P(r^{-1}). |
Hence, as r \to \infty , {N}^{-1} \mathit{\boldsymbol{Q}}_{{\tilde{\mathit{\boldsymbol{\beta}}}}_0}^{*}(\mathit{\boldsymbol{\beta}}) - {N}^{-1} \mathit{\boldsymbol{Q}}(\mathit{\boldsymbol{\beta}})\mathop \to \limits^{} {{\mathbf{0}}} for all {\boldsymbol \beta} \in \Lambda in conditional probability given \mathcal{F}_N .
\check {\mathit{\boldsymbol{\beta}}} is the solution of \mathit{\boldsymbol{Q}}_{{{{\tilde{\boldsymbol \beta}}}_0}}^{two - step}(\mathit{\boldsymbol{\beta}}) = {\mathbf{0}} , we have
\begin{equation} {\mathbf{0}} = \frac{1}{N}\mathit{\boldsymbol{Q}}_{{{\tilde{\mathit{\boldsymbol{{\beta}}}}}_0}}^{two - step}(\check{\mathit{\boldsymbol{\beta}}}) = \frac{r}{{r + {r_0}}}\frac{1}{N}\mathit{\boldsymbol{Q}}_{{\tilde{\boldsymbol \beta}}_0}^{*}({\check{\mathit{\boldsymbol{\beta}}}}) + \frac{{{r_0}}}{{r + {r_0}}}\frac{1}{N}{\mathit{\boldsymbol{Q}}}_{{{\tilde{\mathit{\boldsymbol{\beta}}}}_0}}^{*0}(\check{\mathit{\boldsymbol{\beta}}}). \end{equation} | (A.14) |
By Lemma 4, we have
\frac{1}{N r_0} \sum\limits_{i = 1}^{r_0} \frac{{\widetilde{\bf{\Omega}}}_{i}^*(\mathit{\boldsymbol{\Sigma}}_u, {\hat{\mathit{\boldsymbol{\beta}}}}_{\text{MLE}} + {{{\boldsymbol{s}}}_{r_0}})}{\widetilde{\pi}_i^{\text{opt}}} = \frac{1}{N} \sum\limits_{i = 1}^N {\bf{\Omega}}_{i}^*(\mathit{\boldsymbol{\Sigma}}_u, {\hat{\mathit{\boldsymbol{\beta}}}}_{\text{MLE}}) + o_{P|\mathcal{F}_N}(1) = {\bf{M}_X} + {o_{P|\mathcal{F}_N}(1)}, |
and
\frac{1}{{Nr}}\sum\limits_{i = 1}^r {\frac{{{\widetilde{\bf{\Omega}}}_i^*({{\mathit{\boldsymbol{\Sigma}}}_u},{{\hat{\mathit{\boldsymbol{\beta}}}}_{MLE}} + {{{\boldsymbol{s}}}_{r}})}}{{\widetilde \pi _i^{\text {opt}}}}} = {{\bf{M}}_X} + {o_{P|\mathcal{F}_N}(1)}. |
By Taylor expansion, we have
\begin{equation} \begin{aligned} \frac{1}{N} \mathit{\boldsymbol{Q}}_{\tilde{\mathit{\boldsymbol{\beta}}}_0}^{*}(\check{\mathit{\boldsymbol{{\beta}}}}) & = \frac{1}{N} \mathit{\boldsymbol{Q}}_{\tilde{\boldsymbol \beta}_0}^{*}(\hat{\mathit{\boldsymbol{\beta}}}_{\text{MLE}}) + \frac{1}{Nr} \sum\limits_{i = 1}^r \frac{{\bf{\Omega}}_i^*(\mathit{\boldsymbol{\Sigma}}_u, {\hat{\mathit{\boldsymbol{\beta}}}}_{\text{MLE}} + {{{\boldsymbol{s}}}_{r}})}{\widetilde{\pi}_i^{\text{opt}}} (\check{\mathit{\boldsymbol{\beta}}} - {\hat{\mathit{\boldsymbol{\beta}}}}_{\text{MLE}}) \\ & = \frac{1}{N} \mathit{\boldsymbol{Q}}_{{\tilde{\boldsymbol \beta}_0}}^{*}({\hat{\mathit{\boldsymbol{\beta}}}}_{\text{MLE}}) + {\bf{M}}_X ({\hat{\mathit{\boldsymbol{{\beta}}}}} - {\hat{\mathit{\boldsymbol{\beta}}}}_{\text{MLE}}) + o_{P|\mathcal{F}_N}(1) (\check {\mathit{\boldsymbol{\beta}}} - {\hat{\mathit{\boldsymbol{\beta}}}}_{\text{MLE}}). \end{aligned} \end{equation} | (A.15) |
Similarly,
\begin{equation} \frac{1}{N}{\mathit{\boldsymbol{Q}}}_{{\tilde{\mathit{\boldsymbol{\beta}}}}_0}^{*0}({\check{\mathit{\boldsymbol{\beta}}}}) = \frac{1}{N}\mathit{\boldsymbol{Q}}_{{\tilde{\mathit{\boldsymbol{\beta}}}}_0}^{*0}({\hat{\mathit{\boldsymbol{\beta}}}_{\text{MLE}}}) + {{\bf{M}}_X}(\check{\mathit{\boldsymbol{\beta}}} - {{\hat{\mathit{\boldsymbol{\beta}}}}_{\text{MLE}}}) + {o_{P|\mathcal{F}_N}}(1)(\check{\mathit{\boldsymbol{\beta}}} - {{\hat{\mathit{\boldsymbol{\beta}}}}_{\text{MLE}}}). \end{equation} | (A.16) |
As {{{r_0}}}{{r}^{-1}} \to 0, {N}^{-1} \mathit{\boldsymbol{Q}}_{{{\tilde{\mathit{\boldsymbol{\beta}}}}_0}}^{*0}({{\hat{\mathit{\boldsymbol{\beta}}}}_{\text{MLE}}}) = {O_{P|\mathcal{F}_N}}\left(r_0^{ - \frac{1}{2}}\right) , then
\frac{r_0}{r + r_0} \frac{1}{N} \mathit{\boldsymbol{Q}}_{\tilde{\boldsymbol \beta}_0}^{*0}(\hat{\mathit{\boldsymbol{\beta}}}_{\text{MLE}}) = \frac{r_0}{r + r_0} {O_{P|\mathcal{F}_N}}(r_0^{-\frac{1}{2}}) = {o_{P|\mathcal{F}_N}}(r^{-\frac{1}{2}}). |
Combining this with (A.14)–(A.16), we have
\begin{equation} {\check {\boldsymbol \beta}} - {\hat{\mathit{\boldsymbol{\beta}}}}_{\text{MLE}} = {O_{P|\mathcal{F}_N}} \left( r^{ - \frac{1}{2}} \right) + {o_{P|\mathcal{F}_N}} \left(\left\| {\check {\boldsymbol \beta}} - {\hat{\mathit{\boldsymbol{\beta}}}}_{\text{MLE}} \right\| \right), \end{equation} | (A.17) |
which implies that {\check {\boldsymbol \beta}} - {\hat{\mathit{\boldsymbol{\beta}}}}_{\text{MLE}} = {O_{P|\mathcal{F}_N}} \left(r^{ - \frac{1}{2}} \right) .
Proof of Theorem 2.6. By Lemma 3, \frac{1}{N}{\bf{V}}_{\text {C}}^{ - \frac{1}{2}}\mathit{\boldsymbol{Q}}_{{{\tilde{\mathit{\boldsymbol{\beta}}}}_0}}^{*}({{\hat{\mathit{\boldsymbol{\beta}}}}_{\text{MLE}}})\mathop \to \limits^d N({{\mathbf{0}}}, {\bf I}) , we have
\begin{aligned} \left\| {\bf{V}}_{\text {C}} - {\bf{V}}_{\text {C}}^{\text{opt}} \right\|_S & = \left\| \frac{1}{N^2 r} \sum\limits_{i = 1}^N \frac{\mathit{\boldsymbol{\eta}}_{i}^*(\mathit{\boldsymbol{\Sigma}}_u, \hat{\mathit{\boldsymbol{\beta}}}_{\text{MLE}}) \mathit{\boldsymbol{\eta}}_{i}^{*{\rm T}}(\mathit{\boldsymbol{\Sigma}}_u, {\hat{\mathit{\boldsymbol{\beta}}}}_{\text{MLE}})}{\pi_i^{\text{op}}} - \frac{1}{N^2 r} \sum\limits_{i = 1}^N \frac{\mathit{\boldsymbol{\eta}}_{i}^*(\mathit{\boldsymbol{\Sigma}}_u, {\hat{\mathit{\boldsymbol{\beta}}}}_{\text{MLE}}) {\mathit{\boldsymbol{\eta}}}_{i}^{*{\rm T}}(\mathit{\boldsymbol{\Sigma}}_u, {\hat{\mathit{\boldsymbol{\beta}}}}_{\text{MLE}})}{\pi_i^{\text{opt}}} \right\|_S \\ &\le \frac{1}{N^2 r} \sum\limits_{i = 1}^N \left\| \frac{1}{\pi_i^{\text{op}}} - \frac{1}{\pi_i^{\text{opt}}} \right\| \left\| {\mathit{\boldsymbol{\eta}}}_{i}^*(\mathit{\boldsymbol{\Sigma}}_u, {\hat{\mathit{\boldsymbol{\beta}}}}_{\text{MLE}}) \right\|^2 \\ & = \frac{1}{r} \sum\limits_{i = 1}^N \left\| 1 - \frac{\pi_i^{\text{op}}}{\pi_i^{\text{opt}}} \right\| \frac{\left\| {\mathit{\boldsymbol{\eta}}}_{i}^*(\mathit{\boldsymbol{\Sigma}}_u, {\hat{\mathit{\boldsymbol{\beta}}}}_{\text{MLE}}) \right\|^2}{N^2 \pi_i^{\text{op}}}. \end{aligned} |
Taking \pi _i^{\text{mVc}} as an example, by Assumpion A4, the above equation can be summarized as
\begin{aligned} &\frac{1}{r} \sum\limits_{i = 1}^N \left\| 1 - \frac{\pi_i^{\text{mVc}}}{\pi_i^{\text{mVct}}} \right\| \frac{\left\| \mathit{\boldsymbol{\eta}}_{i}^*(\mathit{\boldsymbol{\Sigma}}_u, {\hat{\mathit{\boldsymbol{\beta}}}}_{\text{MLE}}) \right\|^2}{N^2} \frac{\sum\limits_{j = 1}^N g_j^{\text{mVc}}}{g_i^{\text{mVc}}} \\ = & \frac{1}{r} \sum\limits_{i = 1}^N \left\| 1 - \frac{\pi_i^{\text{mVc}}}{\pi_i^{\text{mVct}}} \right\| \frac{\left\| \mathit{\boldsymbol{\eta}}_{i}^*(\mathit{\boldsymbol{\Sigma}}_u, {\hat{\mathit{\boldsymbol{\beta}}}}_{\text{MLE}}) \right\|^2}{N^2} \frac{\sum\limits_{j = 1}^N \left\| {\mathit{\boldsymbol{\eta}}}_j^*(\mathit{\boldsymbol{\Sigma}}_u, {\hat{\mathit{\boldsymbol{\beta}}}}_{\text{MLE}}) \right\| }{\left\| \mathit{\boldsymbol{\eta}}_i^*(\mathit{\boldsymbol{\Sigma}}_u, {\hat{\mathit{\boldsymbol{\beta}}}}_{\text{MLE}}) \right\| } \\ \le & \frac{1}{r} \sum\limits_{i = 1}^N \left\| 1 - \frac{\pi_i^{\text{mVc}}}{\pi_i^{\text{mVct}}} \right\| \frac{\left\| {\mathit{\boldsymbol{\eta}}}_{i}^*(\mathit{\boldsymbol{\Sigma}}_u, {\hat{\mathit{\boldsymbol{\beta}}}}_{\text{MLE}}) \right\|}{N} \frac{\sum\limits_{j = 1}^N \left\| {\mathit{\boldsymbol{\eta}}}_j^*(\mathit{\boldsymbol{\Sigma}}_u, {\hat{\mathit{\boldsymbol{\beta}}}}_{\text{MLE}}) \right\| }{N} \\ \le &\frac{1}{r} \left( \frac{1}{N} \sum\limits_{i = 1}^N \left\| 1 - \frac{\pi_i^{\text{mVc}}}{\pi_i^{\text{mVct}}} \right\|^2 \right)^{\frac{1}{2}} \left( \sum\limits_{i = 1}^N \frac{\left\| {\mathit{\boldsymbol{\eta}}}_{i}^*(\mathit{\boldsymbol{\Sigma}}_u, {\hat{\mathit{\boldsymbol{\beta}}}}_{\text{MLE}}) \right\|^2}{N} \right)^{\frac{1}{2}} \left(\frac{\sum\limits_{j = 1}^N \left\| {\mathit{\boldsymbol{\eta}}}_j^*(\mathit{\boldsymbol{\Sigma}}_u, {\hat{\mathit{\boldsymbol{\beta}}}}_{\text{MLE}}) \right\| }{N} \right) \\ = & {o_{P\mid\mathcal{F}_N}}\left( {{r^{ - 1}}} \right). \end{aligned} |
Therefore {\left\| {{{\bf{V}}_{\text {C}}} - {\bf{V}}_{\text {C}}^{opt}} \right\|_S} = {o_{P\mid\mathcal{F}_N}}\left({{r^{ - 1}}} \right) , and
\begin{aligned} {\bf{V}}_{\text {opt}}^{-\frac{1}{2}}(\check{\mathit{\boldsymbol{{\beta}}}} - {\hat{\mathit{\boldsymbol{\beta}}}}_{\text{MLE}}) & = - {\bf{V}}_{\text {opt}}^{-\frac{1}{2}}{\bf{M}}_X^{-1}\frac{1}{N}\mathit{\boldsymbol{Q}}_{{\tilde{\mathit{\boldsymbol{\beta}}}}_0}^{two-step}({\hat{\mathit{\boldsymbol{\beta}}}}_{\text{MLE}}) + o_{P|\mathcal{F}_N}(1) \\ & = - {\bf{V}}_{\text {opt}}^{-\frac{1}{2}}{\bf{M}}_X^{-1} \left[ \frac{r}{r + r_0} \frac{1}{N}\mathit{\boldsymbol{Q}}_{{\tilde{\mathit{\boldsymbol{\beta}}}}_0}^{*}(\check{\mathit{\boldsymbol{\beta}}}) + \frac{r_0}{r + r_0} \frac{1}{N}\mathit{\boldsymbol{Q}}_{{\tilde{\mathit{\boldsymbol{\beta}}}}_0}^{*0}(\check{\mathit{\boldsymbol{\beta}}}) \right] + o_{P|\mathcal{F}_N}(1) \\ & = - {\bf{V}}_{\text {opt}}^{-\frac{1}{2}}{\bf{M}}_X^{-1}{\left( {\bf{V}}_{\text {C}}^{\text {opt}} \right)^{\frac{1}{2}}}{\left( {\bf{V}}_{\text {C}}^{\text {opt}} \right)^{-\frac{1}{2}}}\frac{1}{N}\mathit{\boldsymbol{Q}}_{{\tilde{\mathit{\boldsymbol{\beta}}}}_0}^{*}(\check{\mathit{\boldsymbol{\beta}}}) + o_{P|\mathcal{F}_N}(1), \end{aligned} |
which implies that
{\bf{V}}_{\text {opt}}^{ - \frac{1}{2}}{\bf{M}}_X^{ - 1}{\left( {{\bf{V}}_{\text {C}}^{\text {opt}}} \right)^{\frac{1}{2}}}{\left\{ {{\bf{V}}_{\text {opt}}^{ - \frac{1}{2}}{\bf{M}}_X^{ - 1}{{\left( {{\bf{V}}_{\text {C}}^{\text {opt}}} \right)}^{\frac{1}{2}}}} \right\}^{\rm T}} = {\bf{V}}_{\text {opt}}^{ - \frac{1}{2}}{\bf{M}}_X^{ - 1}{\left( {{\bf{V}}_{\text {C}}^{\text {opt}}} \right)^{\frac{1}{2}}}{\left( {{\bf{V}}_{\text {C}}^{{\text {opt}}}} \right)^{\frac{1}{2}}}{\bf{M}}_X^{ - 1}{\bf{V}}_{\text {opt}}^{ - \frac{1}{2}} = {\bf{I}}. |
\\ Therefore
{\bf{V}}_{\text {opt}}^{ - \frac{1}{2}}({\check{\mathit{\boldsymbol{\beta}}}} - {{\mathit{\boldsymbol{\hat {\beta}}}}_{\text{MLE}}})\mathop \to \limits^d N_p({{\mathbf{0}}},{\bf I}). |
[1] |
R. P. Agarwal, Y. M. Chow, Iterative method for fourth order boundary value problem, J. Comput. Appl. Math., 10 (1984), 203–217. doi: 10.1016/0377-0427(84)90058-X
![]() |
[2] |
Z. B. Bai, H. Y. Wang, On positive solutions of some nonlinear four-order beam equations, J. Math. Anal. Appl., 270 (2002), 357–368. doi: 10.1016/S0022-247X(02)00071-9
![]() |
[3] | Z. B. Bai, The upper and lower solution method for some fourth-order boundary value problems, Nonlinear Anal.-Theor.,, 67 (2007), 1704–1709. |
[4] |
G. Bonanno, B. D. Bella, A boundary value problem for fourth-order elastic beam equations, J. Math. Anal. Appl., 343 (2008), 1166–1176. doi: 10.1016/j.jmaa.2008.01.049
![]() |
[5] |
G. Bonanno, B. D. Bella, D. O'Regan, Non-trivial solutions for nonlinear fourth-order elastic beam equations, Comput. Math. Appl., 62 (2011), 1862–1869. doi: 10.1016/j.camwa.2011.06.029
![]() |
[6] | R. Graef, B. Yang, Positive solutions of a nonlinear fourth order boundary value problem, Communications on Applied Nonlinear Analysis, 14 (2007), 61–73. |
[7] |
C. P. Gupta, Existence and uniqueness results for the bending of an elastic beam equation, Appl. Anal., 26 (1988), 289–304. doi: 10.1080/00036818808839715
![]() |
[8] |
P. Korman, Uniqueness and exact multiplicity of solutions for a class of fourth-order semilinear problems, P. Roy. Soc. Edinb. A, 134 (2004), 179–190. doi: 10.1017/S0308210500003140
![]() |
[9] |
B. D. Lou, Positive solutions for nonlinear elastic beam models, International Journal of Mathematics and Mathematical Sciences, 27 (2001), 365–375. doi: 10.1155/S0161171201004203
![]() |
[10] |
R. Y. Ma, L. Xu, Existence of positive solutions of a nonlinear fourth-order boundary value problem, Appl. Math. Lett., 23 (2010), 537–543. doi: 10.1016/j.aml.2010.01.007
![]() |
[11] |
Q. L. Yao, Positive solutions for eigenvalue problems of four-order elastic beam equations, Appl. Math. Lett., 17 (2004), 237–243. doi: 10.1016/S0893-9659(04)90037-7
![]() |
[12] |
Q. L. Yao, Existence of n solutions and/or positive solutions to a semipositone elastic beam equation, Nonlinear Anal.-Theor., 66 (2007), 138–150. doi: 10.1016/j.na.2005.11.016
![]() |
[13] |
Q. L. Yao, positive solutions of nonlinear elastic beam equation rigidly fastened on the left and simply supported on the right, Nonlinear Anal.-Theor., 69 (2008), 1570–1580. doi: 10.1016/j.na.2007.07.002
![]() |
[14] |
C. B. Zhai, R. P. Song, Q. Q. Han, The existence and the uniqueness of symmetric positive solutions for a fourth-order boundary value problem, Comput. Math. Appl., 62 (2011), 2639–2647. doi: 10.1016/j.camwa.2011.08.003
![]() |
[15] |
X. P. Zhang, Existence and iteration of monotone positive solutions for an elastic beam equation with a corner, Nonlinear Anal.-Real, 10 (2009), 2097–2103. doi: 10.1016/j.nonrwa.2008.03.017
![]() |
[16] | K. Deimling, Nonlinear Functional Analysis, Springer-Verlag, Berlin-Heidelberg-Newyork, 1985. |
[17] | D. J. Guo, V. Lakshmikanthan, Nonlinear Problems in Abstract Cones, Academic press, San Diego, 1988. |
[18] | D. J. Guo, Nonlinear Functional Analysis, second edn., Shandong Science and Technology Press, Jinan, 2001 (in Chinese). |
[19] |
H. Amann, Fixed point equations and nonlinear eigenvalue problems in ordered Banach spaces, SIAM Rev., 18 (1976), 620–709. doi: 10.1137/1018114
![]() |
[20] |
G. W. Zhang, J. X. Sun, Positive solutions of m-point boundary value problems, J. Math. Anal. Appl., 291 (2004), 406–418. doi: 10.1016/j.jmaa.2003.11.034
![]() |
uniform | mV | mVc | |||||||
Case | r | Coverage | Length | Coverage | Length | Coverage | Length | ||
Case 1 | 500 | 0.958 | 0.565 | 0.932 | 0.331 | 0.942 | 0.457 | ||
1000 | 0.952 | 0.453 | 0.925 | 0.248 | 0.954 | 0.333 | |||
1500 | 0.960 | 0.387 | 0.920 | 0.206 | 0.964 | 0.274 | |||
2000 | 0.932 | 0.345 | 0.907 | 0.180 | 0.954 | 0.237 | |||
2500 | 0.938 | 0.313 | 0.910 | 0.160 | 0.956 | 0.211 | |||
5000 | 0.964 | 0.302 | 0.908 | 0.148 | 0.937 | 0.202 | |||
Case 2 | 500 | 0.956 | 0.634 | 0.946 | 0.602 | 0.962 | 0.613 | ||
1000 | 0.946 | 0.621 | 0.934 | 0.586 | 0.946 | 0.593 | |||
1500 | 0.927 | 0.597 | 0.954 | 0.551 | 0.962 | 0.561 | |||
2000 | 0.943 | 0.543 | 0.956 | 0.524 | 0.921 | 0.518 | |||
2500 | 0.970 | 0.475 | 0.958 | 0.453 | 0.944 | 0.462 | |||
5000 | 0.963 | 0.438 | 0.932 | 0.417 | 0.947 | 0.441 | |||
Case 3 | 500 | 0.958 | 0.706 | 0.956 | 0.432 | 0.968 | 0.550 | ||
1000 | 0.946 | 0.561 | 0.972 | 0.399 | 0.970 | 0.409 | |||
1500 | 0.944 | 0.479 | 0.968 | 0.321 | 0.960 | 0.329 | |||
2000 | 0.936 | 0.425 | 0.964 | 0.265 | 0.958 | 0.281 | |||
2500 | 0.926 | 0.389 | 0.966 | 0.249 | 0.954 | 0.250 | |||
5000 | 0.915 | 0.356 | 0.947 | 0.220 | 0.942 | 0.236 |
uniform | mV | mVc | |||||||
Case | r | Coverage | Length | Coverage | Length | Coverage | Length | ||
Case 1 | 500 | 0.962 | 0.441 | 0.962 | 0.383 | 0.958 | 0.399 | ||
1000 | 0.944 | 0.352 | 0.964 | 0.291 | 0.964 | 0.304 | |||
1500 | 0.932 | 0.302 | 0.964 | 0.241 | 0.966 | 0.255 | |||
2000 | 0.952 | 0.268 | 0.930 | 0.210 | 0.944 | 0.223 | |||
2500 | 0.946 | 0.244 | 0.958 | 0.188 | 0.974 | 0.201 | |||
5000 | 0.952 | 0.234 | 0.961 | 0.173 | 0.943 | 0.185 | |||
Case 2 | 500 | 0.938 | 0.127 | 0.936 | 0.108 | 0.948 | 0.109 | ||
1000 | 0.936 | 0.102 | 0.946 | 0.082 | 0.934 | 0.082 | |||
1500 | 0.942 | 0.087 | 0.934 | 0.069 | 0.936 | 0.068 | |||
2000 | 0.952 | 0.078 | 0.956 | 0.060 | 0.952 | 0.059 | |||
2500 | 0.946 | 0.071 | 0.932 | 0.053 | 0.944 | 0.053 | |||
5000 | 0.935 | 0.068 | 0.965 | 0.045 | 0.971 | 0.047 | |||
Case 3 | 500 | 0.940 | 0.185 | 0.936 | 0.153 | 0.953 | 0.156 | ||
1000 | 0.950 | 0.148 | 0.954 | 0.113 | 0.958 | 0.118 | |||
1500 | 0.932 | 0.127 | 0.950 | 0.094 | 0.958 | 0.099 | |||
2000 | 0.946 | 0.113 | 0.952 | 0.082 | 0.960 | 0.086 | |||
2500 | 0.942 | 0.103 | 0.932 | 0.073 | 0.950 | 0.077 | |||
5000 | 0.937 | 0.096 | 0.956 | 0.065 | 0.964 | 0.061 |
r | ||||||
Method | 300 | 500 | 800 | 1200 | 1600 | 2000 |
uniform | 0.2993 | 0.3337 | 0.4985 | 0.5632 | 0.8547 | 0.5083 |
mV | 3.5461 | 3.6485 | 3.8623 | 4.1256 | 4.4325 | 5.2365 |
mVc | 3.2852 | 3.3658 | 3.5463 | 3.8562 | 4.0235 | 4.4235 |
Full | 45.9075 |
r | ||||||
Method | 300 | 500 | 800 | 1200 | 1600 | 2000 |
uniform | 0.4213 | 0.4868 | 0.5327 | 0.5932 | 0.7147 | 0.8883 |
mV | 4.6723 | 4.8963 | 5.2369 | 5.6524 | 6.0128 | 6.3567 |
mVc | 4.3521 | 4.6329 | 4.9658 | 5.2156 | 5.7652 | 5.9635 |
Full | 51.2603 |
uniform | mV | mVc | |
\text{Intercept} | -1.6084(0.069) | -1.5998(0.055) | -1.3122(0.052) |
{\check{\beta}}_1^{\text{err}} | 1.2879(0.205) | 1.1880(0.103) | 1.2038(0.097) |
{\check{\beta}}_2^{\text{err}} | 0.0105(0.106) | 0.0104(0.059) | 0.0111(0.046) |
{\check{\beta}}_3^{\text{err}} | 1.0033(0.201) | 0.9217(0.067) | 0.9199(0.054) |
{\check{\beta}}_4^{\text{err}} | 0.2636(0.094) | 0.2698(0.054) | 0.2555(0.063) |
{\check{\beta}}_5^{\text{err}} | 0.9469(0.229) | 0.8741(0.083) | 0.8628(0.076) |
uniform | mV | mVc | |
\text{Intercept} | -8.7934(0.0678) | -8.8105(0.0562) | -8.8135(0.0543) |
{\check{\beta}}_1^{\text{err}} | -0.6123(0.341) | -0.6047(0.142) | -0.6035(0.105) |
{\check{\beta}}_2^{\text{err}} | 0.0712(0.125) | 0.0730(0.064) | 0.0798(0.088) |
{\check{\beta}}_3^{\text{err}} | -0.9321(0.245) | -0.9087(0.067) | -0.9123(0.057) |
{\check{\beta}}_4^{\text{err}} | 1.4618(0.198) | 1.4580(0.054) | 1.4603(0.075) |
{\check{\beta}}_5^{\text{err}} | -0.1435(0.531) | -0.1347(0.242) | -0.1408(0.225) |
uniform | mV | mVc | |||||||
Case | r | Coverage | Length | Coverage | Length | Coverage | Length | ||
Case 1 | 500 | 0.958 | 0.565 | 0.932 | 0.331 | 0.942 | 0.457 | ||
1000 | 0.952 | 0.453 | 0.925 | 0.248 | 0.954 | 0.333 | |||
1500 | 0.960 | 0.387 | 0.920 | 0.206 | 0.964 | 0.274 | |||
2000 | 0.932 | 0.345 | 0.907 | 0.180 | 0.954 | 0.237 | |||
2500 | 0.938 | 0.313 | 0.910 | 0.160 | 0.956 | 0.211 | |||
5000 | 0.964 | 0.302 | 0.908 | 0.148 | 0.937 | 0.202 | |||
Case 2 | 500 | 0.956 | 0.634 | 0.946 | 0.602 | 0.962 | 0.613 | ||
1000 | 0.946 | 0.621 | 0.934 | 0.586 | 0.946 | 0.593 | |||
1500 | 0.927 | 0.597 | 0.954 | 0.551 | 0.962 | 0.561 | |||
2000 | 0.943 | 0.543 | 0.956 | 0.524 | 0.921 | 0.518 | |||
2500 | 0.970 | 0.475 | 0.958 | 0.453 | 0.944 | 0.462 | |||
5000 | 0.963 | 0.438 | 0.932 | 0.417 | 0.947 | 0.441 | |||
Case 3 | 500 | 0.958 | 0.706 | 0.956 | 0.432 | 0.968 | 0.550 | ||
1000 | 0.946 | 0.561 | 0.972 | 0.399 | 0.970 | 0.409 | |||
1500 | 0.944 | 0.479 | 0.968 | 0.321 | 0.960 | 0.329 | |||
2000 | 0.936 | 0.425 | 0.964 | 0.265 | 0.958 | 0.281 | |||
2500 | 0.926 | 0.389 | 0.966 | 0.249 | 0.954 | 0.250 | |||
5000 | 0.915 | 0.356 | 0.947 | 0.220 | 0.942 | 0.236 |
uniform | mV | mVc | |||||||
Case | r | Coverage | Length | Coverage | Length | Coverage | Length | ||
Case 1 | 500 | 0.962 | 0.441 | 0.962 | 0.383 | 0.958 | 0.399 | ||
1000 | 0.944 | 0.352 | 0.964 | 0.291 | 0.964 | 0.304 | |||
1500 | 0.932 | 0.302 | 0.964 | 0.241 | 0.966 | 0.255 | |||
2000 | 0.952 | 0.268 | 0.930 | 0.210 | 0.944 | 0.223 | |||
2500 | 0.946 | 0.244 | 0.958 | 0.188 | 0.974 | 0.201 | |||
5000 | 0.952 | 0.234 | 0.961 | 0.173 | 0.943 | 0.185 | |||
Case 2 | 500 | 0.938 | 0.127 | 0.936 | 0.108 | 0.948 | 0.109 | ||
1000 | 0.936 | 0.102 | 0.946 | 0.082 | 0.934 | 0.082 | |||
1500 | 0.942 | 0.087 | 0.934 | 0.069 | 0.936 | 0.068 | |||
2000 | 0.952 | 0.078 | 0.956 | 0.060 | 0.952 | 0.059 | |||
2500 | 0.946 | 0.071 | 0.932 | 0.053 | 0.944 | 0.053 | |||
5000 | 0.935 | 0.068 | 0.965 | 0.045 | 0.971 | 0.047 | |||
Case 3 | 500 | 0.940 | 0.185 | 0.936 | 0.153 | 0.953 | 0.156 | ||
1000 | 0.950 | 0.148 | 0.954 | 0.113 | 0.958 | 0.118 | |||
1500 | 0.932 | 0.127 | 0.950 | 0.094 | 0.958 | 0.099 | |||
2000 | 0.946 | 0.113 | 0.952 | 0.082 | 0.960 | 0.086 | |||
2500 | 0.942 | 0.103 | 0.932 | 0.073 | 0.950 | 0.077 | |||
5000 | 0.937 | 0.096 | 0.956 | 0.065 | 0.964 | 0.061 |
r | ||||||
Method | 300 | 500 | 800 | 1200 | 1600 | 2000 |
uniform | 0.2993 | 0.3337 | 0.4985 | 0.5632 | 0.8547 | 0.5083 |
mV | 3.5461 | 3.6485 | 3.8623 | 4.1256 | 4.4325 | 5.2365 |
mVc | 3.2852 | 3.3658 | 3.5463 | 3.8562 | 4.0235 | 4.4235 |
Full | 45.9075 |
r | ||||||
Method | 300 | 500 | 800 | 1200 | 1600 | 2000 |
uniform | 0.4213 | 0.4868 | 0.5327 | 0.5932 | 0.7147 | 0.8883 |
mV | 4.6723 | 4.8963 | 5.2369 | 5.6524 | 6.0128 | 6.3567 |
mVc | 4.3521 | 4.6329 | 4.9658 | 5.2156 | 5.7652 | 5.9635 |
Full | 51.2603 |
uniform | mV | mVc | |
\text{Intercept} | -1.6084(0.069) | -1.5998(0.055) | -1.3122(0.052) |
{\check{\beta}}_1^{\text{err}} | 1.2879(0.205) | 1.1880(0.103) | 1.2038(0.097) |
{\check{\beta}}_2^{\text{err}} | 0.0105(0.106) | 0.0104(0.059) | 0.0111(0.046) |
{\check{\beta}}_3^{\text{err}} | 1.0033(0.201) | 0.9217(0.067) | 0.9199(0.054) |
{\check{\beta}}_4^{\text{err}} | 0.2636(0.094) | 0.2698(0.054) | 0.2555(0.063) |
{\check{\beta}}_5^{\text{err}} | 0.9469(0.229) | 0.8741(0.083) | 0.8628(0.076) |
uniform | mV | mVc | |
\text{Intercept} | -8.7934(0.0678) | -8.8105(0.0562) | -8.8135(0.0543) |
{\check{\beta}}_1^{\text{err}} | -0.6123(0.341) | -0.6047(0.142) | -0.6035(0.105) |
{\check{\beta}}_2^{\text{err}} | 0.0712(0.125) | 0.0730(0.064) | 0.0798(0.088) |
{\check{\beta}}_3^{\text{err}} | -0.9321(0.245) | -0.9087(0.067) | -0.9123(0.057) |
{\check{\beta}}_4^{\text{err}} | 1.4618(0.198) | 1.4580(0.054) | 1.4603(0.075) |
{\check{\beta}}_5^{\text{err}} | -0.1435(0.531) | -0.1347(0.242) | -0.1408(0.225) |