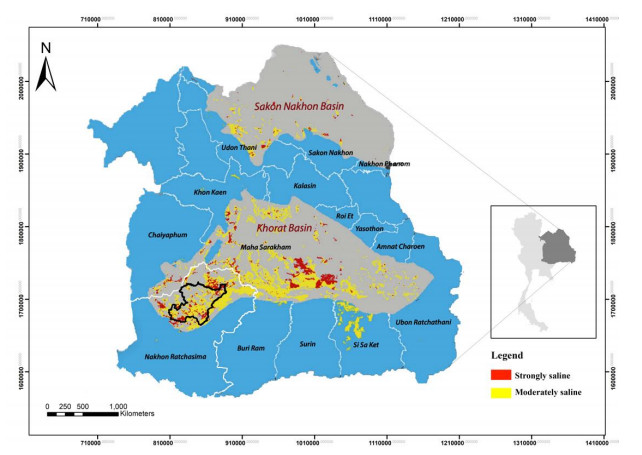
The research purpose is to assess and delineate groundwater potential zones (GWPZs) in the saline soil area in the districts of Non-Thai, Non-Sung, Non-Daeng, Khong, and Kham Sakae Saeng, Nakhon Ratchasima province, Thailand, using remote sensing (RS), geographical information system (GIS), and analytical hierarchy process (AHP) techniques. The GWPZs were created by combining multiple influencing factors such as slope, landforms, annual rainfall, soil texture class, drainage density, geology, hydrogeological unit, land use/land cover, groundwater potential, and normalized difference vegetation index of the study area. The AHP technique was used to determine the weights of various thematic layers to identify the groundwater potential zone. The weights of the thematic layers in descending order consisted of hydrogeological unit (17.61%), geology (17.10%), groundwater potential (12.09%), soil texture class (12.09%), drainage density (8.55%), landforms (8.46%), land use/land cover (6.05%), slope (6.01%), annual rainfall (6.01%), and normalized difference vegetation index (6.01%), respectively. The acceptable consistency ratio (CR) is used to evaluate the reliability of AHP techniques, and which coefficient of determination (R2) of 0.7131 was used to validate the salinity data of 17 groundwater wells. The overall weightage of the AHP technique assessment was classified into 5 categories of the GWPZs including very high potential, high potential, moderate potential, poor potential, and very poor potential. The mostly groundwater quality distribution represented a moderate potential of about 1,101 km2 (46.01%) to a poor potential of about 1,114 km2 (46.57%) from the 2,390 km2 of the study area located throughout the study area especially Kham Sakae Saeng, Non-Thai, and Non-Sung districts.
Citation: Watcharin Phoemphon, Bantita Terakulsatit. Assessment of groundwater potential zones and mapping using GIS/RS techniques and analytic hierarchy process: A case study on saline soil area, Nakhon Ratchasima, Thailand[J]. AIMS Geosciences, 2023, 9(1): 49-67. doi: 10.3934/geosci.2023004
[1] | Nudthawud Homtong, Wisaroot Pringproh, Kankanon Sakmongkoljit, Sattha Srikarom, Rungtiwa Yapun, Ben Wongsaijai . Remote sensing-based groundwater potential evaluation in a fractured-bedrock mountainous area. AIMS Geosciences, 2024, 10(2): 242-262. doi: 10.3934/geosci.2024014 |
[2] | Palavai Venkateswara Rao, Mangalampalli Subrahmanyam, Bakuru Anandagajapathi Raju . Groundwater exploration in hard rock terrains of East Godavari district, Andhra Pradesh, India using AHP and WIO analyses together with geoelectrical surveys. AIMS Geosciences, 2021, 7(2): 244-267. doi: 10.3934/geosci.2021015 |
[3] | Rosazlin Abdullah, Firuza Begham Mustafa, Subha Bhassu, Nur Aziaty Amirah Azhar, Benjamin Ezekiel Bwadi, Nur Syabeera Begum Nasir Ahmad, Aaronn Avit Ajeng . Evaluation of water and soil qualities for giant freshwater prawn farming site suitability by using the AHP and GIS approaches in Jelebu, Negeri Sembilan, Malaysia. AIMS Geosciences, 2021, 7(3): 507-528. doi: 10.3934/geosci.2021029 |
[4] | Maurizio Barbieri, Tiziano Boschetti, Giuseppe Sappa, Francesca Andrei . Hydrogeochemistry and groundwater quality assessment in a municipal solid waste landfill (central Italy). AIMS Geosciences, 2022, 8(3): 467-487. doi: 10.3934/geosci.2022026 |
[5] | Seyed Mohsen Mousavi, Ali Golkarian, Seyed Amir Naghibi, Bahareh Kalantar, Biswajeet Pradhan . GIS-based Groundwater Spring Potential Mapping Using Data Mining Boosted Regression Tree and Probabilistic Frequency Ratio Models in Iran. AIMS Geosciences, 2017, 3(1): 91-115. doi: 10.3934/geosci.2017.1.91 |
[6] | Andre Vervoort . Impact of the closure of a coal district on the environmental issue of long-term surface movements. AIMS Geosciences, 2022, 8(3): 326-345. doi: 10.3934/geosci.2022019 |
[7] | Christopher Talbot, Nico Augustin . Submarine Salt Karst Terrains. AIMS Geosciences, 2016, 2(2): 182-200. doi: 10.3934/geosci.2016.2.182 |
[8] | Diaka Sidibé, Mamadou Diallo, Ahmed Amara Konaté, Muhammad Zaheer . Geotechnical investigations of lead-silver ore processing residues at the Auzelles site, Auvergne (France). AIMS Geosciences, 2024, 10(4): 735-758. doi: 10.3934/geosci.2024036 |
[9] | Emil Drápela . Prevention of damage to sandstone rocks in protected areas of nature in northern Bohemia. AIMS Geosciences, 2021, 7(1): 56-73. doi: 10.3934/geosci.2021003 |
[10] | Natascha T. Torres, Thomas Steinsberger, Helen Droz-Georget, Beat Müller, Helmut Brandl, Peter C. Hauser, Gerhard Furrer . A Novel Method to Quantify Bioavailable Elements and Mobile ATP on Rock Surfaces and Lichens. AIMS Geosciences, 2016, 2(3): 245-258. doi: 10.3934/geosci.2016.3.245 |
The research purpose is to assess and delineate groundwater potential zones (GWPZs) in the saline soil area in the districts of Non-Thai, Non-Sung, Non-Daeng, Khong, and Kham Sakae Saeng, Nakhon Ratchasima province, Thailand, using remote sensing (RS), geographical information system (GIS), and analytical hierarchy process (AHP) techniques. The GWPZs were created by combining multiple influencing factors such as slope, landforms, annual rainfall, soil texture class, drainage density, geology, hydrogeological unit, land use/land cover, groundwater potential, and normalized difference vegetation index of the study area. The AHP technique was used to determine the weights of various thematic layers to identify the groundwater potential zone. The weights of the thematic layers in descending order consisted of hydrogeological unit (17.61%), geology (17.10%), groundwater potential (12.09%), soil texture class (12.09%), drainage density (8.55%), landforms (8.46%), land use/land cover (6.05%), slope (6.01%), annual rainfall (6.01%), and normalized difference vegetation index (6.01%), respectively. The acceptable consistency ratio (CR) is used to evaluate the reliability of AHP techniques, and which coefficient of determination (R2) of 0.7131 was used to validate the salinity data of 17 groundwater wells. The overall weightage of the AHP technique assessment was classified into 5 categories of the GWPZs including very high potential, high potential, moderate potential, poor potential, and very poor potential. The mostly groundwater quality distribution represented a moderate potential of about 1,101 km2 (46.01%) to a poor potential of about 1,114 km2 (46.57%) from the 2,390 km2 of the study area located throughout the study area especially Kham Sakae Saeng, Non-Thai, and Non-Sung districts.
The Khorat Plateau is located in northeastern Thailand and is split into two depositional basins by the Phu Phan Range. The Sakon Nakhon Basin is in the north and the Khorat Basin is in the south (Figure 1). The salinity of water and soil problems in this area are caused by human and natural activities. Human activities that contributed to soil salinization include wastewater from private and municipal septic systems, as well as certain usage of agricultural pesticides. The nature is mainly caused by the evaporitic environment of the Maha Sarakham Formation [1] as shown in Figure 2. Because water can be recharged and discharged through soil and rock [2]. These soil or rock-water interactions affected saline water and soil in the saline soil area, especially during the dry season, exposed on the ground in various locations [2]. The soil salinization problems are a major critical issue that is becoming more widespread in northeastern Thailand, especially in Nakhon Ratchasima province. The major causes of salinity in the study area are salts dissolving in groundwater and accumulating on the ground surface; sodium chloride; and shallow groundwater table depth. Electrical surveys and groundwater map in Nakhon Ratchasima Province by Wannakomol [3] represented those nine districts of Nakhon Ratchasima are affected by the shallow level of saline groundwater including Si Khio, Dan Khun Thot, Phra Thong Kham, Kham Sakae Saeng, Non-Sung, Phimai, Kham Thale So, Muang Nakhon Ratchasima, and Chaloem Phra Kiat districts. These areas are overlayed in the moderate to strongly saline soil of the Khorat Plateau (Figure 1).
The Khorat Plateau is defined by a huge expanse of Mesozoic era (Late Triassic to Cretaceous) continental sedimentary rocks of the Khorat Group [5] from bottom to top (Figure 2A), Nam Phong Formation consists of reddish-brown micaceous sandstone, conglomerate, siltstone, and mudstone. Phu Kradung Formation consists predominantly of maroon siltstone while sandstone and conglomerate are subordinate. Phra Wihan Formation consists of dominant white, coarse-grained, well-sorted sandstone and rare siltstone, mudstone, and conglomerate. Sao Khua Formation consists of an alternation of reddish-brown conglomeratic sandstone with lime-nodule clasts, siltstone, and mudstone. Phu Phan Formation consists mainly of light-buff to brown, medium- to coarse-grained, well-sorted sandstone. Khok Kruat Formation is the end of the Khorat Group accumulation consisting mainly of light grey to light brown, fine to medium-grained micaceous or arkose sandstone interbedded with siltstone and mudstone with subordinate conglomerate beds. After that, the inversion in the Mid-Cretaceous led to a restricted depositional basin containing red beds. Subsequently, the associated rock salt layers of the Maha Sarakham Formation were deposited under a hypersaline land-locked, lacustrine basin in arid conditions. The basin was subsequently covered by the Phu Tok Formation in the Late Cretaceous under an arid desert environment (Figure 2B).
Groundwater aquifers in Nakhon Ratchasima province consisted of unconsolidated and consolidated aquifers: unconsolidated aquifers can be found in alluvial and high terrace deposits, and consolidated aquifers can be found in the Maha Sarakham and Khok Kruat Formation [5] as shown in Figure 2A.
The Maha Sarakham Formation is divided into three distinct depositional members: the Lower Member, the Middle Member, and the Upper Member, which are mainly comprised of evaporites separated by red-colored siliciclastic (Figure 2B). Each of the evaporative members has beds made up of pseudomorphs, the replacement of gypsum by halite. While such beds are widespread in both basins, the replaced beds are the thickest. [1].
The traditional approach to groundwater investigation using geological, hydrogeological, and geophysical approaches is costly due to the excessive cost of drilling, time-consuming, and complicated for regional groundwater exploration fields [6]. Meanwhile, the intercorporate all filed data by using the modeling process constitutes the most effective tool for such analysis to solve modern hydrogeological problems such as SWAT-MODFLOW, GSFLOW, MIKE SHE, and HydroGeoSphere (HGS) are the most common models for surface water-groundwater simulation analysis [7].
Remote Sensing (RS) and Geographic Information Systems (GIS) were the one convenient and simple method to help locate the groundwater potential zones. Because remote sensors cannot directly detect groundwater, its presence is inferred from various surface properties gathered from satellite imagery, such as geology, landforms, soils, land use/land cover, surface water bodies, and others that operate as indicators of groundwater occurrence [8].
The RS and GIS have also proven to be the primary tools for creating thematic maps from satellite images. Various techniques, including weighted overlay analysis, multiple influencing factors, and analytical hierarchy process, can be used to assess groundwater potential zones. The analytical hierarchy process (AHP) technique is one of Multi-Criteria Decision Analysis (MCDA) [9] and is most widely used and popular for utilized to assign the relative relevance of each criterion for zoning groundwater potential recharge regions. MCDA technique was used for defining groundwater prospecting zones [10].
However, few studies have been undertaken in the saline soil area to delineate the potential zones of groundwater occurrence [11]. Therefore, this research aimed to examine and delineate groundwater potential zones by using RS/GIS and the AHP approach according to combining multiple influencing factors such as slope, landforms, annual rainfall, soil texture class, drainage density, geology, hydrogeological unit, land use/land cover, groundwater potential, and normalized difference vegetation index of the study area. This research will provide essential information to the population, government agencies, and the commercial sector to promote sustainable groundwater management and optimal locations for borehole drilling. Furthermore, the results of this study are critical for the proper administration, management, and long-term usage of groundwater resources in the saline soil area of Nakhon Ratchasima province, Thailand.
The saline soil area is in Nakhon Ratchasima province in northeastern Thailand, located between 818000 E to 888000 E and 1664000 N to 1722000N (UTM zone 47 N). The study area is approximately 2,390 km2 and covers the districts of Non-Thai, Non-Sung, Non-Daeng, Khong, and Kham Sakae Saeng, Nakhon Ratchasima province (Figure 3). This is one of many areas affected by the salinity of the Maha Sarakham Formation. The average temperature was 27.40 ℃, with a relative humidity of 71% and an annual rainfall of 1,028.10 ml. Between November and May, the weather is typically dry, with little rain forecast for much of the region. The dry season here is divided into two parts: the cool season (November to February), when it can get chilly at night, and the hot season (March to May) when temperatures can approach 40 ℃. The rainy season lasts from May to November. The southwest monsoon dominates the region, and rainfall is at its heaviest during this season. The rain normally falls in the form of brief showers that last an hour or two. Rainfall can become heavier and more consistent as the rainy season develops, with peak amounts typically occurring in August and September.
In this study, the field data of geological and geophysical investigation [3], geohydrology [4], and groundwater and soil analysis [12] were collected and used to intercorporate all data by using the RS/GIS and analytical hierarchy process (AHP) techniques for assess and delineate groundwater potential zones (GWPZs) in the study area.
Secondary data for groundwater potential mapping in the study area were obtained by examining the literature and available online materials. Ten factors were gathered from online sources. The slope was created from ASTER GDEM [13], the landforms were collected from the SRTM Landform dataset [14], the annual rainfall was collected from Royal Irrigation Department (RID), and the soil texture class was collected from the Soil Texture Classes (USDA system) according to Hengl [15], the drainage density was extracted from stream networks from ASTER GDEM [13], the geology was collected from the Department of Groundwater Resources (DGR), the hydrogeological unit was collected from the Department of Groundwater Resources (DGR), the land use/land cover was collected from the Sentinel-2 Land Use and Land Cover map created by Esri, Microsoft, and Impact Observatory, the groundwater potential was collected from Department of Groundwater Resources (DGR), and the normalized difference vegetation index was collected from Sentinel-2 images were all obtained from reliable sources (Table 1).
No. | Influencing factors | Data type | Scale/Resolution | Source |
1 | Slope | Raster | 30 m | ASTER GDEM |
2 | Landforms | Raster | 90 m | SRTM |
3 | Annual rainfall | XML | - | RID |
4 | Soil texture class | Raster | 10 m | Hengl's dataset |
5 | Drainage density | Vector | 30 m | ASTER GDEM |
6 | Geology | WMS | 1:50,000 | DGR |
7 | Hydrogeological unit | WMS | 1:50,000 | DGR |
8 | Land use /land cover | Raster | 10 m | ESRI |
9 | Groundwater potential | WMS | 1:50,000 | DGR |
10 | Normalized Difference vegetation index | Raster | 10 m | Generated from Sentinel-2 |
Note: ASTER: The Advanced Spaceborne Thermal Emission and Reflection Radiometer, GDEM: Global Digital Elevation Model, RID: Royal Irrigation Department, DGR: Department of Groundwater Resources. |
Thematic layers were projected to the same projections, and raster layers were transformed into vectors (polygons). The drainage density and slope were created in ArcGIS from GDEM. The Google Earth engine and the Python API were used to collect landforms, soil texture class, land use/land cover, and normalized difference vegetation index. Rainfall was collected from Royal Irrigation Department (RID). Geology, groundwater quality, and hydrogeological units were obtained from the Department of Groundwater Resources (DGR) and were digitized in ArcGIS.
Slope, landforms, rainfall, soil texture class, drainage density, geology, hydrogeological unit, land use/land cover, groundwater potential, and normalized difference vegetation index are essential elements in controlling groundwater quality in the study area. As Table 2 shown all thematic layers and their subclasses were classified and weighted based on their influence on groundwater potential. Each thematic layer and subclass were weighted based on the AHP technique as shown by expert judgment of relative intensity of importance (Table 3), and its impact on groundwater potential occurrence. As Table 4 shows the different values of n of Saaty's Random Consistency Index (RCI). A CR of 0.10 or less (for n = 5) is regarded acceptable, as is a CR of 0.09 or less (for n = 4), or 0.05 or less (for n = 3). Otherwise, each criterion's relative relevance will be reconsidered to increase judgmental consistency and avoid inconsistency. These thematic layers were overlaid in ArcGIS's analyst tool. The delineated map was classed into different zones ranging from very poor to very high groundwater quality. To identify the groundwater potentiality in the study area, the map was overlaid with the administrative district of Nakhon Ratchasima. The delineated groundwater potential zones were validated using borehole/well data collected in the field.
No. | Influencing factors | Feature | Groundwater prospect | Feature normalized weight |
1 | Slope | < 11% | 4 | 0.40 |
11–22% | 3 | 0.30 | ||
22–44% | 2 | 0.20 | ||
> 44% | 1 | 0.10 | ||
2 | Landforms | Upper slope (warm) | 2 | 1.11 |
Upper slope (flat) | 2 | 1.26 | ||
Lower slope (warm) | 3 | 1.63 | ||
Lower slope (flat) | 3 | 1.79 | ||
Valley | 4 | 2.16 | ||
Valley (narrow) | 5 | 2.21 | ||
3 | Annual rainfall | Low rainfall | 1 | 0.10 |
Medium rainfall | 2 | 0.20 | ||
High rainfall | 3 | 0.30 | ||
Very High rainfall | 4 | 0.40 | ||
4 | Soil texture class | Clay | 1 | 0.09 |
Clayey Loam | 2 | 0.36 | ||
Sandy Clay Loam | 2 | 0.55 | ||
Loam | 3 | 0.64 | ||
Sandy Loam | 3 | 0.82 | ||
5 | Drainage density | Very Fine | 1 | 0.17 |
Fine | 2 | 0.33 | ||
6 | Geology | Kk | 1 | 0.13 |
KTms | 3 | 0.38 | ||
Q1 | 2 | 0.25 | ||
Q2 | 2 | 0.25 | ||
7 | Hydrogeological unit | Qfd | 1 | 0.25 |
8 | Land use/land cover | Ms | 3 | 0.75 |
No Data | 1 | 0.06 | ||
Water | 5 | 0.28 | ||
Grass | 3 | 0.17 | ||
Flooded Vegetation | 4 | 0.22 | ||
Scrub/Shrub | 3 | 0.17 | ||
Built Area | 1 | 0.06 | ||
9 | Groundwater potential | G2 (Yield 2–10 and TDS 500–1,500 ppm) | 1 | 0.10 |
R2 (Yield 2–10 and TDS > 1,500 ppm) | 2 | 0.20 | ||
G1 (Yield < 2 and TDS 500–1,500 ppm) | 3 | 0.30 | ||
R1 (Yield < 2 and TDS > 1,500 ppm) | 4 | 0.40 | ||
10 | Normalized | Water | 5 | 0.33 |
difference | bare soil | 4 | 0.27 | |
vegetation index | sparse vegetation | 3 | 0.20 | |
moderate vegetation | 2 | 0.13 | ||
dense vegetation | 1 | 0.07 |
Intensity of importance | Definition | Explanation |
1 | Equal importance | Two elements contribute equally to the objective |
3 | Moderate importance | Experience and judgment slightly favor one element over another |
5 | Strong importance | Experience and judgment strongly favor one element over another |
7 | Very strong importance | One element is favored very strongly over another, its dominance is demonstrated in practice |
9 | Extreme importance | The evidence favoring one element over another is of the highest possible order of affirmation |
2, 4, 6, and 8 can be used to express intermediate values | ||
Note: Adapted from Goepel [32]. |
1 | 2 | 3 | 4 | 5 | 6 | 7 | 8 | 9 | 10 | |
0.00 | 0.00 | 0.58 | 0.89 | 1.12 | 1.24 | 1.32 | 1.41 | 1.45 | 1.49 | |
Note: Adapted from Saaty [31]. |
Groundwater occurrences are controlled by geology, structure, geomorphology, and drainage density, while recharge is influenced by land use, rainfall, and infiltration rate [16]. The number of thematic layers used varies based on the availability of data for each researcher. Slope, landforms, annual rainfall, soil texture class, drainage density, geology, hydrogeological unit, land use/land cover, groundwater potential, and normalized difference vegetation index (Table 2) were used in this study to investigate groundwater potential zone analysis. The relationships between these influencing factors are weighted based on past research. A factor with a higher weight has a greater impact, while a factor with a lower weight has a smaller impact on the potential interaction of groundwater. Weighted overlay analysis in ArcGIS is used to compute the integration of these factors with their potential weights.
The slope of a surface is the highest rate of change in height across a region of the surface, and it is the main topographical factor that influences land stability [17]. Because terrain slope is one of the main factors influencing groundwater infiltration into the subsurface, it is an appropriate groundwater indicator [18]. Surface runoff is gradual in moderate slope areas, providing more time for rainwater to percolate, whereas steep slope areas encourage strong runoff, allowing less residence time for rainwater and hence slightly less infiltration [19]. The ASTER Global Digital Elevation Model (GDEM) with a spatial resolution of 30 m was used to create a slope map of the area (Table 1).
The slope map was prepared and classified using ArcGIS spatial analyst tools. The weightage was assigned in the attribute table based on its impact on the potential occurrence of groundwater. The slopes are divided into four categories. Most of the study area has a pleasant slope of 5 degrees or less of 2,115.48 km2 (88.90%), 5–10 degrees of 253.61 km2 (10.66%), 10–20 degrees of 10.41 km2 (0.44%), and greater than 20 degrees of 0.24 km2 (0.01%) as shown in Table 1 and Figure 4A.
Landform investigations are one of the most important parts of assessing both surface and groundwater resources. Landform mapping helps identify and categorize various landforms and structural features that are supportive of groundwater occurrences [20]. An integrated study of landform development and geology can help identify the presence of permeable and porous zones [20]. A landform categorization that leads to the definition of hydro-morphological features is useful for assessing groundwater resources since it considers both morphological and lithological aspects. The study of landforms significantly influences the groundwater region, slope, relief, depth of weathering, type of deposited materials, the thickness of deposition, and the assemblage of various landforms. Landform data collected from The SRTM Landform dataset [14] was given total and individual weightage based on its influence on groundwater occurrence. The landform data was collected and processed using the Google Earth engine and the Python API. The ArcGIS attribute table was used to arrange the values.
In this study, the landform map was divided into six landform features: 11.54 km2 of the upper slope (warm) (0.48%), 888.30 km2 of the upper slope (flat) (37.17%), 4.45 km2 of the lower slope (warm) (0.19%), 1,084.20 km2 of the lower slope (flat) (45.37%), 385.52 km2 of the valley (16.13%), and 15.62 km2 of the valley (narrow) (0.65%) as shown in Table 2 and Figure 4B.
Rainfall is crucial in the hydrologic cycle because it influences groundwater potential. The prospect of groundwater recharge is high when rainfall is high and low when rainfall is low [21]. Annual rainfall is collected by the Royal Irrigation Department (RID). Spatial analyst tools on ArcGIS were utilized to interpolate and reclassify data.
The annual rainfall map was projected after being cropped to the study area's extent and was collected in millimeters and reclassified into four groups according to natural breaks (Jenks): 186–193 mm (Low rainfall) of 154.45 km2 (6.47%), 193–199 mm (Medium rainfall) of 722.25 km2 (30.23%), 199–204 mm (High rainfall) of 890.51 km2 (37.28%), and 204–206 mm (Very High rainfall) of 621.75 km2 (26.03%) as shown in Table 2 and Figure 4C.
Soil is a natural resource that is an influential factor in delineating groundwater potential zones. It also plays an important role in groundwater recharge [22]. Sandy loam has a high infiltration rate and good groundwater potential zones, whereas clay has a low infiltration rate but less groundwater potential. The Google Earth engine and the Python API were used to collect and process the soil texture class.
According to Hengl's [15] study, the soil map was classified into five classes: clay, clayey loam, sandy clay loam, loam, and sandy loam as shown in Table 2 and Figure 4D.
More than one-third of the area is covered by 809.42 km2 of sandy clay loam (SaClLo) (33.87%), 714.10 km2 of loam (Lo) (29.88%), 527.55 km2 of sandy loam (SaLo) (22.07%), 328.14 km2 of clayey loam (ClLo) (13.73%), and 10.66 km2 of clay (Cl) (0.45%).
Drainage density is described as the close spacing of stream networks and their relationship to surface runoff and permeability [23]. Because it is an inverse function of permeability, it is an important parameter in determining the groundwater potential zone. The drainage characteristics of the basin influence the subsurface hydrological condition of any region, which leads to the interpretation of groundwater conditions. Rain infiltration is reduced if the rock is less permeable [24]. The drainage density was determined in ArcGIS' spatial analyst tool under the hydrology tool. ArcMap was used to extract stream networks from the global digital elevation model (GDEM). Following line density preparation, the drainage density was calculated from the derived stream network.
The drainage density map was divided into three density classes based on their effect on groundwater occurrence: 0.00–0.75 km/km2 (Very fine) of 1,131.51 km2 (47.33%), 0.75–1.50 km/km2 (Fine) of 1,245.09 km2 (52.08%), and 1.50–1.86 km/km2 (Coarse) of 13.93 km2 (0.58%) as shown in Table 2 and Figure 4E.
According to Shaban and Khawlie [25], the type of rock exposed to the surface substantially impacts groundwater recharge. Geology influences groundwater recharge by influencing water flow percolation. Geology data was obtained from the Department of Mineral Resources (DMR) in Thailand using the WFS (Web Feature Service) on a 1:50,000 scale then extracted and digitized.
The geology map was divided into four geological units: Pleistocene River terrace deposits (Q1) of 190.24 km2 (7.96%), Quaternary Alluvial deposits (Q2) of 1,266.95 km2 (53.00%), Khok Kruat Formation (Kk) of 10.57 km2 (0.44%), and Maha Sarakham Formation (KTms) of 922.80 km2 (38.60%). Depending on the infiltration capacity and groundwater recharge potential, different scores were assigned to different rock units as shown in Table 2 and Figure 4F.
It is defined as any soil or rock unit or zone that has a particular influence on the storage or circulation of groundwater due to its hydraulic properties. It is regarded as the primary dataset in terms of groundwater potential. An aquifer is a formation or group of formations or part of a formation that contains sufficient saturated permeable material to provide large volumes of water to wells and springs [26]. The hydrogeological unit was derived from Thailand's Department of Mineral Resources (DMR) at a scale of 1:50,000 using the WFS then extracted and digitized.
The hydrogeological unit map was reclassified into two units based on groundwater quality, with different scores assigned to each unit: 93.11 km2 of Floodplain Deposits Aquifer (Qfd) (3.89%), 2,297.45 km2 of Maha Sarakham Aquifer (Ms) (96.11%) as shown in Table 2 and Figure 5A.
It impacts the runoff, infiltration, and groundwater recharge capacity of any watershed or subbasin [27], and it also gives soil information such as soil moisture content, groundwater, surface water, and an indicator of groundwater potential development [28]. Land use and land cover datasets were obtained from the Sentinel–2 Land Use and Land Cover map created by Esri, Microsoft, and Impact Observatory and processed using the google earth engine with the python API.
According to the LULC dataset, the land use/land cover map was classified into seven categories: 24.91 km2 of no data (1.04%), 11.29 km2 of water (0.47%), 1.26 km2 of grass (0.05%), 1,928.06 km2 of flooded vegetation (80.67%), 221.71 km2 of scrub/shrub (9.28%), 1.42 km2 of build area (0.06%), and 201.53 km2 of cloud (8.43%). Cropland received a high score, whereas barren land and settlements with low permeability received a lower score. Land use/land cover was clipped, projected, and weighted in ArcGIS' attribute table as shown in Table 2 and Figure 5B.
A groundwater potential map was created using specific yield and TDS data. Specific yield is an essential factor in land surface models for determining water table depth, which can strongly influence the relationship between soil moisture and groundwater [29]. According to WHO guidelines, total dissolved solids (TDS) of up to 500 ppm is the most desirable and up to 1,500 ppm is the maximum allowed. The high concentration of TDS in the groundwater sample is due to salt leaching from the soil, and residential sewage may percolate into the groundwater [30]. The groundwater potential was obtained using the WFS data from Thailand's Department of Mineral Resources (DMR) on a scale of 1:50,000 to extract and digitized.
The groundwater potential map is divided into four classes according to DMR datasets: 1936.65 km2 of G1 (Yield < 2 and TDS 500–1,500 ppm) (81.01%), 385.41 km2 of G2 (Yield 2–10 and TDS 500–1,500 ppm) (16.12%), 59.96 km2 of R1 (Yield < 2 and TDS > 1,500 ppm) (2.51%), and 8.54 km2 of R2 (Yield 2–10 and TDS > 1,500 ppm) (0.36%) as shown in Table 2 and Figure 5C.
Groundwater levels can be determined using NDVI, particularly in naturally vegetated environments such as forests or shrubland. The NDVI reflected water availability is a major factor in the growth of dense vegetation and vegetation cover. In areas where the water table is shallow, the vegetation is natural, and in situ groundwater observations are unavailable, NDVI may be utilized as a suitable indicator of groundwater storage conditions [31]. NDVI data was gathered from Sentinel–2 images and processed using the Google Earth Engine with the python API.
The NDVI map was reclassified into five classes, and different scores were assigned: 17.57 km2 of 0 or less (water) (0.74%), 3.23 km2 of 0.00–0.03 (bare soil) (0.14%), 2,106.69 km2 of 0.03-0.30 (sparse vegetation) (88.15%), 262.24 km2 of 0.30–0.50 (moderate vegetation) (10.97%), 0.05 km2 of more than 0.50 (dense vegetation) (0.002%) as shown in Table 2 and Figure 5D.
This AHP technique is the most widely used and popular Multi-Criteria Decision Analysis (MCDA) technique for determining groundwater prospecting zones. The AHP technique was developed by Saaty [32] to provide solutions to complex decision-making situations. The weights assigned to various thematic maps and their features were determined based on a review of the literature, previous research, and field experience, which were normalized using Saaty's AHP technique [33]. As shown in Table 5, the analytic hierarchy method evaluates each pair of variables in a specific cluster at a time, based on Saaty's scale of the relative intensity of importance. The final weight of each thematic layer was calculated using the output matrix's principal Eigenvalue (). The consistency of the judgment matrix should be assessed using the consistency index (CI), which is defined as
(1) |
Matrix | Sl | Geol | Lf | AR | STC | DD | HU | LULC | GP | NDVI | Weights |
Sl | 1.00 | 0.33 | 0.71 | 1.00 | 0.50 | 0.71 | 0.33 | 1.00 | 0.50 | 1.00 | 6.01% |
Geol | 2.83 | 1.00 | 2.00 | 2.83 | 1.40 | 2.00 | 1.00 | 2.83 | 1.40 | 2.83 | 17.10% |
Lf | 1.40 | 0.50 | 1.00 | 1.40 | 0.71 | 1.00 | 0.44 | 1.40 | 0.71 | 1.40 | 8.46% |
AR | 1.00 | 0.33 | 0.71 | 1.00 | 0.50 | 0.71 | 0.33 | 1.00 | 0.50 | 1.00 | 6.01% |
STC | 2.00 | 0.71 | 1.40 | 2.00 | 1.00 | 1.40 | 0.71 | 2.00 | 1.00 | 2.00 | 12.09% |
DD | 1.40 | 0.50 | 1.00 | 1.40 | 0.71 | 1.00 | 0.50 | 1.40 | 0.71 | 1.40 | 8.55% |
HU | 3.00 | 1.00 | 2.25 | 3.00 | 1.40 | 2.00 | 1.00 | 2.83 | 1.40 | 3.00 | 17.61% |
LULC | 1.00 | 0.33 | 0.71 | 1.00 | 0.50 | 0.71 | 0.33 | 1.00 | 0.50 | 1.00 | 6.05% |
GP | 2.00 | 0.71 | 1.40 | 2.00 | 1.00 | 1.40 | 0.71 | 2.00 | 1.00 | 2.00 | 12.09% |
NDVI | 1.00 | 0.33 | 0.71 | 1.00 | 0.50 | 0.71 | 0.33 | 1.00 | 0.50 | 1.00 | 6.01% |
Note: Sl: Slope; Geol: Geology; Lf: Landforms; AR: Annual rainfall; STC: Soil texture class; DD: Drainage density; HU: Hydrogeological unit; LULC: Land use/land cover; GP: Groundwater potential; NDVI: Normalized difference vegetation index. |
where is the consistency index, is the maximum of the judgment matrix's principal eigenvalues, which can be determined from the matrix, and n is the matrix's order. The ratio of CI to RCI for the same order matrix is known as the Consistency Ratio (CR) coefficient and is calculated using equation (2).
(2) |
where RCI is the random consistency index.
Table 4 shows the value of RCI acquired from Saaty's random consistency index (RCI) for different values of n. ACR of 0.10 or less (for n = 5) is regarded acceptable, as is a CR of 0.09 or less (for n = 4) or 0.05 or less (for n = 3). Otherwise, each criterion's relative relevance will be reconsidered to increase judgmental consistency and avoid inconsistency.
This GWPI is a dimensionless parameter used to estimate GWPZs in the study area. The weighted linear combination technique is used to calculate the GWPI as shown in equation (3).
(3) |
where is the normalized weight of the thematic layer, is the rank value of each feature class about the class, is the total number of thematic layers, and is the total number of features classes in the thematic layer.
In 2018, Thongwat [12] collected groundwater samples from a well-discharge in the saline soil area of Nakhon Ratchasima province and analyzed salinity content. In this study, the delineated groundwater potential zones were compared with the salinity content from well discharge for finding the coefficient of determination (R2).
The weightage was assigned to thematic layers and estimated values of the normalized weightage using AHP techniques (Table 5). The maximum principal eigenvalue () is 10.0012, the consistency index (CI) is 0.000137, the random consistency index (RCI) is 1.49, and the consistency ratio (CR) coefficient is 0.000092. This CR value result was acceptable due to its being less than 0.10 (for n = 5). In this study, the delineated groundwater potential zones were validated with the groundwater salinity data of well discharge data collected from Thongwat [12]. The coefficient of determination (R2) for 17 well discharges was found to be 0.7131 (Figure 6).
The slope, landforms, annual rainfall, soil texture class, drainage density, geology, hydrogeological unit, land use/land cover, groundwater potential, and normalized difference vegetation index of the study area were overlaid in ArcGIS software to evaluate the groundwater potential zones of the saline soil area. The groundwater potential zones map was created by overlaying 10 thematic layers and using the AHP technique to provide weightage ranking and reclassify the thematic layer in the ArcGIS attribute table. Each thematic layer was weighted according to its feature classes. The weights of the thematic layers result in descending order consisting of hydrogeological unit (17.61%), geology (17.10%), groundwater potential (12.09%), soil texture class (12.09%), drainage density (8.55%), landforms (8.46%), land use/land cover (6.05%), slope (6.01%), annual rainfall (6.01%), and normalized difference vegetation index (6.01%), respectively.
Thematic layers were created using remote sensing (RS) and geographical information system (GIS), and analytical hierarchy process (AHP) techniques were used to determine the weights of various thematic layers to identify the groundwater potential zone (GWPZs) by combining multiple influencing factors namely slope, landforms, annual rainfall, soil texture class, drainage density, geology, hydrogeological unit, land use/land cover, groundwater potential, and normalized difference vegetation index of the study area.
Groundwater potential zones (GWPZs) were reclassified into five categories based on their overall weightage: very high potential, high potential, moderate potential, poor potential, and very poor potential (Figure 7).
(1) The very high potential covered 99.71 km2 (4.16%) of this study area located in the southern part of the Non-Sung district. (2) The high potential covered 40.17 km2 (1.68%) located in the northwestern part of the Non-Thai district and the northeastern part of the Non-Daeng district. (3) The moderate potential covered 1,101.63 km2 (46.01%), which is mostly located in the northern part of Kham Sakae Saeng district, slightly in the northwestern part of the Khong district, and distributed throughout the study area. (4) The poor potential covered 1,114.83 km2 (46.57%) located in the central parts and eastern parts of Non-Thai districts; the northern part of the Non-Sung district; the southern part and eastern parts of Kham Sakae Saeng district; and the central part of Khong district. (5) The very poor potential covered 37.74 km2 (1.58%) located in the northeastern part of the Non-Thai district and the central parts of the Khong district.
The GWPZs were validated using salinity content, which may influence groundwater potential. The GWPZs were compared to salinity content data obtained by Thongwat [12] in 2018. As a result, the research found an inverse relation between salinity content and groundwater potential zones in Nakhon Ratchasima's saline soil area. The zone with high groundwater potential has low salinity, while the zone with low groundwater potential has high salinity.
In future studies, the model's performance could be evaluated using different statistical models. For example, the regression model gives a function that defines the relationship between one or more independent variables and dependent variables, which could improve prediction accuracy. Statistical model combiner provides the ability to take into consideration various criteria such as performance, stability, and parsimony [34].
The research revealed that the hydrogeological unit, geology, soil texture class, hydrological unit, and groundwater potential significantly impacted groundwater potential compared to other thematic layers. Moreover, the groundwater potential distribution represented a moderate potential to a poor potential located throughout the study area especially Kham Sakae Saeng, Non-Thai, and Non-Sung districts. Because of the saline related to the Maha Sarakham Formation (KTms), Maha Sarakham Aquifer (Ms), loam (Lo) and sandy loam (SaLo) of the soil texture class, and R1 (yield < 2 and TDS > 1,500 ppm) of the groundwater potential class. The validated GWPZs map with the observed well discharge data (salinity content). The study is certain, with a high coefficient value of 0.7131.
The results of this research can be used as necessary information for the population, government agencies, and the commercial sector to encourage sustainable groundwater management and optimal locations for field monitoring and groundwater borehole drilling. Furthermore, the results of this study are critical for the effective administration, management, and long-term usage of groundwater resources in the saline soil area of Nakhon Ratchasima province.
This work is supported by the Suranaree University of Technology for permission to publish this paper is gratefully acknowledged. The author is also thankful to Dr. Siripon Kamontum for her advice and assistance in GIS unit. My thanks are also extended to Ms. Wanida Thongwat and Mr. Thanet Phuphulb for their discharge well data.
All authors declare no conflicts of interest.
[1] |
El Tabakh M, Utha-Aroon C, Schreiber BC (1999) Sedimentology of the Cretaceous Maha Sarakham evaporites in the Khorat Plateau of northeastern Thailand. Sediment Geol 123: 31–62. https://doi.org/10.1016/S0037-0738(98)00083-9 doi: 10.1016/S0037-0738(98)00083-9
![]() |
[2] |
Davis S, Whittemore D, Fabryka-Martin J (1998) Uses of Chloride/Bromide Ratios in Studies of Potable Water. Ground Water 36: 338–350. https://doi.org/10.1111/j.1745-6584.1998.tb01099.x doi: 10.1111/j.1745-6584.1998.tb01099.x
![]() |
[3] | Wannakomol A (2012) Mapping of groundwater using an electrical survey in the vicinity of Nakhon Ratchasima province. Suranaree University of Technology Intellectual Repository, Nakhon Ratchasima, Thailand. Available from: http://sutir.sut.ac.th:8080/jspui/handle/123456789/4001 |
[4] | DMR (2015) Saline Soil Area Development in the Northeast: Geological approaches. Division of Mineral Resources Conservation and Management. Available from: https://www.dmr.go.th/wp-content/uploads/2022/10/article_20161011102857-1.pdf |
[5] | Tatong T (2004) Hydrogeology of the Khorat Greater City Area and Investigation of the Causes and Effects of Groundwater and Soil Salinization. Bangkok: Department of Mineral Resources. Available from: http://library.dmr.go.th/elib/cgi-bin/opacexe.exe?op=mmvw&db=Main&sid=undefined&skin=u&usid=undefined&mmid=628&bid=11978 |
[6] | Ndatuwong L, Yadav G (2014) Morphometric Analysis to Infer the Hydrogeological Behaviour in Part of Sonebhadra District, Uttar Pradesh, India, Using Remote Sensing and GIS Technique. Int J Remote Sens GIS 3: 1–7. |
[7] |
Ntona MM, Busico G, Mastrocicco M, et al. (2022) Modeling groundwater and surface water interaction: An overview of current status and future challenges. Sci Total Environ 846: 157355. https://doi.org/10.1016/j.scitotenv.2022.157355 doi: 10.1016/j.scitotenv.2022.157355
![]() |
[8] | Jha MK, Peiffer S (2006) Applications of remote sensing and GIS technologies in groundwater hydrology: past, present, and future, BayCEER Bayreuth. |
[9] |
Vittala SS, Govindaiah S, Gowda HH (2005) Evaluation of groundwater potential zones in the sub-watersheds of north pennar river basin around Pavagada, Karnataka, India using remote sensing and GIS techniques. J Indian Soc Remote Sens 33: 483. https://doi.org/10.1007/BF02990733 doi: 10.1007/BF02990733
![]() |
[10] |
Duguma TA, Duguma GA (2022) Assessment of Groundwater Potential Zones of Upper Blue Nile River Basin Using Multi-Influencing Factors under GIS and RS Environment: A Case Study on Guder Watersheds, Abay Basin, Oromia Region, Ethiopia. Geofluids 2022: 26. https://doi.org/10.1155/2022/1172039 doi: 10.1155/2022/1172039
![]() |
[11] |
Sinanuwong S, Takaya Y (1974) Distribution of Saline Soils in the Khorat Basin of Thailand. Southeast Asian Stud 12: 365–382. https://doi.org/10.20495/tak.12.3_365 doi: 10.20495/tak.12.3_365
![]() |
[12] | Thongwat W (2018) Relationship between soil salinity and chloride content in groundwater in saline soil areas of Nakhon Ratchasima province, Suranaree University of Technology Intellectual Repository. |
[13] | NASA, METI, AIST, et al. (2019) ASTER Global Digital Elevation Model V003 [Data set]. NASA EOSDIS Land Processes DAAC. Available from: https://search.earthdata.nasa.gov/search?q=%20C1711961296-LPCLOUD |
[14] |
Theobald DM, Harrison-Atlas D, Monahan WB, et al. (2015) Ecologically-Relevant Maps of Landforms and Physiographic Diversity for Climate Adaptation Planning. PLOS ONE 10: e0143619. https://doi.org/10.1371/journal.pone.0143619 doi: 10.1371/journal.pone.0143619
![]() |
[15] | Hengl T (2018) Soil texture classes (USDA system) for 6 soil depths (0, 10, 30, 60,100 and 200 cm) at 250 m. OpenAIRE. |
[16] |
Selvam S, Magesh NS, Chidambaram S, et al. (2015) A GIS-based identification of groundwater recharge potential zones using RS and IF technique: a case study in Ottapidaram taluk, Tuticorin district, Tamil Nadu. Environ Earth Sci 73: 3785–3799. https://doi.org/10.1007/s12665-014-3664-0 doi: 10.1007/s12665-014-3664-0
![]() |
[17] | Manjare BS (2014) Identification of groundwater prospecting zones using Remote Sensing and GIS techniques in upper Vena river watersheds Nagpur district, Maharashtra, India. 15th Esri India User Conference, 1–14. |
[18] | Sikakwe G, Ntekim E, Obi D, et al. (2015) Geohydrological study of weathered basement aquifers in Oban Massif and environs Southeastern Nigeria: using Remote Sensing and Geographic Information System Techniques. Geophysics 3: 321–990. |
[19] | Malik S, Rajeshwari D (2011) Delineation of groundwater potential zones in Mewat District. Int J Geomatics Geosci 2: 278–281. |
[20] | Karanth KR, Seshu babu K (1987) Identification of major lineaments on satellite imagery and aerial photographs for the delineation for possible potential groundwater zones in Penukonda and Dharmavaram taluks of Anantapur district. Proceedings of the Joint Indo-US Workshop on Remote Sensing of Water Resources, National Remote Sensing Agency (NRSA), Hyderabad, India, 188–197 |
[21] |
Kumar T, Gautam AK, Kumar T (2014) Appraising the accuracy of GIS-based Multi-criteria decision making technique for delineation of Groundwater potential zones. Water Resour Manage 28: 4449–4466. https://doi.org/10.1007/s11269-014-0663-6 doi: 10.1007/s11269-014-0663-6
![]() |
[22] | Radhakrishnan D, Ramamoorthy P (2014) Delineation of groundwater recharge potential zones in Mailam Block, Villupuram district, Using GIS. Int J Water Res 2: 71–75. |
[23] |
Magesh NS, Chandrasekar N, Soundranayagam JP (2012) Delineation of groundwater potential zones in Theni district, Tamil Nadu, using remote sensing, GIS and MIF techniques. Geosci Front 3: 189–196. https://doi.org/10.1016/j.gsf.2011.10.007 doi: 10.1016/j.gsf.2011.10.007
![]() |
[24] | Hutti B, Nijagunappa R (2011) Identification of Groundwater Potential Zone using Geoinformatics in Ghataprabha basin, North Karnataka, India. Int J Geomatics Geosci 2: 91–109. |
[25] |
Shaban A, Khawlie M, Abdallah C (2006) Use of remote sensing and GIS to determine recharge potential zones: the case of Occidental Lebanon. Hydrogeol J 14: 433–443. https://doi.org/10.1007/s10040-005-0437-6 doi: 10.1007/s10040-005-0437-6
![]() |
[26] |
Lohman AHM, Mentink GM (1972) Some cortical connections of the tegu lizard (Tupinambis teguixin). Brain Res 45: 325–344. https://doi.org/10.1016/0006-8993(72)90466-0 doi: 10.1016/0006-8993(72)90466-0
![]() |
[27] |
Guru B, Seshan K, Bera S (2017) Frequency ratio model for groundwater potential mapping and its sustainable management in cold desert, India. J King Saud Univ Sci 29: 333–347. https://doi.org/10.1016/j.jksus.2016.08.003 doi: 10.1016/j.jksus.2016.08.003
![]() |
[28] |
Ahmad I, Dar MA, Teka AH, et al. (2020) GIS and fuzzy logic techniques-based demarcation of groundwater potential zones: A case study from Jemma River basin, Ethiopia. J Afr Earth Sci 169: 103860. https://doi.org/10.1016/j.jafrearsci.2020.103860 doi: 10.1016/j.jafrearsci.2020.103860
![]() |
[29] |
Lu M, Xu Z, Yang ZL, et al. (2021) A Comprehensive Review of Specific Yield in Land Surface and Groundwater Studies. J Adv Model Earth Syst 13: e2020MS002270. https://doi.org/10.1029/2020MS002270 doi: 10.1029/2020MS002270
![]() |
[30] |
Sarath Prasanth SV, Magesh NS, Jitheshlal KV, et al. (2012) Evaluation of groundwater quality and its suitability for drinking and agricultural use in the coastal stretch of Alappuzha District, Kerala, India. Appl Water Sci 2: 165–175. https://doi.org/10.1007/s13201-012-0042-5 doi: 10.1007/s13201-012-0042-5
![]() |
[31] |
Bhanja SN, Malakar P, Mukherjee A, et al. (2019) Using Satellite-Based Vegetation Cover as Indicator of Groundwater Storage in Natural Vegetation Areas. Geophys Res Lett 46: 8082–8092. https://doi.org/10.1029/2019GL083015 doi: 10.1029/2019GL083015
![]() |
[32] |
Saaty RW (1987) The analytic hierarchy process—what it is and how it is used. Math Modell 9: 161–176. https://doi.org/10.1016/0270-0255(87)90473-8 doi: 10.1016/0270-0255(87)90473-8
![]() |
[33] | Goepel K (2013) Implementing the Analytic Hierarchy Process as a Standard Method for Multi-Criteria Decision Making in Corporate Enterprises—a New AHP Excel Template with Multiple Inputs. Proceedings of the international symposium on the analytic hierarchy process, Malaysia. 1–10. |
[34] |
Razzagh S, Sadeghfam S, Nadiri AA, et al. (2022) Formulation of Shannon entropy model averaging for groundwater level prediction using artificial intelligence models. Int J Environ Sci Technol 19: 6203–6220. https://doi.org/10.1007/s13762-021-03793-2 doi: 10.1007/s13762-021-03793-2
![]() |
1. | Tadie Mulie Asrade, Groundwater potential mapping and its sustainable management using AHP and FR models in the Jedeb watershed, Upper Blue Nile Basin, Ethiopia, 2024, 24, 1606-9749, 3617, 10.2166/ws.2024.226 |
No. | Influencing factors | Data type | Scale/Resolution | Source |
1 | Slope | Raster | 30 m | ASTER GDEM |
2 | Landforms | Raster | 90 m | SRTM |
3 | Annual rainfall | XML | - | RID |
4 | Soil texture class | Raster | 10 m | Hengl's dataset |
5 | Drainage density | Vector | 30 m | ASTER GDEM |
6 | Geology | WMS | 1:50,000 | DGR |
7 | Hydrogeological unit | WMS | 1:50,000 | DGR |
8 | Land use /land cover | Raster | 10 m | ESRI |
9 | Groundwater potential | WMS | 1:50,000 | DGR |
10 | Normalized Difference vegetation index | Raster | 10 m | Generated from Sentinel-2 |
Note: ASTER: The Advanced Spaceborne Thermal Emission and Reflection Radiometer, GDEM: Global Digital Elevation Model, RID: Royal Irrigation Department, DGR: Department of Groundwater Resources. |
No. | Influencing factors | Feature | Groundwater prospect | Feature normalized weight |
1 | Slope | < 11% | 4 | 0.40 |
11–22% | 3 | 0.30 | ||
22–44% | 2 | 0.20 | ||
> 44% | 1 | 0.10 | ||
2 | Landforms | Upper slope (warm) | 2 | 1.11 |
Upper slope (flat) | 2 | 1.26 | ||
Lower slope (warm) | 3 | 1.63 | ||
Lower slope (flat) | 3 | 1.79 | ||
Valley | 4 | 2.16 | ||
Valley (narrow) | 5 | 2.21 | ||
3 | Annual rainfall | Low rainfall | 1 | 0.10 |
Medium rainfall | 2 | 0.20 | ||
High rainfall | 3 | 0.30 | ||
Very High rainfall | 4 | 0.40 | ||
4 | Soil texture class | Clay | 1 | 0.09 |
Clayey Loam | 2 | 0.36 | ||
Sandy Clay Loam | 2 | 0.55 | ||
Loam | 3 | 0.64 | ||
Sandy Loam | 3 | 0.82 | ||
5 | Drainage density | Very Fine | 1 | 0.17 |
Fine | 2 | 0.33 | ||
6 | Geology | Kk | 1 | 0.13 |
KTms | 3 | 0.38 | ||
Q1 | 2 | 0.25 | ||
Q2 | 2 | 0.25 | ||
7 | Hydrogeological unit | Qfd | 1 | 0.25 |
8 | Land use/land cover | Ms | 3 | 0.75 |
No Data | 1 | 0.06 | ||
Water | 5 | 0.28 | ||
Grass | 3 | 0.17 | ||
Flooded Vegetation | 4 | 0.22 | ||
Scrub/Shrub | 3 | 0.17 | ||
Built Area | 1 | 0.06 | ||
9 | Groundwater potential | G2 (Yield 2–10 and TDS 500–1,500 ppm) | 1 | 0.10 |
R2 (Yield 2–10 and TDS > 1,500 ppm) | 2 | 0.20 | ||
G1 (Yield < 2 and TDS 500–1,500 ppm) | 3 | 0.30 | ||
R1 (Yield < 2 and TDS > 1,500 ppm) | 4 | 0.40 | ||
10 | Normalized | Water | 5 | 0.33 |
difference | bare soil | 4 | 0.27 | |
vegetation index | sparse vegetation | 3 | 0.20 | |
moderate vegetation | 2 | 0.13 | ||
dense vegetation | 1 | 0.07 |
Intensity of importance | Definition | Explanation |
1 | Equal importance | Two elements contribute equally to the objective |
3 | Moderate importance | Experience and judgment slightly favor one element over another |
5 | Strong importance | Experience and judgment strongly favor one element over another |
7 | Very strong importance | One element is favored very strongly over another, its dominance is demonstrated in practice |
9 | Extreme importance | The evidence favoring one element over another is of the highest possible order of affirmation |
2, 4, 6, and 8 can be used to express intermediate values | ||
Note: Adapted from Goepel [32]. |
1 | 2 | 3 | 4 | 5 | 6 | 7 | 8 | 9 | 10 | |
0.00 | 0.00 | 0.58 | 0.89 | 1.12 | 1.24 | 1.32 | 1.41 | 1.45 | 1.49 | |
Note: Adapted from Saaty [31]. |
Matrix | Sl | Geol | Lf | AR | STC | DD | HU | LULC | GP | NDVI | Weights |
Sl | 1.00 | 0.33 | 0.71 | 1.00 | 0.50 | 0.71 | 0.33 | 1.00 | 0.50 | 1.00 | 6.01% |
Geol | 2.83 | 1.00 | 2.00 | 2.83 | 1.40 | 2.00 | 1.00 | 2.83 | 1.40 | 2.83 | 17.10% |
Lf | 1.40 | 0.50 | 1.00 | 1.40 | 0.71 | 1.00 | 0.44 | 1.40 | 0.71 | 1.40 | 8.46% |
AR | 1.00 | 0.33 | 0.71 | 1.00 | 0.50 | 0.71 | 0.33 | 1.00 | 0.50 | 1.00 | 6.01% |
STC | 2.00 | 0.71 | 1.40 | 2.00 | 1.00 | 1.40 | 0.71 | 2.00 | 1.00 | 2.00 | 12.09% |
DD | 1.40 | 0.50 | 1.00 | 1.40 | 0.71 | 1.00 | 0.50 | 1.40 | 0.71 | 1.40 | 8.55% |
HU | 3.00 | 1.00 | 2.25 | 3.00 | 1.40 | 2.00 | 1.00 | 2.83 | 1.40 | 3.00 | 17.61% |
LULC | 1.00 | 0.33 | 0.71 | 1.00 | 0.50 | 0.71 | 0.33 | 1.00 | 0.50 | 1.00 | 6.05% |
GP | 2.00 | 0.71 | 1.40 | 2.00 | 1.00 | 1.40 | 0.71 | 2.00 | 1.00 | 2.00 | 12.09% |
NDVI | 1.00 | 0.33 | 0.71 | 1.00 | 0.50 | 0.71 | 0.33 | 1.00 | 0.50 | 1.00 | 6.01% |
Note: Sl: Slope; Geol: Geology; Lf: Landforms; AR: Annual rainfall; STC: Soil texture class; DD: Drainage density; HU: Hydrogeological unit; LULC: Land use/land cover; GP: Groundwater potential; NDVI: Normalized difference vegetation index. |
No. | Influencing factors | Data type | Scale/Resolution | Source |
1 | Slope | Raster | 30 m | ASTER GDEM |
2 | Landforms | Raster | 90 m | SRTM |
3 | Annual rainfall | XML | - | RID |
4 | Soil texture class | Raster | 10 m | Hengl's dataset |
5 | Drainage density | Vector | 30 m | ASTER GDEM |
6 | Geology | WMS | 1:50,000 | DGR |
7 | Hydrogeological unit | WMS | 1:50,000 | DGR |
8 | Land use /land cover | Raster | 10 m | ESRI |
9 | Groundwater potential | WMS | 1:50,000 | DGR |
10 | Normalized Difference vegetation index | Raster | 10 m | Generated from Sentinel-2 |
Note: ASTER: The Advanced Spaceborne Thermal Emission and Reflection Radiometer, GDEM: Global Digital Elevation Model, RID: Royal Irrigation Department, DGR: Department of Groundwater Resources. |
No. | Influencing factors | Feature | Groundwater prospect | Feature normalized weight |
1 | Slope | < 11% | 4 | 0.40 |
11–22% | 3 | 0.30 | ||
22–44% | 2 | 0.20 | ||
> 44% | 1 | 0.10 | ||
2 | Landforms | Upper slope (warm) | 2 | 1.11 |
Upper slope (flat) | 2 | 1.26 | ||
Lower slope (warm) | 3 | 1.63 | ||
Lower slope (flat) | 3 | 1.79 | ||
Valley | 4 | 2.16 | ||
Valley (narrow) | 5 | 2.21 | ||
3 | Annual rainfall | Low rainfall | 1 | 0.10 |
Medium rainfall | 2 | 0.20 | ||
High rainfall | 3 | 0.30 | ||
Very High rainfall | 4 | 0.40 | ||
4 | Soil texture class | Clay | 1 | 0.09 |
Clayey Loam | 2 | 0.36 | ||
Sandy Clay Loam | 2 | 0.55 | ||
Loam | 3 | 0.64 | ||
Sandy Loam | 3 | 0.82 | ||
5 | Drainage density | Very Fine | 1 | 0.17 |
Fine | 2 | 0.33 | ||
6 | Geology | Kk | 1 | 0.13 |
KTms | 3 | 0.38 | ||
Q1 | 2 | 0.25 | ||
Q2 | 2 | 0.25 | ||
7 | Hydrogeological unit | Qfd | 1 | 0.25 |
8 | Land use/land cover | Ms | 3 | 0.75 |
No Data | 1 | 0.06 | ||
Water | 5 | 0.28 | ||
Grass | 3 | 0.17 | ||
Flooded Vegetation | 4 | 0.22 | ||
Scrub/Shrub | 3 | 0.17 | ||
Built Area | 1 | 0.06 | ||
9 | Groundwater potential | G2 (Yield 2–10 and TDS 500–1,500 ppm) | 1 | 0.10 |
R2 (Yield 2–10 and TDS > 1,500 ppm) | 2 | 0.20 | ||
G1 (Yield < 2 and TDS 500–1,500 ppm) | 3 | 0.30 | ||
R1 (Yield < 2 and TDS > 1,500 ppm) | 4 | 0.40 | ||
10 | Normalized | Water | 5 | 0.33 |
difference | bare soil | 4 | 0.27 | |
vegetation index | sparse vegetation | 3 | 0.20 | |
moderate vegetation | 2 | 0.13 | ||
dense vegetation | 1 | 0.07 |
Intensity of importance | Definition | Explanation |
1 | Equal importance | Two elements contribute equally to the objective |
3 | Moderate importance | Experience and judgment slightly favor one element over another |
5 | Strong importance | Experience and judgment strongly favor one element over another |
7 | Very strong importance | One element is favored very strongly over another, its dominance is demonstrated in practice |
9 | Extreme importance | The evidence favoring one element over another is of the highest possible order of affirmation |
2, 4, 6, and 8 can be used to express intermediate values | ||
Note: Adapted from Goepel [32]. |
1 | 2 | 3 | 4 | 5 | 6 | 7 | 8 | 9 | 10 | |
0.00 | 0.00 | 0.58 | 0.89 | 1.12 | 1.24 | 1.32 | 1.41 | 1.45 | 1.49 | |
Note: Adapted from Saaty [31]. |
Matrix | Sl | Geol | Lf | AR | STC | DD | HU | LULC | GP | NDVI | Weights |
Sl | 1.00 | 0.33 | 0.71 | 1.00 | 0.50 | 0.71 | 0.33 | 1.00 | 0.50 | 1.00 | 6.01% |
Geol | 2.83 | 1.00 | 2.00 | 2.83 | 1.40 | 2.00 | 1.00 | 2.83 | 1.40 | 2.83 | 17.10% |
Lf | 1.40 | 0.50 | 1.00 | 1.40 | 0.71 | 1.00 | 0.44 | 1.40 | 0.71 | 1.40 | 8.46% |
AR | 1.00 | 0.33 | 0.71 | 1.00 | 0.50 | 0.71 | 0.33 | 1.00 | 0.50 | 1.00 | 6.01% |
STC | 2.00 | 0.71 | 1.40 | 2.00 | 1.00 | 1.40 | 0.71 | 2.00 | 1.00 | 2.00 | 12.09% |
DD | 1.40 | 0.50 | 1.00 | 1.40 | 0.71 | 1.00 | 0.50 | 1.40 | 0.71 | 1.40 | 8.55% |
HU | 3.00 | 1.00 | 2.25 | 3.00 | 1.40 | 2.00 | 1.00 | 2.83 | 1.40 | 3.00 | 17.61% |
LULC | 1.00 | 0.33 | 0.71 | 1.00 | 0.50 | 0.71 | 0.33 | 1.00 | 0.50 | 1.00 | 6.05% |
GP | 2.00 | 0.71 | 1.40 | 2.00 | 1.00 | 1.40 | 0.71 | 2.00 | 1.00 | 2.00 | 12.09% |
NDVI | 1.00 | 0.33 | 0.71 | 1.00 | 0.50 | 0.71 | 0.33 | 1.00 | 0.50 | 1.00 | 6.01% |
Note: Sl: Slope; Geol: Geology; Lf: Landforms; AR: Annual rainfall; STC: Soil texture class; DD: Drainage density; HU: Hydrogeological unit; LULC: Land use/land cover; GP: Groundwater potential; NDVI: Normalized difference vegetation index. |