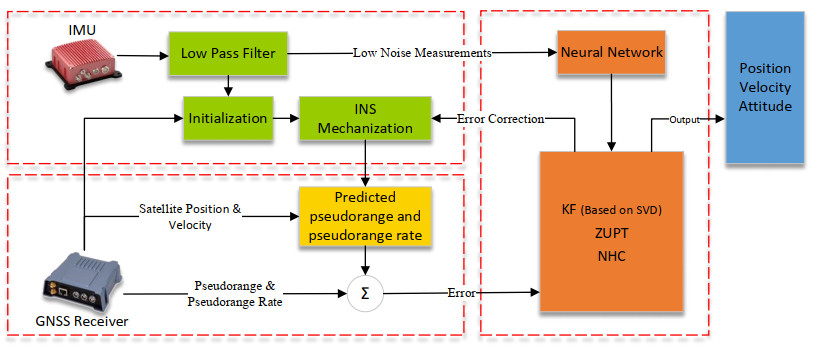
Our recent mapping of the Dras fault zone in the NW Himalaya has answered one of the most anticipated searches in recent times where strike-slip faulting was expected from the geodetic studies. Therefore, the discovery of the fault is a leap towards the understanding of the causes of active faulting in the region, and how the plate tectonic convergence between India and Eurasia is compensated in the interior portions of the Himalayan collision zone, and what does that imply about the overall convergence budget and the associated earthquake hazards. The present work is an extended version of our previous studies on the mapping of the Dras fault zone, and we show details that were either not available or briefly touched. We have used the 30 m shuttle radar topography to map the tectonic geomorphological features that includes the fault scarps, deflected drainage, triangular facets, ridge crests, faulted Quaternary landforms and so on. The results show that oblique strike-slip faulting is active in the suture zone, which suggests that the active crustal deformation is actively compensated in the interior portions of the orogen, and it is not just restricted to the frontal portions. The Dras fault is a major fault that we have interpreted either as a south dipping oblique backthrust or an oblique north dipping normal fault. The fieldwork was conducted in Leh, but it did not reveal any evidence for active faulting, and the fieldwork in the Dras region was not possible because of the politically sensitive nature of border regions where fieldwork is always an uphill task.
Citation: AA Shah, A Rajasekharan, N Batmanathan, Zainul Farhan, Qibah Reduan, JN Malik. Detailed tectonic geomorphology of the Dras fault zone, NW Himalaya[J]. AIMS Geosciences, 2021, 7(3): 390-414. doi: 10.3934/geosci.2021023
[1] | Wenshun Sheng, Jiahui Shen, Qiming Huang, Zhixuan Liu, Zihao Ding . Multi-objective pedestrian tracking method based on YOLOv8 and improved DeepSORT. Mathematical Biosciences and Engineering, 2024, 21(2): 1791-1805. doi: 10.3934/mbe.2024077 |
[2] | H. Thomas Banks, Shuhua Hu, Zackary R. Kenz, Hien T. Tran . A comparison of nonlinear filtering approaches in the context of an HIV model. Mathematical Biosciences and Engineering, 2010, 7(2): 213-236. doi: 10.3934/mbe.2010.7.213 |
[3] | Gerasimos G. Rigatos, Efthymia G. Rigatou, Jean Daniel Djida . Change detection in the dynamics of an intracellular protein synthesis model using nonlinear Kalman filtering. Mathematical Biosciences and Engineering, 2015, 12(5): 1017-1035. doi: 10.3934/mbe.2015.12.1017 |
[4] | Xin Liu, Yingyuan Xiao, Xu Jiao, Wenguang Zheng, Zihao Ling . A novel Kalman Filter based shilling attack detection algorithm. Mathematical Biosciences and Engineering, 2020, 17(2): 1558-1577. doi: 10.3934/mbe.2020081 |
[5] | Weijie Wang, Shaoping Wang, Yixuan Geng, Yajing Qiao, Teresa Wu . An OGI model for personalized estimation of glucose and insulin concentration in plasma. Mathematical Biosciences and Engineering, 2021, 18(6): 8499-8523. doi: 10.3934/mbe.2021420 |
[6] | Junyoung Jang, Kihoon Jang, Hee-Dae Kwon, Jeehyun Lee . Feedback control of an HBV model based on ensemble kalman filter and differential evolution. Mathematical Biosciences and Engineering, 2018, 15(3): 667-691. doi: 10.3934/mbe.2018030 |
[7] | Yukun Tan, Durward Cator III, Martial Ndeffo-Mbah, Ulisses Braga-Neto . A stochastic metapopulation state-space approach to modeling and estimating COVID-19 spread. Mathematical Biosciences and Engineering, 2021, 18(6): 7685-7710. doi: 10.3934/mbe.2021381 |
[8] | Fang Zhu, Wei Liu . A novel medical image fusion method based on multi-scale shearing rolling weighted guided image filter. Mathematical Biosciences and Engineering, 2023, 20(8): 15374-15406. doi: 10.3934/mbe.2023687 |
[9] | Tran Quang-Huy, Phuc Thinh Doan, Nguyen Thi Hoang Yen, Duc-Tan Tran . Shear wave imaging and classification using extended Kalman filter and decision tree algorithm. Mathematical Biosciences and Engineering, 2021, 18(6): 7631-7647. doi: 10.3934/mbe.2021378 |
[10] | Sarita Bugalia, Jai Prakash Tripathi, Hao Wang . Estimating the time-dependent effective reproduction number and vaccination rate for COVID-19 in the USA and India. Mathematical Biosciences and Engineering, 2023, 20(3): 4673-4689. doi: 10.3934/mbe.2023216 |
Our recent mapping of the Dras fault zone in the NW Himalaya has answered one of the most anticipated searches in recent times where strike-slip faulting was expected from the geodetic studies. Therefore, the discovery of the fault is a leap towards the understanding of the causes of active faulting in the region, and how the plate tectonic convergence between India and Eurasia is compensated in the interior portions of the Himalayan collision zone, and what does that imply about the overall convergence budget and the associated earthquake hazards. The present work is an extended version of our previous studies on the mapping of the Dras fault zone, and we show details that were either not available or briefly touched. We have used the 30 m shuttle radar topography to map the tectonic geomorphological features that includes the fault scarps, deflected drainage, triangular facets, ridge crests, faulted Quaternary landforms and so on. The results show that oblique strike-slip faulting is active in the suture zone, which suggests that the active crustal deformation is actively compensated in the interior portions of the orogen, and it is not just restricted to the frontal portions. The Dras fault is a major fault that we have interpreted either as a south dipping oblique backthrust or an oblique north dipping normal fault. The fieldwork was conducted in Leh, but it did not reveal any evidence for active faulting, and the fieldwork in the Dras region was not possible because of the politically sensitive nature of border regions where fieldwork is always an uphill task.
The global navigation satellite system (GNSS), as the primary outdoor positioning method, has always attracted attention from scholars. In recent years, autonomous vehicles and unmanned transport equipment, which are sensitive to positioning performance, have developed rapidly at home and abroad. They have higher navigation and positioning performance requirements, requiring more accurate positioning accuracy and more robust environmental adaptability.
With the development of differential positioning and precise single-point positioning [1, 2, 3], GNSS can already provide satisfactory positioning results. However, the problem is also apparent. For example, the receiver will fail to capture and output incorrect results or not output results when the satellite signal is blocked by buildings and vegetation in urban areas. As an independent closed-loop system, the inertial navigation system (INS) has the advantages of high autonomy and strong anti-interference ability. After initial alignment, it can provide users with more accurate positioning results for a while without external input. However, due to inertial devices' inherent characteristics, INS has an accumulation of errors, so the positioning accuracy decreases with time without external corrections.
The GNSS/INS integrated system is highly complementary to each other. GNSS can correct the INS to prevent the accumulation of INS positioning errors, and INS can compensate for the deficiency that GNSS cannot position under the obstruction. It dramatically improves the performance of the entire positioning system. In loosely coupled GNSS/INS navigation, the GNSS receiver and the INS system work independently of each other, and the respective results are fused through the fusion algorithm [4, 5]. There is a cascade between the filters, and the measurement noise is time-correlated. Therefore, the accuracy of the fusion result is limited [6]. Compared with loosely coupled integration, tightly coupled GNSS/INS navigation has a higher degree of fusion. It uses satellite receiver pseudorange and pseudorange rate for fusion, which has higher positioning accuracy and anti-jamming performance [7, 8, 9]. The deep coupled GNSS/INS has reached hardware-level integration. In addition to the correction of INS errors, it also involves assisting the code loop and carrier loop in the GNSS receiver's baseband signal processing for signal acquisition and tracking through INS information [10]. Therefore, the entire system's dynamic performance and anti-interference ability are better than those of the above two combinations [11, 12].
Information fusion is the primary method of information processing in GNSS/INS integrated navigation. It can be roughly divided into filtering and non-linear optimization methods. The most representative of the filtering algorithms is the Kalman filtering algorithm under the Gaussian assumption and its various extended forms. The nonlinear optimization method is global optimization based on factor graphs. Its processing methods include the classic sum-product algorithm [13] and the latest incremental smoothing algorithm isam, isam2 proposed by Kaess et al. [14, 15].
For positioning algorithms used in vehicles, there are specific prior pieces of information as well. We can assume that under normal circumstances, a vehicle will not have lateral or vertical velocity. Furthermore, zero velocity is also crucial information. When the target is at zero velocity, various states should remain unchanged. This can effectively constrain sensors such as IMU (Inertial Measurement Unit) that have cumulative divergence. In GNSS/INS fusion systems, the most commonly used zero velocity detection algorithms are those based on IMU measurements. These methods include generalized likelihood ratio testing (GLRT) [16, 17]. Acceleration magnitude detection (MAG) [18] and angular rate magnitude detection (ARE) can also involve machine learning approaches or deep learning methods, such as support vector machines (SVM) [19, 20] and neural networks [21, 22, 23].
To ensure calculation accuracy and prevent divergence, there are two main methods: (a) The factorization method, i.e., reducing rounding errors and enhancing the filter's numerical stability through factorization and (b) adaptive filtering algorithm, i.e., adaptively adjusting the covariance matrix and reducing the dependence on prior knowledge.
The Kalman filtering algorithm of factorization has two forms: the Cholesky decomposition form and the singular value decomposition form. The SVD method was first proposed by Wang et al. [24]. The method decomposes the error covariance matrix and uses the decomposition factor to iterate. This method is also called the singular value decomposition-based square root Kalman filter (SVD-SRKF). Kulikova et al. [25] analyzed the shortcomings of the SVD-SRKF algorithm in a document published in 2017. The main shortcoming is that the error covariance matrix update formula is not used to reduce the accumulation of rounding errors. In addition, the noise matrix needs to be a Cholesky decomposition, which requires a rigorous matrix form. Kulikova et al. improved it and proposed the singular value decomposition-based Kalman filter (SVD-KF).
Mehra divided adaptive filtering methods into four categories: Bayesian, maximum likelihood, correlation and covariance matching [26]. Among them, the covariance matching method is the most widely used. It uses the error between the current noise covariance and the theoretical covariance matrix to adaptively adjust the filtering process. In 1998, Mohamed et al. [27] proposed the innovation-based adaptive estimation Kalman filter (IAEAKF) algorithm, which is based on innovations and utilizes maximum likelihood estimation for correction. They derived the correction process and also presented an equivalent form based on residuals. By correcting the noise covariance using innovations or residuals dynamically and without simultaneously correcting state noise and observation noise together, this method mitigates the impact of initialization errors and exhibits stronger interference resistance [28, 29, 30]. Bermudez et al. [31] used the standard deviation of an arithmetically differentiated small-signal segment to update the noise covariance matrix in SVD-based filter in 2020. It is also an adaptive method based on innovation. In 2017, Liu et al. [32] introduced a decay factor into IAEAKF for noise suppression in vehicle GNSS/INS integrated navigation systems. In 2019, Wei Sun proposed a new decay factor calculation method. In 2021, Pan et al. [33] added decay factors to the cubature Kalman filter (CKF) based on SVD to improve the robustness of the algorithm. The applications of decay factors enhance system stability and positioning accuracy and have practical value in certain scenarios. The Sage-Husa filter can be summarized as a Kalman filter based on covariance matching. It is constantly modified and adjusted in the iterative process to stabilize the filter. However, the main disadvantage is that the forgetting factor selection is difficult and the noise covariance is prone to be nonpositive definite before it becomes stable. The general solution is to adopt a suboptimal way to make corrections in the iterative process according to the actual situation [9, 34]. The method proposed in our paper belongs to the algorithm based on covariance matching.
The information filter (IF) algorithm is an equivalent form of the Kalman filtering algorithm, which has certain research value in some application scenarios. IF is concerned with the inverse matrix of the error covariance matrix rather than the matrix itself. Compared with the Kalman filter algorithm, the initialization of IF is more straightforward and the computational complexity of the iterative process is smaller. It is more appropriate to use IF for scenarios with sizeable initial error covariance [35]. In addition, there has been no exploration of the SVD form of IF and its adaptive performance. Therefore, research on the IF algorithm's SVD form is also of great significance.
Figure 1 shows an overall block diagram of the tightly GNSS/INS system. The input consists of raw data from IMU, GNSS observations, and satellite positions and velocities. The IMU data undergo a low-pass filter (LPF) and are used as input for the inertial navigation system (INS) to predict the pseudorange and pseudorange rate. Additionally, a neural network is employed to classify the current motion state of the target. The fusion algorithm is Kalman filtering based on SVD. Since this algorithm is applied to ground vehicles, nonholonomic constraints (NHC) are applied to improve positioning accuracy. Zero velocity update (ZUPT) are also applied in stationary states.
In general, the filtering form based on SVD reduces the accumulation of rounding error and has good filtering performance. The main contributions of this paper are reflected as follows. The Sage-Husa filter method is used to improve the Kalman filter algorithm based on SVD. Then, the decomposed matrixes are used to derive the SVD form of the adaptive method and obtain a more robust filtering method. In response to the characteristics of commonly used filtering forms based on singular values, this paper employs various adaptive methods to enhance the stability of the filter. In addition, due to the performance improvement brought by SVD, this paper will also derive the SVD form of IF. This paper conducts comprehensive experiments to compare the impact of the proposed method and common methods on positioning accuracy.
The structure of the article is as follows. First, it introduces how to perform zero velocity detection using neural networks based on IMU raw data. Second, it introduces the GNSS and INS tightly coupled integrated navigation model and then proposes an improved algorithm. Then, the SVD form of the IF is derived. Finally, the performance of the algorithm is verified by simulation and physical experiments.
Using a neural network for zero velocity determination is a binary classification task. The input consists of raw data from IMU measurements, which include three-axis accelerometer readings and three-axis gyroscope readings. The output indicates the zero-velocity state, distinguishing between stationary and in-motion states. The details on the network can be found in reference [21]. To emphasize the IMU features more effectively, a low-pass filter is applied to the data before feeding it into the network. This filtering aims to remove noise that could affect the network's classification. Here, a second-order digital low-pass filter is chosen, which allows for minimal delay while rapidly attenuating information outside the cutoff frequency.
In general, IMU data first go through the zero-velocity determination network. If the system is in a zero-velocity state at this moment, velocity and heading constraints are applied to maintain the current state.
In the Kalman filter model of tightly coupled GNSS/INS integrated navigation, the state and measurement equation are:
xk=Φk−1xk−1+wk−1 | (1) |
zk=Hkxk+vk | (2) |
where (1) is the equation of state and the subscripts k and k−1 represent moments k and k−1 respectively. State parameter xk=[δφ,δv,δp,ε,∇,bclk]T, δφ is the attitude error of the carrier coordinate system relative to the reference coordinate system. δv and δp are the velocity error and position error, respectively. ε is the bias of the accelerometer, ∇ is the bias of the gyroscope and bclk represents the clock offset and clock drift of the GNSS receiver. Φk is the state transition matrix, which is derived from the INS error equation. The continuous-to-discrete process adopts the first-order approximation. Eq (2) represents the measurement equation, and the measurement vector zk is expressed as follows:
zk=[δρkδ˙ρk]=[(ρ1G−ˆρ1I,ρ2G−ˆρ2I,⋯)T(˙ρ1G−ˆ˙ρ1I,˙ρ2G−ˆ˙ρ2I,⋯)T] | (3) |
where ρiG and ˙ρiG (i=1,2…M, M is the number of visible satellites) are the pseudorange and pseudorange rates of the i-th satellite, respectively. ˆρI and ˆ˙ρI are the pseudorange and pseudorange rates predicted by the INS results. Hk is the measurement matrix. wk and vk are the state noise vector and the measurement noise vector, respectively, and both are assumed to be Gaussian white noise with zero mean. Also, the covariances are represented by Rk and Qk [36].
Section 4.1 first gives the algorithm procedure of SVD-SRKF and SVD-KF and then improves the above two algorithms, adds constraints and proposes a more robust form. Section 4.2 derives a new form of IF, the SVD form of IF.
The SVD-SRKF algorithm procedure is given below, and the detailed derivation process can be found in reference [24].
Initialization process:
P0|0=UP0|0D2P0|0UTP0|0 | (4) |
ˆx0|0=x0|0 | (5) |
Iteration process:
LRKLTRK=R−1k | (6) |
LQKLTQK=Qk | (7) |
ˆxk|k−1=Φk−1ˆxk−1|k−1 | (8) |
[DPk−1|k−1UTPk−1|k−1Φk−1LTQK]=U∗Pk|k−1[D∗Pk|k−10]V∗TPk|k−1 | (9) |
UPk|k−1=V∗Pk|k−1 | (10) |
DPk|k−1=D∗Pk|k−1 | (11) |
[LTRKHkUPk|k−1D−1Pk|k−1]=U∗Pk|k[D∗Pk|k0]V∗TPk|k | (12) |
UPk|k=UPk|k−1V∗Pk|k | (13) |
DPk|k=(D∗Pk|k)−1 | (14) |
Kk=UPk|kD2Pk|kUTPk|kHTkLRKLTRK | (15) |
ˆxk|k=ˆxk|k−1+Kk(zk−Hkˆxk|k−1) | (16) |
where P is the estimated error covariance matrix. Eqs (9)-(11) represent the error matrix prediction process, and Eqs. (12)–(14) are the correction process. Subscript k|k−1 is the filter estimated result, and k|k is the corrected result. P0|0 and x0|0 are the initial error covariance matrix and state vector, respectively. ˆx indicates the predicted value of the state vector. UP and DP are expressed as the SVD factors of the P matrix. DP is the diagonal matrix. U∗, D∗P and V∗P are the temporary factors generated during the SVD process, and D∗ is the diagonal matrix. LR and LQ are the factors obtained after Cholesky decomposition of the inverse matrix of R and matrix Q. K is the Kalman filter gain.
SVD-KF is an improvement of SVD-SRKF. It has some commonalities. The following only gives the key steps of the algorithm. For the specific process, please refer to reference [25].
The SVD-KF has the following improvements compared to SVD-SRKF:
1. Use singular value decomposition of Qk−1 and Rk instead of Cholesky decomposition in SVD-SRKF.
2. The posterior estimation error covariance matrix Pk|k is calculated by Eq (17). Compared with the calculation form in SVD-SRKF, it can guarantee symmetry and positive definiteness.
Pk|k=(I−KkHk)Pk|k−1(I−KkHk)T+KkRkKTk | (17) |
Werries et al. [29] pointed out that it is relatively easier to obtain accurate results for the observed noise covariance Rk in the navigation system. Based on this, assume that Rk is a constant matrix. To improve the robustness of the system, it is hoped to adjust Qk adaptively, so the above two algorithms will be improved throughout the rest of this section.
In order to obtain the form of correction, it is necessary to modify the classical forms. Mohamed et al. [27] proposed and derived the maximum likelihood estimation for the measurement noise covariance:
ˆQk=KkΔzkΔzTkKTk+Pk|k+Φk−1Pk|k−1ΦTk−1 | (18) |
To obtain the SVD form of the likelihood estimation, some transformations are required. Using the new matrix (U, D and L) to replace K and P in the Eq (18):
ˆQk=KkΔzkΔzTkKTk+ξ | (19) |
where ξ can be expressed as
ξ=UPk|kD2Pk|kUTPk|k−Φk−1UPk−1|k−1D2Pk−1|k−1UTPk−1|k−1ΦTk−1 | (20) |
where Kk is shown in Eqs (15) and Δzk is innovation. For the SVD-SRKF method, using ˆQk to correct the noise covariance:
LQKLTQK=(1−dk−1)LQK−1LTQK−1+dk−1(diag(ˆQk)) | (21) |
where LQK−1LTQK−1 and LQKLTQK are the Cholesky decomposition forms before and after correction. dk=(1−b)/(1−bk), b∈[0,1] is expressed as the forgetting factor. The general value is 0.95 0.99. diag(∗) means selecting the value on the diagonal of the matrix *. According to Eq (7) of the SVD-SRKF algorithm procedure, it involves Cholesky decomposition. Therefore, this paper uses a suboptimal method, which is not used ˆQk directly but only modifies the diagonal covariance data to ensure the feasibility of decomposition. If the ˆQk matrix is directly used, then it will result in non-positive definite. The corrected algorithm is called the singular value decomposition-based adaptive square root Kalman filter (SVD-ASRKF) in the rest of the article.
Similar to the SVD-ASRKF method, the following adaptive adjustments are made to Qk in the SVD-SRKF algorithm.
UQkD2QkUTQk=(1−dk−1)UQk−1D2Qk−1UTQk−1+dk−1(ˆQk) | (22) |
where dk−1 and ˆQk have been mentioned above. Because SVD-KF directly performs SVD on Qk, ˆQk is directly used for correction in Eq (22) and the suboptimal form of diagonal correction is not needed. The improved SVD-KF algorithm is called the singular value decomposition-based adaptive Kalman filter (SVD-AKF) in the rest of the article. Figure 2 shows the algorithm flowchart about the SVD-ASRKF and SVD-AKF. The main improved work is focused on the red box selection section and the black box represents the original algorithm flowchart (SVD-SRKF and SVD-KF). As shown above, our work focuses on dynamically correcting the covariance of measurement noise through new matrix (U, D and L) and innovation. In order to express the main flow of tightly GNSS/INS integrated navigation algorithm base on SVD-ASRKF, we summarize it in Algorithm 1.
Algorithm 1 The tightly coupled GNSS/INS navigation algorithm based on SVD-ASRKF |
Input: |
1:UP and DP matrixes from SVD of error covariance matrix P0, initial state vector x0, LR and LQ matrixes from Cholesky decomposition of inverse noise covariance matrix R−1 and noise matrix Q |
2: satellite position, pseudorange, pseudorange rate, IMU raw data |
Output: position, velocity and attitude |
1: if new GNSS data comes then |
2: using current state and satellite information to predict pseudorange and pseudorange rate and calculate residuals using Eq (3) |
3: obtain the Kalman gain using the SVD matrixes in Eq (15) |
4: correct state vector and obtain ˆxk|k |
5: calculate correction about measurement noise covariance by innovation Δzk, UP and DP matrixes |
6: update LQK matrix using Eq (21) |
7: update UP and DP matrix |
8: else |
9: using imu data to predict next state |
10: predict UP and DP matrix using Eq (10) and (11) |
11: end if |
12: return position, velocity and attitude |
The main flow of the tightly coupled navigation algorithm based on SVD-AKF is shown in Algorithm 2. The main different steps compared to Algorithm 1 are steps 6–10.
Algorithm 2 The tightly coupled GNSS/INS navigation algorithm based on SVD-AKF |
Input: |
1:UP and DP matrixes from SVD of error covariance matrix P0, initial state vector x0, UR and DR matrixes from SVD of noise covariance matrix R, UQ and DQ matrixes from SVD of noise covariance matrix Q |
2: satellite position, pseudorange, pseudorange rate, IMU raw data |
Output: position, velocity and attitude |
1: if new GNSS data comes then |
2: using current state and satellite information to predict pseudorange and pseudorange rate and calculate residuals from Eq (3) |
3: obtain the Kalman gain using the SVD matrix |
4: correct state vector and obtain ˆxk|k |
5: calculate correction about measurement noise covariance by innovation Δzk, UP and DP matrix |
6: update UQ and DQ matrix using Eq (22) |
7: update UP and DP matrix |
8: else |
9: using imu data to predict next state |
10: predict UP and DP matrix |
11: end if |
12: return position, velocity and attitude |
The above is the improved algorithm of the SVD-SRKF and SVD-KF algorithms. In practical applications, to simplify the calculation, Eqs (21) and (22) are reanalyzed first. The main problems are the limitation of the number of iterations and the selection of the forgetting factor. For the first problem, when the number of iterations approaches infinity, dk approaches 1−b. The effect of the innovation Δzk decreases, and the current noise information is mainly determined by the past state.
dk→1−b(k→∞) | (23) |
The dk in Eqs (21) and (22) do not need to be iterated all the time. There are two main reasons for this. First, we reduce the computational overhead. Second, it is affected by the limited word length. Therefore, the calculation can be stopped as long as dk reaches an expected small range. Different forget factors might be used in various scenarios, so we use delta dk as the criteria for assessment and the selected delta range is less than 5.0*10-4 in this paper. In addition, the forgetting factor can be selected by the following methods. First, choose a relatively large forgetting factor because the initial matrix Q is generally accurate. Then, the forgetting factor can be adjusted according to certain criteria. The judgment criterion selects the magnitude of the change in the Q square error. Calculate by the following formula:
ek=∑i,j(ˆQk,i,j)2 | (24) |
where ˆQk is the same as in (18) and i and j represent each element in the matrix. When error ek is greater than the expected threshold, it indicates that the deviation of Q is large, and the forgetting factor can be decreased to speed up the adjustment speed. The threshold can be selected three times the square error of the previous state, which is 3ek−1.
In general, the adaptive adjustment of the noise matrix makes the algorithm more robust. In addition, the constraints on Eqs (21) and (22) save computational overhead and are more suitable for engineering implementation.
IF is the equivalent form of the Kalman filter algorithm. Compared with the Kalman filter algorithm, it is more suitable to use IF in scenes with less initial information. Better filtering performance can be obtained by deducing the SVD form of IF.
The classical formula of IF is as follows [35]:
P−1k|k−1=Ak−1−Ak−1(Q−1k−1+Ak−1)−1Ak−1 | (25) |
P−1k|k−1ˆxk|k−1=(I−Ak−1Qk−1)−1(Φ−1k−1TP−1k−1|k−1ˆxk−1|k−1) | (26) |
P−1k|kˆxk|k=P−1k|k−1ˆxk|k−1+HTkR−1kzk | (27) |
P−1k|k=P−1k|k−1+HTkR−1kHk | (28) |
where P−1 is the inverse matrix of error matrix P, which is traditionally called the information matrix, and Ak−1=Φ−1k−1TP−1k−1|k−1Φ−1k−1.
First, decompose P−1k|k
P−1k|k=UP−1k|kD2P−1k|kUTP−1k|k | (29) |
Then, we analyze the prediction process of P−1. Because
Pk|k−1=Φk−1Pk−1|k−1ΦTk−1+Qk−1 | (30) |
Now, we want to solve the factors UP−1k|k-1 and DP−1k|k-1 from Eq (30), make an equivalent change to it and perform SVD on Q.
(P−1k|k−1)−1=Φk−1(P−1k−1|k−1)−1ΦTk+UQk−1D2Qk−1UTQk−1 | (31) |
By substituting Eq (32), which represents the decomposition factor, into (31) and decomposing it, Eq (33) can be obtained.
(P−1k−1|k−1)−1=(UP−1k-1|k-1D2P−1k-1|k-1UTP−1k-1|k-1)−1=UP−1k-1|k-1D−2P−1k-1|k-1UTP−1k-1|k-1 | (32) |
[D−1P−1k-1|k-1UTP−1k-1|k-1Φk−1DQk−1UTQk−1]=U∗P−1k|k-1[D∗P−1k|k-10]V∗TP−1k|k-1 | (33) |
So, we can obtain that:
UP−1k|k-1=V∗P−1k|k-1 | (34) |
DP−1k|k-1=(D∗P−1k|k-1)−1 | (35) |
Then, solve the decomposition factors UP−1k|k and DP−1k|k. Decompose Eq (28):
[DP−1k|k-1UTP−1k|k-1DR−1kUTR−1kHk]=U∗P−1k|k[D∗P−1k|k0]V∗TP−1k|k | (36) |
We know that the right side of the equation is the decomposition factor we need. Which is:
UP−1k|k=V∗P−1k|k | (37) |
DP−1k|k=D∗P−1k|k | (38) |
A new IF form based on SVD is obtained through the above derivation, which is called singular value decomposition based information filters (SVD-IF) in the rest of the article. In summary, the algorithm steps are shown as follows.
Initialization process:
P−10|0=UP−10|0D2P−10|0UTP−10|0 | (39) |
Qk−1=UQk−1D2Qk−1UTQk−1 | (40) |
R−1k=DR−1kUTR−1kHk | (41) |
ˆx0|0=x0|0 | (42) |
Iteration process:
[D−1P−1k-1|k-1UTP−1k-1|k-1Φk−1DQk−1UTQk−1]=U∗P−1k|k-1[D∗P−1k|k-10]V∗TP−1k|k-1 | (43) |
UP−1k|k-1=V∗P−1k|k-1 | (44) |
DP−1k|k-1=(D∗P−1k|k-1)−1 | (45) |
UP−1k|k-1D2P−1k|k-1UTP−1k|k-1ˆxk|k−1=(I−Ak−1UQk−1D2Qk−1UTQk−1)−1(Φ−1k−1TUP−1k-1|k-1D2P−1k-1|k-1UTP−1k-1|k-1ˆxk−1|k−1) | (46) |
where Ak−1 is expressed as Ak−1=Φ−1k−1TUP−1k-1|k-1D2P−1k-1|k-1UTP−1k-1|k-1Φ−1k−1.
[DP−1k|k-1UTP−1k|k-1DR−1kUTR−1kHk]=U∗P−1k|k[D∗P−1k|k0]V∗TP−1k|k | (47) |
UP−1k|k=V∗P−1k|k | (48) |
DP−1k|k=D∗P−1k|k | (49) |
UP−1k|kDP−1k|kUTP−1k|kˆxk|k=UP−1k|k-1D2P−1k|k-1UTP−1k|k-1ˆxk|k−1+HTkDR−1kUTR−1kHkzk | (50) |
We summarize the GNSS/INS navigation algorithm based on SVD-IF in Algorithm 3. Through the SVD of the information matrix of IF, the accumulation of rounding error in the iterative calculation process can be alleviated in the case of limited word length, making the filtering algorithm more stable and the location result more accurate.
Algorithm 3 The tightly coupled GNSS/INS navigation algorithm based on SVD-IF |
Input: |
1: UP−1 and DP−1 matrixes from SVD of inverse error covariance matrix P−10、initial state vector x0、UR−1 and DR−1 matrixes from SVD of inverse noise covariance matrix R−1、UQ and DQ matrix from SVD of noise covariance matrix Q |
2: satellite position, pseudorange, pseudorange rate, IMU raw data |
Output: position, velocity and attitude |
1: if new GNSS data comes then |
2: using current state and satellite information to predict pseudorange and pseudorange rate and calculate residuals |
3: update UP−1 and DP−1 matrixes from Eq (48) and(49) |
4: correct state vector and obtain ˆxk|k |
5: else |
6: predict UP−1 and DP−1 matrixes using Eq (44) and (45) |
7: using imu data to predict next state |
8: end if |
9: return position, velocity and attitude |
To evaluate the performance of the method proposed in this article, this section first conducts a tightly coupled GNSS/INS integrated navigation simulation experiment. Then, it is applied to actual navigation scenarios. In the experiment, the variable's storage width is 32 bits, where the sign bit is 1 bit, the exponent is 8 bits and the mantissa is 23 bits. The methods that need to be simulated and compared are listed:
a) KF: conventional Kalman filter
b) SVD-SRKF: singular value decomposition-based square root Kalman filter
c) SVD-KF: improved SVD-SRKF
d) SVD-ASRKF: the improved SVD-SRKF proposed in this article
e) SVD-AKF: the improved SVD-KF method proposed in this article
f) IF: conventional information filters
g) SVD-IF: the singular value decomposition-based IF proposed in this article
In the tightly coupled GNSS/INS integrated navigation simulation, 50 Monte Carlo experiments were carried out. The GNSS data output frequency is 2 Hz, the IMU frequency is 100 Hz and the sampling time is 60 s. The simulation selects a low dynamic motion scene and the target velocity is 20 m/s. To increase the dynamic performance of the target and make the scene more general, two 90° turns are made at 15 s and 45 s. The random standard variances of the accelerometer and gyroscope are 3 mg and 1°/h, respectively. Other initial parameters are set as shown in Table 1:
Parameters | value |
Accelerometer biases m/s2 | aX=0.0883, aY=−0.1275, aZ=0.0785 |
Gyro biases rad/s | gX=gZ=−8.7×10−4, gY=13×10−4 |
Earth radius m | 6378137 |
Rotation speed of Earth rad/s | 7.292115×10−5rad/s |
Initial velocity error m/s | δVX=δVY=δVZ=0.1 |
Initial position error m | δPX=δPY=δPZ=10 |
Initial angle error ° | ϕX=ϕY=ϕZ=0.03 |
Figure 3 shows the comparison results, selecting the local navigation frame (ENU). In the figure, the X-axis represents the sampling time in seconds and the Y-axis represents the position error in meters. The experimental results show that compared with the conventional Kalman filter, SVD-KF and SVD-SRKF both have better filtering performance. The SVD of the correlation matrix can reduce rounding errors and improve filtering performance. However, there is no significant difference between the filter performance of SVD-KF and SVD-SRKF in this simulation.
Figure 4 is the simulation result of the improved filtering form proposed in this paper. The experimental results show that the method proposed in this paper, due to the real-time correction of the state noise covariance in the iterative process, has higher accuracy and better robustness than the above two methods. It also has a more stable performance in turning where the target's motion state changes quickly.
The positioning result of SVD-IF is shown in Figure 5. It can be concluded that SVD-IF also has higher positioning accuracy and robustness than IF. Especially when the error matrix is large, it is more appropriate to use SVD-IF.
To further prove the performance of the algorithm, a physical experiment is implemented. The GNSS receiver and INS use ProPak6 and IMU-FSAS, which are made by NovAtel. The GNSS receiver output frequency is 1 Hz, and the INS output frequency is 10 Hz. The accelerometer bias and scale factor of INS are 1 mg and 300 ppm, respectively. The gyro bias and scale factors are 0.75°/h and 400 ppm, respectively. The coordinate system uses ECEF, and the reference position adopts the position that has been accurately calibrated. In the static experiment, the ZUPT will be closed.
In addition to static experiments, dynamic experiments were also conducted. In this experiment, a GNSS reference station was set up at a fixed location. The trajectory obtained through real-time kinematic differential positioning is used as the reference trajectory for vehicle movement. The precise coordinates of the GNSS reference station are accurately known. The evaluation metrics are maximum and root mean squared error (RMSE).
The static experiment results are as follows.
Figure 6 compares the positioning error between the conventional Kalman filter and the SVD form filter. In this figure, the X-axis represents the sampling time in seconds, and the Y-axis represents the position error in the ECEF. Through the experimental results, it can be concluded that the Kalman filter based on SVD has better performance in position accuracy.
The positioning results about the improved filtering form are shown in Figure 7. Table 2 shows the detailed positioning results. The RMSE of each method on each coordinate axis is listed. The experimental results show that compared with SVD-SRKF and SVD-KF, the improved filtering forms of SVD-ASRKF and SVD-AKF have smaller positioning errors on each coordinate axis. The Z-axis positioning error decreased the most, with a maximum reduction of 25.7% and a minimum reduction of 6.8%. There is also a 3.3% reduction in the X-axis error.
Algorithm | SVD-SRKF | SVD-KF | SVD-ASRKF | SVD-AKF | SVD-IF | IF | ||||||
X-axis | 6.04 | 6.01 | 5.82 | 5.81 | 6.07 | 6.37 | ||||||
Y-axis | 18.38 | 18.34 | 18.29 | 18.25 | 18.17 | 18.26 | ||||||
Z-axis | 1.94 | 1.91 | 1.44 | 1.78 | 1.94 | 2.26 |
Figure 8 shows the performance comparison of IF and SVD-IF under the above experimental conditions. The experimental results show that SVD-IF has higher accuracy and robustness than IF. The specific error results are listed in Table 2. The RMSE in the X direction decreases by 4.7%.
The dynamic experiment results are as follows.
Zero velocity detection on the target vehicle is performed using a neural network and the results are shown in Figure 9. In the figure, the detection result curve has only two values, "0" and "2". A value of "0" indicates the detection of zero velocity, while a value of "2" indicates that the target is in motion. Due to the presence of high-frequency environmental noise and vibration noise from the vehicle when it is stationary, a low-pass filter is applied before the detector to enhance the detection rate. The experimental results demonstrate that the detection rate reaches 94.73%.
Analyzing the experimental results, it can be observed that when the target transitions from an open sky to a scene in which the GNSS signal is obstructed, the number of visible satellites decreases. In this case, if some of the visible satellites involved in the positioning solution exhibit significant observation errors, it can lead to fluctuations in the final positioning solution. The red rectangular box highlighted in Figures 10 and 11 represents the scenario described above, and the change in the number of satellites is shown in the rectangular box in Figure 12. In Figure 10, the proposed methods have smaller fluctuation. In addition, due to the lack of spatial error compensation for ionosphere and troposphere delay, the trajectories do not align very well. However, absolute positioning accuracy is not the primary focus of the algorithm and does not affect performance comparisons. In Figure 11, it can be observed that the proposed method, compared to the Kalman filtering algorithms based on classical SVD methods, exhibits superior positioning performance in such complex environments. As errors in GNSS observations, the method proposed in this paper adaptively increases the covariance of the measurements. This, in turn, places greater trust in the pure IMU derived results, thereby reducing the impact of erroneous GNSS observations.
The rectangular box highlighted in Figure 13 shows that the convergence speed of SVD-ASRKF is slower compared to other algorithms. There are two reasons for this. First, SVD-ASRKF uses a suboptimal method, which only modifies the diagonal covariance data to ensure the feasibility of decomposition. Second, the GNSS signal consistently fluctuates after algorithm initialization, resulting in slower convergence. In addition, due to the adaptive methods involve solving the correction, the computational workload for the adaptive form is relatively higher compared to classical methods.
Compared to SVD-KF and SVD-SRKF in dynamic scenarios, the method proposed in this paper reduces the maximum values from 52.31 m and 52.41 m to 28.37 m and 30.15 m, respectively. The maximum values were reduced by 45.77% and 42.47%, respectively.
This paper proposes a more robust filtering method for different Kalman filtering forms based on SVD. The noise covariance matrix of SVD-SRKF and SVD-KF is modified dynamically by covariance matching to achieve a better filtering effect. The number of correction processes is constrained and an effective forgetting factor selection strategy is proposed in practical applications. In addition, the SVD form of IF is derived. Monte Carlo simulation experiments and physical experiments show that the RMSE of the proposed Kalman filtering method and SVD-IF positioning results are lower than those of the existing methods. This proves that the proposed method and SVD-IF positioning accuracy are higher and have theoretical reference and engineering value.
To enhance the applicability of the new algorithm, further work can be conducted in the two following areas: (a) researching more effective adaptive methods to solve the slow convergence problem of SVD-ASRKF using suboptimal methods and (b) incorporating Doppler information from GNSS receivers into network training to improve zero velocity detection accuracy.
The authors declare they have not used Artificial Intelligence (AI) tools in the creation of this article.
This work was supported by the Research and Demonstration of Holographic Portrait for Highway Network Structure and Traffic Safety Forewarning and Control Technology in Ningxia, Key R & D Program of NingXia (2023BBF01004).
The authors declare there is no conflict of interest.
[1] | Shah AA, Addly AABM, Samat MIBA (2018) Geomorphic Mapping Reveals NW-SE Extension in NW Himalaya. Arab J Geosci 385-389. |
[2] |
Baker DM, Robert J, Robert S, et al. (1988) Development of the Himalayan frontal thrust zone: Salt Range, Pakistan. Geology 16: 3-7. doi: 10.1130/0091-7613(1988)016<0003:DOTHFT>2.3.CO;2
![]() |
[3] | Yeats RS, Nakata T, Farah A, et al. (1992) The Himalayan frontal fault system. Ann Tecton 6: 85-98. |
[4] |
Wesnousky SG, Kumar S, Mohindra R, et al. (1999) Uplift and convergence along the Himalayan Frontal Thrust of India. Tectonics 18: 967-976. doi: 10.1029/1999TC900026
![]() |
[5] |
Malik JN, Sahoo AK, Shah AA (2010) Paleoseismic evidence from trench investigation along Hajipur fault, Himalayan Frontal Thrust, NW Himalaya: implications of the faulting pattern on landscape evolution and seismic hazard. J Struct Geol 32: 350-361. doi: 10.1016/j.jsg.2010.01.005
![]() |
[6] |
Wesnousky SG, Kumahara Y, Chamlagain D, et al. (2017) Geological observations on large earthquakes along the Himalayan frontal fault near Kathmandu, Nepal. Earth Planet Sci Lett 457: 366-375. doi: 10.1016/j.epsl.2016.10.006
![]() |
[7] |
Coudurier-Curveur A, Tapponnier P, Okal E, et al. (2020) A composite rupture model for the great 1950 Assam earthquake across the cusp of the East Himalayan Syntaxis. Earth Planet Sci Lett 531: 115928. doi: 10.1016/j.epsl.2019.115928
![]() |
[8] |
Cloos M (1993) Lithospheric buoyancy and collisional orogenesis: Subduction of oceanic plateaus, continental margins, island arcs, spreading ridges, and seamounts. Geol Soc Am Bull 105: 715-737. doi: 10.1130/0016-7606(1993)105<0715:LBACOS>2.3.CO;2
![]() |
[9] |
Tapponnier P, Molnar P (1977) Active faulting and tectonics in China. J Geophys Res 82: 2905-2930. doi: 10.1029/JB082i020p02905
![]() |
[10] | Keller EA (1986) Investigation of active tectonics: use of surficial earth processes. Tectonics 1: 136-147. |
[11] | Malik JN, Nakata T (2003) Active faults and related late Quaternary deformation along the northwestern Himalayan frontal zone, India. Ann Geophys 46: 917-936. |
[12] |
Shah AA (2013) Earthquake geology of Kashmir Basin and its implications for future large earthquakes. Int J Earth Sci 102: 1957-1966. doi: 10.1007/s00531-013-0874-8
![]() |
[13] | Shah AA, Sahari S, Navakanesh B, et al. (2021) Tectonic geomorphology of the Jhelum fault zone and its contiguous regions in western Himalaya. Bull Geol Soc Mal 73. |
[14] |
Zhao LS, Xie J (1993) Lateral variations in compressional velocities beneath the Tibetan Plateau from Pn travel time tomography. Geophys J Int 115: 1070-1084. doi: 10.1111/j.1365-246X.1993.tb01510.x
![]() |
[15] |
Yin A (2000) Mode of Cenozoic east‐west extension in Tibet suggesting a common origin of rifts in Asia during the Indo‐Asian collision. J Geophys Res 105: 21745-21759. doi: 10.1029/2000JB900168
![]() |
[16] |
Stevens VL, Avouac JP (2015) Interseismic coupling on the main Himalayan thrust. Geophys Res Lett 42: 5828-5837. doi: 10.1002/2015GL064845
![]() |
[17] |
Shah AA (2015) Kashmir basin fault and its tectonic significance in NW Himalaya, Jammu and Kashmir, India. Int J Earth Sci 104: 1901-1906. doi: 10.1007/s00531-015-1183-1
![]() |
[18] | Sahoo S, Malik JN (2017) Active fault topography along Kangra valley fault in the epicentral zone of 1905 Mw7. 8 earthquake NW Himalaya, India. Quat Int 462: 90-108. |
[19] |
Burg JP, Bouilhol P (2018) Timeline of the South-Tibet—Himalayan belt: the geochronological record of subduction, collision, and underthrusting from zircon and monazite U-Pb ages. Can J Earth Sci 56: 1318-1332 doi: 10.1139/cjes-2018-0174
![]() |
[20] |
Shah AA, Abd MN, Aliudin NAB, et al. (2020) Formation, Rotation, and Present-Day Configuration of Kashmir and Peshawar Basins in NW Himalaya. Front Earth Sci 8: 569771. doi: 10.3389/feart.2020.569771
![]() |
[21] |
Yin A, Harrison TM (2000) Geologic Evolution of the Himalayan-Tibetan Orogen. Annu Rev Earth Planet Sci 28: 211-280. doi: 10.1146/annurev.earth.28.1.211
![]() |
[22] |
Murphy MA, Yin A (2003) Structural evolution and sequence of thrusting in the Tethyan fold-thrust belt and Indus-Yalu suture zone, southwest Tibet. GSA Bull 115: 21-34. doi: 10.1130/0016-7606(2003)115<0021:SEASOT>2.0.CO;2
![]() |
[23] |
Sinclair HD, Jaffey N (2001) Sedimentology of the Indus group, Ladakh, northern India: Implications for the timing of initiation of the palaeo‐Indus River. J Geol Soc 158: 151-162 doi: 10.1144/jgs.158.1.151
![]() |
[24] |
Garzanti E, Haver TV (1988) The Indus clastics: Fore‐arc basin sedimentation in the Ladakh Himalaya (India). Sediment Geol 59: 237-249. doi: 10.1016/0037-0738(88)90078-4
![]() |
[25] |
Searle MP, Pickering KT, Cooper DJW (1990) Restoration and evolution of the intermontane Indus molasse basin, Ladakh Himalaya, India. Tectonophysics 174: 301-314. doi: 10.1016/0040-1951(90)90327-5
![]() |
[26] |
Searle MP (1986) Structural evolution and sequence of thrusting in the High Himalayan, Tibetan—Tethys and Indus suture zones of Zanskar and Ladakh, Western Himalaya. J Struct Geol 8: 923-936. doi: 10.1016/0191-8141(86)90037-4
![]() |
[27] |
Qayyum M, Lawrence RD, Niem AR (1997) Discovery of the palaeo-Indus delta-fan complex. J Geol Soc 154: 753-756. doi: 10.1144/gsjgs.154.5.0753
![]() |
[28] | Nakata T (1989) Active faults of the Himalayas of India and Nepal. Spec Pap Geol Soc Am 232: 243-264. |
[29] | Sahoo S, Malik JN (2017) Active fault topography along Kangra valley fault in the epicentral zone of 1905 Mw7. 8 earthquake NW Himalaya, India. Quat Int 462: 90-108. |
[30] | Shah AA, Syaakiirroh Sahari, Navakanesh B, et al. (2022) Tectonic geomorphology of the Jhelum fault zone and its contiguous regions in western Himalaya. Bull Geol Soc Malay 73. |
[31] |
Shah AA, Navakanesh B (2020) Active tectonics and active faults: Why these terms still lack consensus on definitions. Bull Geol Soc Malay 70: 125-132. doi: 10.7186/bgsm70202010
![]() |
[32] | DiPietro JA, Pogue KR (2004) Tectonostratigraphic subdivisions of the Himalaya: A view from the west. Tectonics 23. |
[33] | Jouanne F, Munawar N, Mugnier JL, et al. (2020) Seismic coupling quantified on inferred décollements beneath the western syntaxis of the Himalaya. Tectonics 39. |
[34] | Shah AA, Rajasekharan A, Batmanathan N, et al. (2021) Dras Fault: a major active fault in Kashmir Himalaya. Bull N Z Soc Earthq Eng 30. |
[35] | Sangode SJ, Phadtare NR, Meshram DC, et al. (2011) A record of lake outburst in the Indus valley of Ladakh Himalaya, India. Curr Sci 100: 1712-1718. |
[36] |
Gansser A (1980) The significance of the Himalayan suture zone. Tectonophysics 62: 37-52. doi: 10.1016/0040-1951(80)90134-1
![]() |
[37] |
Searle MP (1983) Stratigraphy, structure and evolution of the Tibetan-Tethys zone in Zanskar and the Indus suture zone in the Ladakh Himalaya. Earth Environ Sci Trans R Soc Edinb 73: 205-219. doi: 10.1017/S0263593300009688
![]() |
[38] | Ahmad SA, Sahari S, Qadir A, et al. (2020) A critical review of field relationships and gravitational origin of active normal faults in the Kashmir basin, NW Himalaya. J Asian Earth Sci X 100042. |
[39] |
Schiffman C, Bali BS, Szeliga W, et al. (2013) Seismic slip deficit in the Kashmir Himalaya from GPS observations. Geophys Res Lett 40: 5642-5645. doi: 10.1002/2013GL057700
![]() |
[40] |
Kundu B, Yadav RK, Bali BS, et al. (2014) Oblique convergence and slip partitioning in the NW Himalaya: Implications from GPS measurements. Tectonics 33: 2013-2024. doi: 10.1002/2014TC003633
![]() |
[41] |
Bilham R (2019) Himalayan earthquakes: a review of historical seismicity and early 21st century slip potential. Geol Soc Spec Publ 483: 423-482. doi: 10.1144/SP483.16
![]() |
[42] | Omar Pengiran DNA, Shah AA, Abd MN (2020) Active transtensional structures mapped in the west of Karakoram fault (KF), Kashmir Himalayas. 5th International Young Earth Scientists (YES) Congress "Rocking Earth's Future". |
[43] |
Koukouvelas IK, Zygouri V, Papadopoulos GA, et al. (2017) Holocene record of slip-predictable earthquakes on the Kenchreai Fault, Gulf of Corinth, Greece. J Struct Geol 94: 258-274. doi: 10.1016/j.jsg.2016.12.001
![]() |
[44] |
Koukouvelas IK, Zygouri V, Nikolakopoulos K, et al. (2018) Treatise on the tectonic geomorphology of active faults: The significance of using a universal digital elevation model. J Struct Geol 116: 241-252. doi: 10.1016/j.jsg.2018.06.007
![]() |
1. | Li Xue, Yongmin Zhong, Yulan Han, Constrained Cubature Particle Filter for Vehicle Navigation, 2024, 24, 1424-8220, 1228, 10.3390/s24041228 | |
2. | Mohammed AFTATAH , Khalid ZEBBARA , Robust ConvNet-Kalman Filter Integration for Mitigating GPS Jamming and Spoofing Attacks Basing on Inertial Navigation System Data, 2024, 3, 2953-4917, 10.56294/dm2024.405 | |
3. | Zijian Wang, Jianghua Liu, Jinguang Jiang, Jiaji Wu, Qinghai Wang, Jingnan Liu, An Adaptive Combined Filtering Algorithm for Non-Holonomic Constraints with Time-Varying and Thick-Tailed Measurement Noise, 2025, 17, 2072-4292, 1126, 10.3390/rs17071126 |
Algorithm 1 The tightly coupled GNSS/INS navigation algorithm based on SVD-ASRKF |
Input: |
1:UP and DP matrixes from SVD of error covariance matrix P0, initial state vector x0, LR and LQ matrixes from Cholesky decomposition of inverse noise covariance matrix R−1 and noise matrix Q |
2: satellite position, pseudorange, pseudorange rate, IMU raw data |
Output: position, velocity and attitude |
1: if new GNSS data comes then |
2: using current state and satellite information to predict pseudorange and pseudorange rate and calculate residuals using Eq (3) |
3: obtain the Kalman gain using the SVD matrixes in Eq (15) |
4: correct state vector and obtain ˆxk|k |
5: calculate correction about measurement noise covariance by innovation Δzk, UP and DP matrixes |
6: update LQK matrix using Eq (21) |
7: update UP and DP matrix |
8: else |
9: using imu data to predict next state |
10: predict UP and DP matrix using Eq (10) and (11) |
11: end if |
12: return position, velocity and attitude |
Algorithm 2 The tightly coupled GNSS/INS navigation algorithm based on SVD-AKF |
Input: |
1:UP and DP matrixes from SVD of error covariance matrix P0, initial state vector x0, UR and DR matrixes from SVD of noise covariance matrix R, UQ and DQ matrixes from SVD of noise covariance matrix Q |
2: satellite position, pseudorange, pseudorange rate, IMU raw data |
Output: position, velocity and attitude |
1: if new GNSS data comes then |
2: using current state and satellite information to predict pseudorange and pseudorange rate and calculate residuals from Eq (3) |
3: obtain the Kalman gain using the SVD matrix |
4: correct state vector and obtain ˆxk|k |
5: calculate correction about measurement noise covariance by innovation Δzk, UP and DP matrix |
6: update UQ and DQ matrix using Eq (22) |
7: update UP and DP matrix |
8: else |
9: using imu data to predict next state |
10: predict UP and DP matrix |
11: end if |
12: return position, velocity and attitude |
Algorithm 3 The tightly coupled GNSS/INS navigation algorithm based on SVD-IF |
Input: |
1: UP−1 and DP−1 matrixes from SVD of inverse error covariance matrix P−10、initial state vector x0、UR−1 and DR−1 matrixes from SVD of inverse noise covariance matrix R−1、UQ and DQ matrix from SVD of noise covariance matrix Q |
2: satellite position, pseudorange, pseudorange rate, IMU raw data |
Output: position, velocity and attitude |
1: if new GNSS data comes then |
2: using current state and satellite information to predict pseudorange and pseudorange rate and calculate residuals |
3: update UP−1 and DP−1 matrixes from Eq (48) and(49) |
4: correct state vector and obtain ˆxk|k |
5: else |
6: predict UP−1 and DP−1 matrixes using Eq (44) and (45) |
7: using imu data to predict next state |
8: end if |
9: return position, velocity and attitude |
Parameters | value |
Accelerometer biases m/s2 | aX=0.0883, aY=−0.1275, aZ=0.0785 |
Gyro biases rad/s | gX=gZ=−8.7×10−4, gY=13×10−4 |
Earth radius m | 6378137 |
Rotation speed of Earth rad/s | 7.292115×10−5rad/s |
Initial velocity error m/s | δVX=δVY=δVZ=0.1 |
Initial position error m | δPX=δPY=δPZ=10 |
Initial angle error ° | ϕX=ϕY=ϕZ=0.03 |
Algorithm | SVD-SRKF | SVD-KF | SVD-ASRKF | SVD-AKF | SVD-IF | IF | ||||||
X-axis | 6.04 | 6.01 | 5.82 | 5.81 | 6.07 | 6.37 | ||||||
Y-axis | 18.38 | 18.34 | 18.29 | 18.25 | 18.17 | 18.26 | ||||||
Z-axis | 1.94 | 1.91 | 1.44 | 1.78 | 1.94 | 2.26 |
Algorithm 1 The tightly coupled GNSS/INS navigation algorithm based on SVD-ASRKF |
Input: |
1:UP and DP matrixes from SVD of error covariance matrix P0, initial state vector x0, LR and LQ matrixes from Cholesky decomposition of inverse noise covariance matrix R−1 and noise matrix Q |
2: satellite position, pseudorange, pseudorange rate, IMU raw data |
Output: position, velocity and attitude |
1: if new GNSS data comes then |
2: using current state and satellite information to predict pseudorange and pseudorange rate and calculate residuals using Eq (3) |
3: obtain the Kalman gain using the SVD matrixes in Eq (15) |
4: correct state vector and obtain ˆxk|k |
5: calculate correction about measurement noise covariance by innovation Δzk, UP and DP matrixes |
6: update LQK matrix using Eq (21) |
7: update UP and DP matrix |
8: else |
9: using imu data to predict next state |
10: predict UP and DP matrix using Eq (10) and (11) |
11: end if |
12: return position, velocity and attitude |
Algorithm 2 The tightly coupled GNSS/INS navigation algorithm based on SVD-AKF |
Input: |
1:UP and DP matrixes from SVD of error covariance matrix P0, initial state vector x0, UR and DR matrixes from SVD of noise covariance matrix R, UQ and DQ matrixes from SVD of noise covariance matrix Q |
2: satellite position, pseudorange, pseudorange rate, IMU raw data |
Output: position, velocity and attitude |
1: if new GNSS data comes then |
2: using current state and satellite information to predict pseudorange and pseudorange rate and calculate residuals from Eq (3) |
3: obtain the Kalman gain using the SVD matrix |
4: correct state vector and obtain ˆxk|k |
5: calculate correction about measurement noise covariance by innovation Δzk, UP and DP matrix |
6: update UQ and DQ matrix using Eq (22) |
7: update UP and DP matrix |
8: else |
9: using imu data to predict next state |
10: predict UP and DP matrix |
11: end if |
12: return position, velocity and attitude |
Algorithm 3 The tightly coupled GNSS/INS navigation algorithm based on SVD-IF |
Input: |
1: UP−1 and DP−1 matrixes from SVD of inverse error covariance matrix P−10、initial state vector x0、UR−1 and DR−1 matrixes from SVD of inverse noise covariance matrix R−1、UQ and DQ matrix from SVD of noise covariance matrix Q |
2: satellite position, pseudorange, pseudorange rate, IMU raw data |
Output: position, velocity and attitude |
1: if new GNSS data comes then |
2: using current state and satellite information to predict pseudorange and pseudorange rate and calculate residuals |
3: update UP−1 and DP−1 matrixes from Eq (48) and(49) |
4: correct state vector and obtain ˆxk|k |
5: else |
6: predict UP−1 and DP−1 matrixes using Eq (44) and (45) |
7: using imu data to predict next state |
8: end if |
9: return position, velocity and attitude |
Parameters | value |
Accelerometer biases m/s2 | aX=0.0883, aY=−0.1275, aZ=0.0785 |
Gyro biases rad/s | gX=gZ=−8.7×10−4, gY=13×10−4 |
Earth radius m | 6378137 |
Rotation speed of Earth rad/s | 7.292115×10−5rad/s |
Initial velocity error m/s | δVX=δVY=δVZ=0.1 |
Initial position error m | δPX=δPY=δPZ=10 |
Initial angle error ° | ϕX=ϕY=ϕZ=0.03 |
Algorithm | SVD-SRKF | SVD-KF | SVD-ASRKF | SVD-AKF | SVD-IF | IF | ||||||
X-axis | 6.04 | 6.01 | 5.82 | 5.81 | 6.07 | 6.37 | ||||||
Y-axis | 18.38 | 18.34 | 18.29 | 18.25 | 18.17 | 18.26 | ||||||
Z-axis | 1.94 | 1.91 | 1.44 | 1.78 | 1.94 | 2.26 |