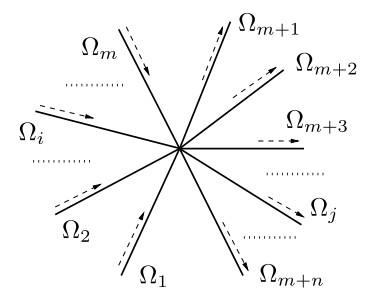
We consider the idealized setting of gradient flow on the population risk for infinitely wide two-layer ReLU neural networks (without bias), and study the effect of symmetries on the learned parameters and predictors. We first describe a general class of symmetries which, when satisfied by the target function f∗ and the input distribution, are preserved by the dynamics. We then study more specific cases. When f∗ is odd, we show that the dynamics of the predictor reduces to that of a (non-linearly parameterized) linear predictor, and its exponential convergence can be guaranteed. When f∗ has a low-dimensional structure, we prove that the gradient flow PDE reduces to a lower-dimensional PDE. Furthermore, we present informal and numerical arguments that suggest that the input neurons align with the lower-dimensional structure of the problem.
Citation: Karl Hajjar, Lénaïc Chizat. On the symmetries in the dynamics of wide two-layer neural networks[J]. Electronic Research Archive, 2023, 31(4): 2175-2212. doi: 10.3934/era.2023112
[1] | Giuseppe Maria Coclite, Carlotta Donadello . Vanishing viscosity on a star-shaped graph under general transmission conditions at the node. Networks and Heterogeneous Media, 2020, 15(2): 197-213. doi: 10.3934/nhm.2020009 |
[2] | John D. Towers . An explicit finite volume algorithm for vanishing viscosity solutions on a network. Networks and Heterogeneous Media, 2022, 17(1): 1-13. doi: 10.3934/nhm.2021021 |
[3] | Boris Andreianov, Kenneth H. Karlsen, Nils H. Risebro . On vanishing viscosity approximation of conservation laws with discontinuous flux. Networks and Heterogeneous Media, 2010, 5(3): 617-633. doi: 10.3934/nhm.2010.5.617 |
[4] | Wen Shen . Traveling wave profiles for a Follow-the-Leader model for traffic flow with rough road condition. Networks and Heterogeneous Media, 2018, 13(3): 449-478. doi: 10.3934/nhm.2018020 |
[5] | Martin Gugat, Mario Sigalotti . Stars of vibrating strings: Switching boundary feedback stabilization. Networks and Heterogeneous Media, 2010, 5(2): 299-314. doi: 10.3934/nhm.2010.5.299 |
[6] |
Giuseppe Maria Coclite, Nicola De Nitti, Mauro Garavello, Francesca Marcellini .
Vanishing viscosity for a |
[7] | Michael Herty, Niklas Kolbe, Siegfried Müller . Central schemes for networked scalar conservation laws. Networks and Heterogeneous Media, 2023, 18(1): 310-340. doi: 10.3934/nhm.2023012 |
[8] | Joachim von Below, José A. Lubary . Isospectral infinite graphs and networks and infinite eigenvalue multiplicities. Networks and Heterogeneous Media, 2009, 4(3): 453-468. doi: 10.3934/nhm.2009.4.453 |
[9] | Alessia Marigo . Equilibria for data networks. Networks and Heterogeneous Media, 2007, 2(3): 497-528. doi: 10.3934/nhm.2007.2.497 |
[10] | Gen Qi Xu, Siu Pang Yung . Stability and Riesz basis property of a star-shaped network of Euler-Bernoulli beams with joint damping. Networks and Heterogeneous Media, 2008, 3(4): 723-747. doi: 10.3934/nhm.2008.3.723 |
We consider the idealized setting of gradient flow on the population risk for infinitely wide two-layer ReLU neural networks (without bias), and study the effect of symmetries on the learned parameters and predictors. We first describe a general class of symmetries which, when satisfied by the target function f∗ and the input distribution, are preserved by the dynamics. We then study more specific cases. When f∗ is odd, we show that the dynamics of the predictor reduces to that of a (non-linearly parameterized) linear predictor, and its exponential convergence can be guaranteed. When f∗ has a low-dimensional structure, we prove that the gradient flow PDE reduces to a lower-dimensional PDE. Furthermore, we present informal and numerical arguments that suggest that the input neurons align with the lower-dimensional structure of the problem.
We consider a family of scalar conservation laws defined on an oriented graph
On the edge
∂tρi+∂xfi(ρi)=0,t>0,x<0,i=1,...,m, | (1) |
and on the outgoing ones
∂tρj+∂xfj(ρj)=0,t>0,x>0,j=m+1,...,m+n. | (2) |
The fluxes
(H.1) for each
(H.2) for any
We augment (1) and (2) with the initial conditions
{ρi(0,x)=ρi,0(x),x<0,i=1,...,m,ρj(0,x)=ρj,0(x),x>0,j=m+1,...,m+n, | (3) |
assuming that
(H.3)
and
Finally, we introduce the necessary conservation assumption at the node, which transforms our family of independent equations into a single problem
m∑i=1fi(ρi(t,0−))=m+n∑j=m+1fj(ρj(t,0+)) for a.e. t≥0. |
Questions related to existence, uniqueness and stability of solutions for problems of this kind have been extensively investigated in recent years, mainly in relation with traffic modeling. The interested reader can refer to [7,13] for an overview of the subject. Here our point of view is different, as we do not focus on a specific model. We consider a parabolic regularization of the problem, similarly to what has been done in [11,10], but instead of enforcing a continuity condition at the node for the regularized solutions, we introduce a more general set of transmission conditions on the parabolic fluxes.
In this work we adopt the following definition of weak solution for the problem (1), (2), and (3). We stress that this definition is for sure not sufficient to ensure uniqueness. On the contrary it fix somehow a minimal set of properties that any reasonable solution is expected to satisfy, see [3] and references therein for a more detailed discussion on this point.
Definition 1.1. Let
(D.1)
(D.2) for every
∫∞0∫0−∞(|ρi−c|∂tφ+sign(ρi−c)(fi(ρi)−fi(c))∂xφ)dtdx+∫0−∞|ρi,0(x)−c|φ(0,x)dx≥0; |
(D.3) for every
∫∞0∫∞0(|ρj−c|∂tφ+sign(ρj−c)(fj(ρR)−fj(c))∂xφ)dtdx+∫∞0|ρj,0(x)−c|φ(0,x)dx≥0; |
(D.4)
In [10] the authors approximated (1), (2), and (3) in the following way
{∂tρi,ε+∂xfi(ρi,ε)=ε∂2xxρi,ε,t>0,x<0,i,∂tρj,ε+∂xfj(ρj,ε)=ε∂2xxρj,ε,t>0,x>0,j,ρi,ε(t,0)=ρj,ε(t,0),t>0,i,j,m∑i=1(fi(ρi,ε(t,0))−ε∂xρi,ε(t,0))=m+n∑j=m+1(fj(ρj,ε(t,0))−ε∂xρj,ε(t,0)),t>0,ρi,ε(0,x)=ρi,0,ε(x),x<0,i,ρj,ε(0,x)=ρj,0,ε(x),x>0,j, | (4) |
where
ρi,ε→ρia.e. in (0,∞)×(−∞,0) andin Lploc((0,∞)×(−∞,0)),1≤p<∞, as ε→0 for every i,ρj,ε→ρja.e. in (0,∞)×(0,∞) and in Lploc((0,∞)×(0,∞)),1≤p<∞, as ε→0 for every j, |
where
In this paper we modify the transmission condition of (4) and inspired by [14] we consider the following viscous approximation of (1), (2), and (3)
{∂tρi,ε+∂xfi(ρi,ε)=ε∂2xxρi,ε,t>0,x<0,i,∂tρj,ε+∂xfj(ρj,ε)=ε∂2xxρj,ε,t>0,x>0,j,fi(ρi,ε(t,0))−ε∂xρi,ε(t,0)=βi(ρ1,ε(t,0),....,ρm+n,ε(t,0)),t>0,i,fj(ρj,ε(t,0))−ε∂xρj,ε(t,0)=βj(ρ1,ε(t,0),....,ρm+n,ε(t,0)),t>0,j,ρi,ε(0,x)=ρi,0,ε(x),x<0,i,ρj,ε(0,x)=ρj,0,ε(x),x>0,j, | (5) |
where, of course,
m∑i=1βi(ρ1,ε(t,0),…,ρm+n,ε(t,0))=m+n∑j=m+1βj(ρ1,ε(t,0),…,ρm+n,ε(t,0)). | (6) |
The additional assumptions we make on the functions
The main result of the paper is the following.
Theorem 1.2. Assume(H.1), (H.2), and (H.3). There exist a sequence
ρi,εk⟶ρi,a.e.andinLploc((0,∞)×(−∞,0)), | (7) |
ρj,εk⟶ρj,a.e.andinLploc((0,∞)×(0,∞)), | (8) |
f1(ρ1),...,fm(ρm)∈BV((0,∞)×(−∞,0)),fm+1(ρm+1),...,fm+n(ρm+n)∈BV((0,∞)×(0,∞)), | (9) |
for every
It worth mentioning that a complete characterization of the limit solution obtained from (4) as
At the moment we are not able to formulate a similar characterization of the limit of (5). In general, however, the limits coming from parabolic regularization subject to the two different kinds of transmission conditions are different.
To show this consider the simple case of a junction with one incoming and one outgoing edges. So we have the conservation law
∂tρ1+∂xf1(ρ1)=0,t>0,x<0, | (10) |
on the incoming edge and
∂tρ2+∂xf2(ρ2)=0,t>0,x>0, | (11) |
on the outgoing one. Assume that
f1(0)=f1(1)=f2(0)=f2(1)=0,f″1,f″2<0,there exists 0<ˇρ<ˆρ<1 and G>0 such that f1(ˆρ)=f2(ˇρ)=G(ˆρ−ˇρ). | (12) |
Consider the simplified version of (5)
{∂tρ1,ε+∂xf1(ρ1,ε)=ε∂2xxρ1,ε,t>0,x<0,∂tρ2,ε+∂xf2(ρ2,ε)=ε∂2xxρ2,ε,t>0,x>0,f1(ρ1,ε(t,0))−ε∂xρ1,ε(t,0)=f2(ρ2,ε(t,0))−ε∂xρ2,ε(t,0)=G(ρ1,ε−ρ2,ε),t>0,ρ1,ε(0,x)=ˆρ,x<0,ρ2,ε(0,x)=ˇρ,x>0. | (13) |
The unique solution of (13) is
ρ1,ε(⋅,⋅)=ˆρ,ρ2,ε(⋅,⋅)=ˇρ,ε>0. | (14) |
Therefore, as
ρ1(⋅,⋅)=ˆρ,ρ2(⋅,⋅)=ˇρ. | (15) |
This stationary solution is not admissible in the sense of the classical vanishing viscosity germ, see [5,Sec. 5], as it consists of a nonclassical shock. However, when dealing with conservation laws with discontinuous flux, it is well known that infinitely many
It is worth noticing that entropy solutions admissible in the sense of a
It is difficult, however, to establish a direct equivalence between the aforementioned results and the one we put forward in this paper. In particular, in the present case we miss information on the boundary layers at the parabolic level and we do not know how the transmission conditions we impose on the parabolic fluxes translates into a condition for the hyperbolic problem.
This means in particular that we have little information on the germ associated to the family of limit solutions obtained in Theorem 1.2 and, so far, we have not been able to prove that this germ is
The paper is organized as follows: Section 2 contains the precise list of assumptions on the initial and transmission conditions in the parabolic problem (5). In Section 3 we present the proofs of all necessary a priori estimates on (5). Finally, in Section 4 we detail the proof of Theorem 1.2.
The initial conditions
Once the functions
ρi,0,ε∈C∞((−∞,0])∩L1(−∞,0),ρj,0,ε∈C∞([0,∞))∩L1(0,∞),ε>0,ρi,0,ε→ρi,0a.e. in (−∞,0) and in Lploc(−∞,0),1≤p<∞, as ε→0,ρj,0,ε→ρj,0a.e. in (0,∞) and in Lploc(0,∞),1≤p<∞, as ε→0,0≤ρi,0,ε,ρj,0,ε≤1,ε>0,‖ρi,0,ε‖L1(−∞,0)≤‖ρi,0‖L1(−∞,0),‖ρj,0,ε‖L1(0,∞)≤‖ρj,0‖L1(0,∞),ε>0,‖ρi,0,ε‖L2(−∞,0)≤‖ρi,0‖L2(−∞,0),‖ρj,0,ε‖L2(0,∞)≤‖ρj,0‖L2(0,∞),ε>0,‖∂xρi,0,ε‖L1(−∞,0)≤TV(ρi,0),‖∂xρj,0,ε‖L1(0,∞)≤TV(ρj,0),ε>0,ε‖∂xρi,0,ε‖L1(−∞,0),ε‖∂2xxρj,0,ε‖L1(0,∞)≤C,ε>0, | (16) |
for some constant
The functions
βi(ρ1,ε(t,0),....,ρm+n,ε(t,0))=m+n∑j=m+1Gi,j(ρi,ε(t,0),ρj,ε(t,0))+ε(m∑h=1Ki,h(ρi,ε(t,0),ρh,ε(t,0))−m+n∑h=1Kh,i(ρh,ε(t,0),ρi,ε(t,0))); | (17) |
for
βj(ρ1,ε(t,0),....,ρm+n,ε(t,0))=m∑i=1Gi,j(ρi,ε(t,0),ρj,ε(t,0))+ε(m+n∑h=m+1Kh,j(ρh,ε(t,0),ρj,ε(t,0))−m+n∑h=1Kj,h(ρj,ε(t,0),ρh,ε(t,0))). | (18) |
The functions
∂vGi,j(⋅,⋅)≤0≤∂uGi,j(⋅,⋅),Gi,j(0,0)=Gi,j(1,1)=0,∂uKh,ℓ(⋅,⋅)≤0≤∂vKh,ℓ(⋅,⋅),Kh,ℓ(0,0)=Kh,ℓ(1,1)=0. | (19) |
In particular, (19) implies
(sign(u)−sign(v))∇Gi,j(⋅,⋅)⋅(u,v)≥0,u,v∈R,(sign(u)−sign(v))∇Kh,ℓ(⋅,⋅)⋅(u,v)≤0,u,v∈R,(sign(u−u′)−sign(v−v′))(Gi,j(u,v)−Gi,j(u′,v′))≥0,u,u′,v,v′∈R,(sign(u−u′)−sign(v−v′))(Kh,ℓ(u,v)−Kh,ℓ(u′,v′))≤0,u,u′,v,v′∈R,(χ(−∞,0)(u)−χ(−∞,0)(v))Gi,j(u,v)≤0,u,v∈R,(χ(−∞,0)(u)−χ(−∞,0)(v))Kh,ℓ(u,v)≥0,u,v∈R, | (20) |
where
This specific form of transmission conditions is reminiscent of the parabolic transmission conditions considered in [14,8], which were originally inspired from the Kedem-Katchalsky conditions for membrane permeability introduced in [16]
Gh,ℓ(u,v)=ch,ℓ(u−v), | (21) |
for some constants
Gh,ℓ(u,v)(u−v)≥0, | (22) |
that allows the authors in [14] to get the
We can observe that the equality (6) holds as
m∑i=1βi(ρ1,ε(t,0),....,ρm+n,ε(t,0))=m∑i=1m+n∑j=m+1Gi,j(ρi,ε(t,0),ρj,ε(t,0))+εm∑i=1(m∑h=1Ki,h(ρi,ε(t,0),ρh,ε(t,0))−m+n∑h=1Kh,i(ρh,ε(t,0),ρi,ε(t,0)))=m∑i=1m+n∑j=m+1(Gi,j(ρi,ε(t,0),ρj,ε(t,0))−εKj,i(ρj,ε(t,0),ρi,ε(t,0))) | (23) |
and analogously
m+n∑j=m+1βj(ρ1,ε(t,0),....,ρm+n,ε(t,0))=m+n∑j=m+1m∑i=1(Gi,j(ρi,ε(t,0),ρj,ε(t,0))−εKj,i(ρj,ε(t,0),ρi,ε(t,0))). | (24) |
This section is devoted to establish a priori estimates, uniform with respect to
For every
Lemma 3.1 (
0≤ρi,ε,ρj,ε≤1,i,j. | (25) |
Proof. Consider the function
η(ξ)=−ξχ(−∞,0)(ξ). |
Since
η′(ξ)=−χ(−∞,0)(ξ), |
using (19) we obtain
ddt(m∑i=1∫0−∞η(ρi,ε)dx+m+n∑j=m+1∫∞0η(ρj,ε)dx)=m∑i=1∫0−∞η′(ρi,ε)∂tρi,εdx+m+n∑j=m+1∫∞0η′(ρj,ε)∂tρj,εdx=−m∑i=1∫0−∞χ(−∞,0)(ρi,ε)∂tρi,εdx−m+n∑j=m+1∫∞0χ(−∞,0)(ρj,ε)∂tρj,εdx=m∑i=1∫0−∞χ(−∞,0)(ρi,ε)∂x(fi(ρi,ε)−ε∂xρi,ε)dx+m+n∑j=m+1∫∞0χ(−∞,0)(ρj,ε)∂x(fj(ρj,ε)−ε∂xρj,ε)dx=m∑i=1χ(−∞,0)(ρi,ε(t,0))(fi(ρi,ε(t,0))−ε∂xρi,ε(t,0))−m+n∑j=m+1χ(−∞,0)(ρj,ε(t,0))(fj(ρj,ε(t,0))−ε∂xρj,ε(t,0))+m∑i=1∫0−∞∂xρi,ε(fi(ρi,ε)−ε∂xρi,ε)dδ{ρi,ε=0}⏟≤0+m+n∑j=m+1∫∞0∂xρj,ε(fj(ρj,ε)−ε∂xρj,ε)dδ{ρj,ε=0}⏟≤0≤m+n∑j=m+1m∑i=1(χ(−∞,0)(ρi,ε(t,0))−χ(−∞,0)(ρj,ε(t,0)))⋅⋅(Gi,j(ρi,ε(t,0),ρj,ε(t,0))−εKj,i(ρj,ε(t,0),ρi,ε(t,0)))≤0, |
where
0≤m∑i=1∫0−∞η(ρi,ε(t,x))dx+m+n∑j=m+1∫∞0η(ρj,ε(t,x))dx≤m∑i=1∫0−∞η(ρi,0,ε)dx+m+n∑j=m+1∫∞0η(ρj,0,ε)dx=0 |
and then
ρi,ε,ρj,ε≥0,i,j, |
that proves the lower bounds in (25). The upper bounds in (25) can be proved in the same way using the function
Lemma 3.2 (
m∑i=1‖ρi,ε(t,⋅)‖L1(−∞,0)+m+n∑j=m+1‖ρj,ε(t,⋅)‖L1(0,∞)≤m∑i=1‖ρi,0‖L1(−∞,0)+m+n∑j=m+1‖ρj,0‖L1(0,∞),t≥0. | (26) |
Proof. Thanks to (5), (23), (24), and (25), we have that
ddt(m∑i=1∫0−∞|ρi,ε|dx+m+n∑j=m+1∫∞0|ρj,ε|dx)=ddt(m∑i=1∫0−∞ρi,εdx+m+n∑j=m+1∫∞0ρj,εdx)=m∑i=1∫0−∞∂tρi,εdx+m+n∑j=m+1∫∞0∂tρj,εdx=−m∑i=1∫0−∞∂x(fi(ρi,ε)−ε∂xρi,ε)dx−m+n∑j=m+1∫∞0∂x(fj(ρj,ε)−ε∂xρj,ε)dx=−m∑i=1βi(ρ1,ε(t,0),…,ρm+n,ε(t,0))+m+n∑j=m+1βj(ρ1,ε(t,0),…,ρm+n,ε(t,0))=0. |
Integrating over
Lemma 3.3 (
m∑i=1‖ρi,ε(t,⋅)‖2L2(−∞,0)+m+n∑j=m+1‖ρj,ε(t,⋅)‖2L2(0,∞)+2ε∫t0(m∑i=1‖∂xρi,ε(s,⋅)‖2L2(−∞,0)+m+n∑j=m+1‖∂xρj,ε(s,⋅)‖2L2(0,∞))ds≤m∑i=1‖ρi,0‖2L2(−∞,0)+m+n∑j=m+1‖ρj,0‖2L2(0,∞)+2(m+n∑ℓ=1‖βℓ‖L∞((0,1)m+n)+m∑i=1‖fi‖L1(0,1))t, | (27) |
for every
Proof. Thanks to (5), we have that
ddt(m∑i=1∫0−∞ρ2i,ε2dx+m+n∑j=m+1∫∞0ρ2j,ε2dx)=m∑i=1∫0−∞ρi,ε∂tρi,εdx+m+n∑j=m+1∫∞0ρj,ε∂tρj,εdx=−m∑i=1∫0−∞ρi,ε∂x(fi(ρi,ε)−ε∂xρi,ε)dx−m+n∑j=m+1∫∞0ρj,ε∂x(fj(ρj,ε)−ε∂xρj,ε)dx=−m∑i=1ρi,ε(t,0)(fi(ρi,ε(t,0))−ε∂xρi,ε(t,0))+m+n∑j=m+1ρj,ε(t,0)(fj(ρj,ε(t,0))−ε∂xρj,ε(t,0))+m∑i=1∫0−∞∂x(∫ρi,ε(t,x)0fi(ξ)dξ)dx+m+n∑j=m+1∫∞0∂x(∫ρj,ε(t,x)0fj(ξ)dξ)dx−εm∑i=1∫0−∞(∂xρi,ε)2dx−εm+n∑j=m+1∫∞0(∂xρj,ε)2dx=m∑i=1ρj,ε(t,0)βi(ρ1,ε(t,0),....,ρm+n,ε(t,0))−m+n∑j=m+1ρi,ε(t,0))βj(ρ1,ε(t,0),....,ρm+n,ε(t,0))+m∑i=1∫ρi,ε(t,0)0fi(ξ)dξ−m+n∑j=m+1∫ρj,ε(t,0)0fj(ξ)dξ⏟≤0−εm∑i=1∫0−∞(∂xρi,ε)2dx−εm+n∑j=m+1∫∞0(∂xρj,ε)2dx≤m+n∑ℓ=1‖βℓ‖L∞((0,1)m+n)+m∑i=1‖fi‖L1(0,1)−εm∑i=1∫0−∞(∂xρi,ε)2dx−εm+n∑j=m+1∫∞0(∂xρj,ε)2dx. |
Integrating over
Lemma 3.4 (
m∑i=1‖∂tρi,ε(t,⋅)‖L1(−∞,0)+m+n∑j=m+1‖∂tρj,ε(t,⋅)‖L1(0,∞)≤(m+n)C+m∑i=1‖f′i‖L∞(0,1)TV(ρi,0)+m+n∑j=m+1‖f′j‖L∞(0,1)TV(ρj,0), | (28) |
for every
Proof. From (5) we get
∂2ttρi,ε+∂x(f′i(ρi,ε)∂tρi,ε)=ε∂3txxρi,ε,∂2ttρj,ε+∂x(f′j(ρj,ε)∂tρj,ε)=ε∂3txxρj,ε,f′i(ρi,ε(t,0))∂tρi,ε(t,0)−ε∂2txρi,ε(t,0)=m+n∑j=m+1∇Gi,j(ρi,ε(t,0),ρj,ε(t,0))⋅(∂tρi,ε(t,0),∂tρj,ε(t,0))+εm∑h=1∇Ki,h(ρi,ε(t,0),ρh,ε(t,0))⋅(∂tρi,ε(t,0),∂tρh,ε(t,0))−εm+n∑h=1∇Kh,i(ρh,ε(t,0),ρi,ε(t,0))⋅(∂tρh,ε(t,0),∂tρi,ε(t,0)),f′j(ρj,ε(t,0))∂tρj,ε(t,0)−ε∂2txρj,ε(t,0)=m∑i=1∇Gi,j(ρi,ε(t,0),ρj,ε(t,0))⋅(∂tρi,ε(t,0),∂tρj,ε(t,0))+εm+n∑h=m+1∇Kh,j(ρh,ε(t,0),ρj,ε(t,0))⋅(∂tρh,ε(t,0),∂tρj,ε(t,0))−εm+n∑h=1∇Kj,h(ρj,ε(t,0),ρh,ε(t,0))⋅(∂tρi,ε(t,0),∂tρh,ε(t,0)). |
Thanks to (20), we have that
ddt(m∑i=1∫0−∞|∂tρi,ε|dx+m+n∑j=m+1∫∞0|∂tρj,ε|dx)=m∑i=1∫0−∞∂2ttρi,εsign(∂tρi,ε)dx+m+n∑j=m+1∫∞0∂2ttρj,εsign(∂tρj,ε)dx=−m∑i=1∫0−∞sign(∂tρi,ε)∂x(f′i(ρi,ε)∂tρi,ε−ε∂2txρi,ε)dx−m+n∑j=m+1∫∞0sign(∂tρj,ε)∂x(f′j(ρj,ε)∂tρj,ε−ε∂2txρj,ε)dx=−m∑i=1sign(∂tρi,ε(t,0))(f′i(ρi,ε(t,0))∂tρi,ε(t,0)−ε∂2txρi,ε(t,0))+m+n∑j=m+1sign(∂tρj,ε(t,0))(f′j(ρj,ε(t,0))∂tρj,ε(t,0)−ε∂2txρj,ε(t,0))+2m∑i=1∫0−∞∂2txρi,ε(f′i(ρi,ε)∂tρi,ε−ε∂2txρi,ε)dδ{∂tρi,ε=0}⏟≤0+2m+n∑j=m+1∫0−∞∂2txρj,ε(f′j(ρj,ε)∂tρj,ε−ε∂2txρj,ε)dδ{∂tρj,ε=0}⏟≤0≤−m∑i=1m+n∑j=m+1(sign(∂tρi,ε(t,0))−sign(∂tρj,ε(t,0)))××∇Gi,j(ρi,ε(t,0),ρj,ε(t,0))⋅(∂tρi,ε(t,0),∂tρj,ε(t,0))+εm∑i=1m+n∑j=m+1(sign(∂tρi,ε(t,0))−sign(∂tρj,ε(t,0)))××∇Kj,i(ρi,ε(t,0),ρj,ε(t,0))⋅(∂tρi,ε(t,0),∂tρj,ε(t,0))≤0, |
where
Integrating over
m∑i=1‖∂tρi,ε(t,⋅)‖L1(−∞,0)+m+n∑j=m+1‖∂tρj,ε(t,⋅)‖L1(0,∞)≤m∑i=1‖∂tρi,ε(0,⋅)‖L1(−∞,0)+m+n∑j=m+1‖∂tρj,ε(0,⋅)‖L1(0,∞)=m∑i=1‖ε∂2xxρi,0,ε−∂xfi(ρi,0,ε)‖L1(−∞,0)+m+n∑j=m+1‖ε∂2xxρj,0,ε−∂xfj(ρj,0,ε)‖L1(0,∞)≤m∑i=1(ε‖∂2xxρi,0,ε‖L1(−∞,0)+‖f′i(ρi,0,ε)‖L∞(−∞,0)‖∂xρi,0,ε‖L1(−∞,0))+m+n∑j=m+1(ε‖∂2xxρj,0,ε‖L1(0,∞)+‖f′j(ρj,0,ε)‖L∞(0,∞)‖∂xρj,0,ε‖L1(0,∞))≤(m+n)C+m∑i=1‖f′i‖L∞(0,1)TV(ρi,0)+m+n∑j=m+1‖f′j‖L∞(0,1)TV(ρj,0), |
that is (28).
Lemma 3.5 (Stability estimate). Let
m∑i=1‖ρi,ε(t,⋅)−¯ρi,ε(t,⋅)‖L1(−∞,0)+m+n∑j=m+1‖ρj,ε(t,⋅)−¯ρj,ε(t,⋅)‖L1(0,∞)≤m∑i=1‖ρi,0,ε−¯ρi,0,ε‖L1(−∞,0)+m+n∑j=m+1‖ρj,0,ε−¯ρj,0,ε‖L1(0,∞),t≥0. | (29) |
Proof. From (5) we get
∂t(ρi,ε−¯ρi,ε)+∂x(fi(ρi,ε)−fi(¯ρi,ε))=ε∂2xx(ρi,ε−¯ρi,ε),∂t(ρj,ε−¯ρj,ε)+∂x(fj(ρj,ε)−fj(¯ρj,ε))=ε∂2xx(ρj,ε−¯ρj,ε). |
Thanks to (5), (20), and (25), we have that
ddt(m∑i=1∫0−∞|ρi,ε−¯ρi,ε|dx+m+n∑j=m+1∫∞0|ρj,ε−¯ρj,ε|dx)=m∑i=1∫0−∞sign(ρi,ε−¯ρi,ε)∂t(ρi,ε−¯ρi,ε)dx+m+n∑j=m+1∫∞0sign(ρj,ε−¯ρj,ε)∂t(ρj,ε−¯ρj,ε)dx=−m∑i=1∫0−∞sign(ρi,ε−¯ρi,ε)∂x((fi(ρi,ε)−fi(¯ρi,ε))−ε∂x(ρi,ε−¯ρi,ε))dx−m+n∑j=m+1∫∞0sign(ρj,ε−¯ρj,ε)∂x((fj(ρj,ε)−fj(¯ρj,ε))−ε∂x(ρj,ε−¯ρj,ε))dx=−m∑i=1m+n∑j=m+1[sign(ρi,ε(t,0)−¯ρi,ε(t,0))−sign(ρj,ε(t,0)−¯ρj,ε(t,0))]××[Gi,j(ρi,ε(t,0),ρj,ε(t,0))−Gi,j(¯ρi,ε(t,0),¯ρj,ε(t,0))]+εm∑i=1m+n∑j=m+1[sign(ρi,ε(t,0)−¯ρi,ε(t,0))−sign(ρj,ε(t,0)−¯ρj,ε(t,0))]××[Kj,i(ρi,ε(t,0),ρj,ε(t,0))−Gi,j(¯ρi,ε(t,0),¯ρj,ε(t,0))]+2m∑i=1∫0−∞∂x(ρi,ε−¯ρi,ε)((fi(ρi,ε)−fi(¯ρi,ε))−ε∂x(ρi,ε−¯ρi,ε))dδ{ρi,ε=¯ρi,ε}⏟≤0+2m+n∑j=m+1∫∞0∂x(ρj,ε−¯ρj,ε)((fi(ρj,ε)−fi(¯ρj,ε))−ε∂x(ρj,ε−¯ρj,ε))dδ{ρj,ε=¯ρj,ε}⏟≤0≤0, |
where we use [6,Lemma 2] and we denote by
Integrating over
The well-posedness of smooth solutions for (5) can be proved following the argument used in [10,Theorem 1.2] to establish the well-posedness of smooth solutions for (4). Indeed, the existence of a linear semigroup of solutions in the linear case (i.e., when
The main result of this section is the following.
Lemma 4.1. Let
ρ1,...,ρm∈L1((0,∞)×(−∞,0))∩L∞((0,∞)×(−∞,0)), | (30) |
ρm+1,...,ρm+n∈L1((0,∞)×(0,∞))∩L∞((0,∞)×(0,∞)), | (31) |
0≤ρℓ≤1,ℓ∈{1,...,m+n}, | (32) |
ρi,εk⟶ρi,a.e.andinLploc((0,∞)×(−∞,0)), | (33) |
ρj,εk⟶ρj,a.e.andinLploc((0,∞)×(0,∞)), | (34) |
for every
m∑i=1‖ρi(t,⋅)‖L1(−∞,0)+m+n∑j=m+1‖ρj(t,⋅)‖L1(0,∞) | (35) |
≤m∑i=1‖ρi,0‖L1(−∞,0)+m+n∑j=m+1‖ρj,0‖L1(0,∞),m∑i=1‖ρi(t,⋅)‖2L2(−∞,0)+m+n∑j=m+1‖ρj(t,⋅)‖2L2(0,∞) | (36) |
≤m∑i=1‖ρi,0‖2L2(−∞,0)+m+n∑j=m+1‖ρj,0‖2L2(0,∞)+2(m+n∑ℓ=1‖βℓ‖L∞((0,1)m+n)+m∑i=1‖fi‖L1(0,1))t,m∑i=1TV(fi(ρi(t,⋅)))+m+n∑j=m+1TV(fj(ρj(t,⋅)))=m∑i=1‖∂tρi(t,⋅)‖M(−∞,0)+m+n∑j=m+1‖∂tρj(t,⋅)‖M(0,∞)≤(m+n)C+m∑i=1‖f′i‖L∞(0,1)TV(ρi,0)+m+n∑j=m+1‖f′j‖L∞(0,1)TV(ρj,0). | (37) |
Thanks to the genuine nonlinearity of
Theorem 4.2 (Tartar). Let
‖vν‖L∞((0,T)×R)≤MT,T,ν>0, |
and the family
{∂tη(vν)+∂xqℓ(vν)}ν>0 |
is compact in
vνn⟶va.e.andinLploc((0,∞)×R),1≤p<∞. |
The following compact embedding of Murat [17] is useful.
Theorem 4.3 (Murat). Let
Ln=L1,n+L2,n, |
where
Proof of Lemma 4.1. Let us fix
Let
∂tη(ρi,ε)+∂xqi(ρi,ε)=ε∂2xxη(ρi,ε)⏟L1,ε−εη″(ρi,ε)(∂xρi,ε)2⏟L2,ε. | (38) |
We claim that
L1,ε⟶0inH−1((0,T)×(−∞,0)),T>0,asε→0,{L2,ε}εisuniformlyboundedinL1((0,T)×(−∞,0)),T>0. | (39) |
Indeed, (25) and (27) imply
‖ε∂xη(ρi,ε)‖L2((0,T)×(−∞,0))≤√ε‖η′‖L∞(0,1)‖√ε∂xρi,ε‖L2((0,∞)×(−∞,0))≤√ε‖η′‖L∞(0,1)(m∑i=1‖ρi,ε,0‖L2(−∞,0)+m+n∑j=m+1‖ρj,ε,0‖L2(0,∞)+√2(m+n∑ℓ=1‖βℓ‖L∞((0,1)m+n)+m∑i=1‖fi‖L1(0,1))T)→0,‖εη″(ρi,ε)(∂xρi,ε)2‖L1((0,T)×(−∞,0))≤‖η″‖L∞(0,1)(m∑i=1‖ρi,ε,0‖2L2(−∞,0)+m+n∑j=m+1‖ρj,ε,0‖2L2(0,∞)+2(m+n∑ℓ=1‖βℓ‖L∞((0,1)m+n)+m∑i=1‖fi‖L1(0,1))T). |
Due to (16), (39) follows. Therefore, Theorems 4.3 and 4.2 give the existence of a subsequence
ρi,εk⟶ρiinLploc((0,∞)×(−∞,0))foranyp∈[1,∞),ρi,εk⟶ρia.e.in(0,∞)×(−∞,0), | (40) |
that guarantees (32) and (33).
Finally, thanks to Lemmas 3.2, 3.3, and 3.4 we have (35), (36), and (37).
Proof of Theorem 1.2.. The first part of the statement related to the convergence of vanishing viscosity approximations has been proved in Lemma 4.1.
Let us fix
Thanks to (3.4) and (33), for all
∫∞0∫0−∞ρi∂tφdxdt=limk∫∞0∫0−∞ρi,εk∂tφdxdt=−limk∫∞0∫0−∞∂tρi,εkφdxdt≤‖φ‖L∞((0,∞)×(−∞,0))((m+n)C+m∑i=1‖f′i‖L∞(0,1)TV(ρi,0)+m+n∑j=m+1‖f′j‖L∞(0,1)TV(ρj,0)), |
therefore
∂tρi∈M((0,∞)×(−∞,0)), | (41) |
where
∂xfi(ρi)∈M((0,∞)×(−∞,0)). | (42) |
Clearly (41) and (42) give (9) and so the trace at the junction
We prove now that the identity
m∑i=1fi(ρi(t,0−))=n+m∑j=m+1fj(ρj(t,0+)) | (43) |
holds for a.e.
Let
0≤rν(x)≤1,rν(0)=1,supp(rν)⊆[0,1ν], | (44) |
for every
From (5) we have that
0=m∑i=1∫∞0∫0−∞(∂tρi,εk+∂xfi(ρi,εk)−εk∂2xxρi,εk)φ(t)˜rν(x)dxdt+m+n∑j=m+1∫∞0∫∞0(∂tρj,εk+∂xfj(ρj,εk)−εk∂2xxρj,εk)φ(t)rν(x)dxdt=−m∑i=1∫∞0∫0−∞(ρi,εkφ′(t)˜rν(x)+fi(ρi,εk)φ(t)˜r′ν(x)−εk∂xρi,εkφ(t)˜r′ν(x))dxdt−m+n∑j=m+1∫∞0∫∞0(ρj,εkφ′(t)rν(x)+fj(ρj,εk)φ(t)r′ν(x)−εk∂xρj,εkφ(t)r′ν(x))dxdt+m∑i=1∫∞0(fi(ρi,εk(t,0))−εk∂xρi,εk(t,0))φ(t)dt−m+n∑j=m+1∫∞0(fj(ρj,εk(t,0))−εk∂xρj,εk(t,0))φ(t)dt=−m∑i=1∫∞0∫0−∞(ρi,εkφ′(t)˜rν(x)+fi(ρi,εk)φ(t)˜r′ν(x)−εk∂xρi,εkφ(t)˜r′ν(x))dxdt−m+n∑j=m+1∫∞0∫∞0(ρj,εkφ′(t)rν(x)+fj(ρj,εk)φ(t)r′ν(x)−εk∂xρj,εkφ(t)r′ν(x))dxdt. |
As
0=−m∑i=1∫∞0∫0−∞(ρiφ′(t)˜rν(x)+fi(ρi)φ(t)˜r′ν(x))dxdt−m+n∑j=m+1∫∞0∫∞0(ρjφ′(t)rν(x)+fj(ρj)φ(t)r′ν(x))dxdt. |
Finally, sending
0=−m∑i=1∫∞0fi(ρi(t,0−))φ(t)dt+m+n∑j=m+1∫∞0fj(ρj(t,0+))φ(t)dt, |
that gives (43).
[1] | N. Cammarata, S. Carter, G. Goh, C. Olah, M. Petrov, L. Schubert, et al., Thread: Circuits, Distill, 5 (2020), e24. |
[2] | M. D. Zeiler, R. Fergus, Visualizing and understanding convolutional networks, in European Conference on Computer Vision, Springer, (2014), 818–833. https://doi.org/10.1007/978-3-319-10590-1_53 |
[3] | I. Goodfellow, Y. Bengio, A. Courville, Deep Learning, MIT Press, 2016. |
[4] | L. Chizat, F. Bach, Implicit bias of gradient descent for wide two-layer neural networks trained with the logistic loss, in Conference on Learning Theory, PMLR, (2020), 1305–1338. |
[5] | S. Wojtowytsch, On the convergence of gradient descent training for two-layer relu-networks in the mean field regime, preprint, arXiv: 2005.13530. |
[6] | S. Mei, A. Montanari, P. M. Nguyen, A mean field view of the landscape of two-layer neural networks, Proc. Natl. Acad. Sci., 115 (2018), E7665–E7671. |
[7] | P. Nguyen, H. T. Pham, A rigorous framework for the mean field limit of multilayer neural networks, preprint, arXiv: 2001.11443. |
[8] | L. Chizat, E. Oyallon, F. Bach, On lazy training in differentiable programming, in Advances in Neural Information Processing Systems, 32 (2019), 1–11. |
[9] | G. Yang, E. J. Hu, Tensor programs iv: Feature learning in infinite-width neural networks, in International Conference on Machine Learning, PMLR, (2021), 11727–11737. |
[10] | A. Jacot, F. Gabriel, C. Hongler, Neural tangent kernel: Convergence and generalization in neural networks, Adv. Neural Inf. Proc. Syst., 31 (2018), 1–10. |
[11] | L. Chizat, F. Bach, On the global convergence of gradient descent for over-parameterized models using optimal transport, in Proceedings of the 32nd International Conference on Neural Information Processing Systems, (2018), 3040–3050. |
[12] | G. Rotskoff, E. Vanden-Eijnden, Parameters as interacting particles: long time convergence and asymptotic error scaling of neural networks, in Advances in Neural Information Processing Systems (eds. S. Bengio, H. Wallach, H. Larochelle, K. Grauman, N. Cesa-Bianchi, R. Garnett), 31 (2018), 1–10. |
[13] |
J. Sirignano, K. Spiliopoulos, Mean field analysis of neural networks: A law of large numbers, SIAM J. Appl. Math., 80 (2020), 725–752. https://doi.org/10.1137/18M1192184 doi: 10.1137/18M1192184
![]() |
[14] | C. Ma, L. Wu, Machine learning from a continuous viewpoint, I, Sci. Chin. Math., 63 (2020), 2233–2266, https://doi.org/10.1007%2Fs11425-020-1773-8. |
[15] | H. Daneshmand, F. Bach, Polynomial-time sparse measure recovery, preprint, arXiv: 2204.07879. |
[16] | F. Bach, Breaking the curse of dimensionality with convex neural networks, J. Mach. Learn. Res., 18 (2017), 629–681. |
[17] |
A. Cloninger, T. Klock, A deep network construction that adapts to intrinsic dimensionality beyond the domain, Neural Networks, 141 (2021), 404–419. https://doi.org/10.1016/j.neunet.2021.06.004 doi: 10.1016/j.neunet.2021.06.004
![]() |
[18] | A. Damian, J. Lee, M. Soltanolkotabi, Neural networks can learn representations with gradient descent, in Conference on Learning Theory, PMLR, (2022), 5413–5452. |
[19] | A. Mousavi-Hosseini, S. Park, M. Girotti, M. Ioannis, M. Erdogdu, Neural networks efficiently learn low-dimensional representations with SGD, preprint, arXiv: 2209.14863. |
[20] |
J. Paccolat, L. Petrini, M. Geiger, K. Tyloo, M. Wyart, Geometric compression of invariant manifolds in neural networks, J. Stat. Mech. Theory Exp., 2021 (2021), 044001. https://doi.org/10.1088/1742-5468/abf1f3 doi: 10.1088/1742-5468/abf1f3
![]() |
[21] | E. Abbe, E. Boix-Adsera, T. Misiakiewicz, The merged-staircase property: a necessary and nearly sufficient condition for sgd learning of sparse functions on two-layer neural networks, preprint, arXiv: 2202.08658. |
[22] | E. Abbe, E. Boix-Adsera, M. S. Brennan, G. Bresler, D. Nagaraj, The staircase property: How hierarchical structure can guide deep learning, Adv. Neural Inf. Proc. Syst., 34 (2021), 26989–27002. |
[23] | Z. Allen-Zhu, Y. Li, Y. Liang, Learning and generalization in overparameterized neural networks, going beyond two layers, in Proceedings of the 33rd International Conference on Neural Information Processing Systems, (2019), 6158–6169. |
[24] | J. Ba, M. A. Erdogdu, T. Suzuki, Z. Wang, D. Wu, G. Yang, High-dimensional asymptotics of feature learning: How one gradient step improves the representation, preprint, arXiv: 2205.01445. |
[25] | G. Yehudai, O. Shamir, On the power and limitations of random features for understanding neural networks, Adv. Neural Inf. Proc. Syst., 32 (2019), 1–11. |
[26] |
B. Bloem-Reddy, Y. W. Teh, Probabilistic symmetries and invariant neural networks, J. Mach. Learn. Res., 21 (2020), 90–91. https://doi.org/10.1109/MMM.2020.3008308 doi: 10.1109/MMM.2020.3008308
![]() |
[27] | I. Ganev, R. Walters, The QR decomposition for radial neural networks, preprint, arXiv: 2107.02550. |
[28] | G. Głuch, R. Urbanke, Noether: The more things change, the more stay the same, preprint, arXiv: 2104.05508. |
[29] | Z. Ji, M. Telgarsky, Gradient descent aligns the layers of deep linear networks, preprint, arXiv: 1810.02032. |
[30] |
T. Gallouët, M. Laborde, L. Monsaingeon, An unbalanced optimal transport splitting scheme for general advection-reaction-diffusion problems, ESAIM Control. Optim. Calc. Var., 25 (2019), 8. https://doi.org/10.1051/cocv/2018001 doi: 10.1051/cocv/2018001
![]() |
[31] |
L. Chizat, Sparse optimization on measures with over-parameterized gradient descent, Math. Program., 194 (2022), 487–532. https://doi.org/10.1007/s10107-021-01636-z doi: 10.1007/s10107-021-01636-z
![]() |
[32] | F. Santambrogio, Optimal transport for applied mathematicians, Birkäuser, NY, 55 (2015), 94. https://doi.org/10.1007/978-3-319-20828-2 |
[33] |
F. Santambrogio, {Euclidean, metric, and Wasserstein} gradient flows: an overview, Bull. Math. Sci., 7 (2017), 87–154. https://doi.org/10.1007/s13373-017-0101-1 doi: 10.1007/s13373-017-0101-1
![]() |
[34] | K. Atkinson, W. Han, Spherical Harmonics and Approximations on the Unit Sphere: An Introduction, Springer, 2012. https://doi.org/10.1007/978-3-642-25983-8 |
1. | Markus Musch, Ulrik Skre Fjordholm, Nils Henrik Risebro, Well-posedness theory for nonlinear scalar conservation laws on networks, 2022, 17, 1556-1801, 101, 10.3934/nhm.2021025 | |
2. | Francesca R. Guarguaglini, Roberto Natalini, Vanishing viscosity approximation for linear transport equations on finite star-shaped networks, 2021, 21, 1424-3199, 2413, 10.1007/s00028-021-00688-0 | |
3. | John D. Towers, An explicit finite volume algorithm for vanishing viscosity solutions on a network, 2022, 17, 1556-1801, 1, 10.3934/nhm.2021021 | |
4. | Ulrik S. Fjordholm, Markus Musch, Nils H. Risebro, Well-Posedness and Convergence of a Finite Volume Method for Conservation Laws on Networks, 2022, 60, 0036-1429, 606, 10.1137/21M145001X | |
5. | Jon Asier Bárcena-Petisco, Márcio Cavalcante, Giuseppe Maria Coclite, Nicola De Nitti, Enrique Zuazua, Control of hyperbolic and parabolic equations on networks and singular limits, 2024, 0, 2156-8472, 0, 10.3934/mcrf.2024015 | |
6. | Dilip Sarkar, Shridhar Kumar, Pratibhamoy Das, Higinio Ramos, Higher-order convergence analysis for interior and boundary layers in a semi-linear reaction-diffusion system networked by a $ k $-star graph with non-smooth source terms, 2024, 19, 1556-1801, 1085, 10.3934/nhm.2024048 |